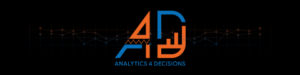
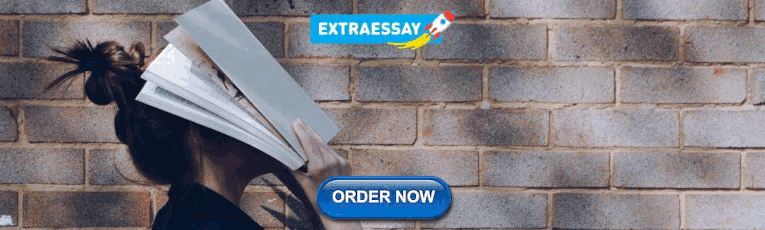
11 Tips For Writing a Dissertation Data Analysis
Since the evolution of the fourth industrial revolution – the Digital World; lots of data have surrounded us. There are terabytes of data around us or in data centers that need to be processed and used. The data needs to be appropriately analyzed to process it, and Dissertation data analysis forms its basis. If data analysis is valid and free from errors, the research outcomes will be reliable and lead to a successful dissertation.
Considering the complexity of many data analysis projects, it becomes challenging to get precise results if analysts are not familiar with data analysis tools and tests properly. The analysis is a time-taking process that starts with collecting valid and relevant data and ends with the demonstration of error-free results.
So, in today’s topic, we will cover the need to analyze data, dissertation data analysis, and mainly the tips for writing an outstanding data analysis dissertation. If you are a doctoral student and plan to perform dissertation data analysis on your data, make sure that you give this article a thorough read for the best tips!
What is Data Analysis in Dissertation?
Dissertation Data Analysis is the process of understanding, gathering, compiling, and processing a large amount of data. Then identifying common patterns in responses and critically examining facts and figures to find the rationale behind those outcomes.
Even f you have the data collected and compiled in the form of facts and figures, it is not enough for proving your research outcomes. There is still a need to apply dissertation data analysis on your data; to use it in the dissertation. It provides scientific support to the thesis and conclusion of the research.
Data Analysis Tools
There are plenty of indicative tests used to analyze data and infer relevant results for the discussion part. Following are some tests used to perform analysis of data leading to a scientific conclusion:
11 Most Useful Tips for Dissertation Data Analysis
Doctoral students need to perform dissertation data analysis and then dissertation to receive their degree. Many Ph.D. students find it hard to do dissertation data analysis because they are not trained in it.
1. Dissertation Data Analysis Services
The first tip applies to those students who can afford to look for help with their dissertation data analysis work. It’s a viable option, and it can help with time management and with building the other elements of the dissertation with much detail.
Dissertation Analysis services are professional services that help doctoral students with all the basics of their dissertation work, from planning, research and clarification, methodology, dissertation data analysis and review, literature review, and final powerpoint presentation.
One great reference for dissertation data analysis professional services is Statistics Solutions , they’ve been around for over 22 years helping students succeed in their dissertation work. You can find the link to their website here .
For a proper dissertation data analysis, the student should have a clear understanding and statistical knowledge. Through this knowledge and experience, a student can perform dissertation analysis on their own.
Following are some helpful tips for writing a splendid dissertation data analysis:
2. Relevance of Collected Data
If the data is irrelevant and not appropriate, you might get distracted from the point of focus. To show the reader that you can critically solve the problem, make sure that you write a theoretical proposition regarding the selection and analysis of data.
3. Data Analysis
For analysis, it is crucial to use such methods that fit best with the types of data collected and the research objectives. Elaborate on these methods and the ones that justify your data collection methods thoroughly. Make sure to make the reader believe that you did not choose your method randomly. Instead, you arrived at it after critical analysis and prolonged research.
On the other hand, quantitative analysis refers to the analysis and interpretation of facts and figures – to build reasoning behind the advent of primary findings. An assessment of the main results and the literature review plays a pivotal role in qualitative and quantitative analysis.
The overall objective of data analysis is to detect patterns and inclinations in data and then present the outcomes implicitly. It helps in providing a solid foundation for critical conclusions and assisting the researcher to complete the dissertation proposal.
4. Qualitative Data Analysis
Qualitative data refers to data that does not involve numbers. You are required to carry out an analysis of the data collected through experiments, focus groups, and interviews. This can be a time-taking process because it requires iterative examination and sometimes demanding the application of hermeneutics. Note that using qualitative technique doesn’t only mean generating good outcomes but to unveil more profound knowledge that can be transferrable.
Presenting qualitative data analysis in a dissertation can also be a challenging task. It contains longer and more detailed responses. Placing such comprehensive data coherently in one chapter of the dissertation can be difficult due to two reasons. Firstly, we cannot figure out clearly which data to include and which one to exclude. Secondly, unlike quantitative data, it becomes problematic to present data in figures and tables. Making information condensed into a visual representation is not possible. As a writer, it is of essence to address both of these challenges.
Qualitative Data Analysis Methods
Following are the methods used to perform quantitative data analysis.
- Deductive Method
This method involves analyzing qualitative data based on an argument that a researcher already defines. It’s a comparatively easy approach to analyze data. It is suitable for the researcher with a fair idea about the responses they are likely to receive from the questionnaires.
- Inductive Method
In this method, the researcher analyzes the data not based on any predefined rules. It is a time-taking process used by students who have very little knowledge of the research phenomenon.
5. Quantitative Data Analysis
Quantitative data contains facts and figures obtained from scientific research and requires extensive statistical analysis. After collection and analysis, you will be able to conclude. Generic outcomes can be accepted beyond the sample by assuming that it is representative – one of the preliminary checkpoints to carry out in your analysis to a larger group. This method is also referred to as the “scientific method”, gaining its roots from natural sciences.
The Presentation of quantitative data depends on the domain to which it is being presented. It is beneficial to consider your audience while writing your findings. Quantitative data for hard sciences might require numeric inputs and statistics. As for natural sciences , such comprehensive analysis is not required.
Quantitative Analysis Methods
Following are some of the methods used to perform quantitative data analysis.
- Trend analysis: This corresponds to a statistical analysis approach to look at the trend of quantitative data collected over a considerable period.
- Cross-tabulation: This method uses a tabula way to draw readings among data sets in research.
- Conjoint analysis : Quantitative data analysis method that can collect and analyze advanced measures. These measures provide a thorough vision about purchasing decisions and the most importantly, marked parameters.
- TURF analysis: This approach assesses the total market reach of a service or product or a mix of both.
- Gap analysis: It utilizes the side-by-side matrix to portray quantitative data, which captures the difference between the actual and expected performance.
- Text analysis: In this method, innovative tools enumerate open-ended data into easily understandable data.
6. Data Presentation Tools
Since large volumes of data need to be represented, it becomes a difficult task to present such an amount of data in coherent ways. To resolve this issue, consider all the available choices you have, such as tables, charts, diagrams, and graphs.
Tables help in presenting both qualitative and quantitative data concisely. While presenting data, always keep your reader in mind. Anything clear to you may not be apparent to your reader. So, constantly rethink whether your data presentation method is understandable to someone less conversant with your research and findings. If the answer is “No”, you may need to rethink your Presentation.
7. Include Appendix or Addendum
After presenting a large amount of data, your dissertation analysis part might get messy and look disorganized. Also, you would not be cutting down or excluding the data you spent days and months collecting. To avoid this, you should include an appendix part.
The data you find hard to arrange within the text, include that in the appendix part of a dissertation . And place questionnaires, copies of focus groups and interviews, and data sheets in the appendix. On the other hand, one must put the statistical analysis and sayings quoted by interviewees within the dissertation.
8. Thoroughness of Data
It is a common misconception that the data presented is self-explanatory. Most of the students provide the data and quotes and think that it is enough and explaining everything. It is not sufficient. Rather than just quoting everything, you should analyze and identify which data you will use to approve or disapprove your standpoints.
Thoroughly demonstrate the ideas and critically analyze each perspective taking care of the points where errors can occur. Always make sure to discuss the anomalies and strengths of your data to add credibility to your research.
9. Discussing Data
Discussion of data involves elaborating the dimensions to classify patterns, themes, and trends in presented data. In addition, to balancing, also take theoretical interpretations into account. Discuss the reliability of your data by assessing their effect and significance. Do not hide the anomalies. While using interviews to discuss the data, make sure you use relevant quotes to develop a strong rationale.
It also involves answering what you are trying to do with the data and how you have structured your findings. Once you have presented the results, the reader will be looking for interpretation. Hence, it is essential to deliver the understanding as soon as you have submitted your data.
10. Findings and Results
Findings refer to the facts derived after the analysis of collected data. These outcomes should be stated; clearly, their statements should tightly support your objective and provide logical reasoning and scientific backing to your point. This part comprises of majority part of the dissertation.
In the finding part, you should tell the reader what they are looking for. There should be no suspense for the reader as it would divert their attention. State your findings clearly and concisely so that they can get the idea of what is more to come in your dissertation.
11. Connection with Literature Review
At the ending of your data analysis in the dissertation, make sure to compare your data with other published research. In this way, you can identify the points of differences and agreements. Check the consistency of your findings if they meet your expectations—lookup for bottleneck position. Analyze and discuss the reasons behind it. Identify the key themes, gaps, and the relation of your findings with the literature review. In short, you should link your data with your research question, and the questions should form a basis for literature.
The Role of Data Analytics at The Senior Management Level
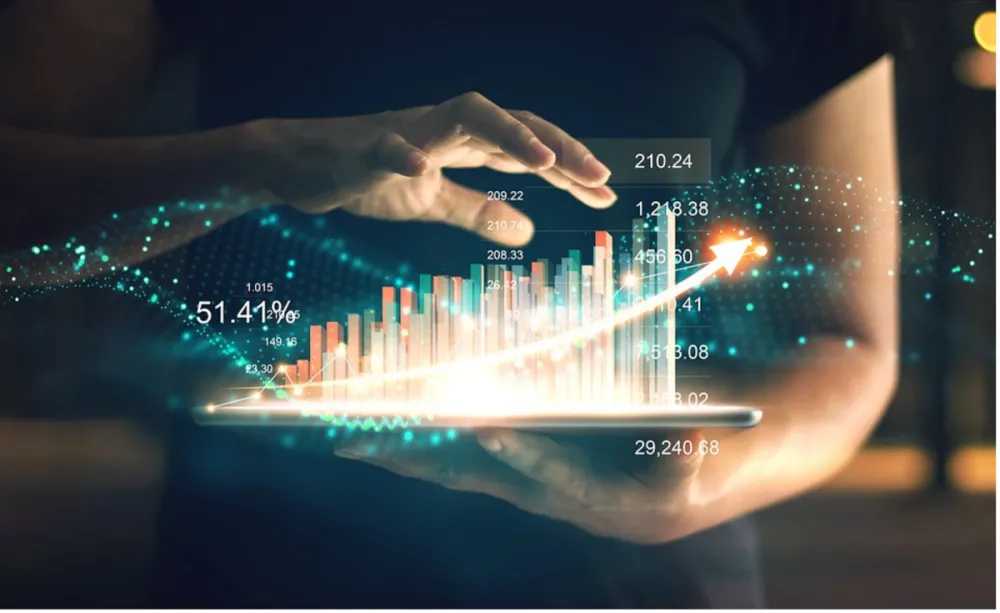
From small and medium-sized businesses to Fortune 500 conglomerates, the success of a modern business is now increasingly tied to how the company implements its data infrastructure and data-based decision-making. According
The Decision-Making Model Explained (In Plain Terms)

Any form of the systematic decision-making process is better enhanced with data. But making sense of big data or even small data analysis when venturing into a decision-making process might
13 Reasons Why Data Is Important in Decision Making
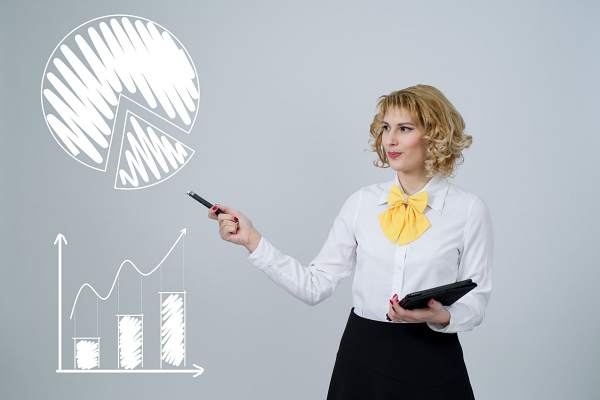
Wrapping Up
Writing data analysis in the dissertation involves dedication, and its implementations demand sound knowledge and proper planning. Choosing your topic, gathering relevant data, analyzing it, presenting your data and findings correctly, discussing the results, connecting with the literature and conclusions are milestones in it. Among these checkpoints, the Data analysis stage is most important and requires a lot of keenness.
In this article, we thoroughly looked at the tips that prove valuable for writing a data analysis in a dissertation. Make sure to give this article a thorough read before you write data analysis in the dissertation leading to the successful future of your research.
Oxbridge Essays. Top 10 Tips for Writing a Dissertation Data Analysis.
Emidio Amadebai
As an IT Engineer, who is passionate about learning and sharing. I have worked and learned quite a bit from Data Engineers, Data Analysts, Business Analysts, and Key Decision Makers almost for the past 5 years. Interested in learning more about Data Science and How to leverage it for better decision-making in my business and hopefully help you do the same in yours.
Recent Posts
Causal vs Evidential Decision-making (How to Make Businesses More Effective)
In today’s fast-paced business landscape, it is crucial to make informed decisions to stay in the competition which makes it important to understand the concept of the different characteristics and...
Bootstrapping vs. Boosting
Over the past decade, the field of machine learning has witnessed remarkable advancements in predictive techniques and ensemble learning methods. Ensemble techniques are very popular in machine...

