
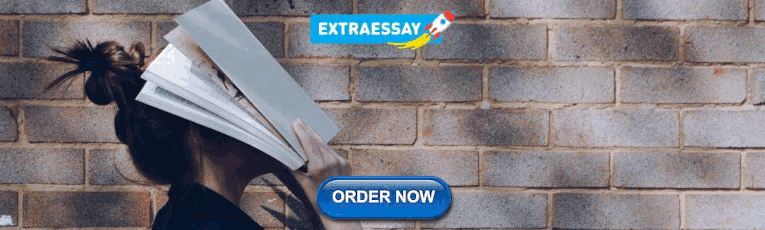
Use of Market Research Panels in Transit (2013)
Chapter: chapter two - literature review.
Below is the uncorrected machine-read text of this chapter, intended to provide our own search engines and external engines with highly rich, chapter-representative searchable text of each book. Because it is UNCORRECTED material, please consider the following text as a useful but insufficient proxy for the authoritative book pages.
8 accommodate new technologies, such as telephone sampling and computerization. Since Frankel and Frankel wrote their article in 1987, the Internet has become another technologi- cal advance, requiring innovations in sampling to accommo- date the technology. The Brick article notes that 10 years ago, no generally accepted method of sampling from the Internet had been established, and that as of the writing of the article in 2011, it was still the case. As developed by Neyman in 1934, probability survey sam- pling became the basis for virtually all sampling theory, with a very specific framework for making inferences. The frame- work assumes that all units in the population can be identified, that every unit has a probability of being selected, and that the probability can be computed for each unit. Once the population is selected, the framework assumes that all char- acteristics can be accurately measured. Non-response fac- tors and the inability to include portions of the population (coverage error) violate the pure assumptions of probability sampling. A variety of techniques has been developed to adjust for non-response and coverage error, such as model- based and design-based sampling methods. Technological advances that have changed sampling meth- ods include: the introduction of telephone surveying, which eventually replaced face-to-face interviews as the primary mode of household surveying; the shift from landline tele- phones to cell phones; and the advent of the Internet. Each of these not only affected methods of sampling, it is intertwined with the others, with changes in one leading to new develop- ments in the others. New Concerns with Traditional Quantitative Research The science of traditional quantitative market research rests on two fundamental assumptions: (1) the people who are surveyed approximate a random probability sample; and (2) the people who are surveyed are able and willing to answer the questions they are asked (Poynter 2010). In addition to these concerns with the theoretical underpinnings of market research, operational concerns are also putting pressure on traditional methodologies. Random Probability Sample The random probability sample is an essential ingredient of traditional market research. Without it, quantitative market This literature review consists of four sections: ⢠The first section provides a brief history of survey sam- pling and the theoretical basis for market research anal- ysis, providing context for what became the standard procedures and expectations of market research. This is followed by an overview of some of the issues facing market research today, and how they are impacting the statistical underpinning of market analysis. ⢠The second section introduces traditional panel surveys and panel survey techniques. A summary of a typical tra- ditional case example, the Puget Sound Transportation Panel Survey, is provided in Appendix A. ⢠The third section introduces relatively newer concepts of online panel research, with definitions particular to online panel surveys and techniques, issues with online panel research, and the special concerns of market research in the public sector. ⢠The fourth section circles back to the concerns raised in the first section, and looks at what lies ahead for the market research industry on these issues. MARKET RESEARCH CONTEXT A Brief History of Survey Sampling In a 2011 special edition of the Public Opinion Quarterly, Brick provides an article on the future of survey sampling (Brick 2011). For the purposes of the article, survey sampling is defined as the methodology for identifying a set of obser- vations from a population and making inferences about the population from those observations. Prior to 1934, full enu- meration was considered necessary to understanding char- acteristics of a population; everyone needed to be contacted. Neyman, in his 1934 article âOn the Two Different Aspects of the Representative Method: The Method of Stratified Sample and the Method of Purposive Selection,â planted the seeds that resulted in the overthrow of full enumeration and established the paradigm of survey sampling. The move to telephone surveying in the mid-20th century was another significant change in survey sampling methods. The pressures for timely and cost-efficient estimates were stimulants for change then, and are even more relevant today. The article by Brick draws from a 1987 article by Frankel and Frankel, âFifty Years of Survey Sampling in the United States.â In the 1987 article, sampling is described as having two phases: (1) the establishment of the basic methods of prob- ability sampling; and (2) innovations in the basic methods to chapter two LITERATURE REVIEW
