Thank you for visiting nature.com. You are using a browser version with limited support for CSS. To obtain the best experience, we recommend you use a more up to date browser (or turn off compatibility mode in Internet Explorer). In the meantime, to ensure continued support, we are displaying the site without styles and JavaScript.
- View all journals
- My Account Login
- Explore content
- About the journal
- Publish with us
- Sign up for alerts
- Review Article
- Open access
- Published: 25 October 2021
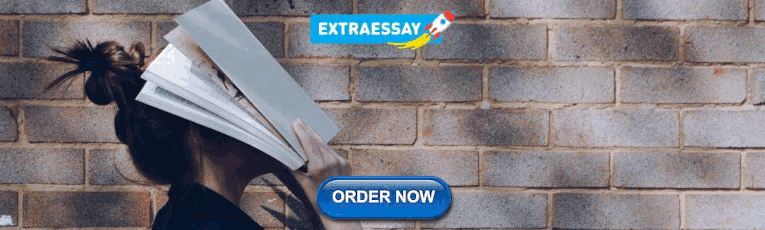
Augmented reality and virtual reality displays: emerging technologies and future perspectives
- Jianghao Xiong 1 ,
- En-Lin Hsiang 1 ,
- Ziqian He 1 ,
- Tao Zhan ORCID: orcid.org/0000-0001-5511-6666 1 &
- Shin-Tson Wu ORCID: orcid.org/0000-0002-0943-0440 1
Light: Science & Applications volume 10 , Article number: 216 ( 2021 ) Cite this article
117k Accesses
471 Citations
36 Altmetric
Metrics details
- Liquid crystals
With rapid advances in high-speed communication and computation, augmented reality (AR) and virtual reality (VR) are emerging as next-generation display platforms for deeper human-digital interactions. Nonetheless, to simultaneously match the exceptional performance of human vision and keep the near-eye display module compact and lightweight imposes unprecedented challenges on optical engineering. Fortunately, recent progress in holographic optical elements (HOEs) and lithography-enabled devices provide innovative ways to tackle these obstacles in AR and VR that are otherwise difficult with traditional optics. In this review, we begin with introducing the basic structures of AR and VR headsets, and then describing the operation principles of various HOEs and lithography-enabled devices. Their properties are analyzed in detail, including strong selectivity on wavelength and incident angle, and multiplexing ability of volume HOEs, polarization dependency and active switching of liquid crystal HOEs, device fabrication, and properties of micro-LEDs (light-emitting diodes), and large design freedoms of metasurfaces. Afterwards, we discuss how these devices help enhance the AR and VR performance, with detailed description and analysis of some state-of-the-art architectures. Finally, we cast a perspective on potential developments and research directions of these photonic devices for future AR and VR displays.
Similar content being viewed by others
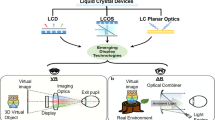
Advanced liquid crystal devices for augmented reality and virtual reality displays: principles and applications
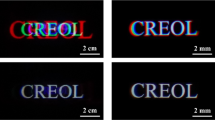
Achromatic diffractive liquid-crystal optics for virtual reality displays
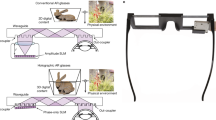
Full-colour 3D holographic augmented-reality displays with metasurface waveguides
Introduction.
Recent advances in high-speed communication and miniature mobile computing platforms have escalated a strong demand for deeper human-digital interactions beyond traditional flat panel displays. Augmented reality (AR) and virtual reality (VR) headsets 1 , 2 are emerging as next-generation interactive displays with the ability to provide vivid three-dimensional (3D) visual experiences. Their useful applications include education, healthcare, engineering, and gaming, just to name a few 3 , 4 , 5 . VR embraces a total immersive experience, while AR promotes the interaction between user, digital contents, and real world, therefore displaying virtual images while remaining see-through capability. In terms of display performance, AR and VR face several common challenges to satisfy demanding human vision requirements, including field of view (FoV), eyebox, angular resolution, dynamic range, and correct depth cue, etc. Another pressing demand, although not directly related to optical performance, is ergonomics. To provide a user-friendly wearing experience, AR and VR should be lightweight and ideally have a compact, glasses-like form factor. The above-mentioned requirements, nonetheless, often entail several tradeoff relations with one another, which makes the design of high-performance AR/VR glasses/headsets particularly challenging.
In the 1990s, AR/VR experienced the first boom, which quickly subsided due to the lack of eligible hardware and digital content 6 . Over the past decade, the concept of immersive displays was revisited and received a new round of excitement. Emerging technologies like holography and lithography have greatly reshaped the AR/VR display systems. In this article, we firstly review the basic requirements of AR/VR displays and their associated challenges. Then, we briefly describe the properties of two emerging technologies: holographic optical elements (HOEs) and lithography-based devices (Fig. 1 ). Next, we separately introduce VR and AR systems because of their different device structures and requirements. For the immersive VR system, the major challenges and how these emerging technologies help mitigate the problems will be discussed. For the see-through AR system, we firstly review the present status of light engines and introduce some architectures for the optical combiners. Performance summaries on microdisplay light engines and optical combiners will be provided, that serve as a comprehensive overview of the current AR display systems.
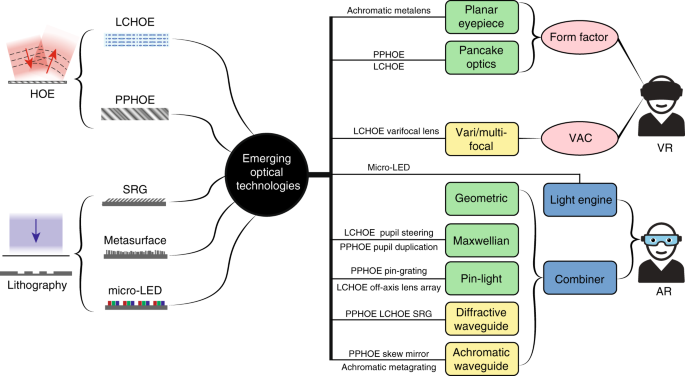
The left side illustrates HOEs and lithography-based devices. The right side shows the challenges in VR and architectures in AR, and how the emerging technologies can be applied
Key parameters of AR and VR displays
AR and VR displays face several common challenges to satisfy the demanding human vision requirements, such as FoV, eyebox, angular resolution, dynamic range, and correct depth cue, etc. These requirements often exhibit tradeoffs with one another. Before diving into detailed relations, it is beneficial to review the basic definitions of the above-mentioned display parameters.
Definition of parameters
Taking a VR system (Fig. 2a ) as an example. The light emitting from the display module is projected to a FoV, which can be translated to the size of the image perceived by the viewer. For reference, human vision’s horizontal FoV can be as large as 160° for monocular vision and 120° for overlapped binocular vision 6 . The intersection area of ray bundles forms the exit pupil, which is usually correlated with another parameter called eyebox. The eyebox defines the region within which the whole image FoV can be viewed without vignetting. It therefore generally manifests a 3D geometry 7 , whose volume is strongly dependent on the exit pupil size. A larger eyebox offers more tolerance to accommodate the user’s diversified interpupillary distance (IPD) and wiggling of headset when in use. Angular resolution is defined by dividing the total resolution of the display panel by FoV, which measures the sharpness of a perceived image. For reference, a human visual acuity of 20/20 amounts to 1 arcmin angular resolution, or 60 pixels per degree (PPD), which is considered as a common goal for AR and VR displays. Another important feature of a 3D display is depth cue. Depth cue can be induced by displaying two separate images to the left eye and the right eye, which forms the vergence cue. But the fixed depth of the displayed image often mismatches with the actual depth of the intended 3D image, which leads to incorrect accommodation cues. This mismatch causes the so-called vergence-accommodation conflict (VAC), which will be discussed in detail later. One important observation is that the VAC issue may be more serious in AR than VR, because the image in an AR display is directly superimposed onto the real-world with correct depth cues. The image contrast is dependent on the display panel and stray light. To achieve a high dynamic range, the display panel should exhibit high brightness, low dark level, and more than 10-bits of gray levels. Nowadays, the display brightness of a typical VR headset is about 150–200 cd/m 2 (or nits).
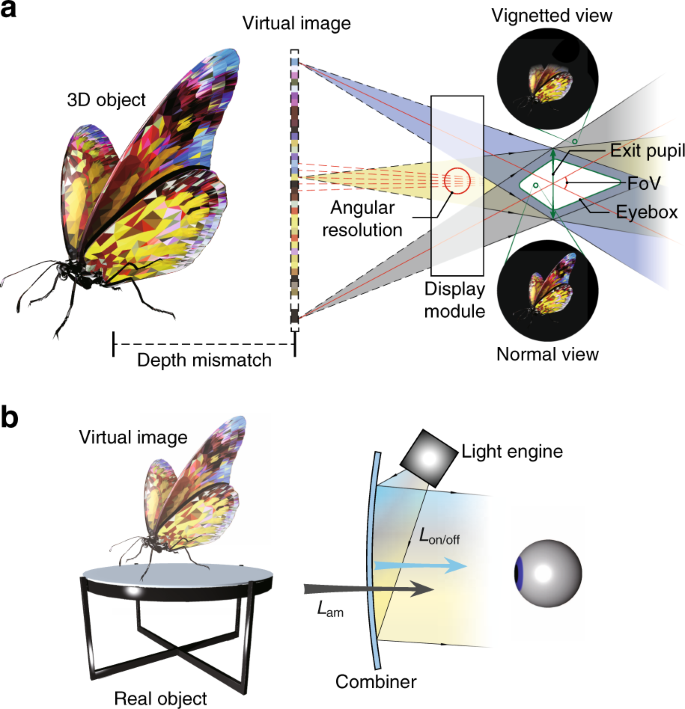
a Schematic of a VR display defining FoV, exit pupil, eyebox, angular resolution, and accommodation cue mismatch. b Sketch of an AR display illustrating ACR
Figure 2b depicts a generic structure of an AR display. The definition of above parameters remains the same. One major difference is the influence of ambient light on the image contrast. For a see-through AR display, ambient contrast ratio (ACR) 8 is commonly used to quantify the image contrast:
where L on ( L off ) represents the on (off)-state luminance (unit: nit), L am is the ambient luminance, and T is the see-through transmittance. In general, ambient light is measured in illuminance (lux). For the convenience of comparison, we convert illuminance to luminance by dividing a factor of π, assuming the emission profile is Lambertian. In a normal living room, the illuminance is about 100 lux (i.e., L am ≈ 30 nits), while in a typical office lighting condition, L am ≈ 150 nits. For outdoors, on an overcast day, L am ≈ 300 nits, and L am ≈ 3000 nits on a sunny day. For AR displays, a minimum ACR should be 3:1 for recognizable images, 5:1 for adequate readability, and ≥10:1 for outstanding readability. To make a simple estimate without considering all the optical losses, to achieve ACR = 10:1 in a sunny day (~3000 nits), the display needs to deliver a brightness of at least 30,000 nits. This imposes big challenges in finding a high brightness microdisplay and designing a low loss optical combiner.
Tradeoffs and potential solutions
Next, let us briefly review the tradeoff relations mentioned earlier. To begin with, a larger FoV leads to a lower angular resolution for a given display resolution. In theory, to overcome this tradeoff only requires a high-resolution-display source, along with high-quality optics to support the corresponding modulation transfer function (MTF). To attain 60 PPD across 100° FoV requires a 6K resolution for each eye. This may be realizable in VR headsets because a large display panel, say 2–3 inches, can still accommodate a high resolution with acceptable manufacture cost. However, for a glasses-like wearable AR display, the conflict between small display size and the high solution becomes obvious as further shrinking the pixel size of a microdisplay is challenging.
To circumvent this issue, the concept of the foveated display is proposed 9 , 10 , 11 , 12 , 13 . The idea is based on that the human eye only has high visual acuity in the central fovea region, which accounts for about 10° FoV. If the high-resolution image is only projected to fovea while the peripheral image remains low resolution, then a microdisplay with 2K resolution can satisfy the need. Regarding the implementation method of foveated display, a straightforward way is to optically combine two display sources 9 , 10 , 11 : one for foveal and one for peripheral FoV. This approach can be regarded as spatial multiplexing of displays. Alternatively, time-multiplexing can also be adopted, by temporally changing the optical path to produce different magnification factors for the corresponding FoV 12 . Finally, another approach without multiplexing is to use a specially designed lens with intended distortion to achieve non-uniform resolution density 13 . Aside from the implementation of foveation, another great challenge is to dynamically steer the foveated region as the viewer’s eye moves. This task is strongly related to pupil steering, which will be discussed in detail later.
A larger eyebox or FoV usually decreases the image brightness, which often lowers the ACR. This is exactly the case for a waveguide AR system with exit pupil expansion (EPE) while operating under a strong ambient light. To improve ACR, one approach is to dynamically adjust the transmittance with a tunable dimmer 14 , 15 . Another solution is to directly boost the image brightness with a high luminance microdisplay and an efficient combiner optics. Details of this topic will be discussed in the light engine section.
Another tradeoff of FoV and eyebox in geometric optical systems results from the conservation of etendue (or optical invariant). To increase the system etendue requires a larger optics, which in turn compromises the form factor. Finally, to address the VAC issue, the display system needs to generate a proper accommodation cue, which often requires the modulation of image depth or wavefront, neither of which can be easily achieved in a traditional geometric optical system. While remarkable progresses have been made to adopt freeform surfaces 16 , 17 , 18 , to further advance AR and VR systems requires additional novel optics with a higher degree of freedom in structure design and light modulation. Moreover, the employed optics should be thin and lightweight. To mitigate the above-mentioned challenges, diffractive optics is a strong contender. Unlike geometric optics relying on curved surfaces to refract or reflect light, diffractive optics only requires a thin layer of several micrometers to establish efficient light diffractions. Two major types of diffractive optics are HOEs based on wavefront recording and manually written devices like surface relief gratings (SRGs) based on lithography. While SRGs have large design freedoms of local grating geometry, a recent publication 19 indicates the combination of HOE and freeform optics can also offer a great potential for arbitrary wavefront generation. Furthermore, the advances in lithography have also enabled optical metasurfaces beyond diffractive and refractive optics, and miniature display panels like micro-LED (light-emitting diode). These devices hold the potential to boost the performance of current AR/VR displays, while keeping a lightweight and compact form factor.
Formation and properties of HOEs
HOE generally refers to a recorded hologram that reproduces the original light wavefront. The concept of holography is proposed by Dennis Gabor 20 , which refers to the process of recording a wavefront in a medium (hologram) and later reconstructing it with a reference beam. Early holography uses intensity-sensitive recording materials like silver halide emulsion, dichromated gelatin, and photopolymer 21 . Among them, photopolymer stands out due to its easy fabrication and ability to capture high-fidelity patterns 22 , 23 . It has therefore found extensive applications like holographic data storage 23 and display 24 , 25 . Photopolymer HOEs (PPHOEs) have a relatively small refractive index modulation and therefore exhibits a strong selectivity on the wavelength and incident angle. Another feature of PPHOE is that several holograms can be recorded into a photopolymer film by consecutive exposures. Later, liquid-crystal holographic optical elements (LCHOEs) based on photoalignment polarization holography have also been developed 25 , 26 . Due to the inherent anisotropic property of liquid crystals, LCHOEs are extremely sensitive to the polarization state of the input light. This feature, combined with the polarization modulation ability of liquid crystal devices, offers a new possibility for dynamic wavefront modulation in display systems.
The formation of PPHOE is illustrated in Fig. 3a . When exposed to an interfering field with high-and-low intensity fringes, monomers tend to move toward bright fringes due to the higher local monomer-consumption rate. As a result, the density and refractive index is slightly larger in bright regions. Note the index modulation δ n here is defined as the difference between the maximum and minimum refractive indices, which may be twice the value in other definitions 27 . The index modulation δ n is typically in the range of 0–0.06. To understand the optical properties of PPHOE, we simulate a transmissive grating and a reflective grating using rigorous coupled-wave analysis (RCWA) 28 , 29 and plot the results in Fig. 3b . Details of grating configuration can be found in Table S1 . Here, the reason for only simulating gratings is that for a general HOE, the local region can be treated as a grating. The observation of gratings can therefore offer a general insight of HOEs. For a transmissive grating, its angular bandwidth (efficiency > 80%) is around 5° ( λ = 550 nm), while the spectral band is relatively broad, with bandwidth around 175 nm (7° incidence). For a reflective grating, its spectral band is narrow, with bandwidth around 10 nm. The angular bandwidth varies with the wavelength, ranging from 2° to 20°. The strong selectivity of PPHOE on wavelength and incident angle is directly related to its small δ n , which can be adjusted by controlling the exposure dosage.
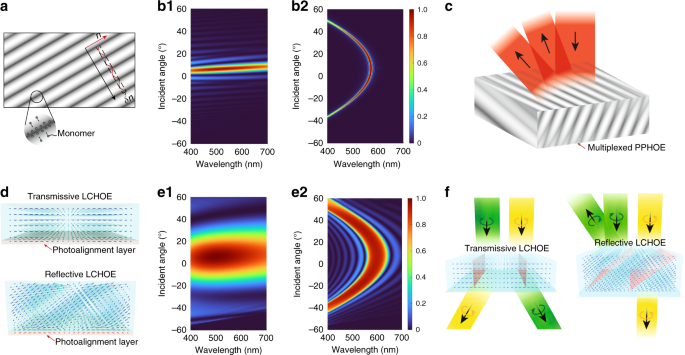
a Schematic of the formation of PPHOE. Simulated efficiency plots for b1 transmissive and b2 reflective PPHOEs. c Working principle of multiplexed PPHOE. d Formation and molecular configurations of LCHOEs. Simulated efficiency plots for e1 transmissive and e2 reflective LCHOEs. f Illustration of polarization dependency of LCHOEs
A distinctive feature of PPHOE is the ability to multiplex several holograms into one film sample. If the exposure dosage of a recording process is controlled so that the monomers are not completely depleted in the first exposure, the remaining monomers can continue to form another hologram in the following recording process. Because the total amount of monomer is fixed, there is usually an efficiency tradeoff between multiplexed holograms. The final film sample would exhibit the wavefront modulation functions of multiple holograms (Fig. 3c ).
Liquid crystals have also been used to form HOEs. LCHOEs can generally be categorized into volume-recording type and surface-alignment type. Volume-recording type LCHOEs are either based on early polarization holography recordings with azo-polymer 30 , 31 , or holographic polymer-dispersed liquid crystals (HPDLCs) 32 , 33 formed by liquid-crystal-doped photopolymer. Surface-alignment type LCHOEs are based on photoalignment polarization holography (PAPH) 34 . The first step is to record the desired polarization pattern in a thin photoalignment layer, and the second step is to use it to align the bulk liquid crystal 25 , 35 . Due to the simple fabrication process, high efficiency, and low scattering from liquid crystal’s self-assembly nature, surface-alignment type LCHOEs based on PAPH have recently attracted increasing interest in applications like near-eye displays. Here, we shall focus on this type of surface-alignment LCHOE and refer to it as LCHOE thereafter for simplicity.
The formation of LCHOEs is illustrated in Fig. 3d . The information of the wavefront and the local diffraction pattern is recorded in a thin photoalignment layer. The volume liquid crystal deposited on the photoalignment layer, depending on whether it is nematic liquid crystal or cholesteric liquid crystal (CLC), forms a transmissive or a reflective LCHOE. In a transmissive LCHOE, the bulk nematic liquid crystal molecules generally follow the pattern of the bottom alignment layer. The smallest allowable pattern period is governed by the liquid crystal distortion-free energy model, which predicts the pattern period should generally be larger than sample thickness 36 , 37 . This results in a maximum diffraction angle under 20°. On the other hand, in a reflective LCHOE 38 , 39 , the bulk CLC molecules form a stable helical structure, which is tilted to match the k -vector of the bottom pattern. The structure exhibits a very low distorted free energy 40 , 41 and can accommodate a pattern period that is small enough to diffract light into the total internal reflection (TIR) of a glass substrate.
The diffraction property of LCHOEs is shown in Fig. 3e . The maximum refractive index modulation of LCHOE is equal to the liquid crystal birefringence (Δ n ), which may vary from 0.04 to 0.5, depending on the molecular conjugation 42 , 43 . The birefringence used in our simulation is Δ n = 0.15. Compared to PPHOEs, the angular and spectral bandwidths are significantly larger for both transmissive and reflective LCHOEs. For a transmissive LCHOE, its angular bandwidth is around 20° ( λ = 550 nm), while the spectral bandwidth is around 300 nm (7° incidence). For a reflective LCHOE, its spectral bandwidth is around 80 nm and angular bandwidth could vary from 15° to 50°, depending on the wavelength.
The anisotropic nature of liquid crystal leads to LCHOE’s unique polarization-dependent response to an incident light. As depicted in Fig. 3f , for a transmissive LCHOE the accumulated phase is opposite for the conjugated left-handed circular polarization (LCP) and right-handed circular polarization (RCP) states, leading to reversed diffraction directions. For a reflective LCHOE, the polarization dependency is similar to that of a normal CLC. For the circular polarization with the same handedness as the helical structure of CLC, the diffraction is strong. For the opposite circular polarization, the diffraction is negligible.
Another distinctive property of liquid crystal is its dynamic response to an external voltage. The LC reorientation can be controlled with a relatively low voltage (<10 V rms ) and the response time is on the order of milliseconds, depending mainly on the LC viscosity and layer thickness. Methods to dynamically control LCHOEs can be categorized as active addressing and passive addressing, which can be achieved by either directly switching the LCHOE or modulating the polarization state with an active waveplate. Detailed addressing methods will be described in the VAC section.
Lithography-enabled devices
Lithography technologies are used to create arbitrary patterns on wafers, which lays the foundation of the modern integrated circuit industry 44 . Photolithography is suitable for mass production while electron/ion beam lithography is usually used to create photomask for photolithography or to write structures with nanometer-scale feature size. Recent advances in lithography have enabled engineered structures like optical metasurfaces 45 , SRGs 46 , as well as micro-LED displays 47 . Metasurfaces exhibit a remarkable design freedom by varying the shape of meta-atoms, which can be utilized to achieve novel functions like achromatic focus 48 and beam steering 49 . Similarly, SRGs also offer a large design freedom by manipulating the geometry of local grating regions to realize desired optical properties. On the other hand, micro-LED exhibits several unique features, such as ultrahigh peak brightness, small aperture ratio, excellent stability, and nanosecond response time, etc. As a result, micro-LED is a promising candidate for AR and VR systems for achieving high ACR and high frame rate for suppressing motion image blurs. In the following section, we will briefly review the fabrication and properties of micro-LEDs and optical modulators like metasurfaces and SRGs.
Fabrication and properties of micro-LEDs
LEDs with a chip size larger than 300 μm have been widely used in solid-state lighting and public information displays. Recently, micro-LEDs with chip sizes <5 μm have been demonstrated 50 . The first micro-LED disc with a diameter of about 12 µm was demonstrated in 2000 51 . After that, a single color (blue or green) LED microdisplay was demonstrated in 2012 52 . The high peak brightness, fast response time, true dark state, and long lifetime of micro-LEDs are attractive for display applications. Therefore, many companies have since released their micro-LED prototypes or products, ranging from large-size TVs to small-size microdisplays for AR/VR applications 53 , 54 . Here, we focus on micro-LEDs for near-eye display applications. Regarding the fabrication of micro-LEDs, through the metal-organic chemical vapor deposition (MOCVD) method, the AlGaInP epitaxial layer is grown on GaAs substrate for red LEDs, and GaN epitaxial layers on sapphire substrate for green and blue LEDs. Next, a photolithography process is applied to define the mesa and deposit electrodes. To drive the LED array, the fabricated micro-LEDs are transferred to a CMOS (complementary metal oxide semiconductor) driver board. For a small size (<2 inches) microdisplay used in AR or VR, the precision of the pick-and-place transfer process is hard to meet the high-resolution-density (>1000 pixel per inch) requirement. Thus, the main approach to assemble LED chips with driving circuits is flip-chip bonding 50 , 55 , 56 , 57 , as Fig. 4a depicts. In flip-chip bonding, the mesa and electrode pads should be defined and deposited before the transfer process, while metal bonding balls should be preprocessed on the CMOS substrate. After that, thermal-compression method is used to bond the two wafers together. However, due to the thermal mismatch of LED chip and driving board, as the pixel size decreases, the misalignment between the LED chip and the metal bonding ball on the CMOS substrate becomes serious. In addition, the common n-GaN layer may cause optical crosstalk between pixels, which degrades the image quality. To overcome these issues, the LED epitaxial layer can be firstly metal-bonded with the silicon driver board, followed by the photolithography process to define the LED mesas and electrodes. Without the need for an alignment process, the pixel size can be reduced to <5 µm 50 .
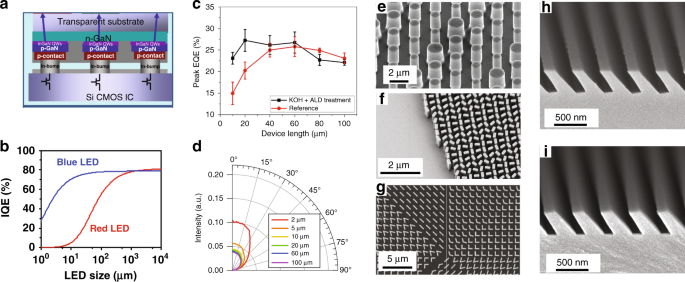
a Illustration of flip-chip bonding technology. b Simulated IQE-LED size relations for red and blue LEDs based on ABC model. c Comparison of EQE of different LED sizes with and without KOH and ALD side wall treatment. d Angular emission profiles of LEDs with different sizes. Metasurfaces based on e resonance-tuning, f non-resonance tuning and g combination of both. h Replication master and i replicated SRG based on nanoimprint lithography. Reproduced from a ref. 55 with permission from AIP Publishing, b ref. 61 with permission from PNAS, c ref. 66 with permission from IOP Publishing, d ref. 67 with permission from AIP Publishing, e ref. 69 with permission from OSA Publishing f ref. 48 with permission from AAAS g ref. 70 with permission from AAAS and h , i ref. 85 with permission from OSA Publishing
In addition to manufacturing process, the electrical and optical characteristics of LED also depend on the chip size. Generally, due to Shockley-Read-Hall (SRH) non-radiative recombination on the sidewall of active area, a smaller LED chip size results in a lower internal quantum efficiency (IQE), so that the peak IQE driving point will move toward a higher current density due to increased ratio of sidewall surface to active volume 58 , 59 , 60 . In addition, compared to the GaN-based green and blue LEDs, the AlGaInP-based red LEDs with a larger surface recombination and carrier diffusion length suffer a more severe efficiency drop 61 , 62 . Figure 4b shows the simulated result of IQE drop in relation with the LED chip size of blue and red LEDs based on ABC model 63 . To alleviate the efficiency drop caused by sidewall defects, depositing passivation materials by atomic layer deposition (ALD) or plasma enhanced chemical vapor deposition (PECVD) is proven to be helpful for both GaN and AlGaInP based LEDs 64 , 65 . In addition, applying KOH (Potassium hydroxide) treatment after ALD can further reduce the EQE drop of micro-LEDs 66 (Fig. 4c ). Small-size LEDs also exhibit some advantages, such as higher light extraction efficiency (LEE). Compared to an 100-µm LED, the LEE of a 2-µm LED increases from 12.2 to 25.1% 67 . Moreover, the radiation pattern of micro-LED is more directional than that of a large-size LED (Fig. 4d ). This helps to improve the lens collection efficiency in AR/VR display systems.
Metasurfaces and SGs
Thanks to the advances in lithography technology, low-loss dielectric metasurfaces working in the visible band have recently emerged as a platform for wavefront shaping 45 , 48 , 68 . They consist of an array of subwavelength-spaced structures with individually engineered wavelength-dependent polarization/phase/ amplitude response. In general, the light modulation mechanisms can be classified into resonant tuning 69 (Fig. 4e ), non-resonant tuning 48 (Fig. 4f ), and combination of both 70 (Fig. 4g ). In comparison with non-resonant tuning (based on geometric phase and/or dynamic propagation phase), the resonant tuning (such as Fabry–Pérot resonance, Mie resonance, etc.) is usually associated with a narrower operating bandwidth and a smaller out-of-plane aspect ratio (height/width) of nanostructures. As a result, they are easier to fabricate but more sensitive to fabrication tolerances. For both types, materials with a higher refractive index and lower absorption loss are beneficial to reduce the aspect ratio of nanostructure and improve the device efficiency. To this end, titanium dioxide (TiO 2 ) and gallium nitride (GaN) are the major choices for operating in the entire visible band 68 , 71 . While small-sized metasurfaces (diameter <1 mm) are usually fabricated via electron-beam lithography or focused ion beam milling in the labs, the ability of mass production is the key to their practical adoption. The deep ultraviolet (UV) photolithography has proven its feasibility for reproducing centimeter-size metalenses with decent imaging performance, while it requires multiple steps of etching 72 . Interestingly, the recently developed UV nanoimprint lithography based on a high-index nanocomposite only takes a single step and can obtain an aspect ratio larger than 10, which shows great promise for high-volume production 73 .
The arbitrary wavefront shaping capability and the thinness of the metasurfaces have aroused strong research interests in the development of novel AR/VR prototypes with improved performance. Lee et al. employed nanoimprint lithography to fabricate a centimeter-size, geometric-phase metalens eyepiece for full-color AR displays 74 . Through tailoring its polarization conversion efficiency and stacking with a circular polarizer, the virtual image can be superimposed with the surrounding scene. The large numerical aperture (NA~0.5) of the metalens eyepiece enables a wide FoV (>76°) that conventional optics are difficult to obtain. However, the geometric phase metalens is intrinsically a diffractive lens that also suffers from strong chromatic aberrations. To overcome this issue, an achromatic lens can be designed via simultaneously engineering the group delay and the group delay dispersion 75 , 76 , which will be described in detail later. Other novel and/or improved near-eye display architectures include metasurface-based contact lens-type AR 77 , achromatic metalens array enabled integral-imaging light field displays 78 , wide FoV lightguide AR with polarization-dependent metagratings 79 , and off-axis projection-type AR with an aberration-corrected metasurface combiner 80 , 81 , 82 . Nevertheless, from the existing AR/VR prototypes, metasurfaces still face a strong tradeoff between numerical aperture (for metalenses), chromatic aberration, monochromatic aberration, efficiency, aperture size, and fabrication complexity.
On the other hand, SRGs are diffractive gratings that have been researched for decades as input/output couplers of waveguides 83 , 84 . Their surface is composed of corrugated microstructures, and different shapes including binary, blazed, slanted, and even analogue can be designed. The parameters of the corrugated microstructures are determined by the target diffraction order, operation spectral bandwidth, and angular bandwidth. Compared to metasurfaces, SRGs have a much larger feature size and thus can be fabricated via UV photolithography and subsequent etching. They are usually replicated by nanoimprint lithography with appropriate heating and surface treatment. According to a report published a decade ago, SRGs with a height of 300 nm and a slant angle of up to 50° can be faithfully replicated with high yield and reproducibility 85 (Fig. 4g, h ).
Challenges and solutions of VR displays
The fully immersive nature of VR headset leads to a relatively fixed configuration where the display panel is placed in front of the viewer’s eye and an imaging optics is placed in-between. Regarding the system performance, although inadequate angular resolution still exists in some current VR headsets, the improvement of display panel resolution with advanced fabrication process is expected to solve this issue progressively. Therefore, in the following discussion, we will mainly focus on two major challenges: form factor and 3D cue generation.
Form factor
Compact and lightweight near-eye displays are essential for a comfortable user experience and therefore highly desirable in VR headsets. Current mainstream VR headsets usually have a considerably larger volume than eyeglasses, and most of the volume is just empty. This is because a certain distance is required between the display panel and the viewing optics, which is usually close to the focal length of the lens system as illustrated in Fig. 5a . Conventional VR headsets employ a transmissive lens with ~4 cm focal length to offer a large FoV and eyebox. Fresnel lenses are thinner than conventional ones, but the distance required between the lens and the panel does not change significantly. In addition, the diffraction artifacts and stray light caused by the Fresnel grooves can degrade the image quality, or MTF. Although the resolution density, quantified as pixel per inch (PPI), of current VR headsets is still limited, eventually Fresnel lens will not be an ideal solution when a high PPI display is available. The strong chromatic aberration of Fresnel singlet should also be compensated if a high-quality imaging system is preferred.
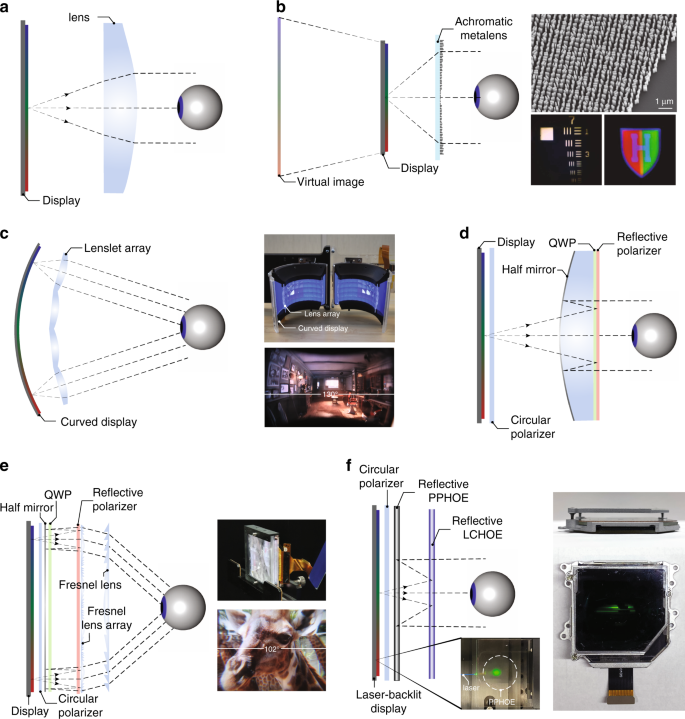
a Schematic of a basic VR optical configuration. b Achromatic metalens used as VR eyepiece. c VR based on curved display and lenslet array. d Basic working principle of a VR display based on pancake optics. e VR with pancake optics and Fresnel lens array. f VR with pancake optics based on purely HOEs. Reprinted from b ref. 87 under the Creative Commons Attribution 4.0 License. Adapted from c ref. 88 with permission from IEEE, e ref. 91 and f ref. 92 under the Creative Commons Attribution 4.0 License
It is tempting to replace the refractive elements with a single thin diffractive lens like a transmissive LCHOE. However, the diffractive nature of such a lens will result in serious color aberrations. Interestingly, metalenses can fulfil this objective without color issues. To understand how metalenses achieve achromatic focus, let us first take a glance at the general lens phase profile \(\Phi (\omega ,r)\) expanded as a Taylor series 75 :
where \(\varphi _0(\omega )\) is the phase at the lens center, \(F\left( \omega \right)\) is the focal length as a function of frequency ω , r is the radial coordinate, and \(\omega _0\) is the central operation frequency. To realize achromatic focus, \(\partial F{{{\mathrm{/}}}}\partial \omega\) should be zero. With a designed focal length, the group delay \(\partial \Phi (\omega ,r){{{\mathrm{/}}}}\partial \omega\) and the group delay dispersion \(\partial ^2\Phi (\omega ,r){{{\mathrm{/}}}}\partial \omega ^2\) can be determined, and \(\varphi _0(\omega )\) is an auxiliary degree of freedom of the phase profile design. In the design of an achromatic metalens, the group delay is a function of the radial coordinate and monotonically increases with the metalens radius. Many designs have proven that the group delay has a limited variation range 75 , 76 , 78 , 86 . According to Shrestha et al. 86 , there is an inevitable tradeoff between the maximum radius of the metalens, NA, and operation bandwidth. Thus, the reported achromatic metalenses at visible usually have limited lens aperture (e.g., diameter < 250 μm) and NA (e.g., <0.2). Such a tradeoff is undesirable in VR displays, as the eyepiece favors a large clear aperture (inch size) and a reasonably high NA (>0.3) to maintain a wide FoV and a reasonable eye relief 74 .
To overcome this limitation, Li et al. 87 proposed a novel zone lens method. Unlike the traditional phase Fresnel lens where the zones are determined by the phase reset, the new approach divides the zones by the group delay reset. In this way, the lens aperture and NA can be much enlarged, and the group delay limit is bypassed. A notable side effect of this design is the phase discontinuity at zone boundaries that will contribute to higher-order focusing. Therefore, significant efforts have been conducted to find the optimal zone transition locations and to minimize the phase discontinuities. Using this method, they have demonstrated an impressive 2-mm-diameter metalens with NA = 0.7 and nearly diffraction-limited focusing for the designed wavelengths (488, 532, 658 nm) (Fig. 5b ). Such a metalens consists of 681 zones and works for the visible band ranging from 470 to 670 nm, though the focusing efficiency is in the order of 10%. This is a great starting point for the achromatic metalens to be employed as a compact, chromatic-aberration-free eyepiece in near-eye displays. Future challenges are how to further increase the aperture size, correct the off-axis aberrations, and improve the optical efficiency.
Besides replacing the refractive lens with an achromatic metalens, another way to reduce system focal length without decreasing NA is to use a lenslet array 88 . As depicted in Fig. 5c , both the lenslet array and display panel adopt a curved structure. With the latest flexible OLED panel, the display can be easily curved in one dimension. The system exhibits a large diagonal FoV of 180° with an eyebox of 19 by 12 mm. The geometry of each lenslet is optimized separately to achieve an overall performance with high image quality and reduced distortions.
Aside from trying to shorten the system focal length, another way to reduce total track is to fold optical path. Recently, polarization-based folded lenses, also known as pancake optics, are under active development for VR applications 89 , 90 . Figure 5d depicts the structure of an exemplary singlet pancake VR lens system. The pancake lenses can offer better imaging performance with a compact form factor since there are more degrees of freedom in the design and the actual light path is folded thrice. By using a reflective surface with a positive power, the field curvature of positive refractive lenses can be compensated. Also, the reflective surface has no chromatic aberrations and it contributes considerable optical power to the system. Therefore, the optical power of refractive lenses can be smaller, resulting in an even weaker chromatic aberration. Compared to Fresnel lenses, the pancake lenses have smooth surfaces and much fewer diffraction artifacts and stray light. However, such a pancake lens design is not perfect either, whose major shortcoming is low light efficiency. With two incidences of light on the half mirror, the maximum system efficiency is limited to 25% for a polarized input and 12.5% for an unpolarized input light. Moreover, due to the existence of multiple surfaces in the system, stray light caused by surface reflections and polarization leakage may lead to apparent ghost images. As a result, the catadioptric pancake VR headset usually manifests a darker imagery and lower contrast than the corresponding dioptric VR.
Interestingly, the lenslet and pancake optics can be combined to further reduce the system form. Bang et al. 91 demonstrated a compact VR system with a pancake optics and a Fresnel lenslet array. The pancake optics serves to fold the optical path between the display panel and the lenslet array (Fig. 5e ). Another Fresnel lens is used to collect the light from the lenslet array. The system has a decent horizontal FoV of 102° and an eyebox of 8 mm. However, a certain degree of image discontinuity and crosstalk are still present, which can be improved with further optimizations on the Fresnel lens and the lenslet array.
One step further, replacing all conventional optics in catadioptric VR headset with holographic optics can make the whole system even thinner. Maimone and Wang demonstrated such a lightweight, high-resolution, and ultra-compact VR optical system using purely HOEs 92 . This holographic VR optics was made possible by combining several innovative optical components, including a reflective PPHOE, a reflective LCHOE, and a PPHOE-based directional backlight with laser illumination, as shown in Fig. 5f . Since all the optical power is provided by the HOEs with negligible weight and volume, the total physical thickness can be reduced to <10 mm. Also, unlike conventional bulk optics, the optical power of a HOE is independent of its thickness, only subject to the recording process. Another advantage of using holographic optical devices is that they can be engineered to offer distinct phase profiles for different wavelengths and angles of incidence, adding extra degrees of freedom in optical designs for better imaging performance. Although only a single-color backlight has been demonstrated, such a PPHOE has the potential to achieve full-color laser backlight with multiplexing ability. The PPHOE and LCHOE in the pancake optics can also be optimized at different wavelengths for achieving high-quality full-color images.
Vergence-accommodation conflict
Conventional VR displays suffer from VAC, which is a common issue for stereoscopic 3D displays 93 . In current VR display modules, the distance between the display panel and the viewing optics is fixed, which means the VR imagery is displayed at a single depth. However, the image contents are generated by parallax rendering in three dimensions, offering distinct images for two eyes. This approach offers a proper stimulus to vergence but completely ignores the accommodation cue, which leads to the well-known VAC that can cause an uncomfortable user experience. Since the beginning of this century, numerous methods have been proposed to solve this critical issue. Methods to produce accommodation cue include multifocal/varifocal display 94 , holographic display 95 , and integral imaging display 96 . Alternatively, elimination of accommodation cue using a Maxwellian-view display 93 also helps to mitigate the VAC. However, holographic displays and Maxwellian-view displays generally require a totally different optical architecture than current VR systems. They are therefore more suitable for AR displays, which will be discussed later. Integral imaging, on the other hand, has an inherent tradeoff between view number and resolution. For current VR headsets pursuing high resolution to match human visual acuity, it may not be an appealing solution. Therefore, multifocal/varifocal displays that rely on depth modulation is a relatively practical and effective solution for VR headsets. Regarding the working mechanism, multifocal displays present multiple images with different depths to imitate the original 3D scene. Varifocal displays, in contrast, only show one image at each time frame. The image depth matches the viewer’s vergence depth. Nonetheless, the pre-knowledge of the viewer’s vergence depth requires an additional eye-tracking module. Despite different operation principles, a varifocal display can often be converted to a multifocal display as long as the varifocal module has enough modulation bandwidth to support multiple depths in a time frame.
To achieve depth modulation in a VR system, traditional liquid lens 97 , 98 with tunable focus suffers from the small aperture and large aberrations. Alvarez lens 99 is another tunable-focus solution but it requires mechanical adjustment, which adds to system volume and complexity. In comparison, transmissive LCHOEs with polarization dependency can achieve focus adjustment with electronic driving. Its ultra-thinness also satisfies the requirement of small form factors in VR headsets. The diffractive behavior of transmissive LCHOEs is often interpreted by the mechanism of Pancharatnam-Berry phase (also known as geometric phase) 100 . They are therefore often called Pancharatnam-Berry optical elements (PBOEs). The corresponding lens component is referred as Pancharatnam-Berry lens (PBL).
Two main approaches are used to switch the focus of a PBL, active addressing and passive addressing. In active addressing, the PBL itself (made of LC) can be switched by an applied voltage (Fig. 6a ). The optical power of the liquid crystal PBLs can be turned-on and -off by controlling the voltage. Stacking multiple active PBLs can produce 2 N depths, where N is the number of PBLs. The drawback of using active PBLs, however, is the limited spectral bandwidth since their diffraction efficiency is usually optimized at a single wavelength. In passive addressing, the depth modulation is achieved through changing the polarization state of input light by a switchable half-wave plate (HWP) (Fig. 6b ). The focal length can therefore be switched thanks to the polarization sensitivity of PBLs. Although this approach has a slightly more complicated structure, the overall performance can be better than the active one, because the PBLs made of liquid crystal polymer can be designed to manifest high efficiency within the entire visible spectrum 101 , 102 .
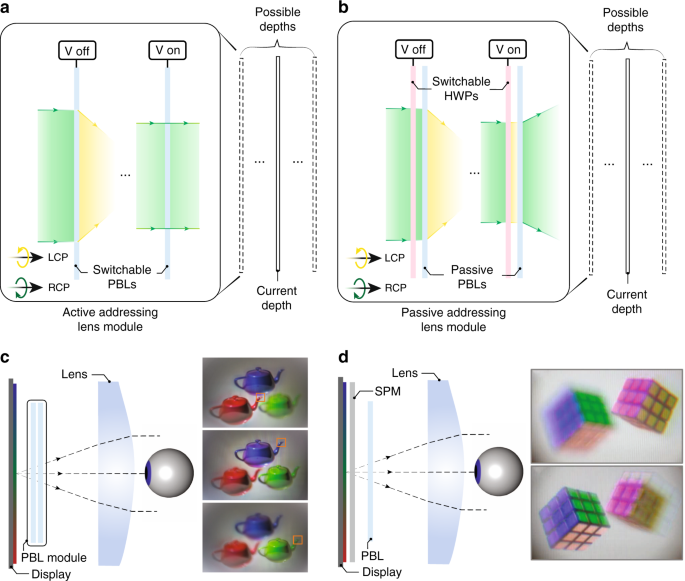
Working principles of a depth switching PBL module based on a active addressing and b passive addressing. c A four-depth multifocal display based on time multiplexing. d A two-depth multifocal display based on polarization multiplexing. Reproduced from c ref. 103 with permission from OSA Publishing and d ref. 104 with permission from OSA Publishing
With the PBL module, multifocal displays can be built using time-multiplexing technique. Zhan et al. 103 demonstrated a four-depth multifocal display using two actively switchable liquid crystal PBLs (Fig. 6c ). The display is synchronized with the PBL module, which lowers the frame rate by the number of depths. Alternatively, multifocal displays can also be achieved by polarization-multiplexing, as demonstrated by Tan et al. 104 . The basic principle is to adjust the polarization state of local pixels so the image content on two focal planes of a PBL can be arbitrarily controlled (Fig. 6d ). The advantage of polarization multiplexing is that it does not sacrifice the frame rate, but it can only support two planes because only two orthogonal polarization states are available. Still, it can be combined with time-multiplexing to reduce the frame rate sacrifice by half. Naturally, varifocal displays can also be built with a PBL module. A fast-response 64-depth varifocal module with six PBLs has been demonstrated 105 .
The compact structure of PBL module leads to a natural solution of integrating it with above-mentioned pancake optics. A compact VR headset with dynamic depth modulation to solve VAC is therefore possible in practice. Still, due to the inherent diffractive nature of PBL, the PBL module face the issue of chromatic dispersion of focal length. To compensate for different focal depths for RGB colors may require additional digital corrections in image-rendering.
Architectures of AR displays
Unlike VR displays with a relatively fixed optical configuration, there exist a vast number of architectures in AR displays. Therefore, instead of following the narrative of tackling different challenges, a more appropriate way to review AR displays is to separately introduce each architecture and discuss its associated engineering challenges. An AR display usually consists of a light engine and an optical combiner. The light engine serves as display image source, while the combiner delivers the displayed images to viewer’s eye and in the meantime transmits the environment light. Some performance parameters like frame rate and power consumption are mainly determined by the light engine. Parameters like FoV, eyebox and MTF are primarily dependent on the combiner optics. Moreover, attributes like image brightness, overall efficiency, and form factor are influenced by both light engine and combiner. In this section, we will firstly discuss the light engine, where the latest advances in micro-LED on chip are reviewed and compared with existing microdisplay systems. Then, we will introduce two main types of combiners: free-space combiner and waveguide combiner.
Light engine
The light engine determines several essential properties of the AR system like image brightness, power consumption, frame rate, and basic etendue. Several types of microdisplays have been used in AR, including micro-LED, micro-organic-light-emitting-diodes (micro-OLED), liquid-crystal-on-silicon (LCoS), digital micromirror device (DMD), and laser beam scanning (LBS) based on micro-electromechanical system (MEMS). We will firstly describe the working principles of these devices and then analyze their performance. For those who are more interested in final performance parameters than details, Table 1 provides a comprehensive summary.