- +44 7897 053596
- [email protected]
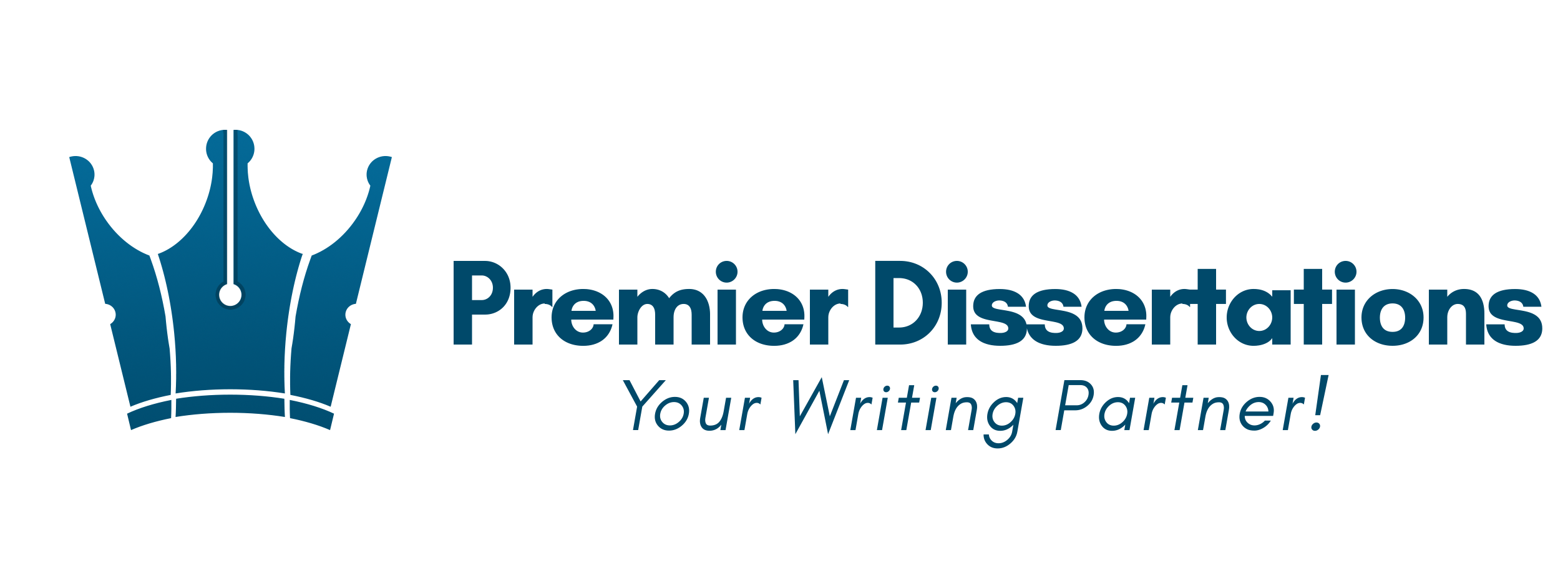
Get an experienced writer start working
Review our examples before placing an order, learn how to draft academic papers, a step-by-step guide to dissertation data analysis.
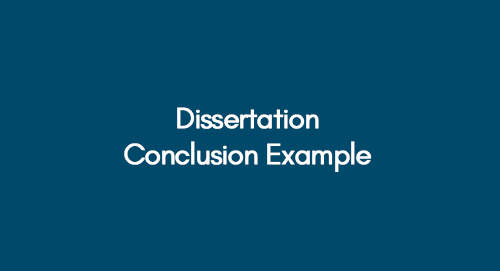
How to Write a Dissertation Conclusion? | Tips & Examples
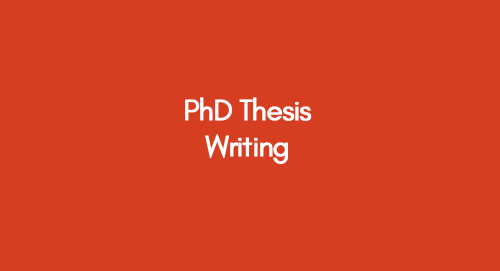
What is PhD Thesis Writing? | Beginner’s Guide
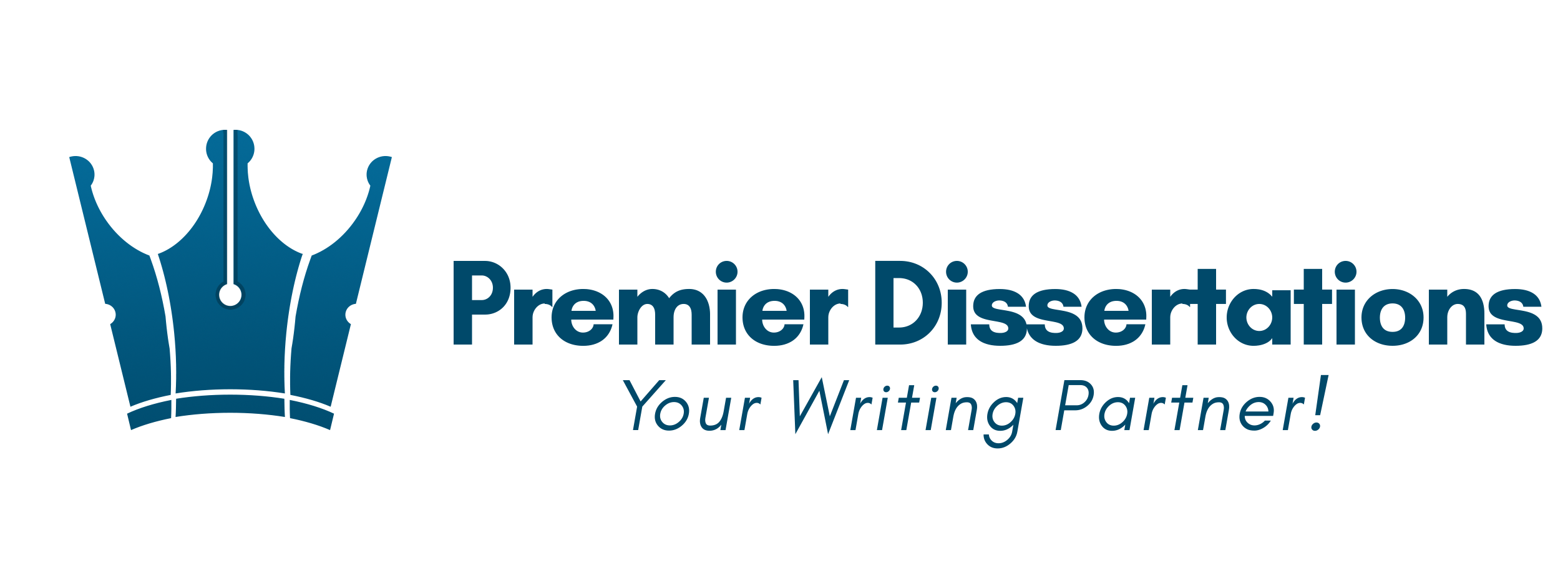
A data analysis dissertation is a complex and challenging project requiring significant time, effort, and expertise. Fortunately, it is possible to successfully complete a data analysis dissertation with careful planning and execution.
As a student, you must know how important it is to have a strong and well-written dissertation, especially regarding data analysis. Proper data analysis is crucial to the success of your research and can often make or break your dissertation.
To get a better understanding, you may review the data analysis dissertation examples listed below;
- Impact of Leadership Style on the Job Satisfaction of Nurses
- Effect of Brand Love on Consumer Buying Behaviour in Dietary Supplement Sector
- An Insight Into Alternative Dispute Resolution
- An Investigation of Cyberbullying and its Impact on Adolescent Mental Health in UK
3-Step Dissertation Process!