9 research studies and analysis cannot be conducted. It is this underpinning of sampling theory that allows the calculation of sampling and expressing confidence in the results, such as results being ±3% with a confidence interval of 95%. As tele- phones became standard in every household, random-digit- dial techniques for landline telephones became the foundation of probabilistic sampling, with a solid theoretical basis. Results for a survey conducted between January and June 2011 by the National Center for Health Statistics found that 31.6% of American homes had only cell phones, and that an additional 16.4% of homes received all or almost all calls on wireless telephones despite also having a landline telephone (Blumbery 2011). Expert disagree, but it has been suggested that if more than 30% of the population has a 0% chance of being selected, then a random probabilistic sample cannot be selected (Brick 2011). The implication is profoundâthat with the incidence of landline phones declining, random-digit-dial telephone survey- ing, the mainstay of traditional quantitative market research, no longer provides a probabilistic sample. Cell phones are considered unsuitable for random-digit-dialing, for a variety of reasons, including the possibility of respondents having more than one cell phone resulting in duplication within the sample; respondent resistance; and legislation that prohibits the practice. Online recruitment is fast and economical, but does not provide a probabilistic sample, as is discussed later in this chapter. Willingness and Ability to Answer Research Questions Market researchers started out assuming that people could answer direct questions about their attitudes and behavior. Early on, it became clear that these questions were difficult to answer, so psychometrics and marketing science method- ologies were developed to facilitate responses and analysis of results. More recently even these techniques have been chal- lenged, as the industry realizes that respondents are unreliable witnesses about themselves. Operational Issues Other problems with the traditional market research process are operational. It is perceived as slow and costly, and increas- ingly, organizations are relying on techniques that may be providing quick results at the expense of quality. The cost of traditional survey research often is driven up by the decline in use of landline telephones, making it more difficult to obtain a traditional random probabilistic sample. In addition, more people have answering machines to screen calls or otherwise refuse to participate, again making it difficult to achieve a suf- ficient sample without additional time and expense. If cost is arguably the single most important factor in the search for new survey techniques, the Internet offers a potential solution, even though it doesnât provide a probabilistic sample. This shift from probability sampling to non-probability sam- pling is a paradigm change of the magnitude of the shift from enumeration to probability sampling theory in 1934âwhich, Brick notes in his article, was spurred by the cost of enumera- tion. Today researchers find themselves in a similar situation, driven by rising costs away from probabilistic sampling toward non-probabilistic sampling. The issues raised here may fundamentally change the way all market research is conducted. How the market research indus- try is responding and what may lie ahead is discussed in the last section of this chapter, âThe Future of Market Research.â TRADITIONAL MARKET RESEARCH PANELS Panel surveys have been conducted for many years, and have been used in the transportation industry for topics such as travel behavior changes and tracking customer satisfaction. The concepts discussed in this section are applicable to both traditional and online panel research. Definition of a Traditional Panel The meaning of a market research panel depends on the con- text, industry, and time period in which the term is being used. The AMA acknowledges this with the following distinctions: ⢠True panel: A sample of respondents who are measured repeatedly over time with respect to the same variables. ⢠Omnibus panel: A sample of respondents who are mea sured repeatedly over time but on variables that change from measurement to measurement [http:// www.marketing power.com/_layouts/Dictionary. aspx?dLetter=P (accessed Mar. 11, 2012)]. Traditional Panel Survey Techniques Traditional panel members were recruited through probabilis- tic sampling techniques so that survey results could be extra p- olated to the general population. Developing and maintaining a panel was an expensive proposition, made more difficult by the challenge of keeping track of people and households as they moved and changed phone numbers. Panel survey research was typically used to determine individual travel behavior changes over time, such as to understand the relation- ship between changes in household characteristics and choice of travel mode. Another use was for âbefore and afterâ studies to measure impacts of a change in policy or service; for exam- ple, adding a new light rail line or carpool lanes. These studies were often conducted by a MPO for the purpose of developing regional travel demand and forecasting models. Panels were rarely set up and maintained for the purpose of ad hoc, on-call market research (omnibus panels). Panel data collection is described as âa survey of a group of preselected respondents who agree to be panel members on a continuous basis for a given period of time and provide demographic data, allowing selection of special groups and
10 cally, each wave consists of the same core questions along with some new questions. In a travel behavior survey, the panel provides information on how the travel behavior of each participant evolves in response to changes in the travel environment, household background, or other factors. Rotating or revolving panel surveys are a combination of repeated and cross-sectional designs, in that they collect panel data on the same sample for a specified number of waves, after which portions of the panel are dropped and replaced with comparable members. The strength of this design is its abil- ity to allow for both short-term panel member analysis and long-term analysis of population and subgroup change. Like repeated cross-sectional designs, rotating panels periodically draw new members from the current population, obtaining similar measurements on them. Benefits of Panel Designs The most important benefit of a panel survey is that it directly measures changes at the individual level and can provide repeated measurements over time. This rich source of infor- mation on personal and household behavior is essential for determining causal relationships between travel behavior and the factors that influence