Working principles
Micro-LED and micro-OLED are self-emissive display devices. They are usually more compact than LCoS and DMD because no illumination optics is required. The fundamentally different material systems of LED and OLED lead to different approaches to achieve full-color displays. Due to the “green gap” in LEDs, red LEDs are manufactured on a different semiconductor material from green and blue LEDs. Therefore, how to achieve full-color display in high-resolution density microdisplays is quite a challenge for micro-LEDs. Among several solutions under research are two main approaches. The first is to combine three separate red, green and blue (RGB) micro-LED microdisplay panels 106 . Three single-color micro-LED microdisplays are manufactured separately through flip-chip transfer technology. Then, the projected images from three microdisplay panels are integrated by a trichroic prism (Fig. 7a ).
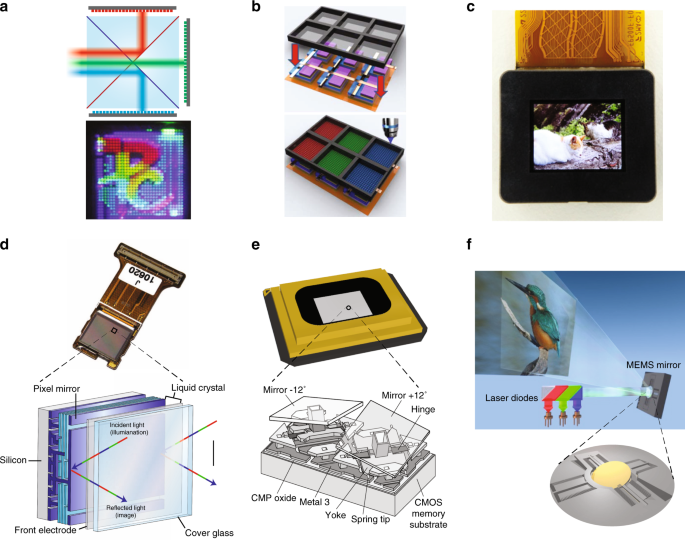
a RGB micro-LED microdisplays combined by a trichroic prism. b QD-based micro-LED microdisplay. c Micro-OLED display with 4032 PPI. Working principles of d LCoS, e DMD, and f MEMS-LBS display modules. Reprinted from a ref. 106 with permission from IEEE, b ref. 108 with permission from Chinese Laser Press, c ref. 121 with permission from Jon Wiley and Sons, d ref. 124 with permission from Spring Nature, e ref. 126 with permission from Springer and f ref. 128 under the Creative Commons Attribution 4.0 License
Another solution is to assemble color-conversion materials like quantum dot (QD) on top of blue or ultraviolet (UV) micro-LEDs 107 , 108 , 109 (Fig. 7b ). The quantum dot color filter (QDCF) on top of the micro-LED array is mainly fabricated by inkjet printing or photolithography 110 , 111 . However, the display performance of color-conversion micro-LED displays is restricted by the low color-conversion efficiency, blue light leakage, and color crosstalk. Extensive efforts have been conducted to improve the QD-micro-LED performance. To boost QD conversion efficiency, structure designs like nanoring 112 and nanohole 113 , 114 have been proposed, which utilize the Förster resonance energy transfer mechanism to transfer excessive excitons in the LED active region to QD. To prevent blue light leakage, methods using color filters or reflectors like distributed Bragg reflector (DBR) 115 and CLC film 116 on top of QDCF are proposed. Compared to color filters that absorb blue light, DBR and CLC film help recycle the leaked blue light to further excite QDs. Other methods to achieve full-color micro-LED display like vertically stacked RGB micro-LED array 61 , 117 , 118 and monolithic wavelength tunable nanowire LED 119 are also under investigation.
Micro-OLED displays can be generally categorized into RGB OLED and white OLED (WOLED). RGB OLED displays have separate sub-pixel structures and optical cavities, which resonate at the desirable wavelength in RGB channels, respectively. To deposit organic materials onto the separated RGB sub-pixels, a fine metal mask (FMM) that defines the deposition area is required. However, high-resolution RGB OLED microdisplays still face challenges due to the shadow effect during the deposition process through FMM. In order to break the limitation, a silicon nitride film with small shadow has been proposed as a mask for high-resolution deposition above 2000 PPI (9.3 µm) 120 .
WOLED displays use color filters to generate color images. Without the process of depositing patterned organic materials, a high-resolution density up to 4000 PPI has been achieved 121 (Fig. 7c ). However, compared to RGB OLED, the color filters in WOLED absorb about 70% of the emitted light, which limits the maximum brightness of the microdisplay. To improve the efficiency and peak brightness of WOLED microdisplays, in 2019 Sony proposed to apply newly designed cathodes (InZnO) and microlens arrays on OLED microdisplays, which increased the peak brightness from 1600 nits to 5000 nits 120 . In addition, OLEDWORKs has proposed a multi-stacked OLED 122 with optimized microcavities whose emission spectra match the transmission bands of the color filters. The multi-stacked OLED shows a higher luminous efficiency (cd/A), but also requires a higher driving voltage. Recently, by using meta-mirrors as bottom reflective anodes, patterned microcavities with more than 10,000 PPI have been obtained 123 . The high-resolution meta-mirrors generate different reflection phases in the RGB sub-pixels to achieve desirable resonant wavelengths. The narrow emission spectra from the microcavity help to reduce the loss from color filters or even eliminate the need of color filters.
LCoS and DMD are light-modulating displays that generate images by controlling the reflection of each pixel. For LCoS, the light modulation is achieved by manipulating the polarization state of output light through independently controlling the liquid crystal reorientation in each pixel 124 , 125 (Fig. 7d ). Both phase-only and amplitude modulators have been employed. DMD is an amplitude modulation device. The modulation is achieved through controlling the tilt angle of bi-stable micromirrors 126 (Fig. 7e ). To generate an image, both LCoS and DMD rely on the light illumination systems, with LED or laser as light source. For LCoS, the generation of color image can be realized either by RGB color filters on LCoS (with white LEDs) or color-sequential addressing (with RGB LEDs or lasers). However, LCoS requires a linearly polarized light source. For an unpolarized LED light source, usually, a polarization recycling system 127 is implemented to improve the optical efficiency. For a single-panel DMD, the color image is mainly obtained through color-sequential addressing. In addition, DMD does not require a polarized light so that it generally exhibits a higher efficiency than LCoS if an unpolarized light source is employed.
MEMS-based LBS 128 , 129 utilizes micromirrors to directly scan RGB laser beams to form two-dimensional (2D) images (Fig. 7f ). Different gray levels are achieved by pulse width modulation (PWM) of the employed laser diodes. In practice, 2D scanning can be achieved either through a 2D scanning mirror or two 1D scanning mirrors with an additional focusing lens after the first mirror. The small size of MEMS mirror offers a very attractive form factor. At the same time, the output image has a large depth-of-focus (DoF), which is ideal for projection displays. One shortcoming, though, is that the small system etendue often hinders its applications in some traditional display systems.
Comparison of light engine performance
There are several important parameters for a light engine, including image resolution, brightness, frame rate, contrast ratio, and form factor. The resolution requirement (>2K) is similar for all types of light engines. The improvement of resolution is usually accomplished through the manufacturing process. Thus, here we shall focus on other three parameters.
Image brightness usually refers to the measured luminance of a light-emitting object. This measurement, however, may not be accurate for a light engine as the light from engine only forms an intermediate image, which is not directly viewed by the user. On the other hand, to solely focus on the brightness of a light engine could be misleading for a wearable display system like AR. Nowadays, data projectors with thousands of lumens are available. But the power consumption is too high for a battery-powered wearable AR display. Therefore, a more appropriate way to evaluate a light engine’s brightness is to use luminous efficacy (lm/W) measured by dividing the final output luminous flux (lm) by the input electric power (W). For a self-emissive device like micro-LED or micro-OLED, the luminous efficacy is directly determined by the device itself. However, for LCoS and DMD, the overall luminous efficacy should take into consideration the light source luminous efficacy, the efficiency of illumination optics, and the efficiency of the employed spatial light modulator (SLM). For a MEMS LBS engine, the efficiency of MEMS mirror can be considered as unity so that the luminous efficacy basically equals to that of the employed laser sources.
As mentioned earlier, each light engine has a different scheme for generating color images. Therefore, we separately list luminous efficacy of each scheme for a more inclusive comparison. For micro-LEDs, the situation is more complicated because the EQE depends on the chip size. Based on previous studies 130 , 131 , 132 , 133 , we separately calculate the luminous efficacy for RGB micro-LEDs with chip size ≈ 20 µm. For the scheme of direct combination of RGB micro-LEDs, the luminous efficacy is around 5 lm/W. For QD-conversion with blue micro-LEDs, the luminous efficacy is around 10 lm/W with the assumption of 100% color conversion efficiency, which has been demonstrated using structure engineering 114 . For micro-OLEDs, the calculated luminous efficacy is about 4–8 lm/W 120 , 122 . However, the lifetime and EQE of blue OLED materials depend on the driving current. To continuously display an image with brightness higher than 10,000 nits may dramatically shorten the device lifetime. The reason we compare the light engine at 10,000 nits is that it is highly desirable to obtain 1000 nits for the displayed image in order to keep ACR>3:1 with a typical AR combiner whose optical efficiency is lower than 10%.
For an LCoS engine using a white LED as light source, the typical optical efficiency of the whole engine is around 10% 127 , 134 . Then the engine luminous efficacy is estimated to be 12 lm/W with a 120 lm/W white LED source. For a color sequential LCoS using RGB LEDs, the absorption loss from color filters is eliminated, but the luminous efficacy of RGB LED source is also decreased to about 30 lm/W due to lower efficiency of red and green LEDs and higher driving current 135 . Therefore, the final luminous efficacy of the color sequential LCoS engine is also around 10 lm/W. If RGB linearly polarized lasers are employed instead of LEDs, then the LCoS engine efficiency can be quite high due to the high degree of collimation. The luminous efficacy of RGB laser source is around 40 lm/W 136 . Therefore, the laser-based LCoS engine is estimated to have a luminous efficacy of 32 lm/W, assuming the engine optical efficiency is 80%. For a DMD engine with RGB LEDs as light source, the optical efficiency is around 50% 137 , 138 , which leads to a luminous efficacy of 15 lm/W. By switching to laser light sources, the situation is similar to LCoS, with the luminous efficacy of about 32 lm/W. Finally, for MEMS-based LBS engine, there is basically no loss from the optics so that the final luminous efficacy is 40 lm/W. Detailed calculations of luminous efficacy can be found in Supplementary Information .
Another aspect of a light engine is the frame rate, which determines the volume of information it can deliver in a unit time. A high volume of information is vital for the construction of a 3D light field to solve the VAC issue. For micro-LEDs, the device response time is around several nanoseconds, which allows for visible light communication with bandwidth up to 1.5 Gbit/s 139 . For an OLED microdisplay, a fast OLED with ~200 MHz bandwidth has been demonstrated 140 . Therefore, the limitation of frame rate is on the driving circuits for both micro-LED and OLED. Another fact concerning driving circuit is the tradeoff between resolution and frame rate as a higher resolution panel means more scanning lines in each frame. So far, an OLED display with 480 Hz frame rate has been demonstrated 141 . For an LCoS, the frame rate is mainly limited by the LC response time. Depending on the LC material used, the response time is around 1 ms for nematic LC or 200 µs for ferroelectric LC (FLC) 125 . Nematic LC allows analog driving, which accommodates gray levels, typically with 8-bit depth. FLC is bistable so that PWM is used to generate gray levels. DMD is also a binary device. The frame rate can reach 30 kHz, which is mainly constrained by the response time of micromirrors. For MEMS-based LBS, the frame rate is limited by the scanning frequency of MEMS mirrors. A frame rate of 60 Hz with around 1 K resolution already requires a resonance frequency of around 50 kHz, with a Q-factor up to 145,000 128 . A higher frame rate or resolution requires a higher Q-factor and larger laser modulation bandwidth, which may be challenging.
Form factor is another crucial aspect for the light engines of near-eye displays. For self-emissive displays, both micro-OLEDs and QD-based micro-LEDs can achieve full color with a single panel. Thus, they are quite compact. A micro-LED display with separate RGB panels naturally have a larger form factor. In applications requiring direct-view full-color panel, the extra combining optics may also increase the volume. It needs to be pointed out, however, that the combing optics may not be necessary for some applications like waveguide displays, because the EPE process results in system’s insensitivity to the spatial positions of input RGB images. Therefore, the form factor of using three RGB micro-LED panels is medium. For LCoS and DMD with RGB LEDs as light source, the form factor would be larger due to the illumination optics. Still, if a lower luminous efficacy can be accepted, then a smaller form factor can be achieved by using a simpler optics 142 . If RGB lasers are used, the collimation optics can be eliminated, which greatly reduces the form factor 143 . For MEMS-LBS, the form factor can be extremely compact due to the tiny size of MEMS mirror and laser module.
Finally, contrast ratio (CR) also plays an important role affecting the observed images 8 . Micro-LEDs and micro-OLEDs are self-emissive so that their CR can be >10 6 :1. For a laser beam scanner, its CR can also achieve 10 6 :1 because the laser can be turned off completely at dark state. On the other hand, LCoS and DMD are reflective displays, and their CR is around 2000:1 to 5000:1 144 , 145 . It is worth pointing out that the CR of a display engine plays a significant role only in the dark ambient. As the ambient brightness increases, the ACR is mainly governed by the display’s peak brightness, as previously discussed.
The performance parameters of different light engines are summarized in Table 1 . Micro-LEDs and micro-OLEDs have similar levels of luminous efficacy. But micro-OLEDs still face the burn-in and lifetime issue when driving at a high current, which hinders its use for a high-brightness image source to some extent. Micro-LEDs are still under active development and the improvement on luminous efficacy from maturing fabrication process could be expected. Both devices have nanosecond response time and can potentially achieve a high frame rate with a well-designed integrated circuit. The frame rate of the driving circuit ultimately determines the motion picture response time 146 . Their self-emissive feature also leads to a small form factor and high contrast ratio. LCoS and DMD engines have similar performance of luminous efficacy, form factor, and contrast ratio. In terms of light modulation, DMD can provide a higher 1-bit frame rate, while LCoS can offer both phase and amplitude modulations. MEMS-based LBS exhibits the highest luminous efficacy so far. It also exhibits an excellent form factor and contrast ratio, but the presently demonstrated 60-Hz frame rate (limited by the MEMS mirrors) could cause image flickering.
Free-space combiners
The term ‘free-space’ generally refers to the case when light is freely propagating in space, as opposed to a waveguide that traps light into TIRs. Regarding the combiner, it can be a partial mirror, as commonly used in AR systems based on traditional geometric optics. Alternatively, the combiner can also be a reflective HOE. The strong chromatic dispersion of HOE necessitates the use of a laser source, which usually leads to a Maxwellian-type system.
Traditional geometric designs
Several systems based on geometric optics are illustrated in Fig. 8 . The simplest design uses a single freeform half-mirror 6 , 147 to directly collimate the displayed images to the viewer’s eye (Fig. 8a ). This design can achieve a large FoV (up to 90°) 147 , but the limited design freedom with a single freeform surface leads to image distortions, also called pupil swim 6 . The placement of half-mirror also results in a relatively bulky form factor. Another design using so-called birdbath optics 6 , 148 is shown in Fig. 8b . Compared to the single-combiner design, birdbath design has an extra optics on the display side, which provides space for aberration correction. The integration of beam splitter provides a folded optical path, which reduces the form factor to some extent. Another way to fold optical path is to use a TIR-prism. Cheng et al. 149 designed a freeform TIR-prism combiner (Fig. 8c ) offering a diagonal FoV of 54° and exit pupil diameter of 8 mm. All the surfaces are freeform, which offer an excellent image quality. To cancel the optical power for the transmitted environmental light, a compensator is added to the TIR prism. The whole system has a well-balanced performance between FoV, eyebox, and form factor. To release the space in front of viewer’s eye, relay optics can be used to form an intermediate image near the combiner 150 , 151 , as illustrated in Fig. 8d . Although the design offers more optical surfaces for aberration correction, the extra lenses also add to system weight and form factor.
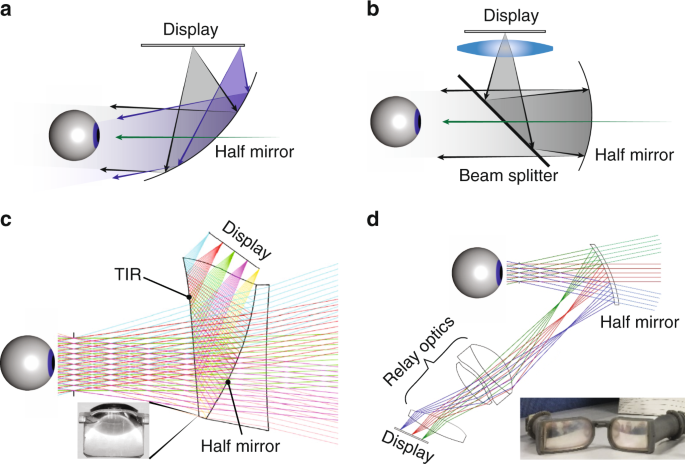
a Single freeform surface as the combiner. b Birdbath optics with a beam splitter and a half mirror. c Freeform TIR prism with a compensator. d Relay optics with a half mirror. Adapted from c ref. 149 with permission from OSA Publishing and d ref. 151 with permission from OSA Publishing
Regarding the approaches to solve the VAC issue, the most straightforward way is to integrate a tunable lens into the optical path, like a liquid lens 152 or Alvarez lens 99 , to form a varifocal system. Alternatively, integral imaging 153 , 154 can also be used, by replacing the original display panel with the central depth plane of an integral imaging module. The integral imaging can also be combined with varifocal approach to overcome the tradeoff between resolution and depth of field (DoF) 155 , 156 , 157 . However, the inherent tradeoff between resolution and view number still exists in this case.
Overall, AR displays based on traditional geometric optics have a relatively simple design with a decent FoV (~60°) and eyebox (8 mm) 158 . They also exhibit a reasonable efficiency. To measure the efficiency of an AR combiner, an appropriate measure is to divide the output luminance (unit: nit) by the input luminous flux (unit: lm), which we note as combiner efficiency. For a fixed input luminous flux, the output luminance, or image brightness, is related to the FoV and exit pupil of the combiner system. If we assume no light waste of the combiner system, then the maximum combiner efficiency for a typical diagonal FoV of 60° and exit pupil (10 mm square) is around 17,000 nit/lm (Eq. S2 ). To estimate the combiner efficiency of geometric combiners, we assume 50% of half-mirror transmittance and the efficiency of other optics to be 50%. Then the final combiner efficiency is about 4200 nit/lm, which is a high value in comparison with waveguide combiners. Nonetheless, to further shrink the system size or improve system performance ultimately encounters the etendue conservation issue. In addition, AR systems with traditional geometric optics is hard to achieve a configuration resembling normal flat glasses because the half-mirror has to be tilted to some extent.
Maxwellian-type systems
The Maxwellian view, proposed by James Clerk Maxwell (1860), refers to imaging a point light source in the eye pupil 159 . If the light beam is modulated in the imaging process, a corresponding image can be formed on the retina (Fig. 9a ). Because the point source is much smaller than the eye pupil, the image is always-in-focus on the retina irrespective of the eye lens’ focus. For applications in AR display, the point source is usually a laser with narrow angular and spectral bandwidths. LED light sources can also build a Maxwellian system, by adding an angular filtering module 160 . Regarding the combiner, although in theory a half-mirror can also be used, HOEs are generally preferred because they offer the off-axis configuration that places combiner in a similar position like eyeglasses. In addition, HOEs have a lower reflection of environment light, which provides a more natural appearance of the user behind the display.
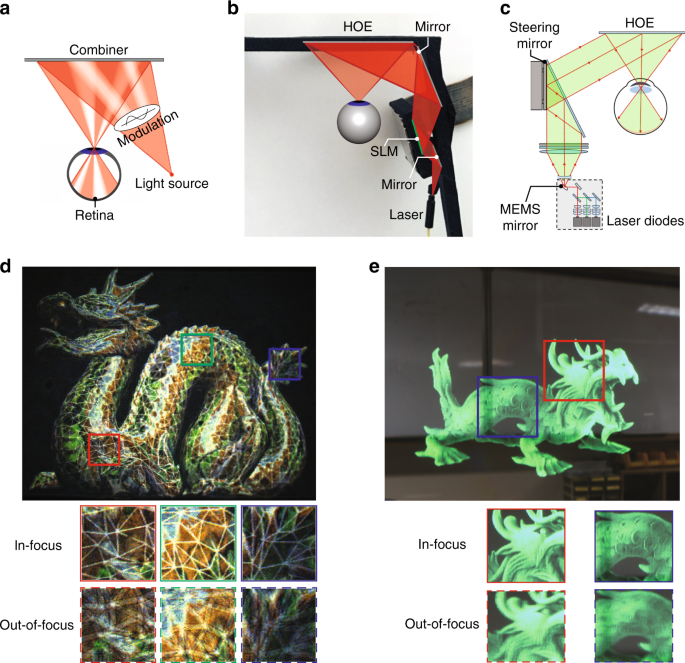
a Schematic of the working principle of Maxwellian displays. Maxwellian displays based on b SLM and laser diode light source and c MEMS-LBS with a steering mirror as additional modulation method. Generation of depth cues by d computational digital holography and e scanning of steering mirror to produce multiple views. Adapted from b, d ref. 143 and c, e ref. 167 under the Creative Commons Attribution 4.0 License
To modulate the light, a SLM like LCoS or DMD can be placed in the light path, as shown in Fig. 9b . Alternatively, LBS system can also be used (Fig. 9c ), where the intensity modulation occurs in the laser diode itself. Besides the operation in a normal Maxwellian-view, both implementations offer additional degrees of freedom for light modulation.
For a SLM-based system, there are several options to arrange the SLM pixels 143 , 161 . Maimone et al. 143 demonstrated a Maxwellian AR display with two modes to offer a large-DoF Maxwellian-view, or a holographic view (Fig. 9d ), which is often referred as computer-generated holography (CGH) 162 . To show an always-in-focus image with a large DoF, the image can be directly displayed on an amplitude SLM, or using amplitude encoding for a phase-only SLM 163 . Alternatively, if a 3D scene with correct depth cues is to be presented, then optimization algorithms for CGH can be used to generate a hologram for the SLM. The generated holographic image exhibits the natural focus-and-blur effect like a real 3D object (Fig. 9d ). To better understand this feature, we need to again exploit the concept of etendue. The laser light source can be considered to have a very small etendue due to its excellent collimation. Therefore, the system etendue is provided by the SLM. The micron-sized pixel-pitch of SLM offers a certain maximum diffraction angle, which, multiplied by the SLM size, equals system etendue. By varying the display content on SLM, the final exit pupil size can be changed accordingly. In the case of a large-DoF Maxwellian view, the exit pupil size is small, accompanied by a large FoV. For the holographic display mode, the reduced DoF requires a larger exit pupil with dimension close to the eye pupil. But the FoV is reduced accordingly due to etendue conservation. Another commonly concerned issue with CGH is the computation time. To achieve a real-time CGH rendering flow with an excellent image quality is quite a challenge. Fortunately, with recent advances in algorithm 164 and the introduction of convolutional neural network (CNN) 165 , 166 , this issue is gradually solved with an encouraging pace. Lately, Liang et al. 166 demonstrated a real-time CGH synthesis pipeline with a high image quality. The pipeline comprises an efficient CNN model to generate a complex hologram from a 3D scene and an improved encoding algorithm to convert the complex hologram to a phase-only one. An impressive frame rate of 60 Hz has been achieved on a desktop computing unit.
For LBS-based system, the additional modulation can be achieved by integrating a steering module, as demonstrated by Jang et al. 167 . The steering mirror can shift the focal point (viewpoint) within the eye pupil, therefore effectively expanding the system etendue. When the steering process is fast and the image content is updated simultaneously, correct 3D cues can be generated, as shown in Fig. 9e . However, there exists a tradeoff between the number of viewpoint and the final image frame rate, because the total frames are equally divided into each viewpoint. To boost the frame rate of MEMS-LBS systems by the number of views (e.g., 3 by 3) may be challenging.
Maxwellian-type systems offer several advantages. The system efficiency is usually very high because nearly all the light is delivered into viewer’s eye. The system FoV is determined by the f /# of combiner and a large FoV (~80° in horizontal) can be achieved 143 . The issue of VAC can be mitigated with an infinite-DoF image that deprives accommodation cue, or completely solved by generating a true-3D scene as discussed above. Despite these advantages, one major weakness of Maxwellian-type system is the tiny exit pupil, or eyebox. A small deviation of eye pupil location from the viewpoint results in the complete disappearance of the image. Therefore, to expand eyebox is considered as one of the most important challenges in Maxwellian-type systems.
Pupil duplication and steering
Methods to expand eyebox can be generally categorized into pupil duplication 168 , 169 , 170 , 171 , 172 and pupil steering 9 , 13 , 167 , 173 . Pupil duplication simply generates multiple viewpoints to cover a large area. In contrast, pupil steering dynamically shifts the viewpoint position, depending on the pupil location. Before reviewing detailed implementations of these two methods, it is worth discussing some of their general features. The multiple viewpoints in pupil duplication usually mean to equally divide the total light intensity. In each time frame, however, it is preferable that only one viewpoint enters the user’s eye pupil to avoid ghost image. This requirement, therefore, results in a reduced total light efficiency, while also conditioning the viewpoint separation to be larger than the pupil diameter. In addition, the separation should not be too large to avoid gap between viewpoints. Considering that human pupil diameter changes in response to environment illuminance, the design of viewpoint separation needs special attention. Pupil steering, on the other hand, only produces one viewpoint at each time frame. It is therefore more light-efficient and free from ghost images. But to determine the viewpoint position requires the information of eye pupil location, which demands a real-time eye-tracking module 9 . Another observation is that pupil steering can accommodate multiple viewpoints by its nature. Therefore, a pupil steering system can often be easily converted to a pupil duplication system by simultaneously generating available viewpoints.
To generate multiple viewpoints, one can focus on modulating the incident light or the combiner. Recall that viewpoint is the image of light source. To duplicate or shift light source can achieve pupil duplication or steering accordingly, as illustrated in Fig. 10a . Several schemes of light modulation are depicted in Fig. 10b–e . An array of light sources can be generated with multiple laser diodes (Fig. 10b ). To turn on all or one of the sources achieves pupil duplication or steering. A light source array can also be produced by projecting light on an array-type PPHOE 168 (Fig. 10c ). Apart from direct adjustment of light sources, modulating light on the path can also effectively steer/duplicate the light sources. Using a mechanical steering mirror, the beam can be deflected 167 (Fig. 10d ), which equals to shifting the light source position. Other devices like a grating or beam splitter can also serve as ray deflector/splitter 170 , 171 (Fig. 10e ).
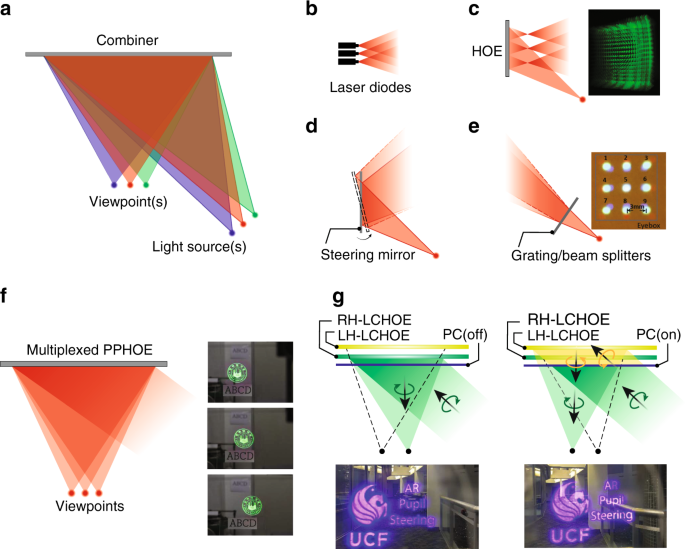
a Schematic of duplicating (or shift) viewpoint by modulation of incident light. Light modulation by b multiple laser diodes, c HOE lens array, d steering mirror and e grating or beam splitters. f Pupil duplication with multiplexed PPHOE. g Pupil steering with LCHOE. Reproduced from c ref. 168 under the Creative Commons Attribution 4.0 License, e ref. 169 with permission from OSA Publishing, f ref. 171 with permission from OSA Publishing and g ref. 173 with permission from OSA Publishing
Nonetheless, one problem of the light source duplication/shifting methods for pupil duplication/steering is that the aberrations in peripheral viewpoints are often serious 168 , 173 . The HOE combiner is usually recorded at one incident angle. For other incident angles with large deviations, considerable aberrations will occur, especially in the scenario of off-axis configuration. To solve this problem, the modulation can be focused on the combiner instead. While the mechanical shifting of combiner 9 can achieve continuous pupil steering, its integration into AR display with a small factor remains a challenge. Alternatively, the versatile functions of HOE offer possible solutions for combiner modulation. Kim and Park 169 demonstrated a pupil duplication system with multiplexed PPHOE (Fig. 10f ). Wavefronts of several viewpoints can be recorded into one PPHOE sample. Three viewpoints with a separation of 3 mm were achieved. However, a slight degree of ghost image and gap can be observed in the viewpoint transition. For a PPHOE to achieve pupil steering, the multiplexed PPHOE needs to record different focal points with different incident angles. If each hologram has no angular crosstalk, then with an additional device to change the light incident angle, the viewpoint can be steered. Alternatively, Xiong et al. 173 demonstrated a pupil steering system with LCHOEs in a simpler configuration (Fig. 10g ). The polarization-sensitive nature of LCHOE enables the controlling of which LCHOE to function with a polarization converter (PC). When the PC is off, the incident RCP light is focused by the right-handed LCHOE. When the PC is turned on, the RCP light is firstly converted to LCP light and passes through the right-handed LCHOE. Then it is focused by the left-handed LCHOE into another viewpoint. To add more viewpoints requires stacking more pairs of PC and LCHOE, which can be achieved in a compact manner with thin glass substrates. In addition, to realize pupil duplication only requires the stacking of multiple low-efficiency LCHOEs. For both PPHOEs and LCHOEs, because the hologram for each viewpoint is recorded independently, the aberrations can be eliminated.
Regarding the system performance, in theory the FoV is not limited and can reach a large value, such as 80° in horizontal direction 143 . The definition of eyebox is different from traditional imaging systems. For a single viewpoint, it has the same size as the eye pupil diameter. But due to the viewpoint steering/duplication capability, the total system eyebox can be expanded accordingly. The combiner efficiency for pupil steering systems can reach 47,000 nit/lm for a FoV of 80° by 80° and pupil diameter of 4 mm (Eq. S2 ). At such a high brightness level, eye safety could be a concern 174 . For a pupil duplication system, the combiner efficiency is decreased by the number of viewpoints. With a 4-by-4 viewpoint array, it can still reach 3000 nit/lm. Despite the potential gain of pupil duplication/steering, when considering the rotation of eyeball, the situation becomes much more complicated 175 . A perfect pupil steering system requires a 5D steering, which proposes a challenge for practical implementation.
Pin-light systems
Recently, another type of display in close relation with Maxwellian view called pin-light display 148 , 176 has been proposed. The general working principle of pin-light display is illustrated in Fig. 11a . Each pin-light source is a Maxwellian view with a large DoF. When the eye pupil is no longer placed near the source point as in Maxwellian view, each image source can only form an elemental view with a small FoV on retina. However, if the image source array is arranged in a proper form, the elemental views can be integrated together to form a large FoV. According to the specific optical architectures, pin-light display can take different forms of implementation. In the initial feasibility demonstration, Maimone et al. 176 used a side-lit waveguide plate as the point light source (Fig. 11b ). The light inside the waveguide plate is extracted by the etched divots, forming a pin-light source array. A transmissive SLM (LCD) is placed behind the waveguide plate to modulate the light intensity and form the image. The display has an impressive FoV of 110° thanks to the large scattering angle range. However, the direct placement of LCD before the eye brings issues of insufficient resolution density and diffraction of background light.
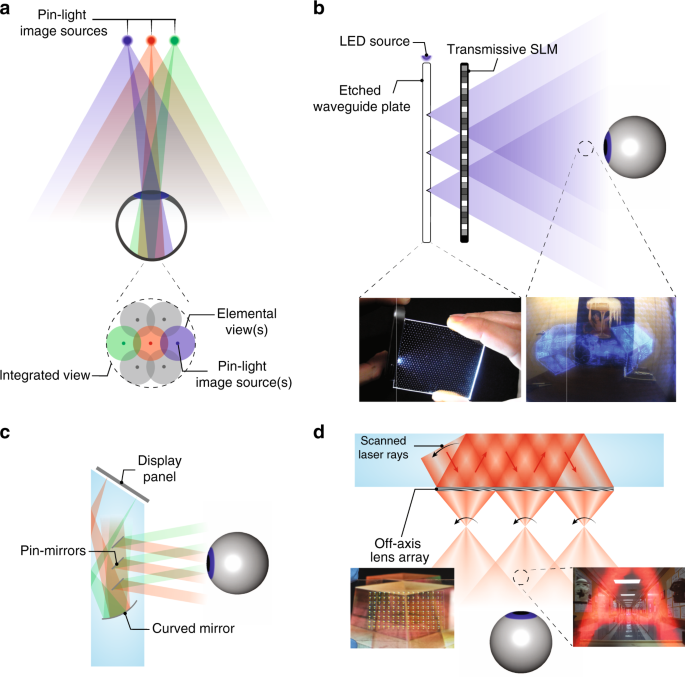
a Schematic drawing of the working principle of pin-light display. b Pin-light display utilizing a pin-light source and a transmissive SLM. c An example of pin-mirror display with a birdbath optics. d SWD system with LBS image source and off-axis lens array. Reprinted from b ref. 176 under the Creative Commons Attribution 4.0 License and d ref. 180 with permission from OSA Publishing
To avoid these issues, architectures using pin-mirrors 177 , 178 , 179 are proposed. In these systems, the final combiner is an array of tiny mirrors 178 , 179 or gratings 177 , in contrast to their counterparts using large-area combiners. An exemplary system with birdbath design is depicted in Fig. 11c . In this case, the pin-mirrors replace the original beam-splitter in the birdbath and can thus shrink the system volume, while at the same time providing large DoF pin-light images. Nonetheless, such a system may still face the etendue conservation issue. Meanwhile, the size of pin-mirror cannot be too small in order to prevent degradation of resolution density due to diffraction. Therefore, its influence on the see-through background should also be considered in the system design.
To overcome the etendue conservation and improve see-through quality, Xiong et al. 180 proposed another type of pin-light system exploiting the etendue expansion property of waveguide, which is also referred as scanning waveguide display (SWD). As illustrated in Fig. 11d , the system uses an LBS as the image source. The collimated scanned laser rays are trapped in the waveguide and encounter an array of off-axis lenses. Upon each encounter, the lens out-couples the laser rays and forms a pin-light source. SWD has the merits of good see-through quality and large etendue. A large FoV of 100° was demonstrated with the help of an ultra-low f /# lens array based on LCHOE. However, some issues like insufficient image resolution density and image non-uniformity remain to be overcome. To further improve the system may require optimization of Gaussian beam profile and additional EPE module 180 .
Overall, pin-light systems inherit the large DoF from Maxwellian view. With adequate number of pin-light sources, the FoV and eyebox can be expanded accordingly. Nonetheless, despite different forms of implementation, a common issue of pin-light system is the image uniformity. The overlapped region of elemental views has a higher light intensity than the non-overlapped region, which becomes even more complicated considering the dynamic change of pupil size. In theory, the displayed image can be pre-processed to compensate for the optical non-uniformity. But that would require knowledge of precise pupil location (and possibly size) and therefore an accurate eye-tracking module 176 . Regarding the system performance, pin-mirror systems modified from other free-space systems generally shares similar FoV and eyebox with original systems. The combiner efficiency may be lower due to the small size of pin-mirrors. SWD, on the other hand, shares the large FoV and DoF with Maxwellian view, and large eyebox with waveguide combiners. The combiner efficiency may also be lower due to the EPE process.
Waveguide combiner
Besides free-space combiners, another common architecture in AR displays is waveguide combiner. The term ‘waveguide’ indicates the light is trapped in a substrate by the TIR process. One distinctive feature of a waveguide combiner is the EPE process that effectively enlarges the system etendue. In the EPE process, a portion of the trapped light is repeatedly coupled out of the waveguide in each TIR. The effective eyebox is therefore enlarged. According to the features of couplers, we divide the waveguide combiners into two types: diffractive and achromatic, as described in the followings.
Diffractive waveguides
As the name implies, diffractive-type waveguides use diffractive elements as couplers. The in-coupler is usually a diffractive grating and the out-coupler in most cases is also a grating with the same period as the in-coupler, but it can also be an off-axis lens with a small curvature to generate image with finite depth. Three major diffractive couplers have been developed: SRGs, photopolymer gratings (PPGs), and liquid crystal gratings (grating-type LCHOE; also known as polarization volume gratings (PVGs)). Some general protocols for coupler design are that the in-coupler should have a relatively high efficiency and the out-coupler should have a uniform light output. A uniform light output usually requires a low-efficiency coupler, with extra degrees of freedom for local modulation of coupling efficiency. Both in-coupler and out-coupler should have an adequate angular bandwidth to accommodate a reasonable FoV. In addition, the out-coupler should also be optimized to avoid undesired diffractions, including the outward diffraction of TIR light and diffraction of environment light into user’s eyes, which are referred as light leakage and rainbow. Suppression of these unwanted diffractions should also be considered in the optimization process of waveguide design, along with performance parameters like efficiency and uniformity.
The basic working principles of diffractive waveguide-based AR systems are illustrated in Fig. 12 . For the SRG-based waveguides 6 , 8 (Fig. 12a ), the in-coupler can be a transmissive-type or a reflective-type 181 , 182 . The grating geometry can be optimized for coupling efficiency with a large degree of freedom 183 . For the out-coupler, a reflective SRG with a large slant angle to suppress the transmission orders is preferred 184 . In addition, a uniform light output usually requires a gradient efficiency distribution in order to compensate for the decreased light intensity in the out-coupling process. This can be achieved by varying the local grating configurations like height and duty cycle 6 . For the PPG-based waveguides 185 (Fig. 12b ), the small angular bandwidth of a high-efficiency transmissive PPG prohibits its use as in-coupler. Therefore, both in-coupler and out-coupler are usually reflective types. The gradient efficiency can be achieved by space-variant exposure to control the local index modulation 186 or local Bragg slant angle variation through freeform exposure 19 . Due to the relatively small angular bandwidth of PPG, to achieve a decent FoV usually requires stacking two 187 or three 188 PPGs together for a single color. The PVG-based waveguides 189 (Fig. 12c ) also prefer reflective PVGs as in-couplers because the transmissive PVGs are much more difficult to fabricate due to the LC alignment issue. In addition, the angular bandwidth of transmissive PVGs in Bragg regime is also not large enough to support a decent FoV 29 . For the out-coupler, the angular bandwidth of a single reflective PVG can usually support a reasonable FoV. To obtain a uniform light output, a polarization management layer 190 consisting of a LC layer with spatially variant orientations can be utilized. It offers an additional degree of freedom to control the polarization state of the TIR light. The diffraction efficiency can therefore be locally controlled due to the strong polarization sensitivity of PVG.
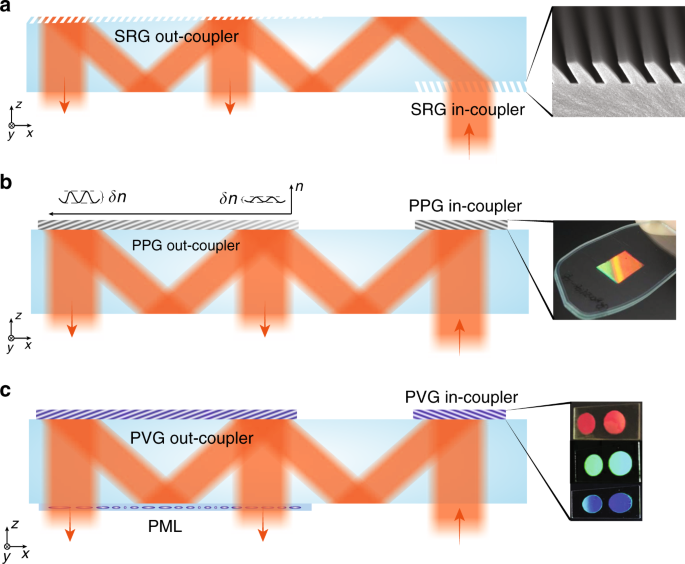
Schematics of waveguide combiners based on a SRGs, b PPGs and c PVGs. Reprinted from a ref. 85 with permission from OSA Publishing, b ref. 185 with permission from John Wiley and Sons and c ref. 189 with permission from OSA Publishing
The above discussion describes the basic working principle of 1D EPE. Nonetheless, for the 1D EPE to produce a large eyebox, the exit pupil in the unexpanded direction of the original image should be large. This proposes design challenges in light engines. Therefore, a 2D EPE is favored for practical applications. To extend EPE in two dimensions, two consecutive 1D EPEs can be used 191 , as depicted in Fig. 13a . The first 1D EPE occurs in the turning grating, where the light is duplicated in y direction and then turned into x direction. Then the light rays encounter the out-coupler and are expanded in x direction. To better understand the 2D EPE process, the k -vector diagram (Fig. 13b ) can be used. For the light propagating in air with wavenumber k 0 , its possible k -values in x and y directions ( k x and k y ) fall within the circle with radius k 0 . When the light is trapped into TIR, k x and k y are outside the circle with radius k 0 and inside the circle with radius nk 0 , where n is the refractive index of the substrate. k x and k y stay unchanged in the TIR process and are only changed in each diffraction process. The central red box in Fig. 13b indicates the possible k values within the system FoV. After the in-coupler, the k values are added by the grating k -vector, shifting the k values into TIR region. The turning grating then applies another k -vector and shifts the k values to near x -axis. Finally, the k values are shifted by the out-coupler and return to the free propagation region in air. One observation is that the size of red box is mostly limited by the width of TIR band. To accommodate a larger FoV, the outer boundary of TIR band needs to be expanded, which amounts to increasing waveguide refractive index. Another important fact is that when k x and k y are near the outer boundary, the uniformity of output light becomes worse. This is because the light propagation angle is near 90° in the waveguide. The spatial distance between two consecutive TIRs becomes so large that the out-coupled beams are spatially separated to an unacceptable degree. The range of possible k values for practical applications is therefore further shrunk due to this fact.
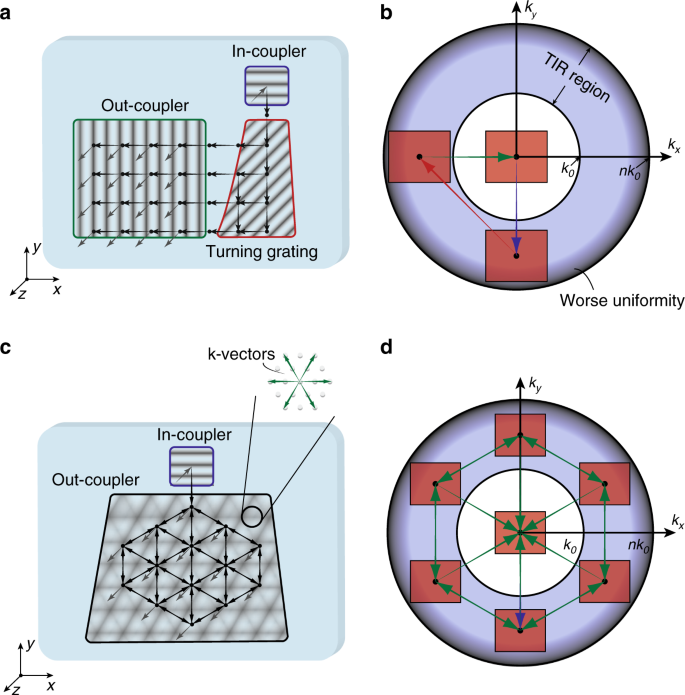
a Schematic of 2D EPE based on two consecutive 1D EPEs. Gray/black arrows indicate light in air/TIR. Black dots denote TIRs. b k-diagram of the two-1D-EPE scheme. c Schematic of 2D EPE with a 2D hexagonal grating d k-diagram of the 2D-grating scheme
Aside from two consecutive 1D EPEs, the 2D EPE can also be directly implemented with a 2D grating 192 . An example using a hexagonal grating is depicted in Fig. 13c . The hexagonal grating can provide k -vectors in six directions. In the k -diagram (Fig. 13d ), after the in-coupling, the k values are distributed into six regions due to multiple diffractions. The out-coupling occurs simultaneously with pupil expansion. Besides a concise out-coupler configuration, the 2D EPE scheme offers more degrees of design freedom than two 1D EPEs because the local grating parameters can be adjusted in a 2D manner. The higher design freedom has the potential to reach a better output light uniformity, but at the cost of a higher computation demand for optimization. Furthermore, the unslanted grating geometry usually leads to a large light leakage and possibly low efficiency. Adding slant to the geometry helps alleviate the issue, but the associated fabrication may be more challenging.
Finally, we discuss the generation of full-color images. One important issue to clarify is that although diffractive gratings are used here, the final image generally has no color dispersion even if we use a broadband light source like LED. This can be easily understood in the 1D EPE scheme. The in-coupler and out-coupler have opposite k -vectors, which cancels the color dispersion for each other. In the 2D EPE schemes, the k -vectors always form a closed loop from in-coupled light to out-coupled light, thus, the color dispersion also vanishes likewise. The issue of using a single waveguide for full-color images actually exists in the consideration of FoV and light uniformity. The breakup of propagation angles for different colors results in varied out-coupling situations for each color. To be more specific, if the red and the blue channels use the same in-coupler, the propagating angle for the red light is larger than that of the blue light. The red light in peripheral FoV is therefore easier to face the mentioned large-angle non-uniformity issue. To acquire a decent FoV and light uniformity, usually two or three layers of waveguides with different grating pitches are adopted.
Regarding the system performance, the eyebox is generally large enough (~10 mm) to accommodate different user’s IPD and alignment shift during operation. A parameter of significant concern for a waveguide combiner is its FoV. From the k -vector analysis, we can conclude the theoretical upper limit is determined by the waveguide refractive index. But the light/color uniformity also influences the effective FoV, over which the degradation of image quality becomes unacceptable. Current diffractive waveguide combiners generally achieve a FoV of about 50°. To further increase FoV, a straightforward method is to use a higher refractive index waveguide. Another is to tile FoV through direct stacking of multiple waveguides or using polarization-sensitive couplers 79 , 193 . As to the optical efficiency, a typical value for the diffractive waveguide combiner is around 50–200 nit/lm 6 , 189 . In addition, waveguide combiners adopting grating out-couplers generate an image with fixed depth at infinity. This leads to the VAC issue. To tackle VAC in waveguide architectures, the most practical way is to generate multiple depths and use the varifocal or multifocal driving scheme, similar to those mentioned in the VR systems. But to add more depths usually means to stack multiple layers of waveguides together 194 . Considering the additional waveguide layers for RGB colors, the final waveguide thickness would undoubtedly increase.
Other parameters special to waveguide includes light leakage, see-through ghost, and rainbow. Light leakage refers to out-coupled light that goes outwards to the environment, as depicted in Fig. 14a . Aside from decreased efficiency, the leakage also brings drawback of unnatural “bright-eye” appearance of the user and privacy issue. Optimization of the grating structure like geometry of SRG may reduce the leakage. See-through ghost is formed by consecutive in-coupling and out-couplings caused by the out-coupler grating, as sketched in Fig. 14b , After the process, a real object with finite depth may produce a ghost image with shift in both FoV and depth. Generally, an out-coupler with higher efficiency suffers more see-through ghost. Rainbow is caused by the diffraction of environment light into user’s eye, as sketched in Fig. 14c . The color dispersion in this case will occur because there is no cancellation of k -vector. Using the k -diagram, we can obtain a deeper insight into the formation of rainbow. Here, we take the EPE structure in Fig. 13a as an example. As depicted in Fig. 14d , after diffractions by the turning grating and the out-coupler grating, the k values are distributed in two circles that shift from the origin by the grating k -vectors. Some diffracted light can enter the see-through FoV and form rainbow. To reduce rainbow, a straightforward way is to use a higher index substrate. With a higher refractive index, the outer boundary of k diagram is expanded, which can accommodate larger grating k -vectors. The enlarged k -vectors would therefore “push” these two circles outwards, leading to a decreased overlapping region with the see-through FoV. Alternatively, an optimized grating structure would also help reduce the rainbow effect by suppressing the unwanted diffraction.