Get 3+ Topics

Dissertation Proposal

Get Final Dissertation
Types of data analysis for dissertation.
The various types of data Analysis in a Dissertation are as follows;
1. Qualitative Data Analysis
Qualitative data analysis is a type of data analysis that involves analyzing data that cannot be measured numerically. This data type includes interviews, focus groups, and open-ended surveys. Qualitative data analysis can be used to identify patterns and themes in the data.
2. Quantitative Data Analysis
Quantitative data analysis is a type of data analysis that involves analyzing data that can be measured numerically. This data type includes test scores, income levels, and crime rates. Quantitative data analysis can be used to test hypotheses and to look for relationships between variables.
3. Descriptive Data Analysis
Descriptive data analysis is a type of data analysis that involves describing the characteristics of a dataset. This type of data analysis summarizes the main features of a dataset.
4. Inferential Data Analysis
Inferential data analysis is a type of data analysis that involves making predictions based on a dataset. This type of data analysis can be used to test hypotheses and make predictions about future events.
5. Exploratory Data Analysis
Exploratory data analysis is a type of data analysis that involves exploring a data set to understand it better. This type of data analysis can identify patterns and relationships in the data.
Time Period to Plan and Complete a Data Analysis Dissertation?
When planning dissertation data analysis, it is important to consider the dissertation methodology structure and time series analysis as they will give you an understanding of how long each stage will take. For example, using a qualitative research method, your data analysis will involve coding and categorizing your data.
This can be time-consuming, so allowing enough time in your schedule is important. Once you have coded and categorized your data, you will need to write up your findings. Again, this can take some time, so factor this into your schedule.
Finally, you will need to proofread and edit your dissertation before submitting it. All told, a data analysis dissertation can take anywhere from several weeks to several months to complete, depending on the project’s complexity. Therefore, starting planning early and allowing enough time in your schedule to complete the task is important.
Essential Strategies for Data Analysis Dissertation
A. Planning
The first step in any dissertation is planning. You must decide what you want to write about and how you want to structure your argument. This planning will involve deciding what data you want to analyze and what methods you will use for a data analysis dissertation.
B. Prototyping
Once you have a plan for your dissertation, it’s time to start writing. However, creating a prototype is important before diving head-first into writing your dissertation. A prototype is a rough draft of your argument that allows you to get feedback from your advisor and committee members. This feedback will help you fine-tune your argument before you start writing the final version of your dissertation.
C. Executing
After you have created a plan and prototype for your data analysis dissertation, it’s time to start writing the final version. This process will involve collecting and analyzing data and writing up your results. You will also need to create a conclusion section that ties everything together.
D. Presenting
The final step in acing your data analysis dissertation is presenting it to your committee. This presentation should be well-organized and professionally presented. During the presentation, you’ll also need to be ready to respond to questions concerning your dissertation.
Data Analysis Tools
Numerous suggestive tools are employed to assess the data and deduce pertinent findings for the discussion section. The tools used to analyze data and get a scientific conclusion are as follows:
a. Excel
Excel is a spreadsheet program part of the Microsoft Office productivity software suite. Excel is a powerful tool that can be used for various data analysis tasks, such as creating charts and graphs, performing mathematical calculations, and sorting and filtering data.
b. Google Sheets
Google Sheets is a free online spreadsheet application that is part of the Google Drive suite of productivity software. Google Sheets is similar to Excel in terms of functionality, but it also has some unique features, such as the ability to collaborate with other users in real-time.
c. SPSS
SPSS is a statistical analysis software program commonly used in the social sciences. SPSS can be used for various data analysis tasks, such as hypothesis testing, factor analysis, and regression analysis.
d. STATA
STATA is a statistical analysis software program commonly used in the sciences and economics. STATA can be used for data management, statistical modelling, descriptive statistics analysis, and data visualization tasks.
SAS is a commercial statistical analysis software program used by businesses and organizations worldwide. SAS can be used for predictive modelling, market research, and fraud detection.
R is a free, open-source statistical programming language popular among statisticians and data scientists. R can be used for tasks such as data wrangling, machine learning, and creating complex visualizations.
g. Python
A variety of applications may be used using the distinctive programming language Python, including web development, scientific computing, and artificial intelligence. Python also has a number of modules and libraries that can be used for data analysis tasks, such as numerical computing, statistical modelling, and data visualization.
Testimonials
Very satisfied students
This is our reason for working. We want to make all students happy, every day. Review us on Sitejabber
Tips to Compose a Successful Data Analysis Dissertation
a. Choose a Topic You’re Passionate About
The first step to writing a successful data analysis dissertation is to choose a topic you’re passionate about. Not only will this make the research and writing process more enjoyable, but it will also ensure that you produce a high-quality paper.
Choose a topic that is particular enough to be covered in your paper’s scope but not so specific that it will be challenging to obtain enough evidence to substantiate your arguments.
b. Do Your Research
data analysis in research is an important part of academic writing. Once you’ve selected a topic, it’s time to begin your research. Be sure to consult with your advisor or supervisor frequently during this stage to ensure that you are on the right track. In addition to secondary sources such as books, journal articles, and reports, you should also consider conducting primary research through surveys or interviews. This will give you first-hand insights into your topic that can be invaluable when writing your paper.
c. Develop a Strong Thesis Statement
After you’ve done your research, it’s time to start developing your thesis statement. It is arguably the most crucial part of your entire paper, so take care to craft a clear and concise statement that encapsulates the main argument of your paper.
Remember that your thesis statement should be arguable—that is, it should be capable of being disputed by someone who disagrees with your point of view. If your thesis statement is not arguable, it will be difficult to write a convincing paper.
d. Write a Detailed Outline
Once you have developed a strong thesis statement, the next step is to write a detailed outline of your paper. This will offer you a direction to write in and guarantee that your paper makes sense from beginning to end.
Your outline should include an introduction, in which you state your thesis statement; several body paragraphs, each devoted to a different aspect of your argument; and a conclusion, in which you restate your thesis and summarize the main points of your paper.
e. Write Your First Draft
With your outline in hand, it’s finally time to start writing your first draft. At this stage, don’t worry about perfecting your grammar or making sure every sentence is exactly right—focus on getting all of your ideas down on paper (or onto the screen). Once you have completed your first draft, you can revise it for style and clarity.
And there you have it! Following these simple tips can increase your chances of success when writing your data analysis dissertation. Just remember to start early, give yourself plenty of time to research and revise, and consult with your supervisor frequently throughout the process.
How Does It Work ?
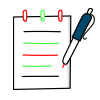
Fill the Form
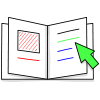
Writer Starts Working
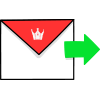
3+ Topics Emailed!
Studying the above examples gives you valuable insight into the structure and content that should be included in your own data analysis dissertation. You can also learn how to effectively analyze and present your data and make a lasting impact on your readers.
In addition to being a useful resource for completing your dissertation, these examples can also serve as a valuable reference for future academic writing projects. By following these examples and understanding their principles, you can improve your data analysis skills and increase your chances of success in your academic career.
You may also contact Premier Dissertations to develop your data analysis dissertation.
For further assistance, some other resources in the dissertation writing section are shared below;
How Do You Select the Right Data Analysis
How to Write Data Analysis For A Dissertation?
How to Develop a Conceptual Framework in Dissertation?
What is a Hypothesis in a Dissertation?
Get an Immediate Response
Discuss your requirments with our writers
WhatsApp Us Email Us Chat with Us
Get 3+ Free Dissertation Topics within 24 hours?
Your Number
Academic Level Select Academic Level Undergraduate Masters PhD
Area of Research
admin farhan
Related posts.
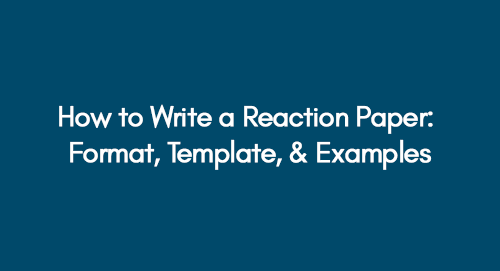
How to Write a Reaction Paper: Format, Template, & Examples
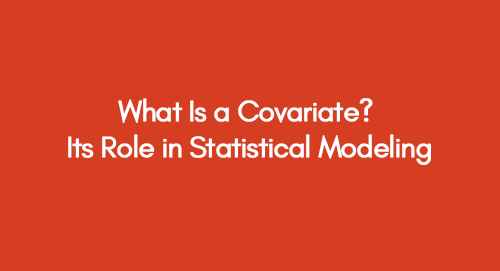
What Is a Covariate? Its Role in Statistical Modeling
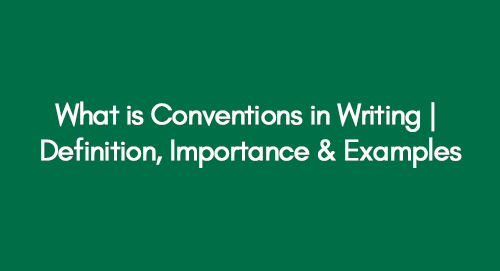
What is Conventions in Writing | Definition, Importance & Examples
Comments are closed.
Reference management. Clean and simple.
How to collect data for your thesis
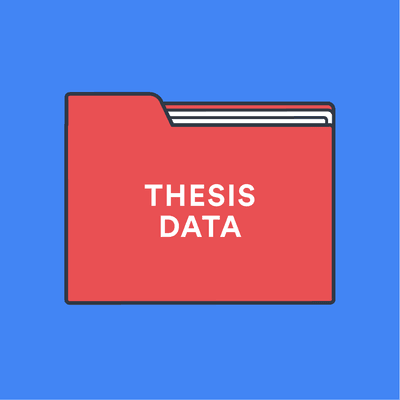
Collecting theoretical data
Search for theses on your topic, use content-sharing platforms, collecting empirical data, qualitative vs. quantitative data, frequently asked questions about gathering data for your thesis, related articles.
After choosing a topic for your thesis , you’ll need to start gathering data. In this article, we focus on how to effectively collect theoretical and empirical data.
Empirical data : unique research that may be quantitative, qualitative, or mixed.
Theoretical data : secondary, scholarly sources like books and journal articles that provide theoretical context for your research.
Thesis : the culminating, multi-chapter project for a bachelor’s, master’s, or doctoral degree.
Qualitative data : info that cannot be measured, like observations and interviews .
Quantitative data : info that can be measured and written with numbers.
At this point in your academic life, you are already acquainted with the ways of finding potential references. Some obvious sources of theoretical material are:
- edited volumes
- conference proceedings
- online databases like Google Scholar , ERIC , or Scopus
You can also take a look at the top list of academic search engines .
Looking at other theses on your topic can help you see what approaches have been taken and what aspects other writers have focused on. Pay close attention to the list of references and follow the bread-crumbs back to the original theories and specialized authors.
Another method for gathering theoretical data is to read through content-sharing platforms. Many people share their papers and writings on these sites. You can either hunt sources, get some inspiration for your own work or even learn new angles of your topic.
Some popular content sharing sites are:
With these sites, you have to check the credibility of the sources. You can usually rely on the content, but we recommend double-checking just to be sure. Take a look at our guide on what are credible sources?
The more you know, the better. The guide, " How to undertake a literature search and review for dissertations and final year projects ," will give you all the tools needed for finding literature .
In order to successfully collect empirical data, you have to choose first what type of data you want as an outcome. There are essentially two options, qualitative or quantitative data. Many people mistake one term with the other, so it’s important to understand the differences between qualitative and quantitative research .
Boiled down, qualitative data means words and quantitative means numbers. Both types are considered primary sources . Whichever one adapts best to your research will define the type of methodology to carry out, so choose wisely.
In the end, having in mind what type of outcome you intend and how much time you count on will lead you to choose the best type of empirical data for your research. For a detailed description of each methodology type mentioned above, read more about collecting data .
Once you gather enough theoretical and empirical data, you will need to start writing. But before the actual writing part, you have to structure your thesis to avoid getting lost in the sea of information. Take a look at our guide on how to structure your thesis for some tips and tricks.
The key to knowing what type of data you should collect for your thesis is knowing in advance the type of outcome you intend to have, and the amount of time you count with.
Some obvious sources of theoretical material are journals, libraries and online databases like Google Scholar , ERIC or Scopus , or take a look at the top list of academic search engines . You can also search for theses on your topic or read content sharing platforms, like Medium , Issuu , or Slideshare .
To gather empirical data, you have to choose first what type of data you want. There are two options, qualitative or quantitative data. You can gather data through observations, interviews, focus groups, or with surveys, tests, and existing databases.
Qualitative data means words, information that cannot be measured. It may involve multimedia material or non-textual data. This type of data claims to be detailed, nuanced and contextual.
Quantitative data means numbers, information that can be measured and written with numbers. This type of data claims to be credible, scientific and exact.
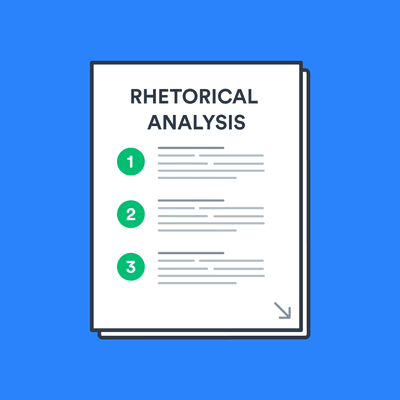
- Cookies & Privacy
- GETTING STARTED
- Introduction
- FUNDAMENTALS

Getting to the main article
Choosing your route
Setting research questions/ hypotheses
Assessment point
Building the theoretical case
Setting your research strategy
Data collection
Data analysis
Data analysis techniques
In STAGE NINE: Data analysis , we discuss the data you will have collected during STAGE EIGHT: Data collection . However, before you collect your data, having followed the research strategy you set out in this STAGE SIX , it is useful to think about the data analysis techniques you may apply to your data when it is collected.
The statistical tests that are appropriate for your dissertation will depend on (a) the research questions/hypotheses you have set, (b) the research design you are using, and (c) the nature of your data. You should already been clear about your research questions/hypotheses from STAGE THREE: Setting research questions and/or hypotheses , as well as knowing the goal of your research design from STEP TWO: Research design in this STAGE SIX: Setting your research strategy . These two pieces of information - your research questions/hypotheses and research design - will let you know, in principle , the statistical tests that may be appropriate to run on your data in order to answer your research questions.
We highlight the words in principle and may because the most appropriate statistical test to run on your data not only depend on your research questions/hypotheses and research design, but also the nature of your data . As you should have identified in STEP THREE: Research methods , and in the article, Types of variables , in the Fundamentals part of Lærd Dissertation, (a) not all data is the same, and (b) not all variables are measured in the same way (i.e., variables can be dichotomous, ordinal or continuous). In addition, not all data is normal , nor is the data when comparing groups necessarily equal , terms we explain in the Data Analysis section in the Fundamentals part of Lærd Dissertation. As a result, you might think that running a particular statistical test is correct at this point of setting your research strategy (e.g., a statistical test called a dependent t-test ), based on the research questions/hypotheses you have set, but when you collect your data (i.e., during STAGE EIGHT: Data collection ), the data may fail certain assumptions that are important to such a statistical test (i.e., normality and homogeneity of variance ). As a result, you have to run another statistical test (e.g., a Wilcoxon signed-rank test instead of a dependent t-test ).
At this stage in the dissertation process, it is important, or at the very least, useful to think about the data analysis techniques you may apply to your data when it is collected. We suggest that you do this for two reasons:
REASON A Supervisors sometimes expect you to know what statistical analysis you will perform at this stage of the dissertation process
This is not always the case, but if you have had to write a Dissertation Proposal or Ethics Proposal , there is sometimes an expectation that you explain the type of data analysis that you plan to carry out. An understanding of the data analysis that you will carry out on your data can also be an expected component of the Research Strategy chapter of your dissertation write-up (i.e., usually Chapter Three: Research Strategy ). Therefore, it is a good time to think about the data analysis process if you plan to start writing up this chapter at this stage.
REASON B It takes time to get your head around data analysis
When you come to analyse your data in STAGE NINE: Data analysis , you will need to think about (a) selecting the correct statistical tests to perform on your data, (b) running these tests on your data using a statistics package such as SPSS, and (c) learning how to interpret the output from such statistical tests so that you can answer your research questions or hypotheses. Whilst we show you how to do this for a wide range of scenarios in the in the Data Analysis section in the Fundamentals part of Lærd Dissertation, it can be a time consuming process. Unless you took an advanced statistics module/option as part of your degree (i.e., not just an introductory course to statistics, which are often taught in undergraduate and master?s degrees), it can take time to get your head around data analysis. Starting this process at this stage (i.e., STAGE SIX: Research strategy ), rather than waiting until you finish collecting your data (i.e., STAGE EIGHT: Data collection ) is a sensible approach.
Final thoughts...
Setting the research strategy for your dissertation required you to describe, explain and justify the research paradigm, quantitative research design, research method(s), sampling strategy, and approach towards research ethics and data analysis that you plan to follow, as well as determine how you will ensure the research quality of your findings so that you can effectively answer your research questions/hypotheses. However, from a practical perspective, just remember that the main goal of STAGE SIX: Research strategy is to have a clear research strategy that you can implement (i.e., operationalize ). After all, if you are unable to clearly follow your plan and carry out your research in the field, you will struggle to answer your research questions/hypotheses. Once you are sure that you have a clear plan, it is a good idea to take a step back, speak with your supervisor, and assess where you are before moving on to collect data. Therefore, when you are ready, proceed to STAGE SEVEN: Assessment point .
Have a language expert improve your writing
Run a free plagiarism check in 10 minutes, automatically generate references for free.
- Knowledge Base
- Methodology
- Data Collection Methods | Step-by-Step Guide & Examples
Data Collection Methods | Step-by-Step Guide & Examples
Published on 4 May 2022 by Pritha Bhandari .
Data collection is a systematic process of gathering observations or measurements. Whether you are performing research for business, governmental, or academic purposes, data collection allows you to gain first-hand knowledge and original insights into your research problem .
While methods and aims may differ between fields, the overall process of data collection remains largely the same. Before you begin collecting data, you need to consider:
- The aim of the research
- The type of data that you will collect
- The methods and procedures you will use to collect, store, and process the data
To collect high-quality data that is relevant to your purposes, follow these four steps.
Table of contents
Step 1: define the aim of your research, step 2: choose your data collection method, step 3: plan your data collection procedures, step 4: collect the data, frequently asked questions about data collection.
Before you start the process of data collection, you need to identify exactly what you want to achieve. You can start by writing a problem statement : what is the practical or scientific issue that you want to address, and why does it matter?
Next, formulate one or more research questions that precisely define what you want to find out. Depending on your research questions, you might need to collect quantitative or qualitative data :
- Quantitative data is expressed in numbers and graphs and is analysed through statistical methods .
- Qualitative data is expressed in words and analysed through interpretations and categorisations.
If your aim is to test a hypothesis , measure something precisely, or gain large-scale statistical insights, collect quantitative data. If your aim is to explore ideas, understand experiences, or gain detailed insights into a specific context, collect qualitative data.
If you have several aims, you can use a mixed methods approach that collects both types of data.
- Your first aim is to assess whether there are significant differences in perceptions of managers across different departments and office locations.
- Your second aim is to gather meaningful feedback from employees to explore new ideas for how managers can improve.
Prevent plagiarism, run a free check.
Based on the data you want to collect, decide which method is best suited for your research.
- Experimental research is primarily a quantitative method.
- Interviews , focus groups , and ethnographies are qualitative methods.
- Surveys , observations, archival research, and secondary data collection can be quantitative or qualitative methods.
Carefully consider what method you will use to gather data that helps you directly answer your research questions.
When you know which method(s) you are using, you need to plan exactly how you will implement them. What procedures will you follow to make accurate observations or measurements of the variables you are interested in?
For instance, if you’re conducting surveys or interviews, decide what form the questions will take; if you’re conducting an experiment, make decisions about your experimental design .
Operationalisation
Sometimes your variables can be measured directly: for example, you can collect data on the average age of employees simply by asking for dates of birth. However, often you’ll be interested in collecting data on more abstract concepts or variables that can’t be directly observed.
Operationalisation means turning abstract conceptual ideas into measurable observations. When planning how you will collect data, you need to translate the conceptual definition of what you want to study into the operational definition of what you will actually measure.
- You ask managers to rate their own leadership skills on 5-point scales assessing the ability to delegate, decisiveness, and dependability.
- You ask their direct employees to provide anonymous feedback on the managers regarding the same topics.
You may need to develop a sampling plan to obtain data systematically. This involves defining a population , the group you want to draw conclusions about, and a sample, the group you will actually collect data from.
Your sampling method will determine how you recruit participants or obtain measurements for your study. To decide on a sampling method you will need to consider factors like the required sample size, accessibility of the sample, and time frame of the data collection.
Standardising procedures
If multiple researchers are involved, write a detailed manual to standardise data collection procedures in your study.
This means laying out specific step-by-step instructions so that everyone in your research team collects data in a consistent way – for example, by conducting experiments under the same conditions and using objective criteria to record and categorise observations.
This helps ensure the reliability of your data, and you can also use it to replicate the study in the future.
Creating a data management plan
Before beginning data collection, you should also decide how you will organise and store your data.
- If you are collecting data from people, you will likely need to anonymise and safeguard the data to prevent leaks of sensitive information (e.g. names or identity numbers).
- If you are collecting data via interviews or pencil-and-paper formats, you will need to perform transcriptions or data entry in systematic ways to minimise distortion.
- You can prevent loss of data by having an organisation system that is routinely backed up.
Finally, you can implement your chosen methods to measure or observe the variables you are interested in.
The closed-ended questions ask participants to rate their manager’s leadership skills on scales from 1 to 5. The data produced is numerical and can be statistically analysed for averages and patterns.
To ensure that high-quality data is recorded in a systematic way, here are some best practices:
- Record all relevant information as and when you obtain data. For example, note down whether or how lab equipment is recalibrated during an experimental study.
- Double-check manual data entry for errors.
- If you collect quantitative data, you can assess the reliability and validity to get an indication of your data quality.
Data collection is the systematic process by which observations or measurements are gathered in research. It is used in many different contexts by academics, governments, businesses, and other organisations.
When conducting research, collecting original data has significant advantages:
- You can tailor data collection to your specific research aims (e.g., understanding the needs of your consumers or user testing your website).
- You can control and standardise the process for high reliability and validity (e.g., choosing appropriate measurements and sampling methods ).
However, there are also some drawbacks: data collection can be time-consuming, labour-intensive, and expensive. In some cases, it’s more efficient to use secondary data that has already been collected by someone else, but the data might be less reliable.
Quantitative research deals with numbers and statistics, while qualitative research deals with words and meanings.
Quantitative methods allow you to test a hypothesis by systematically collecting and analysing data, while qualitative methods allow you to explore ideas and experiences in depth.
Reliability and validity are both about how well a method measures something:
- Reliability refers to the consistency of a measure (whether the results can be reproduced under the same conditions).
- Validity refers to the accuracy of a measure (whether the results really do represent what they are supposed to measure).
If you are doing experimental research , you also have to consider the internal and external validity of your experiment.
In mixed methods research , you use both qualitative and quantitative data collection and analysis methods to answer your research question .
Operationalisation means turning abstract conceptual ideas into measurable observations.
For example, the concept of social anxiety isn’t directly observable, but it can be operationally defined in terms of self-rating scores, behavioural avoidance of crowded places, or physical anxiety symptoms in social situations.
Before collecting data , it’s important to consider how you will operationalise the variables that you want to measure.
Cite this Scribbr article
If you want to cite this source, you can copy and paste the citation or click the ‘Cite this Scribbr article’ button to automatically add the citation to our free Reference Generator.
Bhandari, P. (2022, May 04). Data Collection Methods | Step-by-Step Guide & Examples. Scribbr. Retrieved 3 June 2024, from https://www.scribbr.co.uk/research-methods/data-collection-guide/
Is this article helpful?
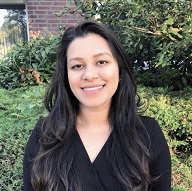
Pritha Bhandari
Other students also liked, qualitative vs quantitative research | examples & methods, triangulation in research | guide, types, examples, what is a conceptual framework | tips & examples.
- Privacy Policy

