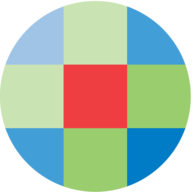
- Subscribe to journal Subscribe
- Get new issue alerts Get alerts
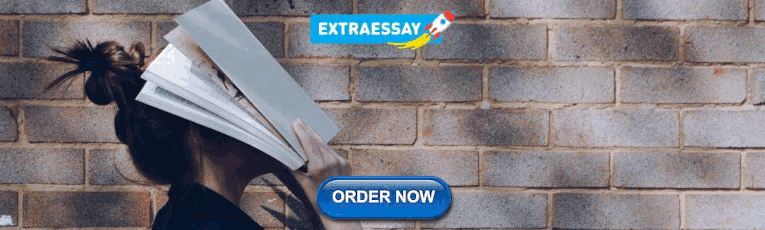
Secondary Logo
Journal logo.
Colleague's E-mail is Invalid
Your message has been successfully sent to your colleague.
Save my selection
Theory Use and Usefulness in Scientific Advancement
Pickler, Rita H.
Rita H. Pickler, PhD, RN, FAAN, is Editor of Nursing Research .
The Editor has no conflicts of interest to disclose.
Accepted for publication November 20, 2017.
Corresponding author: Rita H. Pickler, PhD, RN, FAAN, The FloAnn Sours Easton Professor of Child and Adolescent Health and Director, PhD & MS in Nursing Science Programs, The Ohio State University College of Nursing, 324 Newton Hall, 1585 Neil Avenue Columbus, OH 43210 (e-mail: [email protected] ).
This issue of Nursing Research is focused on theory in nursing, including how theories historically developed in nursing and how they have evolved over time. Also included in this issue are new thoughts on theory development as well as emergent theoretical ideas. But to what end is theory in the rapidly changing scientific world? Do theories and, particularly, “grand” theories of the sort that many of us of a “certain age” were required to “use” in our own first efforts at research lead to scientific advancement? Is theory useful in today’s seemingly atheoretical age where new scientific “discoveries” are announced daily on social media? Do we need theory to advance science?
Like most scientists, I was taught very early in my training that a theory is a set of interrelated concepts and propositions that explains or predicts events or situations. But I also learned that what “counts” as theory could be many different things depending on who you ask. A theory could be “grand” or macro level, setting forth the phenomena of interest to the discipline using rather abstract concepts. A theory could be “grounded,” addressing contextual explanations of very specific phenomena experienced by select people. Or a theory could be “middle range” or meso level, aimed at integrating empirical research and focused on linking macro and micro level concepts and theories. Clear in all of this was the idea that theories are abstract, varying in the extent to which they are conceptually developed and empirically tested. However, in the end, theory development and testing were deemed to be critically important to advancing science.
Scientific advancement is of course critical to any discipline, and nursing is no different; science is generally thought to advance when theories exist that explain and predict phenomena. A full discussion of how science advances is beyond the scope of this editorial. However, whether you think science advances only when current theories fail to explain the phenomena of interest—resulting in a paradigm shift—or at the other extreme that science advances by a steady accumulation of knowledge that occurs with rigorous and repeated theory testing, theory and scientific advancement would appear to interact. But is theory necessary to science? More importantly, should all science or research within a scientific field be focused on theoretical development and testing. I certainly consider myself a scientist. Am I also a theorist?
The answers to these questions, I believe is, “perhaps.” Indeed, perhaps theory is necessary for scientific advancement. This would particularly be the case if theories for nursing science were focused on understanding, explaining, and predicting those phenomena that are of interest to our discipline and, by extension, to our practice. Our conundrum here is that the phenomena of interest in nursing science and practice are highly varied and—importantly—not agreed upon by the discipline. Many years ago, the historically important grand theories identified broad phenomena of interest—person, environment, health, and nursing. In the ensuing years, these broad phenomena have been challenged, modified, or even discounted as meaningless because of their broadness. And yet, it seems that much of what we study as nurse scientists does fall into one or more of these broad categories of phenomena. That begs a further question about the utility of grand theories: Have grand theories resulted in a clear definition of nursing science? However, from an historical perspective, the grand theories have helped advance nursing science by articulating these phenomena, even if we choose to let the phenomena represent almost anything we wish to study.
Thus, we acknowledge the importance of grand theories to explication of phenomena of interest to nursing science. Regrettably, grand theories do not particularly help us to understand relationships among these broad phenomena. Rather, we need middle range or grounded theories to explicate relationships among concepts embedded within these phenomena in order that scientists can test those proposed relationships. But should all our research be focused on the development and testing of these theories? Perhaps. Certainly it would be hard to document that our science is advancing in this way; many research papers do not name a theory upon which the work is based nor identify a theoretical model being tested. Most rare of all are papers reporting on a theory that has been developed. If theory is necessary for scientific advancement, nurse scientists need to pay greater attention to theory use in their research and their research reports. Certainly if theory has been used to inform research, that use should be clearly articulated. And if theories are tested, then scientists should report on how the theory actually performed.
Rather than clear delineation of theory use, much reported nursing science is a collection of empirical studies on many phenomena of interest. Rarely do we have clear understanding of how or why the results of these studies contribute to scientific advancement within the discipline. Perhaps as a scientific community, we do not think this is necessary. Or perhaps we need to encourage theory development using the empirical evidence we have collected over many years and many studies. I might hazard a guess that many of us who have been studying very specific phenomena over a long period of time have sufficient data accumulated from which theories could be developed and tested. Certainly we need more “basic” research to develop and test theories, particularly those that may lead to interventions designed to improve patient outcomes. Greater rigor and precision in theory development and testing is much needed.
Science advances because of and sometimes in spite of our efforts. Theory should help that advancement, providing explanations of phenomena and their relationships. Using theory in research allows us to refine the definitions and scope of the discipline of nursing. Theories may change and the way that they are interpreted may change, but these changes and interpretations based on observations are most likely to result in advancements because they occur within the context of theory. In nursing science, given the broad scope of our interests, we are likely to need many theories to describe, explain, and predict the complexity in the relationships among those concepts.
Theories have long been thought to be the foundations for furthering scientific knowledge and for putting the information gathered through empirical research into practice. Indeed, perhaps more than ever, clear use of theory in research may be even more important to further advance nursing science. Thus, the question is not: should we use theory in our scientific work? The question is: are we making explicit the theories we are using? The papers collected in this issue will hopefully advance science by reminding us of important past theoretical work and providing guidance for the way forward. To each of us engaged in nursing science, our challenge is to carefully consider the function of theory in our own work and to further advance nursing science by making theory use more evident in our research reports.
nursing research; nursing science; nursing theory; scientific advancement
- + Favorites
- View in Gallery
Readers Of this Article Also Read
Key issues in nursing theory: developments, challenges, and future directions, the fundamentals of care framework as a point-of-care nursing theory.
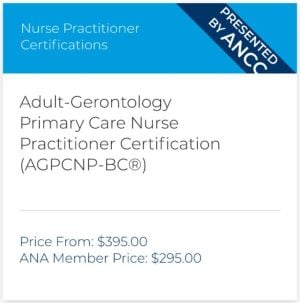
ANA Nursing Resources Hub
Search Resources Hub

What Is Nursing Theory?
3 min read • July, 05 2023
Nursing theories provide a foundation for clinical decision-making. These theoretical models in nursing shape nursing research and create conceptual blueprints, ultimately determining the how and why that drive nurse-patient interactions.
Nurse researchers and scholars naturally develop these theories with the input and influence of other professionals in the field.
Why Is Nursing Theory Important?
Nursing theory concepts are essential to the present and future of the profession. The first nursing theory — Florence Nightingale's Environmental Theory — dates back to the 19th century. Nightingale identified a clear link between a patient's environment (such as clean water, sunlight, and fresh air) and their ability to recover. Her discoveries remain relevant for today's practitioners. As health care continues to develop, new types of nursing theories may evolve to reflect new medicines and technologies.
Education and training showcase the importance of nursing theory. Nurse researchers and scholars share established ideas to ensure industry-wide best practices and patient outcomes, and nurse educators shape their curricula based on this research. When nurses learn these theories, they gain the data to explain the reasoning behind their clinical decision-making. Nurses position themselves to provide the best care by familiarizing themselves with time-tested theories. Recognizing their place in the history of nursing provides a validating sense of belonging within the greater health care system. That helps patients and other health care providers better understand and appreciate nurses’ contributions.
Types of Nursing Theories
Nursing theories fall under three tiers: grand nursing, middle-range, and practical-level theories . Inherent to each is the nursing metaparadigm , which focuses on four components:
- The person (sometimes referred to as the patient or client)
- Their environment (physical and emotional)
- Their health while receiving treatment
- The nurse's approach and attributes
Each of these four elements factors into a specific nursing theory.
Grand Nursing Theories
Grand theories are the broadest of the three theory classifications. They offer wide-ranging perspectives focused on abstract concepts, often stemming from a nurse theorist’s lived experiences or nursing philosophies. Grand nursing theories help to guide research in the field, with studies aiming to explore proposed ideas further.
Hildegard Peplau's Theory of Interpersonal Relations is an excellent example of a grand nursing theory. The theory suggests that for a nurse-patient relationship to be successful, it must go through three phases: orientation, working, and termination. This grand theory is broad in scope and widely applicable to different environments.
Middle-Range Nursing Theories
As the name suggests, middle-range theories lie somewhere between the sweeping scope of grand nursing and a minute focus on practice-level theories. These theories are often phenomena-driven, attempting to explain or predict certain trends in clinical practice. They’re also testable or verifiable through research.
Nurse researchers have applied the concept of Dorothea E. Orem's Self-Care Deficit Theory to patients dealing with various conditions, ranging from hepatitis to diabetes. This grand theory suggests that patients recover most effectively if they actively and autonomously perform self-care.
Practice-Level Nursing Theories
Practice-level theories are more specific to a patient’s needs or goals. These theories guide the treatment of health conditions and situations requiring nursing intervention. Because they’re so specific, these types of nursing theories directly impact daily practices more than other theory classifications. From patient education to practicing active compassion, bedside nurses use these theories in their everyday responsibilities.
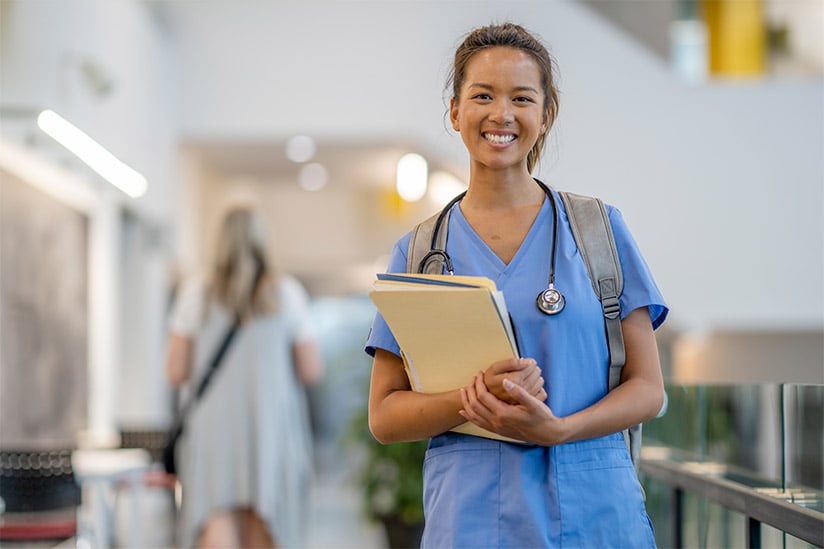
Nursing Theory in Practice
Theory and practice inform each other. Nursing theories determine research that shapes policies and procedures. Nurses constantly apply theories to patient interactions, consciously or due to training. For example, a nurse who aims to provide culturally competent care — through a commitment to ongoing education and open-mindedness — puts Madeleine Leininger's Transcultural Nursing Theory into effect. Because nursing is multifaceted, nurses can draw from multiple theories to ensure the best course of action for a patient.
Applying theory in nursing practice develops nursing knowledge and supports evidence-based practice. A nursing theoretical framework is essential to understand decision-making processes and to promote quality patient care.
Images sourced from Getty Images
Related Resources

Item(s) added to cart
Nursing Theories and Theorists: The Definitive Guide for Nurses
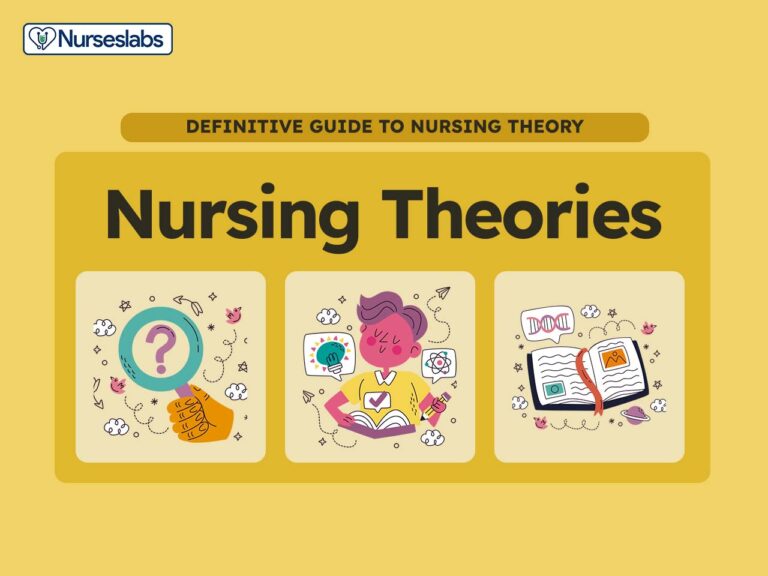
In this guide for nursing theories and nursing theorists , we aim to help you understand what comprises a nursing theory and its importance, purpose, history, types, or classifications, and give you an overview through summaries of selected nursing theories.
Table of Contents
- What are Nursing Theories?
Defining Terms
History of nursing theories, environment, definitions, relational statements, assumptions, why are nursing theories important, in academic discipline, in research, in the profession, grand nursing theories, middle-range nursing theories, practice-level nursing theories, factor-isolating theory, explanatory theory, prescriptive theories, other ways of classifying nursing theories, florence nightingale, hildegard e. peplau, virginia henderson, faye glenn abdellah, ernestine wiedenbach, lydia e. hall, joyce travelbee, kathryn e. barnard, evelyn adam, nancy roper, winifred logan, and alison j. tierney, ida jean orlando, jean watson.
- Marilyn Anne Ray
Patricia Benner
Kari martinsen, katie eriksson, myra estrin levine, martha e. rogers, dorothea e. orem, imogene m. king, betty neuman, sister callista roy, dorothy e. johnson, anne boykin and savina o. schoenhofer, afaf ibrahim meleis, nola j. pender, madeleine m. leininger, margaret a. newman, rosemarie rizzo parse, helen c. erickson, evelyn m. tomlin, and mary ann p. swain, gladys l. husted and james h. husted, ramona t. mercer, merle h. mishel, pamela g. reed, carolyn l. wiener and marylin j. dodd, georgene gaskill eakes, mary lermann burke, and margaret a. hainsworth, phil barker, katharine kolcaba, cheryl tatano beck, kristen m. swanson, cornelia m. ruland and shirley m. moore, wanda de aguiar horta, recommended resources, what are nursing theories.
Nursing theories are organized bodies of knowledge to define what nursing is, what nurses do, and why they do it. Nursing theories provide a way to define nursing as a unique discipline that is separate from other disciplines (e.g., medicine). It is a framework of concepts and purposes intended to guide nursing practice at a more concrete and specific level.
Nursing, as a profession, is committed to recognizing its own unparalleled body of knowledge vital to nursing practice—nursing science. To distinguish this foundation of knowledge, nurses need to identify, develop, and understand concepts and theories in line with nursing. As a science, nursing is based on the theory of what nursing is, what nurses do, and why. Nursing is a unique discipline and is separate from medicine. It has its own body of knowledge on which delivery of care is based.
The development of nursing theory demands an understanding of selected terminologies, definitions, and assumptions.
- Philosophy. These are beliefs and values that define a way of thinking and are generally known and understood by a group or discipline.
- Theory . A belief, policy, or procedure proposed or followed as the basis of action. It refers to a logical group of general propositions used as principles of explanation. Theories are also used to describe, predict, or control phenomena.
- Concept. Concepts are often called the building blocks of theories. They are primarily the vehicles of thought that involve images.
- Models. Models are representations of the interaction among and between the concepts showing patterns. They present an overview of the theory’s thinking and may demonstrate how theory can be introduced into practice.
- Conceptual framework. A conceptual framework is a group of related ideas, statements, or concepts. It is often used interchangeably with the conceptual model and with grand theories .
- Proposition. Propositions are statements that describe the relationship between the concepts.
- Domain . The domain is the perspective or territory of a profession or discipline.
- Process. Processes are organized steps, changes, or functions intended to bring about the desired result.
- Paradigm. A paradigm refers to a pattern of shared understanding and assumptions about reality and the world, worldview, or widely accepted value system.
- Metaparadigm. A metaparadigm is the most general statement of discipline and functions as a framework in which the more restricted structures of conceptual models develop. Much of the theoretical work in nursing focused on articulating relationships among four major concepts: person, environment, health, and nursing.
The first nursing theories appeared in the late 1800s when a strong emphasis was placed on nursing education.
- In 1860, Florence Nightingale defined nursing in her “ Environmental Theory ” as “the act of utilizing the patient’s environment to assist him in his recovery.”
- In the 1950s, there is a consensus among nursing scholars that nursing needed to validate itself through the production of its own scientifically tested body of knowledge.
- In 1952, Hildegard Peplau introduced her Theory of Interpersonal Relations that emphasizes the nurse -client relationship as the foundation of nursing practice.
- In 1955, Virginia Henderson conceptualized the nurse’s role as assisting sick or healthy individuals to gain independence in meeting 14 fundamental needs. Thus her Nursing Need Theory was developed.
- In 1960, Faye Abdellah published her work “Typology of 21 Nursing Problems,” which shifted the focus of nursing from a disease-centered approach to a patient-centered approach.
- In 1962, Ida Jean Orlando emphasized the reciprocal relationship between patient and nurse and viewed nursing’s professional function as finding out and meeting the patient’s immediate need for help.
- In 1968, Dorothy Johnson pioneered the Behavioral System Model and upheld the fostering of efficient and effective behavioral functioning in the patient to prevent illness.
- In 1970, Martha Rogers viewed nursing as both a science and an art as it provides a way to view the unitary human being, who is integral with the universe.
- In 1971, Dorothea Orem stated in her theory that nursing care is required if the client is unable to fulfill biological, psychological, developmental, or social needs.
- In 1971, Imogene King ‘s Theory of Goal attainment stated that the nurse is considered part of the patient’s environment and the nurse-patient relationship is for meeting goals towards good health.
- In 1972, Betty Neuman , in her theory, states that many needs exist, and each may disrupt client balance or stability. Stress reduction is the goal of the system model of nursing practice.
- In 1979, Sr. Callista Roy viewed the individual as a set of interrelated systems that maintain the balance between these various stimuli.
- In 1979, Jean Watson developed the philosophy of caring, highlighted humanistic aspects of nursing as they intertwine with scientific knowledge and nursing practice.
The Nursing Metaparadigm
Four major concepts are frequently interrelated and fundamental to nursing theory: person, environment, health, and nursing. These four are collectively referred to as metaparadigm for nursing .
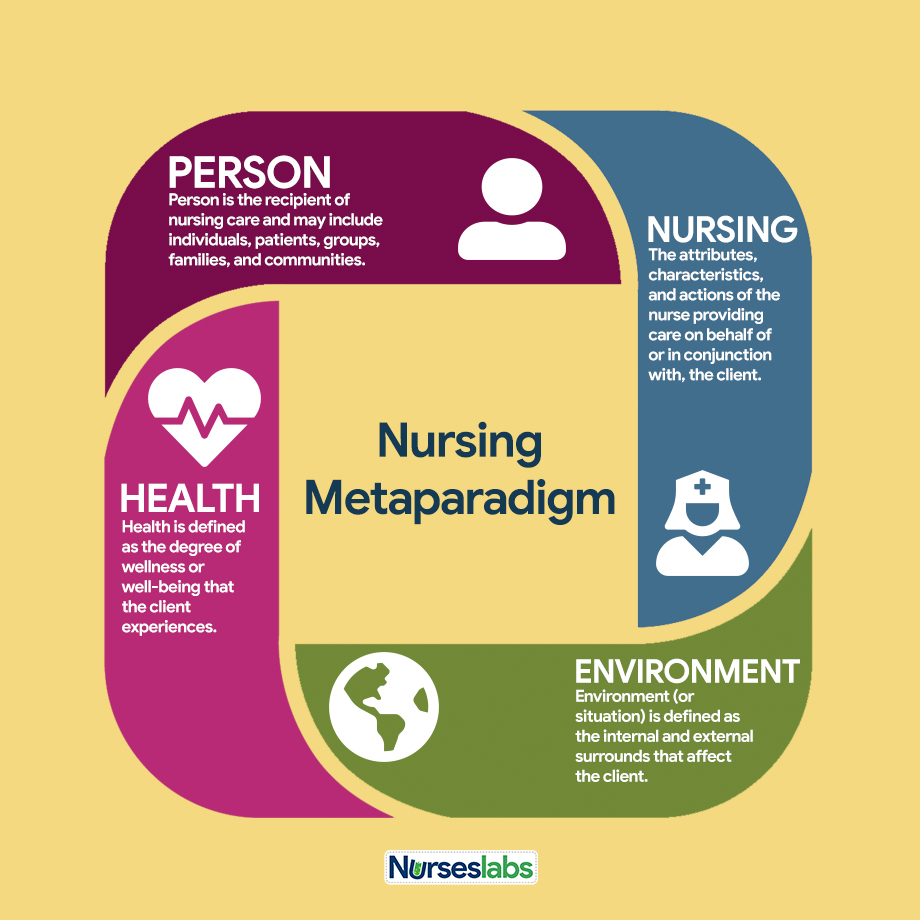
Person (also referred to as Client or Human Beings) is the recipient of nursing care and may include individuals, patients, groups, families, and communities.
Environment (or situation) is defined as the internal and external surroundings that affect the client. It includes all positive or negative conditions that affect the patient, the physical environment, such as families, friends, and significant others, and the setting for where they go for their healthcare.
Health is defined as the degree of wellness or well-being that the client experiences. It may have different meanings for each patient, the clinical setting, and the health care provider.
The nurse’s attributes, characteristics, and actions provide care on behalf of or in conjunction with the client. There are numerous definitions of nursing, though nursing scholars may have difficulty agreeing on its exact definition. The ultimate goal of nursing theories is to improve patient care .
You’ll find that these four concepts are used frequently and defined differently throughout different nursing theories. Each nurse theorist’s definition varies by their orientation, nursing experience , and different factors that affect the theorist’s nursing view. The person is the main focus, but how each theorist defines the nursing metaparadigm gives a unique take specific to a particular theory. To give you an example, below are the different definitions of various theorists on the nursing metaparadigm:
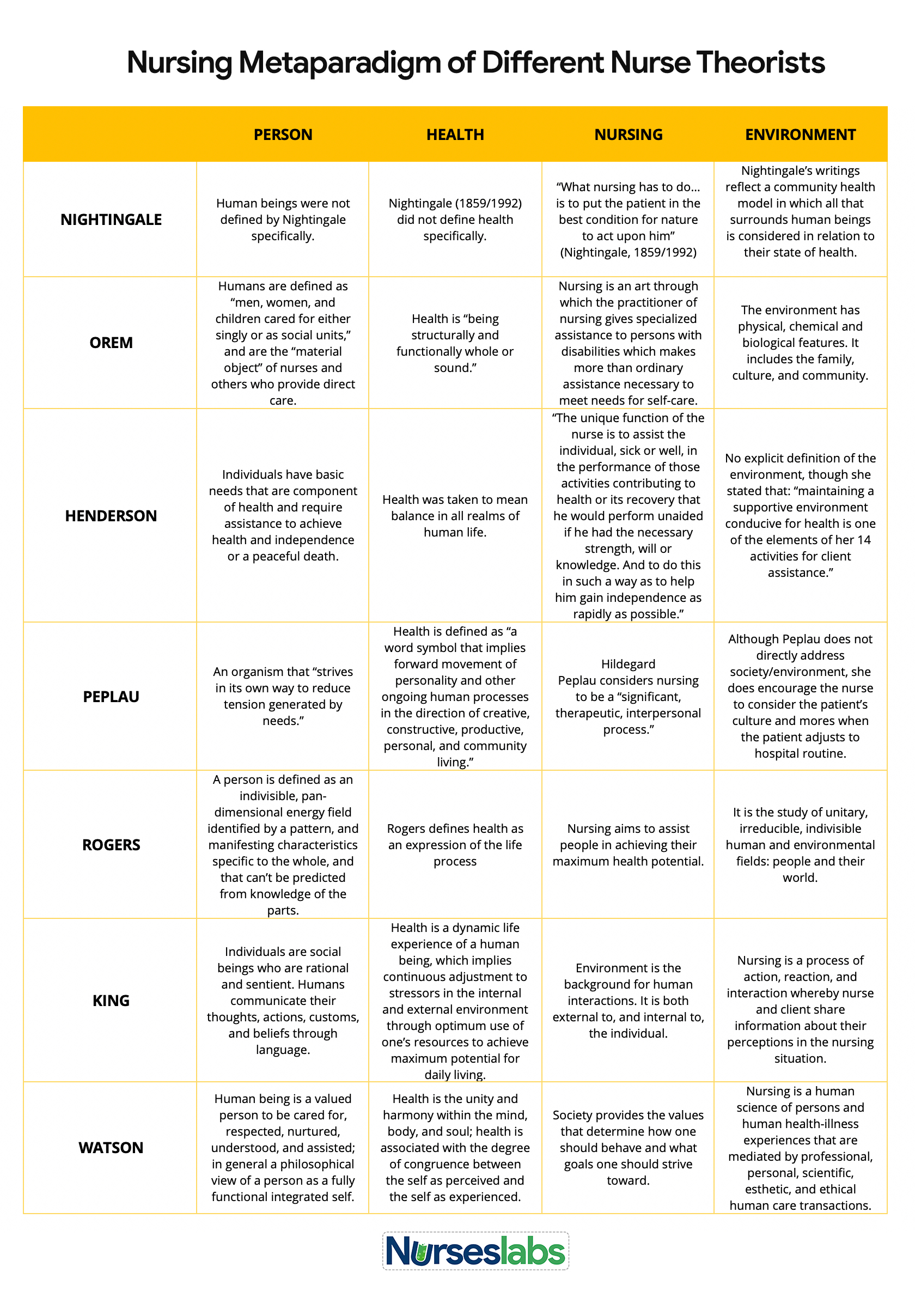
Components of Nursing Theories
For a theory to be a theory, it has to contain concepts, definitions, relational statements, and assumptions that explain a phenomenon. It should also explain how these components relate to each other.