personal travel decisions and developing predictive models for personal travel behavior. This same benefit applies to the ability to measure and under- stand trends in population behavior. Panel studies can be especially useful for before-and-after surveys that measure the impacts of transportation policy and service changes on travel behavior, rider attitudes, and safety. For example, a before-and-after study of the implementation of a new rail line (replacing existing bus service) shows that a shift in mode split occurred after the implementation of the new line. Results using a cross-sectional survey showed a shift from auto to train after opening of the rail line suggesting overall growth in transit use shifting car drivers to rail riders. A panel study measuring individual specific changes captured a shift from bus to car in addition to the shift from car to rail. This finding fundamentally changed the implications of the cross- sectional study: the new service attracted former car drivers, but also shifted former bus riders into cars. Additional benefits of the panel approach include statisti- cal efficiency (it requires a smaller sample size); lower cost (it requires fewer surveys); and speed (easy access to the panel allows faster survey implementation than when a fresh sample must be obtained). Limitations with Panel Designs Three primary limitations of panel surveys are identified: panel attrition, time-in-sample effects, and seam effects. 1. Panel attrition refers to panel member non-response in later waves of data collection. The Puget Sound Trans- portation Panel conducted its first wave of surveys in permitting the use of surveys to monitor responses over timeâ (Elmore-Yalch 1998). This maximizes the use of a sample in that the sampling need be done only once, after which the panel is accessible for future research efforts. Panel member attrition and replacement is an element of maintaining the panel, and is discussed elsewhere in this chapter. The remainder of this section is a summary of An Introduc- tion to Panel Surveys in Transportation Studies (Tourangeau et al. 1997), which provides a solid overview of the basics of traditional panel survey research, especially as applied to travel behavior studies. The report has a four-fold purpose for the development and implementation of travel behavior studies: (1) to highlight the differences between cross-sectional and panel surveys; (2) to discuss the limitations of both cross-sectional and panel sur- veys; (3) to identify situations where panel surveys are the preferred method; and (4) to provide guidelines for design- ing a panel survey and maintaining the panel. A panel survey approach is recommended when the purpose of the survey is to develop travel demand models and forecast future demand; to measure and understand trends in behavior; to assess the impact of a change in transportation policies or services; or to collect timely information on emerging travel issues. Definition of Cross-Sectional and Panel Designs There are two broad types of surveys, cross-sectional and panel surveys. A cross-sectional survey uses a fresh sample each time, whereas a panel survey samples the same persons (or households) over time. In addition, the questions may be the same or change with each survey. This creates four basic approaches to travel behavior surveys. One-time cross-sectional surveys provide a âsnapshotâ of travel behavior at a particular point in time, and show how behavior differs among members of the population, but pro- vide no direct information on how it changes over time. This type of survey makes no attempt to replicate conditions or questions from previous studies, and as a result is not well suited for assessing trends in population behavior. Repeated cross-sectional surveys measure travel behav- ior by repeating the same survey on two or more occasions. In addition to repeating the questions, the sampling is conducted in a similar manner to allow comparisons between or among separate survey efforts. Repeated cross-sectional surveys are sometimes referred to as a âlongitudinal survey designâ because they measure variations in the population over time. A more restrictive definition of a longitudinal survey design is where survey questions are repeated with the same sample over time. Longitudinal panel designs collect information on the same set of variables from the same sample members at two or more points in time. Each time the panel is surveyed, it provides what is called a âwaveâ of data collection. Typi-
11 1989. The fourth round of surveying in 1993 had a par- ticipation rate from the original panel member of about 55%, meaning 45% of the panel had left and needed to be replaced. 2. The time-in-sample effect refers to reporting errors or bias as a result of participants remaining in the panel over time. This is also called condition, rotation bias, or panel fatigue; and generally refers to respondents reporting fewer trips or fewer purchases in later rounds of a panel survey than in earlier ones. 3. Seam effects are another type of reporting error and refer to reporting changes at the beginning or ending of the interval between rounds rather than in other times covered by the interview. Design Issues in Conducting a Panel Survey There are four design issues that need to be considered in conducting a panel survey: definition of the sample unit; the number and spacing of rounds; method of data collection; and sample size. 1. Most traditional travel surveys conducted by MPOs use households as the sampling unit; however, sampling individuals is another option. When a household is the sampling unit, the panel survey sample can become com- plicated as household members are born, die, divorce, or mature and move out. For travel surveys, the report sug- gests using the household as the sampling unit, follow- ing initial respondents to new households, and adding any additional household members to the panel. 