Home » Dissertation – Format, Example and Template
Dissertation – Format, Example and Template
Table of Contents

Dissertation
Definition:
Dissertation is a lengthy and detailed academic document that presents the results of original research on a specific topic or question. It is usually required as a final project for a doctoral degree or a master’s degree.
Dissertation Meaning in Research
In Research , a dissertation refers to a substantial research project that students undertake in order to obtain an advanced degree such as a Ph.D. or a Master’s degree.
Dissertation typically involves the exploration of a particular research question or topic in-depth, and it requires students to conduct original research, analyze data, and present their findings in a scholarly manner. It is often the culmination of years of study and represents a significant contribution to the academic field.
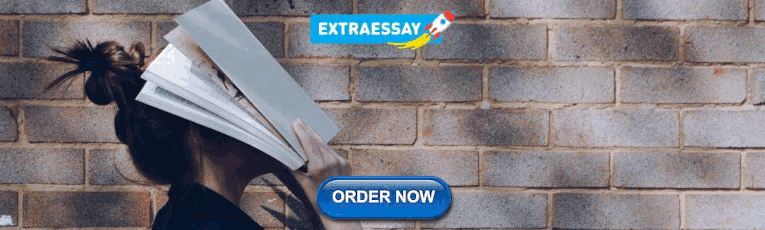
Types of Dissertation
Types of Dissertation are as follows:
Empirical Dissertation
An empirical dissertation is a research study that uses primary data collected through surveys, experiments, or observations. It typically follows a quantitative research approach and uses statistical methods to analyze the data.
Non-Empirical Dissertation
A non-empirical dissertation is based on secondary sources, such as books, articles, and online resources. It typically follows a qualitative research approach and uses methods such as content analysis or discourse analysis.
Narrative Dissertation
A narrative dissertation is a personal account of the researcher’s experience or journey. It typically follows a qualitative research approach and uses methods such as interviews, focus groups, or ethnography.
Systematic Literature Review
A systematic literature review is a comprehensive analysis of existing research on a specific topic. It typically follows a qualitative research approach and uses methods such as meta-analysis or thematic analysis.
Case Study Dissertation
A case study dissertation is an in-depth analysis of a specific individual, group, or organization. It typically follows a qualitative research approach and uses methods such as interviews, observations, or document analysis.
Mixed-Methods Dissertation
A mixed-methods dissertation combines both quantitative and qualitative research approaches to gather and analyze data. It typically uses methods such as surveys, interviews, and focus groups, as well as statistical analysis.
How to Write a Dissertation
Here are some general steps to help guide you through the process of writing a dissertation:
- Choose a topic : Select a topic that you are passionate about and that is relevant to your field of study. It should be specific enough to allow for in-depth research but broad enough to be interesting and engaging.
- Conduct research : Conduct thorough research on your chosen topic, utilizing a variety of sources, including books, academic journals, and online databases. Take detailed notes and organize your information in a way that makes sense to you.
- Create an outline : Develop an outline that will serve as a roadmap for your dissertation. The outline should include the introduction, literature review, methodology, results, discussion, and conclusion.
- Write the introduction: The introduction should provide a brief overview of your topic, the research questions, and the significance of the study. It should also include a clear thesis statement that states your main argument.
- Write the literature review: The literature review should provide a comprehensive analysis of existing research on your topic. It should identify gaps in the research and explain how your study will fill those gaps.
- Write the methodology: The methodology section should explain the research methods you used to collect and analyze data. It should also include a discussion of any limitations or weaknesses in your approach.
- Write the results: The results section should present the findings of your research in a clear and organized manner. Use charts, graphs, and tables to help illustrate your data.
- Write the discussion: The discussion section should interpret your results and explain their significance. It should also address any limitations of the study and suggest areas for future research.
- Write the conclusion: The conclusion should summarize your main findings and restate your thesis statement. It should also provide recommendations for future research.
- Edit and revise: Once you have completed a draft of your dissertation, review it carefully to ensure that it is well-organized, clear, and free of errors. Make any necessary revisions and edits before submitting it to your advisor for review.
Dissertation Format
The format of a dissertation may vary depending on the institution and field of study, but generally, it follows a similar structure:
- Title Page: This includes the title of the dissertation, the author’s name, and the date of submission.
- Abstract : A brief summary of the dissertation’s purpose, methods, and findings.
- Table of Contents: A list of the main sections and subsections of the dissertation, along with their page numbers.
- Introduction : A statement of the problem or research question, a brief overview of the literature, and an explanation of the significance of the study.
- Literature Review : A comprehensive review of the literature relevant to the research question or problem.
- Methodology : A description of the methods used to conduct the research, including data collection and analysis procedures.
- Results : A presentation of the findings of the research, including tables, charts, and graphs.
- Discussion : A discussion of the implications of the findings, their significance in the context of the literature, and limitations of the study.
- Conclusion : A summary of the main points of the study and their implications for future research.
- References : A list of all sources cited in the dissertation.
- Appendices : Additional materials that support the research, such as data tables, charts, or transcripts.
Dissertation Outline
Dissertation Outline is as follows:
Title Page:
- Title of dissertation
- Author name
- Institutional affiliation
- Date of submission
- Brief summary of the dissertation’s research problem, objectives, methods, findings, and implications
- Usually around 250-300 words
Table of Contents:
- List of chapters and sections in the dissertation, with page numbers for each
I. Introduction
- Background and context of the research
- Research problem and objectives
- Significance of the research
II. Literature Review
- Overview of existing literature on the research topic
- Identification of gaps in the literature
- Theoretical framework and concepts
III. Methodology
- Research design and methods used
- Data collection and analysis techniques
- Ethical considerations
IV. Results
- Presentation and analysis of data collected
- Findings and outcomes of the research
- Interpretation of the results
V. Discussion
- Discussion of the results in relation to the research problem and objectives
- Evaluation of the research outcomes and implications
- Suggestions for future research
VI. Conclusion
- Summary of the research findings and outcomes
- Implications for the research topic and field
- Limitations and recommendations for future research
VII. References
- List of sources cited in the dissertation
VIII. Appendices
- Additional materials that support the research, such as tables, figures, or questionnaires.
Example of Dissertation
Here is an example Dissertation for students:
Title : Exploring the Effects of Mindfulness Meditation on Academic Achievement and Well-being among College Students
This dissertation aims to investigate the impact of mindfulness meditation on the academic achievement and well-being of college students. Mindfulness meditation has gained popularity as a technique for reducing stress and enhancing mental health, but its effects on academic performance have not been extensively studied. Using a randomized controlled trial design, the study will compare the academic performance and well-being of college students who practice mindfulness meditation with those who do not. The study will also examine the moderating role of personality traits and demographic factors on the effects of mindfulness meditation.
Chapter Outline:
Chapter 1: Introduction
- Background and rationale for the study
- Research questions and objectives
- Significance of the study
- Overview of the dissertation structure
Chapter 2: Literature Review
- Definition and conceptualization of mindfulness meditation
- Theoretical framework of mindfulness meditation
- Empirical research on mindfulness meditation and academic achievement
- Empirical research on mindfulness meditation and well-being
- The role of personality and demographic factors in the effects of mindfulness meditation
Chapter 3: Methodology
- Research design and hypothesis
- Participants and sampling method
- Intervention and procedure
- Measures and instruments
- Data analysis method
Chapter 4: Results
- Descriptive statistics and data screening
- Analysis of main effects
- Analysis of moderating effects
- Post-hoc analyses and sensitivity tests
Chapter 5: Discussion
- Summary of findings
- Implications for theory and practice
- Limitations and directions for future research
- Conclusion and contribution to the literature
Chapter 6: Conclusion
- Recap of the research questions and objectives
- Summary of the key findings
- Contribution to the literature and practice
- Implications for policy and practice
- Final thoughts and recommendations.
References :
List of all the sources cited in the dissertation
Appendices :
Additional materials such as the survey questionnaire, interview guide, and consent forms.
Note : This is just an example and the structure of a dissertation may vary depending on the specific requirements and guidelines provided by the institution or the supervisor.
How Long is a Dissertation
The length of a dissertation can vary depending on the field of study, the level of degree being pursued, and the specific requirements of the institution. Generally, a dissertation for a doctoral degree can range from 80,000 to 100,000 words, while a dissertation for a master’s degree may be shorter, typically ranging from 20,000 to 50,000 words. However, it is important to note that these are general guidelines and the actual length of a dissertation can vary widely depending on the specific requirements of the program and the research topic being studied. It is always best to consult with your academic advisor or the guidelines provided by your institution for more specific information on dissertation length.
Applications of Dissertation
Here are some applications of a dissertation:
- Advancing the Field: Dissertations often include new research or a new perspective on existing research, which can help to advance the field. The results of a dissertation can be used by other researchers to build upon or challenge existing knowledge, leading to further advancements in the field.
- Career Advancement: Completing a dissertation demonstrates a high level of expertise in a particular field, which can lead to career advancement opportunities. For example, having a PhD can open doors to higher-paying jobs in academia, research institutions, or the private sector.
- Publishing Opportunities: Dissertations can be published as books or journal articles, which can help to increase the visibility and credibility of the author’s research.
- Personal Growth: The process of writing a dissertation involves a significant amount of research, analysis, and critical thinking. This can help students to develop important skills, such as time management, problem-solving, and communication, which can be valuable in both their personal and professional lives.
- Policy Implications: The findings of a dissertation can have policy implications, particularly in fields such as public health, education, and social sciences. Policymakers can use the research to inform decision-making and improve outcomes for the population.
When to Write a Dissertation
Here are some situations where writing a dissertation may be necessary:
- Pursuing a Doctoral Degree: Writing a dissertation is usually a requirement for earning a doctoral degree, so if you are interested in pursuing a doctorate, you will likely need to write a dissertation.
- Conducting Original Research : Dissertations require students to conduct original research on a specific topic. If you are interested in conducting original research on a topic, writing a dissertation may be the best way to do so.
- Advancing Your Career: Some professions, such as academia and research, may require individuals to have a doctoral degree. Writing a dissertation can help you advance your career by demonstrating your expertise in a particular area.
- Contributing to Knowledge: Dissertations are often based on original research that can contribute to the knowledge base of a field. If you are passionate about advancing knowledge in a particular area, writing a dissertation can help you achieve that goal.
- Meeting Academic Requirements : If you are a graduate student, writing a dissertation may be a requirement for completing your program. Be sure to check with your academic advisor to determine if this is the case for you.
Purpose of Dissertation
some common purposes of a dissertation include:
- To contribute to the knowledge in a particular field : A dissertation is often the culmination of years of research and study, and it should make a significant contribution to the existing body of knowledge in a particular field.
- To demonstrate mastery of a subject: A dissertation requires extensive research, analysis, and writing, and completing one demonstrates a student’s mastery of their subject area.
- To develop critical thinking and research skills : A dissertation requires students to think critically about their research question, analyze data, and draw conclusions based on evidence. These skills are valuable not only in academia but also in many professional fields.
- To demonstrate academic integrity: A dissertation must be conducted and written in accordance with rigorous academic standards, including ethical considerations such as obtaining informed consent, protecting the privacy of participants, and avoiding plagiarism.
- To prepare for an academic career: Completing a dissertation is often a requirement for obtaining a PhD and pursuing a career in academia. It can demonstrate to potential employers that the student has the necessary skills and experience to conduct original research and make meaningful contributions to their field.
- To develop writing and communication skills: A dissertation requires a significant amount of writing and communication skills to convey complex ideas and research findings in a clear and concise manner. This skill set can be valuable in various professional fields.
- To demonstrate independence and initiative: A dissertation requires students to work independently and take initiative in developing their research question, designing their study, collecting and analyzing data, and drawing conclusions. This demonstrates to potential employers or academic institutions that the student is capable of independent research and taking initiative in their work.
- To contribute to policy or practice: Some dissertations may have a practical application, such as informing policy decisions or improving practices in a particular field. These dissertations can have a significant impact on society, and their findings may be used to improve the lives of individuals or communities.
- To pursue personal interests: Some students may choose to pursue a dissertation topic that aligns with their personal interests or passions, providing them with the opportunity to delve deeper into a topic that they find personally meaningful.
Advantage of Dissertation
Some advantages of writing a dissertation include:
- Developing research and analytical skills: The process of writing a dissertation involves conducting extensive research, analyzing data, and presenting findings in a clear and coherent manner. This process can help students develop important research and analytical skills that can be useful in their future careers.
- Demonstrating expertise in a subject: Writing a dissertation allows students to demonstrate their expertise in a particular subject area. It can help establish their credibility as a knowledgeable and competent professional in their field.
- Contributing to the academic community: A well-written dissertation can contribute new knowledge to the academic community and potentially inform future research in the field.
- Improving writing and communication skills : Writing a dissertation requires students to write and present their research in a clear and concise manner. This can help improve their writing and communication skills, which are essential for success in many professions.
- Increasing job opportunities: Completing a dissertation can increase job opportunities in certain fields, particularly in academia and research-based positions.
About the author
Muhammad Hassan
Researcher, Academic Writer, Web developer
You may also like