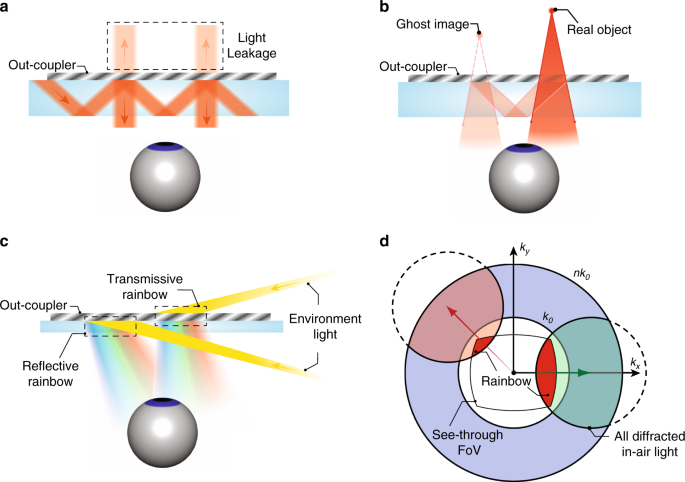
Sketches of formations of a light leakage, b see-through ghost and c rainbow. d Analysis of rainbow formation with k-diagram
Achromatic waveguide
Achromatic waveguide combiners use achromatic elements as couplers. It has the advantage of realizing full-color image with a single waveguide. A typical example of achromatic element is a mirror. The waveguide with partial mirrors as out-coupler is often referred as geometric waveguide 6 , 195 , as depicted in Fig. 15a . The in-coupler in this case is usually a prism to avoid unnecessary color dispersion if using diffractive elements otherwise. The mirrors couple out TIR light consecutively to produce a large eyebox, similarly in a diffractive waveguide. Thanks to the excellent optical property of mirrors, the geometric waveguide usually exhibits a superior image regarding MTF and color uniformity to its diffractive counterparts. Still, the spatially discontinuous configuration of mirrors also results in gaps in eyebox, which may be alleviated by using a dual-layer structure 196 . Wang et al. designed a geometric waveguide display with five partial mirrors (Fig. 15b ). It exhibits a remarkable FoV of 50° by 30° (Fig. 15c ) and an exit pupil of 4 mm with a 1D EPE. To achieve 2D EPE, similar architectures in Fig. 13a can be used by integrating a turning mirror array as the first 1D EPE module 197 . Unfortunately, the k -vector diagrams in Fig. 13b, d cannot be used here because the k values in x-y plane no longer conserve in the in-coupling and out-coupling processes. But some general conclusions remain valid, like a higher refractive index leading to a larger FoV and gradient out-coupling efficiency improving light uniformity.
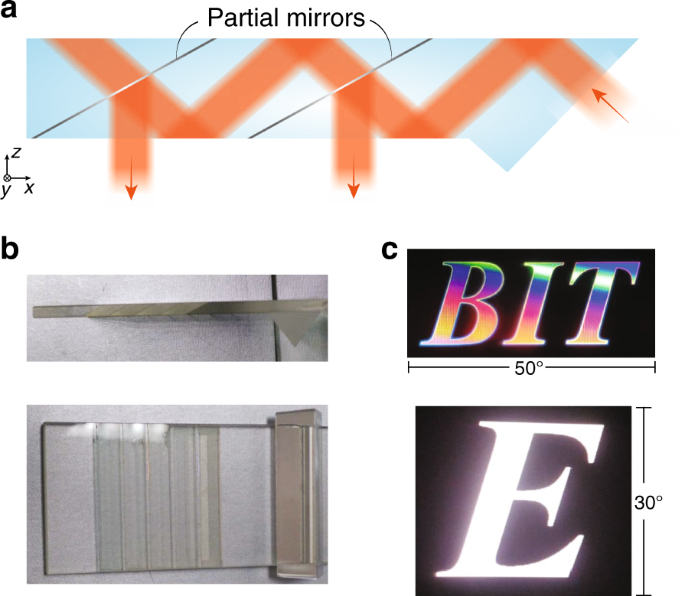
a Schematic of the system configuration. b Geometric waveguide with five partial mirrors. c Image photos demonstrating system FoV. Adapted from b , c ref. 195 with permission from OSA Publishing
The fabrication process of geometric waveguide involves coating mirrors on cut-apart pieces and integrating them back together, which may result in a high cost, especially for the 2D EPE architecture. Another way to implement an achromatic coupler is to use multiplexed PPHOE 198 , 199 to mimic the behavior of a tilted mirror (Fig. 16a ). To understand the working principle, we can use the diagram in Fig. 16b . The law of reflection states the angle of reflection equals to the angle of incidence. If we translate this behavior to k -vector language, it means the mirror can apply any length of k -vector along its surface normal direction. The k -vector length of the reflected light is always equal to that of the incident light. This puts a condition that the k -vector triangle is isosceles. With a simple geometric deduction, it can be easily observed this leads to the law of reflection. The behavior of a general grating, however, is very different. For simplicity we only consider the main diffraction order. The grating can only apply a k -vector with fixed k x due to the basic diffraction law. For the light with a different incident angle, it needs to apply different k z to produce a diffracted light with equal k -vector length as the incident light. For a grating with a broad angular bandwidth like SRG, the range of k z is wide, forming a lengthy vertical line in Fig. 16b . For a PPG with a narrow angular bandwidth, the line is short and resembles a dot. If multiple of these tiny dots are distributed along the oblique line corresponding to a mirror, then the final multiplexed PPGs can imitate the behavior of a tilted mirror. Such a PPHOE is sometimes referred as a skew-mirror 198 . In theory, to better imitate the mirror, a lot of multiplexed PPGs is preferred, while each PPG has a small index modulation δn . But this proposes a bigger challenge in device fabrication. Recently, Utsugi et al. demonstrated an impressive skew-mirror waveguide based on 54 multiplexed PPGs (Fig. 16c, d ). The display exhibits an effective FoV of 35° by 36°. In the peripheral FoV, there still exists some non-uniformity (Fig. 16e ) due to the out-coupling gap, which is an inherent feature of the flat-type out-couplers.
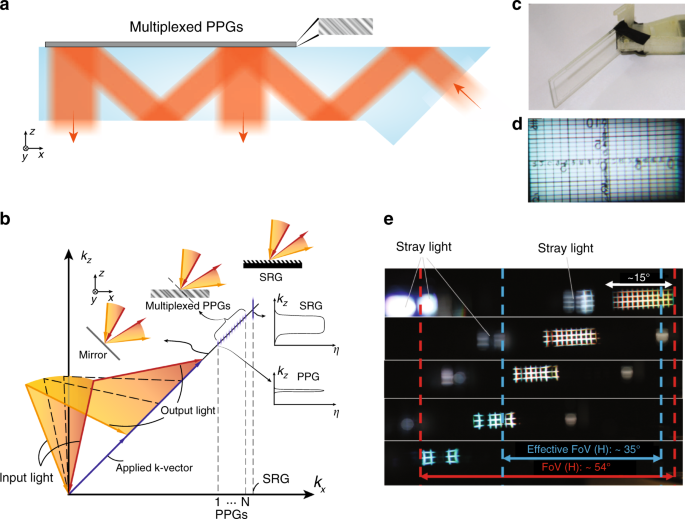
a System configuration. b Diagram demonstrating how multiplexed PPGs resemble the behavior of a mirror. Photos showing c the system and d image. e Picture demonstrating effective system FoV. Adapted from c – e ref. 199 with permission from ITE
Finally, it is worth mentioning that metasurfaces are also promising to deliver achromatic gratings 200 , 201 for waveguide couplers ascribed to their versatile wavefront shaping capability. The mechanism of the achromatic gratings is similar to that of the achromatic lenses as previously discussed. However, the current development of achromatic metagratings is still in its infancy. Much effort is needed to improve the optical efficiency for in-coupling, control the higher diffraction orders for eliminating ghost images, and enable a large size design for EPE.
Generally, achromatic waveguide combiners exhibit a comparable FoV and eyebox with diffractive combiners, but with a higher efficiency. For a partial-mirror combiner, its combiner efficiency is around 650 nit/lm 197 (2D EPE). For a skew-mirror combiner, although the efficiency of multiplexed PPHOE is relatively low (~1.5%) 199 , the final combiner efficiency of the 1D EPE system is still high (>3000 nit/lm) due to multiple out-couplings.
Table 2 summarizes the performance of different AR combiners. When combing the luminous efficacy in Table 1 and the combiner efficiency in Table 2 , we can have a comprehensive estimate of the total luminance efficiency (nit/W) for different types of systems. Generally, Maxwellian-type combiners with pupil steering have the highest luminance efficiency when partnered with laser-based light engines like laser-backlit LCoS/DMD or MEM-LBS. Geometric optical combiners have well-balanced image performances, but to further shrink the system size remains a challenge. Diffractive waveguides have a relatively low combiner efficiency, which can be remedied by an efficient light engine like MEMS-LBS. Further development of coupler and EPE scheme would also improve the system efficiency and FoV. Achromatic waveguides have a decent combiner efficiency. The single-layer design also enables a smaller form factor. With advances in fabrication process, it may become a strong contender to presently widely used diffractive waveguides.
Conclusions and perspectives
VR and AR are endowed with a high expectation to revolutionize the way we interact with digital world. Accompanied with the expectation are the engineering challenges to squeeze a high-performance display system into a tightly packed module for daily wearing. Although the etendue conservation constitutes a great obstacle on the path, remarkable progresses with innovative optics and photonics continue to take place. Ultra-thin optical elements like PPHOEs and LCHOEs provide alternative solutions to traditional optics. Their unique features of multiplexing capability and polarization dependency further expand the possibility of novel wavefront modulations. At the same time, nanoscale-engineered metasurfaces/SRGs provide large design freedoms to achieve novel functions beyond conventional geometric optical devices. Newly emerged micro-LEDs open an opportunity for compact microdisplays with high peak brightness and good stability. Further advances on device engineering and manufacturing process are expected to boost the performance of metasurfaces/SRGs and micro-LEDs for AR and VR applications.
Data availability
All data needed to evaluate the conclusions in the paper are present in the paper. Additional data related to this paper may be requested from the authors.
Cakmakci, O. & Rolland, J. Head-worn displays: a review. J. Disp. Technol. 2 , 199–216 (2006).
Article ADS Google Scholar
Zhan, T. et al. Augmented reality and virtual reality displays: perspectives and challenges. iScience 23 , 101397 (2020).
Rendon, A. A. et al. The effect of virtual reality gaming on dynamic balance in older adults. Age Ageing 41 , 549–552 (2012).
Article Google Scholar
Choi, S., Jung, K. & Noh, S. D. Virtual reality applications in manufacturing industries: past research, present findings, and future directions. Concurrent Eng. 23 , 40–63 (2015).
Li, X. et al. A critical review of virtual and augmented reality (VR/AR) applications in construction safety. Autom. Constr. 86 , 150–162 (2018).
Kress, B. C. Optical Architectures for Augmented-, Virtual-, and Mixed-Reality Headsets (Bellingham: SPIE Press, 2020).
Cholewiak, S. A. et al. A perceptual eyebox for near-eye displays. Opt. Express 28 , 38008–38028 (2020).
Lee, Y. H., Zhan, T. & Wu, S. T. Prospects and challenges in augmented reality displays. Virtual Real. Intell. Hardw. 1 , 10–20 (2019).
Kim, J. et al. Foveated AR: dynamically-foveated augmented reality display. ACM Trans. Graph. 38 , 99 (2019).
Tan, G. J. et al. Foveated imaging for near-eye displays. Opt. Express 26 , 25076–25085 (2018).
Lee, S. et al. Foveated near-eye display for mixed reality using liquid crystal photonics. Sci. Rep. 10 , 16127 (2020).
Yoo, C. et al. Foveated display system based on a doublet geometric phase lens. Opt. Express 28 , 23690–23702 (2020).
Akşit, K. et al. Manufacturing application-driven foveated near-eye displays. IEEE Trans. Vis. Computer Graph. 25 , 1928–1939 (2019).
Zhu, R. D. et al. High-ambient-contrast augmented reality with a tunable transmittance liquid crystal film and a functional reflective polarizer. J. Soc. Inf. Disp. 24 , 229–233 (2016).
Lincoln, P. et al. Scene-adaptive high dynamic range display for low latency augmented reality. In Proc. 21st ACM SIGGRAPH Symposium on Interactive 3D Graphics and Games . (ACM, San Francisco, CA, 2017).
Duerr, F. & Thienpont, H. Freeform imaging systems: fermat’s principle unlocks “first time right” design. Light.: Sci. Appl. 10 , 95 (2021).
Bauer, A., Schiesser, E. M. & Rolland, J. P. Starting geometry creation and design method for freeform optics. Nat. Commun. 9 , 1756 (2018).
Rolland, J. P. et al. Freeform optics for imaging. Optica 8 , 161–176 (2021).
Jang, C. et al. Design and fabrication of freeform holographic optical elements. ACM Trans. Graph. 39 , 184 (2020).
Gabor, D. A new microscopic principle. Nature 161 , 777–778 (1948).
Kostuk, R. K. Holography: Principles and Applications (Boca Raton: CRC Press, 2019).
Lawrence, J. R., O'Neill, F. T. & Sheridan, J. T. Photopolymer holographic recording material. Optik 112 , 449–463 (2001).
Guo, J. X., Gleeson, M. R. & Sheridan, J. T. A review of the optimisation of photopolymer materials for holographic data storage. Phys. Res. Int. 2012 , 803439 (2012).
Jang, C. et al. Recent progress in see-through three-dimensional displays using holographic optical elements [Invited]. Appl. Opt. 55 , A71–A85 (2016).
Xiong, J. H. et al. Holographic optical elements for augmented reality: principles, present status, and future perspectives. Adv. Photonics Res. 2 , 2000049 (2021).
Tabiryan, N. V. et al. Advances in transparent planar optics: enabling large aperture, ultrathin lenses. Adv. Optical Mater. 9 , 2001692 (2021).
Zanutta, A. et al. Photopolymeric films with highly tunable refractive index modulation for high precision diffractive optics. Optical Mater. Express 6 , 252–263 (2016).
Moharam, M. G. & Gaylord, T. K. Rigorous coupled-wave analysis of planar-grating diffraction. J. Optical Soc. Am. 71 , 811–818 (1981).
Xiong, J. H. & Wu, S. T. Rigorous coupled-wave analysis of liquid crystal polarization gratings. Opt. Express 28 , 35960–35971 (2020).
Xie, S., Natansohn, A. & Rochon, P. Recent developments in aromatic azo polymers research. Chem. Mater. 5 , 403–411 (1993).
Shishido, A. Rewritable holograms based on azobenzene-containing liquid-crystalline polymers. Polym. J. 42 , 525–533 (2010).
Bunning, T. J. et al. Holographic polymer-dispersed liquid crystals (H-PDLCs). Annu. Rev. Mater. Sci. 30 , 83–115 (2000).
Liu, Y. J. & Sun, X. W. Holographic polymer-dispersed liquid crystals: materials, formation, and applications. Adv. Optoelectron. 2008 , 684349 (2008).
Xiong, J. H. & Wu, S. T. Planar liquid crystal polarization optics for augmented reality and virtual reality: from fundamentals to applications. eLight 1 , 3 (2021).
Yaroshchuk, O. & Reznikov, Y. Photoalignment of liquid crystals: basics and current trends. J. Mater. Chem. 22 , 286–300 (2012).
Sarkissian, H. et al. Periodically aligned liquid crystal: potential application for projection displays. Mol. Cryst. Liq. Cryst. 451 , 1–19 (2006).
Komanduri, R. K. & Escuti, M. J. Elastic continuum analysis of the liquid crystal polarization grating. Phys. Rev. E 76 , 021701 (2007).
Kobashi, J., Yoshida, H. & Ozaki, M. Planar optics with patterned chiral liquid crystals. Nat. Photonics 10 , 389–392 (2016).
Lee, Y. H., Yin, K. & Wu, S. T. Reflective polarization volume gratings for high efficiency waveguide-coupling augmented reality displays. Opt. Express 25 , 27008–27014 (2017).
Lee, Y. H., He, Z. Q. & Wu, S. T. Optical properties of reflective liquid crystal polarization volume gratings. J. Optical Soc. Am. B 36 , D9–D12 (2019).
Xiong, J. H., Chen, R. & Wu, S. T. Device simulation of liquid crystal polarization gratings. Opt. Express 27 , 18102–18112 (2019).
Czapla, A. et al. Long-period fiber gratings with low-birefringence liquid crystal. Mol. Cryst. Liq. Cryst. 502 , 65–76 (2009).
Dąbrowski, R., Kula, P. & Herman, J. High birefringence liquid crystals. Crystals 3 , 443–482 (2013).
Mack, C. Fundamental Principles of Optical Lithography: The Science of Microfabrication (Chichester: John Wiley & Sons, 2007).
Genevet, P. et al. Recent advances in planar optics: from plasmonic to dielectric metasurfaces. Optica 4 , 139–152 (2017).
Guo, L. J. Nanoimprint lithography: methods and material requirements. Adv. Mater. 19 , 495–513 (2007).
Park, J. et al. Electrically driven mid-submicrometre pixelation of InGaN micro-light-emitting diode displays for augmented-reality glasses. Nat. Photonics 15 , 449–455 (2021).
Khorasaninejad, M. et al. Metalenses at visible wavelengths: diffraction-limited focusing and subwavelength resolution imaging. Science 352 , 1190–1194 (2016).
Li, S. Q. et al. Phase-only transmissive spatial light modulator based on tunable dielectric metasurface. Science 364 , 1087–1090 (2019).
Liang, K. L. et al. Advances in color-converted micro-LED arrays. Jpn. J. Appl. Phys. 60 , SA0802 (2020).
Jin, S. X. et al. GaN microdisk light emitting diodes. Appl. Phys. Lett. 76 , 631–633 (2000).
Day, J. et al. Full-scale self-emissive blue and green microdisplays based on GaN micro-LED arrays. In Proc. SPIE 8268, Quantum Sensing and Nanophotonic Devices IX (SPIE, San Francisco, California, United States, 2012).
Huang, Y. G. et al. Mini-LED, micro-LED and OLED displays: present status and future perspectives. Light.: Sci. Appl. 9 , 105 (2020).
Parbrook, P. J. et al. Micro-light emitting diode: from chips to applications. Laser Photonics Rev. 15 , 2000133 (2021).
Day, J. et al. III-Nitride full-scale high-resolution microdisplays. Appl. Phys. Lett. 99 , 031116 (2011).
Liu, Z. J. et al. 360 PPI flip-chip mounted active matrix addressable light emitting diode on silicon (LEDoS) micro-displays. J. Disp. Technol. 9 , 678–682 (2013).
Zhang, L. et al. Wafer-scale monolithic hybrid integration of Si-based IC and III–V epi-layers—A mass manufacturable approach for active matrix micro-LED micro-displays. J. Soc. Inf. Disp. 26 , 137–145 (2018).
Tian, P. F. et al. Size-dependent efficiency and efficiency droop of blue InGaN micro-light emitting diodes. Appl. Phys. Lett. 101 , 231110 (2012).
Olivier, F. et al. Shockley-Read-Hall and Auger non-radiative recombination in GaN based LEDs: a size effect study. Appl. Phys. Lett. 111 , 022104 (2017).
Konoplev, S. S., Bulashevich, K. A. & Karpov, S. Y. From large-size to micro-LEDs: scaling trends revealed by modeling. Phys. Status Solidi (A) 215 , 1700508 (2018).
Li, L. Z. et al. Transfer-printed, tandem microscale light-emitting diodes for full-color displays. Proc. Natl Acad. Sci. USA 118 , e2023436118 (2021).
Oh, J. T. et al. Light output performance of red AlGaInP-based light emitting diodes with different chip geometries and structures. Opt. Express 26 , 11194–11200 (2018).
Shen, Y. C. et al. Auger recombination in InGaN measured by photoluminescence. Appl. Phys. Lett. 91 , 141101 (2007).
Wong, M. S. et al. High efficiency of III-nitride micro-light-emitting diodes by sidewall passivation using atomic layer deposition. Opt. Express 26 , 21324–21331 (2018).
Han, S. C. et al. AlGaInP-based Micro-LED array with enhanced optoelectrical properties. Optical Mater. 114 , 110860 (2021).
Wong, M. S. et al. Size-independent peak efficiency of III-nitride micro-light-emitting-diodes using chemical treatment and sidewall passivation. Appl. Phys. Express 12 , 097004 (2019).
Ley, R. T. et al. Revealing the importance of light extraction efficiency in InGaN/GaN microLEDs via chemical treatment and dielectric passivation. Appl. Phys. Lett. 116 , 251104 (2020).
Moon, S. W. et al. Recent progress on ultrathin metalenses for flat optics. iScience 23 , 101877 (2020).
Arbabi, A. et al. Efficient dielectric metasurface collimating lenses for mid-infrared quantum cascade lasers. Opt. Express 23 , 33310–33317 (2015).
Yu, N. F. et al. Light propagation with phase discontinuities: generalized laws of reflection and refraction. Science 334 , 333–337 (2011).
Liang, H. W. et al. High performance metalenses: numerical aperture, aberrations, chromaticity, and trade-offs. Optica 6 , 1461–1470 (2019).
Park, J. S. et al. All-glass, large metalens at visible wavelength using deep-ultraviolet projection lithography. Nano Lett. 19 , 8673–8682 (2019).
Yoon, G. et al. Single-step manufacturing of hierarchical dielectric metalens in the visible. Nat. Commun. 11 , 2268 (2020).
Lee, G. Y. et al. Metasurface eyepiece for augmented reality. Nat. Commun. 9 , 4562 (2018).
Chen, W. T. et al. A broadband achromatic metalens for focusing and imaging in the visible. Nat. Nanotechnol. 13 , 220–226 (2018).
Wang, S. M. et al. A broadband achromatic metalens in the visible. Nat. Nanotechnol. 13 , 227–232 (2018).
Lan, S. F. et al. Metasurfaces for near-eye augmented reality. ACS Photonics 6 , 864–870 (2019).
Fan, Z. B. et al. A broadband achromatic metalens array for integral imaging in the visible. Light.: Sci. Appl. 8 , 67 (2019).
Shi, Z. J., Chen, W. T. & Capasso, F. Wide field-of-view waveguide displays enabled by polarization-dependent metagratings. In Proc. SPIE 10676, Digital Optics for Immersive Displays (SPIE, Strasbourg, France, 2018).
Hong, C. C., Colburn, S. & Majumdar, A. Flat metaform near-eye visor. Appl. Opt. 56 , 8822–8827 (2017).
Bayati, E. et al. Design of achromatic augmented reality visors based on composite metasurfaces. Appl. Opt. 60 , 844–850 (2021).
Nikolov, D. K. et al. Metaform optics: bridging nanophotonics and freeform optics. Sci. Adv. 7 , eabe5112 (2021).
Tamir, T. & Peng, S. T. Analysis and design of grating couplers. Appl. Phys. 14 , 235–254 (1977).
Miller, J. M. et al. Design and fabrication of binary slanted surface-relief gratings for a planar optical interconnection. Appl. Opt. 36 , 5717–5727 (1997).
Levola, T. & Laakkonen, P. Replicated slanted gratings with a high refractive index material for in and outcoupling of light. Opt. Express 15 , 2067–2074 (2007).
Shrestha, S. et al. Broadband achromatic dielectric metalenses. Light.: Sci. Appl. 7 , 85 (2018).
Li, Z. Y. et al. Meta-optics achieves RGB-achromatic focusing for virtual reality. Sci. Adv. 7 , eabe4458 (2021).
Ratcliff, J. et al. ThinVR: heterogeneous microlens arrays for compact, 180 degree FOV VR near-eye displays. IEEE Trans. Vis. Computer Graph. 26 , 1981–1990 (2020).
Wong, T. L. et al. Folded optics with birefringent reflective polarizers. In Proc. SPIE 10335, Digital Optical Technologies 2017 (SPIE, Munich, Germany, 2017).
Li, Y. N. Q. et al. Broadband cholesteric liquid crystal lens for chromatic aberration correction in catadioptric virtual reality optics. Opt. Express 29 , 6011–6020 (2021).
Bang, K. et al. Lenslet VR: thin, flat and wide-FOV virtual reality display using fresnel lens and lenslet array. IEEE Trans. Vis. Computer Graph. 27 , 2545–2554 (2021).
Maimone, A. & Wang, J. R. Holographic optics for thin and lightweight virtual reality. ACM Trans. Graph. 39 , 67 (2020).
Kramida, G. Resolving the vergence-accommodation conflict in head-mounted displays. IEEE Trans. Vis. Computer Graph. 22 , 1912–1931 (2016).
Zhan, T. et al. Multifocal displays: review and prospect. PhotoniX 1 , 10 (2020).
Shimobaba, T., Kakue, T. & Ito, T. Review of fast algorithms and hardware implementations on computer holography. IEEE Trans. Ind. Inform. 12 , 1611–1622 (2016).
Xiao, X. et al. Advances in three-dimensional integral imaging: sensing, display, and applications [Invited]. Appl. Opt. 52 , 546–560 (2013).
Kuiper, S. & Hendriks, B. H. W. Variable-focus liquid lens for miniature cameras. Appl. Phys. Lett. 85 , 1128–1130 (2004).
Liu, S. & Hua, H. Time-multiplexed dual-focal plane head-mounted display with a liquid lens. Opt. Lett. 34 , 1642–1644 (2009).
Wilson, A. & Hua, H. Design and demonstration of a vari-focal optical see-through head-mounted display using freeform Alvarez lenses. Opt. Express 27 , 15627–15637 (2019).
Zhan, T. et al. Pancharatnam-Berry optical elements for head-up and near-eye displays [Invited]. J. Optical Soc. Am. B 36 , D52–D65 (2019).
Oh, C. & Escuti, M. J. Achromatic diffraction from polarization gratings with high efficiency. Opt. Lett. 33 , 2287–2289 (2008).
Zou, J. Y. et al. Broadband wide-view Pancharatnam-Berry phase deflector. Opt. Express 28 , 4921–4927 (2020).
Zhan, T., Lee, Y. H. & Wu, S. T. High-resolution additive light field near-eye display by switchable Pancharatnam–Berry phase lenses. Opt. Express 26 , 4863–4872 (2018).
Tan, G. J. et al. Polarization-multiplexed multiplane display. Opt. Lett. 43 , 5651–5654 (2018).
Lanman, D. R. Display systems research at facebook reality labs (conference presentation). In Proc. SPIE 11310, Optical Architectures for Displays and Sensing in Augmented, Virtual, and Mixed Reality (AR, VR, MR) (SPIE, San Francisco, California, United States, 2020).
Liu, Z. J. et al. A novel BLU-free full-color LED projector using LED on silicon micro-displays. IEEE Photonics Technol. Lett. 25 , 2267–2270 (2013).
Han, H. V. et al. Resonant-enhanced full-color emission of quantum-dot-based micro LED display technology. Opt. Express 23 , 32504–32515 (2015).
Lin, H. Y. et al. Optical cross-talk reduction in a quantum-dot-based full-color micro-light-emitting-diode display by a lithographic-fabricated photoresist mold. Photonics Res. 5 , 411–416 (2017).
Liu, Z. J. et al. Micro-light-emitting diodes with quantum dots in display technology. Light.: Sci. Appl. 9 , 83 (2020).
Kim, H. M. et al. Ten micrometer pixel, quantum dots color conversion layer for high resolution and full color active matrix micro-LED display. J. Soc. Inf. Disp. 27 , 347–353 (2019).
Xuan, T. T. et al. Inkjet-printed quantum dot color conversion films for high-resolution and full-color micro light-emitting diode displays. J. Phys. Chem. Lett. 11 , 5184–5191 (2020).
Chen, S. W. H. et al. Full-color monolithic hybrid quantum dot nanoring micro light-emitting diodes with improved efficiency using atomic layer deposition and nonradiative resonant energy transfer. Photonics Res. 7 , 416–422 (2019).
Krishnan, C. et al. Hybrid photonic crystal light-emitting diode renders 123% color conversion effective quantum yield. Optica 3 , 503–509 (2016).
Kang, J. H. et al. RGB arrays for micro-light-emitting diode applications using nanoporous GaN embedded with quantum dots. ACS Applied Mater. Interfaces 12 , 30890–30895 (2020).
Chen, G. S. et al. Monolithic red/green/blue micro-LEDs with HBR and DBR structures. IEEE Photonics Technol. Lett. 30 , 262–265 (2018).
Hsiang, E. L. et al. Enhancing the efficiency of color conversion micro-LED display with a patterned cholesteric liquid crystal polymer film. Nanomaterials 10 , 2430 (2020).
Kang, C. M. et al. Hybrid full-color inorganic light-emitting diodes integrated on a single wafer using selective area growth and adhesive bonding. ACS Photonics 5 , 4413–4422 (2018).
Geum, D. M. et al. Strategy toward the fabrication of ultrahigh-resolution micro-LED displays by bonding-interface-engineered vertical stacking and surface passivation. Nanoscale 11 , 23139–23148 (2019).
Ra, Y. H. et al. Full-color single nanowire pixels for projection displays. Nano Lett. 16 , 4608–4615 (2016).
Motoyama, Y. et al. High-efficiency OLED microdisplay with microlens array. J. Soc. Inf. Disp. 27 , 354–360 (2019).
Fujii, T. et al. 4032 ppi High-resolution OLED microdisplay. J. Soc. Inf. Disp. 26 , 178–186 (2018).
Hamer, J. et al. High-performance OLED microdisplays made with multi-stack OLED formulations on CMOS backplanes. In Proc. SPIE 11473, Organic and Hybrid Light Emitting Materials and Devices XXIV . Online Only (SPIE, 2020).
Joo, W. J. et al. Metasurface-driven OLED displays beyond 10,000 pixels per inch. Science 370 , 459–463 (2020).
Vettese, D. Liquid crystal on silicon. Nat. Photonics 4 , 752–754 (2010).
Zhang, Z. C., You, Z. & Chu, D. P. Fundamentals of phase-only liquid crystal on silicon (LCOS) devices. Light.: Sci. Appl. 3 , e213 (2014).
Hornbeck, L. J. The DMD TM projection display chip: a MEMS-based technology. MRS Bull. 26 , 325–327 (2001).
Zhang, Q. et al. Polarization recycling method for light-pipe-based optical engine. Appl. Opt. 52 , 8827–8833 (2013).
Hofmann, U., Janes, J. & Quenzer, H. J. High-Q MEMS resonators for laser beam scanning displays. Micromachines 3 , 509–528 (2012).
Holmström, S. T. S., Baran, U. & Urey, H. MEMS laser scanners: a review. J. Microelectromechanical Syst. 23 , 259–275 (2014).
Bao, X. Z. et al. Design and fabrication of AlGaInP-based micro-light-emitting-diode array devices. Opt. Laser Technol. 78 , 34–41 (2016).
Olivier, F. et al. Influence of size-reduction on the performances of GaN-based micro-LEDs for display application. J. Lumin. 191 , 112–116 (2017).
Liu, Y. B. et al. High-brightness InGaN/GaN Micro-LEDs with secondary peak effect for displays. IEEE Electron Device Lett. 41 , 1380–1383 (2020).
Qi, L. H. et al. 848 ppi high-brightness active-matrix micro-LED micro-display using GaN-on-Si epi-wafers towards mass production. Opt. Express 29 , 10580–10591 (2021).
Chen, E. G. & Yu, F. H. Design of an elliptic spot illumination system in LED-based color filter-liquid-crystal-on-silicon pico projectors for mobile embedded projection. Appl. Opt. 51 , 3162–3170 (2012).
Darmon, D., McNeil, J. R. & Handschy, M. A. 70.1: LED-illuminated pico projector architectures. Soc. Inf. Disp. Int. Symp . Dig. Tech. Pap. 39 , 1070–1073 (2008).
Essaian, S. & Khaydarov, J. State of the art of compact green lasers for mobile projectors. Optical Rev. 19 , 400–404 (2012).
Sun, W. S. et al. Compact LED projector design with high uniformity and efficiency. Appl. Opt. 53 , H227–H232 (2014).
Sun, W. S., Chiang, Y. C. & Tsuei, C. H. Optical design for the DLP pocket projector using LED light source. Phys. Procedia 19 , 301–307 (2011).
Chen, S. W. H. et al. High-bandwidth green semipolar (20–21) InGaN/GaN micro light-emitting diodes for visible light communication. ACS Photonics 7 , 2228–2235 (2020).
Yoshida, K. et al. 245 MHz bandwidth organic light-emitting diodes used in a gigabit optical wireless data link. Nat. Commun. 11 , 1171 (2020).
Park, D. W. et al. 53.5: High-speed AMOLED pixel circuit and driving scheme. Soc. Inf. Disp. Int. Symp . Dig. Tech. Pap. 41 , 806–809 (2010).
Tan, L., Huang, H. C. & Kwok, H. S. 78.1: Ultra compact polarization recycling system for white light LED based pico-projection system. Soc. Inf. Disp. Int. Symp. Dig. Tech. Pap. 41 , 1159–1161 (2010).
Maimone, A., Georgiou, A. & Kollin, J. S. Holographic near-eye displays for virtual and augmented reality. ACM Trans. Graph. 36 , 85 (2017).
Pan, J. W. et al. Portable digital micromirror device projector using a prism. Appl. Opt. 46 , 5097–5102 (2007).
Huang, Y. et al. Liquid-crystal-on-silicon for augmented reality displays. Appl. Sci. 8 , 2366 (2018).
Peng, F. L. et al. Analytical equation for the motion picture response time of display devices. J. Appl. Phys. 121 , 023108 (2017).
Pulli, K. 11-2: invited paper: meta 2: immersive optical-see-through augmented reality. Soc. Inf. Disp. Int. Symp . Dig. Tech. Pap. 48 , 132–133 (2017).
Lee, B. & Jo, Y. in Advanced Display Technology: Next Generation Self-Emitting Displays (eds Kang, B., Han, C. W. & Jeong, J. K.) 307–328 (Springer, 2021).
Cheng, D. W. et al. Design of an optical see-through head-mounted display with a low f -number and large field of view using a freeform prism. Appl. Opt. 48 , 2655–2668 (2009).
Zheng, Z. R. et al. Design and fabrication of an off-axis see-through head-mounted display with an x–y polynomial surface. Appl. Opt. 49 , 3661–3668 (2010).
Wei, L. D. et al. Design and fabrication of a compact off-axis see-through head-mounted display using a freeform surface. Opt. Express 26 , 8550–8565 (2018).
Liu, S., Hua, H. & Cheng, D. W. A novel prototype for an optical see-through head-mounted display with addressable focus cues. IEEE Trans. Vis. Computer Graph. 16 , 381–393 (2010).
Hua, H. & Javidi, B. A 3D integral imaging optical see-through head-mounted display. Opt. Express 22 , 13484–13491 (2014).
Song, W. T. et al. Design of a light-field near-eye display using random pinholes. Opt. Express 27 , 23763–23774 (2019).
Wang, X. & Hua, H. Depth-enhanced head-mounted light field displays based on integral imaging. Opt. Lett. 46 , 985–988 (2021).
Huang, H. K. & Hua, H. Generalized methods and strategies for modeling and optimizing the optics of 3D head-mounted light field displays. Opt. Express 27 , 25154–25171 (2019).
Huang, H. K. & Hua, H. High-performance integral-imaging-based light field augmented reality display using freeform optics. Opt. Express 26 , 17578–17590 (2018).
Cheng, D. W. et al. Design and manufacture AR head-mounted displays: a review and outlook. Light.: Adv. Manuf. 2 , 24 (2021).
Google Scholar
Westheimer, G. The Maxwellian view. Vis. Res. 6 , 669–682 (1966).
Do, H., Kim, Y. M. & Min, S. W. Focus-free head-mounted display based on Maxwellian view using retroreflector film. Appl. Opt. 58 , 2882–2889 (2019).
Park, J. H. & Kim, S. B. Optical see-through holographic near-eye-display with eyebox steering and depth of field control. Opt. Express 26 , 27076–27088 (2018).
Chang, C. L. et al. Toward the next-generation VR/AR optics: a review of holographic near-eye displays from a human-centric perspective. Optica 7 , 1563–1578 (2020).
Hsueh, C. K. & Sawchuk, A. A. Computer-generated double-phase holograms. Appl. Opt. 17 , 3874–3883 (1978).
Chakravarthula, P. et al. Wirtinger holography for near-eye displays. ACM Trans. Graph. 38 , 213 (2019).
Peng, Y. F. et al. Neural holography with camera-in-the-loop training. ACM Trans. Graph. 39 , 185 (2020).
Shi, L. et al. Towards real-time photorealistic 3D holography with deep neural networks. Nature 591 , 234–239 (2021).
Jang, C. et al. Retinal 3D: augmented reality near-eye display via pupil-tracked light field projection on retina. ACM Trans. Graph. 36 , 190 (2017).
Jang, C. et al. Holographic near-eye display with expanded eye-box. ACM Trans. Graph. 37 , 195 (2018).
Kim, S. B. & Park, J. H. Optical see-through Maxwellian near-to-eye display with an enlarged eyebox. Opt. Lett. 43 , 767–770 (2018).
Shrestha, P. K. et al. Accommodation-free head mounted display with comfortable 3D perception and an enlarged eye-box. Research 2019 , 9273723 (2019).
Lin, T. G. et al. Maxwellian near-eye display with an expanded eyebox. Opt. Express 28 , 38616–38625 (2020).
Jo, Y. et al. Eye-box extended retinal projection type near-eye display with multiple independent viewpoints [Invited]. Appl. Opt. 60 , A268–A276 (2021).
Xiong, J. H. et al. Aberration-free pupil steerable Maxwellian display for augmented reality with cholesteric liquid crystal holographic lenses. Opt. Lett. 46 , 1760–1763 (2021).
Viirre, E. et al. Laser safety analysis of a retinal scanning display system. J. Laser Appl. 9 , 253–260 (1997).
Ratnam, K. et al. Retinal image quality in near-eye pupil-steered systems. Opt. Express 27 , 38289–38311 (2019).
Maimone, A. et al. Pinlight displays: wide field of view augmented reality eyeglasses using defocused point light sources. In Proc. ACM SIGGRAPH 2014 Emerging Technologies (ACM, Vancouver, Canada, 2014).
Jeong, J. et al. Holographically printed freeform mirror array for augmented reality near-eye display. IEEE Photonics Technol. Lett. 32 , 991–994 (2020).
Ha, J. & Kim, J. Augmented reality optics system with pin mirror. US Patent 10,989,922 (2021).
Park, S. G. Augmented and mixed reality optical see-through combiners based on plastic optics. Inf. Disp. 37 , 6–11 (2021).
Xiong, J. H. et al. Breaking the field-of-view limit in augmented reality with a scanning waveguide display. OSA Contin. 3 , 2730–2740 (2020).
Levola, T. 7.1: invited paper: novel diffractive optical components for near to eye displays. Soc. Inf. Disp. Int. Symp . Dig. Tech. Pap. 37 , 64–67 (2006).
Laakkonen, P. et al. High efficiency diffractive incouplers for light guides. In Proc. SPIE 6896, Integrated Optics: Devices, Materials, and Technologies XII . (SPIE, San Jose, California, United States, 2008).
Bai, B. F. et al. Optimization of nonbinary slanted surface-relief gratings as high-efficiency broadband couplers for light guides. Appl. Opt. 49 , 5454–5464 (2010).
Äyräs, P., Saarikko, P. & Levola, T. Exit pupil expander with a large field of view based on diffractive optics. J. Soc. Inf. Disp. 17 , 659–664 (2009).
Yoshida, T. et al. A plastic holographic waveguide combiner for light-weight and highly-transparent augmented reality glasses. J. Soc. Inf. Disp. 26 , 280–286 (2018).
Yu, C. et al. Highly efficient waveguide display with space-variant volume holographic gratings. Appl. Opt. 56 , 9390–9397 (2017).
Shi, X. L. et al. Design of a compact waveguide eyeglass with high efficiency by joining freeform surfaces and volume holographic gratings. J. Optical Soc. Am. A 38 , A19–A26 (2021).
Han, J. et al. Portable waveguide display system with a large field of view by integrating freeform elements and volume holograms. Opt. Express 23 , 3534–3549 (2015).
Weng, Y. S. et al. Liquid-crystal-based polarization volume grating applied for full-color waveguide displays. Opt. Lett. 43 , 5773–5776 (2018).
Lee, Y. H. et al. Compact see-through near-eye display with depth adaption. J. Soc. Inf. Disp. 26 , 64–70 (2018).
Tekolste, R. D. & Liu, V. K. Outcoupling grating for augmented reality system. US Patent 10,073,267 (2018).
Grey, D. & Talukdar, S. Exit pupil expanding diffractive optical waveguiding device. US Patent 10,073, 267 (2019).
Yoo, C. et al. Extended-viewing-angle waveguide near-eye display with a polarization-dependent steering combiner. Opt. Lett. 45 , 2870–2873 (2020).
Schowengerdt, B. T., Lin, D. & St. Hilaire, P. Multi-layer diffractive eyepiece with wavelength-selective reflector. US Patent 10,725,223 (2020).
Wang, Q. W. et al. Stray light and tolerance analysis of an ultrathin waveguide display. Appl. Opt. 54 , 8354–8362 (2015).
Wang, Q. W. et al. Design of an ultra-thin, wide-angle, stray-light-free near-eye display with a dual-layer geometrical waveguide. Opt. Express 28 , 35376–35394 (2020).
Frommer, A. Lumus: maximus: large FoV near to eye display for consumer AR glasses. In Proc. SPIE 11764, AVR21 Industry Talks II . Online Only (SPIE, 2021).
Ayres, M. R. et al. Skew mirrors, methods of use, and methods of manufacture. US Patent 10,180,520 (2019).
Utsugi, T. et al. Volume holographic waveguide using multiplex recording for head-mounted display. ITE Trans. Media Technol. Appl. 8 , 238–244 (2020).
Aieta, F. et al. Multiwavelength achromatic metasurfaces by dispersive phase compensation. Science 347 , 1342–1345 (2015).
Arbabi, E. et al. Controlling the sign of chromatic dispersion in diffractive optics with dielectric metasurfaces. Optica 4 , 625–632 (2017).
Download references
Acknowledgements
The authors are indebted to Goertek Electronics for the financial support and Guanjun Tan for helpful discussions.
Author information
Authors and affiliations.
College of Optics and Photonics, University of Central Florida, Orlando, FL, 32816, USA
Jianghao Xiong, En-Lin Hsiang, Ziqian He, Tao Zhan & Shin-Tson Wu
You can also search for this author in PubMed Google Scholar
Contributions
J.X. conceived the idea and initiated the project. J.X. mainly wrote the manuscript and produced the figures. E.-L.H., Z.H., and T.Z. contributed to parts of the manuscript. S.W. supervised the project and edited the manuscript.
Corresponding author
Correspondence to Shin-Tson Wu .
Ethics declarations
Conflict of interest.
The authors declare no competing interests.
Supplementary information
Supplementary information, rights and permissions.
Open Access This article is licensed under a Creative Commons Attribution 4.0 International License, which permits use, sharing, adaptation, distribution and reproduction in any medium or format, as long as you give appropriate credit to the original author(s) and the source, provide a link to the Creative Commons license, and indicate if changes were made. The images or other third party material in this article are included in the article’s Creative Commons license, unless indicated otherwise in a credit line to the material. If material is not included in the article’s Creative Commons license and your intended use is not permitted by statutory regulation or exceeds the permitted use, you will need to obtain permission directly from the copyright holder. To view a copy of this license, visit http://creativecommons.org/licenses/by/4.0/ .
Reprints and permissions
About this article
Cite this article.
Xiong, J., Hsiang, EL., He, Z. et al. Augmented reality and virtual reality displays: emerging technologies and future perspectives. Light Sci Appl 10 , 216 (2021). https://doi.org/10.1038/s41377-021-00658-8
Download citation
Received : 06 June 2021
Revised : 26 September 2021
Accepted : 04 October 2021
Published : 25 October 2021
DOI : https://doi.org/10.1038/s41377-021-00658-8
Share this article
Anyone you share the following link with will be able to read this content:
Sorry, a shareable link is not currently available for this article.
Provided by the Springer Nature SharedIt content-sharing initiative
This article is cited by
Color liquid crystal grating based color holographic 3d display system with large viewing angle.
- Qiong-Hua Wang
Light: Science & Applications (2024)
Mass-produced and uniformly luminescent photochromic fibers toward future interactive wearable displays
- Manu Gopakumar
- Gun-Yeal Lee
- Gordon Wetzstein
Nature (2024)
Enhancing the color gamut of waveguide displays for augmented reality head-mounted displays through spatially modulated diffraction grating
- Jae-Sang Lee
- Seong-Hyeon Cho
- Young-Wan Choi
Scientific Reports (2024)
Effects of virtual reality exposure therapy on state-trait anxiety in individuals with dentophobia
- Elham Majidi
- Gholamreza Manshaee
Current Psychology (2024)
Quick links
- Explore articles by subject
- Guide to authors
- Editorial policies

- Tools and Resources
- Customer Services
- Original Language Spotlight
- Alternative and Non-formal Education
- Cognition, Emotion, and Learning
- Curriculum and Pedagogy
- Education and Society
- Education, Change, and Development
- Education, Cultures, and Ethnicities
- Education, Gender, and Sexualities
- Education, Health, and Social Services
- Educational Administration and Leadership
- Educational History
- Educational Politics and Policy
- Educational Purposes and Ideals
- Educational Systems
- Educational Theories and Philosophies
- Globalization, Economics, and Education
- Languages and Literacies
- Professional Learning and Development
- Research and Assessment Methods
- Technology and Education
- Share This Facebook LinkedIn Twitter
Article contents
Visual and screen-based research methodologies.
- Cleo Mees Cleo Mees Macquarie University
- and Tom Murray Tom Murray Macquarie University, https://orcid.org/0000-0002-9587-643X
- https://doi.org/10.1093/acrefore/9780190264093.013.1196
- Published online: 23 May 2019
Visual and screen-based research practices have a long history in social-science, humanities, education, and creative-arts based disciplines as methods of qualitative research. While approaches may vary substantially across visual anthropology, sociology, history, media, or cultural studies, in each case visual research technologies, processes, and materials are employed to elicit knowledge that may elude purely textual discursive forms. As a growing body of visual and screen-based research has made previously-latent aspects of the world explicit, there has been a concomitant appreciation that visual practices are multisensory and must also be situated within a broader exploration of embodied knowledge and multisensory (beyond the visual) research practice. As audio-visual projects such as Lucien Castaing-Taylor and Véréna Paravel's Leviathan (2013), Rithy Panh's S-21: The Khmer Rouge Death Machine (2003), and Margaret Loescher’s Cameras at the Addy (2003) all demonstrate, screen-based research practices are both modes of, and routes to, knowledge. These projects also demonstrate ways in which screen-based visual research may differ from research exclusively delivered in written form, most specifically in their capacity to document and audio-visually represent intersubjective, embodied, affective, and dynamic relationships between researchers and the subjects of their research. Increasingly, as a range of fields reveal that the incorporative body works as an integrated “perceptive field” as it processes sensory stimuli, visual and screen-based research practices will fulfil an important role in facilitating scholarly access to intuitive, affective, embodied, and analytical comprehension.
