- Introduction
- Conclusions
- Article Information
New mutations were found among a group of 1699 Omicron viruses that had evolving core haplotypes and rendered the haplotype-based artificial intelligence model unable to identify the variant. In the heat map, wild types, Omicron mutations, and new mutations are coded as 0, 1, and 2 and are colored as white, gray, and blue, respectively.
Among 524 unassigned and unidentifiable viruses, 16 ab initio mutations were found; 8 were expanding, while the rest had negative trajectories. LAMP indicates locally averaged mutation percentage.
eTable 1. A List of Known Variants Assigned by GISAID
eTable 2. Haplotype Frequencies Among Alpha Viruses
eTable 3. Haplotype Frequencies Among Beta Viruses
eTable 4. Haplotype Frequencies Among Delta Viruses
eTable 5. Haplotype Frequencies Among Epsilon Viruses
eTable 6. Haplotype Frequencies Among Eta Viruses
eTable 7. Haplotype Frequencies Among Gamma Viruses
eTable 8. Haplotype Frequencies Among GH/490R Viruses
eTable 9. Haplotype Frequencies Among Iota Viruses
eTable 10. Haplotype Frequencies Among Kappa Viruses
eTable 11. Haplotype Frequencies Among Lambda Viruses
eTable 12. Haplotype Frequencies Among Mu Viruses
eTable 13. Haplotype Frequencies Among Omicron Viruses
eTable 14. Haplotype Frequencies Among Theta Viruses
eTable 15. Haplotype Frequencies Among Zeta Viruses
eTable 16. Concordance Analysis in the Training Set
eTable 17. Concordance Analysis in the Validation Set
eTable 18. Concordance Analysis in the Prospective Set
eTable 19. Four Mixture Variants with Omicron-Delta
eTable 20. Four Mixture Variants with Omicron-Alpha
eTable 21. Four Mixture Variants with Omicron-Zeta
eTable 22. Four Mixture Variants with Omicron-Epsilon
eTable 23. Mixture Variant and Corresponding Lineages
eTable 24. Unidentifiable Omicron Viruses and Corresponding Lineages
eTable 25. New Mutations Among Variant-Unassigned and Unidentifiable Viruses
eFigure 1. Heatmap-Representation of Selected Polymutant Temporal Profile From January 1, 2020 to March 14, 2022 Within Every Variant
eFigure 2. Misclassification Errors by Haplotype-Based Variant Prediction (HVP), When the Prediction Probability Threshold Value is Set at 0.9 to 1
eFigure 3. Temporal Patterns of Sixteen Polymutants Identified From Variant-Unassigned 524 Viruses That Are Unpredictable by HAI, Excluding Those Core Polymutants of All Fourteen Variants
Data Sharing Statement
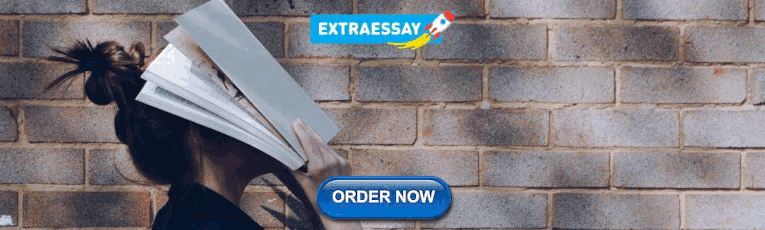
See More About
Sign up for emails based on your interests, select your interests.
Customize your JAMA Network experience by selecting one or more topics from the list below.
- Academic Medicine
- Acid Base, Electrolytes, Fluids
- Allergy and Clinical Immunology
- American Indian or Alaska Natives
- Anesthesiology
- Anticoagulation
- Art and Images in Psychiatry
- Artificial Intelligence
- Assisted Reproduction
- Bleeding and Transfusion
- Caring for the Critically Ill Patient
- Challenges in Clinical Electrocardiography
- Climate and Health
- Climate Change
- Clinical Challenge
- Clinical Decision Support
- Clinical Implications of Basic Neuroscience
- Clinical Pharmacy and Pharmacology
- Complementary and Alternative Medicine
- Consensus Statements
- Coronavirus (COVID-19)
- Critical Care Medicine
- Cultural Competency
- Dental Medicine
- Dermatology
- Diabetes and Endocrinology
- Diagnostic Test Interpretation
- Drug Development
- Electronic Health Records
- Emergency Medicine
- End of Life, Hospice, Palliative Care
- Environmental Health
- Equity, Diversity, and Inclusion
- Facial Plastic Surgery
- Gastroenterology and Hepatology
- Genetics and Genomics
- Genomics and Precision Health
- Global Health
- Guide to Statistics and Methods
- Hair Disorders
- Health Care Delivery Models
- Health Care Economics, Insurance, Payment
- Health Care Quality
- Health Care Reform
- Health Care Safety
- Health Care Workforce
- Health Disparities
- Health Inequities
- Health Policy
- Health Systems Science
- History of Medicine
- Hypertension
- Images in Neurology
- Implementation Science
- Infectious Diseases
- Innovations in Health Care Delivery
- JAMA Infographic
- Law and Medicine
- Leading Change
- Less is More
- LGBTQIA Medicine
- Lifestyle Behaviors
- Medical Coding
- Medical Devices and Equipment
- Medical Education
- Medical Education and Training
- Medical Journals and Publishing
- Mobile Health and Telemedicine
- Narrative Medicine
- Neuroscience and Psychiatry