Appendices – Writing Guide, Types and Examples

Research Techniques – Methods, Types and Examples

APA Table of Contents – Format and Example

Data Analysis – Process, Methods and Types

Research Contribution – Thesis Guide

Research Findings – Types Examples and Writing...

- Study and research support
- Academic skills
Dissertation examples
Listed below are some of the best examples of research projects and dissertations from undergraduate and taught postgraduate students at the University of Leeds We have not been able to gather examples from all schools. The module requirements for research projects may have changed since these examples were written. Refer to your module guidelines to make sure that you address all of the current assessment criteria. Some of the examples below are only available to access on campus.
- Undergraduate examples
- Taught Masters examples
- DSpace@MIT Home
- MIT Libraries
This collection of MIT Theses in DSpace contains selected theses and dissertations from all MIT departments. Please note that this is NOT a complete collection of MIT theses. To search all MIT theses, use MIT Libraries' catalog .
MIT's DSpace contains more than 58,000 theses completed at MIT dating as far back as the mid 1800's. Theses in this collection have been scanned by the MIT Libraries or submitted in electronic format by thesis authors. Since 2004 all new Masters and Ph.D. theses are scanned and added to this collection after degrees are awarded.
MIT Theses are openly available to all readers. Please share how this access affects or benefits you. Your story matters.
If you have questions about MIT theses in DSpace, [email protected] . See also Access & Availability Questions or About MIT Theses in DSpace .
If you are a recent MIT graduate, your thesis will be added to DSpace within 3-6 months after your graduation date. Please email [email protected] with any questions.
Permissions
MIT Theses may be protected by copyright. Please refer to the MIT Libraries Permissions Policy for permission information. Note that the copyright holder for most MIT theses is identified on the title page of the thesis.
Theses by Department
- Comparative Media Studies
- Computation for Design and Optimization
- Computational and Systems Biology
- Department of Aeronautics and Astronautics
- Department of Architecture
- Department of Biological Engineering
- Department of Biology
- Department of Brain and Cognitive Sciences
- Department of Chemical Engineering
- Department of Chemistry
- Department of Civil and Environmental Engineering
- Department of Earth, Atmospheric, and Planetary Sciences
- Department of Economics
- Department of Electrical Engineering and Computer Sciences
- Department of Humanities
- Department of Linguistics and Philosophy
- Department of Materials Science and Engineering
- Department of Mathematics
- Department of Mechanical Engineering
- Department of Nuclear Science and Engineering
- Department of Ocean Engineering
- Department of Physics
- Department of Political Science
- Department of Urban Studies and Planning
- Engineering Systems Division
- Harvard-MIT Program of Health Sciences and Technology
- Institute for Data, Systems, and Society
- Media Arts & Sciences
- Operations Research Center
- Program in Real Estate Development
- Program in Writing and Humanistic Studies
- Science, Technology & Society
- Science Writing
- Sloan School of Management
- Supply Chain Management
- System Design & Management
- Technology and Policy Program
Collections in this community
Doctoral theses, graduate theses, undergraduate theses, recent submissions.
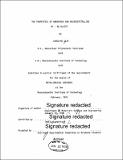
The properties of amorphous and microcrystalline Ni - Nb alloys.

Towards Biologically Plausible Deep Neural Networks
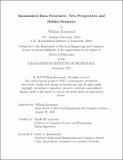
Randomized Data Structures: New Perspectives and Hidden Surprises

UTA researcher recognized for data science leadership
Thursday, May 30, 2024 • Katherine Egan Bennett : contact
Xinlei “Sherry” Wang, director for research in the Division of Data Science at The University of Texas at Arlington, has been named a 2024 fellow of the American Statistical Association (ASA) .
Wang, the Jenkins Garret Endowed Professor of Statistics and Data Science, was selected for her outstanding contributions in developing and applying statistical and computational methods to complex data challenges, for excellence in mentoring doctoral students and junior researchers and for exceptional leadership of data science programs.
Founded in 1839, ASA is the world’s largest community of statisticians and the second oldest continuously operating professional association in the country. ASA fellows have been designated for nearly 100 years, and only up to one-third of 1% of the total association membership can be elected each year.
“I am honored to be elected an ASA fellow and recognized by my profession,” Wang said. “I'm truly grateful for the support I've received from my department, college and University since I joined UTA, which undoubtedly contributed to this achievement.”
Wang came to UTA in January 2023 as director of the College of Science’s newly created Center for Data Science Research and Education. She and her colleagues launched UTA’s first graduate degree in data science in fall 2023.
In January 2024, College of Science Dean Morteza Khaledi appointed Wang as director for research of the new Division of Data Science, which serves as a hub to organize, provide infrastructure support and facilitate growth in instructional and research programs involving data science.
“Dr. Wang is very deserving of this honor of being named an ASA fellow,” Dr. Khaledi said. “This recognition is a testament to her outstanding scholarship. She has played a major role in building and advancing our data science program in the College of Science, and we’re very fortunate to have her here at UTA.”
Wang received a Bachelor of Science in automatic control from the University of Science and Technology of China in 1997, a master’s in statistics from the University of Pittsburgh in 1998 and a doctorate in decision science and statistics from UT Austin in 2002. Her research has been funded by major grants from the National Science Foundation, National Institutes of Health, and the Cancer Prevention and Research Institute of Texas.
She has authored or co-authored more than 90 papers in refereed publications and made more than 75 presentations at conferences and seminars around the world. She has directed Ph.D. dissertations for 19 students, with seven more in progress.
Wang has been an ASA member since 1999 and has served the ASA in various capacities, including as vice president (2009-10) and president (2010-11) of the North Texas Chapter. She will be formally recognized as a 2024 fellow at an awards ceremony at the 2024 Joint Statistical Meetings in Portland, Oregon, in August.
- By Greg Pederson , College of Science
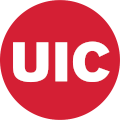
File(s) under embargo
until file(s) become available
Applications of Urban Informatics in Sustainability Planning for Food Waste Management
Degree grantor, degree level, degree name, committee member, submitted date, thesis type, usage metrics.

How To Write The Results/Findings Chapter
For qualitative studies (dissertations & theses).
By: Jenna Crossley (PhD). Expert Reviewed By: Dr. Eunice Rautenbach | August 2021
So, you’ve collected and analysed your qualitative data, and it’s time to write up your results chapter. But where do you start? In this post, we’ll guide you through the qualitative results chapter (also called the findings chapter), step by step.
Overview: Qualitative Results Chapter
- What (exactly) the qualitative results chapter is
- What to include in your results chapter
- How to write up your results chapter
- A few tips and tricks to help you along the way
- Free results chapter template
What exactly is the results chapter?
The results chapter in a dissertation or thesis (or any formal academic research piece) is where you objectively and neutrally present the findings of your qualitative analysis (or analyses if you used multiple qualitative analysis methods ). This chapter can sometimes be combined with the discussion chapter (where you interpret the data and discuss its meaning), depending on your university’s preference. We’ll treat the two chapters as separate, as that’s the most common approach.
In contrast to a quantitative results chapter that presents numbers and statistics, a qualitative results chapter presents data primarily in the form of words . But this doesn’t mean that a qualitative study can’t have quantitative elements – you could, for example, present the number of times a theme or topic pops up in your data, depending on the analysis method(s) you adopt.
Adding a quantitative element to your study can add some rigour, which strengthens your results by providing more evidence for your claims. This is particularly common when using qualitative content analysis. Keep in mind though that qualitative research aims to achieve depth, richness and identify nuances , so don’t get tunnel vision by focusing on the numbers. They’re just cream on top in a qualitative analysis.
So, to recap, the results chapter is where you objectively present the findings of your analysis, without interpreting them (you’ll save that for the discussion chapter). With that out the way, let’s take a look at what you should include in your results chapter.
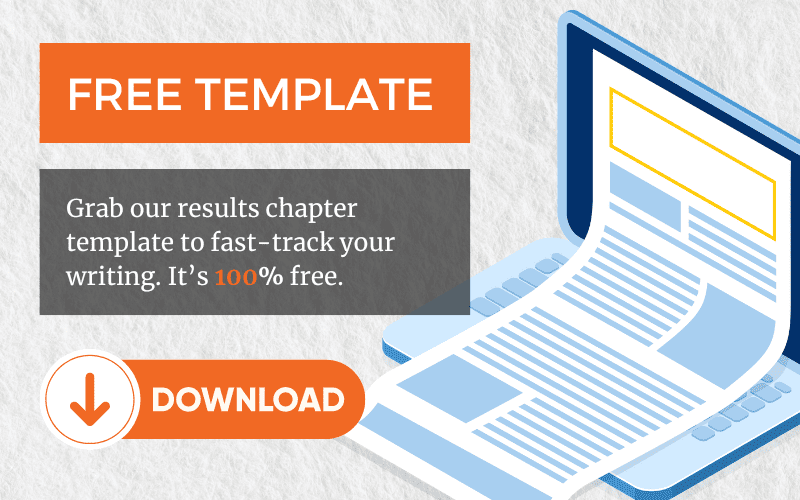
What should you include in the results chapter?
As we’ve mentioned, your qualitative results chapter should purely present and describe your results , not interpret them in relation to the existing literature or your research questions . Any speculations or discussion about the implications of your findings should be reserved for your discussion chapter.
In your results chapter, you’ll want to talk about your analysis findings and whether or not they support your hypotheses (if you have any). Naturally, the exact contents of your results chapter will depend on which qualitative analysis method (or methods) you use. For example, if you were to use thematic analysis, you’d detail the themes identified in your analysis, using extracts from the transcripts or text to support your claims.
While you do need to present your analysis findings in some detail, you should avoid dumping large amounts of raw data in this chapter. Instead, focus on presenting the key findings and using a handful of select quotes or text extracts to support each finding . The reams of data and analysis can be relegated to your appendices.
While it’s tempting to include every last detail you found in your qualitative analysis, it is important to make sure that you report only that which is relevant to your research aims, objectives and research questions . Always keep these three components, as well as your hypotheses (if you have any) front of mind when writing the chapter and use them as a filter to decide what’s relevant and what’s not.
Need a helping hand?
How do I write the results chapter?
Now that we’ve covered the basics, it’s time to look at how to structure your chapter. Broadly speaking, the results chapter needs to contain three core components – the introduction, the body and the concluding summary. Let’s take a look at each of these.
Section 1: Introduction
The first step is to craft a brief introduction to the chapter. This intro is vital as it provides some context for your findings. In your introduction, you should begin by reiterating your problem statement and research questions and highlight the purpose of your research . Make sure that you spell this out for the reader so that the rest of your chapter is well contextualised.
The next step is to briefly outline the structure of your results chapter. In other words, explain what’s included in the chapter and what the reader can expect. In the results chapter, you want to tell a story that is coherent, flows logically, and is easy to follow , so make sure that you plan your structure out well and convey that structure (at a high level), so that your reader is well oriented.
The introduction section shouldn’t be lengthy. Two or three short paragraphs should be more than adequate. It is merely an introduction and overview, not a summary of the chapter.
Pro Tip – To help you structure your chapter, it can be useful to set up an initial draft with (sub)section headings so that you’re able to easily (re)arrange parts of your chapter. This will also help your reader to follow your results and give your chapter some coherence. Be sure to use level-based heading styles (e.g. Heading 1, 2, 3 styles) to help the reader differentiate between levels visually. You can find these options in Word (example below).