- multisensory knowledge
- embodied knowledge
- non-textual discourse
- visual methods
- methodology
Introduction
This article gives an overview of some visual and screen-based methods employed in social sciences, humanities, education, and creative arts research, and explores the unique ways of “knowing” that these methods enable. We begin by providing a historical account of the scholarly uses of visual methods, from their troubled and troublesome origins in the late 19th and early 20th centuries , through to a more recent “visual turn” in the humanities that was driven by the increasing uptake of reflexive, sensory, embodied and participatory approaches to research, and an increasing confidence in the capacity of visual methods to facilitate such approaches. We then go on to describe several ways in which visual and screen-based methods allow researchers to engage with “forms of experience that are either un-securable or much more difficult to secure through other representational forms” (Eisner, as cited in O’Donoghue, 2012 , para. 3). We propose that these methods facilitate multisensory, embodied, personal, empathetic and locomotive routes to knowing about the world. Three examples of visual practice feature in our discussion: Rithy Panh’s documentary film, S-21: The Khmer Rouge Death Machine ( 2003 ); Lucien Castaing-Taylor and Véréna Paravel’s sensory ethnography of a fishing trawler, Leviathan ( 2013 ); and Margaret Loescher’s participatory photography and video project undertaken at “the Addy,” a children’s playground in northern England ( 2003 ). In closing, we consider future developments and remaining questions in the field of visual research.
A Disciplinary Context
In acknowledgement of our own subjectivity, we thought it important to note that we write from backgrounds in documentary filmmaking, performance, and creative practice research informed by history and visual anthropology scholarship. The overview that follows reflects this in several ways.
Firstly, some terminology in this article may be described as “poetic.” This, as Leah Mercer, Julie Robson, and David Fenton ( 2012 ) note, is common in creative arts research, and can help to explain aspects of creative practice “without flattening the liveliness of . . . somatic, aesthetic [approaches]” (p. 16).
Secondly, we write from the understanding that knowledge emerges through context-specific, material practices, and that methodologies appropriate to one context may not be appropriate in others (Barrett & Bolt, 2010 ; Douglas & Gulari, 2015 ; Nelson, 2013 ; Smith & Dean, 2009 ). As such, this article does not aim to be in any way prescriptive.
A History of Visual Research Methodologies
Numerous theorists of visual research have noted the “deep distrust” and “troubled relationship” that social science disciplines have had with visual representations of their key subject areas, such as material culture, social knowledge, and human behavior (Banks, 2001 ; Collier, 1957 ; Pink, 2007 ; Ruby, 2000 ). Indeed, it has been argued that “one of the hallmarks of twentieth-century western thought” was “profound anxiety” toward vision, and its suppression and denigration in favor of textual discourse (Grimshaw & Ravetz, 2005 , pp. 5–6). This suspicion of visual methods has some logic, particularly when we consider the widely discredited 19th-century applications of visual methods (predominantly illustration and photography) employed to advance views based on the superiority of certain races and social classes. This problematic work is most closely associated with various schools of “physical” (rather than cultural) anthropology in France (Paul Broca, Alphonse Bertillon), England (Francis Galton), Germany (Ernst Haeckel, Leo Frobenius), Switzerland (Rudolf Martin), and Austria (Rudolf Pöch), to name just a few of the exponents (Evans, 2010 ; Harper, 1998 ; Morris-Reich, 2013 ). Connections between visual work, colonial aspirations, and state propaganda (particularly during times of war—see Evans, 2010 ) added to concerns regarding the compromising potential of visual materials. Some obvious examples include Leni Riefenstahl’s films in 1930s Germany, and the “Empire Marketing Board” films funded by the British government between 1926 and 1934 , where the producer, John Grierson, put his “Technic [sic] of the Propaganda film” to the creative task of marketing the produce of the British Empire (see Elliot 1931 , pp. 742–744).
Alongside associations with propagandist, racist, and other forms of discriminatory practice, visual media also came to be identified with populist forms of art and entertainment, as well as with less authoritative and intellectual sources of media production, such as tourism and journalism (Grimshaw & Ravetz, 2005 , p. 5). These were all fields with which nascent, professionalizing disciplines wanted no connection. Additionally, within anthropology at least, it has been argued that the preoccupations of mid-20th-century scholarship—with culture as an abstraction in the United States, and with social structure in Britain—had little need for visual tools and methods, as these concerns were much better suited to the analytical form of writing (Banks, 2001 ; Morphy & Banks, 1997 , p. 9). Meanwhile, in sociology, an emphasis by scholars on the statistical analysis of social patterns may account for the dearth of visual research in that field between 1920 and 1960 (Harper, 1988 , p. 58). All of this may serve to account for the 20th-century predominance of writing as a sober, trustworthy, and appropriate form of discourse in which to investigate and describe the world.
This does not mean, however, that visual documentation and the gathering of visual evidence were absent from research practices during this period. What follows is a brief and chronological account of some significant research projects that included visual materials as central to their research aims, beginning with photography in the late 19th and early 20th century .
Photography was integral to a number of early anthropological fieldwork projects, including Alfred Court Haddon’s expeditions to the Torres Strait Islands ( 1888–1899 ), Ryuzo Torii in China, Korea, and Taiwan ( 1895–1911 ), the Hamburg Ethnographic Museum’s South-Seas expedition to the “German” Pacific in 1908–1910 , and Bronislaw Malinowski’s fieldwork in Melanesia ( 1914–1918 ). While moving film was captured during this period, it was not until the 1920s and the work of U.S. documentary pioneer Robert Flaherty and his Russian contemporary Dziga Vertov that “documentary” films began to exploit narrative and descriptive capabilities of the medium that would be inspirational to later visual anthropologists, including French anthropologist and filmmaker Jean Rouch from the 1950s onward (Rouch, 2003 ). Rouch, in turn, developed a method he described as “ cinéma-vérité ” in homage to the “ kino-pravda ” movement of Vertov and others in Russia. His approach would become a key inspiration for later visual anthropologists, in particular because of its reflexive and participatory ethos (MacDougall, 1998 ).
A quick survey of other definitive visual research must suffice to complete this history. Among these must be included the 1930s work of Margaret Mead and Gregory Bateson, who used photography to a then-unprecedented extent in their studies of culture and social organization in Bali (for a discussion on their films of this era, see Henley, 2013 ). In the 1942 work Balinese Character Bateson and Mead ( 1942 , p. xii) described their project in this way:
we were separately engaged in efforts to translate aspects of culture never successfully recorded by the scientist, although often caught by the artist . . . [our work] attempted to communicate all those intangible aspects of culture which had been vaguely referred to as its ethos. . . . By the use of photographs, the wholeness of each piece of behavior can be preserved.
From the mid- 20th century onward, John Collier’s work ( 1957 , 1967 ) was influential in establishing photo-elicitation as a research practice, while American writer Lorraine Hansberry’s photographic study of U.S. southern civil rights issues in The Movement ( 1964 ) and Bruce Davidson’s 1971 study of black “ghetto” life (Bailey & McAtee, 2003 ; Harper, 1998 ) offered examples of how photography could be used as a research tool in sociology. Paolo Freire, the Brazilian educationalist and sociologist who pioneered “dialogic pedagogy” (Freire, 1970 ), was also foundational in his use of photography in a 1973 project designed to investigate the lived experience of Peruvian slum dwellers. Freire asked his subjects to document their lives in photographs rather than in words, a project that has also been influential in the development of “participatory visual methods.”
It would be impossible to conclude a historical overview of the area without reflecting on the “crisis of representation” (Marcus & Fischer, 1986 , pp. 9–12) that engulfed anthropological discourse in the 1970s as it dealt with disciplinary fragmentation, and with accusations of being a discipline of “merely Western significance” and “colonialist” in nature (Asad, 1973 ; Winthrop, 1991 ). These concerns, allied to broader introspection as a result of participation in the Vietnam war, the publication of Bronislaw Malinowski’s diary revealing a dubious regard for his subjects, and the disclosure of clandestine use of social scientists in Latin America and Southeast Asia, precipitated a “crisis of confidence and loss of innocence” for anthropology (Ruby, 1980 , p. 154). This had significant implications for visual research, as it did for the social sciences as a whole. As Jay Ruby ( 1980 ) notes, it was no longer possible for researchers to be “shamans of objectivity” and it has since become widely appreciated that “all serious filmmakers and anthropologists have ethical, aesthetic, and scientific obligations to be reflexive and self-critical about their work” (p. 154).
In response to these challenges researchers began to develop new and increasingly reciprocal relations with their subjects, and to be more reflective about structural power dynamics, authorial positions, and “looking relations” with subjects, often leading to more shared and collaborative forms of authorship (Gaines, 1986 ; Ginsburg, 1994 , 1995 ; Michaels, 1986 ). All of these challenges would also greatly accelerate the future application of visual research practices, leading to what scholars have described as a “pictorial” (Mitchell, 1994 ) or “visual turn” in cultural research (Jay, 2002 ; Pauwels, 2000 ). In what follows, we will advance a position that this emphasis on the visual also encouraged the consideration of sensory, affective, and embodied dimensions to scholarship (Pink, 2009 , 2012 , para. 7; Rose, 2014 , p. 30).
Beyond Textual Approaches to Knowledge
Visual materials, as discussed in the section, “ A History of Visual Research Methodologies ,” have been a component of qualitative and quantitative research methods for a long time. The legitimacy and efficacy of these practices as methodological tools, however, have been an ongoing source of contention. Indeed, for most of the 20th century —if they were employed at all—visual materials and research practices were primarily understood to function as adjuncts to conceptual and text-based knowledge, useful as sources of data, or as “an audiovisual teaching aid,” as Jay Ruby ( 2000 , p. 3) put it. Skepticism of their value, and ridiculing of the idea that visual methods “might become . . . [more] than mere tools in fieldwork” have continued until recently (Wolcott, 1999 , p. 216, emphasis in original). It has become more common, however, for scholars in the social sciences, education, media studies, and creative arts to acknowledge the value of nontextual and nonverbal ways of knowing, mediating, and communicating experience. These methods can bring us into contact with the world in novel and enlightening ways, with images deployed “not merely [as] appendages to the research but rather [as] inseparable components to learning about our social worlds” (Stanczak, 2011 , para. 6).
Visual anthropologists Anna Grimshaw and Amanda Ravetz ( 2005 , pp. 5–6) make a case that the “dominance of linguistic, semiotic and textual models of interpretation” that characterized 20th-century academic practice has recently begun to erode in the face of a “more phenomenologically inflected” and “sensuous scholarship.” Indeed, the uptake of visual methods is wrapped up in a broader sensory and embodied turn in the humanities (Pink, 2012 , para. 7; Rose, 2014 , p. 30), in which the interconnectedness of the senses and the emotive, tacit, corporeal, and ineffable dimensions of knowing are deemed increasingly valid and worthy of investigation.
A problem remains, however, namely that many of these domains of human experience exist “beyond discursive reach” (Grimshaw & Ravetz, 2005 , p. 6), and attempts to investigate them through nonlinguistic means have sometimes been problematic within a “logocentric” university context (Ruby, 1996 , p. 1351). This has been particularly true of creative arts research, where “personally situated, interdisciplinary and diverse and emergent approaches” (Barrett & Bolt, 2010 , p. 2), including research presented in nontextual forms, have been challenged as (in)valid generators of knowledge.
What follows in this article is not intended as a survey of all the nontextual forms of research enquiry and dissemination that exist across performance, the creative arts, education, the humanities and social sciences in the early 21st century . Instead, we wish to concentrate on visual and “screen-based” research (we use this term in order to encompass the wide range of formats and contexts in which visual screen media can be found), in which the medium of research delivery and dissemination is itself screen-based, and in which the world is explored “through the grain” of the visual medium (MacDougall, 1998 , p. 76). For this reason, we will include three case studies of screen-based research from scholar-screen producers, including Véréna Paravel, Lucien Castaing-Taylor, Rithy Panh, and Margaret Loescher. In each case the researchers describe learning about the world and discovering the essence of their specific knowledge quest through the distinctly material, sensory, and social processes of screen production. Their image-making processes were not so much “an aesthetic or scientific performance” as that they formed the very “arena of inquiry” (MacDougall, 1998 , p. 136), an idea that Lucien Castaing-Taylor ( 1996 ) put in a series of rhetorical questions more than two decades ago: “What if film not only constitutes discourse about the world but also (re)presents experience of it? What if film does not say but show ? What if a film does not just describe but depict ? What, then, if it offers not only ‘thin descriptions’ but also ‘thick depictions’?” (p. 86, emphasis in original).
In making an argument for these screen-based research projects as methodologically powerful ways of accessing previously latent understandings, and hence new knowledge, we do not wish to encourage binary oppositions between written and non-written forms of research, or between the increasingly redundant scholarly division between “theory” and “practice.” Rather, we prefer to draw attention to “all the possible variations in the way [these components] can be combined” (Mercer et al., 2012 , p. 11). This is because, in the first instance, many visual research strategies are employed to support what are ultimately text-based qualitative methods and publications (Rose, 2014 ; Stanczak, 2011 ); and in the second instance, because text-based publications can also facilitate sensory and embodied scholarly practices. Laura U. Marks’s work ( 2002 ) on “haptic criticism,” for example, proposes that writing can offer mimetic, tactile, and experiential accounts of the world that are not so much interested in arriving at clear interpretations of events as brushing up closely to experience and “[forming] multiple points of contact [with it]” (p. vx). This suggests that particular routes to knowledge do not so much rely on a choice of medium as on a particular approach to knowing and mediating. With this important qualification, we will now describe what we see as some fundamental aspects of screen-based visual research. We will illustrate these with reference to the three case studies mentioned in the Introduction to this article.
Some Characteristics of Visual “Knowing” and Screen-Based Research
Multisensory knowledge.
The multisensory nature of vision—and an appreciation of the senses as fundamental to how we understand the world and interpret and represent the worlds of others—has become increasingly significant to scholarship in the humanities and social sciences (Pink, 2009 , p. 7). This understanding has led to calls for further scholarly attention to the multisensory body as a research tool (Howes, 2003 , p. 27).
Vivian Sobchack ( 1992 ), in her work on the phenomenology of vision and the spectatorship of screen works, makes the point that “the senses . . . cooperate as a unified system of access. The lived-body does not have senses. It is, rather, sensible. . . . My entire bodily existence is implicated in my vision” (pp. 77–78). It is a point that has been numerously made since Maurice Merleau-Ponty ( 1962 ) described the body as a “synergic system” of interconnected faculties (as cited in Ingold, 2000 , p. 268) where the body works as an integrated “perceptive field” (MacDougall, 1998 , p. 50). Appreciating the interconnectivity of the senses in this way leads to an understanding of the ways in which audiovisual media offer a multisensory (rather than bi-sensory) encounter with the lives and worlds of other beings (Pink, 2012 , paras. 2–5).
As we explore in more depth later, many discussions of the interconnected functioning of the senses are additionally concerned with the way that the act of looking also facilitates a form of touching , a kind of contact with the world that involves (following Merleau-Ponty) mimesis: that is, a “resonance of bodies” that emerges through an imitation of the “postural schema” of other entities (MacDougall, 1998 , p. 53). By enabling mimetic and multisensory encounters, visual media can teach us about the world in distinctly experiential ways that are replete with affective, emotive, and ambiguous dimensions (Rutherford, 2006 , p. 136).
Véréna Paravel and Lucien Castaing-Taylor’s feature-length film, Leviathan , provides a strong example of how a technically “bi-sensory” medium can convey a multisensory understanding of places, people, and processes.
Case Study : Leviathan
Carrying us from night to day, and back into night aboard a fishing trawler, Leviathan consists of a series of long takes, a roaring soundscape, and virtually no human speech. Michael Ungar’s suggestion that the film creates an audiovisual rendition of the experience of being aboard the ship (2017, p. 15) feels apt: we begin the film disoriented, clanking about in the dark, unsure of where we are, or of what we are seeing.
This state of disorientation prompts us to sensorily ascertain the parameters of our environment: its textures, forms, weight, smells, and temperatures. Visual ethnographer Sarah Pink’s argument that we use vision to make multisensory evaluations of materials (such as evaluating whether an object is hot to the touch, heavy to lift, etc.) rings true here: we use the aural and visual materials available to us to develop a multisensory understanding of an unfamiliar environment. Sound, condensation on camera lenses, and flashes of recognizable forms in the maelstrom create sensations of extreme wetness, of hard wind, and hard work. When the camera is pushed underwater, we meet the sharp edges of danger: shards of broken coral flash menacing and close, and we feel the force of water rushing past the ship’s keel. Rather than telling us about this world, the film subjects us to its sensory physicality, giving us an embodied and affective sense of its stakes, and of the elements within it.
Disorientation and ambiguity are key attributes of this work and are intentionally contrary to disciplinary norms that Castaing-Taylor has described as “the discursive and its desire for transparency” (in MacDonald, 2013 , p. 295). Paravel and Castaing-Taylor ( 2013 ) have stated that their “purpose was to give people a very potent aesthetic experience, to give them a glimpse into a reality that they haven’t had first-hand – a protracted, painful, difficult, visceral, profound embodied experience. . . . Our desire was simply to give an experience of an experience . . .” (as cited in Pavsek, 2015 , p. 6).
For those who argue that Leviathan makes a contribution to scholarly knowledge, as we do, its value exists in what each of us extract from this “experience.” Anna Grimshaw ( 2011 ), for example, has argued that it opens “a space between the experiential and propositional, between the perceptual and conceptual” (pp. 257–258), which it does through a disavowal of conventional codes of semiotic screen-based meaning, such as forms of direct address to the audience (voice-over, text, interview), or indirect address through on-screen characters. We are asked to construct meaning through our own sensory experience of the film rather than through a “semiotic coding and decoding” that co-director Paravel believes, “cuts off viewers from the pro-filmic world in the very act of seeming to provide them with authoritative knowledge about it” (as cited in interview with Alvarez, 2012 , para. 13).
Empathetic, Mimetic, and Embodied Knowledge
The notion that looking becomes a sort of touching (mentioned in the sub-section “ Multisensory Knowledge ”) is significantly based on the idea of kinesthetic empathy: the idea that when we look at movement, we are able to mimic that movement in our own bodies and establish a kind of physical contact with it. Knowledge of the world thus emerges from what Anna Gibbs ( 2010 ) calls a “ borrowing of form that might be productively thought of as communication” (p. 193, emphasis added), or even what Sarah Pink ( 2009 ) has called “(audio)visual sensory apprenticeship” (para. 1). This idea is significant because it points to another way in which screen-based research might communicate with audiences.
A central feature of kinesthetic empathy is what neuroscientists Vittorio Gallese and Michele Guerra call “embodied simulation.” This revolves around the activity of mirror neurons in the brain. When a person watches other humans (or animals, or entities) do things—like eat an apple or jump up and down—their mirror neurons fire in exactly the way that they would if they were doing that thing themselves, producing a physiological, empathetic response (Gallese & Guerra, 2012 , p. 184). As Karen Nakamura ( 2013 ) notes, theories of kinesthetic empathy dovetail with theories of synesthesia (or, the ways sensory information can flow across, or trigger, multiple sensory channels at once) (p. 135), further bolstering our understanding of the human body as a “unified system of access” to the world (Sobchack, 1992 , p. 77).
The concept of kinesthetic empathy also has a strong basis in philosophical thought. Philosopher David Abram ( 1996 ), for example, invokes the work of Merleau-Ponty to imagine an epistemology that does not so much aim to achieve a “mastering” overview of the world, as to participate with it. This means entering into a physical “conversation” with things, working with them and mimicking them, such that we “enter into a sympathetic relation with [the world]” and achieve an “attunement or synchronization between [our] own rhythms and the rhythms of the things themselves” (p. 54). In such an epistemology, the sensible is not comprehended by us, but rather “animate[s]” us, and “thinks itself within [us]” (p. 55). Anne Rutherford ( 2003 ), in a similar spirit, describes the effect of mimesis as “a kind of contact—a mode of sensory, tactile perception that . . . closes the gap between the spectator and image” (p. 127). Looking thus provides, through a process of empathy and attunement, a shared sense of physical locomotion as a way of getting closer to the experiences of other entities.
It is important, however, to note the ethical complexities that surround the notion of “empathy”—achieved either through mimetic processes, or any other method. As feminist scholar Sandra Bartky argued in her book, Sympathy and Solidarity ( 2002 ), our capacity to gain access to the experiences (and particularly the suffering) of others will always remains limited. And if, by putting ourselves in the shoes of others, we partially overwrite their experience with our own, then perhaps empathy is not always appropriate, or sufficiently respectful of others’ difference. Such concerns must continue to be explored in accounts of the communicative capacities of audiovisual media.
By accepting this qualification, the significant idea here is that moving with or like the world teaches us about it in an intimate, embodied way, and has the capacity to bring forth both new and remembered knowledge. This might happen through the physical retracing of particular movement pathways in the body and in place (Pink & Leder-Mackley, 2014 , p. 147), through re-enacting or performing historical events (Dening, 1996 ; McCalman & Pickering, 2010 ; Pink & Leder-Mackley, 2014 ) and, as we have described earlier in this section, through the kinesthetic, empathetic, mimetic act of looking.
The potential of movement to bring about new and remembered knowledge is foundational to the visual and re-enactment methods employed in the making of Rithy Panh’s S-21: The Khmer Rouge Death Machine ( 2003 —henceforth S-21 ), as well as to its knowledge claims.
Case Study : S-21
S-21 reunites perpetrators and victims of state-sanctioned torture at the titular “S-21” prison during the repressive rule of the Khmer Rouge between 1975 and 1979 . Panh has explained that the visual methods he used for the film were founded on a belief in the powerful ways that multisensory environments, actions and gestures, and (audio)visual materials could function as “footholds” in the process of knowing and remembering (Oppenheimer, 2012 , p. 244). In the film, the former guards of the Khmer Rouge–run S-21 prison are faced with the enormous challenge of recalling, describing, and reflecting on their crimes. The following account by Panh shows how central the revisiting of sensory states, locations, and actions was to accessing repressed, traumatic, and often ineffable memories:
I met Paul, who does the re-enactment in the film, in his native village. And I understood that this man wanted very much to explain what he had done at S21. But he couldn’t get round to explaining it properly, all his phrases were cut off. So at a certain moment I brought him a map of the camp. And so he said, “oh yes, I was a guard in this part of the building.” So then he was able to explain, but in doing that he made the gestures that you see in the film, which completed the phrases he couldn’t discuss. And it’s then that I discovered that there was another memory, which is the bodily memory. . . . Sometimes the violence is so strong that words don’t suffice to describe it. . . . So it was then that I said to the guard “you can use gestures, you can speak, explain it in any way you wish.” And then that I had the idea [sic] of taking the guard back to S21, which is now a museum of the genocide, and because the guard said that he worked at night there, I took him there at night. I asked at the museum how the building was lit at night—it was lit only by neon—so I cut all the other lighting and just put the neon up there. I sought to create an atmosphere, which recalled the situation, which the guard was actually working in. Sometimes at night they had the radio on with revolutionary songs so that’s why the radio came into it, with the revolutionary songs. . . . I made him listen to the songs. . . . It’s like giving somebody a foothold to get up a mountain. He needs to have these grips . . . in order to achieve what he’s setting out to do, which is to describe his own testimony. (Oppenheimer, 2012 , p. 244)
In addition to recording the film at the location where the atrocities occurred, re-creating sensory and physical environments, and inviting his participants to re-enact what they did as a means to remembering it, Panh also used elicitation devices such as the sprawling archive of photographs, logbooks, and other documents that remained from the prison as props to facilitate remembering (Oppenheimer, 2012 , p. 245). Sensory encounters with these artifacts supported participants in the process of testifying. In the film, we see these processes at work. We see the former prison guards enter the former cells, yell at, handcuff, physically assault, and escort imaginary (and/or remembered) prisoners; we see them leaf through and recite from the logbooks and other archival materials provided by the filmmakers. Doing these tasks helps the guards to start talking about the unspeakable things they participated in many years ago, reflecting Gillian Rose’s observation ( 2014 ) that visual methods allow participants and researchers access to not only aspects of experience that are multisensory, but also to affective or feelingful experiences that are ineffable (p. 28), or difficult to talk about.
Panh’s belief in the power of mimicry to produce empathy and understanding meant that he refused to enter the prison cells with the guards when they were re-enacting their routines. The prisoners had (historically) been chained to the floor, lying down in rows like sardines—and so to walk into the room, Panh, said, would have been akin to stepping on them, throwing into question his moral position as a filmmaker. As Panh put it: “it was instinctive to stop, to hold the camera at the door, not to follow in. Otherwise we’d be walking over the prisoners, if you like. And would knock over into the side of the guards. . . . If I had done, ‘who would I be?’” (Oppenheimer, 2012 , p. 245).
It was a space in which Panh did not belong, either historically or in the process of re-enactment. He literally had no place there, and in the dynamic of re-enactment—which is an environment of mimicry and empathy where affects and emotions might spill from body to body through shared physical movement (Gibbs, 2010 )—his presence would confuse and disturb. His presence would also demand that he be a social “actor,” in which case Panh’s question, “who would I be?” describes a powerful (and impossible) ethical rhetoric. Such dilemmas get to the heart of the methods used in S-21 to access and represent the events of the past in mimetic, embodied, and affective ways.
Screen-Based Research Is Informed by Material Contexts and Is Process-Driven
In much early- 21st-century visual and creative practice research, it is accepted that research outcomes follow from the material contexts and processes of production. This is to say that “thinking” and “making” require material tools, and interactions between these materials and the subjects of research are part of a dynamic that influences both the research processes and outcomes of the work (as argued by Paul Carter in Material Thinking [ 2004 ]). This is also to appreciate that research materials (for example, a camera, a screen) can serve as both the means of investigation, and the means of research dissemination, and that these have their own capacities and limitations.
As just one example, the camera’s ability to objectify others, and the capacity for the embodied presence of researchers and their instruments to shape events and distort “pro-filmic” reality, has been amply noted (Bruzzi, 2000 ; Gaines, 1986 ; MacDougall, 1998 ; Mulvey, 1975 ; Rouch, 2003 ). The critical concept here is that the space between subject and camera/operator is an inherently “intersubjective” and dynamic one. The act of recording and rendering a subject—the particular way in which a place is materialized on screen for example—is in itself a description of the circumstances and decision-making processes embedded (and embodied) within the moment of capture. Sarah Pink ( 2007 ), for example, suggests that the places she films consist of multiple interweaving trajectories, including the trajectory of the camera. In her definition of place (which draws on definitions put forward by Tim Ingold and Doreen Massey), places are not fixed, but reconstituted moment by moment, depending on these variously moving entities and trajectories.
Trinh T. Minh-ha also describes the image capturing process in distinctly relational terms, calling it “an intrinsic activity of image-making and of relation-forming.” She writes that “the subject who films is always caught in the process of relating—or of making and re-presenting—and is not to be found outside that process” (Lippit & Minh-ha, 2012 , para. 15–19). Jean Rouch would agree that the camera instigates many of the movements and responses it captures. For him, the “fundamental problem in social science,” namely that “you distort the answer simply by asking a question” (Georgakas, Gupta, Janda, & Rouch, 1978 , p. 22), must be embraced and openly examined in the screen works one makes.
One outcome of this reflexive awareness is a growing tendency to prioritize participatory and participant-led modes of screen research—methods where subjects have agency to negotiate, and even direct the ways they are represented (Ruby, 1996 , p. 1350). In such approaches, the screen researcher is required to share the filmmaking process with the subjects of the research, and to operate with a “willingness to be decentred in acts of translation” (Clifford, 2000 , p. 56).
Such practices not only help to redress the historical power imbalances implicit in visual and social research, they can also provide a unique form of knowledge in that they record, and give material form to, the negotiation of knowledge and representation between researcher and subject. As Rose ( 2014 ) points out, “taking a photo always entails some sort of negotiated relationship between the person making the image and those being pictured” (p. 29), and the resulting image can, if the researcher allows for it, bear the very imprint of that negotiation. The traces of negotiations surrounding representation, power, and knowledge embedded in participatory visual media may offer us unique routes to thinking about these issues.
All of the case studies invoked in this article were profoundly informed by material and relational processes of production (see Oppenheimer, 2012 , p. 243, and MacInnis, 2013 , p. 60), and the following case study offers an insight into how the materials employed within the research project were part of a negotiated and process-driven method of research.
Case Studies: Cameras at the Addy
Margaret Loescher’s visual ethnography project at “the Addy,” an adventure playground in Hulme, northern England, provides a good example of a collaborative ethnography, in which the subjects have been allowed forms of agency in which to represent themselves. The project explored the ways children navigate and make use of urban spaces, and resulted in the production of a photo essay, a documentary film, and reflective writing.
When Loescher ( 2003 ) set out to film her six- to eight-year-old subjects at play in “the Addy” in an observational style, she noticed that they would—against her intentions—constantly perform to her camera, drawing on pop culture references and in fact using the camera as a “doorway into the world of ‘pop’ culture” (p. 79). After a period of inner resistance to this, she gave the children disposable cameras to represent their own lives and play. Upon looking at the composition of the photographs the children took, she learned that when they were performing to the camera, they were not trying to be someone “other” than their authentic selves, and that these performances were in fact ways of self-identifying in a contemporary, media-saturated cultural landscape, and of “forging relationships with their urban [play] environment” (p. 80).
In addition to the children’s unexpected response to her camera, Loescher’s choice to give them disposable cameras to record their own lives was driven by a discomfort with her own relative power to represent the subjects of her research, particularly given the substantial differences in age and class that she noted between them (p. 77). She reflects that giving the children disposable cameras did something to shift the power balance. Armed with cameras of their own, “[the children] are learning about [the camera] as much as it is learning about them.” This “disarms the camera as a force of categorization and potential oppression and pulls it into the children’s world. It becomes another thing which signifies them as social agents, like the television, the mobile telephones, the football ground and the pop-star poster [that feature] in the photographs taken by the children” (p. 84).
Loescher’s work also foregrounds the sometimes uneasy negotiation of knowledge and representation between researcher and subject, and provides an example of how this might occur “through the very grain of the filmmaking” (MacDougall, 1998 , p. 76). At the beginning of her screen work she includes a recording of her initial interaction with one of her subjects, six-year-old Ainsley. She recalls that this meeting had “an air of uncertainty and mistrust” about it. “I am wondering what this boy is ‘about’. I want to know him and he wants to know me; but I am unsure on what basis we will be ‘knowing’ each other” (Loescher, 2003 , p. 77). A negotiation of the terms on which subject and researcher would “know” each other was then undertaken with and through the camera, and included in public documentation of the research.
Loescher’s work provides a particularly vivid example of a process-driven methodology that is strongly influenced by the interpersonal and material process of recording still and moving images with her subjects. Cameras at the Addy reflects the ways that visual methods are both informed by, and constructive of, relational (and social, and therefore ethical) encounters. Knowledge emerges from these encounters, and resides in them as they unfold.
Event-Based Knowledge
Visual research methods, and screen-based research in particular, can constitute forms of knowing through events rather than through concepts. Addressing observational approaches to documentary and ethnographic filmmaking in particular, MacDougall ( 1998 ) writes:
By focusing on discrete events rather than abstract concepts . . . and by seeking to render faithfully the natural sounds, structure, and duration of events, filmmakers have hoped to provide the viewer with sufficient evidence to judge the film's larger analysis. . . . [These films] are essentially revelatory rather than illustrative, for they explore substance before theory. (p. 126)
MacDougall is here describing the ways in which screen media forms can capture and represent the inherent ambiguity of events and entities and resist clear-cut conclusions about them. MacDougall ( 2006 ) writes that “what we show in images . . . is a different knowledge, stubborn and opaque, but with a capacity for the finest detail. . . . This puts (film) at odds with most academic writing, which, despite its caution and qualifications, is a discourse that advances always toward conclusions” (p. 6).
While images may promise insight and overview (rendering their subjects legible and subject to interpretation), they may equally come with minimal guidelines for how they should be read, and may even resist interpretation—as Laura U. Marks ( 2002 ) has argued in her work on the “haptic” for example. This approach to rendering experience is evident in visual research works like Leviathan . It has been argued that this “different knowledge,” which is inherently subjective and events-based, also signals “a significant epistemological, philosophical, and aesthetic shift . . . founded in a new approach to the world that respect[s] its materiality, its continuity, and fundamental ambiguity” (Grimshaw, 2011 , p. 255). It should be noted that the quality of being ambiguous—or “downright mysterious” as Catherine Russell’s ( 2005 ) critique of Leviathan describes it (p. 28)—is not universally appreciated. Some critics see in this work a conscious “disavowal” of meaning-making, and are concerned about the ethical implication of viewers left to make “sense of that world on their own terms” (Pavsek, 2015 , pp. 8–9). What is certain is that a scholarship that foregrounds “revelation,” and embodied, affective, and sensory experience over discourses of explanation and illustration is unconventional and challenging to traditional scholarship.
Leviathan presents events in a way that some have argued is “analogous to the experience of the filmmaker at the ethnographic site” with a seeming absence of contextualizing that might “clarify or conceptualize that experience” (Ungar, 2017 , p. 14). The lack of obvious discursive strategies, and the “openness” (Russell, 2015 , p. 28) of the authorial and narrative structure leads commentators such as Allan MacInnis to reflect that the film does not seem to have the same “polemical intent” as other films dealing with the suffering of animals in the meat industry. Rather, he feels that the film presents animal death and suffering with a “mixture of brutality and beauty,” which “opens [his] thoughts” (MacInnis, 2013 , pp. 58–59), delaying moral judgement and emphasizing the complexity of its subject(s).
Indeed, in Leviathan , blood flies as marine animals are hacked unceremoniously to pieces, but the film does not seem to incriminate fishermen, or even make clear-cut judgements about fishing as an industry. This could be because the film’s composition frustrates attempts to extract messages or social/political meanings from it (Thain, 2015 , p. 44). This is not only due to the absence of spoken or written guidelines for interpretation (for, as Russell [ 2015 [ notes, “visual and audio material can also be textual” [p. 32]), but also due to the ways the moving images and sounds are assembled to create a landscape of “productive disorientation[s]” (Thain, 2015 , p. 42). As viewers, we may be so consumed with the process of keeping ourselves afloat in the film’s immersive flood of sensory information, that the additional work of judging what we are sensing becomes a lesser priority. Or perhaps it is that the extended, intimate moments we have with the fishermen themselves “amplify” (Rutherford, 2006 , p. 153) our sense of both their unknowability (or opacity), and their humanity.
In fact, the same might be said of the approach to filming the former Khmer Rouge guards in S-21 . In both films, the choice to express (or preserve) the temporal dimension of specific concrete events (a fishing trawler at sea, re-enacted historical scenes) may allow subjects to transform under our sustained gaze. A significant knowledge-based implication of this strategy may be that this kind of scholarship “opens up” contemplative spaces regarding the subjects and “pro-filmic” world being represented to us, rather than configuring a form of knowledge that advances quickly “towards conclusions” (MacDougall, 2006 , p. 6).
Future Developments, Remaining Questions
The final section of this article will make some brief propositions regarding future directions and remaining questions in the area of visual and screen-based research methods.
It seems to us that the ubiquity of screen-based knowledge delivery (despite the continuing dominance of textual discourse in the early 21st century ), together with a growing confidence in the unique knowledge-creation capacities of visual screen-based media methods, as discussed in this article, will facilitate greater instances of audiovisual, nontextual knowledge production. Some of the forms this knowledge production will take are bound to challenge conventional ideas of what constitutes “scholarly knowledge.”
It may be that the knowledge contribution of research incorporating re-enacted, embodied, sensory, affective, and experiential concerns will be sufficiently discrete from existing research categories that new ones are demanded, such as Brad Haseman’s case for “performative” research methods (2006, p. 98), that would stand alongside quantitative and qualitative methodologies. Haseman defines “performative” research outputs as those that embody or enact the questions and concerns they are “about.” These do not need to be delivered in traditional textual form. Yet, much of this “knowledge” may just as easily fall into existing categories of discursive practice—for there is no reason that audiovisual texts be any less “discursive” (putting aside the specific merits and demerits of forms of “discourse”) than written ones.
Much of the research discussed here, and the various “turns” of cultural and scholarly attention, point to a growing diversification of research methods. To take just one example only briefly discussed: the methods that might follow from the concept of knowing as something that emerges in a context-specific process of making (Smith & Dean, 2009 ). In relation to this idea, Tim Ingold ( 2011 ) suggests that in a world consisting of materials on the move —where things do not so much have properties as that they have histories (p. 30)—we can imagine that there will be new epistemological challenges to the things we know, and to our methods for coming to know them.
This article has highlighted research that invites ambiguity, heterogeneity, and uncertainty (Barrett & Bolt, 2010 ; Haseman, 2006 ; Nelson, 2013 ), and debates will certainly continue about the scholarly potential of this kind of research. Ross Gibson ( 2010 ), for example, has noted that responding to experimental and experiential research that often seeks to reveal “tacit” understandings (see Polanyi, 1966 for a description of “tacit knowledge”) of the kind that we have described, requires an “acknowledgement” (a shift in knowledge) that necessitates new critical and analytical strategies of comprehension. We must enable ourselves, he writes, to be “immersed and extracted, involved yet also critically distanced” (Gibson, 2010 , p. 10). In other words, Gibson informs us, the consumption of this research requires both discipline and reflection, and sometimes contradictory processes of intuitive, affective, sober, embodied, and analytical comprehension. The challenge, both for researchers and for those seeking to gain access to the knowledge communicated in these forms of research, is to “entwine the insider’s embodied know-how with the outsider’s analytical precepts” (Gibson, 2010 , p. 11). Paul Carter ( 2010 ), writing in response to claims of a lack of “rigor” in research he describes as “aleatory,” wonders if it is not, on the contrary, “a sign of its sophistication” that this work remains “constitutionally open” in comparison to scientific approaches that “identif[y] power with abstraction and the dematerialisation of thought from the matrix of its production” (p. 16).
As ever, much revolves around questions of support for such practices in a university context (Barrett & Bolt, 2010 ; Carter, 2010 ; Haseman, 2006 ; Nelson, 2013 ; Van Loon, 2014 ), and the ways in which academic cultures, institutions, and governments respond to the challenges of shifting epistemologies and methodologies that seek to investigate the world.
Finally, persistent questions about the ethics and politics of using images will continue to be important as image-making technologies and global political and media landscapes continue to evolve. The ethical dimensions of representation, and of what we do with visual technologies, must always remain integral to the contemplation and revision of visual and screen-based research methods.
Further Reading
- Banks, M. (2001). Visual methods in social research . Thousand Oaks, CA: SAGE.
- Barrett, E. , & Bolt, B. (Eds.). (2010). Practice as research: Approaches to creative arts Enquiry . London, U.K.: I. B. Tauris.
- Carter, P. (2004). Material thinking: The theory and practice of creative research . Carlton, Australia: Melbourne University Publishing.
- Grimshaw, A. , & Ravetz, A. (Eds.). (2005). Visualizing anthropology . Bristol, U.K.: Intellect Books.
- Howes, D. (2003). Sensual relations: Engaging the senses in culture and social theory . Ann Arbor: University of Michigan Press.
- Ingold, T. (2011). Being alive: Essays on movement, knowledge and description . Abingdon, U.K.: Routledge.
- MacDougall, D. (2006). The corporeal image: Film, ethnography and the senses . Princeton, NJ: Princeton University Press.
- Margolis, E. , & Pauwels, L. (Eds.). (2011). The SAGE handbook of visual research methods . London, U.K.: SAGE.
- Marks, L. U. (2002). Touch: Sensuous theory and multisensory media . Minneapolis: University of Minnesota Press.
- Morphy, H. , & Banks, M. (Eds.). (1997). Rethinking visual anthropology . New Haven, CT: Yale University Press.
- Nelson, R. (2013). Practice as research in the arts: Principles, protocols, pedagogies, resistances . New York, NY: Palgrave Macmillan.
- Oliver, J. (Ed.). (2018). Associations: Creative practice and research . Melbourne, Australia: Melbourne University Press.
- Pink, S. (2009). Doing sensory ethnography , London, U.K.: SAGE.
- Prosser, J. (Ed.). (1998). Image based research: A sourcebook for qualitative researchers . London, U.K.: Falmer Press.
- Ruby, J. (2000). Picturing culture: An exploration of film and anthropology . Chicago, IL: University of Chicago Press.
- Smith, H. , & Dean, R. T. (2009). Practice-led research, research-led practice in the creative arts . Edinburgh, U.K.: Edinburgh University Press.
- Sobchack, V. (1992). The address of the eye: A phenomenology of film experience . Princeton, NJ: Princeton University Press.
- Abram, D. (1996). The spell of the sensuous: Perception and language in a more-than-human world . New York, NY: Vintage Books.
- Alvarez, P. (2012). Interview with Verena Paravel and J. P. Sniadecki . Visual and New Media Review, “Cultural Anthropology” website.
- Asad, T. (1973). Anthropology and the colonial encounter . London, U.K.: Ithaca Press.
- Aufderheide, P. (2008). Documentary film: A very short introduction . New York, NY: Oxford University Press.
- Bailey, J. , & McAtee, D. (2003). “Another way of telling”: The use of visual methods in research. International Employment Relations Review , 9 (1), 45–60.
- Banks, M. (2007). Visual data in qualitative research . Thousand Oaks, CA: SAGE.
- Barnouw, E. (1993). Documentary: A history of the non-fiction film (2nd rev. ed.). New York, NY: Oxford University Press.
- Bartky, S. (2002). “Sympathy and Solidarity” and other essays . Lanham, MD: Rowman & Littlefield.
- Bateson, G. , & Mead, M. (1942). Balinese character: A photographic analysis . New York: The New York Academy of Sciences.
- Bolt, B. (2010). The magic is in handling. In E. Barrett , & B. Bolt (Eds.), Practice as research: Approaches to creative arts enquiry (pp. 27–34). London, U.K.: I. B. Tauris.
- Bruzzi, S. (2000). New Documentary—A Critical Introduction (2nd ed.). London, U.K.: Routledge.
- Carter, P. (2010). Interest: The ethics of invention. In E. Barrett , & B. Bolt (Eds.), Practice as Research: Approaches to creative arts enquiry (pp. 15–26). London, U.K.: I. B. Tauris.
- Castaing-Taylor, L. (Director, Producer), & Paravel, V. (Director, Producer). (2013). Leviathan [Motion picture]. New York, NY: Cinema Guild.
- Clifford, J. (2000). An ethnographer in the field. In Coles, A. (Ed.), Site-specificity: The ethnographic turn (pp. 52–73). London, U.K.: Black Dog Press.
- Collier, J. (1957). Photography in anthropology: A report on two experiments. American Anthropologist , 59 (5), 843–859.
- Collier, J. (1967). Visual anthropology: Photography as a research method . Albuquerque: University of New Mexico Press.
- Dening, G. (1996). Performances . Chicago, IL: University of Chicago Press.
- Douglas, A. , & Gulari, M. N. (2015). Understanding experimentation as improvisation in arts research . Qualitative Research Journal , 15 (4), 392–403.
- Eliot, W. (1931). The work of the Empire Marketing Board. Journal of the Royal Society of Arts , 79 (4101), 736–748.
- Evans, A. D. (2010). Anthropology at War: World War I and the science of race in Germany . Chicago, IL: University of Chicago Press.
- Foster, S. L. (2011). Choreographing empathy: Kinesthesia in performance . New York, NY: Routledge.
- Freire, P. (1970). Pedagogy of the oppressed . New York, NY: Herder and Herder.
- Gaines, J. (1986). White privilege and looking relations: Race and gender in feminist film theory. Cultural Critique , 4 (Fall), 59–79.
- Gaines, J. (1999). Political mimesis. In J. Gaines , & M. Renov (Eds.), Collecting visible evidence (pp. 84 – 102). Minneapolis: University of Minnesota Press.
- Gallese, V. , & Guerra, M. (2012). Embodying movies: Embodied simulation and film studies. Cinema , 3 , 183–210.
- Georgakas, D. , Gupta, U. , Janda, J. , & Rouch, J. (1978). The politics of visual anthropology: An interview with Jean Rouch. Cinéaste , 8 (4), 16–24.
- Gibbs, A. (2010). After affect: Sympathy, synchrony and mimetic communication. In M. Gregg , & G. Seigworth (Eds.), The affect theory reader (pp. 186–204). Durham, NC: Duke University Press.
- Gibson, R. (2010). The known world. Text , 14 (2) (Special Issue #8), 1–11.
- Ginsburg, F. (1994). Culture/media: A (mild) polemic. Anthropology Today , 10 (2), 5–15.
- Ginsburg, F. (1995). The parallax effect: The impact of Aboriginal media on ethnographic film. Visual Anthropology Review , 11 (2), 64–76.
- Ginsburg, F. (2002). Screen memories: Signifying the traditional in indigenous media. In F. Ginsburg , L. Abu-Lughod , & B. Larkin (Eds.), Media worlds: Anthropology on new terrain (pp. 39–57). Berkeley: University of California Press.
- Grimshaw, A. (2011). The Bellwether ewe: Recent developments in ethnographic filmmaking and the aesthetics of anthropological inquiry . Cultural Anthropology , 26 (2), 247–262.
- Gubrium, A. , & Krista, H. (2013). Participatory visual and digital methods . Walnut Creek, CA: Left Coast Press.
- Hansen, M. (1999). Benjamin and cinema: Not a one-way street. Critical Inquiry , 25 , 306–343.
- Harper, D. (1988). Visual sociology: Expanding sociological vision. American Sociologist , 19 (1), 54–70.
- Harper, D. (1998). An argument for visual sociology. In J. Prosser (Ed.), Image based research: A sourcebook for qualitative researchers (pp. 24–41). London, U.K.: Falmer Press.
- Haseman, B. (2006). A manifesto for performative research. Media International Australia , 118 (1), 98–106.
- Henley, P. (2013). From documentation to representation: Recovering the films of Margaret Mead and Gregory Bateson. Visual Anthropology , 26 (2), 75–108.
- Howes, D. (2003). Sensual Relations: Engaging the senses in culture and social theory . Ann Arbor: University of Michigan Press.
- Ingold, T. (2000). The perception of the environment: Essays in livelihood, dwelling and skill . London, U.K.: Routledge.
- Ingold, T. (2010). Ways of mind-walking: Reading, writing, painting . Visual Studies , 25 (1), 15–23.
- Jay, M. (2002). That visual turn: The advent of visual culture. Journal of Visual Culture , 1 (1), 87–92.
- Law, J. (2009). Seeing like a survey . Cultural Sociology , 3 (2), 239–256.
- Lippit, A. M. , & Minh-ha, T. T. (2012). When the eye frames red .
- Loescher, M. (2003). Cameras at the Addy . Journal of Media Practice , 3 (2), 75–84.
- MacDonald, S. (2013). American ethnographic film and personal documentary: The Cambridge turn . Berkeley: University of California Press.
- MacDougall, D. (1997). The visual in anthropology. In M. Banks & H. Morphy (Eds.), Rethinking visual anthropology (pp. 276 – 295). London, U.K.: Yale University.
- MacDougall, D. (1998). Transcultural cinema . Princeton, NJ: Princeton University Press.
- MacInnis, A. (2013). The aesthetics of slaughter: Leviathan in context. Cineaction , 91 , 58–64.
- Marcus, G. E. , & Fischer, M. J. (1986). Anthropology as cultural critique: An experimental moment in the human sciences . Chicago, IL: University of Chicago Press.
- McCalman, I. , & Pickering, P. A. (2010). Historical reenactment: From realism to the affective turn . Basingstoke, U.K.: Palgrave Macmillan.
- Mercer, L. , Robson, J. , & Fenton, D. (Eds.). (2012). Live research: Methods of practice-led inquiry in performance . Nerang, Australia: Ladyfinger.