- Notable Notes
- Nutrition, Obesity, Exercise
- Obstetrics and Gynecology
- Occupational Health
- Ophthalmology
- Orthopedics
- Otolaryngology
- Pain Medicine
- Palliative Care
- Pathology and Laboratory Medicine
- Patient Care
- Patient Information
- Performance Improvement
- Performance Measures
- Perioperative Care and Consultation
- Pharmacoeconomics
- Pharmacoepidemiology
- Pharmacogenetics
- Pharmacy and Clinical Pharmacology
- Physical Medicine and Rehabilitation
- Physical Therapy
- Physician Leadership
- Population Health
- Primary Care
- Professional Well-being
- Professionalism
- Psychiatry and Behavioral Health
- Public Health
- Pulmonary Medicine
- Regulatory Agencies
- Reproductive Health
- Research, Methods, Statistics
- Resuscitation
- Rheumatology
- Risk Management
- Scientific Discovery and the Future of Medicine
- Shared Decision Making and Communication
- Sleep Medicine
- Sports Medicine
- Stem Cell Transplantation
- Substance Use and Addiction Medicine
- Surgical Innovation
- Surgical Pearls
- Teachable Moment
- Technology and Finance
- The Art of JAMA
- The Arts and Medicine
- The Rational Clinical Examination
- Tobacco and e-Cigarettes
- Translational Medicine
- Trauma and Injury
- Treatment Adherence
- Ultrasonography
- Users' Guide to the Medical Literature
- Vaccination
- Venous Thromboembolism
- Veterans Health
- Women's Health
- Workflow and Process
- Wound Care, Infection, Healing
Get the latest research based on your areas of interest.
Others also liked.
- Download PDF
- X Facebook More LinkedIn
Zhao LP , Cohen S , Zhao M, et al. Using Haplotype-Based Artificial Intelligence to Evaluate SARS-CoV-2 Novel Variants and Mutations. JAMA Netw Open. 2023;6(2):e230191. doi:10.1001/jamanetworkopen.2023.0191
Manage citations:
© 2024
- Permissions
Using Haplotype-Based Artificial Intelligence to Evaluate SARS-CoV-2 Novel Variants and Mutations
- 1 Public Health Sciences Division, Fred Hutchinson Cancer Research Center, Seattle, Washington
- 2 Vaccine and Infectious Disease Division, Fred Hutchinson Cancer Research Center, Seattle, Washington
- 3 Department of Medicine, University of Washington School of Medicine, Seattle
- 4 QuantFu Inc, Boston, Massachusetts
- 5 Quintepa Computing LLC, Nashville, Tennessee
- 6 Department of Chemistry, Vanderbilt University; Nashville, Tennessee
- 7 Clinical Research Division, Fred Hutchinson Cancer Research Center, Seattle, Washington
Question Could viral genetic mutations and associated haplotypes be used to identify emerging novel SARS-COV-2 variants?
Findings In this cross-sectional study, a haplotype-based artificial intelligence (HAI) model was trained on more than 5 million viral sequences to identify emerging novel SARS-COV-2 variants due to the acquisition of new mutations or mixture of mutations from multiple variants. Applying HAI to 344 901 viral sequences identified 7 mixture variants (eg, Omicron-Alpha, Omicron-Epsilon, Omicron-Zeta, and Alpha-Epsilon) and 16 novel mutations, 8 of which were increasing in prevalence percentage in the earlier part of May 2022.
Meaning The successful application of HAI in this study suggests its utility in identifying novel emerging SARS-COV-2 variants even if such variants have not been observed previously.
Importance Earlier detection of emerging novel SARS-COV-2 variants is important for public health surveillance of potential viral threats and for earlier prevention research. Artificial intelligence may facilitate early detection of SARS-CoV2 emerging novel variants based on variant-specific mutation haplotypes and, in turn, be associated with enhanced implementation of risk-stratified public health prevention strategies.
Objective To develop a haplotype-based artificial intelligence (HAI) model for identifying novel variants, including mixture variants (MVs) of known variants and new variants with novel mutations.
Design, Setting, and Participants This cross-sectional study used serially observed viral genomic sequences globally (prior to March 14, 2022) to train and validate the HAI model and used it to identify variants arising from a prospective set of viruses from March 15 to May 18, 2022.
Main Outcomes and Measures Viral sequences, collection dates, and locations were subjected to statistical learning analysis to estimate variant-specific core mutations and haplotype frequencies, which were then used to construct an HAI model to identify novel variants.