A term given to describe an idea or response about an event, a situation, a process, a group of events, or a group of situations. Phenomena may be temporary or permanent. Nursing theories focus on the phenomena of nursing.
Interrelated concepts define a theory. Concepts are used to help describe or label a phenomenon. They are words or phrases that identify, define, and establish structure and boundaries for ideas generated about a particular phenomenon. Concepts may be abstract or concrete.
- Abstract Concepts . Defined as mentally constructed independently of a specific time or place.
- Concrete Concepts . Are directly experienced and related to a particular time or place.
Definitions are used to convey the general meaning of the concepts of the theory. Definitions can be theoretical or operational.
- Theoretical Definitions . Define a particular concept based on the theorist’s perspective.
- Operational Definitions . States how concepts are measured.
Relational statements define the relationships between two or more concepts. They are the chains that link concepts to one another.
Assumptions are accepted as truths and are based on values and beliefs. These statements explain the nature of concepts, definitions, purpose, relationships, and structure of a theory.
Nursing theories are the basis of nursing practice today. In many cases, nursing theory guides knowledge development and directs education, research, and practice. Historically, nursing was not recognized as an academic discipline or as a profession we view today. Before nursing theories were developed, nursing was considered to be a task-oriented occupation. The training and function of nurses were under the direction and control of the medical profession. Let’s take a look at the importance of nursing theory and its significance to nursing practice:
- Nursing theories help recognize what should set the foundation of practice by explicitly describing nursing.
- By defining nursing, a nursing theory also helps nurses understand their purpose and role in the healthcare setting.
- Theories serve as a rationale or scientific reasons for nursing interventions and give nurses the knowledge base necessary for acting and responding appropriately in nursing care situations.
- Nursing theories provide the foundations of nursing practice, generate further knowledge, and indicate which direction nursing should develop in the future (Brown, 1964).
- By providing nurses a sense of identity, nursing theory can help patients, managers, and other healthcare professionals to acknowledge and understand the unique contribution that nurses make to the healthcare service (Draper, 1990).
- Nursing theories prepare the nurses to reflect on the assumptions and question the nursing values, thus further defining nursing and increasing the knowledge base.
- Nursing theories aim to define, predict, and demonstrate nursing phenomenon (Chinn and Jacobs, 1978).
- It can be regarded as an attempt by the nursing profession to maintain and preserve its professional limits and boundaries.
- Nursing theories can help guide research and informing evidence-based practice.
- Provide a common language and terminology for nurses to use in communication and practice.
- Serves as a basis for the development of nursing education and training programs.
- In many cases, nursing theories guide knowledge development and directs education, research, and practice, although each influences the others. (Fitzpatrick and Whall, 2005).
Purposes of Nursing Theories
The primary purpose of theory in nursing is to improve practice by positively influencing the health and quality of life of patients. Nursing theories are essential for the development and advancement of the nursing profession. Nursing theories are also developed to define and describe nursing care, guide nursing practice, and provide a basis for clinical decision-making . In the past, the accomplishments of nursing led to the recognition of nursing in an academic discipline, research, and profession.
Much of the earlier nursing programs identified the major concepts in one or two nursing models, organized the concepts, and build an entire nursing curriculum around the created framework. These models’ unique language was typically introduced into program objectives, course objectives, course descriptions, and clinical performance criteria. The purpose was to explain the fundamental implications of the profession and enhance the profession’s status.
The development of theory is fundamental to the research process, where it is necessary to use theory as a framework to provide perspective and guidance to the research study. Theory can also be used to guide the research process by creating and testing phenomena of interest. To improve the nursing profession’s ability to meet societal duties and responsibilities, there needs to be a continuous reciprocal and cyclical connection with theory, practice, and research. This will help connect the perceived “gap” between theory and practice and promote the theory-guided practice.
Clinical practice generates research questions and knowledge for theory. In a clinical setting, its primary contribution has been the facilitation of reflecting, questioning, and thinking about what nurses do. Because nurses and nursing practice are often subordinate to powerful institutional forces and traditions, introducing any framework that encourages nurses to reflect on, question, and think about what they do provide an invaluable service.
Classification of Nursing Theories
There are different ways to categorize nursing theories. They are classified depending on their function, levels of abstraction, or goal orientation.
By Abstraction
There are three major categories when classifying nursing theories based on their level of abstraction: grand theory, middle-range theory, and practice-level theory.
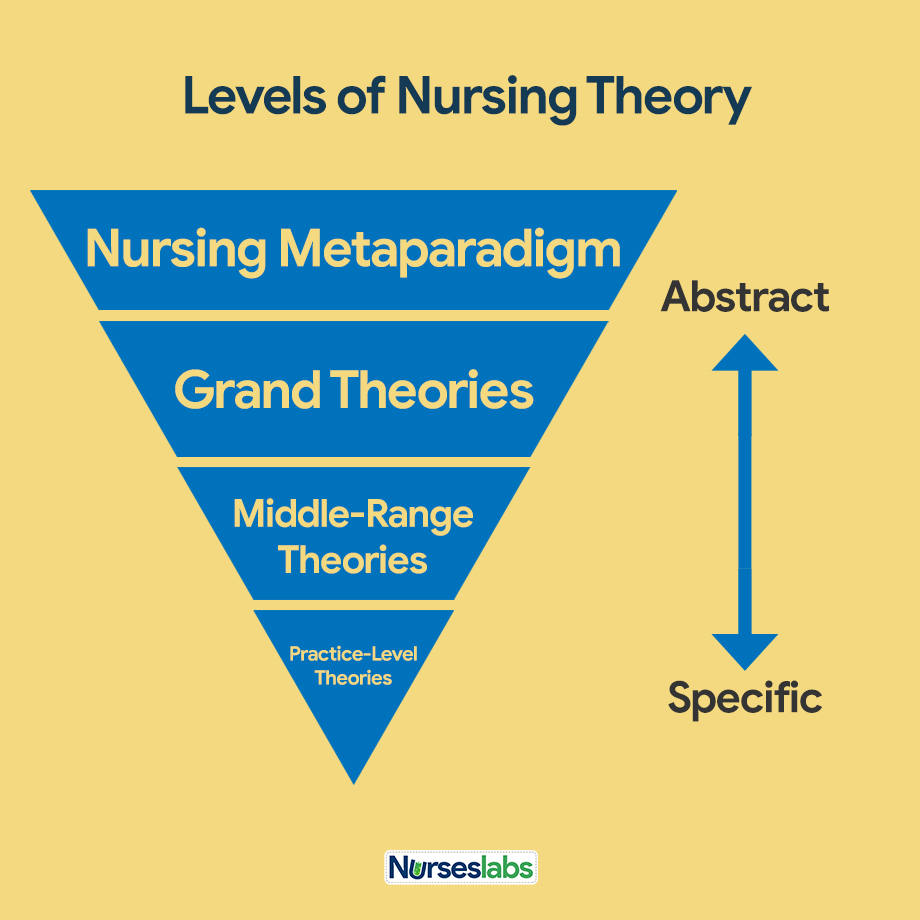
- Grand theories are abstract, broad in scope, and complex, therefore requiring further research for clarification.
- Grand nursing theories do not guide specific nursing interventions but rather provide a general framework and nursing ideas.
- Grand nursing theorists develop their works based on their own experiences and their time, explaining why there is so much variation among theories.
- Address the nursing metaparadigm components of person, nursing, health, and environment.
- More limited in scope (compared to grand theories) and present concepts and propositions at a lower level of abstraction. They address a specific phenomenon in nursing.
- Due to the difficulty of testing grand theories, nursing scholars proposed using this level of theory.
- Most middle-range theories are based on a grand theorist’s works, but they can be conceived from research, nursing practice, or the theories of other disciplines.
- Practice nursing theories are situation-specific theories that are narrow in scope and focuses on a specific patient population at a specific time.
- Practice-level nursing theories provide frameworks for nursing interventions and suggest outcomes or the effect of nursing practice.
- Theories developed at this level have a more direct effect on nursing practice than more abstract theories.
- These theories are interrelated with concepts from middle-range theories or grand theories.
By Goal Orientation
Theories can also be classified based on their goals. They can be descriptive or prescriptive .
Descriptive Theories
- Descriptive theories are the first level of theory development. They describe the phenomena and identify its properties and components in which it occurs.
- Descriptive theories are not action-oriented or attempt to produce or change a situation.
- There are two types of descriptive theories: factor-isolating theory and explanatory theory .
- Also known as category-formulating or labeling theory.
- Theories under this category describe the properties and dimensions of phenomena.
- Explanatory theories describe and explain the nature of relationships of certain phenomena to other phenomena.
- Address the nursing interventions for a phenomenon, guide practice change, and predict consequences.
- Includes propositions that call for change.
- In nursing, prescriptive theories are used to anticipate the outcomes of nursing interventions.
Classification According to Meleis
Afaf Ibrahim Meleis (2011), in her book Theoretical Nursing: Development and Progress , organizes the major nurse theories and models using the following headings: needs theories, interaction theories, and outcome theories. These categories indicate the basic philosophical underpinnings of the theories.
- Needs-Based Theories. The needs theorists were the first group of nurses who thought of giving nursing care a conceptual order. Theories under this group are based on helping individuals to fulfill their physical and mental needs. Theories of Orem, Henderson, and Abdella are categorized under this group. Need theories are criticized for relying too much on the medical model of health and placing the patient in an overtly dependent position.
- Interaction Theories. These theories emphasized nursing on the establishment and maintenance of relationships. They highlighted the impact of nursing on patients and how they interact with the environment, people, and situations. Theories of King, Orlando, and Travelbee are grouped under this category.
- Outcome Theories . These theories describe the nurse as controlling and directing patient care using their knowledge of the human physiological and behavioral systems. The nursing theories of Johnson , Levine , Rogers , and Roy belong to this group.
Classification According to Alligood
In her book, Nursing Theorists and Their Work, Raile Alligood (2017) categorized nursing theories into four headings: nursing philosophy, nursing conceptual models, nursing theories and grand theories, and middle-range nursing theories.
- Nursing Philosophy . It is the most abstract type and sets forth the meaning of nursing phenomena through analysis, reasoning, and logical presentation. Works of Nightingale, Watson, Ray, and Benner are categorized under this group.
- Nursing Conceptual Models . These are comprehensive nursing theories that are regarded by some as pioneers in nursing. These theories address the nursing metaparadigm and explain the relationship between them. Conceptual models of Levine, Rogers, Roy, King, and Orem are under this group.
- Grand Nursing Theories. Are works derived from nursing philosophies, conceptual models, and other grand theories that are generally not as specific as middle-range theories. Works of Levine, Rogers, Orem, and King are some of the theories under this category.
- Middle-Range Theories. Are precise and answer specific nursing practice questions . They address the specifics of nursing situations within the model’s perspective or theory from which they are derived. Examples of Middle-Range theories are that of Mercer, Reed, Mishel, and Barker.
List of Nursing Theories and Theorists
You’ve learned from the previous sections the definition of nursing theory, its significance in nursing, and its purpose in generating a nursing knowledge base. This section will give you an overview and summary of the various published works in nursing theory (in chronological order). Deep dive into learning about the theory by clicking on the links provided for their biography and comprehensive review of their work.
See Also: Florence Nightingale: Environmental Theory and Biography
- Founder of Modern Nursing and Pioneer of the Environmental Theory.
- Defined Nursing as “the act of utilizing the environment of the patient to assist him in his recovery.”
- Stated that nursing “ought to signify the proper use of fresh air, light, warmth, cleanliness, quiet, and the proper selection and administration of diet – all at the least expense of vital power to the patient.”
- Identified five (5) environmental factors: fresh air, pure water, efficient drainage, cleanliness or sanitation, and light or direct sunlight.
See Also: Hildegard Peplau: Interpersonal Relations Theory
- Pioneered the Theory of Interpersonal Relations
- Peplau’s theory defined Nursing as “An interpersonal process of therapeutic interactions between an individual who is sick or in need of health services and a nurse specially educated to recognize, respond to the need for help.”
- Her work is influenced by Henry Stack Sullivan, Percival Symonds, Abraham Maslow , and Neal Elgar Miller.
- It helps nurses and healthcare providers develop more therapeutic interventions in the clinical setting.
See Also: Virginia Henderson: Nursing Need Theory
- Developed the Nursing Need Theory
- Focuses on the importance of increasing the patient’s independence to hasten their progress in the hospital.
- Emphasizes the basic human needs and how nurses can assist in meeting those needs.
- “The nurse is expected to carry out a physician’s therapeutic plan, but individualized care is the result of the nurse’s creativity in planning for care.”
See Also: Faye Glenn Abdellah: 21 Nursing Problems Theory
- Developed the 21 Nursing Problems Theory
- “Nursing is based on an art and science that molds the attitudes, intellectual competencies, and technical skills of the individual nurse into the desire and ability to help people, sick or well, cope with their health needs.”
- Changed the focus of nursing from disease-centered to patient-centered and began to include families and the elderly in nursing care.
- The nursing model is intended to guide care in hospital institutions but can also be applied to community health nursing, as well.
- Developed The Helping Art of Clinical Nursing conceptual model.
- Definition of nursing reflects on nurse-midwife experience as “People may differ in their concept of nursing, but few would disagree that nursing is nurturing or caring for someone in a motherly fashion.”
- Guides the nurse action in the art of nursing and specified four elements of clinical nursing: philosophy, purpose, practice, and art.
- Clinical nursing is focused on meeting the patient’s perceived need for help in a vision of nursing that indicates considerable importance on the art of nursing.
See Also: Lydia Hall: Care, Cure, Core Theory
- Developed the Care, Cure, Core Theory is also known as the “ Three Cs of Lydia Hall . “
- Hall defined Nursing as the “participation in care, core and cure aspects of patient care , where CARE is the sole function of nurses, whereas the CORE and CURE are shared with other members of the health team.”
- The major purpose of care is to achieve an interpersonal relationship with the individual to facilitate the development of the core.
- The “care” circle defines a professional nurse’s primary role, such as providing bodily care for the patient. The “core” is the patient receiving nursing care. The “cure” is the aspect of nursing that involves the administration of medications and treatments.
- States in her Human-to-Human Relationship Model that the purpose of nursing was to help and support an individual, family, or community to prevent or cope with the struggles of illness and suffering and, if necessary, to find significance in these occurrences, with the ultimate goal being the presence of hope.
- Nursing was accomplished through human-to-human relationships.
- Extended the interpersonal relationship theories of Peplau and Orlando.
- Developed the Child Health Assessment Model .
- Concerns improving the health of infants and their families.
- Her findings on parent-child interaction as an important predictor of cognitive development helped shape public policy.
- She is the founder of the Nursing Child Assessment Satellite Training Project (NCAST), which produces and develops research-based products, assessment , and training programs to teach professionals, parents, and other caregivers the skills to provide nurturing environments for young children.
- Borrows from psychology and human development and focuses on mother-infant interaction with the environment.
- Contributed a close link to practice that has modified the way health care providers assess children in light of the parent-child relationship.
- Focuses on the development of models and theories on the concept of nursing.
- Includes the profession’s goal, the beneficiary of the professional service, the role of the professional, the source of the beneficiary’s difficulty, the intervention of the professional, and the consequences.
- A good example of using a unique basis of nursing for further expansion.
- A Model for Nursing Based on a Model of Living
- Logan produced a simple theory, “which actually helped bedside nurses.”
- The trio collaborated in the fourth edition of The Elements of Nursing: A Model for Nursing Based on a Model of Living and prepared a monograph entitled The Roper-Logan-Tierney Model of Nursing: Based on Activities of Daily Living.
- Includes maintaining a safe environment, communicating, breathing, eating and drinking, eliminating, personal cleansing and dressing , controlling body temperature, mobilizing, working and playing, expressing sexuality, sleeping , and dying .
See Also: Ida Jean Orlando: Nursing Process Theory
- She developed the Nursing Process Theory.
- “Patients have their own meanings and interpretations of situations, and therefore nurses must validate their inferences and analyses with patients before drawing conclusions.”
- Allows nurses to formulate an effective nursing care plan that can also be easily adapted when and if any complexity comes up with the patient.
- According to her, persons become patients requiring nursing care when they have needs for help that cannot be met independently because of their physical limitations, negative reactions to an environment, or experience that prevents them from communicating their needs.
- The role of the nurse is to find out and meet the patient’s immediate needs for help.
See Also: Jean Watson: Theory of Human Caring
- She pioneered the Philosophy and Theory of Transpersonal Caring .
- “Nursing is concerned with promoting health, preventing illness, caring for the sick, and restoring health.”
- Mainly concerns with how nurses care for their patients and how that caring progresses into better plans to promote health and wellness, prevent illness and restore health.
- Focuses on health promotion , as well as the treatment of diseases.
- Caring is central to nursing practice and promotes health better than a simple medical cure.
Marilyn Anne Ray
- Developed the Theory of Bureaucratic Caring
- “Improved patient safety , infection control, reduction in medication errors , and overall quality of care in complex bureaucratic health care systems cannot occur without knowledge and understanding of complex organizations, such as the political and economic systems, and spiritual-ethical caring, compassion and right action for all patients and professionals.”
- Challenges participants in nursing to think beyond their usual frame of reference and envision the world holistically while considering the universe as a hologram.
- Presents a different view of how health care organizations and nursing phenomena interrelate as wholes and parts in the system.
- Caring, Clinical Wisdom, and Ethics in Nursing Practice
- “The nurse-patient relationship is not a uniform, professionalized blueprint but rather a kaleidoscope of intimacy and distance in some of the most dramatic, poignant, and mundane moments of life.”
- Attempts to assert and reestablish nurses’ caring practices when nurses are rewarded more for efficiency, technical skills, and measurable outcomes.
- States that caring practices are instilled with knowledge and skill regarding everyday human needs.
- Philosophy of Caring
- “Nursing is founded on caring for life, on neighborly love, […]At the same time, the nurse must be professionally educated.”
- Human beings are created and are beings for whom we may have administrative responsibility.
- Caring, solidarity, and moral practice are unavoidable realities.
- Theory of Carative Caring
- “Caritative nursing means that we take ‘caritas’ into use when caring for the human being in health and suffering […] Caritative caring is a manifestation of the love that ‘just exists’ […] Caring communion, true caring, occurs when the one caring in a spirit of caritas alleviates the suffering of the patient.”
- The ultimate goal of caring is to lighten suffering and serve life and health.
- Inspired many in the Nordic countries and used it as the basis of research, education, and clinical practice.
See Also: Myra Estrin Levine: Conservation Model for Nursing
- According to the Conservation Model , “Nursing is human interaction.”
- Provides a framework within which to teach beginning nursing students.
- Logically congruent, externally and internally consistent, has breadth and depth, and is understood, with few exceptions, by professionals and consumers of health care.
See Also: Martha Rogers: Theory of Unitary Human Beings
- In Roger’s Theory of Human Beings , she defined Nursing as “an art and science that is humanistic and humanitarian.
- The Science of Unitary Human Beings contains two dimensions: the science of nursing, which is the knowledge specific to the field of nursing that comes from scientific research; and the art of nursing, which involves using nursing creatively to help better the lives of the patient.
- A patient can’t be separated from his or her environment when addressing health and treatment.
See Also: Dorothea E. Orem: Self-Care Theory
- In her Self-Care Theory , she defined Nursing as “The act of assisting others in the provision and management of self-care to maintain or improve human functioning at the home level of effectiveness.”
- Focuses on each individual’s ability to perform self-care .
- Composed of three interrelated theories: (1) the theory of self-care , (2) the self-care deficit theory, and (3) the theory of nursing systems, which is further classified into wholly compensatory, partially compensatory, and supportive-educative.
See Also: Imogene M. King: Theory of Goal Attainment
- Conceptual System and Middle-Range Theory of Goal Attainment
- “Nursing is a process of action, reaction and interaction by which nurse and client share information about their perception in a nursing situation” and “a process of human interactions between nurse and client whereby each perceives the other and the situation, and through communication , they set goals, explore means, and agree on means to achieve goals.”
- Focuses on this process to guide and direct nurses in the nurse-patient relationship, going hand-in-hand with their patients to meet good health goals.
- Explains that the nurse and patient go hand-in-hand in communicating information, set goals together, and then take actions to achieve those goals.
See Also: Betty Neuman: Neuman’s Systems Model
- In Neuman’s System Model , she defined nursing as a “unique profession in that is concerned with all of the variables affecting an individual’s response to stress.”
- The focus is on the client as a system (which may be an individual, family, group, or community) and on the client’s responses to stressors.
- The client system includes five variables (physiological, psychological, sociocultural, developmental, and spiritual). It is conceptualized as an inner core (basic energy resources) surrounded by concentric circles that include lines of resistance, a normal defense line, and a flexible line of defense.
See Also: Sister Callista Roy: Adaptation Model of Nursing
- In Adaptation Model , Roy defined nursing as a “health care profession that focuses on human life processes and patterns and emphasizes the promotion of health for individuals, families, groups, and society as a whole.”
- Views the individual as a set of interrelated systems that strives to maintain a balance between various stimuli.
- Inspired the development of many middle-range nursing theories and adaptation instruments.
See Also: Dorothy E. Johnson: Behavioral Systems Model
- The Behavioral System Model defined Nursing as “an external regulatory force that acts to preserve the organization and integrate the patients’ behaviors at an optimum level under those conditions in which the behavior constitutes a threat to the physical or social health or in which illness is found.”
- Advocates to foster efficient and effective behavioral functioning in the patient to prevent illness and stresses the importance of research-based knowledge about the effect of nursing care on patients.
- Describes the person as a behavioral system with seven subsystems: the achievement, attachment-affiliative, aggressive-protective, dependency, ingestive, eliminative, and sexual subsystems.
- The Theory of Nursing as Caring: A Model for Transforming Practice
- Nursing is an “exquisitely interwoven” unity of aspects of the discipline and profession of nursing.
- Nursing’s focus and aim as a discipline of knowledge and a professional service are “nurturing persons living to care and growing in caring.”
- Caring in nursing is “an altruistic, active expression of love, and is the intentional and embodied recognition of value and connectedness.”
- Transitions Theory
- It began with observations of experiences faced as people deal with changes related to health, well-being, and the ability to care for themselves.
- Types of transitions include developmental, health and illness, situational, and organizational.
- Acknowledges the role of nurses as they help people go through health/illness and life transitions.
- Focuses on assisting nurses in facilitating patients’, families’, and communities’ healthy transitions.
See Also: Nola Pender: Health Promotion Model
- Health Promotion Model
- Describes the interaction between the nurse and the consumer while considering the role of the health promotion environment.
- It focuses on three areas: individual characteristics and experiences, behavior-specific cognitions and affect, and behavioral outcomes.
- Describes the multidimensional nature of persons as they interact within their environment to pursue health.
See Also: Madeleine M. Leininger: Transcultural Nursing Theory
- Culture Care Theory of Diversity and Universality
- Defined transcultural nursing as “a substantive area of study and practice focused on comparative cultural care (caring) values, beliefs, and practices of individuals or groups of similar or different cultures to provide culture-specific and universal nursing care practices in promoting health or well-being or to help people to face unfavorable human conditions, illness, or death in culturally meaningful ways.”