- Merleau-Ponty, M. (1962). Phenomenology of perception . London, U.K.: Routledge.
- Michaels, E. , & Australian Institute of Aboriginal Studies (1986). The Aboriginal invention of television in Central Australia, 1982-1986 . Canberra: Australian Institute of Aboriginal Studies.
- Miles, A. (2015, November). About 7am . Paper presented at the Dialogues and Atmospheres Symposium, RMIT and Macquarie University, Melbourne, Australia.
- Minh-ha, T. T. (Director), & Bourdier, J.-P. (Co-Producer). (1982). Reassemblage: From firelight to the screen [Motion picture]. New York, NY: Women Make Movies.
- Mitchell, W. J. T. (1994). Picture theory . Chicago, IL: University of Chicago Press.
- Morris-Reich, A. (2013). Anthropology, standardization and measurement: Rudolf Martin and anthropometric photography. British Journal for the History of Science , 46 (3), 487–516.
- Mulvey, L. (1975). Visual pleasure and narrative cinema. Screen , 16 (3), 6–18.
- Murphy, S. (2012). Writing practice. In L. Mercer , J. Robson , & D. Fenton (Eds.), Live research: Methods of practice-led inquiry in performance (pp. 164–174). Nerang, Australia: Ladyfinger.
- Nakamura, K. (2013). Making sense of sensory ethnography: The sensual and the multisensory . American Anthropologist , 115 (1), 132–144.
- Nichols, B. (1991). Representing reality: Issues and concepts in documentary . Bloomington: Indiana University Press.
- Nichols, B. (2010). Introduction to documentary (2nd ed.). Bloomington: Indiana University Press.
- O’Donoghue, D. (2012). Doing and disseminating visual research: Visual arts-based approaches. In E. Margolis , & L. Pauwels (Eds.), The SAGE handbook of visual research methods . London, U.K.: SAGE.
- Oppenheimer, J. (2012). Perpetrators’ testimony and the restoration of humanity: S-21 , Rithy Panh. In J. Ten Brink , & J. Oppenheimer (Eds.), Killer images: Documentary film, memory and the performance of violence (pp. 243–255). London, U.K.: Wallflower Press.
- Oppenheimer, J. (Director), & Sørensen, S. B. (Producer). (2012). The act of killing [Motion picture]. Norway, Denmark, U.K.: Det Danske Filminstitut, Dogwoof Pictures.
- Panh, R. (Director), Couteau, C. (Producer), & Hastier, D. (Producer). (2003). S-21: The Khmer Rouge death machine [Motion picture]. Cambodia, France: Institut National de l’Audiovisuel, First Run Features.
- Pauwels, L. (2000). Taking the visual turn in research and scholarly communication: key issues in developing a more visually literate (social) science . Visual Sociology , 15 (1), 7–14.
- Pavsek, C. (2015). Leviathan and the experience of sensory ethnography . Visual Anthropology Review , 31 (1), 4–11.
- Pink, S. (2007). Walking with video. Visual Studies , 22 (3), 240–252.
- Pink, S. (2009). Visualising emplacement: Visual methods for multisensory scholars . In Doing sensory ethnography . London, U.K.: SAGE.
- Pink, S. (2012). A multisensory approach to visual methods . In E. Margolis , & L. Pauwels (Eds.), The SAGE handbook of visual research methods . London, U.K.: SAGE.
- Pink, S. , & Leder-Mackley, K. (2012). Video and a sense of the invisible: Approaching domestic energy consumption through the sensory home. Sociological Research Online , 17 (1), 1–19.
- Pink, S. , & Leder-Mackley, K. (2014). Re-enactment methodologies for everyday life research: Art therapy insights for video ethnography . Visual Studies , 29 (2), 146–154.
- Polanyi, M. (1966). The tacit dimension . London, U.K.: Routledge.
- Rose, G. (2014). On the relation between “Visual Research Methods” and contemporary visual culture. Sociological Review , 62 , 24–46.
- Rouch, J. (2003). The camera and man. In S. Field (Ed.), Cine-ethnography (pp. 29–46). Minneapolis: University of Minnesota Press.
- Ruby, J. (1980). Exposing yourself: Reflexivity, anthropology, and film. Semiotica , 30 (1/2), 153–179.
- Ruby, J. (1996). Visual anthropology. In D. Levinson , & M. Ember (Eds.), Encyclopedia of cultural anthropology (Vol. 4, pp. 1345–1351). New York, NY: Henry Holt.
- Russell, C. (2015). Leviathan and the discourse of sensory ethnography: Spleen et idéal . Visual Anthropology Review , 31 (1), 27–34.
- Rutherford, A. (2003). The poetics of a potato: Documentary that gets under the skin. Metro: Media & Education Magazine , 137 (Summer 2003), 126–131.
- Rutherford, A. (2006). “What makes a film tick?”: Cinematic affect, materiality and mimetic innervation (Doctoral dissertation). University of Western Sydney.
- Stanczak, G. C. (2011). Introduction: Images, methodologies, and generating social knowledge . In Visual research methods . Thousand Oaks, CA: SAGE.
- Taussig, M. (1993). Mimesis and alterity: A particular history of the senses . New York, NY: Routledge.
- Thain, A. (2015). A bird’s-eye view of Leviathan . Visual Anthropology Review , 31 (1), 41–48.
- Taylor, L. (1996). Iconophobia. Transition , 69 , 64–88.
- Ungar, M. (2017). Castaing-Taylor and Paravel’s GoPro sensorium: Leviathan (2012), experimental documentary, and subjective sounds. Journal of Film and Video , 69 (3), 3–18.
- Van Loon, J. (2014). The play of research: What creative writing has to teach the academy. TEXT , 18 (1).
- Winston, B. (1988). The tradition of the victim in Griersonian documentary. In A. Rosenthal (Ed.), New challenges for documentary (pp. 269–287). Berkeley: University of California Press.
- Winston, B. (2008). Claiming the real II: Documentary: Grierson and beyond (2nd ed.). New York, NY: Palgrave Macmillan.
- Winthrop, R. H. (1991). Dictionary of concepts in cultural anthropology . Westport, Ireland: Greenwood.
- Wolcott, H. F. (1999). Ethnography: A way of seeing . London, U.K.: AltaMira Press.
Printed from Oxford Research Encyclopedias, Education. Under the terms of the licence agreement, an individual user may print out a single article for personal use (for details see Privacy Policy and Legal Notice).
date: 22 May 2024
- Cookie Policy
- Privacy Policy
- Legal Notice
- Accessibility
- [66.249.64.20|185.80.151.9]
- 185.80.151.9
Character limit 500 /500
- Review article
- Open access
- Published: 07 January 2021
How can basic research on spatial cognition enhance the visual accessibility of architecture for people with low vision?
- Sarah H. Creem-Regehr ORCID: orcid.org/0000-0001-7740-1118 1 ,
- Erica M. Barhorst-Cates 2 ,
- Margaret R. Tarampi 3 ,
- Kristina M. Rand 1 &
- Gordon E. Legge 4
Cognitive Research: Principles and Implications volume 6 , Article number: 3 ( 2021 ) Cite this article
4861 Accesses
5 Citations
4 Altmetric
Metrics details
People with visual impairment often rely on their residual vision when interacting with their spatial environments. The goal of visual accessibility is to design spaces that allow for safe travel for the large and growing population of people who have uncorrectable vision loss, enabling full participation in modern society. This paper defines the functional challenges in perception and spatial cognition with restricted visual information and reviews a body of empirical work on low vision perception of spaces on both local and global navigational scales. We evaluate how the results of this work can provide insights into the complex problem that architects face in the design of visually accessible spaces.
Significance
Architects and designers face the challenge of creating spaces that are accessible for all users, following the principles of Universal Design. The proportion of the population who have uncorrectable visual impairment is large and growing, and most of these individuals rely on their residual vision to travel within spaces. Thus, designing for visual accessibility is a significant practical problem that should be informed by research on visual perception and spatial cognition. The work discussed in this paper presents an empirical approach to identifying when and how visual information is used to perceive and act on local and global features of spaces under severely restricted vision. These basic research approaches have the potential to inform design decisions that could improve the health and well-being of people with low vision and extend more broadly to enhance safety and effective use of designed spaces by all people.
Introduction
Millions of people across the world have low vision , defined as significant uncorrectable visual impairment that impacts essential everyday tasks. Notably, people with low vision have useful residual visual capabilities and often rely on vision as a primary source of information guiding perception and action within their environments. Given this reliance on vision, an important goal in the design of spaces is to increase visual accessibility , to enable the design of environments that support safe and efficient travel for those with visual impairment. Visual accessibility is necessary for full participation within our society, as the ability to travel effectively through one’s environment is critical for independence in accomplishing daily tasks. Limitations in independent mobility due to vision loss lead to debilitating consequences related to quality of life, such as social isolation, reduced opportunities for education and employment, and economic disadvantage.
The goal of this paper is to evaluate how basic research in space perception and spatial cognition can inform the practical design of architectural spaces to improve visual accessibility for people with low vision. First, we provide a background on the prevalence of low vision and the “dimensions” of low vision (reduced acuity, reduced contrast sensitivity, and visual field loss) that are likely to affect space perception and spatial cognition. We discuss the possible effects of reduced visual information on the recruitment of other sensory modalities and the motor system for gathering spatial information, as well as the impact of navigation with low vision on higher-level attention and memory processes. Second, we provide a critical review of studies of low vision concerned with perception on local and global spatial scales, a distinction important to theories of spatial representation and navigation (Ekstrom and Isham 2017 ; Montello 1993 ; Wolbers and Wiener 2014 ). Third, we review the concept of Universal Design and the need to design for visual accessibility analogous to more familiar approaches of designing for physical accessibility. We consider the challenges that architects and lighting designers face in working at multiple scales of space and argue that an understanding of spatial processing with reduced visual information could inform design decisions.
Low vision: prevalence and functional consequences
Estimates of the prevalence of visual impairment vary depending on criteria used, but by all accounts, the number of people who have uncorrectable vision loss is startling. About 441.5 million people are visually impaired worldwide, but only a small percentage (about 8%) have total blindness (Bourne et al. 2017 ) and most are characterized as having low vision . People with low vision have some remaining functional vision and use their residual visual capabilities for many tasks, including reading, object recognition, mobility, and navigation. Low vision is characterized as visual acuity less than 20/40 or a visual field of less than 20°. Clinical diagnosis of severe to profound visual impairment is often defined as 20/200 to 20/1000. In the USA, the statutory definition for legal blindness is defined as best-corrected visual acuity of 20/200 in the better eye or a visual field of no more than 20° (Giudice 2018 ). Recent estimates in the USA show about 5.7 million Americans with uncorrectable impaired vision, and this number is projected to double by 2050 (Chan et al. 2018 ). The number of adults in the USA at risk for vision loss (as defined by factors of older age, diabetes, eye disease) increased by 28 million from 2002 to 2017 to a total of 93 million adults at risk (Saydah et al. 2020 ). This high prevalence and increased risk for low vision should be of significant concern, particularly as associated limitations in the ability and motivation to travel independently are highly related to increased social isolation, depression, and economic disadvantages (Giudice 2018 ; Marston and Golledge 2003 ; Nyman et al. 2010 ).
While the dimensions of low vision are often reported clinically in terms of acuity and contrast sensitivity levels and extent of field of view, Footnote 1 in the work described here we attempt to demonstrate the functional relationship between characterizations of vision loss and spatial behavior. Functioning actively within built spaces relies on the ability to detect and identify environmental geometry such as steps, pillars, or benches so that they do not become mobility hazards. These environmental features also serve a role in providing spatial context such as frames of reference or landmarks to aid in spatial updating, keeping track of one’s current location and orientation in space while moving. Figure 1 provides illustrations of the effect of reduced acuity and contrast sensitivity and reduced peripheral field of view on visibility and use of environmental features. The top two images show a hallway scene with normal acuity (a) and under a simulated acuity of logMAR 1.1 (20/250 Snellen) and Pelli-Robson score of 1.0 (b) (Thompson et al. 2017 ). In the low vision image, the near table is still recognizable, the mid-distance table is detectable as some sort of feature but is not recognizable, and the more distant tables are essentially invisible. The bottom pair of images shows a normal view of a hallway (c) and a simulation of peripheral field loss (d), with a remaining field of 7.5°. While central field acuity and contrast sensitivity are unaffected, tasks such as finding the second door on the right are made much more difficult.
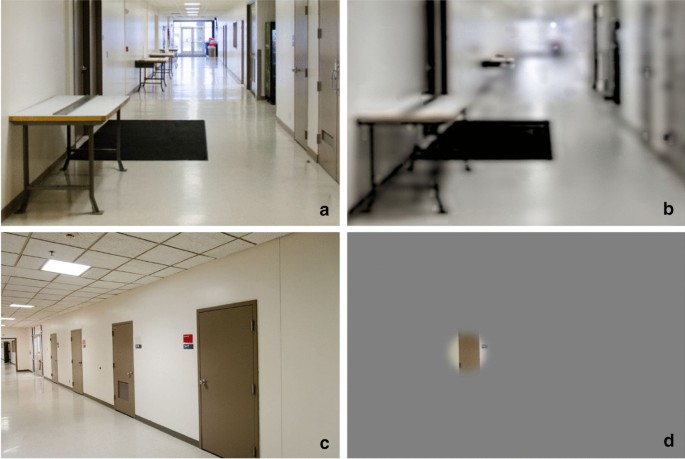
Hallway scenes with normal vision and simulated low vision show possible effects on visibility and use of environmental features for spatial behavior. Photographs by William B. Thompson
One primary approach to assess the impact of low vision on these components of space perception and navigation has been to artificially reduce acuity, contrast sensitivity, or visual field in those with normal vision and test perception and spatial cognition in controlled but real-world laboratory settings. We use the term simulated low vision to describe these artificial reductions, but it is not our intention to convey a specific pathology or assume an accurate representation of the subjective experience of low vision. These studies create viewing restrictions with goggles fitted with occlusion foils or theatrical lighting filters. Much of the experimental work described in this paper falls within the range of severe to profound simulated low vision. Admittedly, simulations using artificial restrictions with normally sighted people do not reproduce behavioral adaptations to vision loss or capture the wide individual variability in low-vision conditions. However, as demonstrated in this review, low vision simulations are a valuable approach because they provide a controlled and less-variable way to assess effects of reductions in visibility of environmental features. This review focuses on the generic effects of reduced spatial resolution, contrast, and field on perceptual interpretation and spatial cognition. While there may be some interactions between specific diagnostic categories, such as glaucoma or macular degeneration, and the cognitive and perceptual factors we are considering, we expect that similar cognitive and perceptual limitations are shared quite broadly across low-vision conditions. We also review some work testing people with actual low vision, showing qualitatively similar effects on perception and recognition of features as found with the low vision simulations.
Predictions about abilities to identify and use environmental features for safe and efficient travel can be informed by the limitations of visual information. For example, those with reduced acuity and contrast sensitivity should have more stringent requirements for angular size of objects and their contrast with surrounding surfaces in order to detect and recognize objects. Reduced acuity and contrast sensitivity should also impact the information that can be used for perceiving scale and distance, such as reliance on high-contrast boundaries rather than high-resolution textures. These features serve as the building blocks for spatial updating and higher-level spatial representations of one’s environment, so we also expect to see influences of low vision on spatial cognition. For example, many models of navigation emphasize visual landmarks (e.g., Chan et al. 2012 ; Chrastil and Warren 2015 ; Ekstrom 2015 ; Epstein and Vass 2014 ) and environmental geometry (Marchette et al. 2014 ; Mou and McNamara 2002 ) as providing frames of reference for spatial learning. Here, in addition to reduced acuity and contrast sensitivity, field of view should also play a role, as it should be more difficult to perceive the scale and shape of large-scale environmental geometry or encode global configurations when experienced in multiple restricted visual snapshots (Fortenbaugh et al. 2007 , 2008 ; Kelly et al. 2008 ; Sturz et al. 2013 ). Importantly, landmark recognition, self-localization, and formation and use of long-term spatial knowledge all involve some amount of attentional resources (Lindberg and Gärling 1982 ), and low vision increases these attentional demands (Pigeon and Marin-Lamellet 2015 ). Low-vision mobility itself requires attentional resources which compete with the attention needed to form spatial memories (Rand et al. 2015 ). We also consider the important role of non-visual body-based information (specifically proprioceptive and vestibular) for spatial updating and spatial learning, that is relied on by both individuals who are normally sighted and those with visual impairment (Giudice 2018 ). Much of the work reviewed here does not focus on auditory or tactile sensory input, although other work suggests that spatialized sound (Giudice et al. 2008 ) and tactile-audio interfaces (Giudice and Palani 2014 ) have the potential to support and enhance spatial navigation performance for people with vision loss.
Impact of low vision on space perception: use of local features
Much of the early research on perception of environmental features in the context of low vision was focused on obstacle avoidance while moving through spaces. This work suggested that visual field loss was a major contributor to safely avoiding visual hazards during locomotion, whereas acuity and contrast sensitivity were less important (e.g., Kuyk et al. 1998 ; Long et al. 1990 ; Marron and Bailey 1982 , Pelli 1987 ). While essential for mobility, obstacle avoidance during walking relies on dynamic cues for distance and self-motion and, as a task, may not reveal the critical contribution of acuity and contrast needed for perception of environmental features from a distance (Ludt and Goodrich 2002 ). From static viewpoints or farther distances, irregularities of ground plane surfaces such as steps and ramps, as well as environmental objects such as benches, posts, and signs may not be visible given low contrast with surrounding surfaces or smaller angular size. Reduced acuity and contrast can affect familiar size cues and perspective-based information used for perceiving distance and scale by reducing high-frequency detail and texture gradients (see Fig. 2 ). These surfaces and objects can become hazards when not detected, recognized, or localized, and their visibility is important to consider when designing for visual accessibility.
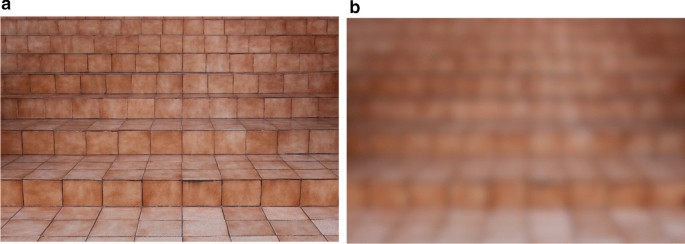
Steps viewed with normal vision ( a ) as compared to simulated degraded acuity and contrast sensitivity ( b ) demonstrating loss of detail and texture gradient of steps
To begin to understand the impact of visibility of ground-plane irregularities on visual accessibility, Legge et al. ( 2010 ) created a long sidewalk inside of an indoor windowless classroom that could be interrupted by a step or ramp, as shown in Fig. 3 . The goal was to test detection and recognition of these steps and ramps in the context of manipulations of lighting direction, target-background contrast, and viewing distance, at different levels of simulated acuity and contrast sensitivity loss created through restricted viewing goggles (referred to as “blur”), as these were predicted to influence the visibility of the cues used to distinguish the environmental feature (see Table 1 for details about local cue studies). Several take-home messages emerged. Steps up were more visible than steps down, and visibility could be helped by enhancing contrast between the riser and contiguous surface with directional lighting. Local image features such as discontinuities in edge contours of a walkway at a step boundary were sources of information highly dependent on viewing distance and contrast (see L-junction in Fig. 4 ). Finally, viewers used the height of the end of the walkway in their visual field to distinguish between a ramp up and a ramp down, showing that the cue of height in the picture plane may be more reliable than local ground surface cues to those with blurred vision because it is less dependent on acuity. Further studies using the same paradigm asked whether providing a high contrast checkerboard texture on the sidewalk would facilitate recognition of the environmental geometry under blur viewing conditions (Bochsler et al. 2012 ). Surprisingly, presence of the surface texture detracted from accuracy in the severe blur condition. Apparently, the transition contrast cue shown to be used to recognize a step up was masked by the high-contrast texture edges from the checkerboard pattern. Similarly, the texture under severe blur appears to mask the L-junction that could be used as a cue to step down (see Fig. 4 ). People with moderate to severe low vision also participated in the same ramps and steps paradigm (Bochsler et al. 2013 ). Overall, they outperformed the normally sighted participants with simulated low vision from Legge et al. ( 2010 ), but the effects of distance, target type, and locomotion were qualitatively similar for the low vision and normal vision participants. Furthermore, environmental objects themselves can become hazards if they are not detected or recognized. Kallie et al. ( 2012 ) identified advantages in object identification for specific shapes and colors that depended on lighting conditions, as well as for larger and closer objects.
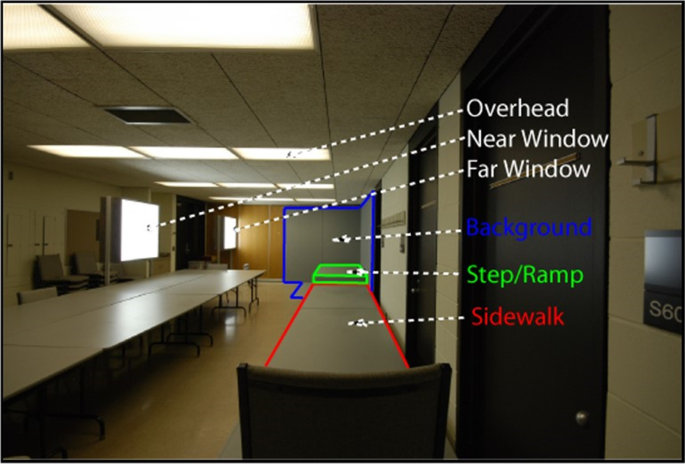
Adapted from Legge et al. ( 2010 )
The constructed sidewalk and room used for the steps and ramps studies.
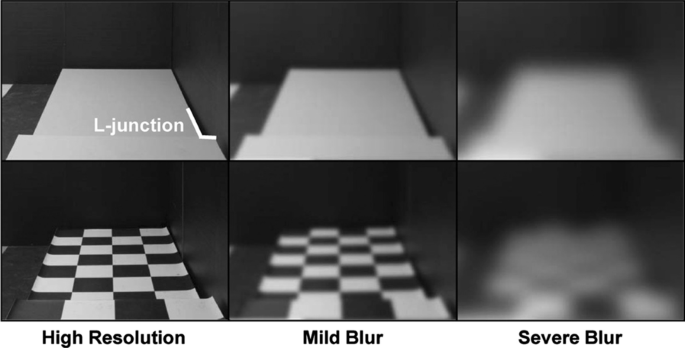
Reprinted with permission from Wolters Kluwer Health, Inc. The Creative Commons license does not apply to this content
The step-down target used in Bochsler et al. ( 2012 ).
The visibility of features is important not only for recognition of surfaces and objects, but also for spatial localization. Successful independent navigation depends on the ability to perceive distances and locations of environmental features, and update 3D representations of space with self-movement. Several studies have used low vision simulation paradigms to examine the perception of distance and size in room-sized environments. For example, in Tarampi et al. ( 2010 ), participants viewed targets in a large indoor room at distances up to 6 m and then walked directly or indirectly to targets while blindfolded. These “blind-walking” tasks are a type of visually directed action measure that indicates perceived distance. Indirect walking involves walking initially in one direction and then on a cue, turning and walking to the target location. Because preplanning motor strategies would be difficult in this unpredictable task, it is a good test of the viewer’s abilities to update their self-location with respect to the environment. Although targets were just barely visible, participants surprisingly showed accurate blind walking to these locations that was comparable to performance in normal vision conditions, revealing relatively intact distance perception, although with increased variability. One explanation for this relatively good performance despite severely degraded vision is that viewers used the visual horizon as a salient cue for judging distance. Sedgwick ( 1983 ) defined the horizon-distance relation or the use of angle of declination between the horizon and a target object as a mechanism for a viewer standing on the ground surface to recover absolute egocentric distance to a location on the ground (see Fig. 5 ).
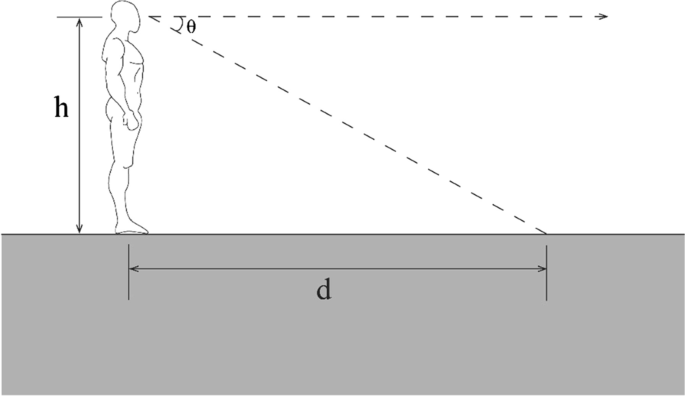
For a viewer standing on a ground plane, the distance ( d ) to locations on the ground can be computed using the horizon-distance relation (angle of declination), scaled by eye height ( h ): d = h cot θ . “Human body front and side” image by Nanoxyde licensed under CC BY-SA 3.0
When a viewer is standing on the ground, the distance to a location on the ground can be computed as a function of one’s eye height and the angle between the line of sight to the horizon and the line of sight to the object. For indoor spaces, the floor-wall boundary plays the role of the visible horizon. Rand et al. ( 2011 ) tested the role of the visual horizon as a cue in a low vision context by artificially manipulating the floor-wall boundary in a large classroom. Because viewers in this study wore blur goggles, it was possible to raise the visible boundary between the floor and wall by hanging material on the wall that matched the floor. When the “horizon” was raised, the angle of declination to the target increased, and as predicted, viewers judged the distance to targets on the ground to be closer. Figure 6 shows a real-world example of this effect. The black carpet on the floor and wall become indistinguishable under blurred viewing conditions, leading to a misperception of the visual horizon and potential errors in perceived distance.
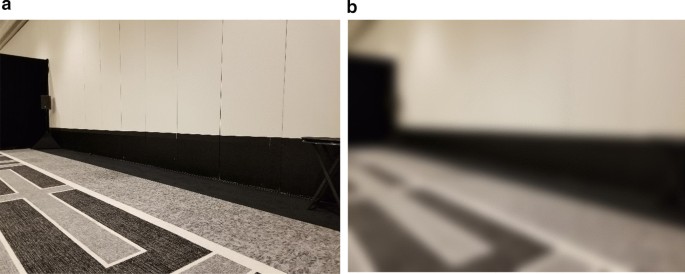
Photograph credit: Margaret Tarampi
Conference Room at Loews Miami Beach Hotel in Miami Beach FL USA under normal vision ( a ) and simulated low vision ( b ).
Further support for the importance of ground surface cues for distance in low vision comes from a study that manipulated the visual information for whether an object is in contact with the ground (Rand et al. 2012 ). Objects that we interact with often make contact with the ground plane, but that point of ground contact may not always be visible, particularly under blurred viewing conditions. For example, furniture may have small or transparent legs, or stands on which objects or signs rest may have low contrast with the ground surface. Gibson’s ( 1950 ) ground theory of perception and insightful demonstrations (see Fig. 7 ) posit that in the absence of cues to suggest that a target is off the ground, viewers will judge distance assuming that the target is in direct contact with the ground. Thus, a target that is off the ground, but assumed to be on the ground, will be perceived to be at a farther distance, consistent with the location on the ground plane that it occludes. In the context of visual accessibility, if the ground contact of an object is not visible, the misperception of the distance of that object could lead to critical collision hazards. Rand et al. ( 2012 ) tested whether manipulating the visibility of the ground-contact support for an object off the ground would lead to the predicted misperception of distance. Participants viewed targets placed on stands that were visible or not due to manipulation of high or low contrast between the stand and the ground plane and manipulations of simulated degraded acuity and contrast sensitivity (see Fig. 8 ). With normal viewing, the stands were visible and distance and size judgments to the targets were accurate. Viewing with blur goggles, the low-contrast gray stand became undetectable and distance and size of the target were overestimated, consistent with Gibson’s predictions of ground theory. These studies demonstrate the importance of the visibility of information for grounding targets when they are located above the ground surface. We will return to this finding in the discussion of implications for design.
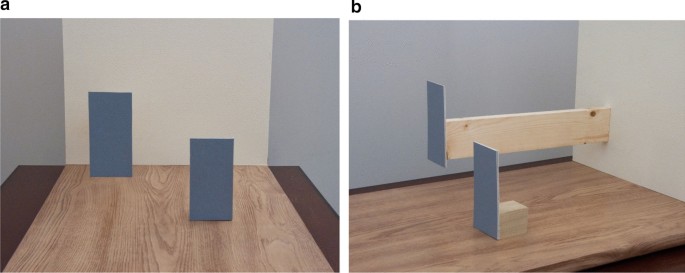
Images motivated by Gibson ( 1950 ) demonstration showing that in the absence of visual information specifying lack of contact with a support surface, a target that is off the surface is perceived to be on the surface but farther away ( a ). Image ( b ) shows the actual configuration in which both objects are the same distance from the camera and the left object is raised off the surface. Created by William B. Thompson
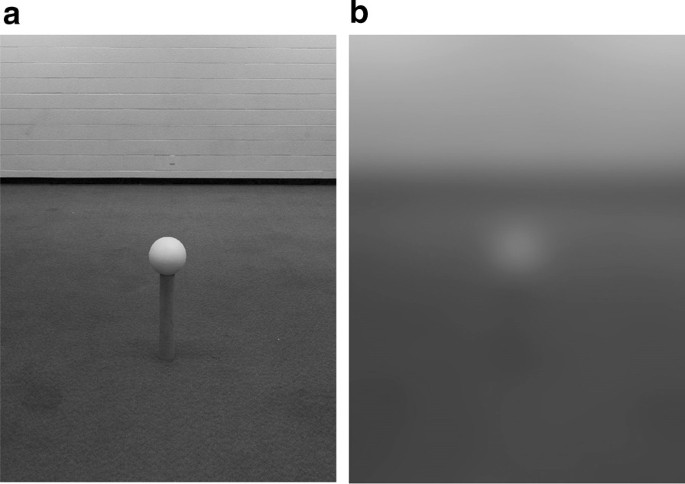
Adapted from Rand et al. ( 2012 ) with permission from Brill
The gray stand is detectable with normal viewing ( a ), but undetectable under degraded vision ( b ). Viewing with blur goggles led to overestimation of distance and size of the target.
Impact of low vision on spatial cognition: global spatial features and locomotion
Thus far we have described the impact of low vision on the visibility of local features, demonstrating that severely blurred vision can impair visual perception of irregularities in surfaces such as ramps and steps, large-scale objects, and perception of distance to objects off the ground. These components are important to understanding spatial perception from static viewpoints at scales immediately surrounding the viewer that can be perceived without locomotion, defined as vista space (Montello 1993 ). However, much interaction with space entails actively traveling through it, requiring perception of distance traveled as well as memory for important landmarks, such as a bathroom or emergency exit. These global features of space are vital to consider for spatial navigation, a complex activity that involves perceptual, sensorimotor, and higher-level cognitive processes. There is a large literature on understanding navigation at both sensorimotor and higher cognitive levels in normally sighted people (for reviews see Chrastil and Warren 2012 ; Ekstrom et al. 2018 ) as well as in blind individuals (Giudice 2018 ; Loomis et al. 1993 ). Normally sighted individuals tend to rely on visual information when it is available and reliable (Zhao and Warren 2015 ), and studies with blind and blindfolded individuals sometimes reveal intact abilities to use non-visual information (Loomis et al. 1993 ; Mittelstaedt and Mittelstaedt 2001 ). However, the residual visual capacity in low vision raises important questions about how people navigate and remember important landmarks when visual information may be present but degraded, an area of research that has received much less attention in the literature.
The environments used to test the impact of low vision on navigation have ranged from simple one-legged paths, to 3 segment spatial updating tasks, to large-scale environments that vary in complexity from long narrow hallways to open environments requiring many turns (see Table 2 for details of global-feature studies). We generally see that low vision type and severity interact with task complexity to influence performance. Whereas the study of local features has focused primarily on the interaction of vision with reduced acuity with surface, geometry, and lighting conditions, examination of global features has extended to simulated peripheral field loss. Reduced peripheral field of view impacts use of global features in spatial cognition in numerous ways, including distance estimation (Fortenbaugh et al. 2007 , 2008 ), perception of global configurations of spatial layout (Yamamoto and Philbeck 2013 ), encoding and use of environmental geometry as a frame of reference (Kelly et al. 2008 ; Sturz et al. 2013 ), and increasing cognitive load (Barhorst-Cates et al. 2016 ).
Legge et al. ( 2016a , b ) measured the impact of low vision on both distance and direction estimates in a simple spatial updating task using a three-segment path completion task in seven different sized rooms (see Fig. 9 ). Surprisingly, none of the reduced vision conditions impaired distance estimates compared to normal vision, but severe blur impaired direction estimates. The automatically acquired information about self-location from real walking (Rieser 1989 ) may have been sufficient for accurate spatial updating except in the severely blurred vision. In other works, a comparison of spatial updating performance between blind, low vision, and normally sighted age-matched controls showed a surprising lack of difference between groups, suggesting that vision was not necessary for accurate performance in a simple spatial updating situation (Legge et al. ( 2016a , b ). Non-visual (body-based) cues (vestibular, proprioceptive) may be used by individuals with both simulated and natural low vision, which allow for overall accurate performance in spatial updating. However, this spatial updating paradigm was relatively simple, requiring participants to process only three distance segments and two turns. Theories of leaky integration assert that increases in distance traveled and number of turns result in greater error accumulation (Lappe et al. 2007 ). While normally sighted individuals can use landmarks to “reset” their path integration when it accumulates error (e.g., Zhao and Warren 2015 ), this capability may not be available to individuals with low vision who do not have access to visual landmarks in the same way, especially in cases of severe acuity or field restriction. Effects of low vision on navigation may thus be more apparent in more complex navigation tasks (longer distances, more turns) that include more opportunity for error accumulation. Rand et al. ( 2015 ) tested spaces on the scale referred to as environmental space (Montello 1993 ), which require greater interaction to represent and cannot be experienced from a single location of the observer. These experiments compared spatial memory accuracy for individuals with simulated acuity and contrast sensitivity degradation after navigating through a large indoor building to those individuals’ own performance with normal vision. Memory for the location of landmarks pointed out along the path was worse in the blurred vision condition compared to the normal vision condition. Using a similar paradigm, decrements in memory accuracy were shown when restricting peripheral field of view (FOV), but only when restricted to severe levels around 4° (Barhorst-Cates et al. ( 2016 ).
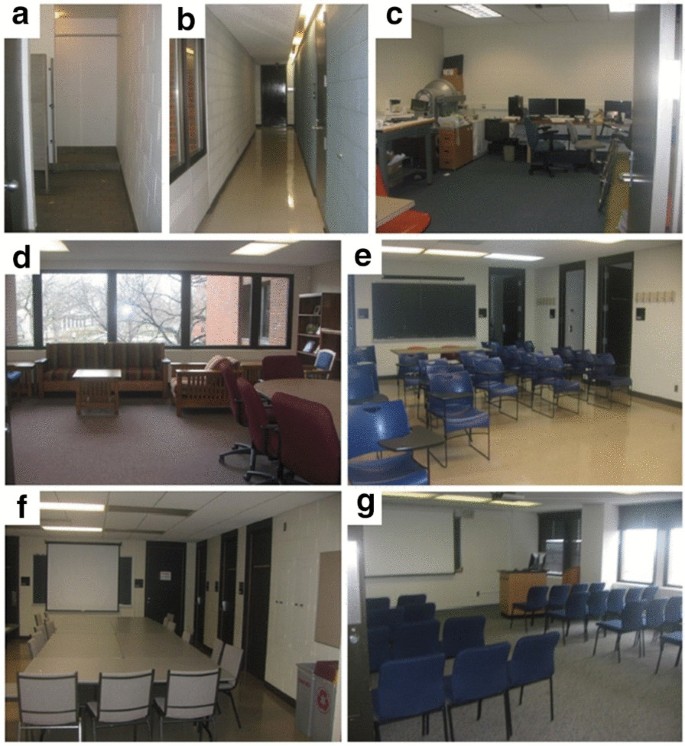
Rooms used in Legge et al. ( 2016a ). Licensed by Creative Commons 4.0
To explain these deficits in performance on spatial cognition tasks with simulated low vision, several studies have tested hypotheses related to perception (Fortenbaugh et al. 2007 , 2008 ; Legge et al. 2016a , b ; Rand et al. 2019 ), attentional demands (Rand et al. 2015 ), and environmental complexity (Barhorst-Cates et al. 2019 ). There is some support for perceptual distortions that could influence more global spatial tasks. For example, participants with simulations of severe acuity reduction and restricted peripheral field misperceive the size of the rooms they are in (Legge 2016a , b ). Room size estimates might be impaired because of difficulty in perceiving the wall-floor boundary, as seen in Rand et al. ( 2011 ). Severe blur results in reductions in visibility of the wall-floor boundary and restricted FOV require a viewer to use more head or eye movements (Yamamoto and Philbeck 2013 ) to perceive the relationship between the wall and the floor, influencing automatic estimates of angle of declination between line of sight and the wall-floor boundary. But surprisingly, actual low vision and normally sighted subjects showed no difference in room size estimates, in contrast to blind individuals who performed at near-chance levels (Legge et al. 2016b ). The discrepant results in simulated compared to actual low vision individuals may be explained by the greater severity of vision reduction in the simulated groups or compensatory perceptual strategies in those with visual impairment (Rieser et al. 1992 ). Another perceptual explanation is that observers misperceive distance traveled while navigating with visual impairment. A series of experiments by Rand et al. ( 2019 ) supports this idea, showing that severe blur results in overestimation of distance traveled and increases the perception of speed of self-motion. Restricted FOV also impairs distance estimates, often resulting in underestimation (Fortenbaugh et al. 2007 , 2008 ).
Beyond explanations based on perception, low vision could influence the cognitive resources needed for spatial learning while navigating. Rand et al. ( 2015 ) provided evidence for an account of mobility monitoring , which posits that attentional demands from locomotion detract from cognitive resources that could be devoted to spatial learning. They implemented a condition that was designed to reduce cognitive demand associated with safe walking by having the experimenter guide the participant and found better memory compared to an unguided condition, both with severe blur. Further, performance on a concurrent auditory reaction time task was faster while guided, indicating reduced cognitive load, and participants reported less anxiety in the guided condition. These data suggested that mobility-related attentional demands influence spatial learning during low vision navigation, beyond the influence of the visual deficit itself. This is an important finding considering the prevalence of mobility deficits in low vision (Marron and Bailey 1982 ). Reducing mobility demands can allow more cognitive resources to be devoted to spatial learning. This effect was replicated in an older adult sample, showing an even stronger effect of guidance on improving spatial memory (Barhorst-Cates et al. ( 2017 )). Mobility is more attentionally demanding for older adults even with normal vision (for a review, see Li and Lindenberger 2002 ), and these data suggest that mobility challenges combined with added attentional demands of low vision may be particularly deleterious for spatial memory in older adults. Effects of attentional demands also extend to navigating with restricted FOV (Barhorst-Cates et al. 2016 ), where attentional demands increase at moderate levels of FOV restriction.
Recent studies with restricted FOV during spatial learning have tested the impact of active navigation and active search (e.g., looking for named targets at uncertain locations) for targets (Barhorst-Cates et al. 2020 ) and environmental complexity (Barhorst-Cates et al. 2019 ). In a comparison of walking and wheelchair locomotion with 10° FOV, spatial memory performance was similar, suggesting that proprioceptive feedback from walking itself does not aid spatial learning (see also Legge et al. 2016a ). A possible explanation is the significant mobility challenges faced with restricted FOV locomotion (Jansen et al. 2010 , 2011 ; Turano et al. 2004 ). While spatial learning could have been facilitated by walking (see Chrastil and Warren 2013 ), being pushed in a wheelchair may also have facilitated learning by reducing the attentional demands associated with low vision mobility, leading to equivalent performance in the two conditions. Attentional demands were also found to increase with restricted FOV when active search for targets was required, although there were not detrimental effects on spatial memory. However, there may be a critical role for environmental complexity (e.g., more clutter, irregularity in structure) in effects on spatial memory when navigating with restricted FOV. The above-described studies all took place in a campus building with long hallways, with 3–4 turn paths. In contrast, indoor navigation often occurs in less structured, more complex contexts that require more turns in open spaces, such as a hotel lobby or convention center. A study addressed this question of environmental regularity using a museum setting, finding decreased memory and increased attentional load with a less severe 10° FOV restriction (Barhorst-Cates et al. 2019 ). Open environments, like museums, introduce mobility and visual complexity demands that pose unique challenges to navigation with restricted FOV, more so than environments with structured hallways, where spatial learning during navigation is largely unimpaired except at extreme FOV restrictions.
Implications for visually accessible architectural design
We conceptualize visual accessibility as parallel to the well-established notion of physical accessibility. Architects are required by law to comply with accessibility guidelines put forward by the Americans with Disabilities Act (ADA), which primarily focuses on providing physical access for those with physical disabilities, such as the inclusion of elevators and ramps and modification of paths and entrances. The ADA does also include guidelines addressing sensory abilities, but these are primarily focused on signage (e.g., the inclusion of Braille) and other forms of communication. In visual accessibility, we emphasize how vision is used to travel safely through environmental spaces, to perceive environmental features, to update one’s position in the environment, and to learn the layout of spaces. Both physical and visual accessibility closely relate to the Principles of Universal Design for architecture—that the key features of environmental spaces that support its function and mobility should be useful to all people (Mace 1985 ). Steinfeld and Maisel’s ( 2012 ) updated definition of Universal Design emphasizes the process “that enables and empowers a diverse population by improving human performance, health and wellness, and social participation”. This revised view acknowledges that designs might not meet all needs, but states that the process brings designs closer to including the needs of as many people as possible. Even though design for visual accessibility focuses on the use of vision (which may not include people who are completely blind), it is an example of this process.
Why is it difficult to take perceptual and cognitive factors into account when designing spaces to enhance accessibility for people with low vision? One reason is that the preponderance of research in the field of architecture is focused on “how buildings are built” corresponding to the second half of the architecture design process, i.e., construction, materiality, and building systems, that have led to innovative and provocative spaces such as Frank Gehry’s Guggenheim Museum Bilbao. Some of these design decisions can unintentionally compromise visibility for low vision, such as creating low-contrast features or glare from skylights or other glass exteriors. While architects are trained to address the challenge of balancing many factors from aesthetics to sustainability to function, some design decisions may unknowingly affect visual accessibility. In contrast, research informing the first half of the architecture design process corresponding to “what is built” has received less attention until recently (Chong et al. 2010 ). There are exciting movements in architecture that take a human-centered approach to design for human health and well-being, such as the WELL Building Standard ( https://www.wellcertified.com/ ) and Fitwel ( https://www.fitwel.org/ ), as well as academic cross-disciplinary fields focused on the human within spaces, such as the Academy of Neuroscience for Architecture ( http://www.anfarch.org/ ) and the emerging area of Human-Building Interaction (e.g., https://www.intelligentenvironments.usc.edu/ ). These movements draw on and extend work of the interdisciplinary field of Environmental Psychology begun over 50 years ago (Canter and Craik 1981 ; Craik 1973 ). Progress toward universal design supporting the functions of built spaces can be seen in the example of the useful set of design guidelines for built environments put forward by the Low Vision Design Committee of the National Institute of Building Sciences (NIBS) in 2015 ( https://www.nibs.org/page/lvdc_guidelines ) and lighting guidelines put forward by the Illuminating Engineering Society (Barker et al. 2016 ). A number of the NIBS guidelines relate to the ideas of visual accessibility and the perception of local and global features for spatial behavior and could be informed by basic science approaches such as the methods described above. For example, the guidelines suggest avoiding patterns on flooring that could be mistaken for steps and placing ottomans or tables that are low or have transparent parts. The basic research described here establishes a scientific foundation for more general and future guidance in these directions.
Together, the body of work on perceiving local and global features in low vision contexts provides some initial insights and recommendations for architectural design that can enhance visual accessibility. These are summarized in Tables 1 and 2 . Beginning with the basic features supporting travel through spaces such as sidewalks, corridors, and stairways, research has identified challenges that could inform design. The “ramps and steps” work identified that enhancing the contrast at step transitions with directional lighting helped detection, but that providing high contrast texture on these surfaces hurt detection. The research also shows that while the subtle image cues of discontinuities in edge contours are very susceptible to changes in viewing conditions, cues that are less dependent on acuity facilitate perception of these environmental features. One good example is the cue of height in the picture plane for the identification of ramps, which was useful in blurred viewing conditions even at relatively shallow ramps. For perception of absolute scale that informs localization of these features, the visual horizon combined with eye height is readily used even in severely blurred viewing conditions. Low vision distance perception studies showed that even when viewers could just barely detect the presence of the object, they relied on vision of the floor-wall boundary to inform distance judgments. This finding is significant, as it suggests that if interior design is such that low contrast (or no contrast as in the black carpet and wall intersection in Fig. 6 ) impairs the perception of the floor-wall boundary, observers are likely to misperceive spatial locations and possibly room size as well. These examples along with empirical work emphasize the importance of high contrast at the floor-wall boundary . Research on objects as hazards supports some of the initial guidelines from the NIBS about visibility of features in terms of size and placement of environmental objects such as signs, poles, or furniture. For example, the visibility of object-ground contact matters. One study showed quantitatively that when viewers could no longer detect the object’s attachment to the ground, they perceived the object to be at a different location. Broadly for detection of objects, contrast matters for visibility with blurred vision, but more subtly, the contrast between object and background is dependent on lighting arrangement . Shape of environmental objects could also be considered, as curved objects were generally more visible than straight-edged objects under blur viewing conditions. Finally, an object’s angular size could be taken into account in the design of paths for pedestrians.
Basic research on perception of global features used to support spatial updating and spatial learning is in some ways consistent with the focus on local features summarized above. Those with simulated or actual low vision show relatively intact abilities to judge room size and update self-location after traversing simple paths within vista scale spaces, unless under extreme acuity/contrast sensitivity degradation. This is likely because of the ability to use salient wall-floor boundaries as well as non-visual body-based information for spatial updating . Blur does influence dynamic perception of distance traveled which may contribute to errors in learning of spatial layout while navigating. In environmental-scale navigation tasks, we have identified consistent effects of increased attentional demands for mobility associated with decreased accuracy for remembered locations. This occurs with both reduced acuity and contrast sensitivity and severely reduced peripheral field. While these are very different visual deficits, they both impact the automaticity of walking and show that designers should consider the associated cognitive factors that accompany the complex interaction of visual parameters . Navigating with visual impairment involves constant spatial problem solving (Giudice 2018 ) and associated increased anxiety about travel. The findings from the museum study (Barhorst-Cates et al. 2020 ) suggest that more complex environments and navigation paths may raise different issues in visual accessibility. Possibilities for reducing cognitive demands during travel might be to ensure unobstructed corridors and walkways and consider the impact of placement of highly visible landmarks and signs that could be used from a distance.
From a theoretical perspective, the research on global spatial features also suggests that non-visual spatial information can be used to solve navigation tasks (Giudice 2018 ). Loomis et al. ( 2013 ) propose an “amodal hypothesis” that accounts for functional equivalence, or similar behavioral performance in spatial tasks regardless of the sensory channels through which spatial information is conveyed. A body of research suggests that in many circumstances we act similarly in spaces that are conveyed by haptic stimuli, auditory stimuli, spatial language, or by vision. However, when designing for visual accessibility, it is important to consider the increased uncertainty that comes with reliance on degraded visual information that parallels what is known for use with non-visual information. For example, haptic perception can provide information about potential obstacles and distances, but only within the range that can be reached with the arm or long cane. Auditory perception provides cues for locations of objects at greater distances, but is less precise in specifying distance, direction, and self-motion (Giudice 2018 ). Similarly, low vision navigators with reduced acuity and/or contrast sensitivity also experience uncertainty in the available visual information and this uncertainty increases dramatically with the greater distances and complexity of spatial problem solving inherent in acting over larger-scale environments.