Results Through training on more than 5 million viral sequences, an HAI model was built, and its identification performance was validated on an independent validation set of more than 5 million viruses. Its identification performance was assessed on a prospective set of 344 901 viruses. In addition to achieving an accuracy of 92.8% (95% CI within 0.1%), the HAI model identified 4 Omicron MVs (Omicron-Alpha, Omicron-Delta, Omicron-Epsilon, and Omicron-Zeta), 2 Delta MVs (Delta-Kappa and Delta-Zeta), and 1 Alpha-Epsilon MV, among which Omicron-Epsilon MVs were most frequent (609/657 MVs [92.7%]). Furthermore, the HAI model found that 1699 Omicron viruses had unidentifiable variants given that these variants acquired novel mutations. Lastly, 524 variant-unassigned and variant-unidentifiable viruses carried 16 novel mutations, 8 of which were increasing in prevalence percentages as of May 2022.
Conclusions and Relevance In this cross-sectional study, an HAI model found SARS-COV-2 viruses with MV or novel mutations in the global population, which may require closer examination and monitoring. These results suggest that HAI may complement phylogenic variant assignment, providing additional insights into emerging novel variants in the population.
The COVID-19 pandemic is gradually shifting to an endemic phase with continuously circulating SARS-COV-2 variants globally. The presence of multiple viral variants increases co-infection risks, which may lead to recombinants (eg, an Alpha-Omicron mixture) as new, emerging variants. 1 - 5 In addition, every infection could recombine with mutations from other viruses, 3 host genetic sequences, 6 or zoonotic events, 7 with saltational outcomes that may lead to new variants. Most mutations are functionally neutral, appearing and waning randomly, but some may persist because they impart increased transmissibility or virulence. Therefore, detecting new variants is of importance, for example, to facilitate early viral control measures and enhanced lead time to research and develop effective preventive and treatment strategies.
Prevailing methods of identifying variants assign sequenced viruses to known clades and lineages using phylogenic methods. 8 - 11 When a group of viral clades or lineages emerges rapidly and exhibits excessive transmissibility, virulence, or evasion of host immunity, these variants are classified as variants of interest and variants of concern by an expert panel of the World Health Organization (WHO) 12 and are classified further as variants being monitored or variants of high consequence by the US Centers for Disease Control and Prevention (CDC). 13 Currently, phylogenic methods 14 - 16 are routinely applied in classifying all viruses, and assigned lineages and clades are accepted by WHO 17 and the CDC 13 to identify variants and declare the emergence of new variants. However, such variant assignments may be uncertain when multiple variants are recombined and the assumption of branching phylogenic trees, required by most phylogenic methods in use, is violated. Ignoring this violation could bias phylogenetic inferences. 18 , 19 When applied to classifying SARS-COV-2, conventional phylogenic analysis may force assignment of a recombinant variant to an existing variant (ie, a misclassification error) or may miss the recombinant variant (ie, a missing data error).
There are alternative approaches for identifying mutations in SARS-COV-2. One approach is to estimate mutational drivers of individual genes based on amino acid substitutions in individual SARS-COV-2 genes. 20 Another approach is an empirical statistical learning strategy (SLS) that selects individual polymorphic amino acid sites (hereafter, polymutants ), models their temporal patterns over time, and identifies haplotypes based on a set of polymutants that share synchronized expansion patterns. 21 The primary limitation of these 2 alternative approaches is the lack of direct linkage of specific mutations or polymutants with variant assignments, which makes interpretation difficult.
Using existing analytic approaches and the large viral sequence database at Global Initiative on Sharing Avian Influenza Data (GISAID), 22 - 24 we sought to build a haplotype-based artificial intelligence (HAI) model for identifying SARS-COV-2 novel variants using variant-specific polymutants and their haplotypes. In addition to identifying variants, the HAI model was designed to discover novel variants with no need for the branching phylogenic trees assumption. Conceptually, the HAI model learned from the large collection of viral sequences in GISAID to identify core polymutants that were specific to viral variants. Through a haplotype analysis, the HAI model estimated haplotype frequencies of variant-specific core polymutant haplotypes. Applying Bayes’ theorem, HAI computed identification probabilities corresponding to all known variants. By a chosen threshold probability, estimated variant identification probabilities were used to identify the variant under which each virus should be classified, including variant-unidentifiable viruses with novel mutations. If variant identification was ambiguous, with 2 or more identification probabilities greater than a prespecified threshold, the result implied that the viral genome had appreciable probabilities of carrying corresponding variant-specific core haplotypes (ie, a mixture of corresponding variants), possibly due to recombination. From GISAID, we obtained 10.5 million viral sequences (downloaded on March 14, 2022), with half as training set and the rest as a validation set, to develop and validate the HAI model. To demonstrate its identification performance, we used pooled data to build the final HAI model and applied it to a prospective set of 344 901 viruses collected from March 15 to May 18, 2022. Using identification results from the prospective set, we explored mixture variants (MVs) and viruses with novel mutations to gain insights into emerging SARS-COV-2 variants.
Because GISAID data may be considered as observational routinely collected health data, they are reported following the Reporting of Studies Conducted Using Observational Routinely-collected Health Data (RECORD) guideline. 22 This study was determined to be exempt from review by Fred Hutchinson Research Center institution review board and informed consent was waived because the identity of the human participants cannot readily by ascertained directly or through identifiers linked to the participants, in accordance with 45 CFR §46.104(d)(4).