2. The number and spacing of survey rounds depends on factors such as the rate of changes in travel behavior and the need for up-to-date information. If changes in travel behavior are the result of external factors, such as rap- idly increasing gas prices, or if administrative reporting requires monthly or quarterly updates, this may shorten the intervals between survey waves. Panel travel surveys are collected at six-month or annual intervals, balancing the potential for respondent burden with the desire for regular data collection. The report recommends annual data collection for travel behavior studies. 3. Data collection methods differ in terms of cost, cover- age of the population, response rates, and data quality (inconsistent or missing data). In-person data collec- tion is typically the most expensive, but produces the highest percentage of coverage, highest response rates, and potentially most accurate data, as the interviewer can assist the respondent. Telephone data collection tends to be the next most expensive methodology, and eliminates the population without a telephone. This used to be limited since almost all households had a landline phone, but since the report was written the per- centage of mobile phone-only households has grown significantly. Data collection by mail is the cheapest of the three traditional modes, but has the lowest response rates and poorest data quality. [Since the report was written, Internet surveying has become another inex- pensive alternative method of data collection. Online surveying is covered in other portions of the literature review.] The report recommends using the telephone for data collection in the first wave of a travel behavior panel study and considering less expensive methods for successive waves, if necessary. 4. Selecting the sample size requires specifying the desired level of precision for the survey estimates. The preci- sion level is determined by the requirements for ana- lyzing the goals and objectives of the survey, typically rates of change in travel behavior at the household or sub-regional level. After the level of precision is deter- mined, traditional statistical formulas can be applied to determine the sample size, which is then adjusted for anticipated non-response, attrition, and eligibility rates. Issues with Maintaining the Panel The report points up three issues that need to be considered in terms of maintaining a panel: freshening the sample, main- taining high response rates across waves, and modifying the questionnaires across rounds. 1. âFreshening the sampleâ is the process of adding new panel members over time to ensure that the sample accurately reflects changes in the population from newly formed households or those who have recently moved to the study area. The longer the panel is con- tinued, the less likely it is to represent the study area. The report suggests that, if a panel continues for more than five years or there is significant in-migration to the study area, a supplemental sample be implemented. Another reason for freshening the sample is to off- set attrition, recruiting new panel members compa- rable to those who drop out and thereby maintaining the panel make-up and sample size for the duration of the panel effort. The report suggests that the initial sample size be large enough to accommodate antici- pated attrition in later waves, and that steps are taken to minimize attrition. Replacement of panel members should only be done as a last resort. 2. There are three techniques for maintaining high response rates: tracing people or households who move; main- taining contact with panel members between rounds; and providing incentives for participation. Methods of tracing panel members who move include mailing a let- ter several months in advance of the next wave request- ing updated contact information; and asking the post office to provide new addresses rather than forwarding the mail, to ensure that the contact files get updated. If new contact information is not provided, researchers may attempt a manual search through existing databases. The report suggests that a protocol be developed at the outset of the survey effort to track respondents between waves and reduce attrition. Another way of reducing attrition is to maintain respondent interest and contact information between
12 waves by sending postcards, holiday greetings, and sur- vey results. Incentives such as small amounts of cash can also be helpful. Cross-sectional surveys have shown that a small prepaid incentive (for example, a $2 bill) is effective in increasing participation rates and reducing attrition. Unfortunately, there was limited research at the time as to the effect of incentives on panel surveys over time. It is noted that non-respondents in one wave may still participate in the next, so that only those who refuse to respond to more than one round of the study would be dropped from the panel. 3. A defining element of a traditional panel survey is the ability to administer the same questions to panel mem- bers over time, which is what provides the direct mea- surement of change that is so valuable to travel behavior studies. Two situations arise that may make it neces- sary to modify the questionnaire across waves. First, a new issue may arise that can be advantageously posed to the panel. This then becomes a cross-sectional sur- vey, where the data are collected once. If the question is repeated in later waves, it becomes part of the panel effort. Although this is easy, fast, and less expensive than conducting a separate study, it can add to respon- dent fatigue by making the questionnaire longer. For this reason, it is suggested that new questions be kept to a minimum. The second reason for changing a question that there is a problem with the question itself (e.g., it is poorly worded, yields unreliable results, or becomes irrelevant). In this instance, it is important to revise the question as soon as possible. The report recommends that a calibration study be done to determine