While the basic research has provided some support for the NIBS design recommendations for low vision, guidelines or intuitive practices can only take us so far toward the goal of visual accessibility. As noted throughout, there is variability in performance across spatial scenarios because of the difficulty in predicting the complex interaction between lighting conditions, environmental geometry, surface materials, and visual deficits. It is important to note that architects do not purposely design in ways that would exclude any population of users. Most often, if there are problematic spaces, it is reflective of lack of knowledge to the specific issues of those select populations. With the multitude of considerations that architects must integrate into the design (e.g., building program/function, structure, building systems, codes, zoning), moving to Universal Design through the consideration of low vision issues is a challenge.
Future directions for designing visually accessible spaces
Basic research in low vision perception identifies both capabilities and limitations associated with spatial cognition and navigation in visually restricted contexts. There are still many open questions as to the influence of type and severity of vision loss on the functional capabilities underlying independent travel. A future goal should be to test a wide range of low vision individuals on the types of paradigms that have been developed. This would serve to generalize beyond simulated low vision by varying the extent of visual impairment in ways that naturally occur with age or eye disease as well as account for the role of experience and strategies that people with low vision have. Notably, the “blur” created with restricted viewing goggles in many of the studies reduced acuity and contrast sensitivity together in ways that are not necessarily representative of specific forms of low vision. The simulations also independently limited acuity/contrast sensitivity or visual field loss, while many people with low vision experience both types of deficits together. Thus, there are clear benefits to expanding empirical work to include the diversity of low vision conditions in research on visual accessibility.
As we described earlier, the prevalence of low vision is growing worldwide, and the health and well-being of this population depends on the ability to have access to spaces in ways that promote independent travel. Future work in the design of visually accessible spaces must consider that visual impairment does not exist in isolation from other health problems. The prevalence of many eye diseases (e.g., age-related macular degeneration, glaucoma) is highly correlated with age, and there is evidence for comorbidities with cognitive impairments, hearing impairments, and depression (Whitson et al. 2011 ). Other comorbidities exist with physical disabilities such as the peripheral neuropathies associated with diabetes-related visual impairment (Tesfaye et al. 2010 ) or the increased likelihood of requiring a walker or wheelchair with age. Future directions of research should consider the diversity and individual differences inherent in a population with low vision.
There is potential in new assistive technologies that could supplement visually accessible design and facilitate the space perception and spatial cognition needed for safe and efficient navigation. However, the development of these technologies requires a human-centered design approach (O'Modhrain et al. 2015 ) that considers realistic scenarios and usability of visually impaired users—an approach that is not always typical of the designers (Giudice 2018 ). Furthermore, effective design of assistive technologies needs to be informed by an understanding of the perceptual and cognitive processes that underlie spatial representation and navigation (Giudice 2018 ; Loomis et al. 2012 ). For tasks that we define here as relying on global features, such as spatial updating and navigation along more complex routes, speech-enabled GPS-based navigation devices may be used to provide information about spatial layout, position, and orientation information. These systems currently work best outdoors, and assistive technology still needs to be developed for indoor wayfinding (Giudice 2018 ; Legge et al. 2013 ). An important consideration for the use of any type of assistive device is the additional cognitive processing required. As described in the spatial learning studies reviewed here, navigation with restricted viewing is inherently more cognitively demanding. The additional cognitive load required for use of an assistive technology could negate its positive effects. Future work is needed to understand the multisensory spatial information that is used in complex wayfinding and navigation tasks so that it can be conveyed and used effectively.
Availability of data and materials
Not applicable.
Acuity is the ability to detect fine-scale patterns and is often clinically measured in terms of LogMAR (Bailey-Lovie chart) which is the logarithm of the minimum angle of resolution (Bailey and Lovie-Kitchin 2013 ). A logMAR value of 0 indicates normal acuity (20/20 Snellen), and larger values correspond to lower acuity (logMAR 1.0 = 20/200 Snellen). In this paper, we report both logMAR and Snellen values. Increases in the denominator of the Snellen fraction correspond to decreases in acuity. The Pelli–Robson Contrast Sensitivity chart (Pelli et al. 1988 ) measures contrast sensitivity (the ability to see small changes in luminance) as the threshold lowest contrast for letter recognition of black/gray letters on a white background. A value of 2.0 is normal contrast sensitivity, and the value decreases with loss of contrast sensitivity. Field of view is the amount of the environment that is visible at one time and is described in terms of degrees of visual angle.
Abbreviations
Field of view
Bailey, I. L., & Lovie-Kitchin, J. E. (2013). Visual acuity testing. From the laboratory to the clinic. Vision Research, 90, 2–9.
Article PubMed Google Scholar
Barker, B., Brawley, B., Burnett, D., Cook, G., Crawford, D., Davies, L., et al. (2016). Lighting and the visual environment for seniors and the low vision population . New York: American National Standards Institute and Illuminating Engineering Society of North America.
Google Scholar
Barhorst-Cates, E. M., Rand, K. M., & Creem-Regehr, S. H. (2016). The effects of restricted peripheral field of view on spatial learning while navigating. PLoS ONE, 11 (10), e0163785.
Article PubMed PubMed Central Google Scholar
Barhorst-Cates, E. M., Rand, K. M., & Creem-Regehr, S. H. (2017). Let me be your guide: Physical guidance improves spatial learning for older adults with simulated low vision. Experimental Brain Research, 235 (11), 3307–3317.
Barhorst-Cates, E. M., Rand, K. M., & Creem-Regehr, S. H. (2019). Navigating with peripheral field loss in a museum: Learning impairments due to environmental complexity. Cognitive Research: Principles and Implications, 4 (1), 1–10.
Barhorst-Cates, E. M., Rand, K. M., & Creem-Regehr, S. H. (2020). Does active learning benefit spatial memory during navigation with restricted peripheral field? Attention, Perception, & Psychophysics, 82, 3033–3047.
Article Google Scholar
Bochsler, T. M., Legge, G. E., Gage, R., & Kallie, C. S. (2013). Recognition of ramps and steps by people with low vision. Investigative Ophthalmology & Visual Science, 54 (1), 288–294.
Bochsler, T. M., Legge, G. E., Kallie, C. S., & Gage, R. (2012). Seeing steps and ramps with simulated low acuity: Impact of texture and locomotion. Optometry and Vision Science, 89 (9), E1299.
Bourne, R. R., Flaxman, S. R., Braithwaite, T., Cicinelli, M. V., Das, A., Jonas, J. B., et al. (2017). Magnitude, temporal trends, and projections of the global prevalence of blindness and distance and near vision impairment: A systematic review and meta-analysis. The Lancet Global Health, 5 (9), e888–e897.
Canter, D. V., & Craik, K. H. (1981). Environmental psychology. Journal of Environmental Psychology, 1 (1), 1–11.
Chan, E., Baumann, O., Bellgrove, M. A., & Mattingley, J. B. (2012). From objects to landmarks: The function of visual location information in spatial navigation. Frontiers in Psychology, 3, 304.
PubMed PubMed Central Google Scholar
Chan, T., Friedman, D. S., Bradley, C., & Massof, R. (2018). Estimates of incidence and prevalence of visual impairment, low vision, and blindness in the United States. JAMA Ophthalmology, 136 (1), 12–19.
Chong, G. H., Brandt, R., & Martin, W. M. (2010). Design informed: Driving innovation with evidence-based design . New York: Wiley.
Chrastil, E. R., & Warren, W. H. (2012). Active and passive contributions to spatial learning. Psychonomic Bulletin & Review, 19 (1), 1–23.
Chrastil, E. R., & Warren, W. H. (2013). Active and passive spatial learning in human navigation: Acquisition of survey knowledge. Journal of Experimental Psychology: Learning, Memory, and Cognition, 39 (5), 1520.
PubMed Google Scholar
Chrastil, E. R., & Warren, W. H. (2015). Active and passive spatial learning in human navigation: Acquisition of graph knowledge. Journal of Experimental Psychology: Learning, Memory & Cognition, 41 (4), 1162–1178.
Craik, K. H. (1973). Environmental psychology. Annual Review of Psychology, 24 (1), 403–422.
Ekstrom, A. D. (2015). Why vision is important to how we navigate. Hippocampus, 25 (6), 731–735.
Ekstrom, A. D., & Isham, E. A. (2017). Human spatial navigation: Representations across dimensions and scales. Current Opinion in Behavioral Sciences, 17, 84–89.
Ekstrom, A. D., Spiers, H. J., Bohbot, V. D., & Rosenbaum, R. S. (2018). Human spatial navigation . Princeton: Princeton University Press.
Book Google Scholar
Epstein, R. A., & Vass, L. K. (2014). Neural systems for landmark-based wayfinding in humans. Philosophical Transactions of the Royal Society B, 369 (1635), 20120533.
Fortenbaugh, F. C., Hicks, J. C., Hao, L., & Turano, K. A. (2007). Losing sight of the bigger picture: Peripheral field loss compresses representations of space. Vision Research, 47, 2506–2520.
Fortenbaugh, F. C., Hicks, J. C., & Turano, K. A. (2008). The effect of peripheral visual field loss on representations of space: Evidence for distortion and adaptation. Investigative Ophthalmology & Visual Science, 49 (6), 2765–2772.
Gibson, J. J. (1950). The perception of the visual world . Boston: Houghton Mifflin.
Giudice, N. A. (2018). Navigating without vision: Principles of blind spatial cognition. In D. R. Montello (Ed.), Handbook of behavioral and cognitive geography (pp. 260–288). Cheltenham: Edward Elgar Publishing.
Chapter Google Scholar
Giudice, N. A., Marston, J. R., Klatzky, R. L., Loomis, J. M., & Golledge, R. G. (2008). Environmental learning without vision: Effects of cognitive load on interface design. In Paper presented at the 9th international conference on low vision, Montreal, Quebec, Canada .
Giudice, N. A., & Palani, H. P. (2014). Evaluation of non-visual panning operations using touch-screen devices. In Proceedings of the 16th international ACM SIGACCESS conference on computers & accessibility (ASSETS'14) (pp. 293–294).
Jansen, S. E., Toet, A., & Werkhoven, P. J. (2010). Obstacle crossing with lower visual field restriction: Shifts in strategy. Journal of Motor Behavior, 43 (1), 55–62.
Jansen, S. E., Toet, A., & Werkhoven, P. J. (2011). Human locomotion through a multiple obstacle environment: Strategy changes as a result of visual field limitation. Experimental Brain Research, 212 (3), 449–456.
Kallie, C. S., Legge, G. E., & Yu, D. (2012). Identification and detection of simple 3D objects with severely blurred vision. Investigative Ophthalmology & Visual Science, 53 (3), 7997–8005.
Kelly, J. W., McNamara, T. P., Bodenheimer, B., Carr, T. H., & Rieser, J. J. (2008). The shape of human navigation: How environmental geometry is used in the maintenance of spatial orientation. Cognition, 109, 281–286.
Kuyk, T., Elliott, J. L., & Fuhr, P. (1998). Visual correlates of mobility in real world settings in older adults with low vision. Optometry and Vision Science, 75 (7), 538–547.
Lappe, M., Jenkin, M., & Harris, L. R. (2007). Travel distance estimation from visual motion by leaky path integration. Experimental Brain Research, 180 (1), 35–48.
Legge, G. E., Beckmann, P. J., Tjan, B. S., Havey, G., Kramer, K., Rolkosky, D., et al. (2013). Indoor navigation by people with visual impairment using a digital sign system. PLoS ONE, 8 (10), e76783.
Legge, G. E., Gage, R., Baek, Y., & Bochsler, T. M. (2016a). Indoor spatial updating with reduced visual information. PLoS ONE, 11 (3), e0150708. https://doi.org/10.1371/journal.pone.0150708
Legge, G. E., Granquist, C., Baek, Y., & Gage, R. (2016b). Indoor spatial updating with impaired vision. Investigative Ophthalmology & Visual Science, 57 (15), 6757–6765. https://doi.org/10.1167/iovs.16-20226
Legge, G. E., Yu, D., Kallie, C. S., Bochsler, T. M., & Gage, R. (2010). Visual accessibility of ramps and steps. Journal of Vision, 10 (11), 8.
Li, K. Z., & Lindenberger, U. (2002). Relations between aging sensory/sensorimotor and cognitive functions. Neuroscience & Biobehavioral Reviews, 26 (7), 777–783.
Lindberg, E., & Gärling, T. (1982). Acquisition of locational information about reference points during locomotion: The role of central information processing. Scandinavian Journal of Psychology, 23 (1), 207–218.
Long, R. G., Rieser, J. J., & Hill, E. W. (1990). Mobility in individuals with moderate visual impairments. Journal of Visual Impairment & Blindness, 84, 111–118.
Loomis, J. L., Klatzky, R. L., & Giudice, N. A. (2012). Sensory substitution of vision: Importance of perceptual and cognitive processing. In R. Manduchi & S. Kurniawan (Eds.), Assistive technology for blindness and low vision (pp. 162–191). Boca Raton: CRC Press.
Loomis, J. M., Klatzky, R. L., & Giudice, N. A. (2013). Representing 3D space in working memory: Spatial images from vision, hearing, touch, and language. In S. Lacey & R. Lawson (Eds.), Multisensory imagery (pp. 131–155). Berlin: Springer.
Loomis, J. M., Klatzky, R. L., Golledge, R. G., Cicinelli, J. G., Pellegrino, J. W., & Fry, P. A. (1993). Nonvisual navigation by blind and sighted: Assessment of path integration ability. Journal of Experimental Psychology: General, 122 (1), 73–91.
Ludt, R., & Goodrich, G. L. (2002). Change in visual perception detection distances for low vision travelers as a result of dynamic visual assessment and training. Journal of Visual Impairment, 96 (1), 7–21.
Mace, R. (1985). Universal design: Barrier free environments for everyone. Designers West, 33 (1), 147–152.
Marchette, S. A., Vass, L. K., Ryan, J., & Epstein, R. A. (2014). Anchoring the neural compass: Coding of local spatial reference frames in human medial parietal lobe. Nature Neuroscience, 17 (11), 1598–1606.
Marron, J. A., & Bailey, I. L. (1982). Visual factors and orientation-mobility performance. Journal of Optometry and Physiological Optics, 59 (5), 413–426.
Marston, J. R., & Golledge, R. G. (2003). The hidden demand for participation in activities and travel by persons who are visually impaired. Journal of Visual Impairment & Blindness, 97 (8), 475–488.
Mittelstaedt, M.-L., & Mittelstaedt, H. (2001). Idiothetic navigation in humans: Estimation of path length. Experimental Brain Research, 139 (3), 318–332.
Montello, D. R. (1993). Scale and multiple psychologies of space. In Spatial information theory: A theoretical basis for GIS. Proceedings of COSIT '93. Lecture notes in computer science (vol. 716, pp. 312–321).
Mou, W., & McNamara, T. P. (2002). Intrinsic frames of reference in spatial memory. Journal of Experimental Psychology: Learning, Memory, and Cognition, 28, 162–170.
Nyman, S. R., Gosney, M. A., & Victor, C. R. (2010). Psychosocial impact of visual impairment in working-age adults. British Journal of Opthalmology, 94, 1427–1431.
O’Modhrain, S., Giudice, N. A., Gardner, J. A., & Legge, G. E. (2015). Designing media for visually impaired users of refreshable touch displays: Possibilities and pitfalls. IEEE Transactions on Haptics, 8 (3), 248–257.
Pelli, D. G. (1987). The visual requirements of mobility. In G. C. Woo (Ed.), Low vision: Principles and applications (pp. 134–146). Berlin: Springer.
Pelli, D. G., Robson, J. G., & Wilkins, A. J. (1988). The design of a new letter chart for measuring contrast sensitivity. Clinical Vision Sciences, 2, 187–199.
Pigeon, C., & Marin-Lamellet, C. (2015). Evaluation of the attentional capacities and working memory of early and late blind persons. Acta Psychologica, 155, 1–7.
Rand, K. M., Barhorst-Cates, E. M., Kiris, E., Thompson, W. B., & Creem-Regehr, S. H. (2019). Going the distance and beyond: simulated low vision increases perception of distance traveled during locomotion. Psychological Research Psychologische Forschung, 83 (7), 1349–1362.
Rand, K. M., Creem-Regehr, S. H., & Thompson, W. B. (2015). Spatial learning while navigating with severely degraded viewing: The role of attention and mobility monitoring. Journal of Experimental Psychology: Human Perception & Performance, 41 (3), 649–664.
Rand, K. M., Tarampi, M. R., Creem-Regehr, S. H., & Thompson, W. B. (2011). The importance of a visual horizon for distance judgments under severely degraded vision. Perception , 40 (2), 143–154.
Rand, K. M., Tarampi, M. R., Creem-Regehr, S. H., & Thompson, W. B. (2012). The influence of ground contact and visible horizon on perception of distance and size under severely degraded vision. Seeing and Perceiving, 25 (5), 425–447.
Rieser, J. J. (1989). Access to knowledge of spatial structure at novel points of observation. Journal of Experimental Psychology: Learning, Memory, and Cognition, 15 (6), 1157–1165.
Rieser, J. J., Hill, E. W., Talor, C. R., Bradfield, A., & Rosen, S. (1992). Visual experience, visual field size, and the development of nonvisual sensitivity to the spatial structure of outdoor neighborhoods explored by walking. Journal of Experimental Psychology: General, 121 (2), 210–221.
Saydah, S. H., Gerzoff, R. B., Saaddine, J. B., Zhang, X., & Cotch, M. F. (2020). Eye care among US adults at high risk for vision loss in the United States in 2002 and 2017. JAMA Ophthalmology, 138, 479–489.
Sedgwick, H. A. (1983). Environment-centered representation of spatial layout: Available information from texture and perspective. In J. Beck, B. Hope, & A. Rosenfeld (Eds.), Human and machine vision (pp. 425–458). New York: Academic Press.
Steinfeld, E., & Maisel, J. (2012). Universal design: Creating inclusive environments . New York: Wiley.
Sturz, B. R., Kilday, Z. A., & Bodily, K. D. (2013). Does constraining field of view prevent extraction of geometric cues for humans during virtual-environment reorientation? Journal of Experimental Psychology: Animal Behavior Processes, 39 (4), 390–396.
Tarampi, M. R., Creem-Regehr, S. H., & Thompson, W. B. (2010). Intact spatial updating with severely degraded vision. Attention, Perception, & Psychophysics, 72 (1), 23–27.
Tesfaye, S., Boulton, A. J., Dyck, P. J., Freeman, R., Horowitz, M., Kempler, P., et al. (2010). Diabetic neuropathies: Update on definitions, diagnostic criteria, estimation of severity, and treatments. Diabetes Care, 33 (10), 2285–2293.
Thompson, W. B., Legge, G. E., Kersten, D. J., Shakespeare, R. A., & Lei, Q. (2017). Simulating visibility under reduced acuity and contrast sensitivity. Journal of the Optical Society of America A. Optics and Image Science, 34 (4), 583–593.
Turano, K. A., Broman, A. T., Bandeen-Roche, K., Munoz, B., Rubin, G. S., & West, S. K. (2004). Association of visual field loss and mobility performance in older adults: Salisbury Eye Evaluation Study. Optometry & Vision Science, 81 (5), 298–307.
Whitson, H. E., Ansah, D., Sanders, L. L., Whitaker, D., Potter, G. G., Cousins, S. W., et al. (2011). Comorbid cognitive impairment and functional trajectories in low vision rehabilitation for macular disease. Aging Clinical and Experimental Research, 23 (5–6), 343–350.
Wolbers, T., & Wiener, J. M. (2014). Challenges for identifying the neural mechanisms that support spatial navigation: the impact of spatial scale. Frontiers in Human Neuroscience, 8, 571.
Yamamoto, N., & Philbeck, J. W. (2013). Peripheral vision benefits spatial learning by guiding eye movements. Memory & Cognition, 41 (1), 109–121.
Zhao, M., & Warren, W. H. (2015). How you get there from here: Interaction of visual landmarks and path integration in human navigation. Psychological Science, 26 (6), 915–924.
Download references
Acknowledgements
We thank William B. Thompson for providing several figures, Eric Egenolf for discussions on national and international building codes, and Caitlyn Barhorst for discussions on design implications.
This research was supported by the National Eye Institute of the National Institutes of Health under Award Number R01EY017835. The content is solely the responsibility of the authors and does not necessarily represent the official views of the National Institutes of Health. The funders had no role in study design, data collection and analysis, decision to publish, or preparation of the manuscript.
Author information
Authors and affiliations.
Department of Psychology, University of Utah, Salt Lake City, UT, USA
Sarah H. Creem-Regehr & Kristina M. Rand
Moss Rehabilitation Research Institute, Elkins Park, PA, USA
Erica M. Barhorst-Cates
Department of Psychology, University of Hartford, West Hartford, CT, USA
Margaret R. Tarampi
Department of Psychology, University of Minnesota, Minneapolis, MN, USA
Gordon E. Legge
You can also search for this author in PubMed Google Scholar
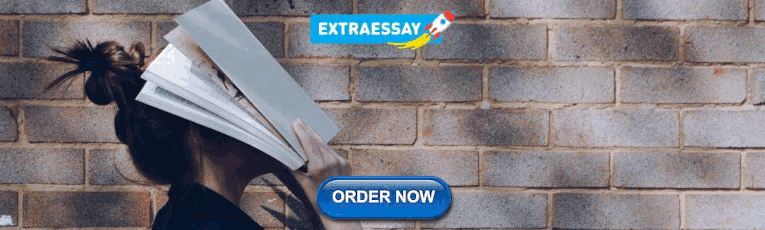
Contributions
SC, EB, MT, KR, and GL wrote and edited the manuscript. All authors read and approved the final manuscript.
Corresponding author
Correspondence to Sarah H. Creem-Regehr .
Ethics declarations
Ethics approval and consent to participate, consent for publication, competing interests.
The authors declare that they have no competing interests.
Additional information
Publisher's note.
Springer Nature remains neutral with regard to jurisdictional claims in published maps and institutional affiliations.
Rights and permissions
Open Access This article is licensed under a Creative Commons Attribution 4.0 International License, which permits use, sharing, adaptation, distribution and reproduction in any medium or format, as long as you give appropriate credit to the original author(s) and the source, provide a link to the Creative Commons licence, and indicate if changes were made. The images or other third party material in this article are included in the article's Creative Commons licence, unless indicated otherwise in a credit line to the material. If material is not included in the article's Creative Commons licence and your intended use is not permitted by statutory regulation or exceeds the permitted use, you will need to obtain permission directly from the copyright holder. To view a copy of this licence, visit http://creativecommons.org/licenses/by/4.0/ .
Reprints and permissions
About this article
Cite this article.
Creem-Regehr, S.H., Barhorst-Cates, E.M., Tarampi, M.R. et al. How can basic research on spatial cognition enhance the visual accessibility of architecture for people with low vision?. Cogn. Research 6 , 3 (2021). https://doi.org/10.1186/s41235-020-00265-y
Download citation
Received : 25 June 2020
Accepted : 19 November 2020
Published : 07 January 2021
DOI : https://doi.org/10.1186/s41235-020-00265-y
Share this article
Anyone you share the following link with will be able to read this content:
Sorry, a shareable link is not currently available for this article.
Provided by the Springer Nature SharedIt content-sharing initiative
- Space perception
- Spatial cognition
- Bibliography
- More Referencing guides Blog Automated transliteration Relevant bibliographies by topics
- Automated transliteration
- Relevant bibliographies by topics
- Referencing guides
Machine Learning: Algorithms, Real-World Applications and Research Directions
- Review Article
- Published: 22 March 2021
- Volume 2 , article number 160 , ( 2021 )
Cite this article
- Iqbal H. Sarker ORCID: orcid.org/0000-0003-1740-5517 1 , 2
510k Accesses
1445 Citations
23 Altmetric
Explore all metrics
In the current age of the Fourth Industrial Revolution (4 IR or Industry 4.0), the digital world has a wealth of data, such as Internet of Things (IoT) data, cybersecurity data, mobile data, business data, social media data, health data, etc. To intelligently analyze these data and develop the corresponding smart and automated applications, the knowledge of artificial intelligence (AI), particularly, machine learning (ML) is the key. Various types of machine learning algorithms such as supervised, unsupervised, semi-supervised, and reinforcement learning exist in the area. Besides, the deep learning , which is part of a broader family of machine learning methods, can intelligently analyze the data on a large scale. In this paper, we present a comprehensive view on these machine learning algorithms that can be applied to enhance the intelligence and the capabilities of an application. Thus, this study’s key contribution is explaining the principles of different machine learning techniques and their applicability in various real-world application domains, such as cybersecurity systems, smart cities, healthcare, e-commerce, agriculture, and many more. We also highlight the challenges and potential research directions based on our study. Overall, this paper aims to serve as a reference point for both academia and industry professionals as well as for decision-makers in various real-world situations and application areas, particularly from the technical point of view.
Similar content being viewed by others
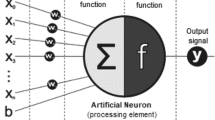
Deep Learning: A Comprehensive Overview on Techniques, Taxonomy, Applications and Research Directions
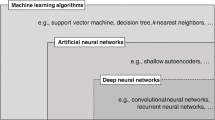
Machine learning and deep learning
What Is Machine Learning?
Avoid common mistakes on your manuscript.
Introduction
We live in the age of data, where everything around us is connected to a data source, and everything in our lives is digitally recorded [ 21 , 103 ]. For instance, the current electronic world has a wealth of various kinds of data, such as the Internet of Things (IoT) data, cybersecurity data, smart city data, business data, smartphone data, social media data, health data, COVID-19 data, and many more. The data can be structured, semi-structured, or unstructured, discussed briefly in Sect. “ Types of Real-World Data and Machine Learning Techniques ”, which is increasing day-by-day. Extracting insights from these data can be used to build various intelligent applications in the relevant domains. For instance, to build a data-driven automated and intelligent cybersecurity system, the relevant cybersecurity data can be used [ 105 ]; to build personalized context-aware smart mobile applications, the relevant mobile data can be used [ 103 ], and so on. Thus, the data management tools and techniques having the capability of extracting insights or useful knowledge from the data in a timely and intelligent way is urgently needed, on which the real-world applications are based.
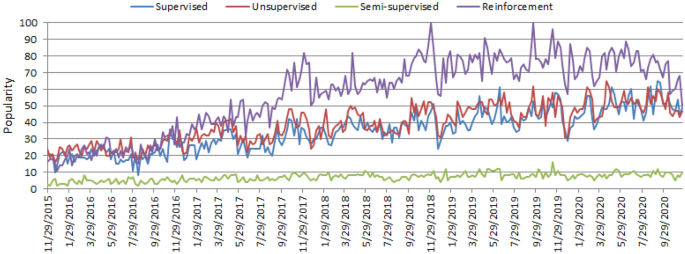
The worldwide popularity score of various types of ML algorithms (supervised, unsupervised, semi-supervised, and reinforcement) in a range of 0 (min) to 100 (max) over time where x-axis represents the timestamp information and y-axis represents the corresponding score
Artificial intelligence (AI), particularly, machine learning (ML) have grown rapidly in recent years in the context of data analysis and computing that typically allows the applications to function in an intelligent manner [ 95 ]. ML usually provides systems with the ability to learn and enhance from experience automatically without being specifically programmed and is generally referred to as the most popular latest technologies in the fourth industrial revolution (4 IR or Industry 4.0) [ 103 , 105 ]. “Industry 4.0” [ 114 ] is typically the ongoing automation of conventional manufacturing and industrial practices, including exploratory data processing, using new smart technologies such as machine learning automation. Thus, to intelligently analyze these data and to develop the corresponding real-world applications, machine learning algorithms is the key. The learning algorithms can be categorized into four major types, such as supervised, unsupervised, semi-supervised, and reinforcement learning in the area [ 75 ], discussed briefly in Sect. “ Types of Real-World Data and Machine Learning Techniques ”. The popularity of these approaches to learning is increasing day-by-day, which is shown in Fig. 1 , based on data collected from Google Trends [ 4 ] over the last five years. The x - axis of the figure indicates the specific dates and the corresponding popularity score within the range of \(0 \; (minimum)\) to \(100 \; (maximum)\) has been shown in y - axis . According to Fig. 1 , the popularity indication values for these learning types are low in 2015 and are increasing day by day. These statistics motivate us to study on machine learning in this paper, which can play an important role in the real-world through Industry 4.0 automation.
In general, the effectiveness and the efficiency of a machine learning solution depend on the nature and characteristics of data and the performance of the learning algorithms . In the area of machine learning algorithms, classification analysis, regression, data clustering, feature engineering and dimensionality reduction, association rule learning, or reinforcement learning techniques exist to effectively build data-driven systems [ 41 , 125 ]. Besides, deep learning originated from the artificial neural network that can be used to intelligently analyze data, which is known as part of a wider family of machine learning approaches [ 96 ]. Thus, selecting a proper learning algorithm that is suitable for the target application in a particular domain is challenging. The reason is that the purpose of different learning algorithms is different, even the outcome of different learning algorithms in a similar category may vary depending on the data characteristics [ 106 ]. Thus, it is important to understand the principles of various machine learning algorithms and their applicability to apply in various real-world application areas, such as IoT systems, cybersecurity services, business and recommendation systems, smart cities, healthcare and COVID-19, context-aware systems, sustainable agriculture, and many more that are explained briefly in Sect. “ Applications of Machine Learning ”.
Based on the importance and potentiality of “Machine Learning” to analyze the data mentioned above, in this paper, we provide a comprehensive view on various types of machine learning algorithms that can be applied to enhance the intelligence and the capabilities of an application. Thus, the key contribution of this study is explaining the principles and potentiality of different machine learning techniques, and their applicability in various real-world application areas mentioned earlier. The purpose of this paper is, therefore, to provide a basic guide for those academia and industry people who want to study, research, and develop data-driven automated and intelligent systems in the relevant areas based on machine learning techniques.
The key contributions of this paper are listed as follows:
To define the scope of our study by taking into account the nature and characteristics of various types of real-world data and the capabilities of various learning techniques.
To provide a comprehensive view on machine learning algorithms that can be applied to enhance the intelligence and capabilities of a data-driven application.
To discuss the applicability of machine learning-based solutions in various real-world application domains.
To highlight and summarize the potential research directions within the scope of our study for intelligent data analysis and services.
The rest of the paper is organized as follows. The next section presents the types of data and machine learning algorithms in a broader sense and defines the scope of our study. We briefly discuss and explain different machine learning algorithms in the subsequent section followed by which various real-world application areas based on machine learning algorithms are discussed and summarized. In the penultimate section, we highlight several research issues and potential future directions, and the final section concludes this paper.
Types of Real-World Data and Machine Learning Techniques
Machine learning algorithms typically consume and process data to learn the related patterns about individuals, business processes, transactions, events, and so on. In the following, we discuss various types of real-world data as well as categories of machine learning algorithms.
Types of Real-World Data
Usually, the availability of data is considered as the key to construct a machine learning model or data-driven real-world systems [ 103 , 105 ]. Data can be of various forms, such as structured, semi-structured, or unstructured [ 41 , 72 ]. Besides, the “metadata” is another type that typically represents data about the data. In the following, we briefly discuss these types of data.
Structured: It has a well-defined structure, conforms to a data model following a standard order, which is highly organized and easily accessed, and used by an entity or a computer program. In well-defined schemes, such as relational databases, structured data are typically stored, i.e., in a tabular format. For instance, names, dates, addresses, credit card numbers, stock information, geolocation, etc. are examples of structured data.
Unstructured: On the other hand, there is no pre-defined format or organization for unstructured data, making it much more difficult to capture, process, and analyze, mostly containing text and multimedia material. For example, sensor data, emails, blog entries, wikis, and word processing documents, PDF files, audio files, videos, images, presentations, web pages, and many other types of business documents can be considered as unstructured data.
Semi-structured: Semi-structured data are not stored in a relational database like the structured data mentioned above, but it does have certain organizational properties that make it easier to analyze. HTML, XML, JSON documents, NoSQL databases, etc., are some examples of semi-structured data.
Metadata: It is not the normal form of data, but “data about data”. The primary difference between “data” and “metadata” is that data are simply the material that can classify, measure, or even document something relative to an organization’s data properties. On the other hand, metadata describes the relevant data information, giving it more significance for data users. A basic example of a document’s metadata might be the author, file size, date generated by the document, keywords to define the document, etc.
In the area of machine learning and data science, researchers use various widely used datasets for different purposes. These are, for example, cybersecurity datasets such as NSL-KDD [ 119 ], UNSW-NB15 [ 76 ], ISCX’12 [ 1 ], CIC-DDoS2019 [ 2 ], Bot-IoT [ 59 ], etc., smartphone datasets such as phone call logs [ 84 , 101 ], SMS Log [ 29 ], mobile application usages logs [ 137 ] [ 117 ], mobile phone notification logs [ 73 ] etc., IoT data [ 16 , 57 , 62 ], agriculture and e-commerce data [ 120 , 138 ], health data such as heart disease [ 92 ], diabetes mellitus [ 83 , 134 ], COVID-19 [ 43 , 74 ], etc., and many more in various application domains. The data can be in different types discussed above, which may vary from application to application in the real world. To analyze such data in a particular problem domain, and to extract the insights or useful knowledge from the data for building the real-world intelligent applications, different types of machine learning techniques can be used according to their learning capabilities, which is discussed in the following.
Types of Machine Learning Techniques
Machine Learning algorithms are mainly divided into four categories: Supervised learning, Unsupervised learning, Semi-supervised learning, and Reinforcement learning [ 75 ], as shown in Fig. 2 . In the following, we briefly discuss each type of learning technique with the scope of their applicability to solve real-world problems.
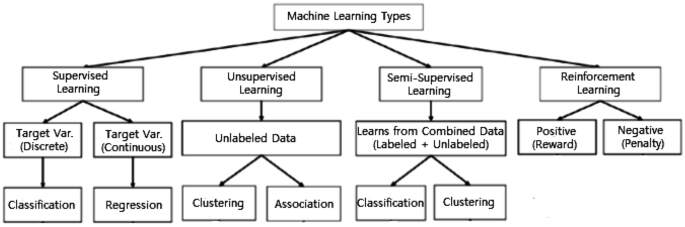
Various types of machine learning techniques
Supervised: Supervised learning is typically the task of machine learning to learn a function that maps an input to an output based on sample input-output pairs [ 41 ]. It uses labeled training data and a collection of training examples to infer a function. Supervised learning is carried out when certain goals are identified to be accomplished from a certain set of inputs [ 105 ], i.e., a task-driven approach . The most common supervised tasks are “classification” that separates the data, and “regression” that fits the data. For instance, predicting the class label or sentiment of a piece of text, like a tweet or a product review, i.e., text classification, is an example of supervised learning.
Unsupervised: Unsupervised learning analyzes unlabeled datasets without the need for human interference, i.e., a data-driven process [ 41 ]. This is widely used for extracting generative features, identifying meaningful trends and structures, groupings in results, and exploratory purposes. The most common unsupervised learning tasks are clustering, density estimation, feature learning, dimensionality reduction, finding association rules, anomaly detection, etc.
Semi-supervised: Semi-supervised learning can be defined as a hybridization of the above-mentioned supervised and unsupervised methods, as it operates on both labeled and unlabeled data [ 41 , 105 ]. Thus, it falls between learning “without supervision” and learning “with supervision”. In the real world, labeled data could be rare in several contexts, and unlabeled data are numerous, where semi-supervised learning is useful [ 75 ]. The ultimate goal of a semi-supervised learning model is to provide a better outcome for prediction than that produced using the labeled data alone from the model. Some application areas where semi-supervised learning is used include machine translation, fraud detection, labeling data and text classification.
Reinforcement: Reinforcement learning is a type of machine learning algorithm that enables software agents and machines to automatically evaluate the optimal behavior in a particular context or environment to improve its efficiency [ 52 ], i.e., an environment-driven approach . This type of learning is based on reward or penalty, and its ultimate goal is to use insights obtained from environmental activists to take action to increase the reward or minimize the risk [ 75 ]. It is a powerful tool for training AI models that can help increase automation or optimize the operational efficiency of sophisticated systems such as robotics, autonomous driving tasks, manufacturing and supply chain logistics, however, not preferable to use it for solving the basic or straightforward problems.
Thus, to build effective models in various application areas different types of machine learning techniques can play a significant role according to their learning capabilities, depending on the nature of the data discussed earlier, and the target outcome. In Table 1 , we summarize various types of machine learning techniques with examples. In the following, we provide a comprehensive view of machine learning algorithms that can be applied to enhance the intelligence and capabilities of a data-driven application.
Machine Learning Tasks and Algorithms
In this section, we discuss various machine learning algorithms that include classification analysis, regression analysis, data clustering, association rule learning, feature engineering for dimensionality reduction, as well as deep learning methods. A general structure of a machine learning-based predictive model has been shown in Fig. 3 , where the model is trained from historical data in phase 1 and the outcome is generated in phase 2 for the new test data.
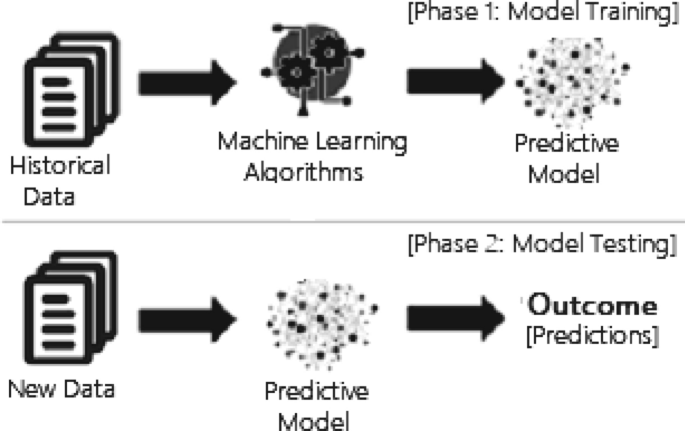
A general structure of a machine learning based predictive model considering both the training and testing phase
Classification Analysis
Classification is regarded as a supervised learning method in machine learning, referring to a problem of predictive modeling as well, where a class label is predicted for a given example [ 41 ]. Mathematically, it maps a function ( f ) from input variables ( X ) to output variables ( Y ) as target, label or categories. To predict the class of given data points, it can be carried out on structured or unstructured data. For example, spam detection such as “spam” and “not spam” in email service providers can be a classification problem. In the following, we summarize the common classification problems.
Binary classification: It refers to the classification tasks having two class labels such as “true and false” or “yes and no” [ 41 ]. In such binary classification tasks, one class could be the normal state, while the abnormal state could be another class. For instance, “cancer not detected” is the normal state of a task that involves a medical test, and “cancer detected” could be considered as the abnormal state. Similarly, “spam” and “not spam” in the above example of email service providers are considered as binary classification.
Multiclass classification: Traditionally, this refers to those classification tasks having more than two class labels [ 41 ]. The multiclass classification does not have the principle of normal and abnormal outcomes, unlike binary classification tasks. Instead, within a range of specified classes, examples are classified as belonging to one. For example, it can be a multiclass classification task to classify various types of network attacks in the NSL-KDD [ 119 ] dataset, where the attack categories are classified into four class labels, such as DoS (Denial of Service Attack), U2R (User to Root Attack), R2L (Root to Local Attack), and Probing Attack.
Multi-label classification: In machine learning, multi-label classification is an important consideration where an example is associated with several classes or labels. Thus, it is a generalization of multiclass classification, where the classes involved in the problem are hierarchically structured, and each example may simultaneously belong to more than one class in each hierarchical level, e.g., multi-level text classification. For instance, Google news can be presented under the categories of a “city name”, “technology”, or “latest news”, etc. Multi-label classification includes advanced machine learning algorithms that support predicting various mutually non-exclusive classes or labels, unlike traditional classification tasks where class labels are mutually exclusive [ 82 ].
Many classification algorithms have been proposed in the machine learning and data science literature [ 41 , 125 ]. In the following, we summarize the most common and popular methods that are used widely in various application areas.
Naive Bayes (NB): The naive Bayes algorithm is based on the Bayes’ theorem with the assumption of independence between each pair of features [ 51 ]. It works well and can be used for both binary and multi-class categories in many real-world situations, such as document or text classification, spam filtering, etc. To effectively classify the noisy instances in the data and to construct a robust prediction model, the NB classifier can be used [ 94 ]. The key benefit is that, compared to more sophisticated approaches, it needs a small amount of training data to estimate the necessary parameters and quickly [ 82 ]. However, its performance may affect due to its strong assumptions on features independence. Gaussian, Multinomial, Complement, Bernoulli, and Categorical are the common variants of NB classifier [ 82 ].
Linear Discriminant Analysis (LDA): Linear Discriminant Analysis (LDA) is a linear decision boundary classifier created by fitting class conditional densities to data and applying Bayes’ rule [ 51 , 82 ]. This method is also known as a generalization of Fisher’s linear discriminant, which projects a given dataset into a lower-dimensional space, i.e., a reduction of dimensionality that minimizes the complexity of the model or reduces the resulting model’s computational costs. The standard LDA model usually suits each class with a Gaussian density, assuming that all classes share the same covariance matrix [ 82 ]. LDA is closely related to ANOVA (analysis of variance) and regression analysis, which seek to express one dependent variable as a linear combination of other features or measurements.
Logistic regression (LR): Another common probabilistic based statistical model used to solve classification issues in machine learning is Logistic Regression (LR) [ 64 ]. Logistic regression typically uses a logistic function to estimate the probabilities, which is also referred to as the mathematically defined sigmoid function in Eq. 1 . It can overfit high-dimensional datasets and works well when the dataset can be separated linearly. The regularization (L1 and L2) techniques [ 82 ] can be used to avoid over-fitting in such scenarios. The assumption of linearity between the dependent and independent variables is considered as a major drawback of Logistic Regression. It can be used for both classification and regression problems, but it is more commonly used for classification.
K-nearest neighbors (KNN): K-Nearest Neighbors (KNN) [ 9 ] is an “instance-based learning” or non-generalizing learning, also known as a “lazy learning” algorithm. It does not focus on constructing a general internal model; instead, it stores all instances corresponding to training data in n -dimensional space. KNN uses data and classifies new data points based on similarity measures (e.g., Euclidean distance function) [ 82 ]. Classification is computed from a simple majority vote of the k nearest neighbors of each point. It is quite robust to noisy training data, and accuracy depends on the data quality. The biggest issue with KNN is to choose the optimal number of neighbors to be considered. KNN can be used both for classification as well as regression.
Support vector machine (SVM): In machine learning, another common technique that can be used for classification, regression, or other tasks is a support vector machine (SVM) [ 56 ]. In high- or infinite-dimensional space, a support vector machine constructs a hyper-plane or set of hyper-planes. Intuitively, the hyper-plane, which has the greatest distance from the nearest training data points in any class, achieves a strong separation since, in general, the greater the margin, the lower the classifier’s generalization error. It is effective in high-dimensional spaces and can behave differently based on different mathematical functions known as the kernel. Linear, polynomial, radial basis function (RBF), sigmoid, etc., are the popular kernel functions used in SVM classifier [ 82 ]. However, when the data set contains more noise, such as overlapping target classes, SVM does not perform well.
Decision tree (DT): Decision tree (DT) [ 88 ] is a well-known non-parametric supervised learning method. DT learning methods are used for both the classification and regression tasks [ 82 ]. ID3 [ 87 ], C4.5 [ 88 ], and CART [ 20 ] are well known for DT algorithms. Moreover, recently proposed BehavDT [ 100 ], and IntrudTree [ 97 ] by Sarker et al. are effective in the relevant application domains, such as user behavior analytics and cybersecurity analytics, respectively. By sorting down the tree from the root to some leaf nodes, as shown in Fig. 4 , DT classifies the instances. Instances are classified by checking the attribute defined by that node, starting at the root node of the tree, and then moving down the tree branch corresponding to the attribute value. For splitting, the most popular criteria are “gini” for the Gini impurity and “entropy” for the information gain that can be expressed mathematically as [ 82 ].
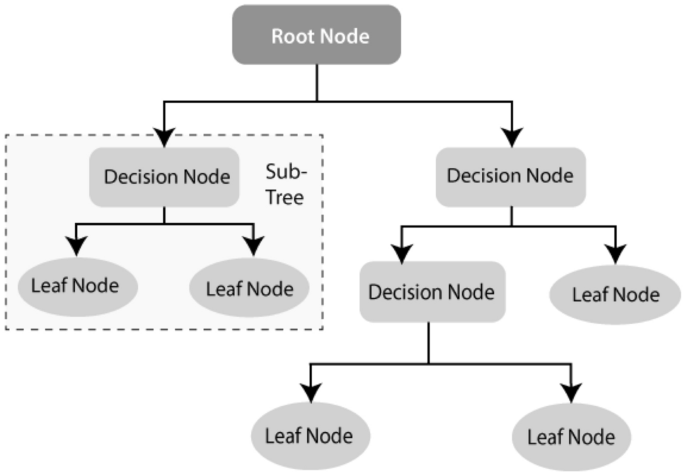
An example of a decision tree structure
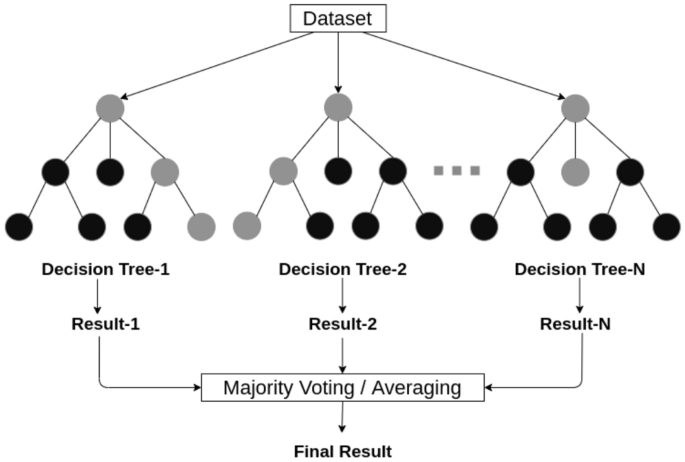
An example of a random forest structure considering multiple decision trees
Random forest (RF): A random forest classifier [ 19 ] is well known as an ensemble classification technique that is used in the field of machine learning and data science in various application areas. This method uses “parallel ensembling” which fits several decision tree classifiers in parallel, as shown in Fig. 5 , on different data set sub-samples and uses majority voting or averages for the outcome or final result. It thus minimizes the over-fitting problem and increases the prediction accuracy and control [ 82 ]. Therefore, the RF learning model with multiple decision trees is typically more accurate than a single decision tree based model [ 106 ]. To build a series of decision trees with controlled variation, it combines bootstrap aggregation (bagging) [ 18 ] and random feature selection [ 11 ]. It is adaptable to both classification and regression problems and fits well for both categorical and continuous values.
Adaptive Boosting (AdaBoost): Adaptive Boosting (AdaBoost) is an ensemble learning process that employs an iterative approach to improve poor classifiers by learning from their errors. This is developed by Yoav Freund et al. [ 35 ] and also known as “meta-learning”. Unlike the random forest that uses parallel ensembling, Adaboost uses “sequential ensembling”. It creates a powerful classifier by combining many poorly performing classifiers to obtain a good classifier of high accuracy. In that sense, AdaBoost is called an adaptive classifier by significantly improving the efficiency of the classifier, but in some instances, it can trigger overfits. AdaBoost is best used to boost the performance of decision trees, base estimator [ 82 ], on binary classification problems, however, is sensitive to noisy data and outliers.