Section 2: Body
Before we get started on what to include in the body of your chapter, it’s vital to remember that a results section should be completely objective and descriptive, not interpretive . So, be careful not to use words such as, “suggests” or “implies”, as these usually accompany some form of interpretation – that’s reserved for your discussion chapter.
The structure of your body section is very important , so make sure that you plan it out well. When planning out your qualitative results chapter, create sections and subsections so that you can maintain the flow of the story you’re trying to tell. Be sure to systematically and consistently describe each portion of results. Try to adopt a standardised structure for each portion so that you achieve a high level of consistency throughout the chapter.
For qualitative studies, results chapters tend to be structured according to themes , which makes it easier for readers to follow. However, keep in mind that not all results chapters have to be structured in this manner. For example, if you’re conducting a longitudinal study, you may want to structure your chapter chronologically. Similarly, you might structure this chapter based on your theoretical framework . The exact structure of your chapter will depend on the nature of your study , especially your research questions.
As you work through the body of your chapter, make sure that you use quotes to substantiate every one of your claims . You can present these quotes in italics to differentiate them from your own words. A general rule of thumb is to use at least two pieces of evidence per claim, and these should be linked directly to your data. Also, remember that you need to include all relevant results , not just the ones that support your assumptions or initial leanings.
In addition to including quotes, you can also link your claims to the data by using appendices , which you should reference throughout your text. When you reference, make sure that you include both the name/number of the appendix , as well as the line(s) from which you drew your data.
As referencing styles can vary greatly, be sure to look up the appendix referencing conventions of your university’s prescribed style (e.g. APA , Harvard, etc) and keep this consistent throughout your chapter.
Section 3: Concluding summary
The concluding summary is very important because it summarises your key findings and lays the foundation for the discussion chapter . Keep in mind that some readers may skip directly to this section (from the introduction section), so make sure that it can be read and understood well in isolation.
In this section, you need to remind the reader of the key findings. That is, the results that directly relate to your research questions and that you will build upon in your discussion chapter. Remember, your reader has digested a lot of information in this chapter, so you need to use this section to remind them of the most important takeaways.
Importantly, the concluding summary should not present any new information and should only describe what you’ve already presented in your chapter. Keep it concise – you’re not summarising the whole chapter, just the essentials.
Tips for writing an A-grade results chapter
Now that you’ve got a clear picture of what the qualitative results chapter is all about, here are some quick tips and reminders to help you craft a high-quality chapter:
- Your results chapter should be written in the past tense . You’ve done the work already, so you want to tell the reader what you found , not what you are currently finding .
- Make sure that you review your work multiple times and check that every claim is adequately backed up by evidence . Aim for at least two examples per claim, and make use of an appendix to reference these.
- When writing up your results, make sure that you stick to only what is relevant . Don’t waste time on data that are not relevant to your research objectives and research questions.
- Use headings and subheadings to create an intuitive, easy to follow piece of writing. Make use of Microsoft Word’s “heading styles” and be sure to use them consistently.
- When referring to numerical data, tables and figures can provide a useful visual aid. When using these, make sure that they can be read and understood independent of your body text (i.e. that they can stand-alone). To this end, use clear, concise labels for each of your tables or figures and make use of colours to code indicate differences or hierarchy.
- Similarly, when you’re writing up your chapter, it can be useful to highlight topics and themes in different colours . This can help you to differentiate between your data if you get a bit overwhelmed and will also help you to ensure that your results flow logically and coherently.
If you have any questions, leave a comment below and we’ll do our best to help. If you’d like 1-on-1 help with your results chapter (or any chapter of your dissertation or thesis), check out our private dissertation coaching service here or book a free initial consultation to discuss how we can help you.
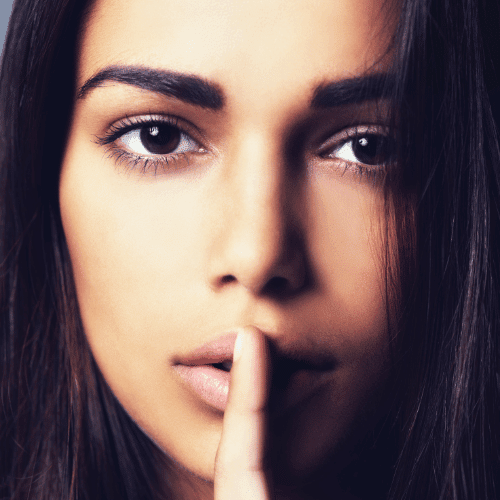
Psst... there’s more!
This post was based on one of our popular Research Bootcamps . If you're working on a research project, you'll definitely want to check this out ...
You Might Also Like:
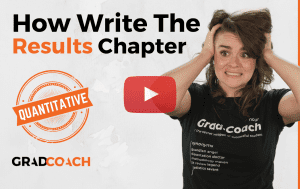
20 Comments
This was extremely helpful. Thanks a lot guys
Hi, thanks for the great research support platform created by the gradcoach team!
I wanted to ask- While “suggests” or “implies” are interpretive terms, what terms could we use for the results chapter? Could you share some examples of descriptive terms?
I think that instead of saying, ‘The data suggested, or The data implied,’ you can say, ‘The Data showed or revealed, or illustrated or outlined’…If interview data, you may say Jane Doe illuminated or elaborated, or Jane Doe described… or Jane Doe expressed or stated.
I found this article very useful. Thank you very much for the outstanding work you are doing.
What if i have 3 different interviewees answering the same interview questions? Should i then present the results in form of the table with the division on the 3 perspectives or rather give a results in form of the text and highlight who said what?
I think this tabular representation of results is a great idea. I am doing it too along with the text. Thanks
That was helpful was struggling to separate the discussion from the findings
this was very useful, Thank you.
Very helpful, I am confident to write my results chapter now.
It is so helpful! It is a good job. Thank you very much!
Very useful, well explained. Many thanks.
Hello, I appreciate the way you provided a supportive comments about qualitative results presenting tips
I loved this! It explains everything needed, and it has helped me better organize my thoughts. What words should I not use while writing my results section, other than subjective ones.
Thanks a lot, it is really helpful
Thank you so much dear, i really appropriate your nice explanations about this.
Thank you so much for this! I was wondering if anyone could help with how to prproperly integrate quotations (Excerpts) from interviews in the finding chapter in a qualitative research. Please GradCoach, address this issue and provide examples.
what if I’m not doing any interviews myself and all the information is coming from case studies that have already done the research.
Very helpful thank you.
This was very helpful as I was wondering how to structure this part of my dissertation, to include the quotes… Thanks for this explanation
This is very helpful, thanks! I am required to write up my results chapters with the discussion in each of them – any tips and tricks for this strategy?
Submit a Comment Cancel reply
Your email address will not be published. Required fields are marked *
Save my name, email, and website in this browser for the next time I comment.
- Print Friendly
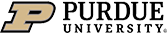
Adversarial Attacks On Graph Convolutional Transformer With EHR Data
This research explores adversarial attacks on Graph Convolutional Transformer (GCT) models that utilize Electronic Health Record (EHR) data. As deep learning models become increasingly integral to healthcare, securing their robustness against adversarial threats is critical. This research assesses the susceptibility of GCT models to specific adversarial attacks, namely the Fast Gradient Sign Method (FGSM) and the Jacobian-based Saliency Map Attack (JSMA). It examines their effect on the model’s prediction of mortality and readmission. Through experiments conducted with the MIMIC-III and eICU datasets, the study finds that although the GCT model exhibits superior performance in processing EHR data under normal conditions, its accuracy drops when subjected to adversarial conditions—from an accuracy of 86% with test data to about 57% and an area under the curve (AUC) from 0.86 to 0.51. These findings averaged across both datasets and attack methods, underscore the urgent need for effective adversarial defense mechanisms in AI systems used in healthcare. This thesis contributes to the field by identifying vulnerabilities and suggesting various strategies to enhance the resilience of GCT models against adversarial manipulations.
Degree Type
- Master of Science
- Computer and Information Technology
Campus location
- West Lafayette
Advisor/Supervisor/Committee Chair
Additional committee member 2, additional committee member 3, usage metrics.
- Deep learning
- Adversarial machine learning

News and views for the UB community
- Stories >
IBM director of research explains how AI can help companies leverage data
research news

Dario Gil, IBM senior vice president and director of research, discusses “Exploring the Future of AI for Maximum Industry Impact” at the sixth edition of the UB | AI Chat Series, held at UB’s Center of Excellence in Bioinformatics and Life Sciences on the Downtown Campus. Photo: Douglas Levere
By TOM DINKI
Published June 3, 2024
Dario Gil, IBM senior vice president and director of research, wants you to think about artificial intelligence differently.
While large language models like ChatGPT and their ability to converse may get much of the attention, Gil says the real value of AI is in the unprecedented amount of data that it can analyze and what we as humans then do with that analysis.
“Don’t be an AI user, be an AI value creator,” Gil told an audience of UB faculty, students and stakeholders Friday on the Downtown Campus. “Use the technology but think more structurally about how to create value in whatever domain you are in, whether you’re a professor or student or an institution. A lot of this is going to be taking the data in your fields and going on this journey of unlocking its true value by embracing the power of this new representation and fully exploiting what it gives us.”
Gil gave the keynote address of the sixth edition of the UB | AI Chat Series, titled “Exploring the Future of AI for Maximum Industry Impact.”
The event included a panel featuring Gil and other officials from some of the nation’s leading businesses. Moderated by Kemper Lewis, dean of the School of Engineering and Applied Sciences, the panelists discussed the ways their companies are currently using AI and how they’ll use it in the future.

UB engineering dean Kemper Lewis (far right, at the podium) moderates a panel of industry leaders that includes (from left) Jose Pinto, Chris Tolomeo and Dario Gil. Photo: Douglas Levere
A clear consensus emerged: AI will help companies take full advantage of their data.
Gil, who directs IBM’s strategies in AI, semiconductors and quantum computing, explained how AI, neural networks in particular, transform our information — documents, images and even speech — and transform them into what’s known in the AI field as tokens. Foundational models can intake tens of trillions of these tokens, worth many terabytes of data.
“When you actually represent information in that fashion, you can establish semantic connections [much easier]. In this high-dimensional space, the distance between those representations tells you something about how connected those pieces of information are,” Gil said. “While we can only think in three dimensions, the machines don’t care how many dimensions there are. So it turns out to be a very powerful way to establish connections.”
Another panelist, Chris Tolomeo, senior vice president and head of banking services for M&T Bank, says the company is already using AI to confirm new customers’ identities and catch check fraud.
“I think the promise of AI will simplify things to make it much easier for both our customers and our employees,” he said.
However, he also offered caution.
“There’s high expectations that things will happen very quickly, and history tells us technology tends to be very difficult and expensive,” he said. “Not to downplay the potential, but making sure we’re doing things with the right expectations.”
AI will also transform health care and research. Allison Brashear, vice president for health sciences and dean of the Jacobs School of Medicine and Biomedical Sciences, envisions AI conducting predictive modeling for who is at risk for Alzheimer’s disease or a heart attack.
“That’s the aspiration here: Go from fixing people after they are sick to fixing people before they are sick,” Brashear said.