the effect of any core changes. Weighting the Panel Data The final section of the report deals with how to weight panel survey data. Weighting is done to account for differences in the probability of being selected, to compensate for differences in response rates across subgroups, and to adjust for random or systematic departures from the composition of the popula- tion. Weighting is done at two points: after the initial wave, following the procedures for standard cross-sectional surveys; and then after each wave to account for changes in the panel membership. Although weighting is fairly straightforward for the first wave, subsequent waves can be complicated if the sampling unit is a household, as is typical of travel behav- ior panel studies. Elements that must be taken into account are how to treat households who add or lose members over the course of the panel; and how to define a ârespondingâ or ânon-respondingâ household, for example, whether all survey waves are completed by all household members or only cer- tain household members. It is sometimes necessary to gener- ate different weights for different survey analyses. Detailed guidelines for developing panel survey weights are provided in the report appendices. ONLINE MARKET RESEARCH PANELS This section will discuss the types of online panels, sampling strategies, and issues and concerns with using the Internet for market research purposes. The current literature reviewed in this synthesis discusses sampling and recruitment for online panels using the Internet, e-mail, or other new technologies, such as quick response (QR) codes scanned by a smart phone. Multi-frame sampling, where a mix of sampling techniques is used for developing the panel, poses additional issues which are only now being explored and disseminated within the market research industry. Because this is an emerging area of research, this literature review does not include multi-frame sampling. Types of Online Panels Three types of panels are discussed by Poynter in his 2010 book, The Handbook of Online and Social Media Research: Tools and Techniques for Market Researchers. The first is a traditional panel, typically called a client panel or in-house panel, developed to meet specific criteria and recruited either in-house by the agency or through the assistance of a vendor. The panel can be recruited through a variety of techniques, including telephone; in-person intercepts (on a vehicle, or on the street); through existing agency customer databases; or online, through the agency website or pop-up invitations to join the panel. The critical elements of this type of panel are the definition and control that is exercised by the agency, and the intention for the agency to maintain the panel over time. An online access panel, also referred to as an access panel or online panel, is developed by independent market research firms and can provide samples for most markets that have a significant volume of research activity. The researcher provides the panel company with the desired sample speci- fication, and then either the researcher provides a link to the online survey, or the panel company scripts and hosts the online survey. The third type of panel survey is an online research com- munity, also known as a market research online community or MROC, which combines attributes of panel research with elements of a social media community. Although it is sometimes grouped with social media techniques, the online research community has been included here because it meets the definition of âa group of persons selected for the purpose of collecting data for the analysis of some aspect of the group or area.â In-House Panels As the name implies, in-house panels are owned by the research department of the agency, and are not purchased from a vendorâs existing panel. The in-house panel is used
13 for market research, not public relations, marketing or sales; and panel members are aware that they will be contacted for research, insight, and advice. The primary advantages of in-house panels are cost savings, speed of feedback, and control over the panel. Disadvantages include the workload required to manage a panel and that the possibility that panel members may become sensitized to the research approaches. In-house panels can be conducted simply from a list of people and an off-the-shelf survey program using e-mail and a way to unsubscribe from the panel. For small-budget projects or a low-key exploratory concept, a simple approach may be the most appropriate. More sophisticated panel management may require methods to prevent people from signing up mul- tiple times, the ability to draw sub-samples, protocols for han- dling and managing incentives, panel member replacement strategies, quotas on survey responses, online focus groups or bulletin board groups, and rules for creating an online panel community. The more sophisticated the approach, the more advanta- geous it is to contract with a vendor to run the panel. Using internal staff may make the research more responsive to man- agement needs while saving in consultant fees. A vendor, how- ever, can handle more work without overburdening agency staff, using employees familiar with the latest thinking and best practices. These different strengths often lead to a strong partnership between the vendor and staff. Traditionally, panel research was done with standard ques- tionnaires, implemented by means of mail or telephone. New developments in technology and the Internet have made it easy to expand the activities of a panel even further, creating online focus groups, photo projects where panel members take pictures with their cell phones and upload them to an agency website, brainstorming through collaborative systems such as âwikiâ sites, and quick âfun pollsâ that encourage participa- tion, generate panel engagement, and provide almost instant answers to questions of the moment. Tips for using an in-house panel include: 1. Manage the expectations of panel members by letting them know at the outset how many surveys/activities they should expect. 