- Involves learning and understanding various cultures regarding nursing and health-illness caring practices, beliefs, and values to implement significant and efficient nursing care services to people according to their cultural values and health-illness context.
- It focuses on the fact that various cultures have different and unique caring behaviors and different health and illness values, beliefs, and patterns of behaviors.
- Health as Expanding Consciousness
- “Nursing is the process of recognizing the patient in relation to the environment, and it is the process of the understanding of consciousness.”
- “The theory of health as expanding consciousness was stimulated by concern for those for whom health as the absence of disease or disability is not possible . . . “
- Nursing is regarded as a connection between the nurse and patient, and both grow in the sense of higher levels of consciousness.
- Human Becoming Theory
- “Nursing is a science, and the performing art of nursing is practiced in relationships with persons (individuals, groups, and communities) in their processes of becoming.”
- Explains that a person is more than the sum of the parts, the environment, and the person is inseparable and that nursing is a human science and art that uses an abstract body of knowledge to help people.
- It centered around three themes: meaning, rhythmicity, and transcendence.
- Modeling and Role-Modeling
- “Nursing is the holistic helping of persons with their self-care activities in relation to their health . . . The goal is to achieve a state of perceived optimum health and contentment.”
- Modeling is a process that allows nurses to understand the unique perspective of a client and learn to appreciate its importance.
- Role-modeling occurs when the nurse plans and implements interventions that are unique for the client.
- Created the Symphonological Bioethical Theory
- “Symphonology (from ‘ symphonia ,’ a Greek word meaning agreement) is a system of ethics based on the terms and preconditions of an agreement.”
- Nursing cannot occur without both nurse and patient. “A nurse takes no actions that are not interactions.”
- Founded on the singular concept of human rights, the essential agreement of non-aggression among rational people forms the foundation of all human interaction.
- Maternal Role Attainment—Becoming a Mother
- “Nursing is a dynamic profession with three major foci: health promotion and prevention of illness, providing care for those who need professional assistance to achieve their optimal level of health and functioning, and research to enhance the knowledge base for providing excellent nursing care.”
- “Nurses are the health professionals having the most sustained and intense interaction with women in the maternity cycle.”
- Maternal role attainment is an interactional and developmental process occurring over time. The mother becomes attached to her infant, acquires competence in the caretaking tasks involved in the role, and expresses pleasure and gratification. (Mercer, 1986).
- Provides proper health care interventions for nontraditional mothers for them to favorably adopt a strong maternal identity.
- Uncertainty in Illness Theory
- Presents a comprehensive structure to view the experience of acute and chronic illness and organize nursing interventions to promote optimal adjustment.
- Describes how individuals form meaning from illness-related situations.
- The original theory’s concepts were organized in a linear model around the following three major themes: Antecedents of uncertainty, Process of uncertainty appraisal, and Coping with uncertainty.
- Self-Transcendence Theory
- Self-transcendence refers to the fluctuation of perceived boundaries that extend the person (or self) beyond the immediate and constricted views of self and the world (Reed, 1997).
- Has three basic concepts: vulnerability, self-transcendence, and well-being.
- Gives insight into the developmental nature of humans associated with health circumstances connected to nursing care.
- Theory of Illness Trajectory
- “The uncertainty surrounding a chronic illness like cancer is the uncertainty of life writ large. By listening to those who are tolerating this exaggerated uncertainty, we can learn much about the trajectory of living.”
- Provides a framework for nurses to understand how cancer patients stand uncertainty manifested as a loss of control.
- Provides new knowledge on how patients and families endure uncertainty and work strategically to reduce uncertainty through a dynamic flow of illness events, treatment situations, and varied players involved in care organization.
- Theory of Chronic Sorrow
- “Chronic sorrow is the presence of pervasive grief -related feelings that have been found to occur periodically throughout the lives of individuals with chronic health conditions, their family caregivers and the bereaved.”
- This middle-range theory defines the aspect of chronic sorrow as a normal response to the ongoing disparity created by the loss.
- Barker’s Tidal Model of Mental Health Recovery is widely used in mental health nursing.
- It focuses on nursing’s fundamental care processes, is universally applicable, and is a practical guide for psychiatry and mental health nursing.
- Draws on values about relating to people and help others in their moments of distress. The values of the Tidal Model are revealed in the Ten Commitments: Value the voice, Respect the language, Develop genuine curiosity, Become the apprentice, Use the available toolkit, Craft the step beyond, Give the gift of time, Reveal personal wisdom, Know that change is constant, and Be transparent.
- Theory of Comfort
- “Comfort is an antidote to the stressors inherent in health care situations today, and when comfort is enhanced, patients and families are strengthened for the tasks ahead. Also, nurses feel more satisfied with the care they are giving.”
- Patient comfort exists in three forms: relief, ease, and transcendence. These comforts can occur in four contexts: physical, psychospiritual, environmental, and sociocultural.
- As a patient’s comfort needs change, the nurse’s interventions change, as well.
- Postpartum Depression Theory
- “The birth of a baby is an occasion for joy—or so the saying goes […] But for some women, joy is not an option.”
- Described nursing as a caring profession with caring obligations to persons we care for, students, and each other.
- Provides evidence to understand and prevent postpartum depression .
- Theory of Caring
- “Caring is a nurturing way of relating to a valued other toward whom one feels a personal sense of commitment and responsibility.”
- Defines nursing as informed caring for the well-being of others.
- Offers a structure for improving up-to-date nursing practice, education, and research while bringing the discipline to its traditional values and caring-healing roots.
- Peaceful End-of-Life Theory
- The focus was not on death itself but on providing a peaceful and meaningful living in the time that remained for patients and their significant others.
- The purpose was to reflect the complexity involved in caring for terminally ill patients.
- Also known as Wanda Horta, she introduced the concepts of nursing that are accepted in Brazil.
- Wrote the book Nursing Process which presents relevance to the various fields of Nursing practice for providing a holistic view of the patient.
- Her work was recognized in all the teaching institutions called the Theory of Basic Human Needs . It is based on Maslow’s Theory of Human Motivation, whose primary concept is the hierarchy of Basic Human Needs (BHN).
- Horta’s Theory of Basic Human Needs is considered the highest point of her work, and the summary of all her research concludes sickness as a science and art of assisting a human being in meeting basic human needs, making the patient independent of this assistance through education in recovery, maintenance, and health promotion .
- Classified basic human needs into three main dimensions – psychobiological, psychosocial and psychospiritual – and establishes a relationship between the concepts of human being, environment, and nursing.
- The theory describes nursing as an element of a healthcare team and states that it can function efficiently through a scientific method. Horta referred this method as the nursing process .
- She defined the nursing process as the dynamics of systematic and interrelated actions to assist human beings. It is characterized by six phases: nursing history, nursing diagnosis , assistance plan, care plan or nursing prescription, evolution, and prognosis.
Recommended books and resources to learn more about nursing theory:
Disclosure: Included below are affiliate links from Amazon at no additional cost from you. We may earn a small commission from your purchase. For more information, check out our privacy policy .
- Nursing Theorists and Their Work (10th Edition) by Alligood Nursing Theorists and Their Work, 10th Edition provides a clear, in-depth look at nursing theories of historical and international significance. Each chapter presents a key nursing theory or philosophy, showing how systematic theoretical evidence can enhance decision making, professionalism, and quality of care.
- Knowledge Development in Nursing: Theory and Process (11th Edition) Use the five patterns of knowing to help you develop sound clinical judgment. This edition reflects the latest thinking in nursing knowledge development and adds emphasis to real-world application. The content in this edition aligns with the new 2021 AACN Essentials for Nursing Education.
- Nursing Knowledge and Theory Innovation, Second Edition: Advancing the Science of Practice (2nd Edition) This text for graduate-level nursing students focuses on the science and philosophy of nursing knowledge development. It is distinguished by its focus on practical applications of theory for scholarly, evidence-based approaches. The second edition features important updates and a reorganization of information to better highlight the roles of theory and major philosophical perspectives.
- Nursing Theories and Nursing Practice (5th Edition) The only nursing research and theory book with primary works by the original theorists. Explore the historical and contemporary theories that are the foundation of nursing practice today. The 5th Edition, continues to meet the needs of today’s students with an expanded focus on the middle range theories and practice models.
- Strategies for Theory Construction in Nursing (6th Edition) The clearest, most useful introduction to theory development methods. Reflecting vast changes in nursing practice, it covers advances both in theory development and in strategies for concept, statement, and theory development. It also builds further connections between nursing theory and evidence-based practice.
- Middle Range Theory for Nursing (4th Edition) This nursing book’s ability to break down complex ideas is part of what made this book a three-time recipient of the AJN Book of the Year award. This edition includes five completely new chapters of content essential for nursing books. New exemplars linking middle range theory to advanced nursing practice make it even more useful and expand the content to make it better.
- Nursing Research: Methods and Critical Appraisal for Evidence-Based Practice This book offers balanced coverage of both qualitative and quantitative research methodologies. This edition features new content on trending topics, including the Next-Generation NCLEX® Exam (NGN).
- Nursing Research (11th Edition) AJN award-winning authors Denise Polit and Cheryl Beck detail the latest methodologic innovations in nursing, medicine, and the social sciences. The updated 11th Edition adds two new chapters designed to help students ensure the accuracy and effectiveness of research methods. Extensively revised content throughout strengthens students’ ability to locate and rank clinical evidence.
Recommended site resources related to nursing theory:
- Nursing Theories and Theorists: The Definitive Guide for Nurses MUST READ! In this guide for nursing theories, we aim to help you understand what comprises a nursing theory and its importance, purpose, history, types or classifications, and give you an overview through summaries of selected nursing theories.
Other resources related to nursing theory:
- Betty Neuman: Neuman Systems Model
- Dorothea Orem: Self-Care Deficit Theory
- Dorothy Johnson: Behavioral System Model
- Faye Abdellah: 21 Nursing Problems Theory
- Florence Nightingale: Environmental Theory
- Hildegard Peplau: Interpersonal Relations Theory
- Ida Jean Orlando: Deliberative Nursing Process Theory
- Imogene King: Theory of Goal Attainment
- Jean Watson: Theory of Human Caring
- Lydia Hall: Care, Cure, Core Nursing Theory
- Madeleine Leininger: Transcultural Nursing Theory
- Martha Rogers: Science of Unitary Human Beings
- Myra Estrin Levine: The Conservation Model of Nursing
- Nola Pender: Health Promotion Model
- Sister Callista Roy: Adaptation Model of Nursing
- Virginia Henderson: Nursing Need Theory
Suggested readings and resources for this study guide :
- Alligood, M., & Tomey, A. (2010). Nursing theorists and their work, seventh edition (No ed.). Maryland Heights: Mosby-Elsevier.
- Alligood, M. R. (2017). Nursing Theorists and Their Work-E-Book . Elsevier Health Sciences.
- Barnard, K. E. (1984). Nursing research related to infants and young children. In Annual review of nursing research (pp. 3-25). Springer, Berlin, Heidelberg.
- Brown, H. I. (1979). Perception, theory, and commitment: The new philosophy of science . University of Chicago Press. [ Link ]
- Brown M (1964) Research in the development of nursing theory: the importance of a theoretical framework in nursing research. Nursing Research.
- Camacho, A. C. L. F., & Joaquim, F. L. (2017). Reflections based on Wanda Horta on the basic instruments of nursing. Rev Enferm UFPE [Internet], 11(12), 5432-8.
- Chinn, P. L., & Jacobs, M. K. (1978). A model for theory development in nursing. Advances in Nursing Science , 1 (1), 1-12. [ Link ]
- Colley, S. (2003). Nursing theory: its importance to practice. Nursing Standard (through 2013), 17(46), 33. [ Link ]
- Fawcett, J. (2005). Criteria for evaluation of theory. Nursing science quarterly, 18(2), 131-135. [ Link ]
- Fitzpatrick, J. J., & Whall, A. L. (Eds.). (1996). Conceptual models of nursing: Analysis and application . Connecticut, Norwalk: Appleton & Lange.
- Kaplan, A. (2017). The conduct of inquiry: Methodology for behavioural science . Routledge. [ Link ]
- Meleis, A. I. (2011). Theoretical nursing: Development and progress . Lippincott Williams & Wilkins.
- Neuman, B. M., & Fawcett, J. (2002). The Neuman systems model .
- Nightingale F (1860) Notes on Nursing. New York NY, Appleton.
- Perão, O. F., Zandonadi, G. C., Rodríguez, A. H., Fontes, M. S., Nascimento, E. L. P., & Santos, E. K. A. (2017). Patient safety in an intensive care unit according to Wanda Horta’s theory. Cogitare Enfermagem, 22(3), e45657.
- Peplau H (1988) The art and science of nursing: similarities, differences, and relations. Nursing Science Quarterly
- Rogers M (1970) An Introduction to the Theoretical Basis of Nursing. Philadelphia PA, FA Davis.
51 thoughts on “Nursing Theories and Theorists: The Definitive Guide for Nurses”
Great work indeed
Amazing and simple post I have ever come across about nursing theories.
Thank you for the simplicity
where do i find the reference page in apa format?
The reference listed below the article is in APA format.
i love this. insightful. Comprehensive ,Well researched .
Thank you for these theories they are a life saver and simplified. My school require us to write about 2 nursing theorist from memory for a Comprehensive exam in which if you do not pass it you are required to wait for a year to retake the exam.
Merci beaucoup, puisque je suis très satisfait.
I’m pleased to congratulate you about your work! I really appreciate it! From: Cameroon
An entire’s semester worth of a nursing theory class, expertly and succinctly summarized in one paper. I wish my instructor were as easy to understand. Good work.
I thought this was in a chronological order based on their published works date? Then why Orlando’s theory comes at the later part? Can someone englighten me please because I am making a timeline for our project.
Great job. Very clear and succinct.
I like it. Well explained!
easy to understand and very helpful
thankyou very much.
The article was beneficial to me to understand nursing theories
This is amazing and I love it so enriching!
Thanks for the article may God bless you more Plus More Power and Protection
Thanks so much
Please can someone help me with a nursing theory related to “teamwork” please
Thank you so much !
I loved the text and saw that the nursing theorist Wanda Aguiar Horta, a Brazilian nurse and great theorist regarding basic human needs, was not included.
I suggest reviewing and including it to be more complete.
If you need, I can help with inclusion!
Best Regards
Hi João Carlos, we’d love to hear about her work. Please send us the details via our contact page: https://nurseslabs.com/contact/
Excellent study guide! Detailed, Informative and Valued! Thank you!
hi can someone help me which theorist can relate in Ear, Nose, Throat nursing care.
Wonderful contribution of shared knowledge- now how do we get the word out for nurses that are not able to afford a BSN?
Thanks for the work. It’s very helpful
This has helped me understand theories a bit better, however, there is one that is eluding me. Where does the normative theory fit in?
very educative.I have understood theories more than before.Thanks
hard work. great work in deed
I love reading your material, plain concise and easy
Very informative, more knowledgeable about the theorist
Thank you for your information. This material is great and when I have looked for material for nursing theory. I got is material with complete
A big hand of applause 👏🏿 This is a treasure for nurses of the world. Thank you so much
Hi G. ALex,
Wow, thanks for the awesome feedback! 😊 Super glad you found it to be a treasure. Just curious, was there a particular section that stood out to you or something you’d love to see more of? Always keen to hear what resonates with fellow nurses!
This is really hard work put together in a very easy to understand way.Thank you so much.It came handy
Hi Sigala, Thanks a ton for noticing the effort! 😊 Super happy to hear it came in handy for you. If you ever have suggestions or topics you’d like to see, give me a shout. Cheers to making things understandable!
Absolutely helpful. Thank you.
So glad to hear the nursing theories guide was a hit for you! 😊 If you have any other topics or questions in mind, just give a shout. Always here to help. Keep rocking your studies! Thanks Ishe!
Am happy, to read these theories, very educating. Am going to make use of it when caring for my patients. GREAT NURSES GREAT! I LOVE YOU ALL.
Hi Eboh, I’m thrilled to hear you’re excited about applying these nursing theories in practice! They can really enhance the care we provide. It’s all about putting that knowledge to good use. By the way, which theory resonated with you the most, or which do you see being most applicable in your day-to-day patient care?
How do I relate one of the theories to effective management of intravenous lines? Which theory and how to relate to the above?
Hi wanted to ask you who wrote this page who is the autor because i need to write them on footnotes and i can’t find autor of the page,neither the year it was published. Thank you. Btw this article was really helpful i never understood nursing theories this good.
Hey there Innaya, I’m glad to hear the article on nursing theories was so helpful to you! Here’s how you can cite it in APA format:
Vera, M. (2019, September 11). Nursing Theories and Theorists: The Definitive Guide for Nurses Nurseslabs. https://nurseslabs.com/nursing-theories/
If you need any more help with citations or have other questions, feel free to ask. Happy to assist!
Please is there an app I could download all these from?
Hi Felicia, Thanks for your interest! As of now, we don’t have a dedicated app for downloading our content. However, our website is mobile-friendly, so you can easily access all our resources from your smartphone or tablet browser.
wonderful insights, and very precise and easy to understand, I even got to know and learn about other new theorists of Nursing I didn’t know before.
Thank you so much for this wonderful work.
Its so amazing and very helpful. Please how can I cite any of these theory using Vancouver?
thanls for good informatiom need to explain example
Great!. Useful information to the lecturers, and educators toward delivery info to our young generation nursing.
Leave a Comment Cancel reply
Log in using your username and password
- Search More Search for this keyword Advanced search
- Latest content
- Current issue
- Write for Us
- BMJ Journals
You are here
- Volume 27, Issue 1
- Induction, deduction and abduction
- Article Text
- Article info
- Citation Tools
- Rapid Responses
- Article metrics

- http://orcid.org/0000-0003-4308-4219 David Barrett 1 ,
- http://orcid.org/0000-0003-0157-5319 Ahtisham Younas 2 , 3
- 1 Department of Health Sciences , University of York , York , UK
- 2 Memorial University of Newfoundland , St. John's , Newfoundland , Canada
- 3 Swat College of Nursing , Pakistan
- Correspondence to Professor David Barrett, Department of Health Sciences, University of York, York, UK; david.i.barrett{at}york.ac.uk
https://doi.org/10.1136/ebnurs-2023-103873
Statistics from Altmetric.com
Request permissions.
If you wish to reuse any or all of this article please use the link below which will take you to the Copyright Clearance Center’s RightsLink service. You will be able to get a quick price and instant permission to reuse the content in many different ways.
- Nursing Research
Researchers often refer to the type of ‘reasoning’ that they have used to support their analysis and reach conclusions within their study. For example, Krick and colleagues completed a study that supported the development of an outcome framework for measuring the effectiveness of digital nursing technologies. 1 They reported completing the analysis through combining ‘an inductive and deductive approach’ (p1), but what do these terms mean? How can these methods of reasoning support nursing practice, and guide the development and appraisal of research evidence?
This article will explore inductive and deductive reasoning and their place in nursing research. We will also explore a third approach to reasoning—abductive reasoning—which is arguably less well-known than induction and deduction, but just as prevalent and important in nursing practice and nursing research.
Inductive reasoning
Induction, or inductive reasoning, involves the identification of cues and the collection of data to develop general theories or hypotheses. For this reason, inductive reasoning is often described as being ‘bottom-up’ reasoning. In the paper by Krick and colleagues mentioned previously, the inductive element of their work was taking findings from individual studies in a scoping review and using these to ‘inductively derive’ a first draft of their digital nursing outcomes framework. 1
It is easy to see how this study—and many other qualitative pieces of nursing research—frame themselves as having used inductive reasoning, with data from individuals building piece-by-piece into a general theory or overview of a phenomenon. However, the inductive nature of qualitative research is not universally accepted. Bergdahl and Berterö, discuss the ‘myth’ of inductive reasoning in qualitative research. 4 Though they agree that inductive methods require using direct observations or experiments to develop a general theory, they suggest that this is not what qualitative researchers do. Instead, they argue that the codes and themes developed during qualitative research are not tested scientifically, so do not represent true inductive reasoning. Instead, the generation of theory in qualitative research—they argue—requires a ‘creative leap’ which is well beyond the scope of an inductive approach. 4
Deductive reasoning
In their critique of induction in qualitative research, Bergdahl and Berterö argue that qualitative researchers should be more open to a deductive approach. 4 At a superficial level, deductive reasoning can be viewed as the opposite to induction, with specific conclusions drawn from general theories. So, with inductive reasoning often conceptualised as ‘bottom-up’, deductive reasoning can be viewed as ‘top-down’.
Some researchers have applied deductive reasoning within qualitative research as advocated by Bergdahl and Berterö, often by analysing qualitative data in the context of existing theory. A 2022 study by Andersson and colleagues explored critical care nurses’ experiences of working during the first phase of the COVID-19 pandemic. However, rather than starting their analysis of qualitative interviews with a ‘blank canvas’ (as would be the case in a study using inductive reasoning), the authors applied an existing person-centred practice framework as a lens through which the data could be better understood and interpreted. 5
Often, we see deductive reasoning linked to the hypothesis-testing nature of quantitative nursing research. A typical example is from Chang and colleagues, who completed a randomised controlled trial (RCT) in which the impact of a simulation-based mobile app on student learning was evaluated. 6 The authors developed a set of hypotheses (the ‘general theory’ that forms the foundation of deductive reasoning) related to student learning and satisfaction and then tested whether these were supported through analysis of outcomes in the intervention (mobile app) and control (usual paper-based learning) groups. Through analysis of findings, the authors demonstrated the use of a deductive approach to confirm a theory-based hypothesis. 6
Abductive reasoning
The two approaches discussed so far—induction and deduction—would seem to provide the foundation for much of what nurse researchers seek to do. Induction allows us to build theory from data, and deduction allows us to test theory and hypotheses. However, there is a third approach to reasoning — abduction—which is under-represented in the nursing evidence base, despite its importance to both research and practice. 7
With abduction, we generate new ideas from, and recognise meaning in, the information that is available to us. 8 The key point here is that we ‘generate’ new ideas, rather than test or verify them in the way that we should when using inductive or deductive reasoning. Abductive reasoning therefore plays an important part in both quantitative and qualitative research.
From a quantitative perspective, it is often abductive reasoning that we use to first develop the hypothesis or theory that we can then test deductively. A set of observations about an element of nursing practice may lead us—through abductive reasoning—to develop a hypothesis that best explains what we have seen. We can then apply the hypothetico-deductive approach to test this, as with the RCT by Chang and colleagues described earlier.
In qualitative research, abduction may offer the solution to the ‘myth’ of inductive reasoning proposed by Bergdahl and Berterö. The ‘creative leap’ that they state qualitative researchers must make to turn their data into general theories, 4 aligns well with the moment of discovery often associated with abductive reasoning.
Though abductive reasoning is a critical part of nursing practice and research, there is ongoing discussion about how effective it is when used as the only form of reasoning. Given the importance of producing research that supports evidence-based nursing care, it could be argued that suppositions based on abduction alone require testing by inductive and deductive methods before they can be generalised and implemented with confidence. 7
This will depend to an extent on what we look to do with the insights provided by abductive reasoning: we would not, for example, introduce a new nursing intervention based only on the ‘best fit’ hypothesis of abduction. We would want to robustly test this hypothesis through deductive and inductive methods to ensure that practice was evidence-based. However, where abduction leads us towards understanding the experiences of those in our care, or of nurses themselves, it may be that—even without inductive or deductive substantiation—these insights can offer valuable guidance.
In this paper, we have explored three approaches to reasoning—inductive, deductive and abductive—and how they can be applied in nursing research. The main characteristics of these approaches are summarised in table 1 . The key message for nurse researchers is that each approach has an important part to play in the generation, testing and implementation of new knowledge. In many cases, the research process will require nurses to deploy all three approaches, so an understanding of each is an important part of any nurse’s knowledge base.
- View inline
Summary of inductive, deductive and abductive reasoning
- Seibert K , et al
- Alteren J ,
- Hermstad M ,
- Nerdal L , et al
- Bergdahl E ,
- Andersson M ,
- Chang H-Y ,
- Chang Y-C , et al
- Karlsen B ,
- Hillestad TM ,
Twitter @barrett1972, @@Ahtisham04
Funding The authors have not declared a specific grant for this research from any funding agency in the public, commercial or not-for-profit sectors.
Competing interests None declared.
Provenance and peer review Not commissioned; internally peer reviewed.
Read the full text or download the PDF:
Information
- Author Services
Initiatives
You are accessing a machine-readable page. In order to be human-readable, please install an RSS reader.
All articles published by MDPI are made immediately available worldwide under an open access license. No special permission is required to reuse all or part of the article published by MDPI, including figures and tables. For articles published under an open access Creative Common CC BY license, any part of the article may be reused without permission provided that the original article is clearly cited. For more information, please refer to https://www.mdpi.com/openaccess .
Feature papers represent the most advanced research with significant potential for high impact in the field. A Feature Paper should be a substantial original Article that involves several techniques or approaches, provides an outlook for future research directions and describes possible research applications.