Extreme gradient boosting (XGBoost): Gradient Boosting, like Random Forests [ 19 ] above, is an ensemble learning algorithm that generates a final model based on a series of individual models, typically decision trees. The gradient is used to minimize the loss function, similar to how neural networks [ 41 ] use gradient descent to optimize weights. Extreme Gradient Boosting (XGBoost) is a form of gradient boosting that takes more detailed approximations into account when determining the best model [ 82 ]. It computes second-order gradients of the loss function to minimize loss and advanced regularization (L1 and L2) [ 82 ], which reduces over-fitting, and improves model generalization and performance. XGBoost is fast to interpret and can handle large-sized datasets well.
Stochastic gradient descent (SGD): Stochastic gradient descent (SGD) [ 41 ] is an iterative method for optimizing an objective function with appropriate smoothness properties, where the word ‘stochastic’ refers to random probability. This reduces the computational burden, particularly in high-dimensional optimization problems, allowing for faster iterations in exchange for a lower convergence rate. A gradient is the slope of a function that calculates a variable’s degree of change in response to another variable’s changes. Mathematically, the Gradient Descent is a convex function whose output is a partial derivative of a set of its input parameters. Let, \(\alpha\) is the learning rate, and \(J_i\) is the training example cost of \(i \mathrm{th}\) , then Eq. ( 4 ) represents the stochastic gradient descent weight update method at the \(j^\mathrm{th}\) iteration. In large-scale and sparse machine learning, SGD has been successfully applied to problems often encountered in text classification and natural language processing [ 82 ]. However, SGD is sensitive to feature scaling and needs a range of hyperparameters, such as the regularization parameter and the number of iterations.
Rule-based classification : The term rule-based classification can be used to refer to any classification scheme that makes use of IF-THEN rules for class prediction. Several classification algorithms such as Zero-R [ 125 ], One-R [ 47 ], decision trees [ 87 , 88 ], DTNB [ 110 ], Ripple Down Rule learner (RIDOR) [ 125 ], Repeated Incremental Pruning to Produce Error Reduction (RIPPER) [ 126 ] exist with the ability of rule generation. The decision tree is one of the most common rule-based classification algorithms among these techniques because it has several advantages, such as being easier to interpret; the ability to handle high-dimensional data; simplicity and speed; good accuracy; and the capability to produce rules for human clear and understandable classification [ 127 ] [ 128 ]. The decision tree-based rules also provide significant accuracy in a prediction model for unseen test cases [ 106 ]. Since the rules are easily interpretable, these rule-based classifiers are often used to produce descriptive models that can describe a system including the entities and their relationships.
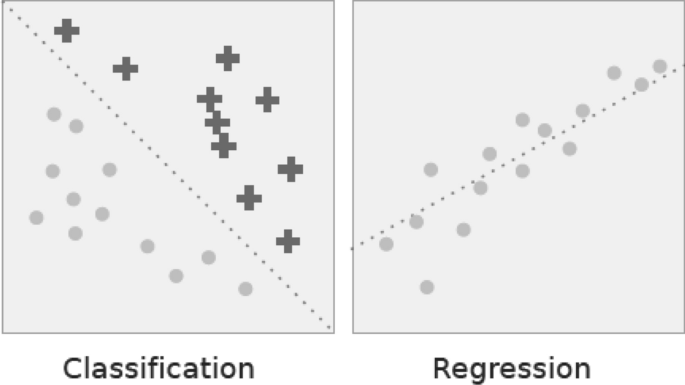
Classification vs. regression. In classification the dotted line represents a linear boundary that separates the two classes; in regression, the dotted line models the linear relationship between the two variables
Regression Analysis
Regression analysis includes several methods of machine learning that allow to predict a continuous ( y ) result variable based on the value of one or more ( x ) predictor variables [ 41 ]. The most significant distinction between classification and regression is that classification predicts distinct class labels, while regression facilitates the prediction of a continuous quantity. Figure 6 shows an example of how classification is different with regression models. Some overlaps are often found between the two types of machine learning algorithms. Regression models are now widely used in a variety of fields, including financial forecasting or prediction, cost estimation, trend analysis, marketing, time series estimation, drug response modeling, and many more. Some of the familiar types of regression algorithms are linear, polynomial, lasso and ridge regression, etc., which are explained briefly in the following.
Simple and multiple linear regression: This is one of the most popular ML modeling techniques as well as a well-known regression technique. In this technique, the dependent variable is continuous, the independent variable(s) can be continuous or discrete, and the form of the regression line is linear. Linear regression creates a relationship between the dependent variable ( Y ) and one or more independent variables ( X ) (also known as regression line) using the best fit straight line [ 41 ]. It is defined by the following equations:
where a is the intercept, b is the slope of the line, and e is the error term. This equation can be used to predict the value of the target variable based on the given predictor variable(s). Multiple linear regression is an extension of simple linear regression that allows two or more predictor variables to model a response variable, y, as a linear function [ 41 ] defined in Eq. 6 , whereas simple linear regression has only 1 independent variable, defined in Eq. 5 .
Polynomial regression: Polynomial regression is a form of regression analysis in which the relationship between the independent variable x and the dependent variable y is not linear, but is the polynomial degree of \(n^\mathrm{th}\) in x [ 82 ]. The equation for polynomial regression is also derived from linear regression (polynomial regression of degree 1) equation, which is defined as below:
Here, y is the predicted/target output, \(b_0, b_1,... b_n\) are the regression coefficients, x is an independent/ input variable. In simple words, we can say that if data are not distributed linearly, instead it is \(n^\mathrm{th}\) degree of polynomial then we use polynomial regression to get desired output.
LASSO and ridge regression: LASSO and Ridge regression are well known as powerful techniques which are typically used for building learning models in presence of a large number of features, due to their capability to preventing over-fitting and reducing the complexity of the model. The LASSO (least absolute shrinkage and selection operator) regression model uses L 1 regularization technique [ 82 ] that uses shrinkage, which penalizes “absolute value of magnitude of coefficients” ( L 1 penalty). As a result, LASSO appears to render coefficients to absolute zero. Thus, LASSO regression aims to find the subset of predictors that minimizes the prediction error for a quantitative response variable. On the other hand, ridge regression uses L 2 regularization [ 82 ], which is the “squared magnitude of coefficients” ( L 2 penalty). Thus, ridge regression forces the weights to be small but never sets the coefficient value to zero, and does a non-sparse solution. Overall, LASSO regression is useful to obtain a subset of predictors by eliminating less important features, and ridge regression is useful when a data set has “multicollinearity” which refers to the predictors that are correlated with other predictors.
Cluster Analysis
Cluster analysis, also known as clustering, is an unsupervised machine learning technique for identifying and grouping related data points in large datasets without concern for the specific outcome. It does grouping a collection of objects in such a way that objects in the same category, called a cluster, are in some sense more similar to each other than objects in other groups [ 41 ]. It is often used as a data analysis technique to discover interesting trends or patterns in data, e.g., groups of consumers based on their behavior. In a broad range of application areas, such as cybersecurity, e-commerce, mobile data processing, health analytics, user modeling and behavioral analytics, clustering can be used. In the following, we briefly discuss and summarize various types of clustering methods.
Partitioning methods: Based on the features and similarities in the data, this clustering approach categorizes the data into multiple groups or clusters. The data scientists or analysts typically determine the number of clusters either dynamically or statically depending on the nature of the target applications, to produce for the methods of clustering. The most common clustering algorithms based on partitioning methods are K-means [ 69 ], K-Mediods [ 80 ], CLARA [ 55 ] etc.
Density-based methods: To identify distinct groups or clusters, it uses the concept that a cluster in the data space is a contiguous region of high point density isolated from other such clusters by contiguous regions of low point density. Points that are not part of a cluster are considered as noise. The typical clustering algorithms based on density are DBSCAN [ 32 ], OPTICS [ 12 ] etc. The density-based methods typically struggle with clusters of similar density and high dimensionality data.
Hierarchical-based methods: Hierarchical clustering typically seeks to construct a hierarchy of clusters, i.e., the tree structure. Strategies for hierarchical clustering generally fall into two types: (i) Agglomerative—a “bottom-up” approach in which each observation begins in its cluster and pairs of clusters are combined as one, moves up the hierarchy, and (ii) Divisive—a “top-down” approach in which all observations begin in one cluster and splits are performed recursively, moves down the hierarchy, as shown in Fig 7 . Our earlier proposed BOTS technique, Sarker et al. [ 102 ] is an example of a hierarchical, particularly, bottom-up clustering algorithm.
Grid-based methods: To deal with massive datasets, grid-based clustering is especially suitable. To obtain clusters, the principle is first to summarize the dataset with a grid representation and then to combine grid cells. STING [ 122 ], CLIQUE [ 6 ], etc. are the standard algorithms of grid-based clustering.
Model-based methods: There are mainly two types of model-based clustering algorithms: one that uses statistical learning, and the other based on a method of neural network learning [ 130 ]. For instance, GMM [ 89 ] is an example of a statistical learning method, and SOM [ 22 ] [ 96 ] is an example of a neural network learning method.
Constraint-based methods: Constrained-based clustering is a semi-supervised approach to data clustering that uses constraints to incorporate domain knowledge. Application or user-oriented constraints are incorporated to perform the clustering. The typical algorithms of this kind of clustering are COP K-means [ 121 ], CMWK-Means [ 27 ], etc.
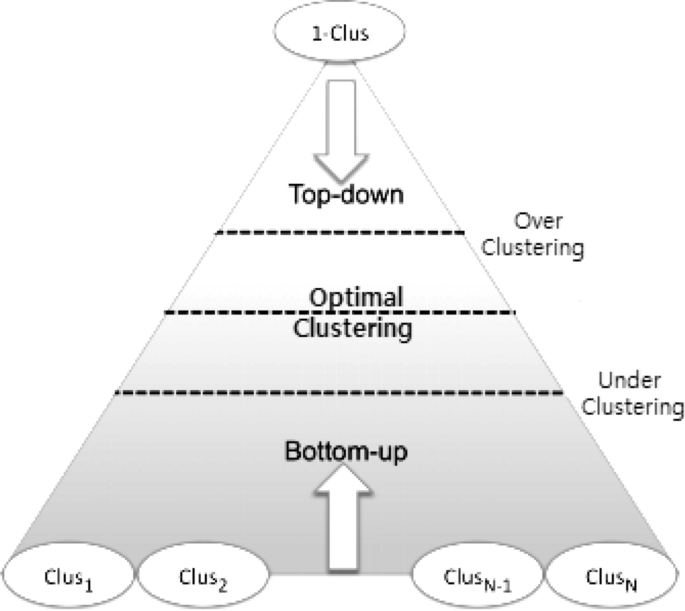
A graphical interpretation of the widely-used hierarchical clustering (Bottom-up and top-down) technique
Many clustering algorithms have been proposed with the ability to grouping data in machine learning and data science literature [ 41 , 125 ]. In the following, we summarize the popular methods that are used widely in various application areas.
K-means clustering: K-means clustering [ 69 ] is a fast, robust, and simple algorithm that provides reliable results when data sets are well-separated from each other. The data points are allocated to a cluster in this algorithm in such a way that the amount of the squared distance between the data points and the centroid is as small as possible. In other words, the K-means algorithm identifies the k number of centroids and then assigns each data point to the nearest cluster while keeping the centroids as small as possible. Since it begins with a random selection of cluster centers, the results can be inconsistent. Since extreme values can easily affect a mean, the K-means clustering algorithm is sensitive to outliers. K-medoids clustering [ 91 ] is a variant of K-means that is more robust to noises and outliers.
Mean-shift clustering: Mean-shift clustering [ 37 ] is a nonparametric clustering technique that does not require prior knowledge of the number of clusters or constraints on cluster shape. Mean-shift clustering aims to discover “blobs” in a smooth distribution or density of samples [ 82 ]. It is a centroid-based algorithm that works by updating centroid candidates to be the mean of the points in a given region. To form the final set of centroids, these candidates are filtered in a post-processing stage to remove near-duplicates. Cluster analysis in computer vision and image processing are examples of application domains. Mean Shift has the disadvantage of being computationally expensive. Moreover, in cases of high dimension, where the number of clusters shifts abruptly, the mean-shift algorithm does not work well.
DBSCAN: Density-based spatial clustering of applications with noise (DBSCAN) [ 32 ] is a base algorithm for density-based clustering which is widely used in data mining and machine learning. This is known as a non-parametric density-based clustering technique for separating high-density clusters from low-density clusters that are used in model building. DBSCAN’s main idea is that a point belongs to a cluster if it is close to many points from that cluster. It can find clusters of various shapes and sizes in a vast volume of data that is noisy and contains outliers. DBSCAN, unlike k-means, does not require a priori specification of the number of clusters in the data and can find arbitrarily shaped clusters. Although k-means is much faster than DBSCAN, it is efficient at finding high-density regions and outliers, i.e., is robust to outliers.
GMM clustering: Gaussian mixture models (GMMs) are often used for data clustering, which is a distribution-based clustering algorithm. A Gaussian mixture model is a probabilistic model in which all the data points are produced by a mixture of a finite number of Gaussian distributions with unknown parameters [ 82 ]. To find the Gaussian parameters for each cluster, an optimization algorithm called expectation-maximization (EM) [ 82 ] can be used. EM is an iterative method that uses a statistical model to estimate the parameters. In contrast to k-means, Gaussian mixture models account for uncertainty and return the likelihood that a data point belongs to one of the k clusters. GMM clustering is more robust than k-means and works well even with non-linear data distributions.
Agglomerative hierarchical clustering: The most common method of hierarchical clustering used to group objects in clusters based on their similarity is agglomerative clustering. This technique uses a bottom-up approach, where each object is first treated as a singleton cluster by the algorithm. Following that, pairs of clusters are merged one by one until all clusters have been merged into a single large cluster containing all objects. The result is a dendrogram, which is a tree-based representation of the elements. Single linkage [ 115 ], Complete linkage [ 116 ], BOTS [ 102 ] etc. are some examples of such techniques. The main advantage of agglomerative hierarchical clustering over k-means is that the tree-structure hierarchy generated by agglomerative clustering is more informative than the unstructured collection of flat clusters returned by k-means, which can help to make better decisions in the relevant application areas.
Dimensionality Reduction and Feature Learning
In machine learning and data science, high-dimensional data processing is a challenging task for both researchers and application developers. Thus, dimensionality reduction which is an unsupervised learning technique, is important because it leads to better human interpretations, lower computational costs, and avoids overfitting and redundancy by simplifying models. Both the process of feature selection and feature extraction can be used for dimensionality reduction. The primary distinction between the selection and extraction of features is that the “feature selection” keeps a subset of the original features [ 97 ], while “feature extraction” creates brand new ones [ 98 ]. In the following, we briefly discuss these techniques.
Feature selection: The selection of features, also known as the selection of variables or attributes in the data, is the process of choosing a subset of unique features (variables, predictors) to use in building machine learning and data science model. It decreases a model’s complexity by eliminating the irrelevant or less important features and allows for faster training of machine learning algorithms. A right and optimal subset of the selected features in a problem domain is capable to minimize the overfitting problem through simplifying and generalizing the model as well as increases the model’s accuracy [ 97 ]. Thus, “feature selection” [ 66 , 99 ] is considered as one of the primary concepts in machine learning that greatly affects the effectiveness and efficiency of the target machine learning model. Chi-squared test, Analysis of variance (ANOVA) test, Pearson’s correlation coefficient, recursive feature elimination, are some popular techniques that can be used for feature selection.
Feature extraction: In a machine learning-based model or system, feature extraction techniques usually provide a better understanding of the data, a way to improve prediction accuracy, and to reduce computational cost or training time. The aim of “feature extraction” [ 66 , 99 ] is to reduce the number of features in a dataset by generating new ones from the existing ones and then discarding the original features. The majority of the information found in the original set of features can then be summarized using this new reduced set of features. For instance, principal components analysis (PCA) is often used as a dimensionality-reduction technique to extract a lower-dimensional space creating new brand components from the existing features in a dataset [ 98 ].
Many algorithms have been proposed to reduce data dimensions in the machine learning and data science literature [ 41 , 125 ]. In the following, we summarize the popular methods that are used widely in various application areas.
Variance threshold: A simple basic approach to feature selection is the variance threshold [ 82 ]. This excludes all features of low variance, i.e., all features whose variance does not exceed the threshold. It eliminates all zero-variance characteristics by default, i.e., characteristics that have the same value in all samples. This feature selection algorithm looks only at the ( X ) features, not the ( y ) outputs needed, and can, therefore, be used for unsupervised learning.
Pearson correlation: Pearson’s correlation is another method to understand a feature’s relation to the response variable and can be used for feature selection [ 99 ]. This method is also used for finding the association between the features in a dataset. The resulting value is \([-1, 1]\) , where \(-1\) means perfect negative correlation, \(+1\) means perfect positive correlation, and 0 means that the two variables do not have a linear correlation. If two random variables represent X and Y , then the correlation coefficient between X and Y is defined as [ 41 ]
ANOVA: Analysis of variance (ANOVA) is a statistical tool used to verify the mean values of two or more groups that differ significantly from each other. ANOVA assumes a linear relationship between the variables and the target and the variables’ normal distribution. To statistically test the equality of means, the ANOVA method utilizes F tests. For feature selection, the results ‘ANOVA F value’ [ 82 ] of this test can be used where certain features independent of the goal variable can be omitted.
Chi square: The chi-square \({\chi }^2\) [ 82 ] statistic is an estimate of the difference between the effects of a series of events or variables observed and expected frequencies. The magnitude of the difference between the real and observed values, the degrees of freedom, and the sample size depends on \({\chi }^2\) . The chi-square \({\chi }^2\) is commonly used for testing relationships between categorical variables. If \(O_i\) represents observed value and \(E_i\) represents expected value, then
Recursive feature elimination (RFE): Recursive Feature Elimination (RFE) is a brute force approach to feature selection. RFE [ 82 ] fits the model and removes the weakest feature before it meets the specified number of features. Features are ranked by the coefficients or feature significance of the model. RFE aims to remove dependencies and collinearity in the model by recursively removing a small number of features per iteration.
Model-based selection: To reduce the dimensionality of the data, linear models penalized with the L 1 regularization can be used. Least absolute shrinkage and selection operator (Lasso) regression is a type of linear regression that has the property of shrinking some of the coefficients to zero [ 82 ]. Therefore, that feature can be removed from the model. Thus, the penalized lasso regression method, often used in machine learning to select the subset of variables. Extra Trees Classifier [ 82 ] is an example of a tree-based estimator that can be used to compute impurity-based function importance, which can then be used to discard irrelevant features.
Principal component analysis (PCA): Principal component analysis (PCA) is a well-known unsupervised learning approach in the field of machine learning and data science. PCA is a mathematical technique that transforms a set of correlated variables into a set of uncorrelated variables known as principal components [ 48 , 81 ]. Figure 8 shows an example of the effect of PCA on various dimensions space, where Fig. 8 a shows the original features in 3D space, and Fig. 8 b shows the created principal components PC1 and PC2 onto a 2D plane, and 1D line with the principal component PC1 respectively. Thus, PCA can be used as a feature extraction technique that reduces the dimensionality of the datasets, and to build an effective machine learning model [ 98 ]. Technically, PCA identifies the completely transformed with the highest eigenvalues of a covariance matrix and then uses those to project the data into a new subspace of equal or fewer dimensions [ 82 ].
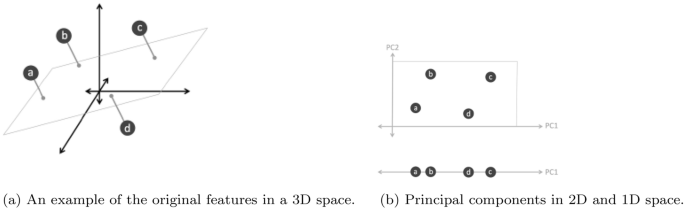
An example of a principal component analysis (PCA) and created principal components PC1 and PC2 in different dimension space
Association Rule Learning
Association rule learning is a rule-based machine learning approach to discover interesting relationships, “IF-THEN” statements, in large datasets between variables [ 7 ]. One example is that “if a customer buys a computer or laptop (an item), s/he is likely to also buy anti-virus software (another item) at the same time”. Association rules are employed today in many application areas, including IoT services, medical diagnosis, usage behavior analytics, web usage mining, smartphone applications, cybersecurity applications, and bioinformatics. In comparison to sequence mining, association rule learning does not usually take into account the order of things within or across transactions. A common way of measuring the usefulness of association rules is to use its parameter, the ‘support’ and ‘confidence’, which is introduced in [ 7 ].
In the data mining literature, many association rule learning methods have been proposed, such as logic dependent [ 34 ], frequent pattern based [ 8 , 49 , 68 ], and tree-based [ 42 ]. The most popular association rule learning algorithms are summarized below.
AIS and SETM: AIS is the first algorithm proposed by Agrawal et al. [ 7 ] for association rule mining. The AIS algorithm’s main downside is that too many candidate itemsets are generated, requiring more space and wasting a lot of effort. This algorithm calls for too many passes over the entire dataset to produce the rules. Another approach SETM [ 49 ] exhibits good performance and stable behavior with execution time; however, it suffers from the same flaw as the AIS algorithm.
Apriori: For generating association rules for a given dataset, Agrawal et al. [ 8 ] proposed the Apriori, Apriori-TID, and Apriori-Hybrid algorithms. These later algorithms outperform the AIS and SETM mentioned above due to the Apriori property of frequent itemset [ 8 ]. The term ‘Apriori’ usually refers to having prior knowledge of frequent itemset properties. Apriori uses a “bottom-up” approach, where it generates the candidate itemsets. To reduce the search space, Apriori uses the property “all subsets of a frequent itemset must be frequent; and if an itemset is infrequent, then all its supersets must also be infrequent”. Another approach predictive Apriori [ 108 ] can also generate rules; however, it receives unexpected results as it combines both the support and confidence. The Apriori [ 8 ] is the widely applicable techniques in mining association rules.
ECLAT: This technique was proposed by Zaki et al. [ 131 ] and stands for Equivalence Class Clustering and bottom-up Lattice Traversal. ECLAT uses a depth-first search to find frequent itemsets. In contrast to the Apriori [ 8 ] algorithm, which represents data in a horizontal pattern, it represents data vertically. Hence, the ECLAT algorithm is more efficient and scalable in the area of association rule learning. This algorithm is better suited for small and medium datasets whereas the Apriori algorithm is used for large datasets.
FP-Growth: Another common association rule learning technique based on the frequent-pattern tree (FP-tree) proposed by Han et al. [ 42 ] is Frequent Pattern Growth, known as FP-Growth. The key difference with Apriori is that while generating rules, the Apriori algorithm [ 8 ] generates frequent candidate itemsets; on the other hand, the FP-growth algorithm [ 42 ] prevents candidate generation and thus produces a tree by the successful strategy of ‘divide and conquer’ approach. Due to its sophistication, however, FP-Tree is challenging to use in an interactive mining environment [ 133 ]. Thus, the FP-Tree would not fit into memory for massive data sets, making it challenging to process big data as well. Another solution is RARM (Rapid Association Rule Mining) proposed by Das et al. [ 26 ] but faces a related FP-tree issue [ 133 ].
ABC-RuleMiner: A rule-based machine learning method, recently proposed in our earlier paper, by Sarker et al. [ 104 ], to discover the interesting non-redundant rules to provide real-world intelligent services. This algorithm effectively identifies the redundancy in associations by taking into account the impact or precedence of the related contextual features and discovers a set of non-redundant association rules. This algorithm first constructs an association generation tree (AGT), a top-down approach, and then extracts the association rules through traversing the tree. Thus, ABC-RuleMiner is more potent than traditional rule-based methods in terms of both non-redundant rule generation and intelligent decision-making, particularly in a context-aware smart computing environment, where human or user preferences are involved.
Among the association rule learning techniques discussed above, Apriori [ 8 ] is the most widely used algorithm for discovering association rules from a given dataset [ 133 ]. The main strength of the association learning technique is its comprehensiveness, as it generates all associations that satisfy the user-specified constraints, such as minimum support and confidence value. The ABC-RuleMiner approach [ 104 ] discussed earlier could give significant results in terms of non-redundant rule generation and intelligent decision-making for the relevant application areas in the real world.
Reinforcement Learning
Reinforcement learning (RL) is a machine learning technique that allows an agent to learn by trial and error in an interactive environment using input from its actions and experiences. Unlike supervised learning, which is based on given sample data or examples, the RL method is based on interacting with the environment. The problem to be solved in reinforcement learning (RL) is defined as a Markov Decision Process (MDP) [ 86 ], i.e., all about sequentially making decisions. An RL problem typically includes four elements such as Agent, Environment, Rewards, and Policy.
RL can be split roughly into Model-based and Model-free techniques. Model-based RL is the process of inferring optimal behavior from a model of the environment by performing actions and observing the results, which include the next state and the immediate reward [ 85 ]. AlphaZero, AlphaGo [ 113 ] are examples of the model-based approaches. On the other hand, a model-free approach does not use the distribution of the transition probability and the reward function associated with MDP. Q-learning, Deep Q Network, Monte Carlo Control, SARSA (State–Action–Reward–State–Action), etc. are some examples of model-free algorithms [ 52 ]. The policy network, which is required for model-based RL but not for model-free, is the key difference between model-free and model-based learning. In the following, we discuss the popular RL algorithms.
Monte Carlo methods: Monte Carlo techniques, or Monte Carlo experiments, are a wide category of computational algorithms that rely on repeated random sampling to obtain numerical results [ 52 ]. The underlying concept is to use randomness to solve problems that are deterministic in principle. Optimization, numerical integration, and making drawings from the probability distribution are the three problem classes where Monte Carlo techniques are most commonly used.
Q-learning: Q-learning is a model-free reinforcement learning algorithm for learning the quality of behaviors that tell an agent what action to take under what conditions [ 52 ]. It does not need a model of the environment (hence the term “model-free”), and it can deal with stochastic transitions and rewards without the need for adaptations. The ‘Q’ in Q-learning usually stands for quality, as the algorithm calculates the maximum expected rewards for a given behavior in a given state.
Deep Q-learning: The basic working step in Deep Q-Learning [ 52 ] is that the initial state is fed into the neural network, which returns the Q-value of all possible actions as an output. Still, when we have a reasonably simple setting to overcome, Q-learning works well. However, when the number of states and actions becomes more complicated, deep learning can be used as a function approximator.
Reinforcement learning, along with supervised and unsupervised learning, is one of the basic machine learning paradigms. RL can be used to solve numerous real-world problems in various fields, such as game theory, control theory, operations analysis, information theory, simulation-based optimization, manufacturing, supply chain logistics, multi-agent systems, swarm intelligence, aircraft control, robot motion control, and many more.
Artificial Neural Network and Deep Learning
Deep learning is part of a wider family of artificial neural networks (ANN)-based machine learning approaches with representation learning. Deep learning provides a computational architecture by combining several processing layers, such as input, hidden, and output layers, to learn from data [ 41 ]. The main advantage of deep learning over traditional machine learning methods is its better performance in several cases, particularly learning from large datasets [ 105 , 129 ]. Figure 9 shows a general performance of deep learning over machine learning considering the increasing amount of data. However, it may vary depending on the data characteristics and experimental set up.
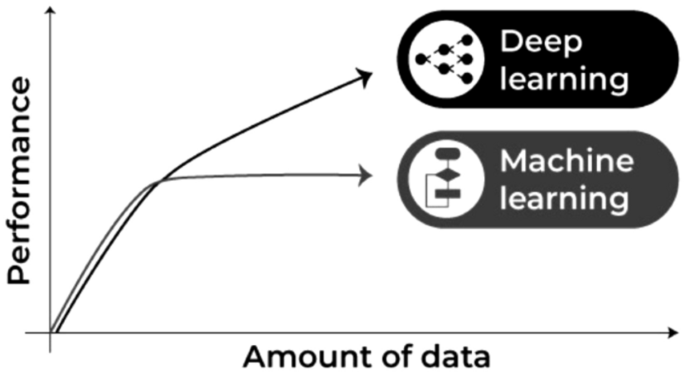
Machine learning and deep learning performance in general with the amount of data
The most common deep learning algorithms are: Multi-layer Perceptron (MLP), Convolutional Neural Network (CNN, or ConvNet), Long Short-Term Memory Recurrent Neural Network (LSTM-RNN) [ 96 ]. In the following, we discuss various types of deep learning methods that can be used to build effective data-driven models for various purposes.
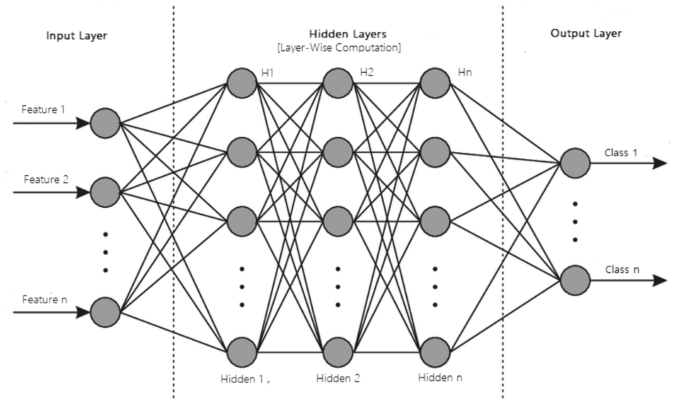
A structure of an artificial neural network modeling with multiple processing layers
MLP: The base architecture of deep learning, which is also known as the feed-forward artificial neural network, is called a multilayer perceptron (MLP) [ 82 ]. A typical MLP is a fully connected network consisting of an input layer, one or more hidden layers, and an output layer, as shown in Fig. 10 . Each node in one layer connects to each node in the following layer at a certain weight. MLP utilizes the “Backpropagation” technique [ 41 ], the most “fundamental building block” in a neural network, to adjust the weight values internally while building the model. MLP is sensitive to scaling features and allows a variety of hyperparameters to be tuned, such as the number of hidden layers, neurons, and iterations, which can result in a computationally costly model.
CNN or ConvNet: The convolution neural network (CNN) [ 65 ] enhances the design of the standard ANN, consisting of convolutional layers, pooling layers, as well as fully connected layers, as shown in Fig. 11 . As it takes the advantage of the two-dimensional (2D) structure of the input data, it is typically broadly used in several areas such as image and video recognition, image processing and classification, medical image analysis, natural language processing, etc. While CNN has a greater computational burden, without any manual intervention, it has the advantage of automatically detecting the important features, and hence CNN is considered to be more powerful than conventional ANN. A number of advanced deep learning models based on CNN can be used in the field, such as AlexNet [ 60 ], Xception [ 24 ], Inception [ 118 ], Visual Geometry Group (VGG) [ 44 ], ResNet [ 45 ], etc.
LSTM-RNN: Long short-term memory (LSTM) is an artificial recurrent neural network (RNN) architecture used in the area of deep learning [ 38 ]. LSTM has feedback links, unlike normal feed-forward neural networks. LSTM networks are well-suited for analyzing and learning sequential data, such as classifying, processing, and predicting data based on time series data, which differentiates it from other conventional networks. Thus, LSTM can be used when the data are in a sequential format, such as time, sentence, etc., and commonly applied in the area of time-series analysis, natural language processing, speech recognition, etc.
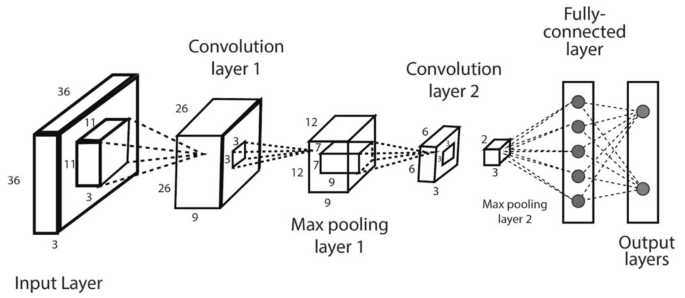
An example of a convolutional neural network (CNN or ConvNet) including multiple convolution and pooling layers
In addition to these most common deep learning methods discussed above, several other deep learning approaches [ 96 ] exist in the area for various purposes. For instance, the self-organizing map (SOM) [ 58 ] uses unsupervised learning to represent the high-dimensional data by a 2D grid map, thus achieving dimensionality reduction. The autoencoder (AE) [ 15 ] is another learning technique that is widely used for dimensionality reduction as well and feature extraction in unsupervised learning tasks. Restricted Boltzmann machines (RBM) [ 46 ] can be used for dimensionality reduction, classification, regression, collaborative filtering, feature learning, and topic modeling. A deep belief network (DBN) is typically composed of simple, unsupervised networks such as restricted Boltzmann machines (RBMs) or autoencoders, and a backpropagation neural network (BPNN) [ 123 ]. A generative adversarial network (GAN) [ 39 ] is a form of the network for deep learning that can generate data with characteristics close to the actual data input. Transfer learning is currently very common because it can train deep neural networks with comparatively low data, which is typically the re-use of a new problem with a pre-trained model [ 124 ]. A brief discussion of these artificial neural networks (ANN) and deep learning (DL) models are summarized in our earlier paper Sarker et al. [ 96 ].
Overall, based on the learning techniques discussed above, we can conclude that various types of machine learning techniques, such as classification analysis, regression, data clustering, feature selection and extraction, and dimensionality reduction, association rule learning, reinforcement learning, or deep learning techniques, can play a significant role for various purposes according to their capabilities. In the following section, we discuss several application areas based on machine learning algorithms.
Applications of Machine Learning
In the current age of the Fourth Industrial Revolution (4IR), machine learning becomes popular in various application areas, because of its learning capabilities from the past and making intelligent decisions. In the following, we summarize and discuss ten popular application areas of machine learning technology.
Predictive analytics and intelligent decision-making: A major application field of machine learning is intelligent decision-making by data-driven predictive analytics [ 21 , 70 ]. The basis of predictive analytics is capturing and exploiting relationships between explanatory variables and predicted variables from previous events to predict the unknown outcome [ 41 ]. For instance, identifying suspects or criminals after a crime has been committed, or detecting credit card fraud as it happens. Another application, where machine learning algorithms can assist retailers in better understanding consumer preferences and behavior, better manage inventory, avoiding out-of-stock situations, and optimizing logistics and warehousing in e-commerce. Various machine learning algorithms such as decision trees, support vector machines, artificial neural networks, etc. [ 106 , 125 ] are commonly used in the area. Since accurate predictions provide insight into the unknown, they can improve the decisions of industries, businesses, and almost any organization, including government agencies, e-commerce, telecommunications, banking and financial services, healthcare, sales and marketing, transportation, social networking, and many others.
Cybersecurity and threat intelligence: Cybersecurity is one of the most essential areas of Industry 4.0. [ 114 ], which is typically the practice of protecting networks, systems, hardware, and data from digital attacks [ 114 ]. Machine learning has become a crucial cybersecurity technology that constantly learns by analyzing data to identify patterns, better detect malware in encrypted traffic, find insider threats, predict where bad neighborhoods are online, keep people safe while browsing, or secure data in the cloud by uncovering suspicious activity. For instance, clustering techniques can be used to identify cyber-anomalies, policy violations, etc. To detect various types of cyber-attacks or intrusions machine learning classification models by taking into account the impact of security features are useful [ 97 ]. Various deep learning-based security models can also be used on the large scale of security datasets [ 96 , 129 ]. Moreover, security policy rules generated by association rule learning techniques can play a significant role to build a rule-based security system [ 105 ]. Thus, we can say that various learning techniques discussed in Sect. Machine Learning Tasks and Algorithms , can enable cybersecurity professionals to be more proactive inefficiently preventing threats and cyber-attacks.
Internet of things (IoT) and smart cities: Internet of Things (IoT) is another essential area of Industry 4.0. [ 114 ], which turns everyday objects into smart objects by allowing them to transmit data and automate tasks without the need for human interaction. IoT is, therefore, considered to be the big frontier that can enhance almost all activities in our lives, such as smart governance, smart home, education, communication, transportation, retail, agriculture, health care, business, and many more [ 70 ]. Smart city is one of IoT’s core fields of application, using technologies to enhance city services and residents’ living experiences [ 132 , 135 ]. As machine learning utilizes experience to recognize trends and create models that help predict future behavior and events, it has become a crucial technology for IoT applications [ 103 ]. For example, to predict traffic in smart cities, parking availability prediction, estimate the total usage of energy of the citizens for a particular period, make context-aware and timely decisions for the people, etc. are some tasks that can be solved using machine learning techniques according to the current needs of the people.
Traffic prediction and transportation: Transportation systems have become a crucial component of every country’s economic development. Nonetheless, several cities around the world are experiencing an excessive rise in traffic volume, resulting in serious issues such as delays, traffic congestion, higher fuel prices, increased CO \(_2\) pollution, accidents, emergencies, and a decline in modern society’s quality of life [ 40 ]. Thus, an intelligent transportation system through predicting future traffic is important, which is an indispensable part of a smart city. Accurate traffic prediction based on machine and deep learning modeling can help to minimize the issues [ 17 , 30 , 31 ]. For example, based on the travel history and trend of traveling through various routes, machine learning can assist transportation companies in predicting possible issues that may occur on specific routes and recommending their customers to take a different path. Ultimately, these learning-based data-driven models help improve traffic flow, increase the usage and efficiency of sustainable modes of transportation, and limit real-world disruption by modeling and visualizing future changes.
Healthcare and COVID-19 pandemic: Machine learning can help to solve diagnostic and prognostic problems in a variety of medical domains, such as disease prediction, medical knowledge extraction, detecting regularities in data, patient management, etc. [ 33 , 77 , 112 ]. Coronavirus disease (COVID-19) is an infectious disease caused by a newly discovered coronavirus, according to the World Health Organization (WHO) [ 3 ]. Recently, the learning techniques have become popular in the battle against COVID-19 [ 61 , 63 ]. For the COVID-19 pandemic, the learning techniques are used to classify patients at high risk, their mortality rate, and other anomalies [ 61 ]. It can also be used to better understand the virus’s origin, COVID-19 outbreak prediction, as well as for disease diagnosis and treatment [ 14 , 50 ]. With the help of machine learning, researchers can forecast where and when, the COVID-19 is likely to spread, and notify those regions to match the required arrangements. Deep learning also provides exciting solutions to the problems of medical image processing and is seen as a crucial technique for potential applications, particularly for COVID-19 pandemic [ 10 , 78 , 111 ]. Overall, machine and deep learning techniques can help to fight the COVID-19 virus and the pandemic as well as intelligent clinical decisions making in the domain of healthcare.
E-commerce and product recommendations: Product recommendation is one of the most well known and widely used applications of machine learning, and it is one of the most prominent features of almost any e-commerce website today. Machine learning technology can assist businesses in analyzing their consumers’ purchasing histories and making customized product suggestions for their next purchase based on their behavior and preferences. E-commerce companies, for example, can easily position product suggestions and offers by analyzing browsing trends and click-through rates of specific items. Using predictive modeling based on machine learning techniques, many online retailers, such as Amazon [ 71 ], can better manage inventory, prevent out-of-stock situations, and optimize logistics and warehousing. The future of sales and marketing is the ability to capture, evaluate, and use consumer data to provide a customized shopping experience. Furthermore, machine learning techniques enable companies to create packages and content that are tailored to the needs of their customers, allowing them to maintain existing customers while attracting new ones.
NLP and sentiment analysis: Natural language processing (NLP) involves the reading and understanding of spoken or written language through the medium of a computer [ 79 , 103 ]. Thus, NLP helps computers, for instance, to read a text, hear speech, interpret it, analyze sentiment, and decide which aspects are significant, where machine learning techniques can be used. Virtual personal assistant, chatbot, speech recognition, document description, language or machine translation, etc. are some examples of NLP-related tasks. Sentiment Analysis [ 90 ] (also referred to as opinion mining or emotion AI) is an NLP sub-field that seeks to identify and extract public mood and views within a given text through blogs, reviews, social media, forums, news, etc. For instance, businesses and brands use sentiment analysis to understand the social sentiment of their brand, product, or service through social media platforms or the web as a whole. Overall, sentiment analysis is considered as a machine learning task that analyzes texts for polarity, such as “positive”, “negative”, or “neutral” along with more intense emotions like very happy, happy, sad, very sad, angry, have interest, or not interested etc.
Image, speech and pattern recognition: Image recognition [ 36 ] is a well-known and widespread example of machine learning in the real world, which can identify an object as a digital image. For instance, to label an x-ray as cancerous or not, character recognition, or face detection in an image, tagging suggestions on social media, e.g., Facebook, are common examples of image recognition. Speech recognition [ 23 ] is also very popular that typically uses sound and linguistic models, e.g., Google Assistant, Cortana, Siri, Alexa, etc. [ 67 ], where machine learning methods are used. Pattern recognition [ 13 ] is defined as the automated recognition of patterns and regularities in data, e.g., image analysis. Several machine learning techniques such as classification, feature selection, clustering, or sequence labeling methods are used in the area.
Sustainable agriculture: Agriculture is essential to the survival of all human activities [ 109 ]. Sustainable agriculture practices help to improve agricultural productivity while also reducing negative impacts on the environment [ 5 , 25 , 109 ]. The sustainable agriculture supply chains are knowledge-intensive and based on information, skills, technologies, etc., where knowledge transfer encourages farmers to enhance their decisions to adopt sustainable agriculture practices utilizing the increasing amount of data captured by emerging technologies, e.g., the Internet of Things (IoT), mobile technologies and devices, etc. [ 5 , 53 , 54 ]. Machine learning can be applied in various phases of sustainable agriculture, such as in the pre-production phase - for the prediction of crop yield, soil properties, irrigation requirements, etc.; in the production phase—for weather prediction, disease detection, weed detection, soil nutrient management, livestock management, etc.; in processing phase—for demand estimation, production planning, etc. and in the distribution phase - the inventory management, consumer analysis, etc.
User behavior analytics and context-aware smartphone applications: Context-awareness is a system’s ability to capture knowledge about its surroundings at any moment and modify behaviors accordingly [ 28 , 93 ]. Context-aware computing uses software and hardware to automatically collect and interpret data for direct responses. The mobile app development environment has been changed greatly with the power of AI, particularly, machine learning techniques through their learning capabilities from contextual data [ 103 , 136 ]. Thus, the developers of mobile apps can rely on machine learning to create smart apps that can understand human behavior, support, and entertain users [ 107 , 137 , 140 ]. To build various personalized data-driven context-aware systems, such as smart interruption management, smart mobile recommendation, context-aware smart searching, decision-making that intelligently assist end mobile phone users in a pervasive computing environment, machine learning techniques are applicable. For example, context-aware association rules can be used to build an intelligent phone call application [ 104 ]. Clustering approaches are useful in capturing users’ diverse behavioral activities by taking into account data in time series [ 102 ]. To predict the future events in various contexts, the classification methods can be used [ 106 , 139 ]. Thus, various learning techniques discussed in Sect. “ Machine Learning Tasks and Algorithms ” can help to build context-aware adaptive and smart applications according to the preferences of the mobile phone users.
In addition to these application areas, machine learning-based models can also apply to several other domains such as bioinformatics, cheminformatics, computer networks, DNA sequence classification, economics and banking, robotics, advanced engineering, and many more.
Challenges and Research Directions
Our study on machine learning algorithms for intelligent data analysis and applications opens several research issues in the area. Thus, in this section, we summarize and discuss the challenges faced and the potential research opportunities and future directions.
In general, the effectiveness and the efficiency of a machine learning-based solution depend on the nature and characteristics of the data, and the performance of the learning algorithms. To collect the data in the relevant domain, such as cybersecurity, IoT, healthcare and agriculture discussed in Sect. “ Applications of Machine Learning ” is not straightforward, although the current cyberspace enables the production of a huge amount of data with very high frequency. Thus, collecting useful data for the target machine learning-based applications, e.g., smart city applications, and their management is important to further analysis. Therefore, a more in-depth investigation of data collection methods is needed while working on the real-world data. Moreover, the historical data may contain many ambiguous values, missing values, outliers, and meaningless data. The machine learning algorithms, discussed in Sect “ Machine Learning Tasks and Algorithms ” highly impact on data quality, and availability for training, and consequently on the resultant model. Thus, to accurately clean and pre-process the diverse data collected from diverse sources is a challenging task. Therefore, effectively modifying or enhance existing pre-processing methods, or proposing new data preparation techniques are required to effectively use the learning algorithms in the associated application domain.
To analyze the data and extract insights, there exist many machine learning algorithms, summarized in Sect. “ Machine Learning Tasks and Algorithms ”. Thus, selecting a proper learning algorithm that is suitable for the target application is challenging. The reason is that the outcome of different learning algorithms may vary depending on the data characteristics [ 106 ]. Selecting a wrong learning algorithm would result in producing unexpected outcomes that may lead to loss of effort, as well as the model’s effectiveness and accuracy. In terms of model building, the techniques discussed in Sect. “ Machine Learning Tasks and Algorithms ” can directly be used to solve many real-world issues in diverse domains, such as cybersecurity, smart cities and healthcare summarized in Sect. “ Applications of Machine Learning ”. However, the hybrid learning model, e.g., the ensemble of methods, modifying or enhancement of the existing learning techniques, or designing new learning methods, could be a potential future work in the area.
Thus, the ultimate success of a machine learning-based solution and corresponding applications mainly depends on both the data and the learning algorithms. If the data are bad to learn, such as non-representative, poor-quality, irrelevant features, or insufficient quantity for training, then the machine learning models may become useless or will produce lower accuracy. Therefore, effectively processing the data and handling the diverse learning algorithms are important, for a machine learning-based solution and eventually building intelligent applications.
In this paper, we have conducted a comprehensive overview of machine learning algorithms for intelligent data analysis and applications. According to our goal, we have briefly discussed how various types of machine learning methods can be used for making solutions to various real-world issues. A successful machine learning model depends on both the data and the performance of the learning algorithms. The sophisticated learning algorithms then need to be trained through the collected real-world data and knowledge related to the target application before the system can assist with intelligent decision-making. We also discussed several popular application areas based on machine learning techniques to highlight their applicability in various real-world issues. Finally, we have summarized and discussed the challenges faced and the potential research opportunities and future directions in the area. Therefore, the challenges that are identified create promising research opportunities in the field which must be addressed with effective solutions in various application areas. Overall, we believe that our study on machine learning-based solutions opens up a promising direction and can be used as a reference guide for potential research and applications for both academia and industry professionals as well as for decision-makers, from a technical point of view.
Canadian institute of cybersecurity, university of new brunswick, iscx dataset, http://www.unb.ca/cic/datasets/index.html/ (Accessed on 20 October 2019).
Cic-ddos2019 [online]. available: https://www.unb.ca/cic/datasets/ddos-2019.html/ (Accessed on 28 March 2020).
World health organization: WHO. http://www.who.int/ .
Google trends. In https://trends.google.com/trends/ , 2019.
Adnan N, Nordin Shahrina Md, Rahman I, Noor A. The effects of knowledge transfer on farmers decision making toward sustainable agriculture practices. World J Sci Technol Sustain Dev. 2018.
Agrawal R, Gehrke J, Gunopulos D, Raghavan P. Automatic subspace clustering of high dimensional data for data mining applications. In: Proceedings of the 1998 ACM SIGMOD international conference on Management of data. 1998; 94–105
Agrawal R, Imieliński T, Swami A. Mining association rules between sets of items in large databases. In: ACM SIGMOD Record. ACM. 1993;22: 207–216
Agrawal R, Gehrke J, Gunopulos D, Raghavan P. Fast algorithms for mining association rules. In: Proceedings of the International Joint Conference on Very Large Data Bases, Santiago Chile. 1994; 1215: 487–499.
Aha DW, Kibler D, Albert M. Instance-based learning algorithms. Mach Learn. 1991;6(1):37–66.
Article Google Scholar
Alakus TB, Turkoglu I. Comparison of deep learning approaches to predict covid-19 infection. Chaos Solit Fract. 2020;140:
Amit Y, Geman D. Shape quantization and recognition with randomized trees. Neural Comput. 1997;9(7):1545–88.