GISAID is a central data portal for storing genomic sequences for coronaviruses in the COVID-19 pandemic. 23 , 25 , 26 Given the large sample size and rapid accumulation of viral sequences at GISAID, we designed this study in 2 phases. The first phase was to train and validate an HAI model, while the second phase was to assess the performance of HAI on a prospectively collected set of viruses.
Accessing GISAID on March 14, 2022, we retrieved all available samples collected between January 1, 2020, and March 14, 2022 (10 450 718 samples). We filtered out samples if viral sequences had fewer than 27 000 nucleotides (119 277 samples [1.1%]), collection dates were incomplete (290 917 samples [2.8%]), or collection dates were prior to January 1, 2020 (33 samples [0.01%]), netting a total of 10 051 620 viruses for this development. By random sampling, half were selected into the training set and the rest into the validation set. For the second-phase analysis, we retrieved samples collected by May 18, 2022; excluded samples collected prior to March 14, 2022; and retained 344 901 viruses in the prospective data set.
GISAID aligns submitted viral sequences, translates these to amino acids, assigns lineages, extracts mutations (substituting mutations, insertions, and deletions), and disseminates assigned lineages, clades, variants, and sequence mutating amino acids through patient-specific metadata. Mutating amino acids, if they have 3 or more observations, are extracted as viral polymutants to be analyzed. Multiple polymutants from a single virus form a polymutant haplotype because an RNA virus is single stranded. As of May 18 2022, there were 14 variants officially assigned at GISAID (eTable 1 in Supplement 1 ).
Metadata included sample collection location and date. The location was organized by continent, country, region, and subregion and had no missing data. A fraction of collection dates were missing completely or partially. Location and date information allowed geographic and temporal analysis of polymutant haplotypes.
We applied an SLS to develop an HAI model, details of which are provided in eMethods in Supplement 1 . Briefly, the SLS included a generalized additive model that was used to select variant-specific polymutants, a haplotype analysis to estimate frequencies of core haplotypes within each variant, a Bayes probabilities to estimate variant-specific posterior probabilities, and an unsupervised learning technique to organize temporal patterns.
SARS-COV-2 viruses are classified in clades and lineages by GISAID based on whole viral genome sequences 27 and are assigned to variants by GISAID (eTable 1 in Supplement 1 ). Characteristically, each variant has a group of amino acid substitutions (ie, variant-specific polymutants). To identify such polymutants, we used the training set and extracted polymorphic amino acids from viruses of a specific variant. By comparing observed amino acids against their references, SLS recognized whether amino acids are substitutions and created a binary mutation indicator of 1 or 0, respectively. Associating mutation indicators with collection dates via a generalized additive model, SLS modeled temporal expansions of individual amino acids, based on which locally averaged mutation percentages (LAMP) over time (see eFigure 1 in Supplement 1 for variant-specific expansions) were estimated along with a P value quantifying whether temporal trends were significant. We considered a substitution as a variant-specific polymutant if its P value was less than. 05 and its maximum LAMP at any time exceeded 10% or if the mean LAMP was greater than 0.5. For all SARS-COV-2 variants (Alpha, Beta, Delta, Epsilon, Eta, Gamma, GH/490R, Iota, Kappa, Lambda, Mu, Omicron, Theta, and Zeta), SLS identified 19, 20, 33, 14, 14, 21, 24, 21, 25, 21, 32, 63, 26, and 10 polymutants, respectively (eTable 2-15 in Supplement 1 ). Using viral sequences, SLS performed a haplotype analysis to estimate haplotype frequencies, referred to as frequencies of core variant haplotypes (listed in eTables 2-15 in Supplement 1 ). Empirically, proportions of SARS-COV-2 variants in the general population were estimated in the training set, denoted as f (variant = v ).
By the Bayes theorem, HAI computes probabilities of observing a variant v , given viral genome (ie, polymutant haplotype), via the following formula:
p (Variant = v | h ) = [ f ( h |Variant = v ) f (Variant = v )]/ f ( h |Unassigned) f (Unassigned) + Σ v f ( h | v ) f ( v )
in which the summation Σ v is over all 14 known variants, haplotype frequency f (h | variant = v ) and variant proportion f ( variant = v ) are empirically estimated from the training set, in addition to f ( h | Unassigned) and f (Unassigned) for variant-unassigned viruses. For each viral sequence, HAI computed an array of variant probabilities. Given the threshold value p v = 0.99 for classifying a variant, HAI classified a virus to variant v if the corresponding probability was greater than p v . On the training set, we tabulated concordances of HAI classifications and GISAID assigned variants, which are displayed as a 16 by 15 contingency table (eTable 16 in Supplement 1 ), that is, 14 known variants and an unassigned virus by GISAID, and 14 identified known variants, 1 unidentifiable variant, and MVs that may be recombinants. For all 5 025 810 virus sequences, the concordance rate of HAI and GISAID variant assignments was 4 326 921 sequences (86.1%), while the discordance rate was nearly zero (5026 sequences [<0.1%]) ( Table 1 ). Among 543 402 unassigned viruses, 175 434 viruses (3.5%) viruses were assigned to 1 known variant and 7633 viruses (0.2%)that were not assigned variants were identified as MVs. Meanwhile, for 4 482 408 viruses with assigned variants, 159 272 viruses (3.6%) were identified as MVs and 7633 viruses (0.1%) were deemed unidentifiable. Finally, 360 335 viruses (7.2%) received no variant assignment by GISAID or identification by HAI. Note that we profiled the choice of threshold value p v from 0.90 to 1.00 and found that the choice of 0.99 was associated with a minimum number of 53 discordances (eFigure 2 in Supplement 1 ). Additionally, note that use of concordance and discordance was suboptimal given that identified variants were not present in the training set.