Feature papers are submitted upon individual invitation or recommendation by the scientific editors and must receive positive feedback from the reviewers.
Editor’s Choice articles are based on recommendations by the scientific editors of MDPI journals from around the world. Editors select a small number of articles recently published in the journal that they believe will be particularly interesting to readers, or important in the respective research area. The aim is to provide a snapshot of some of the most exciting work published in the various research areas of the journal.
Original Submission Date Received: .
- Active Journals
- Find a Journal
- Proceedings Series
- For Authors
- For Reviewers
- For Editors
- For Librarians
- For Publishers
- For Societies
- For Conference Organizers
- Open Access Policy
- Institutional Open Access Program
- Special Issues Guidelines
- Editorial Process
- Research and Publication Ethics
- Article Processing Charges
- Testimonials
- Preprints.org
- SciProfiles
- Encyclopedia
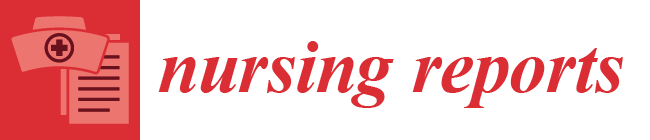
Article Menu

- Subscribe SciFeed
- Author Biographies
- Google Scholar
- on Google Scholar
- Table of Contents
Find support for a specific problem in the support section of our website.
Please let us know what you think of our products and services.
Visit our dedicated information section to learn more about MDPI.
JSmol Viewer
Head nurse leadership: facilitators and barriers to adherence to infection prevention and control programs—a qualitative study protocol †.
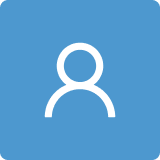
1. Introduction
Theoretical framework, 2. materials and methods, 2.1. objective.
- Describe the thought processes that motivate healthcare professionals in the care provided for reducing HAIs.
- Identify the barriers and facilitators to the implementation of IPC programs in clinical practice.
2.2. Design
2.3. recruitment, 2.4. participants, 2.5. data collection, 2.6. instruments.
- The appropriate use of standard precautions in the management of HAIs caused by devices such as Central Line-Associated Bloodstream Infection (CLABSI) and Catheter-Associated Urinary Tract Infection (CAUTI).
- The appropriate use of standard and additional precautions in the management of device HAIs caused by MDROs.
- The use or non-use of cohorting staff in the case of patients with an HAI due to MDRO.
- The management of in-hospital transport of a patient with an HAI caused by an MDRO.
- The adherence of out-of-ward consultants to the proper use of standard and additional precautions in the case of a patient with HAI due to MDROs.
- The management of environmental sanitation and the relations between healthcare personnel and out-of-hospital cleaners (e.g., patients’ cleaning order from non-infected to infected).
2.7. Data Analysis
2.8. methodical rigor, 2.9. reliability, 2.10. ethical considerations and dissemination, 3. discussion, limitations, 4. conclusions, supplementary materials, author contributions, institutional review board statement, informed consent statement, data availability statement, public involvement statement, guidelines and standards statement, use of artificial intelligence, conflicts of interest.
- König, E.; Medwed, M.; Pux, C.; Uhlmann, M.; Schippinger, W.; Krause, R.; Zollner-Schwetz, I. Prospective surveillance of healthcare-associated infections in residents in four long-term care facilities in Graz, Austria. Antibiotics 2021 , 10 , 544. [ Google Scholar ] [ CrossRef ] [ PubMed ]
- WHO Investing in Education, Jobs and Leadership ; WHO: Geneva, Switzerland, 2020.
- Allegranzi, B.; Nejad, S.B.; Combescure, C.; Graafmans, W.; Attar, H.; Donaldson, L.; Pittet, D. Articles Burden of endemic health-care-associated infection in developing countries: Systematic review and meta-analysis. Lancet 2011 , 377 , 228–269. [ Google Scholar ] [ CrossRef ] [ PubMed ]
- European Centre for Disease Prevention and Control. Healthcare-Associated Infections: Surgical Site Infections 2018–2020 ; European Centre for Disease Prevention and Control: Solna, Sweden, 2023. [ Google Scholar ]
- WHO. Guidelines on Core Components of Infection Prevention and Control Programmes at the National and Acute Health Care Facility Level ; WHO: Geneva, Switzerland, 2016. [ Google Scholar ]
- Warshawsky, N.E. A Complexity-Informed Model to Guide Nurse Manager Practice. Nurs. Adm. Q. 2020 , 44 , 198–204. [ Google Scholar ] [ CrossRef ]
- Zingg, W.; Holmes, A.; Dettenkofer, M.; Goetting, T.; Secci, F.; Clack, L.; Allegranzi, B.; Magiorakos, A.-P.; Pittet, D. Hospital organisation, management, and structure for prevention of health-care-associated infection: A systematic review and expert consensus. Lancet Infect. Dis. 2015 , 15 , 212–224. [ Google Scholar ] [ CrossRef ] [ PubMed ]
- Cummings, G.G.; Lee, S.; Tate, K.; Penconek, T.; Micaroni, S.P.; Paananen, T.; Chatterjee, G.E. The essentials of nursing leadership: A systematic review of factors and educational interventions influencing nursing leadership. Int. J. Nurs. Stud. 2021 , 115 , 103842. [ Google Scholar ] [ CrossRef ] [ PubMed ]
- Mitchell, B.G.; Gardner, A.; Stone, P.W.; Hall, L.; Pogorzelska-Maziarz, M. Hospital Staffing and Health Care–Associated Infections: A Systematic Review of the Literature. Jt. Comm. J. Qual. Patient Saf. 2018 , 44 , 613–622. [ Google Scholar ] [ CrossRef ] [ PubMed ]
- van Buijtene, A.; Foster, D. Does a hospital culture influence adherence to infection prevention and control and rates of healthcare associated infection? A literature review. J. Infect. Prev. 2019 , 20 , 5–17. [ Google Scholar ] [ CrossRef ]
- Ferreira, T.D.M.; Mesquita, G.R.; Melo, G.C.; Oliveira, M.S.; Bucci, A.F.; Porcari, T.A.; Teles, M.G.; Altafini, J.; Dias, F.C.P.; Gasparino, R.C. The influence of nursing leadership styles on the outcomes of patients, professionals and institutions: An integrative review. J. Nurs. Manag. 2022 , 30 , 936–953. [ Google Scholar ] [ CrossRef ] [ PubMed ]
- Saint, S.; Kowalski, C.P.; Banaszak-Holl, J.; Forman, J.; Damschroder, L.; Krein, S.L. The Importance of Leadership in Preventing Healthcare-Associated Infection: Results of a Multisite Qualitative Study. Infect. Control Hosp. Epidemiol. 2010 , 31 , 901–907. [ Google Scholar ] [ CrossRef ] [ PubMed ]
- Cummings, G.G.; Tate, K.; Lee, S.; Wong, C.A.; Paananen, T.; Micaroni, S.P.; Chatterjee, G.E. Leadership styles and outcome patterns for the nursing workforce and work environment: A systematic review. Int. J. Nurs. Stud. 2018 , 85 , 19–60. [ Google Scholar ] [ CrossRef ]
- Cziraki, K.; Wong, C.; Kerr, M.; Finegan, J. Leader empowering behaviour: Relationships with nurse and patient outcomes. Leadersh. Health Serv. 2020 , 33 , 397–415. [ Google Scholar ] [ CrossRef ]
- Labrague, L.J.; Al Sabei, S.D.; AbuAlRub, R.F.; Burney, I.A.; Al Rawajfah, O. Authentic leadership, nurse-assessed adverse patient events and quality of care: The mediating role of nurses’ safety actions. J. Nurs. Manag. 2021 , 29 , 2152–2162. [ Google Scholar ] [ CrossRef ] [ PubMed ]
- Scammell, J.M.E.; Apostolo, J.L.A.; Bianchi, M.; Costa, R.D.P.; Jack, K.; Luiking, M.; Nilsson, S. Learning to lead: A scoping review of undergraduate nurse education. J. Nurs. Manag. 2020 , 28 , 756–765. [ Google Scholar ] [ CrossRef ] [ PubMed ]
- Hegarty, J.; Murphy, S.; Creedon, S.; Wills, T.; Savage, E.; Barry, F.; Smiddy, M.; Coffey, A.; Burton, A.; O’Brien, D.; et al. Leadership perspective on the implementation of guidelines on healthcare-associated infections. BMJ Lead. 2019 , 3 , 43–51. [ Google Scholar ] [ CrossRef ]
- Gobbi, M. Nursing leadership in the European landscape: Influence, reality and politics. J. Res. Nurs. 2014 , 19 , 636–646. [ Google Scholar ] [ CrossRef ]
- Fiorini, J.; Zaghini, F.; Mannocci, A.; Sili, A. Nursing leadership in clinical practice, its efficacy and repercussion on nursing-sensitive outcomes: A cross-sectional multicentre protocol study. J. Nurs. Manag. 2022 , 30 , 3178–3188. [ Google Scholar ] [ CrossRef ] [ PubMed ]
- Zaghini, F.; Vellone, E.; Maurici, M.; Sestili, C.; Mannocci, A.; Ercoli, E.; Magnavita, N.; La Torre, G.; Alvaro, R.; Sili, A. The influence of work context and organizational well-being on psychophysical health of healthcare providers. Med. Lav. 2020 , 111 , 306–320. [ Google Scholar ] [ PubMed ]
- Shankar Balakrishnan, V. Newsdesk WHO’s First Global Infection Prevention and Control Report ; WHO: Geneva, Switzerland, 2022; Volume 22. [ Google Scholar ]
- Storr, J.; the WHO Guidelines Development Group; Twyman, A.; Zingg, W.; Damani, N.; Kilpatrick, C.; Reilly, J.; Price, L.; Egger, M.; Grayson, M.L.; et al. Core components for effective infection prevention and control programmes: New WHO evidence-based recommendations. Antimicrob. Resist. Infect. Control 2017 , 6 , 6. [ Google Scholar ] [ CrossRef ]
- Suetens, C.; Latour, K.; Kärki, T.; Ricchizzi, E.; Kinross, P.; Moro, M.L.; Jans, B.; Hopkins, S.; Hansen, S.; Lyytikäinen, O.; et al. Prevalence of healthcare-associated infections, estimated incidence and composite antimicrobial resistance index in acute care hospitals and long-term care facilities: Results from two European point prevalence surveys, 2016 to 2017. Eurosurveillance 2018 , 23 , 1800516. [ Google Scholar ] [ CrossRef ]
- Asif, M.; Jameel, A.; Hussain, A.; Hwang, J.; Sahito, N. Linking transformational leadership with nurse-assessed adverse patient outcomes and the quality of care: Assessing the role of job satisfaction and structural empowerment. Int. J. Environ. Res. Public Health 2019 , 16 , 2381. [ Google Scholar ] [ CrossRef ]
- Boamah, S.A.; Laschinger, H.K.S.; Wong, C.; Clarke, S. Effect of transformational leadership on job satisfaction and patient safety outcomes. Nurs. Outlook 2018 , 66 , 180–189. [ Google Scholar ] [ CrossRef ] [ PubMed ]
- Adams, J.M. Influence of Nurse Leader Practice Characteristics on Patient Outcomes: Results from a Multi-State Study. Nurs. Econ. 2018 , 36 , 259–267. [ Google Scholar ]
- Fischer, J.P.; Nichols, C. Leadership practices and patient outcomes in Magnet ® vs. non-Magnet hospitals. Nurs. Manag. 2019 , 50 , 26–31. [ Google Scholar ] [ CrossRef ] [ PubMed ]
- Dekker, M.; Jongerden, I.; de Bruijne, M.; Jelsma, J.; Vandenbroucke-Grauls, C.; van Mansfeld, R. Strategies to improve the implementation of infection control link nurse programmes in acute-care hospitals. J. Hosp. Infect. 2022 , 128 , 54–63. [ Google Scholar ] [ CrossRef ] [ PubMed ]
- Wong, C.A.; Giallonardo, L.M. Authentic leadership and nurse-assessed adverse patient outcomes. J. Nurs. Manag. 2013 , 21 , 740–752. [ Google Scholar ] [ CrossRef ] [ PubMed ]
- Landerfelt, P.E.; Lewis, A.; Li, Y.; Cimiotti, J.P. Nursing leadership and the reduction of catheter-associated urinary tract infection. Am. J. Infect. Control 2020 , 48 , 1546–1548. [ Google Scholar ] [ CrossRef ] [ PubMed ]
- Charmaz, K. Constructing Grounded Theory: A Practical Guide through Qualitative Analysis ; Sage Publications: London, UK, 2006. [ Google Scholar ]
- Strauss, A.L.; Corbin, J.M. Basic of Qualitative Research: Techniques and Procedures for Developing Grounded Theory , 3rd ed.; Sage Publications: Thousand Oaks, CA, USA, 1998. [ Google Scholar ]
- Tong, A.; Sainsbury, P.; Craig, J. Consolidated criteria for reporting qualitative research (COREQ): A 32-item checklist for interviews and focus groups. Int. J. Qual. Health Care 2007 , 19 , 349–357. [ Google Scholar ] [ CrossRef ] [ PubMed ]
- World Health Organization. Infection Prevention and Control Assessment Framework at the Facility Level (IPCAF) ; WHO: Geneva, Switzerland, 2018. [ Google Scholar ]
- Polit, D.F.; Beck, C.T. Generalization in quantitative and qualitative research: Myths and strategies. Int. J. Nurs. Stud. 2010 , 47 , 1451–1458. [ Google Scholar ] [ CrossRef ] [ PubMed ]
- Bruchez, S.A.; Duarte, G.C.; Sadowski, R.A.; Custódio da Silva Filho, A.; Fahning, W.E.; Belini Nishiyama, S.A.; Bronharo Tognim, M.C.; Cardoso, C.L. Assessing the Hawthorne effect on hand hygiene compliance in an intensive care unit. Infect. Prev. Pract. 2020 , 2 , 100049. [ Google Scholar ] [ CrossRef ]
- World Health Organization. Global Report on Infection Prevention and Control ; WHO: Geneva, Switzerland, 2022. [ Google Scholar ]
- Mortari, L. Cercare il rigore metodologico per una ricerca pedagogica scientificamente fondata. Educ. Sci. Soc. 2010 , 1 , 143–156. [ Google Scholar ]
- Sandelowski, M.; Barroso, J. Reading Qualitative Studies. Int. J. Qual. Methods 2002 , 1 , 74–108. [ Google Scholar ] [ CrossRef ]
- Mohajan, H.; Mohajan, D.; Kumar Mohajan, H. Munich Personal RePEc Archive Constructivist Grounded Theory: A New Research Approach in Social Science. Res. Adv. Educ. 2022 , 1 , 8–16. [ Google Scholar ] [ CrossRef ]
- Olmos-Vega, F.M.; Stalmeijer, R.E.; Varpio, L.; Kahlke, R. A practical guide to reflexivity in qualitative research: AMEE Guide No. 149. Med. Teach. 2022 , 45 , 241–251. [ Google Scholar ] [ CrossRef ] [ PubMed ]
- World Medical Association. World Medical Association Declaration of Helsinki: Ethical principles for medical research involving human subjects. JAMA 2013 , 310 , 2191–2194. [ Google Scholar ] [ CrossRef ] [ PubMed ]
- Charon, M.J. Symbolic Interactionism ; Prentice Hall: San Francisco, CA, USA, 2010. [ Google Scholar ]
- Kouzes, J.; Posner, B. The Leadership Challenge: How to Make Extraordinary Things Happen in Organizations , 6th ed.; Jossey-Bass: San Francisco, CA, USA, 2017. [ Google Scholar ]
Click here to enlarge figure
Objectives | Main Questions | Supplementary Questions/Points of Attention |
---|---|---|
Background knowledge of the head nurse/nurse | Can you tell me about your education and work experience as a nurse and/or as a head nurse in the ward? | |
Knowledge of the subject that is being studied | What do you think of when I say healthcare-associated infections/community infections/MDRO? | |
Knowledge of the most important aspects of the prevention and control of HAI/HAI due to MDRO/community-acquired infections in clinical practice | Can you tell me about an event that occurred in your ward in the last month with a patient who was diagnosed with HAI/HAI from MDRO/community infection? | What did you think about this situation? What did you do at the time? How did you arrive at this solution? What were the alternatives to this solution? Can you describe to me the reaction of the patient/caregiver/relative to the diagnosis of HAI/HAI from MDRO/community infection? Do you think this could have been avoided? |
Head Nurse’s Knowledge of Leadership Strategies for Managing and Controlling HAIs/HAs from MDROs/Communicable Infections | Can you tell me what strategies you use to increase your team’s adherence to hand hygiene/isolation of the patient with an HAI from an MDRO? Can you describe the key precautions and behaviors you use to manage a patient with HAI caused by MDRO? | Were these decisions shared with your staff or the staff of the IPC? Were they standard procedures or custom procedures? What was the role played by these professionals? Did you consider other hypotheses/interventions? Was this the first time you faced this situation? |
Understand the thought processes that accompany and motivate contextualized decisions in your care setting | From your point of view, what are the strategies for communication and management of the problem of infection within your team? | (Contextualize the question about the organizational context and the patient’s clinical care needs.) How intense are these sharing and confrontation moments? Do you have an agreement with the physician or IPC nurses about the management of these issues? How do your staff approach these strategies? |
Knowledge of barriers to IPC programs in clinical practice | From your perspective, what barriers and facilitators in your hospital might influence the implementation of IPC programs? | From your point of view, what could be changed in the implementation of IPC programs in your hospital? How do you think the relationship with specialist nurses of infectious risk could be defined? Give me an example of a clinical care process that most exposed patients to HAIs or MDRO HAIs. What strategies do you think your hospital uses to ensure safe and quality care for patients and staff? |
Learning about health professionals’ experiences, expectations, and motivations | What was it like for you to participate in this interview? Do you have any questions? |
The statements, opinions and data contained in all publications are solely those of the individual author(s) and contributor(s) and not of MDPI and/or the editor(s). MDPI and/or the editor(s) disclaim responsibility for any injury to people or property resulting from any ideas, methods, instructions or products referred to in the content. |
Share and Cite
Cappelli, E.; Fiorini, J.; Zaghini, F.; Canzan, F.; Sili, A. Head Nurse Leadership: Facilitators and Barriers to Adherence to Infection Prevention and Control Programs—A Qualitative Study Protocol. Nurs. Rep. 2024 , 14 , 1849-1858. https://doi.org/10.3390/nursrep14030138
Cappelli E, Fiorini J, Zaghini F, Canzan F, Sili A. Head Nurse Leadership: Facilitators and Barriers to Adherence to Infection Prevention and Control Programs—A Qualitative Study Protocol. Nursing Reports . 2024; 14(3):1849-1858. https://doi.org/10.3390/nursrep14030138
Cappelli, Eva, Jacopo Fiorini, Francesco Zaghini, Federica Canzan, and Alessandro Sili. 2024. "Head Nurse Leadership: Facilitators and Barriers to Adherence to Infection Prevention and Control Programs—A Qualitative Study Protocol" Nursing Reports 14, no. 3: 1849-1858. https://doi.org/10.3390/nursrep14030138
Article Metrics
Article access statistics, supplementary material.
ZIP-Document (ZIP, 78 KiB)
Further Information
Mdpi initiatives, follow mdpi.
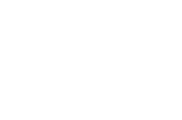
Subscribe to receive issue release notifications and newsletters from MDPI journals

An official website of the United States government
The .gov means it’s official. Federal government websites often end in .gov or .mil. Before sharing sensitive information, make sure you’re on a federal government site.
The site is secure. The https:// ensures that you are connecting to the official website and that any information you provide is encrypted and transmitted securely.
- Publications
- Account settings
- My Bibliography
- Collections
- Citation manager
Save citation to file
Email citation, add to collections.
- Create a new collection
- Add to an existing collection
Add to My Bibliography
Your saved search, create a file for external citation management software, your rss feed.
- Search in PubMed
- Search in NLM Catalog
- Add to Search
Who uses nursing theory? A univariate descriptive analysis of five years' research articles
Affiliation.
- 1 Nursing PhD Program, University of Jordan, Amman, Jordan. [email protected]
- PMID: 20950408
- DOI: 10.1111/j.1471-6712.2010.00835.x
Background: Since the early 1950s, nursing leaders have worked diligently to build the Scientific Discipline of Nursing, integrating Theory, Research and Practice. Recently, the role of theory has again come into question, with some scientists claiming nurses are not using theory to guide their research, with which to improve practice.
Aims: The purposes of this descriptive study were to determine: (i) Were nursing scientists' research articles in leading nursing journals based on theory? (ii) If so, were the theories nursing theories or borrowed theories? (iii) Were the theories integrated into the studies, or were they used as organizing frameworks?
Methods: Research articles from seven top ISI journals were analysed, excluding regularly featured columns, meta-analyses, secondary analysis, case studies and literature reviews. The authors used King's dynamic Interacting system and Goal Attainment Theory as an organizing framework. They developed consensus on how to identify the integration of theory, searching the Title, Abstract, Aims, Methods, Discussion and Conclusion sections of each research article, whether quantitative or qualitative.
Results: Of 2857 articles published in the seven journals from 2002 to, and including, 2006, 2184 (76%) were research articles. Of the 837 (38%) authors who used theories, 460 (55%) used nursing theories, 377 (45%) used other theories: 776 (93%) of those who used theory integrated it into their studies, including qualitative studies, while 51 (7%) reported they used theory as an organizing framework for their studies. Closer analysis revealed theory principles were implicitly implied, even in research reports that did not explicitly report theory usage.
Conclusions: Increasing numbers of nursing research articles (though not percentagewise) continue to be guided by theory, and not always by nursing theory. Newer nursing research methods may not explicitly state the use of nursing theory, though it is implicitly implied.
© 2010 The Authors. Scandinavian Journal of Caring Sciences © 2010 Nordic College of Caring Science.
PubMed Disclaimer
Similar articles
- Critical theory: critical methodology to disciplinary foundations in nursing. Mill JE, Allen MN, Morrow RA. Mill JE, et al. Can J Nurs Res. 2001 Sep;33(2):109-27. Can J Nurs Res. 2001. PMID: 11928332 Review.
- [Cooperation between practice and academia: the self-concept of nursing and scientific experts]. Panfil EM. Panfil EM. Pflege Z. 2007 Jun;60(6):324-7. Pflege Z. 2007. PMID: 17608044 German.
- Knowledge for the good of the individual and society: linking philosophy, disciplinary goals, theory, and practice. McCurry MK, Revell SM, Roy SC. McCurry MK, et al. Nurs Philos. 2010 Jan;11(1):42-52. doi: 10.1111/j.1466-769X.2009.00423.x. Nurs Philos. 2010. PMID: 20017882
- Nursing theory and practice: connecting the dots. Marrs JA, Lowry LW. Marrs JA, et al. Nurs Sci Q. 2006 Jan;19(1):44-50. doi: 10.1177/0894318405283547. Nurs Sci Q. 2006. PMID: 16407599 Review.
- Bourdieu's theory of practice and its potential in nursing research. Rhynas SJ. Rhynas SJ. J Adv Nurs. 2005 Apr;50(2):179-86. doi: 10.1111/j.1365-2648.2005.03377.x. J Adv Nurs. 2005. PMID: 15788082 Review.
- Development of Nursing Research in Saudi Arabia: Implications for Policies and Practice. Aboshaiqah A, Alammar K, Alenezi A, Majrashi B, Alshamlani Y, Alshehari A, Alanazi NH. Aboshaiqah A, et al. Nurs Rep. 2023 Sep 9;13(3):1216-1224. doi: 10.3390/nursrep13030104. Nurs Rep. 2023. PMID: 37755347 Free PMC article. Review.
- Climate Change, Environmental Health, and Challenges for Nursing Discipline. Portela Dos Santos O, Melly P, Joost S, Verloo H. Portela Dos Santos O, et al. Int J Environ Res Public Health. 2023 Apr 28;20(9):5682. doi: 10.3390/ijerph20095682. Int J Environ Res Public Health. 2023. PMID: 37174199 Free PMC article.
- Multi-professional ethical competence in healthcare - an ethical practice model. Koskinen C, Kaldestad K, Rossavik BD, Ree Jensen A, Bjerga G. Koskinen C, et al. Nurs Ethics. 2022 Jun;29(4):1003-1013. doi: 10.1177/09697330211062986. Epub 2022 Feb 25. Nurs Ethics. 2022. PMID: 35212250 Free PMC article.
- Theory-Based Advanced Nursing Practice: A Practice Update on the Application of Orem's Self-Care Deficit Nursing Theory. Yip JYC. Yip JYC. SAGE Open Nurs. 2021 Apr 20;7:23779608211011993. doi: 10.1177/23779608211011993. eCollection 2021 Jan-Dec. SAGE Open Nurs. 2021. PMID: 33959682 Free PMC article.