From left: Provost A. Scott Weber; Dario Gil, IBM senior vice president and director of research; Venu Govindaraju, vice president for research and economic development; and Jinjun Xiong, SUNY Empire Innovation Professor and director of the Institute for Artificial Intelligence and Data Science, pose for a photo at the UB | AI Chat Series. Photo: Douglas Levere
With UB recently being named the home of Empire AI and its supercomputing center, Gil also offered some insight on what the university can expect. IBM just recently built another supercomputing center for AI in Texas.
“You’re going to go on this journey with Empire AI of creating foundational infrastructure that will allow people in their own fields to go and create their own foundation models or build from ones in the community and expand it,” he said. “This is going to be a collaborative and community endeavor, and of course to do this, not only do you need to have data and expertise, you need a lot of computing power.”
Last month, IBM open-sourced its series of AI foundation models, Granite.
“The future of AI is to be open. This is an indispensable element to make it safe, innovative and distribute economic benefits to the world,” Gil said. “It is indispensable for the future of universities. If we go down a path where AI becomes closed … it’s going to be a real catastrophe for universities.”
Numbers, Facts and Trends Shaping Your World
Read our research on:
Full Topic List
Regions & Countries
- Publications
- Our Methods
- Short Reads
- Tools & Resources
Read Our Research On:
How Americans and Israelis view one another and the U.S. role in the Israel-Hamas war
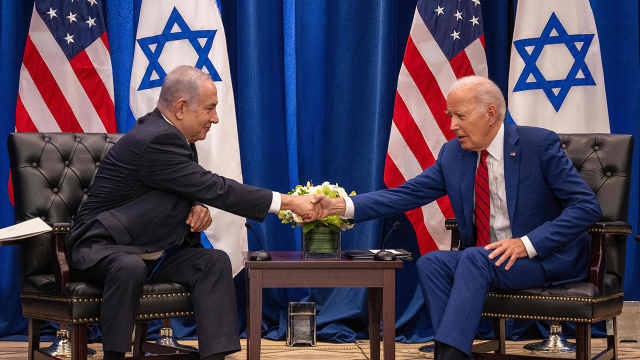
The United States continues to support Israel in its war against Hamas, but relations appear increasingly strained between the two countries and their leaders . The war also seems to have affected public opinion in both countries: Americans and Israelis now see one another’s leaders more negatively than in the recent past, and other key views have shifted as well.
Below, we explore American and Israeli public opinion in detail. This analysis is based on two Pew Research Center surveys of U.S. adults – one conducted in February and one in early April – as well as a survey of Israeli adults conducted in March and early April.
Pew Research Center analyzed Americans’ and Israelis’ attitudes on a variety of questions related to the Israel-Hamas war. The recent U.S. data is from two polls: a survey of 12,693 U.S. adults conducted Feb. 13-25, 2024, and a survey of 3,600 U.S. adults conducted April 1-7, 2024. The data for Israel is from a survey of 1,001 Israeli adults conducted March 3-April 4, 2024.
Most of the respondents in the February survey of U.S. adults (10,642) are members of the Center’s American Trends Panel (ATP). The ATP is an online survey panel that is recruited through national, random sampling of residential addresses, which gives nearly all U.S. adults a chance of selection. Read more about the ATP’s methodology .
The remaining 2,051 respondents are members of three other survey panels – Ipsos’ KnowledgePanel, SSRS’s Opinion Panel, and NORC at the University of Chicago’s AmeriSpeak Panel – who were interviewed because they identify as Jewish or Muslim.
The February survey “oversampled” (i.e., interviewed a disproportionately large number of) Jews and Muslims to provide more reliable estimates of their views. But these groups are not overrepresented in the national figures reported, because we adjusted for the oversampling in the weighting of the data.
Although the February survey included questions about where U.S. adults were born and whether they identify as Arab or of Arab origin, there was insufficient sample size to analyze Arab Americans or people of Palestinian descent separately. In that survey , only about a quarter of U.S. Muslims identified as Arab or of Arab ancestry. As a result, in our U.S. analysis, we describe differences between religious groups, rather than differences in opinion between Arab Americans and Jewish Americans. In contrast, our surveys in Israel have sufficient samples to allow comparisons between Israeli Arabs and Israeli Jews.
Both U.S. surveys are weighted to be representative of the U.S. adult population by gender, race, ethnicity, partisan affiliation, education, religious affiliation and other categories.
The survey of Israeli adults was conducted face-to-face. Interviews were conducted in Hebrew and Arabic, and the survey is representative of the adult population ages 18 and older, excluding those in East Jerusalem and non-sanctioned outposts. (The survey also does not include the West Bank or Gaza.) The survey included an oversample of Arabs in Israel. It was subsequently weighted to be representative of the Israeli adult population with the following variables: gender by ethnicity, age by ethnicity, education, region, urbanicity and probability of selection of respondent.
Here are the February and April questions and responses for the United States used for this analysis, and the questions and responses for Israel . Here are the February and April survey methodologies for the U.S. , and the survey methodology for Israel .
Americans have less confidence in Israeli Prime Minister Benjamin Netanyahu than Israelis have in U.S. President Joe Biden. Only 30% of U.S. adults express some or a lot of confidence in Netanyahu, while around half (53%) have not too much or no confidence in him. An additional 15% say they have not heard of him.
By contrast, more than half of Israeli adults (57%) have some or a great deal of confidence in Biden to do the right thing regarding world affairs, whereas 42% have little or no confidence in him.
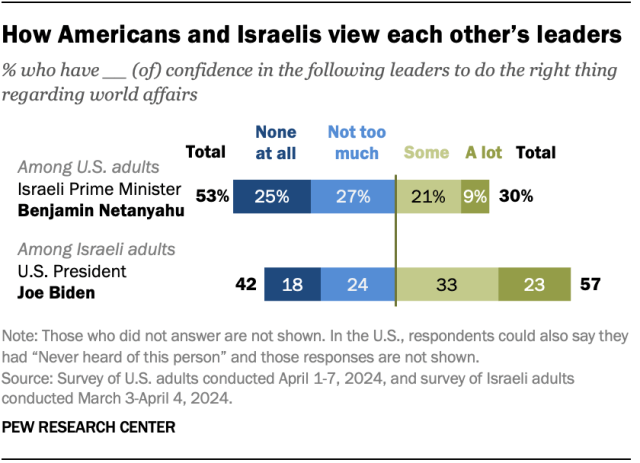
Americans’ views of Netanyahu have turned more negative over the past year. The share of Americans with little or no confidence in Netanyahu to do the right thing regarding world affairs is up 11 percentage points since March 2023 (42% then, 53% today).
Democrats are more likely than Republicans to have a negative view of Netanyahu. Approximately seven-in-ten Democrats and Democratic-leaning independents (71%) have little or no confidence in him, up from 56% in 2023. Around a third of Republicans and GOP leaners (34%) now take this view – up from 29% in 2023.
Related: A growing share of Americans have little or no confidence in Netanyahu
Israelis’ opinions of Biden have also turned more negative. While 57% of Israelis express a lot or some confidence in Biden, that’s down from 68% last year . The share of Israelis who have no confidence at all in Biden has doubled, from 9% to 18%.
Israeli Jews and Arabs differ widely in their views of the U.S. president: 66% of Jews have confidence in Biden, but only 21% of Arabs agree. Among both groups, overall confidence in Biden has decreased by 10 points since last year.
Older Israelis, as well as those in the ideological center or on the right, have more confidence in Biden than younger and left-leaning Israelis do.
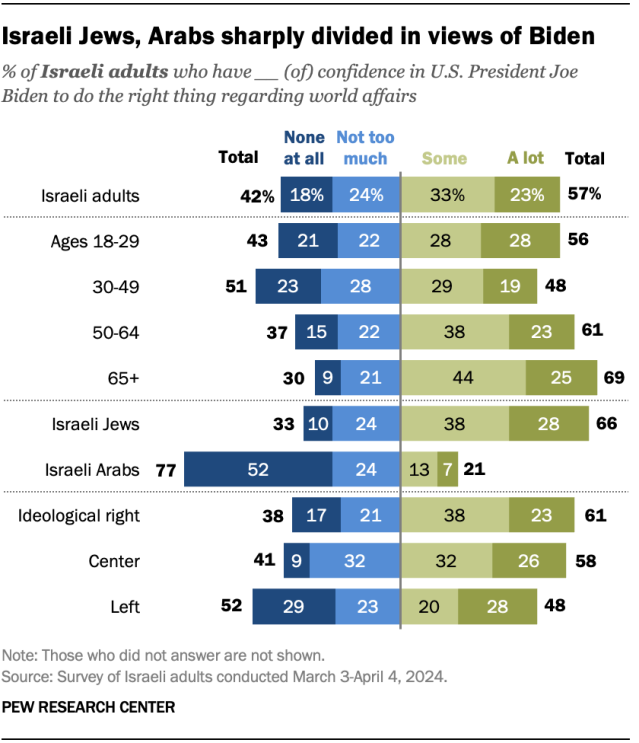
The share of Israelis with a favorable opinion of the U.S. has declined. While a broad majority of Israelis (77%) continue to see the U.S. favorably, this is down 10 points since last year. This marks the lowest overall rating of the U.S. in Israeli public opinion since 2011, based on our nearly two-decade trend .
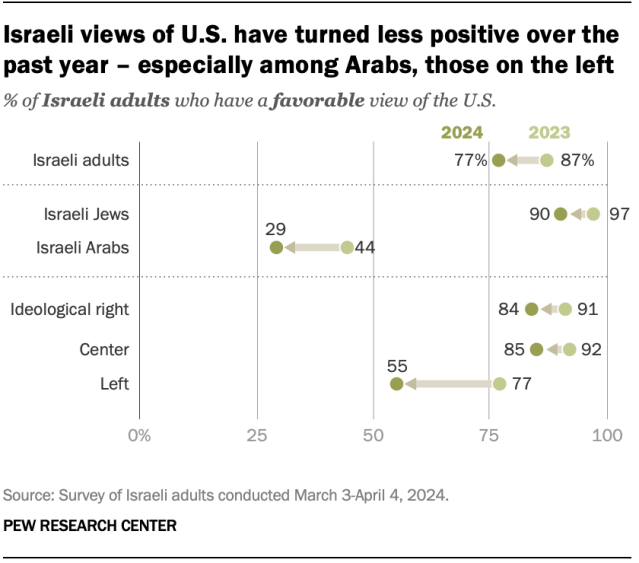
Views of the U.S. are significantly more positive among Jewish Israelis (90%) than Arab Israelis (29%). They are also much more positive among Israelis who place themselves on the ideological right (85%) or in the center (84%) than on the left (55%).
Since last year, views of the U.S. have soured among most demographic groups in Israel, but particularly among Arab Israelis and people on the ideological left.
Americans’ views of the Israeli people are broadly positive, but far fewer express favorable views of the Israeli government . In our recent U.S. polling, we have asked separately about views of the Israeli government and the Israeli people , rather than ask a single question about views of Israel. ( Past research suggests that views of the country tend to fall somewhere between views of its people and its government.)
In our survey this past February, 64% of Americans had a favorable view of the Israeli people, while 41% voiced a favorable view of the Israeli government. Both of these figures were down modestly from 2022. Views of the Israeli people have ticked downward more among younger Americans than among older ones.
Americans and Israelis alike are divided over whether Biden is favoring one side too much in the Israel-Hamas war. In our February survey, Americans were about evenly split as to whether Biden was favoring the Israelis too much (22%), favoring the Palestinians too much (16%) or striking the right balance (21%). However, the largest share of all – 40% – was not sure.
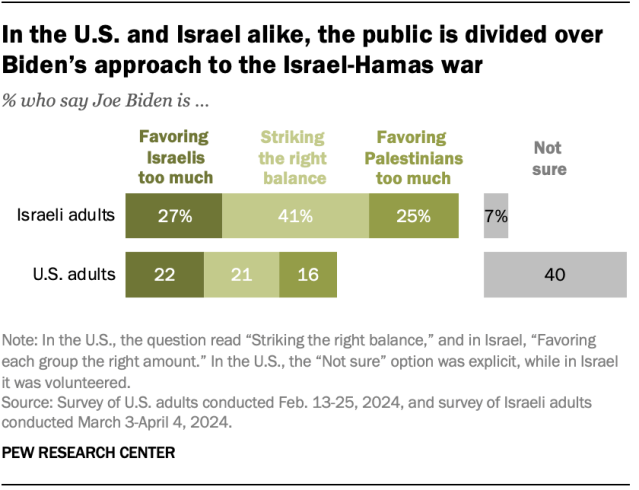
In Israel, 41% of adults say Biden is striking the right balance, but nearly equal shares say the U.S. president favors the Israelis too much (27%) or favors Palestinians too much (25%). Just 7% of Israelis aren’t sure or did not answer the question. (It’s important to note that U.S. respondents took the survey online and were given an explicit “Don’t know” option. Israeli respondents, interviewed in person, were not. Previous research shows that offering an explicit “Don’t know” option in online surveys is a better comparison with face-to-face survey data.)
In both the U.S. and Israel , people on the ideological left are more likely than those on the right to say Biden is favoring Israelis too much (in the U.S., ideology was measured as liberal, moderate and conservative).
In the U.S., Muslim Americans are far more likely than Jewish Americans to say Biden favors the Israelis too much (60% vs. 13%). In Israel, Arabs (86%) are much more likely than Jews to say the same (86% vs. 11%). (In the U.S., we “oversampled” Muslim and Jewish Americans but did not have sufficient sample size to analyze Arab Americans. For more details on the sampling, read the “How we did this” box.)
Around seven-in-ten Israelis (72%) want to see the U.S. play a major diplomatic role in resolving the Israel-Hamas war, but Americans are more divided. In the U.S., a majority of Americans (55%) also say the U.S. should play a role in ending the war, but more favor a minor role than a major one (35% vs. 20%). Another 27% want the U.S. to play no role at all – a view shared by only 10% of Israelis.
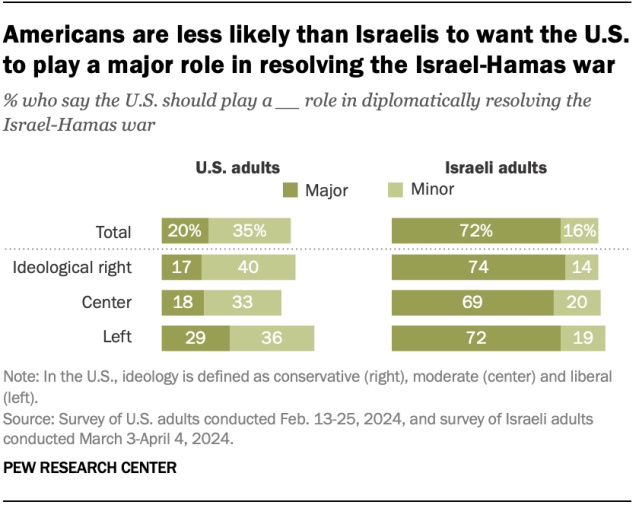
Americans on the ideological left are more supportive of the U.S. playing a major role than are those in the center or on the right. In Israel, U.S. involvement is broadly popular across the ideological spectrum.
In the U.S., 45% of Jews think the U.S. should play a major role in resolving the war, compared with 27% of Muslims. In Israel, Jews and Arabs are both generally supportive of the U.S. playing a major role (74% and 62%, respectively).
Note: Here are the February and April questions and responses for the United States used for this analysis, and the questions and responses for Israel . Here are the February and April survey methodologies for the U.S. , and the survey methodology for Israel .
- Israel Global Image
- U.S. Global Image
- War & International Conflict
- World Leaders
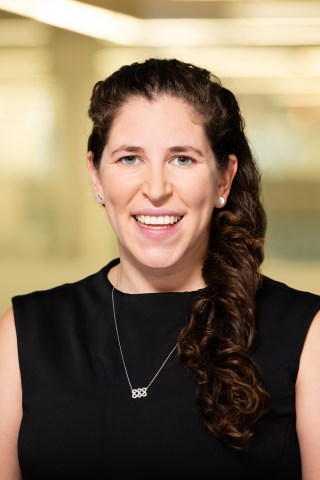
Laura Silver is an associate director focusing on global attitudes at Pew Research Center .
In East Asia, many people see China’s power and influence as a major threat
How views of the u.s., china and their leaders have changed over time, comparing views of the u.s. and china in 24 countries, poles and hungarians differ over views of russia and the u.s., 6 facts about how mexicans view the u.s. and their own country, most popular.
1615 L St. NW, Suite 800 Washington, DC 20036 USA (+1) 202-419-4300 | Main (+1) 202-857-8562 | Fax (+1) 202-419-4372 | Media Inquiries
Research Topics
- Email Newsletters
ABOUT PEW RESEARCH CENTER Pew Research Center is a nonpartisan fact tank that informs the public about the issues, attitudes and trends shaping the world. It conducts public opinion polling, demographic research, media content analysis and other empirical social science research. Pew Research Center does not take policy positions. It is a subsidiary of The Pew Charitable Trusts .
© 2024 Pew Research Center