2. Let panel members know you value their participation and that they are making a difference. 3. Recognize that panels will usually be skewed toward members who are knowledgeable about the product or service, and that they may not represent the opinion of the general public. 4. Complement conventional incentives (such as cash) with intrinsic rewards, such as information about upcoming events or new products before it hits the general market. Online Access Panels Online access panels have fundamentally changed how mar- ket research is conducted. An online access panel âis a col- lection of potential respondents who have signed up with a vendor which provides people for market research surveys.â These respondents are aware that they are joining a market research panel, and that they will be receiving invitations to online surveys. The vendor keeps some information on the panel members so that it can draw samples, if requested, but does not share this information with the client. Panel mainte- nance, including the provision of incentives, is the vendorâs responsibility. In selecting a panel vendor, six factors need to be considered: 1. Does the vendor provide only the sample, or will it also host surveys? If so, can the brand image on the survey maintain the agencyâs brand, or does it become folded into the vendorâs survey branding? 2. What is the quality of the panel? Not all panels are created equal, and the results can vary based on the panel used. ESOMAR formulated â26 Questionsâ (later, â28 Questionsâ) for agencies to ask vendors in order to understand their procedures and the potential quality of the survey results. The questions can be found at: http://www.esomar.org/index.php/ 26-questions.html. 3. In looking at vendor costs, caution must be exercised to ensure that price quotes are on similar services so they can be correctly compared. 4. Make sure that the vendor has the capacity to complete the study, including any potential future waves of the study. It is common practice for panel survey vendors to outsource a portion of or even the entire project to another firm if they do not have the resources to com- plete it as scheduled. Outsourcing to another panel sur- vey firm can result in double-sampling people who are members of both panels. More importantly, because dif- ferent panels often have varying results, this can lead to confusion as to whether an apparent change is real or a reflection of the panel used. 5. The more data a vendor has on its panel members, the more closely a survey can be targeted to the appropriate respondents. This results in fewer respondents being screened out and a shorter survey with fewer necessary questions. 6. As with any service, it is helpful to have a supportive vendor who is willing to stay late if needed, help clean up errors, and respond quickly to issues and concerns. After selecting a vendor, it is essential to ensure a good working relationship. This can be facilitated by: ⢠Clarifying the quote for the project to make sure it includes all work needed;
14 ⢠Booking the fielding time for the job as soon as the ven- dor is selected so there is flexibility if dates need to be changed for holidays, computer maintenance, etc.; and ⢠Developing and agreeing on the timeline, including final- izing the sample specification, scripting the survey or sending the link to the survey, having a soft launch to test the survey, agreeing on the full implementation and end date, and specifying the frequency of communica- tion with the panel company, especially regarding prob- lems that may occur. Once the survey is in the field, it is important to monitor progress and report any issues immediately to the panel ven- dor, including problems reaching the target quotas for com- pleted surveys. The sooner action is taken, the easier it will be to rectify the issue. It is advisable to work closely with the vendor supplying the panel to take advantage of its experi- ence with data issues with long surveys and improving the survey experience. Online Research Communities Using social media to create online research communities or MROCs for research purposes is a relatively new field. Research communities have been offered by third-party ven- dors since about 2000, but did not become widely used until about 2006. Online research communities typically have a few hundred members, and straddle the divide between quantita- tive and qualitative research. The communities can be short- term, developed for one research question and then dissolved; or can be a long-term resource, allowing research on a wide variety of topics over a period of six months or more. The benefits of online research communities are that they provide access to the authentic voice of the customer; go beyond the numbers to provide qualitative discussion; provide quick turnaround at a low marginal cost because the sampling and recruitment is complete; and create an active dialogue with the customers, letting them feel they âmake a difference.â These communities can either be open to anyone who wishes to join (within the requirements of screening criteria, such as age or geographic location), or closed, in which case panel members are invited to participate. It is important to note that open communities tend to be more about involvement, advo- cacy, and transparency rather than insight and research. Incentives are important to maintaining a high level of par- ticipation for all types of research panels; however, several issues are to be considered when structuring an incentive pro- gram. (It should be noted that it is illegal for some public agen- cies to use incentives.) The argument for using incentives is that they represent a small payment for the time and contributions of the panel members, and may be necessary to obtain the level of engage- ment needed to make the community succeed. The type of incentive (cash versus intrinsic rewards) must