- Nursing process from theory to practice: Evidence from the implementation of "Coming back to existence caring model" in burn wards. Lotfi M, Zamanzadeh V, Khodayari-Zarnaq R, Mobasseri K. Lotfi M, et al. Nurs Open. 2021 Sep;8(5):2794-2800. doi: 10.1002/nop2.856. Epub 2021 Mar 24. Nurs Open. 2021. PMID: 33764005 Free PMC article.
- Search in MeSH
LinkOut - more resources
Full text sources.
- Ovid Technologies, Inc.
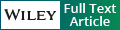
- Citation Manager
NCBI Literature Resources
MeSH PMC Bookshelf Disclaimer
The PubMed wordmark and PubMed logo are registered trademarks of the U.S. Department of Health and Human Services (HHS). Unauthorized use of these marks is strictly prohibited.
- Systematic Review
- Open access
- Published: 16 July 2024
Public health education using social learning theory: a systematic scoping review
- Ting Liu 1 ,
- Patrick Cheong-Iao Pang 1 &
- Chi-Kin Lam 1
BMC Public Health volume 24 , Article number: 1906 ( 2024 ) Cite this article
401 Accesses
Metrics details
Public health education (PHE) in social environments plays a crucial role in mitigating the impact of public health events, especially with the recent surge in global incidents. Social learning theory (SLT) provides a strong theoretical foundation for implementing PHE. The objective of this study is to conduct a systematic scoping review of PHE using SLT, synthesizing the target populations, types of research, main findings, and future directions.
The study followed the Preferred Reporting Items for Systematic Reviews and Meta-Analysis Extension for Scoping Review (PRISMA-ScR) guidelines. We conducted a comprehensive search of five electronic databases (Web of Science, Scopus, PubMed, ProQuest, and APA PsycInfo) for English articles related to PHE using SLT. Two reviewers independently screened the titles and abstracts. Descriptive statistics were utilized to analyze the characteristics of the articles included in the study, followed by a comprehensive narrative analysis of the results.
Research on PHE using SLT mainly focuses on adolescents, students, special patients, and vulnerable populations. The study sample includes seven research types and nine commonly used experimental methods. Four modes of PHE using SLT are identified, along with four types of summarized research results.
PHE research based on SLT can be prioritized for preventing widespread infectious diseases, spreading fundamental public health information, and assisting patients with particular illnesses. To enhance the implementation of PHE, researchers and policymakers should integrate online and offline health education resources, ensure the accessibility of up-to-date information, and leverage digital technologies in PHE. More highly interactive and participatory health education courses will be established in social learning environments to encourage public participation in PHE.
Peer Review reports
Public health as the science and technology of improving the environment, preventing disease, prolonging life expectancy, promoting physical and mental health, and fostering the development of individual potential through organized social activities [ 1 ]. Therefore, the social aspect of public health has to be considered in practice. Public health is also based on the principles of social justice and equity which aims to address the underlying factors that affect public health, including policies and practices [ 2 ]. PHE is the outcome of the simultaneous growth of medical education and the advancement of modern medical careers, especially in the field of medical education. PHE is classified into four categories in the most recent academic research: promoting physical and mental health, developing healthy behaviors, enhancing environmental health, and preventing and controlling diseases [ 3 ]. In recent years, the emergence of global public health crises has had a significant negative impact on governments and the public. In light of the recent global COVID-19 pandemic, it has become clear that the general public lacks public health knowledge, which highlights the importance of strengthening PHE. In order to mitigate the significant impact and consequences of different public health events on the general public and society, it is crucial for governments to prioritize PHE. At the social level, the general public is the target audience for PHE. Through PHE, the government and relevant organizations can effectively raise public awareness of public health and enhance the public's ability to respond effectively to various public health hazards. Therefore, it is necessary to conduct PHE within the social environment.
SLT, which developed from behaviorism, is a social psychological theory that examines human learning in social environments. It was proposed by American psychologist Albert Bandura in 1952. It primarily focuses on the interaction between the learning environment, cognition, and behavior in influencing people's learning behavior, emphasizing the significance of the learning environment and individual behavioral factors [ 4 ]. The theory suggests that individuals can achieve effective learning outcomes by creating a conducive learning environment, engaging in continuous observation, and self-directed learning [ 5 ]. In recent years, most of the research related to SLT has focused on the fields of behavioristics, criminology, and psychology, with limited application in the field of public health. However, there are very few studies based on SLT, which may hinder the effective implementation of public health services. While a small number of public health studies utilize SLT, they primarily focus on higher education and professional talent development in public health, with limited emphasis on public and social PHE studies. SLT’s theoretical support for PHE is necessary for two reasons. Firstly, the public learning environment of PHE is situated in the social public space. Although the implementation of PHE in the public social space is more flexible and covers a wider range of education, the learning environment of a traditional classroom is more stable than that of PHE. Therefore, SLT is necessary to help address the challenges that PHE may encounter during the implementation of social public space. Secondly, traditional PHE is conducted under the supervision of schools and teachers, while public-facing PHE lacks supervision and relies more on individual learning behavior and self-learning efficiency. SLT can enhance learning efficiency by boosting learning motivation and personal learning behavior, thereby promoting the implementation effectiveness of PHE.
PHE is facing numerous challenges worldwide, with the most prominent ones being the dissemination of inaccurate health information [ 6 ], the rapidly evolving global health challenges, and the unequal distribution of health education resources. Among them, the gap and inequality in health education resources are worsening [ 7 ], particularly concerning the quantity and quality of educational resources [ 8 ]. Set against such a background, SLT provides the theoretical support to address these current challenges facing PHE. Our work aims to understand individual learning behavior in social learning environments for learning public health knowledge, which can help to overcome the current challenges in PHE.
Given the importance of using SLT in PHE, this study integrates the SLT perspective and seeks to investigate the impact of SLT-based learning environments and individual behavioral factors on PHE within social contexts. Additionally, this study aims to examine the distinguishing features of PHE in both online and offline settings.
Research question
To accomplish the research objective, the following research questions have been formulated:
What are the target groups and research topics in this field of research?
What types of research and data analysis methods were utilized in these studies?
Which PHE models using SLT were used in these studies?
What are their findings and results?
What are the future trends and suggestions
Search strategy
The reports in this systematic scoping review adhere to the Preferred Reporting Items for Systematic Reviews and Meta-Analyses Extension for Scoping Review (PRISMA-ScR) guidelines [ 9 ]. This review also adheres to Arksey and O'Malley's five-stage framework [ 10 ]. A three-phase search strategy appropriate for scoping studies is utilized. The initial phase involved a restricted search on PubMed and ProQuest. The search terms are repeatedly generated from the research subjects and related theoretical concepts. In the second phase, from our inception until October 16, 2023, we conducted searches using specific terms in the following five databases: Web of Science (WOS), Scopus, PubMed, ProQuest, and APA PsycInfo. The following strings are used to conduct a Boolean search across the four databases. WOS, PubMed, ProQuest and APA PsycInfo with the Booleans “AND”: All fields “public health education” AND “social learning theory”. Scopus with the Booleans “AND”: Title, Abstract, Keywords “public health education” AND “social learning theory”. Limited to articles published between January 2014 and September 2023. The third phase also involves manually searching the full text of all selected articles, as well as references from other similar review studies.
Data selection and extraction
All retrieved records are exported to Zotero software, and any duplicate entries are removed. Two independent reviewers (TL and PP) initially screen the title and abstract of the article based on the inclusion criteria. Differences between two reviewers shall be resolved through negotiation with the third reviewer (CL). All standards are based on SLT, creating a social learning environment, and public-oriented health education. (1) This study is guided by SLT. (2) It focuses on educational behavior within the social environment. (3) The research is intended for the general public rather than professionals or students. Only articles, reviews, conference papers, early access, and proceeding papers are included. Detailed criteria for inclusion and exclusion are included in Table 1 .
Data charting
We developed a data extraction form following the methodology guidance for scoping review provided by the Joanna Briggs Institute and made additional adjustments to the form after piloting five articles [ 11 ]. The items on the form included author, year, country, target groups, research topic, research and data analysis methods, model (a model for implementing PHE), findings, and outcomes. The data were graphed by two independent reviewers, and any sections that resulted in disagreement were resolved by consulting with a senior reviewer.
Collating, summarizing and reporting the results
The data were collected, summarized, and analyzed, and descriptive statistics were used to characterize the sample articles. The findings were presented descriptively, accompanied by graphs and charts. The results were interpreted through a narrative synthesis to address the research questions targeted by the review. All the authors collectively confirmed the results.
As shown in Fig. 1 , a total of 4949 articles were retrieved through the systematic search. After using the Zotero software to exclude duplicate articles, 2584 articles remained. After two reviewers independently screened the article titles and abstracts, 2493 articles that were not directly related to the research topic were excluded. Additionally, 11 articles that were not in English were also excluded. The remaining 80 articles were independently assessed in full by two reviewers, and 43 articles were excluded. Finally, 37 articles were identified for inclusion in the scoping review for this systematic review (see Table 2 [ 12 , 13 , 14 , 15 , 16 , 17 , 18 , 19 , 20 , 21 , 22 , 23 , 24 , 25 , 26 , 27 , 28 , 29 , 30 , 31 , 32 , 33 , 34 , 35 , 36 , 37 , 38 , 39 , 40 , 41 , 42 , 43 , 44 , 45 , 46 , 47 , 48 ]).
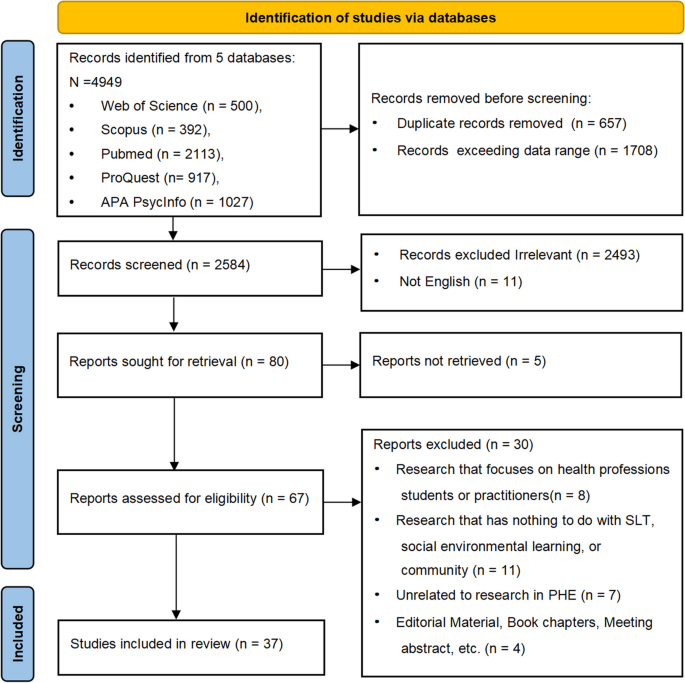
PRISMA flow diagram of selection process
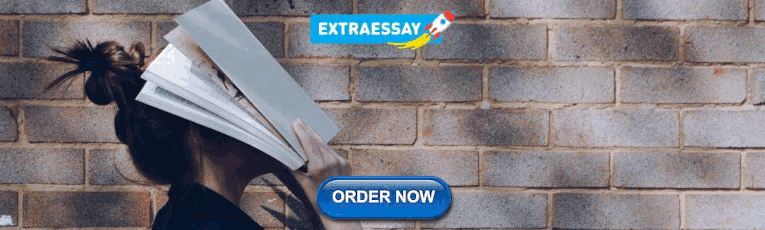
Characteristics of studies
Countries of publications.
Of the 37 studies, the United States, the United Kingdom, Australia, China, and Canada were the top five countries in terms of the number of papers, with the remaining studies mainly originating from six other countries. As shown in Fig. 2 , the United States has the highest number of research projects among all countries, totaling 17. This indicates that the authors from the United States provide the most contributions to the research in SLT and PHE.
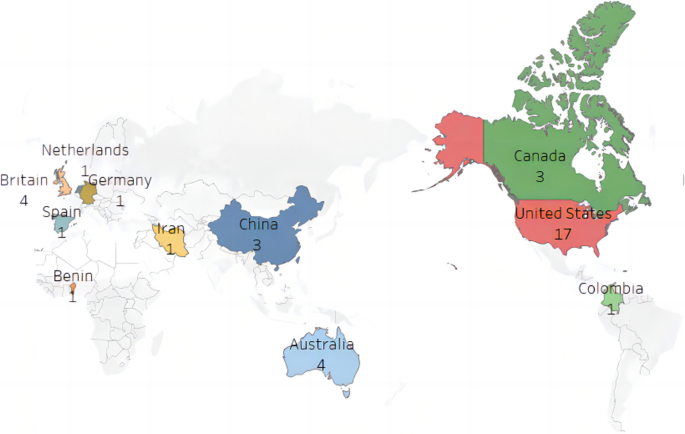
Distribution of 37 articles by country
Target groups of the studies
The studies covered 12 different target groups, including adolescents, students, vulnerable groups, community members, parents of children, young women, special patient groups, villagers, non-professional health workers, ethnic minorities, children in good health, and children with specific diseases. As shown in Fig. 3 . Adolescents and students were the primary focuses of the research. The health of vulnerable groups and community members were also a concern. There have been relatively few studies on children, but a significant number of studies have focused on children with rare diseases and their parents. Relatively little research has been done on groups such as young females, special patients, ethnic minorities, rural areas, and non-professional health workers.
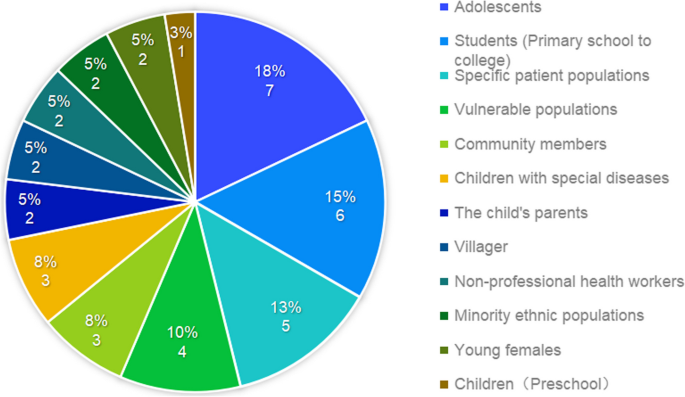
Types of target groups for studies
Research topics
Research articles can be categorized into the following six themes: (1) sex education, encompassing HIV/AIDS prevention and treatment, as well as sex education and contraception; (2) special diseases, including diabetes, COVID-19, cancer, etc.; (3) rare diseases, such as Lyme disease in adolescents, mine pneumoconiosis, rural people snake bites, etc.; (4) non-disease health behavior education for adolescents, involving drinking, violence, smoking, etc.; (5) general public education, covering a wide range of health topics; (6) spiritual and mental health education research, such as adolescents' and college students' mental health, personal mental health, etc. The details of these topics are shown in Table 3 .
Types of studies
Sampling research is the most common method in intervention research, followed by quantitative and qualitative research. The four studies combined qualitative and quantitative methods. Three studies conducted sample reviews, while the other two focused on descriptive and case studies, respectively. In addition, two literature reviews and a systematic review are included. In contrast, the utilization of case studies and descriptive studies is more limited, as shown in Fig. 4 .
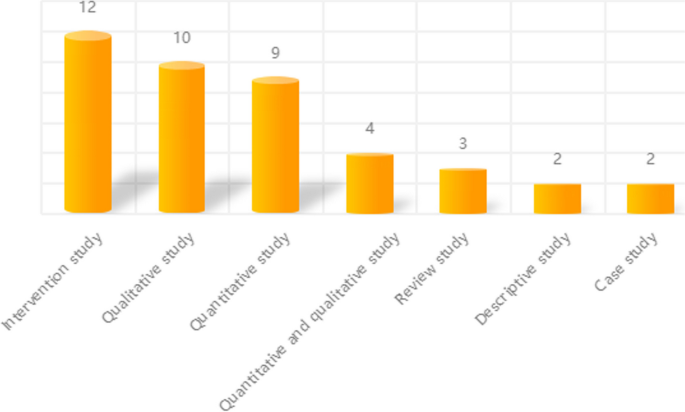
Methods and intervention forms of studies
There are nine experimental methods commonly used in research, as shown in Fig. 5 . Among these experimental methods, interviews, surveys, questionnaires, and (cluster) randomized controlled trials were widely used. Focus groups were used for qualitative studies, while T-tests and Chi-square tests were employed for quantitative studies. In addition, the methods of extended linear model, observation, and triangulation were also used.
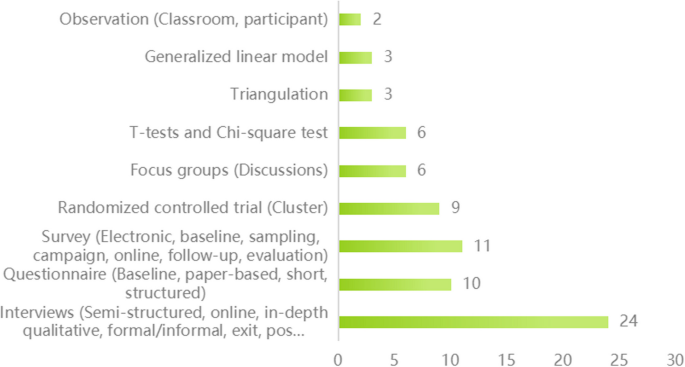
Types of research and data analysis methods
In this study, we primarily identified four models for implementing PHE (Table 4 ). The first model involves offline intervention, followed by the production of materials related to PHE. Another type of intervention is online intervention. Finally, adults, serving as role models for healthy behavior among adolescents, evaluated and screened health education content posted on social media.
Definition of the effectiveness of PHE
Among the 37 studies, 18 defined the effectiveness of PHE through questionnaires, interviews, and scales after implementing health interventions like randomized controlled trials. They measured the effectiveness of interventions by establishing learning efficacy standards across various dimensions. Gottfredson et al. verified the effectiveness of family health education by tracking research subjects and measuring the positive interaction among adolescent gender, parental involvement time, and other variables 39. 5 studies defined the effectiveness of PHE based on participants' engagement in health education courses and acceptance of interventions. Garcia et al.'s study utilized interviews to assess the acceptability of intervention measures among participants, thus determining the effectiveness of PHE 31. 14 studies did not explicitly mention how to define the effectiveness of PHE. According to the analysis of the results of the effectiveness definition of the included study samples, it can be concluded that the criteria of the researchers' definition of the effectiveness of PHE are relatively unclear. There is no single measure or factor that can gauge the effectiveness of PHE. Defining effectiveness solely in terms of engagement and acceptance of PHE interventions and health courses is not comprehensive.
Main findings and results of studies
First, offline education environments in PHE interventions led by governments, schools, communities, or other organizations can effectively enhance health education and promote the dissemination of PHE. Providing educational guidance or courses to the public or to specific patients and their families has a positive impact on their health. Implementing PHE in communities and schools can promote students' physical and mental health and reduce unhealthy behaviors.
On the other hand, online PHE has played an effective role in disseminating health knowledge, bridging social gaps, and promoting timely prevention. Online platforms, including websites, apps, communities, forums, and knowledge bases, effectively share PHE information. Social media and apps are effectively delivering health education messages.
Finally, adult health behaviors can influence adolescent health behaviors, making it crucial for adolescents to establish positive examples of healthy behaviors. Monitoring and screening health information on social platforms is essential to prevent minors from accessing inaccurate information. Therefore, the elimination of boundaries between academic education, schools, students, and classrooms has enhanced the effectiveness of public health communication in the school environment.
PHE in a social learning environment
In the implementation of the PHE project, we may face various challenges, such as dealing with diverse individuals from different backgrounds 12. Factors contributing to intervention failure include changing views due to external circumstances. This makes it necessary to adapt interventions to evolving conditions and diverse educational goals. The target populations in PHE interventions are broad and diverse. To promote acceptance and comprehension of complex public health knowledge among diverse audiences, relying solely on traditional classrooms is insufficient. PHE should be implemented in a diverse social environment to attract a wider audience and provide comprehensive health education. This will help PHE reach a wider audience and achieve the best educational outcomes.
PHE should be conducted in a wider and inclusive social setting, with community public spaces being suitable venues. Community public spaces are commonly chosen by researchers for PHE implementation. These spaces allow people to engage in their daily activities and seek assistance conveniently from local community organizations. Communities share commonalities, including the natural and social environment, and may face similar public health problems. Therefore, implementing quick, easy, and flexible PHE measures in the community environment is a suitable solution to address the complexity of the target population during implementation.
Studies show that community-based public health engagement programs are feasible and effective 23. particularly in addressing regional diabetes issues 25. Community cohesion and a sense of belonging promote active participation in community activities. Successful implementation of PHE in community settings requires collaboration among community workers, government or non-governmental organizations, health workers, and community members. Community participatory PHE enhances health literacy and has been proven effective. The advantage of PHE in a social setting is the opportunity for full participation and interaction. Creating a conducive educational environment and supporting observational learning are crucial for PHE effectiveness. Participatory art education, such as participatory theater, has shown beneficial impacts on health education [ 49 ].
SDOH also have a significant effect on PHE. SDOH refers to the economic and social conditions that affect changes in the health status of individuals and groups [ 50 ]. The influence of individual behavior on social learning in SLT is closely related to the economic and social conditions that affect changes in individual and group health status in SDOH. Individual learning behavior is influenced not only by the learning environment, motivation, and other factors but also by personal income, education level, occupation, and other factors. Therefore, utilizing SDOH to examine PHE can assist us in accurately identifying the key factors influencing health and implementing appropriate health education and interventions. The distribution of social perception factors is often influenced by mainstream political ideology and public policies in the region [ 51 ]. The Special Health Status Report highlights the impact of social inequalities on health, including differences in factors such as income, education level, occupation, and living environment that contribute to inequitable health outcomes. Hollederer et al. mentioned that the unemployed are a vulnerable group in society, and the health status of the unemployed is often worse. However, reaching the unemployed with prevention and health promotion efforts is challenging 34. In PHE, the application of SDOH helps to promote equitable and inclusive health education, focusing on the health needs of vulnerable groups and reducing health inequalities. Parents of children with specific diseases belong to the category of vulnerable groups who have limited access to equitable health resources and require additional support from society, government, and policymakers 17. SDOH emphasizes multifaceted influences, including individual, societal, and system-level factors. Cross-sectoral and interdisciplinary partnerships are essential for the public health sector to effectively respond to health challenges. Lamb et al. applied an interdisciplinary participatory art approach to community PHE research with indigenous youth 19. Promote cooperation among all stakeholders from a health and wellness perspective to create a healthy environment and enhance public health. Based on PHE, comprehensive health intervention strategies can be developed. These strategies may include improving socio-economic conditions, promoting education and career development, enhancing the living environment, and strengthening community support. Such integrated intervention strategies can influence individual health behaviors and outcomes more comprehensively. Therefore, the application of SDOH in PHE contributes to a deeper understanding of the root causes and influencing factors of health problems. It can also contribute to the effective implementation of public health policies and interventions, ultimately achieving the goal of promoting overall social health and equity.
Observational learning based on SLT is significant in PHE, particularly in AIDS prevention education 28. Research supports the effectiveness of observational learning in health education. Adolescents’ health concepts and behaviors are strongly influenced by their external environment, with parents or caregivers having the greatest influence 30. Minors acquire public health knowledge through observational learning in social settings and may adopt unhealthy behaviors from adults. However, they may not have the ability to discern whether adults’ decisions are right or wrong. Studies show that even if parents smoke, it may not necessarily impact minors’ behavior. Nevertheless, adults play a vital role in educating minors about healthy behaviors and should serve as positive examples 38.
Offline interventions for PHE with SLT
In this review, offline PHE interventions were commonly used. Offline interventions refer to PHE initiatives and behaviors carried out by local communities, schools, or organizations. These include the development of intervention policies, strategies, and models by the government, communities, schools, or relevant private organizations. Implementing appropriate health measures, educational models, or activities through offline modes can enhance the success and reach of PHE. Additionally, health intervention programs have been effective in addressing spiritual and mental health issues in specific populations 33. Offline interventions are also effective on campus, with integrated interventions successfully preventing youth violence in schools 20. Implementing offline interventions in school communities can reduce unhealthy habits and promote students' mental health, behavior control, and wellness. Furthermore, PHE interventions can also influence the behavior of health workers and target groups 14.
Offline implementation of PHE can also be achieved through organizing PHE activities, campaigns, workshops, courses, and conferences. These methods are commonly utilized in research. Through organizing various offline PHE activities, the enthusiasm of the public to participate in health education can be effectively enhanced, and the learning efficiency of health knowledge can be improved in an atmosphere of interactive learning. Studies have shown that when people participate in interactive learning, their ability to discover, imagine, and construct is improved, and their learning enthusiasm is enhanced [ 52 ].
The implementation of PHE guidance and curricula can have a positive impact on the general public, as well as on patients with specific diseases. Studies have found that short-term public health classroom learning programs based on SLT can effectively improve children's understanding of health knowledge and influence their health attitudes and behaviors 26. Other studies suggest that PHE in schools should aim to eliminate the divisions between health education and academic education, teachers and students, classrooms and schools, and schools and families 14. In other words, it is necessary to break the traditional classroom education mode and overcome the limitations of the traditional classroom in space, time, form, and content through more flexible and diversified education methods.