Using the same data-processing protocol, we extracted all variant-specific core haplotypes of selected polymutants in the validation set. Using estimated haplotype frequencies and variant proportions, we computed the variant identification probability by the previously described equation. With the chosen threshold, we identified the virus to be a known variant, a mixture of known variants, or an unidentifiable variant. Comparing identifiable variants (by rows) against variant assignment (by columns) by GISAID, we tabulated their concordances and discordances ( Table 2 ). Concordant assignments of known variants by HAI and GISAID are shown along the diagonal line. Results from the concordance analysis in the validation data set were comparable to those in the training data set ( Table 1 ). For example, the estimated concordance between identified and assigned variants was 86.1% and 86.3% in training and validation sets, respectively. We evaluated the concordance between HAI and GISAID, which was measured by a κ statistic, 28 measuring concordances between GISAID assignments and HAI identifications of 14 known variants, yielding a κ value of nearly 1.00. The κ value, after including unassigned and mixture or unidentifiable viruses, was 0.91.
The successful validation suggested that HAI-identified variants were highly concordant with GISAID assignments. Integrated variant assignment and identification provided additional insights into emerging novel variants. To evaluate practical utility, we pooled training and validation sets to build a final HAI model with 10 051 620 viral sequences and repeated the same SLS process, except estimating variant proportions with viruses collected from March 15, 2021, to March 14, 2022. The concordance analysis of HAI and GISAID variant assignment on the full data set is shown in eTable 17 in Supplement 1 , and estimated concordance and discordance rates were comparable to training set results ( Table 1 ).
Applying the final HAI model to 344 901 prospectively collected viruses, we found that the most common variant was Omicron (343 592 viruses [99.6%]), while there were 2 Alpha, 180 Delta, and 1 Lambda variant viruses (eTable 18 in Supplement 1 ; Table 3 ); 1126 viruses were not assigned to any variants. HAI, on the other hand, identified additional variants (Epsilon, Eta, and Zeta) and 2227 MVs (eTable 18 in Supplement 1 ). To assess which MVs were likely recombinants, we applied a postidentification procedure (eMethods in Supplement 1 ) under the assumption that if a mixture was from recombination, it must include unique core polymutants to the corresponding variants in the mixture. Most MVs had only Omicron polymutants (647 of 657 variants [98.5%]) ( Table 3 ), and no MVs had polymutants from 3 or more variants; the remaining MVS were classified as 1 of 7 specific MVs (3 Delta-Kappa, 2 Delta-Zeta, 10 Alpha-Epsilon, 25 Omicron-Alpha, 3 Omicron-Delta, 609 Omicron-Epsilon, and 10 Omicron-Zeta MVs). Finally, the HAI model left 2227 viruses unidentified, which included 4 Delta and 1699 Omicron variants. Concordance and discordance rates were 92.776% (95% CI, 92.775%-92.777%) and 0.004% (95% CI, 0.003%-0.005%), respectively ( Table 1 ). Through a formal concordance κ analysis, the κ value for known variants was estimated at 0.96 (95% CI, 0.97-1.00).
Co-infection could lead to the recombination of 2 variants and the formation of a recombinant, which could empirically be observed as an MV. To identify specific mixtures, we defined a specific MV if the virus carried at least 1 mutating polymutant unique to respective variants. The application of postidentification processing identified a set of potential recombinants ( Table 3 ). The most frequently occurring recombinant type among all 657 MVs was Omicron-Epsilon (609 recombinants [92.7%]). Among all recombinants, the likely most well-known and controversial recombinant is the Omicron-Delta recombinant. 29 - 31 Profiling Delta and Omicron polymutants on these 2 recombinants (eTable 19 in Supplement 1 ), we found that the virus carried L452R and I82T polymutants unique to Delta, while the remaining polymutants were unique to Omicron. Similarly, the Omicron-Alpha recombinants carried T183I, S982A, R52I, D3L, and S235F mutations unique to Alpha, while Omicron-Zeta recombinants carried L71F, A119S, and M234I mutations unique to Zeta (eTable 20 in Supplement 1 ). Omicron-Epsilon recombinants carried T85I, I65V, L452R, R57H, and T205I mutations unique to Epsilon (eTable 21 in Supplement 1 ). To profile the epidemiological distribution of Omicron-Epsilon recombinants, we tabulated their geographic and temporal distribution with respect to collection date and location (eTable 22 in Supplement 1 ).