Ankerst M, Breunig MM, Kriegel H-P, Sander J. Optics: ordering points to identify the clustering structure. ACM Sigmod Record. 1999;28(2):49–60.
Anzai Y. Pattern recognition and machine learning. Elsevier; 2012.
MATH Google Scholar
Ardabili SF, Mosavi A, Ghamisi P, Ferdinand F, Varkonyi-Koczy AR, Reuter U, Rabczuk T, Atkinson PM. Covid-19 outbreak prediction with machine learning. Algorithms. 2020;13(10):249.
Article MathSciNet Google Scholar
Baldi P. Autoencoders, unsupervised learning, and deep architectures. In: Proceedings of ICML workshop on unsupervised and transfer learning, 2012; 37–49 .
Balducci F, Impedovo D, Pirlo G. Machine learning applications on agricultural datasets for smart farm enhancement. Machines. 2018;6(3):38.
Boukerche A, Wang J. Machine learning-based traffic prediction models for intelligent transportation systems. Comput Netw. 2020;181
Breiman L. Bagging predictors. Mach Learn. 1996;24(2):123–40.
Article MATH Google Scholar
Breiman L. Random forests. Mach Learn. 2001;45(1):5–32.
Breiman L, Friedman J, Stone CJ, Olshen RA. Classification and regression trees. CRC Press; 1984.
Cao L. Data science: a comprehensive overview. ACM Comput Surv (CSUR). 2017;50(3):43.
Google Scholar
Carpenter GA, Grossberg S. A massively parallel architecture for a self-organizing neural pattern recognition machine. Comput Vis Graph Image Process. 1987;37(1):54–115.
Chiu C-C, Sainath TN, Wu Y, Prabhavalkar R, Nguyen P, Chen Z, Kannan A, Weiss RJ, Rao K, Gonina E, et al. State-of-the-art speech recognition with sequence-to-sequence models. In: 2018 IEEE International Conference on Acoustics, Speech and Signal Processing (ICASSP), 2018 pages 4774–4778. IEEE .
Chollet F. Xception: deep learning with depthwise separable convolutions. In: Proceedings of the IEEE conference on computer vision and pattern recognition, pages 1251–1258, 2017.
Cobuloglu H, Büyüktahtakın IE. A stochastic multi-criteria decision analysis for sustainable biomass crop selection. Expert Syst Appl. 2015;42(15–16):6065–74.
Das A, Ng W-K, Woon Y-K. Rapid association rule mining. In: Proceedings of the tenth international conference on Information and knowledge management, pages 474–481. ACM, 2001.
de Amorim RC. Constrained clustering with minkowski weighted k-means. In: 2012 IEEE 13th International Symposium on Computational Intelligence and Informatics (CINTI), pages 13–17. IEEE, 2012.
Dey AK. Understanding and using context. Person Ubiquit Comput. 2001;5(1):4–7.
Eagle N, Pentland AS. Reality mining: sensing complex social systems. Person Ubiquit Comput. 2006;10(4):255–68.
Essien A, Petrounias I, Sampaio P, Sampaio S. Improving urban traffic speed prediction using data source fusion and deep learning. In: 2019 IEEE International Conference on Big Data and Smart Computing (BigComp). IEEE. 2019: 1–8. .
Essien A, Petrounias I, Sampaio P, Sampaio S. A deep-learning model for urban traffic flow prediction with traffic events mined from twitter. In: World Wide Web, 2020: 1–24 .
Ester M, Kriegel H-P, Sander J, Xiaowei X, et al. A density-based algorithm for discovering clusters in large spatial databases with noise. Kdd. 1996;96:226–31.
Fatima M, Pasha M, et al. Survey of machine learning algorithms for disease diagnostic. J Intell Learn Syst Appl. 2017;9(01):1.
Flach PA, Lachiche N. Confirmation-guided discovery of first-order rules with tertius. Mach Learn. 2001;42(1–2):61–95.
Freund Y, Schapire RE, et al. Experiments with a new boosting algorithm. In: Icml, Citeseer. 1996; 96: 148–156
Fujiyoshi H, Hirakawa T, Yamashita T. Deep learning-based image recognition for autonomous driving. IATSS Res. 2019;43(4):244–52.
Fukunaga K, Hostetler L. The estimation of the gradient of a density function, with applications in pattern recognition. IEEE Trans Inform Theory. 1975;21(1):32–40.
Article MathSciNet MATH Google Scholar
Goodfellow I, Bengio Y, Courville A, Bengio Y. Deep learning. Cambridge: MIT Press; 2016.
Goodfellow I, Pouget-Abadie J, Mirza M, Xu B, Warde-Farley D, Ozair S, Courville A, Bengio Y. Generative adversarial nets. In: Advances in neural information processing systems. 2014: 2672–2680.
Guerrero-Ibáñez J, Zeadally S, Contreras-Castillo J. Sensor technologies for intelligent transportation systems. Sensors. 2018;18(4):1212.
Han J, Pei J, Kamber M. Data mining: concepts and techniques. Amsterdam: Elsevier; 2011.
Han J, Pei J, Yin Y. Mining frequent patterns without candidate generation. In: ACM Sigmod Record, ACM. 2000;29: 1–12.
Harmon SA, Sanford TH, Sheng X, Turkbey EB, Roth H, Ziyue X, Yang D, Myronenko A, Anderson V, Amalou A, et al. Artificial intelligence for the detection of covid-19 pneumonia on chest ct using multinational datasets. Nat Commun. 2020;11(1):1–7.
He K, Zhang X, Ren S, Sun J. Spatial pyramid pooling in deep convolutional networks for visual recognition. IEEE Trans Pattern Anal Mach Intell. 2015;37(9):1904–16.
He K, Zhang X, Ren S, Sun J. Deep residual learning for image recognition. In: Proceedings of the IEEE conference on computer vision and pattern recognition, 2016: 770–778.
Hinton GE. A practical guide to training restricted boltzmann machines. In: Neural networks: Tricks of the trade. Springer. 2012; 599-619
Holte RC. Very simple classification rules perform well on most commonly used datasets. Mach Learn. 1993;11(1):63–90.
Hotelling H. Analysis of a complex of statistical variables into principal components. J Edu Psychol. 1933;24(6):417.
Houtsma M, Swami A. Set-oriented mining for association rules in relational databases. In: Data Engineering, 1995. Proceedings of the Eleventh International Conference on, IEEE.1995:25–33.
Jamshidi M, Lalbakhsh A, Talla J, Peroutka Z, Hadjilooei F, Lalbakhsh P, Jamshidi M, La Spada L, Mirmozafari M, Dehghani M, et al. Artificial intelligence and covid-19: deep learning approaches for diagnosis and treatment. IEEE Access. 2020;8:109581–95.
John GH, Langley P. Estimating continuous distributions in bayesian classifiers. In: Proceedings of the Eleventh conference on Uncertainty in artificial intelligence, Morgan Kaufmann Publishers Inc. 1995; 338–345
Kaelbling LP, Littman ML, Moore AW. Reinforcement learning: a survey. J Artif Intell Res. 1996;4:237–85.
Kamble SS, Gunasekaran A, Gawankar SA. Sustainable industry 4.0 framework: a systematic literature review identifying the current trends and future perspectives. Process Saf Environ Protect. 2018;117:408–25.
Kamble SS, Gunasekaran A, Gawankar SA. Achieving sustainable performance in a data-driven agriculture supply chain: a review for research and applications. Int J Prod Econ. 2020;219:179–94.
Kaufman L, Rousseeuw PJ. Finding groups in data: an introduction to cluster analysis, vol. 344. John Wiley & Sons; 2009.
Keerthi SS, Shevade SK, Bhattacharyya C, Radha Krishna MK. Improvements to platt’s smo algorithm for svm classifier design. Neural Comput. 2001;13(3):637–49.
Khadse V, Mahalle PN, Biraris SV. An empirical comparison of supervised machine learning algorithms for internet of things data. In: 2018 Fourth International Conference on Computing Communication Control and Automation (ICCUBEA), IEEE. 2018; 1–6
Kohonen T. The self-organizing map. Proc IEEE. 1990;78(9):1464–80.
Koroniotis N, Moustafa N, Sitnikova E, Turnbull B. Towards the development of realistic botnet dataset in the internet of things for network forensic analytics: bot-iot dataset. Fut Gen Comput Syst. 2019;100:779–96.
Krizhevsky A, Sutskever I, Hinton GE. Imagenet classification with deep convolutional neural networks. In: Advances in neural information processing systems, 2012: 1097–1105
Kushwaha S, Bahl S, Bagha AK, Parmar KS, Javaid M, Haleem A, Singh RP. Significant applications of machine learning for covid-19 pandemic. J Ind Integr Manag. 2020;5(4).
Lade P, Ghosh R, Srinivasan S. Manufacturing analytics and industrial internet of things. IEEE Intell Syst. 2017;32(3):74–9.
Lalmuanawma S, Hussain J, Chhakchhuak L. Applications of machine learning and artificial intelligence for covid-19 (sars-cov-2) pandemic: a review. Chaos Sol Fract. 2020:110059 .
LeCessie S, Van Houwelingen JC. Ridge estimators in logistic regression. J R Stat Soc Ser C (Appl Stat). 1992;41(1):191–201.
LeCun Y, Bottou L, Bengio Y, Haffner P. Gradient-based learning applied to document recognition. Proc IEEE. 1998;86(11):2278–324.
Liu H, Motoda H. Feature extraction, construction and selection: A data mining perspective, vol. 453. Springer Science & Business Media; 1998.
López G, Quesada L, Guerrero LA. Alexa vs. siri vs. cortana vs. google assistant: a comparison of speech-based natural user interfaces. In: International Conference on Applied Human Factors and Ergonomics, Springer. 2017; 241–250.
Liu B, HsuW, Ma Y. Integrating classification and association rule mining. In: Proceedings of the fourth international conference on knowledge discovery and data mining, 1998.
MacQueen J, et al. Some methods for classification and analysis of multivariate observations. In: Proceedings of the fifth Berkeley symposium on mathematical statistics and probability, 1967;volume 1, pages 281–297. Oakland, CA, USA.
Mahdavinejad MS, Rezvan M, Barekatain M, Adibi P, Barnaghi P, Sheth AP. Machine learning for internet of things data analysis: a survey. Digit Commun Netw. 2018;4(3):161–75.
Marchand A, Marx P. Automated product recommendations with preference-based explanations. J Retail. 2020;96(3):328–43.
McCallum A. Information extraction: distilling structured data from unstructured text. Queue. 2005;3(9):48–57.
Mehrotra A, Hendley R, Musolesi M. Prefminer: mining user’s preferences for intelligent mobile notification management. In: Proceedings of the International Joint Conference on Pervasive and Ubiquitous Computing, Heidelberg, Germany, 12–16 September, 2016; pp. 1223–1234. ACM, New York, USA. .
Mohamadou Y, Halidou A, Kapen PT. A review of mathematical modeling, artificial intelligence and datasets used in the study, prediction and management of covid-19. Appl Intell. 2020;50(11):3913–25.
Mohammed M, Khan MB, Bashier Mohammed BE. Machine learning: algorithms and applications. CRC Press; 2016.
Book Google Scholar
Moustafa N, Slay J. Unsw-nb15: a comprehensive data set for network intrusion detection systems (unsw-nb15 network data set). In: 2015 military communications and information systems conference (MilCIS), 2015;pages 1–6. IEEE .
Nilashi M, Ibrahim OB, Ahmadi H, Shahmoradi L. An analytical method for diseases prediction using machine learning techniques. Comput Chem Eng. 2017;106:212–23.
Yujin O, Park S, Ye JC. Deep learning covid-19 features on cxr using limited training data sets. IEEE Trans Med Imaging. 2020;39(8):2688–700.
Otter DW, Medina JR , Kalita JK. A survey of the usages of deep learning for natural language processing. IEEE Trans Neural Netw Learn Syst. 2020.
Park H-S, Jun C-H. A simple and fast algorithm for k-medoids clustering. Expert Syst Appl. 2009;36(2):3336–41.
Liii Pearson K. on lines and planes of closest fit to systems of points in space. Lond Edinb Dublin Philos Mag J Sci. 1901;2(11):559–72.
Pedregosa F, Varoquaux G, Gramfort A, Michel V, Thirion B, Grisel O, Blondel M, Prettenhofer P, Weiss R, Dubourg V, et al. Scikit-learn: machine learning in python. J Mach Learn Res. 2011;12:2825–30.
MathSciNet MATH Google Scholar
Perveen S, Shahbaz M, Keshavjee K, Guergachi A. Metabolic syndrome and development of diabetes mellitus: predictive modeling based on machine learning techniques. IEEE Access. 2018;7:1365–75.
Santi P, Ram D, Rob C, Nathan E. Behavior-based adaptive call predictor. ACM Trans Auton Adapt Syst. 2011;6(3):21:1–21:28.
Polydoros AS, Nalpantidis L. Survey of model-based reinforcement learning: applications on robotics. J Intell Robot Syst. 2017;86(2):153–73.
Puterman ML. Markov decision processes: discrete stochastic dynamic programming. John Wiley & Sons; 2014.
Quinlan JR. Induction of decision trees. Mach Learn. 1986;1:81–106.
Quinlan JR. C4.5: programs for machine learning. Mach Learn. 1993.
Rasmussen C. The infinite gaussian mixture model. Adv Neural Inform Process Syst. 1999;12:554–60.
Ravi K, Ravi V. A survey on opinion mining and sentiment analysis: tasks, approaches and applications. Knowl Syst. 2015;89:14–46.
Rokach L. A survey of clustering algorithms. In: Data mining and knowledge discovery handbook, pages 269–298. Springer, 2010.
Safdar S, Zafar S, Zafar N, Khan NF. Machine learning based decision support systems (dss) for heart disease diagnosis: a review. Artif Intell Rev. 2018;50(4):597–623.
Sarker IH. Context-aware rule learning from smartphone data: survey, challenges and future directions. J Big Data. 2019;6(1):1–25.
Sarker IH. A machine learning based robust prediction model for real-life mobile phone data. Internet Things. 2019;5:180–93.
Sarker IH. Ai-driven cybersecurity: an overview, security intelligence modeling and research directions. SN Comput Sci. 2021.
Sarker IH. Deep cybersecurity: a comprehensive overview from neural network and deep learning perspective. SN Comput Sci. 2021.
Sarker IH, Abushark YB, Alsolami F, Khan A. Intrudtree: a machine learning based cyber security intrusion detection model. Symmetry. 2020;12(5):754.
Sarker IH, Abushark YB, Khan A. Contextpca: predicting context-aware smartphone apps usage based on machine learning techniques. Symmetry. 2020;12(4):499.
Sarker IH, Alqahtani H, Alsolami F, Khan A, Abushark YB, Siddiqui MK. Context pre-modeling: an empirical analysis for classification based user-centric context-aware predictive modeling. J Big Data. 2020;7(1):1–23.
Sarker IH, Alan C, Jun H, Khan AI, Abushark YB, Khaled S. Behavdt: a behavioral decision tree learning to build user-centric context-aware predictive model. Mob Netw Appl. 2019; 1–11.
Sarker IH, Colman A, Kabir MA, Han J. Phone call log as a context source to modeling individual user behavior. In: Proceedings of the 2016 ACM International Joint Conference on Pervasive and Ubiquitous Computing (Ubicomp): Adjunct, Germany, pages 630–634. ACM, 2016.
Sarker IH, Colman A, Kabir MA, Han J. Individualized time-series segmentation for mining mobile phone user behavior. Comput J Oxf Univ UK. 2018;61(3):349–68.
Sarker IH, Hoque MM, MdK Uddin, Tawfeeq A. Mobile data science and intelligent apps: concepts, ai-based modeling and research directions. Mob Netw Appl, pages 1–19, 2020.
Sarker IH, Kayes ASM. Abc-ruleminer: user behavioral rule-based machine learning method for context-aware intelligent services. J Netw Comput Appl. 2020; page 102762
Sarker IH, Kayes ASM, Badsha S, Alqahtani H, Watters P, Ng A. Cybersecurity data science: an overview from machine learning perspective. J Big Data. 2020;7(1):1–29.
Sarker IH, Watters P, Kayes ASM. Effectiveness analysis of machine learning classification models for predicting personalized context-aware smartphone usage. J Big Data. 2019;6(1):1–28.
Sarker IH, Salah K. Appspred: predicting context-aware smartphone apps using random forest learning. Internet Things. 2019;8:
Scheffer T. Finding association rules that trade support optimally against confidence. Intell Data Anal. 2005;9(4):381–95.
Sharma R, Kamble SS, Gunasekaran A, Kumar V, Kumar A. A systematic literature review on machine learning applications for sustainable agriculture supply chain performance. Comput Oper Res. 2020;119:
Shengli S, Ling CX. Hybrid cost-sensitive decision tree, knowledge discovery in databases. In: PKDD 2005, Proceedings of 9th European Conference on Principles and Practice of Knowledge Discovery in Databases. Lecture Notes in Computer Science, volume 3721, 2005.
Shorten C, Khoshgoftaar TM, Furht B. Deep learning applications for covid-19. J Big Data. 2021;8(1):1–54.
Gökhan S, Nevin Y. Data analysis in health and big data: a machine learning medical diagnosis model based on patients’ complaints. Commun Stat Theory Methods. 2019;1–10
Silver D, Huang A, Maddison CJ, Guez A, Sifre L, Van Den Driessche G, Schrittwieser J, Antonoglou I, Panneershelvam V, Lanctot M, et al. Mastering the game of go with deep neural networks and tree search. nature. 2016;529(7587):484–9.
Ślusarczyk B. Industry 4.0: Are we ready? Polish J Manag Stud. 17, 2018.
Sneath Peter HA. The application of computers to taxonomy. J Gen Microbiol. 1957;17(1).
Sorensen T. Method of establishing groups of equal amplitude in plant sociology based on similarity of species. Biol Skr. 1948; 5.
Srinivasan V, Moghaddam S, Mukherji A. Mobileminer: mining your frequent patterns on your phone. In: Proceedings of the International Joint Conference on Pervasive and Ubiquitous Computing, Seattle, WA, USA, 13-17 September, pp. 389–400. ACM, New York, USA. 2014.
Szegedy C, Liu W, Jia Y, Sermanet P, Reed S, Anguelov D, Erhan D, Vanhoucke V, Rabinovich A. Going deeper with convolutions. In: Proceedings of the IEEE conference on computer vision and pattern recognition. 2015; pages 1–9.
Tavallaee M, Bagheri E, Lu W, Ghorbani AA. A detailed analysis of the kdd cup 99 data set. In. IEEE symposium on computational intelligence for security and defense applications. IEEE. 2009;2009:1–6.
Tsagkias M. Tracy HK, Surya K, Vanessa M, de Rijke M. Challenges and research opportunities in ecommerce search and recommendations. In: ACM SIGIR Forum. volume 54. NY, USA: ACM New York; 2021. p. 1–23.
Wagstaff K, Cardie C, Rogers S, Schrödl S, et al. Constrained k-means clustering with background knowledge. Icml. 2001;1:577–84.
Wang W, Yang J, Muntz R, et al. Sting: a statistical information grid approach to spatial data mining. VLDB. 1997;97:186–95.
Wei P, Li Y, Zhang Z, Tao H, Li Z, Liu D. An optimization method for intrusion detection classification model based on deep belief network. IEEE Access. 2019;7:87593–605.
Weiss K, Khoshgoftaar TM, Wang DD. A survey of transfer learning. J Big data. 2016;3(1):9.
Witten IH, Frank E. Data Mining: Practical machine learning tools and techniques. Morgan Kaufmann; 2005.
Witten IH, Frank E, Trigg LE, Hall MA, Holmes G, Cunningham SJ. Weka: practical machine learning tools and techniques with java implementations. 1999.
Wu C-C, Yen-Liang C, Yi-Hung L, Xiang-Yu Y. Decision tree induction with a constrained number of leaf nodes. Appl Intell. 2016;45(3):673–85.
Wu X, Kumar V, Quinlan JR, Ghosh J, Yang Q, Motoda H, McLachlan GJ, Ng A, Liu B, Philip SY, et al. Top 10 algorithms in data mining. Knowl Inform Syst. 2008;14(1):1–37.
Xin Y, Kong L, Liu Z, Chen Y, Li Y, Zhu H, Gao M, Hou H, Wang C. Machine learning and deep learning methods for cybersecurity. IEEE Access. 2018;6:35365–81.
Xu D, Yingjie T. A comprehensive survey of clustering algorithms. Ann Data Sci. 2015;2(2):165–93.
Zaki MJ. Scalable algorithms for association mining. IEEE Trans Knowl Data Eng. 2000;12(3):372–90.
Zanella A, Bui N, Castellani A, Vangelista L, Zorzi M. Internet of things for smart cities. IEEE Internet Things J. 2014;1(1):22–32.
Zhao Q, Bhowmick SS. Association rule mining: a survey. Singapore: Nanyang Technological University; 2003.
Zheng T, Xie W, Xu L, He X, Zhang Y, You M, Yang G, Chen Y. A machine learning-based framework to identify type 2 diabetes through electronic health records. Int J Med Inform. 2017;97:120–7.
Zheng Y, Rajasegarar S, Leckie C. Parking availability prediction for sensor-enabled car parks in smart cities. In: Intelligent Sensors, Sensor Networks and Information Processing (ISSNIP), 2015 IEEE Tenth International Conference on. IEEE, 2015; pages 1–6.
Zhu H, Cao H, Chen E, Xiong H, Tian J. Exploiting enriched contextual information for mobile app classification. In: Proceedings of the 21st ACM international conference on Information and knowledge management. ACM, 2012; pages 1617–1621
Zhu H, Chen E, Xiong H, Kuifei Y, Cao H, Tian J. Mining mobile user preferences for personalized context-aware recommendation. ACM Trans Intell Syst Technol (TIST). 2014;5(4):58.
Zikang H, Yong Y, Guofeng Y, Xinyu Z. Sentiment analysis of agricultural product ecommerce review data based on deep learning. In: 2020 International Conference on Internet of Things and Intelligent Applications (ITIA), IEEE, 2020; pages 1–7
Zulkernain S, Madiraju P, Ahamed SI. A context aware interruption management system for mobile devices. In: Mobile Wireless Middleware, Operating Systems, and Applications. Springer. 2010; pages 221–234
Zulkernain S, Madiraju P, Ahamed S, Stamm K. A mobile intelligent interruption management system. J UCS. 2010;16(15):2060–80.
Download references
Author information
Authors and affiliations.
Swinburne University of Technology, Melbourne, VIC, 3122, Australia
Iqbal H. Sarker
Department of Computer Science and Engineering, Chittagong University of Engineering & Technology, 4349, Chattogram, Bangladesh
You can also search for this author in PubMed Google Scholar
Corresponding author
Correspondence to Iqbal H. Sarker .
Ethics declarations
Conflict of interest.
The author declares no conflict of interest.
Additional information
Publisher's note.
Springer Nature remains neutral with regard to jurisdictional claims in published maps and institutional affiliations.
This article is part of the topical collection “Advances in Computational Approaches for Artificial Intelligence, Image Processing, IoT and Cloud Applications” guest edited by Bhanu Prakash K N and M. Shivakumar.
Rights and permissions
Reprints and permissions
About this article
Sarker, I.H. Machine Learning: Algorithms, Real-World Applications and Research Directions. SN COMPUT. SCI. 2 , 160 (2021). https://doi.org/10.1007/s42979-021-00592-x
Download citation
Received : 27 January 2021
Accepted : 12 March 2021
Published : 22 March 2021
DOI : https://doi.org/10.1007/s42979-021-00592-x
Share this article
Anyone you share the following link with will be able to read this content:
Sorry, a shareable link is not currently available for this article.
Provided by the Springer Nature SharedIt content-sharing initiative
- Machine learning
- Deep learning
- Artificial intelligence
- Data science
- Data-driven decision-making
- Predictive analytics
- Intelligent applications
- Find a journal
- Publish with us
- Track your research
Academia.edu no longer supports Internet Explorer.
To browse Academia.edu and the wider internet faster and more securely, please take a few seconds to upgrade your browser .
- We're Hiring!
- Help Center
Visual Basic 6.0
- Most Cited Papers
- Most Downloaded Papers
- Newest Papers
- Save to Library
- Last »
- Pemrograman Database Follow Following
- Pemrograman VB 6.0 Follow Following
- Tutorial Visual Basic 6.0 Follow Following
- Belajar Vb 6 Follow Following
- Computer Security Follow Following
- Visual Basic Programming Language Follow Following
- Computer Science Follow Following
- Teknik Informatika Follow Following
- Education Follow Following
- Programmation Java Netbeans Follow Following
Enter the email address you signed up with and we'll email you a reset link.
- Academia.edu Publishing
- We're Hiring!
- Help Center
- Find new research papers in:
- Health Sciences
- Earth Sciences
- Cognitive Science
- Mathematics
- Computer Science
- Academia ©2024
- Privacy Policy

Home » Research Paper – Structure, Examples and Writing Guide
Research Paper – Structure, Examples and Writing Guide
Table of Contents

Research Paper
Definition:
Research Paper is a written document that presents the author’s original research, analysis, and interpretation of a specific topic or issue.
It is typically based on Empirical Evidence, and may involve qualitative or quantitative research methods, or a combination of both. The purpose of a research paper is to contribute new knowledge or insights to a particular field of study, and to demonstrate the author’s understanding of the existing literature and theories related to the topic.
Structure of Research Paper
The structure of a research paper typically follows a standard format, consisting of several sections that convey specific information about the research study. The following is a detailed explanation of the structure of a research paper:
The title page contains the title of the paper, the name(s) of the author(s), and the affiliation(s) of the author(s). It also includes the date of submission and possibly, the name of the journal or conference where the paper is to be published.
The abstract is a brief summary of the research paper, typically ranging from 100 to 250 words. It should include the research question, the methods used, the key findings, and the implications of the results. The abstract should be written in a concise and clear manner to allow readers to quickly grasp the essence of the research.
Introduction
The introduction section of a research paper provides background information about the research problem, the research question, and the research objectives. It also outlines the significance of the research, the research gap that it aims to fill, and the approach taken to address the research question. Finally, the introduction section ends with a clear statement of the research hypothesis or research question.
Literature Review
The literature review section of a research paper provides an overview of the existing literature on the topic of study. It includes a critical analysis and synthesis of the literature, highlighting the key concepts, themes, and debates. The literature review should also demonstrate the research gap and how the current study seeks to address it.
The methods section of a research paper describes the research design, the sample selection, the data collection and analysis procedures, and the statistical methods used to analyze the data. This section should provide sufficient detail for other researchers to replicate the study.
The results section presents the findings of the research, using tables, graphs, and figures to illustrate the data. The findings should be presented in a clear and concise manner, with reference to the research question and hypothesis.
The discussion section of a research paper interprets the findings and discusses their implications for the research question, the literature review, and the field of study. It should also address the limitations of the study and suggest future research directions.
The conclusion section summarizes the main findings of the study, restates the research question and hypothesis, and provides a final reflection on the significance of the research.
The references section provides a list of all the sources cited in the paper, following a specific citation style such as APA, MLA or Chicago.
How to Write Research Paper
You can write Research Paper by the following guide:
- Choose a Topic: The first step is to select a topic that interests you and is relevant to your field of study. Brainstorm ideas and narrow down to a research question that is specific and researchable.
- Conduct a Literature Review: The literature review helps you identify the gap in the existing research and provides a basis for your research question. It also helps you to develop a theoretical framework and research hypothesis.
- Develop a Thesis Statement : The thesis statement is the main argument of your research paper. It should be clear, concise and specific to your research question.
- Plan your Research: Develop a research plan that outlines the methods, data sources, and data analysis procedures. This will help you to collect and analyze data effectively.
- Collect and Analyze Data: Collect data using various methods such as surveys, interviews, observations, or experiments. Analyze data using statistical tools or other qualitative methods.
- Organize your Paper : Organize your paper into sections such as Introduction, Literature Review, Methods, Results, Discussion, and Conclusion. Ensure that each section is coherent and follows a logical flow.
- Write your Paper : Start by writing the introduction, followed by the literature review, methods, results, discussion, and conclusion. Ensure that your writing is clear, concise, and follows the required formatting and citation styles.
- Edit and Proofread your Paper: Review your paper for grammar and spelling errors, and ensure that it is well-structured and easy to read. Ask someone else to review your paper to get feedback and suggestions for improvement.
- Cite your Sources: Ensure that you properly cite all sources used in your research paper. This is essential for giving credit to the original authors and avoiding plagiarism.
Research Paper Example
Note : The below example research paper is for illustrative purposes only and is not an actual research paper. Actual research papers may have different structures, contents, and formats depending on the field of study, research question, data collection and analysis methods, and other factors. Students should always consult with their professors or supervisors for specific guidelines and expectations for their research papers.
Research Paper Example sample for Students:
Title: The Impact of Social Media on Mental Health among Young Adults
Abstract: This study aims to investigate the impact of social media use on the mental health of young adults. A literature review was conducted to examine the existing research on the topic. A survey was then administered to 200 university students to collect data on their social media use, mental health status, and perceived impact of social media on their mental health. The results showed that social media use is positively associated with depression, anxiety, and stress. The study also found that social comparison, cyberbullying, and FOMO (Fear of Missing Out) are significant predictors of mental health problems among young adults.
Introduction: Social media has become an integral part of modern life, particularly among young adults. While social media has many benefits, including increased communication and social connectivity, it has also been associated with negative outcomes, such as addiction, cyberbullying, and mental health problems. This study aims to investigate the impact of social media use on the mental health of young adults.
Literature Review: The literature review highlights the existing research on the impact of social media use on mental health. The review shows that social media use is associated with depression, anxiety, stress, and other mental health problems. The review also identifies the factors that contribute to the negative impact of social media, including social comparison, cyberbullying, and FOMO.
Methods : A survey was administered to 200 university students to collect data on their social media use, mental health status, and perceived impact of social media on their mental health. The survey included questions on social media use, mental health status (measured using the DASS-21), and perceived impact of social media on their mental health. Data were analyzed using descriptive statistics and regression analysis.
Results : The results showed that social media use is positively associated with depression, anxiety, and stress. The study also found that social comparison, cyberbullying, and FOMO are significant predictors of mental health problems among young adults.
Discussion : The study’s findings suggest that social media use has a negative impact on the mental health of young adults. The study highlights the need for interventions that address the factors contributing to the negative impact of social media, such as social comparison, cyberbullying, and FOMO.
Conclusion : In conclusion, social media use has a significant impact on the mental health of young adults. The study’s findings underscore the need for interventions that promote healthy social media use and address the negative outcomes associated with social media use. Future research can explore the effectiveness of interventions aimed at reducing the negative impact of social media on mental health. Additionally, longitudinal studies can investigate the long-term effects of social media use on mental health.
Limitations : The study has some limitations, including the use of self-report measures and a cross-sectional design. The use of self-report measures may result in biased responses, and a cross-sectional design limits the ability to establish causality.
Implications: The study’s findings have implications for mental health professionals, educators, and policymakers. Mental health professionals can use the findings to develop interventions that address the negative impact of social media use on mental health. Educators can incorporate social media literacy into their curriculum to promote healthy social media use among young adults. Policymakers can use the findings to develop policies that protect young adults from the negative outcomes associated with social media use.
References :
- Twenge, J. M., & Campbell, W. K. (2019). Associations between screen time and lower psychological well-being among children and adolescents: Evidence from a population-based study. Preventive medicine reports, 15, 100918.
- Primack, B. A., Shensa, A., Escobar-Viera, C. G., Barrett, E. L., Sidani, J. E., Colditz, J. B., … & James, A. E. (2017). Use of multiple social media platforms and symptoms of depression and anxiety: A nationally-representative study among US young adults. Computers in Human Behavior, 69, 1-9.
- Van der Meer, T. G., & Verhoeven, J. W. (2017). Social media and its impact on academic performance of students. Journal of Information Technology Education: Research, 16, 383-398.
Appendix : The survey used in this study is provided below.
Social Media and Mental Health Survey
- How often do you use social media per day?
- Less than 30 minutes
- 30 minutes to 1 hour
- 1 to 2 hours
- 2 to 4 hours
- More than 4 hours
- Which social media platforms do you use?
- Others (Please specify)
- How often do you experience the following on social media?
- Social comparison (comparing yourself to others)
- Cyberbullying
- Fear of Missing Out (FOMO)
- Have you ever experienced any of the following mental health problems in the past month?
- Do you think social media use has a positive or negative impact on your mental health?
- Very positive
- Somewhat positive
- Somewhat negative
- Very negative
- In your opinion, which factors contribute to the negative impact of social media on mental health?
- Social comparison
- In your opinion, what interventions could be effective in reducing the negative impact of social media on mental health?
- Education on healthy social media use
- Counseling for mental health problems caused by social media
- Social media detox programs
- Regulation of social media use
Thank you for your participation!
Applications of Research Paper
Research papers have several applications in various fields, including:
- Advancing knowledge: Research papers contribute to the advancement of knowledge by generating new insights, theories, and findings that can inform future research and practice. They help to answer important questions, clarify existing knowledge, and identify areas that require further investigation.
- Informing policy: Research papers can inform policy decisions by providing evidence-based recommendations for policymakers. They can help to identify gaps in current policies, evaluate the effectiveness of interventions, and inform the development of new policies and regulations.
- Improving practice: Research papers can improve practice by providing evidence-based guidance for professionals in various fields, including medicine, education, business, and psychology. They can inform the development of best practices, guidelines, and standards of care that can improve outcomes for individuals and organizations.
- Educating students : Research papers are often used as teaching tools in universities and colleges to educate students about research methods, data analysis, and academic writing. They help students to develop critical thinking skills, research skills, and communication skills that are essential for success in many careers.
- Fostering collaboration: Research papers can foster collaboration among researchers, practitioners, and policymakers by providing a platform for sharing knowledge and ideas. They can facilitate interdisciplinary collaborations and partnerships that can lead to innovative solutions to complex problems.
When to Write Research Paper
Research papers are typically written when a person has completed a research project or when they have conducted a study and have obtained data or findings that they want to share with the academic or professional community. Research papers are usually written in academic settings, such as universities, but they can also be written in professional settings, such as research organizations, government agencies, or private companies.
Here are some common situations where a person might need to write a research paper:
- For academic purposes: Students in universities and colleges are often required to write research papers as part of their coursework, particularly in the social sciences, natural sciences, and humanities. Writing research papers helps students to develop research skills, critical thinking skills, and academic writing skills.
- For publication: Researchers often write research papers to publish their findings in academic journals or to present their work at academic conferences. Publishing research papers is an important way to disseminate research findings to the academic community and to establish oneself as an expert in a particular field.
- To inform policy or practice : Researchers may write research papers to inform policy decisions or to improve practice in various fields. Research findings can be used to inform the development of policies, guidelines, and best practices that can improve outcomes for individuals and organizations.
- To share new insights or ideas: Researchers may write research papers to share new insights or ideas with the academic or professional community. They may present new theories, propose new research methods, or challenge existing paradigms in their field.
Purpose of Research Paper
The purpose of a research paper is to present the results of a study or investigation in a clear, concise, and structured manner. Research papers are written to communicate new knowledge, ideas, or findings to a specific audience, such as researchers, scholars, practitioners, or policymakers. The primary purposes of a research paper are:
- To contribute to the body of knowledge : Research papers aim to add new knowledge or insights to a particular field or discipline. They do this by reporting the results of empirical studies, reviewing and synthesizing existing literature, proposing new theories, or providing new perspectives on a topic.
- To inform or persuade: Research papers are written to inform or persuade the reader about a particular issue, topic, or phenomenon. They present evidence and arguments to support their claims and seek to persuade the reader of the validity of their findings or recommendations.
- To advance the field: Research papers seek to advance the field or discipline by identifying gaps in knowledge, proposing new research questions or approaches, or challenging existing assumptions or paradigms. They aim to contribute to ongoing debates and discussions within a field and to stimulate further research and inquiry.
- To demonstrate research skills: Research papers demonstrate the author’s research skills, including their ability to design and conduct a study, collect and analyze data, and interpret and communicate findings. They also demonstrate the author’s ability to critically evaluate existing literature, synthesize information from multiple sources, and write in a clear and structured manner.
Characteristics of Research Paper
Research papers have several characteristics that distinguish them from other forms of academic or professional writing. Here are some common characteristics of research papers:
- Evidence-based: Research papers are based on empirical evidence, which is collected through rigorous research methods such as experiments, surveys, observations, or interviews. They rely on objective data and facts to support their claims and conclusions.
- Structured and organized: Research papers have a clear and logical structure, with sections such as introduction, literature review, methods, results, discussion, and conclusion. They are organized in a way that helps the reader to follow the argument and understand the findings.
- Formal and objective: Research papers are written in a formal and objective tone, with an emphasis on clarity, precision, and accuracy. They avoid subjective language or personal opinions and instead rely on objective data and analysis to support their arguments.
- Citations and references: Research papers include citations and references to acknowledge the sources of information and ideas used in the paper. They use a specific citation style, such as APA, MLA, or Chicago, to ensure consistency and accuracy.
- Peer-reviewed: Research papers are often peer-reviewed, which means they are evaluated by other experts in the field before they are published. Peer-review ensures that the research is of high quality, meets ethical standards, and contributes to the advancement of knowledge in the field.
- Objective and unbiased: Research papers strive to be objective and unbiased in their presentation of the findings. They avoid personal biases or preconceptions and instead rely on the data and analysis to draw conclusions.
Advantages of Research Paper
Research papers have many advantages, both for the individual researcher and for the broader academic and professional community. Here are some advantages of research papers:
- Contribution to knowledge: Research papers contribute to the body of knowledge in a particular field or discipline. They add new information, insights, and perspectives to existing literature and help advance the understanding of a particular phenomenon or issue.
- Opportunity for intellectual growth: Research papers provide an opportunity for intellectual growth for the researcher. They require critical thinking, problem-solving, and creativity, which can help develop the researcher’s skills and knowledge.
- Career advancement: Research papers can help advance the researcher’s career by demonstrating their expertise and contributions to the field. They can also lead to new research opportunities, collaborations, and funding.
- Academic recognition: Research papers can lead to academic recognition in the form of awards, grants, or invitations to speak at conferences or events. They can also contribute to the researcher’s reputation and standing in the field.
- Impact on policy and practice: Research papers can have a significant impact on policy and practice. They can inform policy decisions, guide practice, and lead to changes in laws, regulations, or procedures.
- Advancement of society: Research papers can contribute to the advancement of society by addressing important issues, identifying solutions to problems, and promoting social justice and equality.
Limitations of Research Paper
Research papers also have some limitations that should be considered when interpreting their findings or implications. Here are some common limitations of research papers:
- Limited generalizability: Research findings may not be generalizable to other populations, settings, or contexts. Studies often use specific samples or conditions that may not reflect the broader population or real-world situations.
- Potential for bias : Research papers may be biased due to factors such as sample selection, measurement errors, or researcher biases. It is important to evaluate the quality of the research design and methods used to ensure that the findings are valid and reliable.
- Ethical concerns: Research papers may raise ethical concerns, such as the use of vulnerable populations or invasive procedures. Researchers must adhere to ethical guidelines and obtain informed consent from participants to ensure that the research is conducted in a responsible and respectful manner.
- Limitations of methodology: Research papers may be limited by the methodology used to collect and analyze data. For example, certain research methods may not capture the complexity or nuance of a particular phenomenon, or may not be appropriate for certain research questions.
- Publication bias: Research papers may be subject to publication bias, where positive or significant findings are more likely to be published than negative or non-significant findings. This can skew the overall findings of a particular area of research.
- Time and resource constraints: Research papers may be limited by time and resource constraints, which can affect the quality and scope of the research. Researchers may not have access to certain data or resources, or may be unable to conduct long-term studies due to practical limitations.
About the author
Muhammad Hassan
Researcher, Academic Writer, Web developer
You may also like

How to Cite Research Paper – All Formats and...

Data Collection – Methods Types and Examples

Delimitations in Research – Types, Examples and...

Research Paper Format – Types, Examples and...

Research Process – Steps, Examples and Tips

Research Design – Types, Methods and Examples
Subscribe to the PwC Newsletter
Join the community, add a new evaluation result row, visual question answering (vqa).
778 papers with code • 66 benchmarks • 115 datasets
Visual Question Answering (VQA) is a task in computer vision that involves answering questions about an image. The goal of VQA is to teach machines to understand the content of an image and answer questions about it in natural language.
Image Source: visualqa.org

Benchmarks Add a Result

Most implemented papers
Grad-cam: visual explanations from deep networks via gradient-based localization.

For captioning and VQA, we show that even non-attention based models can localize inputs.
Bottom-Up and Top-Down Attention for Image Captioning and Visual Question Answering
peteanderson80/bottom-up-attention • CVPR 2018
Top-down visual attention mechanisms have been used extensively in image captioning and visual question answering (VQA) to enable deeper image understanding through fine-grained analysis and even multiple steps of reasoning.
ParlAI: A Dialog Research Software Platform

We introduce ParlAI (pronounced "par-lay"), an open-source software platform for dialog research implemented in Python, available at http://parl. ai.
VQA: Visual Question Answering
Given an image and a natural language question about the image, the task is to provide an accurate natural language answer.
A simple neural network module for relational reasoning
Relational reasoning is a central component of generally intelligent behavior, but has proven difficult for neural networks to learn.
Stacked Attention Networks for Image Question Answering
zcyang/imageqa-san • CVPR 2016
Thus, we develop a multiple-layer SAN in which we query an image multiple times to infer the answer progressively.
ECG Heartbeat Classification: A Deep Transferable Representation
Electrocardiogram (ECG) can be reliably used as a measure to monitor the functionality of the cardiovascular system.
Show, Ask, Attend, and Answer: A Strong Baseline For Visual Question Answering
This paper presents a new baseline for visual question answering task.
BLIP-2: Bootstrapping Language-Image Pre-training with Frozen Image Encoders and Large Language Models
The cost of vision-and-language pre-training has become increasingly prohibitive due to end-to-end training of large-scale models.
Dynamic Memory Networks for Visual and Textual Question Answering
Neural network architectures with memory and attention mechanisms exhibit certain reasoning capabilities required for question answering.
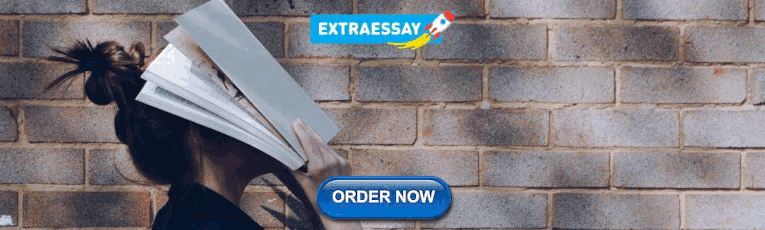
IMAGES
VIDEO
COMMENTS
Explore the latest full-text research PDFs, articles, conference papers, preprints and more on VISUAL BASIC PROGRAMMING. Find methods information, sources, references or conduct a literature ...
Visual basic , Visual Studio , Analysis of multimodal texts, in particular sequential visual narrative forms such as picturebooks , film and graphic novels , Visual Basic 6.0. CalHypso: An ArcGIS extension to calculate hypsometric curves and their statistical moments. Applications to drainage basin analysis in SE Spain.
Visual Basic. Visual Basic is one of the most widely used programming languages in the world. The major reason for its popularity is that it allows programmers to create Windows applications quickly and easily. The origins of Visual Basic are found in a programming language created in 1964 by John Kemeny and Thomas Kurtz.
beta-VAE: Learning Basic Visual Concepts with a Constrained Variational Framework. 6 code implementations • ICLR 2017 . Learning an interpretable factorised representation of the independent data generative factors of the world without supervision is an important precursor for the development of artificial intelligence that is able to learn and reason in the same way that humans do.
With the wizards included in the GUI designer, you can easily set formatting, grouping, charting, and other criteria. The research on Visual Basic Programming. connectivity with Crystal Reports is completely attached with database system. In this research we can attach programming language, database with Crystal Reports.
This paper presents a systematic literature review that examines the role of visual and textual programming languages when learning to program, particularly as a First Programming Language. ... Twenty-nine papers inform the first research question, with 24 informing the second. It is important to note that the level of contribution that some ...
YING BAI, PhD, is a Professor in the Department of Computer Science and Engineering at Johnson C. Smith University where he received the Grantsperson of the Year Award in 2009. A former senior software engineer in the field of automatic control and testing equipment, Dr. Bai is a Senior Member of IEEE and a member of ACM, and has published ten books and numerous papers on software engineering ...
With rapid advances in high-speed communication and computation, augmented reality (AR) and virtual reality (VR) are emerging as next-generation display platforms for deeper human-digital ...
London, U.K.: AltaMira Press. Visual and screen-based research practices have a long history in social-science, humanities, education, and creative-arts based disciplines as methods of qualitative research. While approaches may vary substantially across visual anthropology, sociology, history, media, or cultural studies, in each case visual ...
View Visual Basic 2010 Research Papers on Academia.edu for free.
Thus, designing for visual accessibility is a significant practical problem that should be informed by research on visual perception and spatial cognition. The work discussed in this paper presents an empirical approach to identifying when and how visual information is used to perceive and act on local and global features of spaces under ...
Visual analysis is the basic unit of art historical writing. Sources as varied as art magazines, scholarly books, and undergraduate research papers rely on concise and detailed visual analyses. You may encounter a visual analysis as an assignment itself; or you may write one as part of a longer research paper.
Create a spot-on reference in APA, MLA, Chicago, Harvard, and other styles. Consult the top 50 journal articles for your research on the topic 'Visual Basic (VBA).'. Next to every source in the list of references, there is an 'Add to bibliography' button. Press on it, and we will generate automatically the bibliographic reference to the chosen ...
The purpose of this paper is, therefore, to provide a basic guide for those academia and industry people who want to study, research, and develop data-driven automated and intelligent systems in the relevant areas based on machine learning techniques. The key contributions of this paper are listed as follows:
Blealtan/efficient-kan • • 30 Apr 2024. Inspired by the Kolmogorov-Arnold representation theorem, we propose Kolmogorov-Arnold Networks (KANs) as promising alternatives to Multi-Layer Perceptrons (MLPs). 2,576. 1.12 stars / hour. Paper. Code. Papers With Code highlights trending Machine Learning research and the code to implement it.
The File System Object (FSO) object model provides an object-based tool for working with folders and files. Using "object.method" syntax, it exposes a comprehensive set of properties and methods to perform file system operations such as... more. Download. by James Hitz. 2. Visual Basic 6.0 , File System Objects.
1. Paper. Code. Visual Prompting is the task of streamlining computer vision processes by harnessing the power of prompts, inspired by the breakthroughs of text prompting in NLP. This innovative approach involves using a few visual prompts to swiftly convert an unlabeled dataset into a deployed model, significantly reducing development time for ...
Definition: Research Paper is a written document that presents the author's original research, analysis, and interpretation of a specific topic or issue. It is typically based on Empirical Evidence, and may involve qualitative or quantitative research methods, or a combination of both. The purpose of a research paper is to contribute new ...
153 papers with code • 21 benchmarks • 26 datasets. Visual Object Tracking is an important research topic in computer vision, image understanding and pattern recognition. Given the initial state (centre location and scale) of a target in the first frame of a video sequence, the aim of Visual Object Tracking is to automatically obtain the ...
The creation of this benchmark dataset and the advances in object recognition that have been possible as a result are described, and the state-of-the-art computer vision accuracy with human accuracy is compared. Expand. 35,061. [PDF] Semantic Scholar extracted view of "Exploring the Usage of Pre-trained Features for Stereo Matching" by Jiawei ...
775 papers with code • 66 benchmarks • 115 datasets. Visual Question Answering (VQA) is a task in computer vision that involves answering questions about an image. The goal of VQA is to teach machines to understand the content of an image and answer questions about it in natural language. Image Source: visualqa.org.