Online interventions for PHE with SLT
Online PHE interventions are widely used and offer several advantages. They provide flexibility in terms of time and location, enabling individuals to access public health knowledge at their convenience. Online platforms have a broader reach compared to offline interventions, which are limited by the number of participants. Online PHE interventions can be continuous, allowing for updates and improvements in educational content. These interventions are particularly important for individuals facing mobility barriers and caregivers who struggle to attend offline activities. Research shows that online platforms serve as essential resources for health information and social support for people with specific diseases and their caregivers. Users rely on these platforms to gain knowledge, make informed decisions, and learn from the experiences of others 16. Online health education platforms have proven effective in supporting parents of children with special diseases and treatment needs 37 [ 53 ]. Optimizing digital health literacy among young people is critical due to their heavy use of the internet 27. Enhancing online platforms is necessary to cater to the educational needs of young people. Studies have also demonstrated the effectiveness of providing distance health education to young individuals 24.
Online platform researchers and designers gather user feedback to understand their needs and preferences, enabling them to create customized tools 30. Creating online virtual multidisciplinary communities of practice is an innovative approach to PHE, ensuring high-quality public health services for patients with specific diseases in specific areas 20. It is crucial for the online platform to fully meet user needs in order to retain users 22. Educational video games can complement other media in child health education programs, positively impacting children's health education 18. Videos and programs distributed through social media or other online platforms also contribute positively to PHE 18.
Individual social learning behavior and PHE
According to the research, PHE interventions can be categorized into two forms: online and offline. These interventions have different impacts on individual learning behaviors in the social environment, which can be further classified into two main aspects:
PHE interventions can be categorized into online and offline forms. In the societal learning environment, an individual's learning behavior in PHE is influenced by two objective factors: the temporal factor and the spatial factor. Offline PHE requires individuals to go to a specific location at specific times, which limits their ability to participate in and complete their education. Offline interventions also have limited coverage and lack long-term continuity. On the other hand, online PHE provides flexibility and is not significantly impacted by timing and location restrictions. It requires more preparation, but it offers learners more control and convenience. Online platforms enable a focus on evolving social dynamics, as well as individual and group differences, in public health and service delivery.
In the social learning environment, the distinction between active and passive learning affects individual learning behaviors in PHE. Participants in PHE activities need to have a high level of learning initiative in order to successfully complete the educational process. Offline PHE requires individuals to actively seek out and participate in educational activities, contributing to their own learning initiative. Additionally, offline interventions organized by reputable institutions enhance participation and interactivity, thereby improving learners’ efficiency and motivation. Incorporating participatory visual arts activities in offline interventions for dementia patients has shown positive impacts on cognitive, social, and psychological functioning [ 54 ]. Jensen et al. study also supports the benefits of participating in artistic and cultural activities for sexual health [ 55 ]. On the other hand, online health education interventions do not require a high level of learning initiative, as individuals can access knowledge whenever needed. However, accessing health information online carries the risk of encountering incorrect information, which is a disadvantage of online PHE.
Online and offline forms of PHE have their own advantages and disadvantages, and integrating both forms can fully leverage their strengths. Some studies have integrated online learning modules with traditional face-to-face courses to educate learners on cancer and health. The results showed that participants who engaged in both online and offline learning were motivated to modify their health behaviors in order to reduce the risk of cancer 29. Whether PHE is conducted online or offline, both the intervener and education recipients need to have motivation and enthusiasm to promote the completion of educational activities. To enhance the effectiveness and engagement of PHE initiatives, researchers and government personnel should consider integrating multiple health education models. This approach will help achieve PHE goals and maximize its impact.
Future trends in exploration
Future PHE research can focus on several areas, including prevention of large-scale infectious diseases, basic public health knowledge, health education for individuals with specific diseases and their families, as well as for vulnerable groups such as ethnic minorities and persons with disabilities. There is a lack of studies on the elderly population, despite the increasing global aging trend and the health challenges they face. Researchers should prioritize addressing the health concerns of the elderly and develop strategies for providing convenient and comprehensible PHE for this age group, particularly in chronic diseases, underlying conditions, and self-care. Adolescent health education and sex education remain important topics, but research may also shift towards including special patients, minority groups, and members of the broader community. The focus should be broadened to not overlook the health interests of minority populations. Additionally, PHE in the social environment should expand to include mental health, given the increasing mental and psychological pressures people face and their impact on personal and professional lives. Spiritual and mental health education should be emphasized in PHE.
In the future, the focus on social learning environments can be on enhancing public enthusiasm and participation in PHE by creating engaging and participatory educational atmospheres in community spaces. PHE interventions may combine online and offline approaches and collaborate with comprehensive intervention models to develop educational materials. Offline PHE can promote interaction and participation, increasing learners' motivation. Online PHE can enhance the dissemination of public health knowledge through user-friendly platforms and multimedia forms like AI interactive video games and electronic comics/animations. This combination of online and offline education, along with appropriate PHE materials, can greatly enhance understanding and facilitate widespread dissemination of PHE. The integrated use of these three forms can have a significant impact on PHE behavior in the social environment.
Strengths and limitations
Introducing SLT into the field of PHE, the study explores the relationship between individual learning and behavior in social settings, focusing on the effectiveness of offline and online PHE. The study highlights the importance of removing boundaries in school settings and promoting cross-cutting PHE. This perspective on cross-disciplinary implementation offers a novel approach to enhancing the comprehensiveness and dissemination of PHE. It also presents a clear focus and direction for future PHE research based on SLT, which includes an emphasis on preventing large-scale infectious diseases, providing health education for patients with specific diseases, and addressing health issues among the elderly population. It is unique in the selection of research topics, methodological framework, and prospects of research conclusions, providing distinctive contributions and values for the research and practice of PHE. However, this review has several limitations. First, this review does not include non-English publications or gray literature from related fields. As a result, some pertinent studies may have been overlooked. Secondly, while each scope review is subject to our assessment, no individual quality assessment score is provided for each sample article. The reason for this is that such an assessment would not add value to the review.
We have reviewed 37 studies of SLT based PHE. Most of them focus on adolescents and students, as well as sexual and disease-specific education, while it is fewer to study minorities and vulnerable groups, such young females and specific diseases. For PHE implementation, the combination of both online and offline PHE can be more effective by improving the real-time accessibility of health knowledge dissemination, and encouraging individuals to participate in PHE. The development of integrated PHE intervention models and diverse educational materials can promote the dissemination of public health knowledge and encourage broad participation. As suggested in our review, it is feasible and effective to offer participatory PHE in social learning settings, particularly within the community. The social learning environment should focus on fostering an interactive and participatory educational climate to increase public interest and participation in PHE. Additionally, researchers should prioritize the prevention of a wide range of infectious diseases, the dissemination of basic public health information, and health education for vulnerable populations, individuals with specific diseases, and their families. In conclusion, this work provides important references for further strengthening the implementation strategy and practice of PHE based on SLT.
Availability of data and materials
The author confirms that all data generated or analysed during this study are included in this published article. Furthermore, primary and secondary sources and data supporting the findings of this study were all publicly available at the time of submission.
Public health in England. The report of the committee of inquiry into the future development of the public health function. London: H.M.S.O; 1988.
Google Scholar
Public health: A conceptual framework. Available from: https://www.cpha.ca/public-health-conceptual-framework . Cited 2024 Jan 24.
Mukhalalati B, Elshami S, Eljaam M, Hussain FN, Bishawi AH. Applications of social theories of learning in health professions education programs: a scoping review. Front Med. 2022;9:9. https://doi.org/10.3389/fmed.2022.912751 .
Article Google Scholar
Bandura A. Social learning theory. Englewood Cliffs, N.J: Prentice-Hall; 1977.
McCullough CA. Social Learning Theory and behavioral therapy: considering human behaviors within the social and cultural context of individuals and families. Social Work in Public Health. 2011;26(5):471–81. https://doi.org/10.1080/19371918.2011.591629 .
Moorhead SA, Hazlett DE, Harrison L, Carroll JK, Irwin A, Hoving C. A new dimension of health care: systematic review of the uses, benefits, and limitations of social media for health communication. J Med Internet Res. 2013;15(4):e85. https://doi.org/10.2196/jmir.1933 .
Article PubMed PubMed Central Google Scholar
Gaze JP, Oetjen R. “Perceptions of Diversity in the Largest Overseas US Navy Hospital.” Divers Equality Health Care 11. 2014;(2). https://doi.org/10.21767/2049-5471.100018 .
Zodpey SP, Negandhi H, Yeravdekar R. Future directions for public health education reforms in India. Front Public Health. 2014. https://doi.org/10.3389/fpubh.2014.00068 .
Moher D, Liberati A, Tetzlaff J, Altman DG. Preferred reporting items for systematic reviews and meta-analyses: the prisma statement. Int J Surg. 2010;8(5):336–41. https://doi.org/10.1016/j.ijsu.2010.02.007 .
Article PubMed Google Scholar
Arksey H, O’Malley L. Scoping studies: Towards a methodological framework. Int J Soc Res Methodol. 2005;8(1):19–32. https://doi.org/10.1080/1364557032000119616 .
Peters MDJ, Marnie C, Tricco AC, Pollock D, Munn Z, Alexander L, et al. Updated methodological guidance for the conduct of scoping reviews. JBI Evidence Synthesis. 2020;18(10):2119–26. https://doi.org/10.11124/jbies-20-00167 .
Molassiotis A, Xie YJ, Leung AY, Ho GW, Li Y, Leung PH-M, et al. A community-based participatory research approach to developing and testing social and behavioural interventions to reduce the spread of SARS-COV-2: A protocol for the ‘COPAR for COVID’ programme of research with five interconnected studies in the Hong Kong context. Int J Environ Res Pub Health. 2022;19(20):13392. https://doi.org/10.3390/ijerph192013392 .
Tancred T, Paparini S, Melendez-Torres GJ, Thomas J, Fletcher A, Campbell R, et al. A systematic review and synthesis of theories of change of school-based interventions integrating health and academic education as a novel means of preventing violence and substance use among students. Syst Rev. 2018;7(1). https://doi.org/10.1186/s13643-018-0862-y .
Akers AY, Skolnik A, DiFiore G, Harding J, Timko CA. Feasibility Study of a health coaching intervention to improve contraceptive continuation in adolescent and Young Adult Women in Philadelphia, Pennsylvania. Perspect Sex Reprod Health. 2021;53(3–4):27–43. https://doi.org/10.1363/psrh.12188 .
Nyblade L, Srinivasan K, Mazur A, Raj T, Patil DS, Devadass D, et al. HIV stigma reduction for health facility staff: Development of a blended- learning intervention. Front Public Health. 2018;6. https://doi.org/10.3389/fpubh.2018.00165 .
Moreno-Leguizamon C, Tovar-Restrepo M, Irazábal C, Locke C. Learning alliance methodology: contributions and challenges for multicultural planning in health service provision: a case study in Kent. UK Plann Theory Pract. 2015;16(1):79–96. https://doi.org/10.1080/14649357.2014.990403 .
Karns CM, Todis B, Glenn E, Glang A, Wade SL, Riddle I, et al. Seeking out social learning: Online self-education in parents of children with intellectual and developmental disabilities. Intellect Dev Disabil. 2022;60(4):303–15. https://doi.org/10.1352/1934-9556-60.4.303 .
Warren E, Melendez-Torres GJ, Viner R, Bonell C. Using qualitative research to explore intervention mechanisms: Findings from the trial of the learning together whole-school health intervention. Trials. 2020;21(1). https://doi.org/10.1186/s13063-020-04688-2 .
Lamb P. “we need to do public health differently in this community”: A reflexive analysis of participatory video, affective relations, and the policy process. Agenda. 2020;35(1):42–53. https://doi.org/10.1080/10130950.2020.1844460 .
Teti M, David I, Myroniuk TW, Schatz E. A qualitative evaluation of a campus wide covid-19 Health Education campaign: intent, impact, and ideas for the future. Health Educ J. 2022;81(7):807–22. https://doi.org/10.1177/00178969221122917 .
Sood A, Assad N, Jarrell W, Kalishman S, Le Suer K, Murillo S, et al. A virtual community-of-practice approach by rural stakeholders in managing pneumoconiosis in the USA: A cross-sectional analysis. Rural Remote Health. 2020. https://doi.org/10.22605/rrh5784 .
Burman C, Mendoza Diaz A, Leslie A, Goldthorp K, Jubb B, Simms AR, et al. Critical success factors for school-based integrated health care models: learnings from an Australian example. Health Promot J Austr. 2023;34(4):775–83. https://doi.org/10.1002/hpja.690 .
Zogbochi V, Edoh T, Hounsou JT, Ameduit AK, Alahassa B. Detecting the mobility of patient with chronic diseases in online health communities using ant colony optimization algorithm ensure patient’s safety and diseases awareness based on reliable medical education material. 2018 1st International Conference on Smart Cities and Communities (SCCIC). 2018. https://doi.org/10.1109/sccic.2018.8584556 .
Solomon P, Salbach NM, O’Brien KK, Nixon S, Baxter L, Gervais N. Evaluation of a community-based self-management program to increase access to rehabilitation for people living with HIV. Journal of the International Association of Providers of AIDS Care (JIAPAC). 2019;18:232595821988333. https://doi.org/10.1177/2325958219883334 .
Denehy M, Crawford G, Leavy J, Nimmo L, Jancey J. Formative research to develop theory-based messages for a Western Australian child drowning prevention television campaign: study protocol: Table 1. BMJ Open. 2016;6(5). https://doi.org/10.1136/bmjopen-2015-010033 .
Morrison J, Akter K, Jennings HM, Nahar T, Kuddus A, Shaha SK, et al. Participatory learning and action to address type 2 diabetes in rural Bangladesh: a qualitative process evaluation. BMC Endocr Dis. 2019;19(1). https://doi.org/10.1186/s12902-019-0447-3 .
Shadick NA, Zibit MJ, Nardone E, DeMaria A, Iannaccone CK, Cui J. A school-based intervention to increase lyme disease preventive measures among elementary school-aged children. Vector-Borne Zoonotic Dis. 2016;16(8):507–15. https://doi.org/10.1089/vbz.2016.1942 .
Taba M, Allen TB, Caldwell PHY, Skinner SR, Kang M, McCaffery K, et al. Adolescents’ self-efficacy and digital health literacy: a cross-sectional mixed methods study. BMC Public Health. 2022;22(1). https://doi.org/10.1186/s12889-022-13599-7 .
Javier SJ, Abrams JA, Moore MP, Belgrave FZ. Condom use efficacy and sexual communication skills among African American college women. Health Promot Pract. 2016;19(2):287–94. https://doi.org/10.1177/1524839916676253 .
Cueva K, Revels L, Cueva M, Lanier AP, Dignan M, Viswanath K, et al. Culturally-relevant online cancer education modules empower Alaska’s community health aides/practitioners to disseminate cancer information and reduce cancer risk. J Cancer Educ. 2017;33(5):1102–9. https://doi.org/10.1007/s13187-017-1217-4 .
Garcia JM, Shurack R, Leahy N, Brazendale K, Lee E, Lawrence S. Feasibility of a remote-based nutrition education and culinary skills program for young adults with autism spectrum disorder. J Nutr Educ Behav. 2023;55(3):215–23. https://doi.org/10.1016/j.jneb.2022.11.002 .
Trujillo EM, Suárez DE, Lema M, Londoño A. How adolescents learn about risk perception and behavior in regards to alcohol use in light of social learning theory: a qualitative study in Bogotá, Colombia. Int J Adolesc Med Health. 2015;27(1):3–9. https://doi.org/10.1515/ijamh-2014-0003 .
Hakojärvi HR, Selänne L, Salanterä S. Child involvement in oral health education interventions - a systematic review of randomised controlled studies. Community Dent Health. 2019;36(4):286–92. https://doi.org/10.1922/CDH_Hakojarvi07 .
Hollederer A, Jahn HJ, Klein D. Jobs program Germany for health promotion among the unemployed in the community setting with institutions for employment promotion (jobsprogramdtl): study protocol for a randomized controlled trial. BMC Public Health. 2021;21(1). https://doi.org/10.1186/s12889-021-10251-8 .
Vives-Cases C, Davo-Blanes MC, Ferrer-Cascales R, Sanz-Barbero B, Albaladejo-Blázquez N, Sánchez-San Segundo M, et al. Lights4violence: a quasi-experimental educational intervention in six European countries to promote positive relationships among adolescents. BMC Public Health. 2019;19(1). https://doi.org/10.1186/s12889-019-6726-0 .
Article CAS PubMed PubMed Central Google Scholar
Chen M-F, Wang R-H, Hung S-L. Predicting health-promoting self-care behaviors in people with pre-diabetes by applying bandura social learning theory. Appl Nurs Res. 2015;28(4):299–304. https://doi.org/10.1016/j.apnr.2015.01.001 .
Ghoreishi M-S, Vahedian-shahroodi M, Jafari A, Tehranid H. Self-care behaviors in patients with type 2 diabetes: Education intervention base on social cognitive theory. Diabetes Metab Syndr. 2019;13(3):2049–56. https://doi.org/10.1016/j.dsx.2019.04.045 .
DeHoff BA, Staten LK, Rodgers RC, Denne SC. The role of online social support in supporting and educating parents of young children with special health care needs in the United States: a scoping review. J Med Internet Res. 2016;18(12). https://doi.org/10.2196/jmir.6722 .
Gottfredson NC, Hussong AM, Ennett ST, Rothenberg WA. The role of parental engagement in the intergenerational transmission of smoking behavior and identity. J Adolesc Health. 2017;60(5):599–605. https://doi.org/10.1016/j.jadohealth.2016.11.004 .
Hickling A, Mallory KD, Wilson KE, Del Fernandes R, Fuselli P, Reed N. The Youth Concussion Awareness Network (YOU-CAN) - a school-based peer-led intervention to improve concussion reporting and social support: the protocol for a cluster randomized trial. BMC Public Health. 2020;20(1). https://doi.org/10.1186/s12889-020-8244-5 .
Williams O, Swierad E. A multisensory multilevel health education model for diverse communities. Int J Environ Res Public Health. 2019;16(5):872. https://doi.org/10.3390/ijerph16050872 .
Horrell LN, Kneipp SM, Ahn S, Towne SD, Mingo CA, Ory MG, et al. Chronic disease self-management education courses: utilization by low-income, middle-aged participants. Int J Equity Health. 2017;16(1):114. https://doi.org/10.1186/s12939-017-0604-0 .
Beaujean DJ, Gassner F, Wong A, Steenbergen JE, Crutzen R, Ruwaard D. Education on tick bite and Lyme Borreliosis Prevention, aimed at schoolchildren in the Netherlands: Comparing the effects of an online educational video game versus a leaflet or no intervention. BMC Public Health. 2016;16(1). https://doi.org/10.1186/s12889-016-3811-5 .
Vitagliano LA, Wester KL, Jones CT, Wyrick DL, Vermeesch AL. Group nature-based mindfulness interventions: nature-based mindfulness training for college students with anxiety. Int J Environ Res Public Health. 2023;20(2):1451. https://doi.org/10.3390/ijerph20021451 .
Li Y, Yan X. How could peers in online health community help improve health behavior. Int J Environ Res Public Health. 2020;17(9):2995. https://doi.org/10.3390/ijerph17092995 .
Mahmood MA, Halliday D, Cumming R, Thwin KT, Myitzu M, White J, et al. Inadequate knowledge about snakebite envenoming symptoms and application of harmful first aid methods in the community in high snakebite incidence areas of Myanmar. PLoS Negl Trop Dis. 2019;13(2). https://doi.org/10.1371/journal.pntd.0007171 .
Caballero A, Leath KJ, Staton AD. Strategic combination of theory, plain language, and trusted messengers contribute to COVID-19 vaccine uptake: lessons learned from development and dissemination of a community toolkit. Vaccines. 2023;11(6):1064. https://doi.org/10.3390/vaccines11061064 .
Farber EW, Ali MK, Van Sickle KS, Kaslow NJ. Psychology in patient-centered medical homes: reducing health disparities and promoting health equity. Am Psychol. 2017;72(1):28–41. https://doi.org/10.1037/a0040358 .
Leung J, Som A, McMorrow L, Zickuhr L, Wolbers J, Bain K, et al. Rethinking the difficult patient: formative qualitative study using participatory theater to improve physician-patient communication in rheumatology. JMIR Formative Res. 2023;7. https://doi.org/10.2196/40573 .
Braveman P, Gottlieb L. The social determinants of health: it’s time to consider the causes of the causes. Public Health Rep. 2014;129:19–31. https://doi.org/10.1177/00333549141291S206 .
Raphael D, Bryant T, Mikkonen J, Raphael A. ocial determinants of health: the Canadian facts. Oshawa: Ontario Tech University Faculty of Health Sciences and Toronto: York University School of Health Policy and Management; 2020.
Oprea CL. Interactive and creative learning of the adults. Procedia Soc Behav Sci. 2014;142:493–8. https://doi.org/10.1016/j.sbspro.2014.07.654 .
Pang PCI, Munsie M, Chang S, Tanner C, Walker C. Participatory design and evaluation of the “Stem Cells Australia” website for delivering complex health knowledge: mixed methods study. J Med Internet Res. 2023;25:e44733. https://doi.org/10.2196/44733 .
Cavalcanti Barroso A, Rai HK, Sousa L, Orrell M, Schneider J. Participatory visual arts activities for people with dementia: a review. Perspect Public Health. 2020;142(1):22–31. https://doi.org/10.1177/1757913920948916 .
Jensen A, Folker AP, Lindström M, Ekholm O. Arts and culture engagement for health: a danish population-based study. Public Health. 2023;225:120–6. https://doi.org/10.1016/j.puhe.2023.09.012 .
Download references
Acknowledgements
The authors acknowledge the advice and assistance from Prof. Ying Lau from the Chinese University of Hong Kong in preparing this paper.
This research was funded by Macao Science and Technology Development Fund (funding ID: 0088/2023/ITP2) and Macao Polytechnic University research grant (project code: RP/FCA-10/2022).
Author information
Authors and affiliations.
Faculty of Applied Sciences, Macao Polytechnic University, Macao, China
Ting Liu, Patrick Cheong-Iao Pang & Chi-Kin Lam
You can also search for this author in PubMed Google Scholar
Contributions
P.P. and T.L. proposed and designed this study. C.L. helped with data analyses and resolved disagreements in the review process. T.L. wrote the manuscript with the assistance from P.P. P.P. supervised and provided resources for this work. All authors discussed the results and contributed to the final manuscript.
Corresponding author
Correspondence to Patrick Cheong-Iao Pang .
Ethics declarations
Ethics approval and consent to participate.
Not applicable.
Consent for publication
Competing interests.
The authors declare no competing interests.
Additional information
Publisher’s note.
Springer Nature remains neutral with regard to jurisdictional claims in published maps and institutional affiliations.
Rights and permissions
Open Access This article is licensed under a Creative Commons Attribution 4.0 International License, which permits use, sharing, adaptation, distribution and reproduction in any medium or format, as long as you give appropriate credit to the original author(s) and the source, provide a link to the Creative Commons licence, and indicate if changes were made. The images or other third party material in this article are included in the article's Creative Commons licence, unless indicated otherwise in a credit line to the material. If material is not included in the article's Creative Commons licence and your intended use is not permitted by statutory regulation or exceeds the permitted use, you will need to obtain permission directly from the copyright holder. To view a copy of this licence, visit http://creativecommons.org/licenses/by/4.0/ . The Creative Commons Public Domain Dedication waiver ( http://creativecommons.org/publicdomain/zero/1.0/ ) applies to the data made available in this article, unless otherwise stated in a credit line to the data.
Reprints and permissions
About this article
Cite this article.
Liu, T., Pang, P.CI. & Lam, CK. Public health education using social learning theory: a systematic scoping review. BMC Public Health 24 , 1906 (2024). https://doi.org/10.1186/s12889-024-19333-9
Download citation
Received : 25 January 2024
Accepted : 02 July 2024
Published : 16 July 2024
DOI : https://doi.org/10.1186/s12889-024-19333-9
Share this article
Anyone you share the following link with will be able to read this content:
Sorry, a shareable link is not currently available for this article.
Provided by the Springer Nature SharedIt content-sharing initiative
- Public health education
- Social learning theory
- Systematic scoping review
BMC Public Health
ISSN: 1471-2458
- General enquiries: [email protected]
Suggestions or feedback?
MIT News | Massachusetts Institute of Technology
- Machine learning
- Sustainability
- Black holes
- Classes and programs
Departments
- Aeronautics and Astronautics
- Brain and Cognitive Sciences
- Architecture
- Political Science
- Mechanical Engineering
Centers, Labs, & Programs
- Abdul Latif Jameel Poverty Action Lab (J-PAL)
- Picower Institute for Learning and Memory
- Lincoln Laboratory
- School of Architecture + Planning
- School of Engineering
- School of Humanities, Arts, and Social Sciences
- Sloan School of Management
- School of Science
- MIT Schwarzman College of Computing
Large language models don’t behave like people, even though we may expect them to
Press contact :, media download.