Crosstabulating MVs with assigned lineages (eTable 23 in Supplement 1 ), we noted that Delta recombinants with Kappa and Zeta variants were more frequently assigned to AY lineages and Omicron recombinants were more frequently assigned BA lineages. An Omicron-Epsilon recombinant was assigned to BA.4, while an Omicron-Alpha recombinant was assigned to BA.5.
Among 343 592 Omicron viruses, 1699 viruses were found to be unidentifiable by HAI because the observed haplotypes were not part of any previously observed Omicron core haplotype. Thus, we hypothesized that some Omicron viruses may have rapidly acquired new mutations. To identify new mutations acquired by these Omicron viruses, we applied an unsupervised learning technique to organize a matrix of mutation indicators for amino acids in reference virus, Omicron-specific mutations, and newly acquired mutations ( Figure 1 ). Biclustering of polymutant similarities was associated with clustered viruses (O1, O2, O3, and O4) and clustered Omicron polymutants (G1, G2, G3, and G4). Other than viruses in cluster O4, most viruses displayed sporadic mutations; however, S371 in the spike protein acquired a new mutation, S371F, while most Omicron viruses exhibited S371L mutations, in addition to a few random substitutions (Y, A, C, and deletion). E484, S477, T478, Q493, Y505, Q498, and N501 also acquired comparatively few such mutations. To gain insights into the mutation at S371, we crosstabulated collection dates and countries and found that this mutation was first sequenced in Europe and was spreading to other countries. Viruses in the group O4 were assigned to lineages and Omicron, but no polymutants were listed, which may be associated with data processing errors at GISAID.
In crosstabulating cluster group (O1, O2, O3, and O4) with lineages (eTable 24 in Supplement 1 ), we found that most viruses in the O1 group belonged to BA.1 and BA.2, but the group also included 1 BA.4 and 3 BA.5 variants, in addition to including the 8 XE variant. The viruses in group O2 were predominantly BA.1 variants, while those in group O3 were predominantly BA.2 variants.
Among all 1126 unassigned viruses, 524 viruses were deemed unidentifiable by the HAI model. These unassigned and unidentifiable viruses may have acquired novel mutations. Applying SLS, we modeled the temporal expansions of polymutants in this set and selected 56 polymutants by their significant and substantial temporal expansions ( P value < .05 and LAMP max > 0.5). Excluding polymutants that were part of variant-specific core polymutants, we found 16 new polymutants (N-E31, N-R32, N-S33, NS3-H78, NSP1-F143, NSP1-K141, NSP1-S142, NSP2-F356, NSP6-F108. NSP6-G107, NSP6-L105, NSP6-S106, spike-A684, spike-I68, spike-L24, and spike-P25) (eTable 25 in Supplement 1 ). Application of unsupervised learning yielded 6 groups of polymutants by their temporal trends (eFigure 3 in Supplement 1 ). Visually, 8 polymutants (NSP1-K141/S142/F143, NS3-H78, and spike-L24/P25/I68/A684) in groups 1, 3, and 4 were expanding ( Figure 2 ), while the remaining polymutants, with varying LAMP levels (NSP2-F356, NSP6-L105/S106/G107/F108, and N-E31/R32/S33) were declining ( Figure 2 ). L24 and P25 of the spike protein were expanding at faster trajectories, while H78 of NS3 was expanding rapidly. There were 2 spike polymutants (I68 and A684) and 3 polymutants (K141, S142, F143) that overlapped with NSP1 that were increasing. The remaining 8 polymutants, with varying LAMP levels, were declining.
In this cross-sectional study, we described an HAI model for identifying novel SARS-COV-2 variants that was trained and validated with approximately 10 million viral sequences. Applying HAI to a prospective set of viruses collected between March 15 and May 18, 2022, we found that the HAI model achieved 93% concordance with GISAID assignments, with a 0.003% discordance rate. The HAI model was able to identify MVs and variants with novel mutations. From more than 340 000 viruses, the HAI model identified 7 unique MVs (Omicron-Alpha, Omicron-Delta, Omicron-Epsilon, Omicron-Zeta, Alpha-Epsilon, Delta-Kappa, and Delta-Zeta). It was also of interest to discover that Omicron polymutants continued to acquire novel mutations. For example, S371 in the spike protein was commonly substituted with S371L among Omicron viruses but was subsequently increasingly substituted by S371F. These S371L/F mutations, commonly observed for BA.1 and BA.2, may have been associated with a perturbation of spike trimer conformational dynamics. 32 Additionally, 8 novel mutations (NSP1-K141/S142/F143, NS3-H78, and spike-L24/P25/I68/A684) appeared to be increasing in prevalence recently and may require careful monitoring.
HAI treated GISAID assignment as a standard criterion in the training process, although some assignments may be subject to misclassification errors. Fortunately, such misassignments may be few in the current GISAID given that co-infections were exceptionally rare until recent months. Hence, imperfect training data may have had limited impact on the validity of the HAI. Furthermore, its empirical nature, relying on statistical learning strategies, tends to be robust despite a few misclassification errors.
The HAI method may be routinely used to identify important MVs in the future. For example, the Delta variant carries mutations that are associated with disease severity and hospitalization risk. 33 While Delta-Omicron recombinants are rare thus far, a highly transmissible variant, like Omicron, if recombining with virulent variants, 33 would be cause for concern. Hence, early identification of such MVs may be crucial for effective public health planning.