also be con- sidered. A chance to win a transit pass or seeing the results immediately upon completing an instant poll are examples of incentives. Finally, the agency must decide how to allocate the incentives. Options include giving all members an incen- tive regardless of participation levels; giving members who participate in a specified time frame the incentive; offering a chance to win a prize; and awarding a prize to the âbestâ contribution in a specified time frame. Agencies should avoid starting with a high-value incentive, because lowering the incentive later will seem to panel members that the agency is taking away a benefit, resulting in a loss of participation. As with all research techniques, the online community can be developed and maintained either in-house or through a vendor. Online research communities require significant and continuous management. Even if the community is maintained by a vendor, significant input by staff is needed to ensure that the community is addressing issues of concern to the agency. The advantages of a having a research-only community are that it can be much smaller than broader-topic communities, and members may be more open if they know they will not be âsold toâ another interest. Opening the community up to other department managers may result in too many surveys and e-mails being sent to members, with research being pushed aside in favor of other topics. Likewise, it is important not to allow community members to usurp the purpose of the research community for their own agendas. Part of managing the community is monitoring and ending any member activ- ity that begins to create an agenda separate from that of the agency, even removing a panel member if necessary. The steps to and guidelines for setting up an online com- munity include determining: ⢠What type of community is best (short versus long term, open versus closed, and the number of members); ⢠The âlook and feelâ (i.e., makeup) of the community; ⢠Community tools; ⢠Methods of recruiting members; ⢠Terms and conditions (including intellectual prop- erty, member expectations, restricted activities, anti- community behavior, privacy and safety, incentive rules, eligibility, data protection and privacy), and the ability to change terms and conditions; ⢠Methods of moderating and managing communities (moderator function, community plan, dealing with neg- ativity, creating member engagement); and ⢠Requirements for finding and delivering insights. The rapid pace of change among social media makes it difficult to project how this type of research activity will be conducted in the future. Four considerations are identified in Poyntonâs book: 1. Market research organizations typically do not allow activities that would influence the outcome of the
15 research. Because interaction and relationships built between community members and the sponsoring com- munity agency may sensitize panel members to organi- zational issues, MROCs may be declared ânot research.â 2. Currently, online research communities are used for more qualitative work rather than large-scale quantita- tive work. The ability to expand online research to larger projects (e.g., international research) will increase this as a mainstream research tool. 3. Respondent fatigue may set in, resulting in a less engaged community. This may be especially true if panel mem- bers belong to more than one community. 4. Alternative (not research-based) methods may be more successful, such as having a very large community that can serve both marketing and research functions, or tap- ping into other existing communities to conduct research rather than establishing one specific to the organization. One of the primary concerns with online research commu- nities has been that the relationship with the organization may cause heightened brand awareness and affinity, and that this will lead to a positive bias in research results. However, Aus- tin notes in an article in Quirkâs Marketing Research Media (Austin 2012) that while engagement builds a relationship with the company, community members remain candid and critical despite their relationship with the brand. If anything, members became slightly more critical as their tenure length- ened, not less. The article recommends that in moving to a new research paradigm, organizations make two changes from the traditional research approach to take advantage of this finding: trade anonymity for transparency because transparency builds engagement; and trade distance for relationship because rela- tionship creates candor. Together, the community members âwork harder, they share more and they stay engaged in the research longer.â Online Panel Sampling Techniques A few online panels employ traditional random sampling tech- niques, such as random-digit-dialing, and then conduct the research online; but the majority of panels are recruited using a non-probability approach online, such as pop-up or web ban- ner ads. The AAPOR Report on Online Panels (Baker 2010) covers both types of panels. This review will cover probability and non-probability sampling techniques as they relate to panels; it also discusses âriver sampling,â although it is not a panel sampling technique, per se. Lastly, it provides an over- view of strategies for adjusting non-probability samples to represent a population. Probability sampling techniques for online survey research have been slow to be adopted, despite being around for more than 20 years. The recruitment is similar to voluntary, non- probabilistic samples, except that the initial contact is based on probabilistic sampling techniques such as random-digit- dialing, or other techniques for which the population is known. Computers may sometimes be provided to persons with no online access to remove bias that might exist from only includ- ing persons or households with Internet access. Once the sample is determined, panels are built and maintained in the same way, regardless of whether they are probability- or