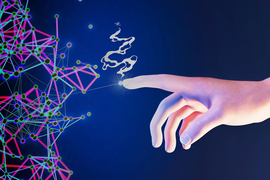
*Terms of Use:
Images for download on the MIT News office website are made available to non-commercial entities, press and the general public under a Creative Commons Attribution Non-Commercial No Derivatives license . You may not alter the images provided, other than to crop them to size. A credit line must be used when reproducing images; if one is not provided below, credit the images to "MIT."
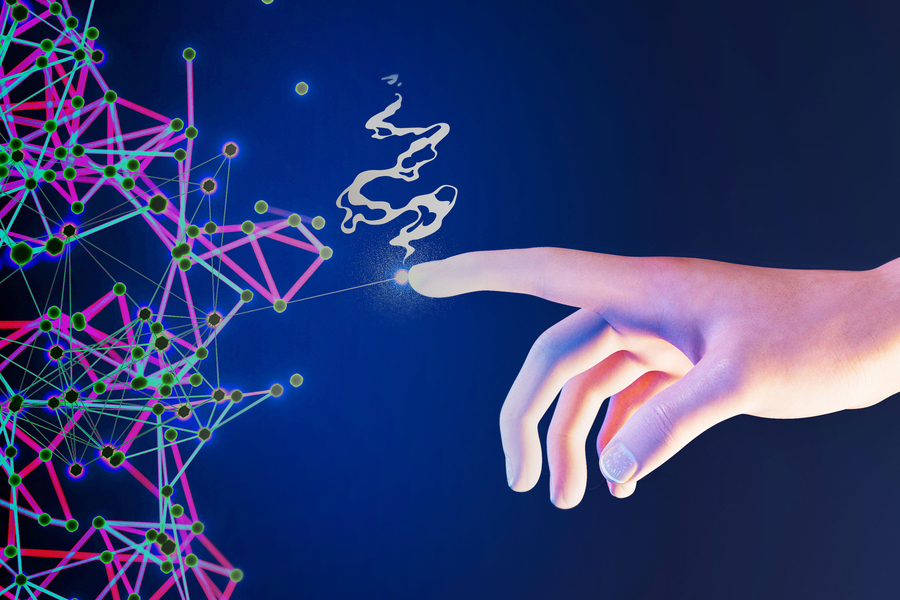
Previous image Next image
One thing that makes large language models (LLMs) so powerful is the diversity of tasks to which they can be applied. The same machine-learning model that can help a graduate student draft an email could also aid a clinician in diagnosing cancer.
However, the wide applicability of these models also makes them challenging to evaluate in a systematic way. It would be impossible to create a benchmark dataset to test a model on every type of question it can be asked.
In a new paper , MIT researchers took a different approach. They argue that, because humans decide when to deploy large language models, evaluating a model requires an understanding of how people form beliefs about its capabilities.
For example, the graduate student must decide whether the model could be helpful in drafting a particular email, and the clinician must determine which cases would be best to consult the model on.
Building off this idea, the researchers created a framework to evaluate an LLM based on its alignment with a human’s beliefs about how it will perform on a certain task.
They introduce a human generalization function — a model of how people update their beliefs about an LLM’s capabilities after interacting with it. Then, they evaluate how aligned LLMs are with this human generalization function.
Their results indicate that when models are misaligned with the human generalization function, a user could be overconfident or underconfident about where to deploy it, which might cause the model to fail unexpectedly. Furthermore, due to this misalignment, more capable models tend to perform worse than smaller models in high-stakes situations.
“These tools are exciting because they are general-purpose, but because they are general-purpose, they will be collaborating with people, so we have to take the human in the loop into account,” says study co-author Ashesh Rambachan, assistant professor of economics and a principal investigator in the Laboratory for Information and Decision Systems (LIDS).
Rambachan is joined on the paper by lead author Keyon Vafa, a postdoc at Harvard University; and Sendhil Mullainathan, an MIT professor in the departments of Electrical Engineering and Computer Science and of Economics, and a member of LIDS. The research will be presented at the International Conference on Machine Learning.
Human generalization
As we interact with other people, we form beliefs about what we think they do and do not know. For instance, if your friend is finicky about correcting people’s grammar, you might generalize and think they would also excel at sentence construction, even though you’ve never asked them questions about sentence construction.
“Language models often seem so human. We wanted to illustrate that this force of human generalization is also present in how people form beliefs about language models,” Rambachan says.
As a starting point, the researchers formally defined the human generalization function, which involves asking questions, observing how a person or LLM responds, and then making inferences about how that person or model would respond to related questions.
If someone sees that an LLM can correctly answer questions about matrix inversion, they might also assume it can ace questions about simple arithmetic. A model that is misaligned with this function — one that doesn’t perform well on questions a human expects it to answer correctly — could fail when deployed.
With that formal definition in hand, the researchers designed a survey to measure how people generalize when they interact with LLMs and other people.
They showed survey participants questions that a person or LLM got right or wrong and then asked if they thought that person or LLM would answer a related question correctly. Through the survey, they generated a dataset of nearly 19,000 examples of how humans generalize about LLM performance across 79 diverse tasks.
Measuring misalignment
They found that participants did quite well when asked whether a human who got one question right would answer a related question right, but they were much worse at generalizing about the performance of LLMs.
“Human generalization gets applied to language models, but that breaks down because these language models don’t actually show patterns of expertise like people would,” Rambachan says.
People were also more likely to update their beliefs about an LLM when it answered questions incorrectly than when it got questions right. They also tended to believe that LLM performance on simple questions would have little bearing on its performance on more complex questions.
In situations where people put more weight on incorrect responses, simpler models outperformed very large models like GPT-4.
“Language models that get better can almost trick people into thinking they will perform well on related questions when, in actuality, they don’t,” he says.
One possible explanation for why humans are worse at generalizing for LLMs could come from their novelty — people have far less experience interacting with LLMs than with other people.
“Moving forward, it is possible that we may get better just by virtue of interacting with language models more,” he says.
To this end, the researchers want to conduct additional studies of how people’s beliefs about LLMs evolve over time as they interact with a model. They also want to explore how human generalization could be incorporated into the development of LLMs.
“When we are training these algorithms in the first place, or trying to update them with human feedback, we need to account for the human generalization function in how we think about measuring performance,” he says.
In the meanwhile, the researchers hope their dataset could be used a benchmark to compare how LLMs perform related to the human generalization function, which could help improve the performance of models deployed in real-world situations.
“To me, the contribution of the paper is twofold. The first is practical: The paper uncovers a critical issue with deploying LLMs for general consumer use. If people don’t have the right understanding of when LLMs will be accurate and when they will fail, then they will be more likely to see mistakes and perhaps be discouraged from further use. This highlights the issue of aligning the models with people's understanding of generalization,” says Alex Imas, professor of behavioral science and economics at the University of Chicago’s Booth School of Business, who was not involved with this work. “The second contribution is more fundamental: The lack of generalization to expected problems and domains helps in getting a better picture of what the models are doing when they get a problem ‘correct.’ It provides a test of whether LLMs ‘understand’ the problem they are solving.”
This research was funded, in part, by the Harvard Data Science Initiative and the Center for Applied AI at the University of Chicago Booth School of Business.
Share this news article on:
Related links.
- Ashesh Rambachan
- Laboratory for Information and Decision Systems
- Department of Electrical Engineering and Computer Science
- Department of Economics
Related Topics
- Computer science and technology
- Artificial intelligence
- Human-computer interaction
- Laboratory for Information and Decision Systems (LIDS)
- School of Humanities Arts and Social Sciences
Related Articles
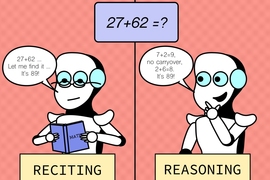
Reasoning skills of large language models are often overestimated
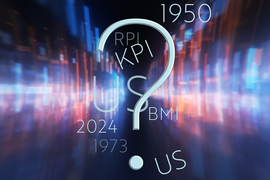
Technique improves the reasoning capabilities of large language models
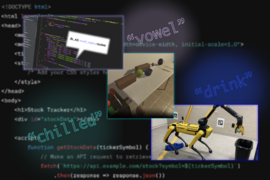
Natural language boosts LLM performance in coding, planning, and robotics
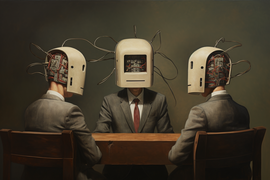
Multi-AI collaboration helps reasoning and factual accuracy in large language models
Previous item Next item
More MIT News

Edgerton Center hosts workshop for deaf high school students in STEM
Read full story →

MIT Global SCALE Network expands by adding center at Loughborough University
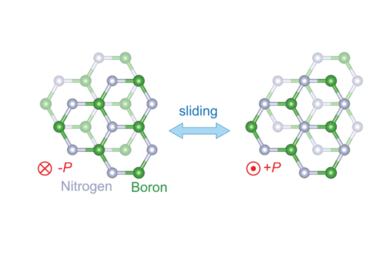
New transistor’s superlative properties could have broad electronics applications
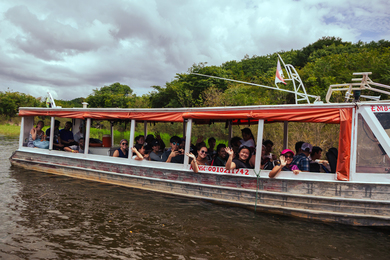
When learning at MIT means studying thousands of miles away
Flying high to enable sustainable delivery, remote care
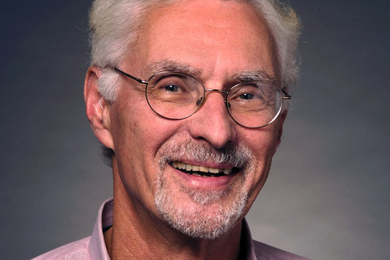
Professor Emeritus Ralph Gakenheimer, mobility planner and champion of international development, dies at 89
- More news on MIT News homepage →
Massachusetts Institute of Technology 77 Massachusetts Avenue, Cambridge, MA, USA
- Map (opens in new window)
- Events (opens in new window)
- People (opens in new window)
- Careers (opens in new window)
- Accessibility
- Social Media Hub
- MIT on Facebook
- MIT on YouTube
- MIT on Instagram
Nursing aide turned sniper: Thomas Crooks' mysterious plot to kill Trump
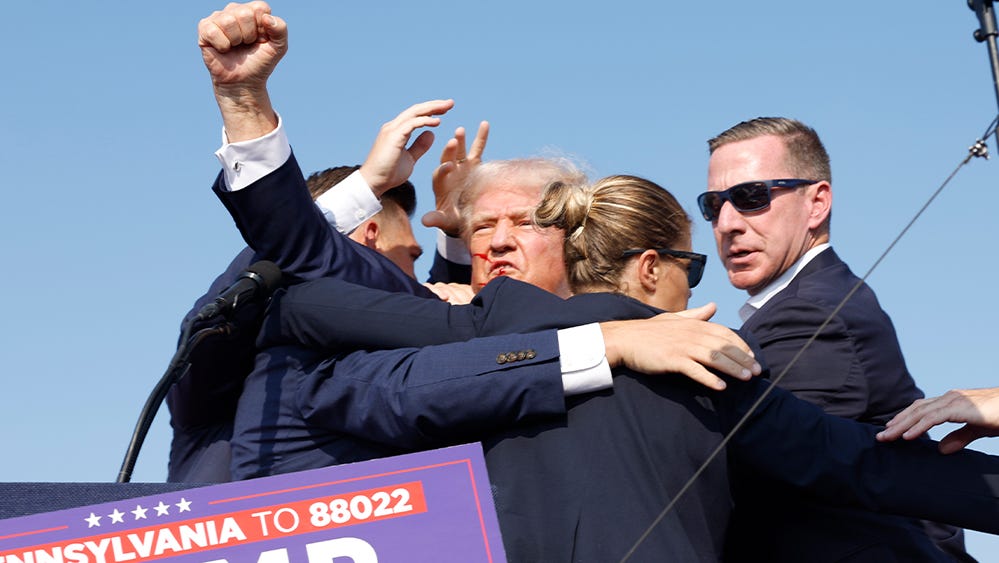
BUTLER, Pa. – Donald Trump and would-be assassin Thomas Crooks started on their violent collision course long before the former president's political rally ended in gunshots and death.
Crooks, 20, was a one-time registered Republican, a nursing home worker with no criminal record, shy in school, and living in a decent middle-class neighborhood in suburban Pennsylvania with his parents. Trump, 78, was eyeing Crooks' state as a key battleground – but not in the way that anyone envisioned on Saturday.
Riding high on polls showing that he's got a strong chance of toppling President Joe Biden, the former president had been campaigning for reelection in swing states, and Pennsylvania is a key prize. Trump won the state in 2016 but lost it four years later.
And on July 3, Trump's campaign announced he would hold a rally at the Butler Farm Show grounds, about 30 miles north of Pittsburgh.
"Pennsylvania has been ravaged by monumental surges in violent crime as a direct result of Biden’s and Democrats’ pro-criminal policies," Trump's campaign said in announcing the event, noting that when he's elected, he'll "re-establish law and order in Pennsylvania!"
The Saturday attack on Trump turned the heated rhetoric of the 2024 presidential campaign freshly violent. Authorities said bullets fired from Crooks' AR-15 style rifle about 150 yards away grazed Trump's ear, killed a rally attendee as he dove to protect his family, and critically wounded two others. Secret Service agents killed Crooks moments later.
Attack planned well in advance
Investigators are still seeking Crooks' motive – despite his Republican leanings, he had donated recently to a progressive voter-turnout campaign in 2021 – but indicated he'd planned the attack well in advance.
The shooting marks the first assassination attempt against a former or current U.S. president since President Ronald Reagan was injured in a March 1981 shooting at a Washington, D.C., hotel.
There are many questions about why Crooks turned into a would-be presidential assassin, firing indiscriminately into hordes of political supporters.
FBI special agent Kevin Rojek said on a call with media that law enforcement located "a suspicious device" when they searched Crooks' vehicle and that it's being analyzed at the FBI crime lab.
"As far as the actions of the shooter immediately prior to the event and any interaction that he may have had with law enforcement, we're still trying to flesh out those details now," Rojek said.
None of Crooks' shocked neighbors or high school classmates described him as violent or that he in any way signaled he was intent on harming Trump. Sunday morning, reporters and curious locals swarmed the leafy streets of the home where Crooks lived with his parents in Bethel Park, about 50 miles from the shooting scene.
Those who knew him described a quiet young man who often walked to work at a nearby nursing home. One classmate said he was bullied and often ate alone in high school.
Sunday morning, neighbor Cathy Caplan, 45, extended her morning walk about a quarter mile to glimpse what was happening outside Crooks’ home.“It came on the morning news and I was like ‘I know that street,’” said Caplan, who works for the local school district. "It feels like something out of a movie.”
Dietary aide turned deadly killer
Authorities say they are examining Crooks' phone, social media and online activity for motivation. They said he carried no identification and his body had to be identified via DNA and biometric confirmation.
Although no possible motive has yet been released, Crooks nevertheless embodies the achingly familiar profile of an American mass shooter: a young white man, isolated from peers and armed with a high-powered rifle. His attack was one of at least 59 shootings in the United States on Saturday, according to the Gun Violence Archive.
According to records and online posts of the ceremony, Crooks graduated from Bethel Park High School, about 42 miles from Butler County, on June 3, 2022. That same day, Trump met briefly with investigators at his Mar-a-Lago club in Florida as they examined whether he improperly took classified documents with him when he left the White House.
A classmate remembered Crooks as a frequent target of bullies. Kids picked on him for wearing camouflage to class and his quiet demeanor, Jason Kohler, 21, said. Crooks usually ate lunch alone, Kohler said.
Crooks worked as a dietary aide at the Bethel Park Skilled Nursing and Rehabilitation, less than a mile from his home. In a statement provided to USA TODAY on Sunday, Marcie Grimm, the facility's administrator, said she was "shocked and saddened to learn of his involvement."
Neighbor Dean Sierka, 52, has known Crooks and his parents for years. The families live a few doors apart on a winding suburban street, and Sierka’s daughter, who attended elementary, middle and high school with Crooks, remembers him as quiet and shy. Sierka said they saw Crooks at least once a week, often when he was walking to the nursing home from his parents' three-bedroom brick house.
"You wouldn’t have expected this," Sierka said. "The parents and the family are all really nice people."
"It's crazy," he added.
Secret Service role: Did they do enough?
Founded in 1865, the Secret Service is supposed to stop this kind of attack, and dozens of agents were present Saturday. As the former president and presumptive Republican presidential nominee, Trump's public appearances are managed by the Secret Service, which works with local law enforcement to develop security plans and crowd-management protocols.
In the days before the event, the agency's experts would have scouted the location, identified security vulnerabilities, and designed a perimeter to keep Trump and rally attendees safe. Congress and the Secret Service are now investigating how Crooks was able to get so close to the former president, and several witnesses reported seeing him in the area with the gun before Trump took the stage.
As the event doors opened at 1 p.m., the temperature was already pushing close to 90, and ticketed attendees oozed through metal detectors run by members of the Secret Service's uniformed division. Similar to airport security screenings, rallygoers emptied their pockets to prove they weren't carrying guns or other weapons.
Media reports indicate the Secret Service had in place, as usual, a counter-sniper team scanning the surrounding area for threats.
In an exclusive interview, former Secret Service Director Julia Pierson told USA TODAY that maintaining such a sniper security perimeter is part of the agency's responsibility for safeguarding protectees like Trump from harm. She said agents typically consider 1,000 yards to be the minimum safe distance for sniper attacks.
The Secret Service has confirmed that it is investigating how Crooks got so close to Trump, who took the stage shortly after 6 p.m. Officials say Crooks' rifle was legally obtained but have not yet released specifics.
Outside the venue at that time, Greg Smith says he tried desperately to get the attention of police. He told the BBC that he and his friends saw a man crawling along a roof overlooking the rally. Other witnesses said they also saw a man atop the American Glass Research building outside the official event security perimeter, well within the range of a 5.56 rifle bullet.
"We noticed the guy bear-crawling up the roof of the building beside us, 50 feet away from us," Smith told the BBC. "He had a rifle, we could clearly see him with a rifle."
Smith told the BBC that the Secret Service eventually saw him and his friends pointing at the man on the roof.
"I'm thinking to myself, why is Trump still speaking, why have they not pulled him off the stage?" Smith said. "Next thing you know, five shots rang out."
From his nearby deck, Trump supporter Pat English watched as the former president took the stage to Lee Greenwood's "God Bless the U.S.A.," and attendees raised their cell phones to record.
English had taken his grandson to see the rally earlier but left when it got too hot. From his deck, they listened as Trump began speaking at 6:05 p.m., backed by a crown of red-hatted MAGA supporters waving "fire Joe Biden" signs.
And then gunfire began.
Boom, boom, boom
"I heard a 'boom, boom, boom' and then screams,” English said Sunday. "I could see people running and the police run in."
Trump was saying the word "happened" as the first pop rang out. He reached up to grab his ear as two more shots echoed, and the crowd behind him – and Trump himself – ducked. Plainclothes Secret Service agents piled atop the president as a fusillade of shots rang out, apparently the Secret Service killing Crooks.
The crowd screamed, and the venue's sound system picked up the agents atop Trump planning to move the former president to safety. One yelled, "shooter's down. Let's move, let's move."
The agents then helped Trump back to his feet as they shielded him on all sides.
The sound system then picked up Trump's voice: "Wait, wait," he said, before turning to the audience and triumphantly raising his fist to yell "fight, fight" as the crowd cheered, blood streaming down his face.
By 6:14 p.m. Trump's motorcade was racing from the scene, and in a later statement, Trump's campaign said he was checked out at a local medical facility.
"I was shot with a bullet that pierced the upper part of my right ear," Trump said in a statement. "I knew immediately that something was wrong in that I heard a whizzing sound, shots, and immediately felt the bullet ripping through the skin. Much bleeding took place, so I realized then what was happening."
Firefighter 'hero' gunned down
Outside of the Butler Township Administration Office Sunday afternoon, Pennsylvania Gov. Josh Shapiro identified the rally attendee killed by Crooks as Corey Comperatore, a firefighter, father of two and longtime Trump supporter.
“Corey died a hero,” Shapiro said. “Corey dove on his family to protect them last night at this rally. Corey was the very best of us. May his memory be a blessing.”
Two other Pennsylvanians are still undergoing treatment for their injuries, Shapiro said.
Pennsylvania State Police identified two wounded attendees David Dutch, 57, of New Kensington, and James Copenhaver, 74, of Moon Township. Both are hospitalized and listed in stable condition. Shapiro said he spoke with the family of one victim and received a message from the other.
Biden spoke briefly with Trump on Saturday night, and the president condemned the assassination attempt as “sick.” He said there’s no place for political violence in the U.S. and called on Americans to unite together to condemn it.
But earlier in the week, Biden told campaign donors in a private phone call it was time to stop talking about his own disastrous presidential debate performance and start targeting Trump instead.
"I have one job and that's to beat Donald Trump," Biden said. "We're done talking about the (June 27) debate. It's time to put Trump in the bullseye."
Republicans across the country have used similar language to attack their opponents over the years, and political scientists say violent rhetoric used worldwide almost invariably leads to physical violence.
On Sunday, someone parked a truck-mounted electronic billboard at the gates to the Butler Farm Show grounds reading "Democrats attempted assassination," along with a picture of Trump clutching an American flag, his face overlaid with a bullseye crosshairs.
Authorities say they have not yet determined a motive for Crooks' attack. But in a statement, Trump declared the shooting an act of evil and thanked God for preventing the unthinkable.
"We will fear not, but instead remain resilient in our faith and defiant in the face of wickedness," Trump said.
And he said he'd be back on the campaign trail for the Republican National Convention in Milwaukee, which starts Monday.
"Based on yesterday’s terrible events, I was going to delay my trip to Wisconsin, and the Republican National Convention, by two days," Trump said on his Truth Social account Sunday, "but have just decided that I cannot allow a 'shooter,' or potential assassin, to force change to scheduling, or anything else."
Contributing: David Jackson, Aysha Bagchi, Christopher Cann, Bryce Buyakie, Emily Le Coz, Josh Meyer, USA TODAY Network
How the assassination attempt unfolded : Graphics, maps, audio analysis show what happened

An official website of the United States government
The .gov means it’s official. Federal government websites often end in .gov or .mil. Before sharing sensitive information, make sure you’re on a federal government site.
The site is secure. The https:// ensures that you are connecting to the official website and that any information you provide is encrypted and transmitted securely.
- Publications
- Account settings
Preview improvements coming to the PMC website in October 2024. Learn More or Try it out now .
- Advanced Search
- Journal List
- HHS Author Manuscripts

The Evolution of Nursing Research
Jacqueline m. stolley.
Trinity College of Nursing, Moline, Illinois
THE RESEARCH CULTURE in nursing has evolved in the last 150 years, beginning with Nightingale’s work in the mid-1850s and culminating in the creation of the National Institute of Nursing Research (NINR) at the National Institues of Health (NIH). This article highlights nursing’s efforts to facilitate the growth of the research culture by developing theory, establishing the importance of a research-based practice, advancing education, and providing avenues for dissemination of research. Similarities with the chiropractic profession are discussed, along with a commentary by Cheryl Hawk, D.C, Ph.D.
EVOLUTION OF NURSING RESEARCH
The development of a research culture in nursing in many ways parallels that of chiropractic, and by reviewing key aspects of the evolution of the science of nursing, there are lessons to be learned, and mistakes to be avoided. Nursing research has changed dramatically in the past 150 years, beginning with Florence Nightingale in the 19th century. Clearly, nursing research has not always had the influence and significance it holds today. In fact, for a number of years after Nightingale’s work, little is found in the literature concerning nursing research. This is perhaps due to the past perception of nursing as an apprenticeship in a task-oriented caring profession ( 1 ). Although research was conducted with respect to nursing education and administration in the first half of the 20th century, it was not until the 1950s that nursing research began the advancement that has been seen in the past three decades. This is due to many factors: an increase in the number of nurses with advanced academic preparation, the establishment of vehicles for dissemination of nursing research, federal funding and support for nursing research, and the upgrading of research skills in faculty and students. This article provides a brief review of the development of research in nursing, and along with it, the theory that has guided that process.
NURSING THEORY DEVELOPMENT
As with other practice professions, nursing requires a knowledge foundation that is based on theory and derived from systematic research. The first nursing theorist, Florence Nightingale, created detailed reports of both medical and nursing matters as chief nurse for the British in the Crimean War in the mid-1850s. Nightingale noted that “… apprehension, uncertainty, waiting, expectation, fear of surprise, do a patient more harm than any exertion” (p. 6) ( 2 ). As a result, Nightingale’s conceptualization of nursing included the need to have an understanding of the laws of nature, the prevention of disease, and the use of personal power. She viewed persons as both physical and spiritual beings, emphasizing the importance of the environment and the need to care for the patient, not the disease. With her emphasis on the environment, changes in nutrition, hydration, and sanitation resulted, and mortality rates dropped drastically during the Crimean War ( 3 ). In subsequent years, Nightingale developed “laws of nursing” that formed the basis for nursing science and guided nursing education in the United States from 1850 to the 1950s ( 4 ).