The approached described in this study is complementary to phylogenic-based variant assignment by GISAID, with the added benefit of timely identification of novel variants that may not otherwise become apparent at early stages. Rapid identification of these variants via the HAI, in addition to geographic and temporal localization, may facilitate correlation of specific variants with clinical outcomes assessable through electronic health records. 24 , 34 It has the potential to inform a broad range of public health strategies, including heightened surveillance, diagnostics, therapeutics, and even vaccine strategies depending on the variant haplotype.
While the HAI model demonstrated clear advantages, we need to be mindful of this study’s limitations. Perhaps the most substantial limitation was that an identified MV may not necessarily arise from recombination due to co-infection. An alternative process is that reinfection may lead to an MV. Sequence contamination may falsify an MV, but such MVs may be rare (1 or 2 copies). Hence, identified MVs may need to be investigated experimentally. Another limitation is that the current HAI is trained and validated with global data collected over the past 2 years. Its identification performance may need to be optimized for specific geographic regions, and it may need to be updated continuously to incorporate newly collected viral sequences. For example, since May 18, 2022, Omicron has evolved into multiple lineages, and HAI may need to account for these lineages. Additionally, our HAI model has several tunable parameters, which may be associated with identification performance. Further research may be necessary to improve robustness and performance of HAI identifications.
In this cross-sectional study, we described an HAI model to detect novel SARS-COV-2 variants. Applying HAI to 344 901 sequences submitted to GISAID globally from March 15 to May 18, 2022, we found that several new MVs were circulating globally and that several novel mutations were expanding recently. We have implemented the HAI model in a web-based calculator 35 for use by the community to facilitate discovery of novel variants.
Accepted for Publication: January 5, 2023.
Published: February 21, 2023. doi:10.1001/jamanetworkopen.2023.0191
Open Access: This is an open access article distributed under the terms of the CC-BY License . © 2023 Zhao LP et al. JAMA Network Open .
Corresponding Authors: Lue Ping Zhao, PhD, Public Health Sciences Division, Fred Hutchinson Cancer Research Center, 1100 Fairview Ave N, Seattle, WA 98109 ( [email protected] ); Lawrence Corey, MD, Vaccine and Infectious Disease Division, Fred Hutchinson Cancer Research Center, 1100 Fairview Ave N, Seattle, WA 98109 ( [email protected] ).
Author Contributions: Dr L. Zhao had full access to all of the data in the study and takes responsibility for the integrity of the data and the accuracy of the data analysis.
Concept and design: L. Zhao, Cohen, Payne, Jerome, Corey.
Acquisition, analysis, or interpretation of data: L. Zhao, Cohen, M. Zhao, Madeleine, Lybrand, Geraghty, Jerome.
Drafting of the manuscript: L. Zhao, Cohen, M. Zhao, Payne, Lybrand.
Critical revision of the manuscript for important intellectual content: L. Zhao, Cohen, Madeleine, Payne, Lybrand, Geraghty, Jerome, Corey.
Statistical analysis: L. Zhao.
Obtained funding: Geraghty, Jerome, Corey.
Administrative, technical, or material support: Cohen, M. Zhao, Payne, Jerome.
Supervision: Cohen, Jerome, Corey.
Conflict of Interest Disclosures: None reported.
Funding/Support: This study was supported by grants UM1 AI68614 and UM1 AI068635 from the National Institutes of Health National Institute of Allergy and Infectious Diseases.
Role of the Funder/Sponsor: The National Institutes of Health National Institute of Allergy and Infectious Diseases had no role in the design and conduct of the study; collection, management, analysis, and interpretation of the data; preparation, review, or approval of the manuscript; and decision to submit the manuscript for publication.
Data Sharing Statement: See Supplement 2 .
Additional Contributions: The authors would like to acknowledge all individuals and affiliated laboratories that contributed viral sequence data to the Global Initiative on Sharing Avian Influenza Data (GISAID) by May 18, 2022, and equally to those at GISAID who assemble and organize this enormous data resource for the world community to learn about SARS-COV-2. Additionally, we would like to thank Craig A Magaret, MS (Fred Hutchinson Cancer Center), and Dan Tenenbaum (Fred Hutchinson Cancer Centernce (HAI), via Fred Hutchinson Cancer Center. They were not compensated for these contributions.
- Register for email alerts with links to free full-text articles
- Access PDFs of free articles
- Manage your interests
- Save searches and receive search alerts
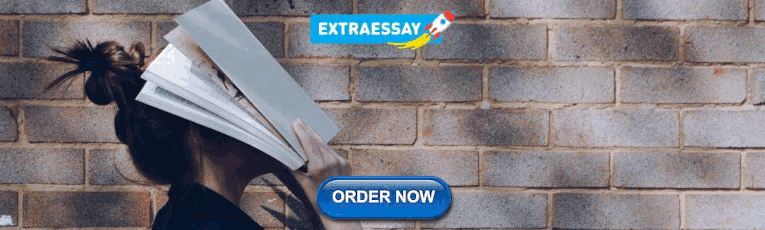
cppreference.com
Std::variant<types...>:: operator=.