Personalized oxygenation could improve outcomes for patients on ventilators
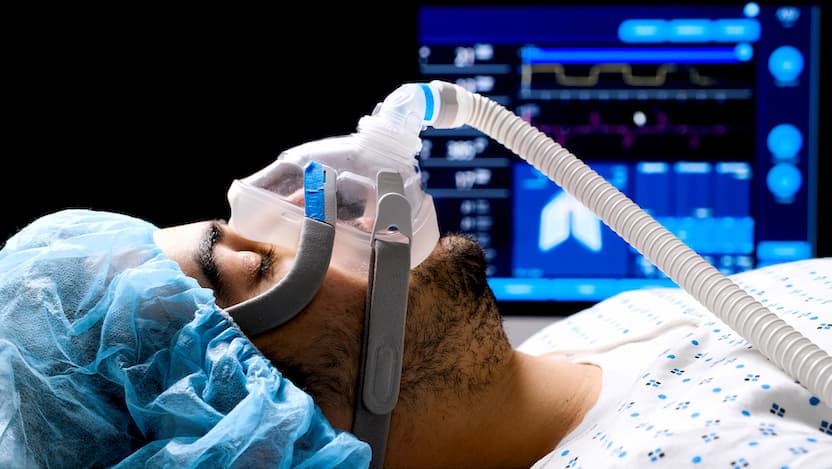
Supplemental oxygen is among the most widely prescribed therapies in the world, with an estimated 13 to 20 million patients worldwide requiring oxygen delivery by mechanical ventilation each year. Mechanical ventilation — a form of life support — is a technology that moves breathable air into and out of the lungs, acting like a bellows. Ventilators have moved far beyond the “iron lung” machines some people might picture; now, apparatuses have progressed to sophisticated, compact digital machines that deliver oxygen through a small plastic tube that goes down the throat.
Despite technological advancements, the correct amount of oxygen to deliver to each patient has remained a guessing game. Clinicians prescribe oxygen levels by using devices that record SpO 2 saturation, which measures the amount of oxygen in a patient’s blood. However, prior research was unable to establish whether a higher or lower SpO 2 target is better for patients.
“The standard of care is to maintain oxygen saturation between 88 and 100; within that range, doctors have had to choose an oxygen level for ventilation without having high-quality data to inform their decision-making,” said Kevin Buell, MBBS, a pulmonary and critical care fellow at the University of Chicago Medicine. “Whether we like it or not, making that decision for each patient exposes them to the potential benefits or harms of the chosen oxygen level.”
To take the guesswork out of ventilation, Buell and a group of other researchers used a machine learning model to study whether the effects of different oxygen levels depend on individual patients’ characteristics. The results, published in JAMA , suggest that personalized oxygenation targets could reduce mortality — which could have far-reaching impacts on critical care.
Previously, some research groups conducted randomized trials to investigate whether higher or lower oxygen levels are better for patients overall, but most produced no clear answer. Buell and his collaborators hypothesized that instead of indicating that oxygen levels don’t affect patient outcomes, the neutral results might indicate that the treatment outcomes for different oxygen levels varied by patient and simply averaged to zero effect in randomized trials.
As personalized medicine continues gaining traction, there is a growing interest in using machine learning to make predictions for individual patients. In the context of mechanical ventilation, these models could potentially use specific patient characteristics to predict an ideal oxygen level for each patient. These characteristics included age, sex, heart rate, body temperature and reason for being admitted to an Intensive Care Unit (ICU).
“We set out to create an evidence-based, personalized prediction of who would benefit from a lower or higher oxygen target when they go on a ventilator,” said Buell, a joint first author on the study.
Those previous randomized trials didn’t go to waste — Buell and his collaborators used data from those studies to design and train their machine learning model. After the model was developed using trial data collected in the U.S., the collaborators applied it to data from patients across the world in Australia and New Zealand. For patients who received oxygenation that fell within the target range the machine learning model predicted to be beneficial for them, mortality could have decreased by 6.4% overall.
It’s impossible to generalize predictions based on a single characteristic — for example, not all patients with brain injuries will benefit from lower oxygen saturation even though the data skew in that direction — which is why clinicians need a tool like the researchers’ machine learning model to piece together the mosaic of each patient’s needs. However, Buell pointed out that although the algorithm itself is complicated, the variables healthcare teams would input are all familiar clinical variables, making it easy for anyone to implement this kind of tool in the future.
At UChicago Medicine, healthcare teams can already use algorithms directly integrated into the electronic health record (EHR) system to inform other areas of clinical decision-making. Buell hopes mechanical ventilation can one day function the same way. For hospitals that might not have the resources to integrate machine learning into an EHR, he even envisions creating a web-based application that would allow clinicians to type in patient characteristics and obtain a prediction that way — like an online calculator. A lot of validation, testing and refinement needs to happen before clinical implementation can become a reality, but the end goal makes that future research well worth the investment.
In an editorial that accompanied the article’s publication, renowned critical care expert Derek Angus, MD, wrote: “If the results are true and generalizable, then the consequences are staggering. If one could instantly assign every patient into their appropriate group of predicted benefit or harm and assign their oxygen target accordingly, the intervention would theoretically yield the greatest single improvement in lives saved from critical illness in the history of the field.”
The study, “ Individualized Treatment Effects of Oxygen Targets in Mechanically Ventilated Critically Ill Adults ,” was published in the Journal of the American Medical Association in March 2024. Co-authors include Kevin G. Buell, Alexandra B. Spicer, Jonathan D. Casey, Kevin P. Seitz, Edward T. Qian, Emma J. Graham Linck, Wesley H. Self, Todd W. Rice, Pratik Sinha, Paul J. Young, Matthew W. Semler and Matthew M. Churpek.
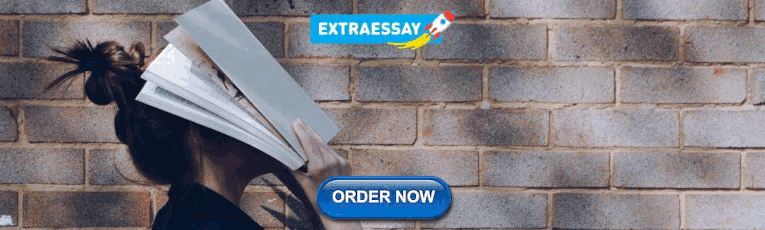
IMAGES
VIDEO
COMMENTS
And place questionnaires, copies of focus groups and interviews, and data sheets in the appendix. On the other hand, one must put the statistical analysis and sayings quoted by interviewees within the dissertation. 8. Thoroughness of Data. It is a common misconception that the data presented is self-explanatory.
The results chapter (also referred to as the findings or analysis chapter) is one of the most important chapters of your dissertation or thesis because it shows the reader what you've found in terms of the quantitative data you've collected. It presents the data using a clear text narrative, supported by tables, graphs and charts.
Types of Data Analysis for Dissertation. The various types of data Analysis in a Dissertation are as follows; 1. Qualitative Data Analysis. Qualitative data analysis is a type of data analysis that involves analyzing data that cannot be measured numerically. This data type includes interviews, focus groups, and open-ended surveys.
Empirical data: unique research that may be quantitative, qualitative, or mixed.. Theoretical data: secondary, scholarly sources like books and journal articles that provide theoretical context for your research.. Thesis: the culminating, multi-chapter project for a bachelor's, master's, or doctoral degree.. Qualitative data: info that cannot be measured, like observations and interviews.
This article is a practical guide to conducting data analysis in general literature reviews. The general literature review is a synthesis and analysis of published research on a relevant clinical issue, and is a common format for academic theses at the bachelor's and master's levels in nursing, physiotherapy, occupational therapy, public health and other related fields.
Craft a convincing dissertation or thesis research proposal. Write a clear, compelling introduction chapter. Undertake a thorough review of the existing research and write up a literature review. Undertake your own research. Present and interpret your findings. Draw a conclusion and discuss the implications.
Your research methodology discusses and explains the data collection and analysis methods you used in your research. A key part of your thesis, dissertation, or research paper, the methodology chapter explains what you did and how you did it, allowing readers to evaluate the reliability and validity of your research and your dissertation topic.
An understanding of the data analysis that you will carry out on your data can also be an expected component of the Research Strategy chapter of your dissertation write-up (i.e., usually Chapter Three: Research Strategy). Therefore, it is a good time to think about the data analysis process if you plan to start writing up this chapter at this ...
Fast-Track Your Data Analysis, Today. Enter your details below, pop us an email, or book an introductory consultation. If you are a human seeing this field, please leave it empty. Get 1-on-1 help analysing and interpreting your qualitative or quantitative dissertation or thesis data from the experts at Grad Coach. Book online now.
Chapter 3 of the dissertation provides the reader with a detailed description of the components of the method that will be used in the research. This chapter helps the reader to judge if the method used in the research provided an adequate opportunity to examine the research questions and hypotheses.
In any research, the methodology chapter is one of the key components of your dissertation. It provides a detailed description of the methods you used to conduct your research and helps readers understand how you obtained your data and how you plan to analyze it. This section is crucial for replicating the study and validating its results.
The method you choose will depend on your research objectives and questions. These are the most common qualitative data analysis methods to help you complete your dissertation: 2. Content analysis: This method is used to analyze documented information from texts, email, media and tangible items.
A dissertation is a long-form piece of academic writing based on original research conducted by you. It is usually submitted as the final step in order to finish a PhD program. Your dissertation is probably the longest piece of writing you've ever completed. It requires solid research, writing, and analysis skills, and it can be intimidating ...
Table of contents. Step 1: Define the aim of your research. Step 2: Choose your data collection method. Step 3: Plan your data collection procedures. Step 4: Collect the data. Frequently asked questions about data collection.
the research setting, the sample, instrumen-tation (if relevant), and methods of data collection and analysis used. • Rationale and significance: Rationale is the justification for the study presented as a logical argument. Significance addresses the benefits that may be derived from doing the study, thereby reaffirming the research purpose.
A research design is a strategy for answering your research question using empirical data. Creating a research design means making decisions about: Your overall research objectives and approach. Whether you'll rely on primary research or secondary research. Your sampling methods or criteria for selecting subjects. Your data collection methods.
Dissertation typically involves the exploration of a particular research question or topic in-depth, and it requires students to conduct original research, analyze data, and present their findings in a scholarly manner. It is often the culmination of years of study and represents a significant contribution to the academic field.
Over the last 80 years, ProQuest has built the world's most comprehensive and renowned dissertations program. ProQuest Dissertations & Theses Global (PQDT Global), continues to grow its repository of 5 million graduate works each year, thanks to the continued contribution from the world's universities, creating an ever-growing resource of emerging research to fuel innovation and new insights.
For students and novice researchers, the choice of qualitative approach and subsequent alignment among problems, research questions, data collection, and data analysis can be particularly tricky. ... Writing a Successful Thesis or Dissertation: Tips and Strategies for Students in the Social and Behavioral Sciences. 2008. SAGE Research Methods.
Dissertation examples. Listed below are some of the best examples of research projects and dissertations from undergraduate and taught postgraduate students at the University of Leeds We have not been able to gather examples from all schools. The module requirements for research projects may have changed since these examples were written.
Gustave Flaubert. CHAPTER 3: RESEARCH METHODOLOGY. 3.1 Introduction. As it is indicated in the title, this chapter includes the research methodology of. the dissertation. In more details, in this ...
MIT's DSpace contains more than 58,000 theses completed at MIT dating as far back as the mid 1800's. Theses in this collection have been scanned by the MIT Libraries or submitted in electronic format by thesis authors. Since 2004 all new Masters and Ph.D. theses are scanned and added to this collection after degrees are awarded.
In January 2024, College of Science Dean Morteza Khaledi appointed Wang as director for research of the new Division of Data Science, which serves as a hub to organize, provide infrastructure support and facilitate growth in instructional and research programs involving data science. ... She has directed Ph.D. dissertations for 19 students ...
I am a Professional Assignments ,Thesis , Essay, Dissertation, Projects, Emperical, Questionaire , Data Analysis, and Research writer. I am available for Complete courses of all the basic subjects as well.
In summary, this dissertation research concludes that UI benefits sustainability planning by enriching urban research and sustainability planning data, improving analytics for effective communications, and informing collective decisions. The interdisciplinary approaches enable system-thinking to balance social, economic, and environmental ...
The results chapter in a dissertation or thesis (or any formal academic research piece) is where you objectively and neutrally present the findings of your qualitative analysis (or analyses if you used multiple qualitative analysis methods ). This chapter can sometimes be combined with the discussion chapter (where you interpret the data and ...
This research explores adversarial attacks on Graph Convolutional Transformer (GCT) models that utilize Electronic Health Record (EHR) data. As deep learning models become increasingly integral to healthcare, securing their robustness against adversarial threats is critical. This research assesses the susceptibility of GCT models to specific adversarial attacks, namely the Fast Gradient Sign ...
Dario Gil, IBM senior vice president and director of research, wants you to think about artificial intelligence differently. While large language models like ChatGPT and their ability to converse may get much of the attention, Gil says the real value of AI is in the unprecedented amount of data that it can analyze and what we as humans then do with that analysis.
Pew Research Center analyzed Americans' and Israelis' attitudes on a variety of questions related to the Israel-Hamas war. The recent U.S. data is from two polls: a survey of 12,693 U.S. adults conducted Feb. 13-25, 2024, and a survey of 3,600 U.S. adults conducted April 1-7, 2024. The data for Israel is from a survey of 1,001 Israeli ...
However, prior research was unable to establish whether a higher or lower SpO 2 target is better for patients. "The standard of care is to maintain oxygen saturation between 88 and 100; within that range, doctors have had to choose an oxygen level for ventilation without having high-quality data to inform their decision-making," said Kevin ...