In the 1960s, nursing theory was used to guide teaching rather than research or practice. This was a natural outgrowth of nursing’s earlier focus on education and professional identity. Additionally, the National League for Nursing (the professional accrediting body) stipulated a conceptual framework for curriculum. Paradigmatic concepts integral to nursing were identified as person, environment, health , and nursing ( 5 – 7 ), and scientific energies were spent developing curriculum that corresponded to existing theories ( 7 ). At this point in time, nurse educators began to urge students to “care for the whole person” and textbooks underscored the importance of “holism” in nursing, with subtitles such as “The Biopsychosocial Approach.” Nurse authors acknowledged multiple causality in human illness, but all too often research, curricula, and textbooks reflected linear cause-and-effect models rather than multivariate approaches.
The 1950s and 1960s saw the development of theories explaining the art and science of nursing. Hildegard Peplau published Interpersonal Relations in Nursing ( 8 ) in 1952, based on her work as a psychiatric nurse. Other theories. included Levine’s Conservation Principles of Nursing ( 9 ) in, 1967; Roger’s Introduction to the Theoretical Basis of Nursing ( 10 ) in 1970, and The Science of Unitary Man ( 11 ) in 1980, followed by The Science of Unitary Human Beings, a Paradigm for Nursing ( 12 ) in 1983. Imogene King published A Theory for Nursing: Systems, Concepts, Process ( 13 ) in 1981, and Sister Calista Roy published her adaptation model ( 14 ) in 1980. These “grand” theories were complex and key concepts were hard to measure empirically. Thus it was difficult to test these early nursing theories through research. With the emphasis on clinical nursing research, the recent trend has been to develop and test midrange theories that describe patient problems and nursing practice.
NURSING RESEARCH DEVELOPMENT
In 1859, Nightingale used the battlefield hospitals of the Crimean War as her research laboratory, using an epidemiological process to describe the morbidity and mortality of sick and injured soldiers. Her pioneering epidemiological research and statistical methodology (documenting the relationship between the environment and health status of soldiers) was the hallmark of scientific investigation in nursing ( 4 , 15 ).
An historical review from 1900 to 1949 reveals that nursing research in the United States (see Table 1 ) was in its infancy, focusing on nursing education, nurses, nursing students, and ways to organize nurses’ work. As noted earlier, at this time, nursing theory was discussed solely as a means of developing and organizing educational curriculum. Early educators were unable to develop educational programs that both represented a nursing perspective and helped students focus on nursing concepts and problems rather than medical concepts and problems. In the first half of this century, groups were formed to answer such questions as: what is nursing, what do nurses do, and how unique is nursing from other health science disciplines? Professional debates raged as to whether nursing was merely a “poor stepsister” of medicine or whether it was part of the biological, natural, or physical sciences ( 4 ). Research during this period was essentially nonexistent in terms of nursing practice.
Nursing: Historical Developments in Nursing Theory and Research
Date | Development |
---|---|
1850 | Florence Nightingale: first nurse researcher; first nurse theorist |
Late 1800s–1900 | ‘Dark Ages’ |
Nursing viewed as an apprenticeship | |
Little focus on research-based practice | |
1900–1949 | (AJN) published |
Research focus: nursing education and administration | |
Questions: What is nursing? What do nurses do? How is nursing unique? | |
Debates: nursing vs. medicine as separate disciplines | |
1950s | Growing emphasis on need to identify a body of nursing knowledge |
journal established (1952) | |
American Nurses Foundation | |
Federal support for funding | |
1960s | Number of nurse researchers miniscule |
Early funding for nursing doctorate education — “hyphenated nurse” | |
Emphasis on nursing’s rightful place in the university setting | |
Nursing theory to guide practice: person, environment, health, and nursing | |
Beginning of “Grand” Theory development | |
1970s | Nursing educators aware of need to develop new knowledge |
American Nurses’ Association (ANA) Commission on Nursing Research | |
Journals published: , and | |
Increase in doctoral programs in nursing education | |
1980s | (CURN) project published |
National Center for Nursing for Nursing Research (NCNR) established within the | |
National Institutes of Health (NIH) | |
Journals published: and | |
Research focus: patients and patient behavior | |
Empirical modes of inquiry (logical positivitist) | |
Beginnings of qualitative research (phenomenology and ethnography | |
Better preparation for nurse researchers | |
Federal funding $16.2 million at NCNR | |
1990s | published |
Clinical Practice Guidelines (AHCPR) | |
journal published | |
NCNR renamed the National Institute for Nursing Research (NINR) in 1993 | |
Nursing doctoral programs in 65 institutions | |
Emphasis on clinical research | |
Research-based practice-research utilization, evidence-based practice | |
Multiple modes of inquiry | |
Emphasis on explanatory and predictive-level studies (vs. descriptive and exploratory) | |
published in 1998 | |
Federal funding $55 million at NINR | |
1995–1999 | Community-based nursing models |
NINR research priorities: effectiveness of nursing interventions in HIV/AIDS; cognitive impairment; living with chronic illness; biobehavioral factors related to immunocompetence | |
2000 | Chronic illnesses |
NINR research priorities: quality and cost effectiveness of care; health promotion and disease prevention; management of symptoms; adaptation to new technologies; health disparities; palliative care at the end of life budget: over $70 million |
It was not until the 1980s that nursing devoted a sizeable portion of its research effort to patients and patient behavior, an emphasis that emerged logically as nurses began to recognize the interplay between behavior and rehabilitation or recovery from illness. Historically, nurses searched for single causative agents when promoting health or preventing illness, even as they acknowledged the contributions of multiple other factors. Predominant modes of inquiry relied on early in the development of a culture of nursing research were empirical (logical positivist). Nurse researchers modeled themselves after colleagues in the basic and biomedical sciences, perhaps in an effort to seek scientific validation. Only during the 1980s and 1990s did nurses increasingly use qualitative research methods, such as phenomenology and ethnography, to explain complex human phenomena. Therefore, nurse researchers are just beginning to respond to the need to view human problems in less reductionistic terms when the research questions call for a holistic combination of quantitative and qualitative research methodologies ( 16 ). Over the past two decades, many nurses have pursued further education, consultation, or research to enhance their understanding and ability to respond constructively to patient behavior. For example, by the mid-1980s, there was a sizable increase in nursing studies of individuals and families experiencing developmental, environmental, or illness-generated crisis situations involving both acute and long-term stress responses ( 17 – 20 ).
During the 1990s, nursing practice underwent a clinical revolution in response to societal, medical, scientific, and technologic advances. Changes in nursing practice began to result from nursing research (e.g., research-based practice guidelines) as the efforts of individuals both in and outside of nursing (e.g., National Academy of Science, National Institute for Nursing Research, and major foundations) coalesced to stimulate and support clinical nursing research. Concurrently, there was a new surge of interest among nurses themselves in redefining the problems of their practice and delineating the gaps in knowledge underpinning their practice base. As noted earlier, the current decade has been marked by interest in multiple modes of inquiry (qualitative and quantitative) for a practice discipline which must address complex human phenomena. In the past, the type of research questions most often addressed through nursing research were of a descriptive or exploratory nature. However, nurse researchers are now going beyond “what is” and “how” questions and are addressing more explanatory or predictive-level questions using methodologically rigorous experimental and quasi-experimental designs as they redefine clinical problems and systematically address gaps in their knowledge base. After becoming established in the research arena, nursing researchers have expanded to incorporate and collaborate on interdisciplinary studies, health care systems and health services research, and taxonomies such as Nursing Intervention Classification (NIC) ( 21 ) and Nursing Outcomes Classification (NOC) ( 22 ). The taxonomies represent efforts to define what nurses do and outcomes sensitive to nursing interventions.
The culture of nursing research has now advanced to the point where consideration can be given not just to the conduct of research, but also to its application in practice. The conduct of research is not the end, but rather a means through which practice is improved by utilizing research findings. Research utilization is the process of conveying and applying research-based knowledge to impact or change existing practices in the health care system ( 23 ). The primary components of research utilization involve summarizing knowledge generated through research; imparting the research knowledge to nurses, other health professionals, policymakers, and consumers of health care; and accomplishing desired outcomes for patients, their families, and health care providers and agencies. Models for research utilization were developed in the 1970s, beginning with the Western Interstate Commission for Higher Education in Nursing (WCHEN) Regional Program for Nursing Development ( 24 ). Other models include the Conduct and Utilization of Research in Nursing (CURN) project ( 25 ), the Stetler/Marram model ( 26 ), the retrieval and application of research in nursing (RARIN) model ( 27 ) and the Iowa model of research in practice ( 28 ). The primary goal of research utilization programs is to make research findings an integral part of nursing practice, assuring research-based care delivery models. Research utilization is an excellent model for application of research findings to practice by advanced practice nurses.
An important trend is the use of research findings to serve as the basis for treatment decision making called evidence-based practice ( 29 ). Using this process, a question involving treatment is developed, and determination of the adequacy of current research is made. If the research base is adequate, it is synthesized, protocols are developed and applied, and evaluation is completed. Through these efforts, the nursing profession, in partnership with other professions, bridges the gap between research and practice to improve patient care.
EDUCATIONAL ADVANCEMENT
Early nursing education took place in hospital training programs (nursing diploma), modeled on Florence Nightingale’s work in the United Kingdom ( 30 ). In 1915, nursing’s educational accrediting body, the National League for Nursing (NLN) called for university-level education. Baccalaureate programs in nursing emerged in 1923 at Yale University and Western Reserve University, but the majority of nursing education took place in hospital-based diploma programs. In 1971, the first community college programs for nursing education opened, providing graduates with an associate degree in nursing. Today, entry into nursing practice takes place primarily in associate degree programs, with baccalaureate programs second. Gradually, diploma programs have decreased in number, and few exist today. Associate degree programs may introduce nursing students to research, but baccalaureate programs included nursing research in the upper division curriculum. From 1900 to the 1960s, most nursing leaders obtained their graduate-level preparation in schools of education ( 30 ). For many years, the Master’s degree was considered the terminal degree in nursing.
The number of nurses whose career was devoted to research was miniscule in the 1960s. Indeed, even by the 1970s only about 400 nurses in the United States held a doctoral degree ( 31 ). In 1955, the Nursing Research Grants and Fellowship Program of the Division of Nursing, United States Public Health Service (USPHS) was established. This program awarded grants for nursing research projects, nursing research fellowships, and nurse-scientist graduate training ( 32 ). Early funding was for nurses to obtain their doctorates in fields outside of nursing, because there were no nursing doctoral programs available. As a result, nurse-physiologists, nurse-anthropologists, and so forth emerged in the field. They were educated to conduct research, but often stayed in the field of their doctorate and did not apply their research efforts to nursing care problems.The emphasis during this period continued to be on establishing nursing’s rightful place in the academic setting of the university. As nursing became integrated into university life during the 1970s, nursing faculty became aware of their responsibility to develop new knowledge, and in many university-based schools of nursing, faculty members began to prepare both themselves and their students to become investigators ( 33 ).
Currently, three types of doctoral degrees in nursing are available. A Nursing Doctorate (ND), first established at Case Western Reserve University in 1979, was designed to be equivalent to the Doctor of Medicine degree, providing students preparation for the practice of generalized nursing and future leadership, but not for advanced practice. Professional doctorates, Doctor of Nursing Science (DNS, DNSc, DSN), emphasized advanced clinical, administrative, or policy-related practice and leadership. The Doctor of Nursing Science degree focuses on applied rather than basic research, and on applying and testing new knowledge in practice. Although the Doctor of Philosophy (PhD) was first available to nurses at Teachers College at Columbia University in the 1920s, interest in doctoral education was rekindled in the 1970s. The number of doctoral programs in nursing has increased from zero in the 1950s to over 65 institutions, three-fourths of which are academic doctorates (PhD) that prepare graduates for a lifetime of scholarship and research ( 34 ). More recently, nurses in academic settings have been encouraged to obtain postdoctoral research training with support available through both individual (F32) and institutional (T32) traineeships through the National Institutes of Health (NIH).
RESEARCH FUNDING AND SUPPORT
Several factors stimulated the growth of nursing research in the 1980s and 1990s. Perhaps the most important factor was the creation in 1986 of the National Center for Nursing Research (NCNR) in the United States Public Health Service (USPHS). The development of this Center resulted from intense political action by the American Nurses’ Association (ANA) ( 23 ). The primary aim of the NCNR was “the conduct, support, and dissemination of information regarding basic and clinical nursing research, training and other programs in patient care research” (p. 2) ( 35 ). Prior to the establishment of the NCNR, most of the federal funds supporting research were designated for medical studies that concentrated on the diagnosis and cure of disease. Thus, creation of the NCNR was a major achievement for nurse researchers. In 1993, the NCNR became the National Institute of Nursing Research (NINR), strengthening nursing’s position by giving the Center institute status within the NIH. This advance served to put nursing into the mainstream of research activities and on more equal status with scientists and other health professions. With the establishment of the Center and then the Institute, federal funding for nursing research has grown. In 1986, the NCNR had a budget of $16.2 million. In 1996, the budget for the NINR was about $55 million ( 1 ), more than a threefold increase over a decade. The NINR elected to foster five research priorities for 1995 through 1999: community-based nursing models, effectiveness of nursing interventions in HIV/AIDS, cognitive impairment, living with chronic illness, and biobehavioral factors related to immunocompetence ( 22 ).
The NINR’s strategic plan for the next millenium includes funding nursing research on chronic illnesses (e.g., improving adherence to chemotherapy, pain relief), quality and cost effectiveness of care, health promotion and disease prevention, management of symptoms (e.g., gender differences in response to therapeutics, managing the pain cycle), health disparities (e.g., cultural sensitivity), adaptation to new technologies (e.g., transplants), and palliative care at the end of life. Special allocations and Requests for Applications (RFA) have facilitated research in these target areas, although investigator-initiated research topics are funded if they are significant to nursing or patient care. The projected budget for NINR for the year 2000 is over $70 million, which is approximately distributed as follows: 73% for extramural research project grants; 8% for pre- and postdoctoral training; 3.5% for career development; 3.5% for Core Centers in specialized areas of research inquiry; 3% for the intramural program. Planning research for the next 5 years and into the next century is a welcome challenge for the NINR and the scientific community ( 36 ).
RESEARCH DISSEMINATION
Significant milestones in the development of nursing science began in the mid-1950s (see Table 1 ). From 1950 to 1959, there was growing emphasis on the need to identify a body of knowledge for the developing profession of nursing in order to justify its presence in post-World War II universities ( 37 ). Not only was the first journal of Nursing Research established in 1952; several textbooks related to nursing research were also published. Another critical step in the evolution of the culture of nursing science was the establishment of the American Nurses Foundation by the American Nurses Association specifically to promote nursing research. During the 1950s, regional research conferences were instituted for the first time, and federal support of nursing research began ( 4 , 33 , 37 ). All these elements were essential to the development of a science of nursing.
Several new nursing research journals, including Applied Nursing Research (ANR), were instituted in the late 1980s. ANR publishes research reports of special significance to nurse clinicians ( 1 ). Increasingly, clinical specialty (i.e., Heart and Lung, Journal of Gerontological Nursing ) journals are publishing data-based articles as well.
Another important event in the development and dissemination of nursing theory and research was the creation of the Annual Review of Nursing Research in 1983. This publication includes critical analyses of research pertinent to nursing and health, including nursing practice, nursing care delivery, nursing education, and the nursing profession. Chapters systematically assess knowledge development in nursing, encourage the use of research findings in practice, and provide direction for future research ( 22 ). More recently, scholars have joined to create the Encyclopedia of Nursing Research ( 38 ), a publication that provides a comprehensive overview of research studies, the history of nursing research, and the evolution of theory development in nursing.
The next century challenges nursing research with critical imperatives for improving health care. Changes in our nation’s population and their needs and expectations will impact the direction of nursing research. Consumers are becoming more involved in managing their own health care, and practitioners are continually adjusting to new technologies as well as innovative health care systems. The broad spectrum of nursing research encompasses both clinical and basic investigations with the patient as the central focus. Nursing must concentrate on making certain that our valuable scientific findings are incorporated into practice and focus on developing the next generation of nurse researchers ( 36 ).
The research culture in chiropractic is similar to where nursing research was in its early years. To move chiropractic research forward will require many of the same changes that occurred in nursing, such as educational advancement, collaboration in academic settings, federal acknowledgement and support, and development of more avenues for research dissemination to practitioners. Just as nursing had to overcome significant barriers such as attitudes and low educational and professional status, so, too, will the chiropractic profession have to strive to develop a research tradition in order to integrate research as part of its practice culture.
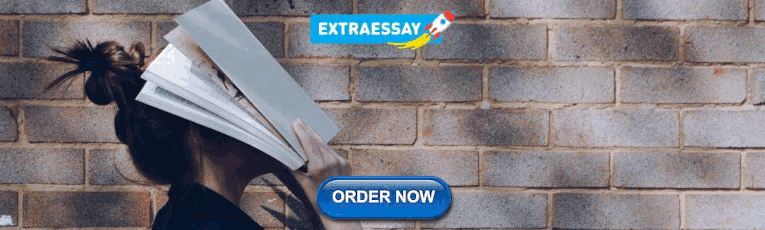
IMAGES
VIDEO
COMMENTS
Many researchers have commended the self-care deficit nursing theory (SCDNT) developed by Orem as a means of improving patients' health outcomes through nurses' contributions. However, experimental research has investigated specific aspects of SCDNT, such as self-care agency and self-care requisites, rather than how the construct is practiced ...
Abstract. Background: Nursing theory-guided practice helps improve the quality of nursing care because it allows nurses to articulate what they do for patients and why they do it. However, the usefulness of nursing theory-guided practice has been questioned and more emphasis has been placed on evidence-based nursing and traditional practice.
Nursing is considered a young science, which indicates that nursing theories are still in the process of being developed. 6 The present nursing theories were selected to represent useful approaches to expand this discussion. We believe that placing the present nursing situation in the context of nursing practice and research findings is a ...
Eighteen of the PhD theses were based on theoretical approaches from philosophy, ethics, pedagogy, medicine or biology as a primary perspective. Nursing theories, in their conventional definition, have a limited presence in the theses examined. Keywords: doctoral students, nurses, nursing, PhD, research, The University of Edinburgh.
Many researchers have recommended the self-care deficit nursing theory (SCDNT) developed by Orem (1995) to improve patients' health outcomes in terms of the nurses' contributions. Experimental studies on this theory include assessing the value of SCDNT in reducing fatigue in patients with multiple sclerosis (Afrasiabifar et al., 2016) and an evaluation of SCDNT-based care in improving the ...
The Neuman Systems Model was developed to create a structure to depict nursing clients as complex systems, in constant energy exchange with their environments, comprised of multiple interrelated parts. It has been widely used in practice, education, research, and administration. An analysis of the theory, based upon Meleis' methods, was ...
Abstract. This replication and critique addresses ongoing development and use of middle range theory since considering this body of nursing knowledge 18 years ago. Middle range theory is appreciated as essential to the structure of nursing knowledge. Nine middle range theories that demonstrate ongoing use by the theory authors are analyzed ...
Research article. First published online December 3, 2019. Patient-Centered Medical (Health) Home: Nursing Theory-Guided Policy Perspectives ... If policies are not formulated with the use of nursing theories and models, then nurses are practicing from views and policies from other health disciplines and in a way that is "generalized" for ...
Parse categorized nursing theories into totality and simultaneity paradigms, with the former viewing the person as a combination of biological, psychological, social and spiritual features, in constant interaction with the environment to accomplish goals and maintain balance: The goals of nursing in the totality paradigm focus on health promotion, care and cure of the sick, and prevention of ...
Detours and dead ends for theory development involved metatheory debates and specific events. Challenges identified relate to rapid changes in society, healthcare, and science. A pathway for the future is presented in a figure with its description of the structure of nursing knowledge. Discussion: The potential of this structure for developing ...
Often the most difficult part of a research study is preparing the proposal based around a theoretical or philosophical framework. Graduate students '…express confusion, a lack of knowledge, and frustration with the challenge of choosing a theoretical framework and understanding how to apply it'.1 However, the importance in understanding and applying a theoretical framework in research ...
A nurse educator looks back at the influence of nursing theorists on today's evidence-based practice. Figure. TODAY'S CLINICAL setting is filled with discussion and implementation of evidence-based practice, but this wasn't always the case. 1 Theorists have elevated the science of nursing to its modern prominence with decades of research.
The application of nursing scientific knowledge in practice is possible in the use of nursing theories and models in care ... Who uses nursing theory? A univariate descriptive analysis of five years' research articles. Scandinavian Journal of Caring Sciences, 25 (2), 404-409. 10.1111/j.1471-6712.2010.00835.x.
Nursing theories are the basic. foundations that help nurses in terms of determining the. needs and achieve goals to advance nursing care and its. delivery (Colley, 2003). Furthermore, its ...
Greater rigor and precision in theory development and testing is much needed. Science advances because of and sometimes in spite of our efforts. Theory should help that advancement, providing explanations of phenomena and their relationships. Using theory in research allows us to refine the definitions and scope of the discipline of nursing.
3 min read • July, 05 2023. Nursing theories provide a foundation for clinical decision-making. These theoretical models in nursing shape nursing research and create conceptual blueprints, ultimately determining the how and why that drive nurse-patient interactions. Nurse researchers and scholars naturally develop these theories with the ...
Theories are important in disciplines. A discipline is a "realm, sphere, or domain. It is a branch of knowledge ordered through the theories and methods evolving from more than one worldview of the phenomenon of concern" (Parse, 1997, p. 74).Theories serve to "describe, explain, and predict phenomena in a discipline" (King, 1997, p. 23).). Scholars and scientists utilize concepts ...
Nursing theories can help guide research and informing evidence-based practice. Provide a common language and terminology for nurses to use in communication and practice. Serves as a basis for the development of nursing education and training programs. In many cases, nursing theories guide knowledge development and directs education, research ...
Introduction. Theories can be useful to nurse-researchers as guides for conducting research (Bartholomew & Mullen, 2011; Rodgers, 2005).A theory offers a set of concepts and propositions that can be applied consistently and examined systematically across studies of clinical problems (Meleis, 2012).Admittedly, not all research should be theory-guided; some research is conducted to generate ...
Deductive reasoning. In their critique of induction in qualitative research, Bergdahl and Berterö argue that qualitative researchers should be more open to a deductive approach.4 At a superficial level, deductive reasoning can be viewed as the opposite to induction, with specific conclusions drawn from general theories. So, with inductive reasoning often conceptualised as 'bottom-up ...
Background: The effective management of Healthcare-Associated Infections (HAIs) relies on the implementation of good practice across the entire multidisciplinary team. The organizational context and the role of head nurses influence the team's performance and behavior. Understanding how decision-making processes influence healthcare professionals' behavior in the management of HAIs could ...
Results: Of 2857 articles published in the seven journals from 2002 to, and including, 2006, 2184 (76%) were research articles. Of the 837 (38%) authors who used theories, 460 (55%) used nursing theories, 377 (45%) used other theories: 776 (93%) of those who used theory integrated it into their studies, including qualitative studies, while 51 ...
Data charting. We developed a data extraction form following the methodology guidance for scoping review provided by the Joanna Briggs Institute and made additional adjustments to the form after piloting five articles [].The items on the form included author, year, country, target groups, research topic, research and data analysis methods, model (a model for implementing PHE), findings, and ...
This descriptive, exploratory study involved a review of 747 doctoral papers to determine whether nursing students are using nursing or non-nursing theory to guide their research. The findings revealed that although 86.9% of doctoral students used theory, just 31.7% used nursing-specific theory to guide their dissertation study or capstone project.
Spoiler alert! We're discussing important plot points and the ending of this summer's tornado-chasing thriller "Twisters" (in theaters now), so blow on down the road if you haven't seen it yet ...
1. Introduction. Nursing in hypertension care has been shown to comprise counselling about lifestyle changes, blood pressure measurement, and being a translator for the physician [].A more detailed description of the nursing interventions is presented by Hong [].When a nurse is a member of a team with other healthcare professionals in the care of the hypertensive patient, a reduction in blood ...
The research will be presented at the International Conference on Machine Learning. Human generalization. As we interact with other people, we form beliefs about what we think they do and do not know. For instance, if your friend is finicky about correcting people's grammar, you might generalize and think they would also excel at sentence ...
BUTLER, Pa. - Donald Trump and would-be assassin Thomas Crooks started on their violent collision course long before the former president's political rally ended in gunshots and death. Crooks ...
Go to: Abstract. THE RESEARCH CULTURE in nursing has evolved in the last 150 years, beginning with Nightingale's work in the mid-1850s and culminating in the creation of the National Institute of Nursing Research (NINR) at the National Institues of Health (NIH). This article highlights nursing's efforts to facilitate the growth of the ...