Assigns a new value to an existing variant object.
- If both * this and rhs are valueless by exception, does nothing.
- Otherwise, if rhs is valueless, but * this is not, destroys the value contained in * this and makes it valueless.
- Otherwise, if rhs holds the same alternative as * this , assigns the value contained in rhs to the value contained in * this . If an exception is thrown, * this does not become valueless: the value depends on the exception safety guarantee of the alternative's copy assignment.
- Otherwise, if the alternative held by rhs is either nothrow copy constructible or not nothrow move constructible (as determined by std::is_nothrow_copy_constructible and std::is_nothrow_move_constructible , respectively), equivalent to this - > emplace < rhs. index ( ) > ( * std:: get_if < rhs. index ( ) > ( std:: addressof ( rhs ) ) ) . * this may become valueless_by_exception if an exception is thrown on the copy-construction inside emplace .
- Otherwise, equivalent to this - > operator = ( variant ( rhs ) ) .
- Otherwise, if rhs holds the same alternative as * this , assigns std :: move ( * std:: get_if < j > ( std:: addressof ( rhs ) ) ) to the value contained in * this , with j being index() . If an exception is thrown, * this does not become valueless: the value depends on the exception safety guarantee of the alternative's move assignment.
- Otherwise (if rhs and * this hold different alternatives), equivalent to this - > emplace < rhs. index ( ) > ( std :: move ( * std:: get_if < rhs. index ( ) > ( std:: addressof ( rhs ) ) ) ) . If an exception is thrown by T_i 's move constructor, * this becomes valueless_by_exception .
- Determines the alternative type T_j that would be selected by overload resolution for the expression F ( std:: forward < T > ( t ) ) if there was an overload of imaginary function F ( T_i ) for every T_i from Types... in scope at the same time, except that:
- An overload F ( T_i ) is only considered if the declaration T_i x [ ] = { std:: forward < T > ( t ) } ; is valid for some invented variable x ;
- If * this already holds a T_j , assigns std:: forward < T > ( t ) to the value contained in * this . If an exception is thrown, * this does not become valueless: the value depends on the exception safety guarantee of the assignment called.
- Otherwise, if std:: is_nothrow_constructible_v < T_j, T > || ! std:: is_nothrow_move_constructible_v < T_j > is true , equivalent to this - > emplace < j > ( std:: forward < T > ( t ) ) . * this may become valueless_by_exception if an exception is thrown on the initialization inside emplace .
- Otherwise, equivalent to this - > emplace < j > ( T_j ( std:: forward < T > ( t ) ) ) .
This overload participates in overload resolution only if std:: decay_t < T > (until C++20) std:: remove_cvref_t < T > (since C++20) is not the same type as variant and std:: is_assignable_v < T_j & , T > is true and std:: is_constructible_v < T_j, T > is true and the expression F ( std:: forward < T > ( t ) ) (with F being the above-mentioned set of imaginary functions) is well formed.
[ edit ] Parameters
[ edit ] return value, [ edit ] exceptions, [ edit ] example.
Possible output:
[ edit ] Defect reports
The following behavior-changing defect reports were applied retroactively to previously published C++ standards.
[ edit ] See also
- conditionally noexcept
- Recent changes
- Offline version
- What links here
- Related changes
- Upload file
- Special pages
- Printable version
- Permanent link
- Page information
- In other languages
- This page was last modified on 12 October 2023, at 13:04.
- This page has been accessed 68,830 times.
- Privacy policy
- About cppreference.com
- Disclaimers

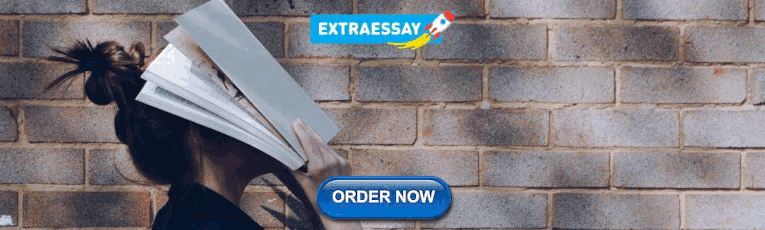
IMAGES
COMMENTS
Ignoring this violation could bias phylogenetic inferences. 18,19 When applied to classifying SARS-COV-2, conventional phylogenic analysis may force assignment of a recombinant variant to an existing variant (ie, a misclassification error) or may miss the recombinant variant (ie, a missing data error).
template<class T >constexpr variant& operator=( T&& t )noexcept(/* see below */); (since C++20) Assigns a new value to an existing variant object. 1) Copy-assignment: If both *this and rhs are valueless by exception, does nothing. Otherwise, if rhs is valueless, but *this is not, destroys the value contained in *this and makes it valueless ...
Edit. Ok, I mulled over this and I think I found a better solution that comes closer to what you had in mind: Public Sub LetSet(ByRef variable As Variant, ByVal value As Variant) If IsObject(value) Then. Set variable = value. Else. variable = value. End If. End Sub.