non- probability-based. A probability-based sample is more expen- sive to develop than a non-probabilistic sample. Consequently, systematic replacement or the replacement of panel members lost through attrition is also more costly. The benefit is that a panel can be built that represents the general population and allows analysis of results based on probability theory. Non-probability and volunteer online panel members are recruited through a variety of techniques, all of which involve self-selection. The invitations to join a panel can be delivered online (through pop-up or banner advertisements), in maga- zines, on television, or through any other medium where the target population is likely to see the advertisement. The recruit- ment entices respondents by offering an incentive, talking about the fun of taking surveys, or other proven techniques. A common practice in the industry for developing online panels is through co-registration agreements. An organization will compile e-mail lists of its website visitors and ask if they would like to receive offers from partner agencies. The e-mail list is then sold to a research panel company. Off-line recruit- ment strategies include purchasing an organizationâs cus- tomer contact database and asking participants in a telephone survey if they would like to become part of an online panel for future surveys. A technique used for both online and off-line recruitment is to ask existing panel members to refer their friends and relatives, sometimes offering a reward for each new panel member recruited. No two panels are recruited the same way, and the panel research companies carefully guard their methodologies for recruiting panel members. River sampling is an online technique that uses pop-up sur- veys, banner ads, or other methods to attract survey respon- dents when they are needed. In river sampling, the ad presents a survey invitation to site visitors and then directs or âdown- streamsâ them to another, unrelated website to complete the survey. (Using this analogy, a panel would be a pond or reser- voir sample.) Knowing on which websites to place the ads is critical to the success of river sampling. This technique is not related to developing a panel, although sometimes the respon- dent is invited to join a panel at the completion of the sur- vey. There is generally a reward of some kind for completing the survey, such as cash, online merchant gift cards, frequent flyer miles, etc. This type of sampling may be on the rise as researchers seek larger and more diverse sample pools, and to get respondents who are less frequently surveyed than those provided through online access panels. The AAPOR report provides an overview of strategies for adjusting self-selected (non-probability-based) online panels, and reviews complex weighting, quotas, benchmarking, and modeling methodologies for creating a more representative
16 sample. Complex weighting uses detailed information about the population to balance respondents so that they mirror the population. Quotas, which match key demographics of the respondents with the demographics of the target population, are the most common technique. Benchmarking keeps the sample specifications the same over multiple waves, under the assumption that any changes are the result of changes in the element being measured, regardless of whether the sample is representative of the population. Modeling refers to linking the benchmark results to the real world to model what a sur- vey score of X means in terms of actual outcomes. When applying statistical significance testing to the panel sample, it is important to recognize that the significance is not how representative it is of the population, but of the panel. âThe error statistics indicate how likely it is that another sample from the same panel will be different, which is a valid and rele- vant measure of reliabilityâ (Poynter, p. 74). It is not, however, an estimate of the population sampling error, as is commonly understood with traditional random (probabilistic) sampling. Response rates for online access panels have little impact on how representative the research is, but do provide a measure of the quality of the panel. Issues and Concerns with Online Panel Surveys: AAPOR Report on Online Panels Online surveys have grown rapidly because of the lower cost, faster turnaround time, and greater reliability in building tar- geted samples, at the same time that traditional survey research methods are plagued by increasing costs, higher non-response rates, and coverage concerns. The quality of online access panel survey data came into focus in 2006 when the VP of Proctor and Gambleâs Global Consumer Market Knowledge gave a presentation on the range of problems P&G had faced with online access panel reliability. It fielded a survey twice with the same panel, two weeks apart, with results that pointed to two different business conclusions. This focused the market research industryâs attention on the need to provide understand- ing, guidance, and research on the topic of online research. The traditional probabilistic sample, such as random-digit- dialing, is the underpinning of market research. Probabilistic samples are based on the probability of being selected out of a specified population (such as households within the city lim- its). Based on probability theory, the results can be projected to the population with a statistical level of certainty. Online panel surveys typically use non-probability samples, which are a significant departure from traditional methods. The AAPOR Report on Online Panels, produced by the AAPOR task force on opt-in online panels, is a seminal work on concerns and issues with online panel (i.e., non-probability sample) survey research. The scope was to âprovide key infor- mation and recommendations about whether and when opt-in panels might be best utilized and how best to judge their qualityâ (Baker 2010). Sampling Error, Coverage Error, and Non-Response Bias A sample is, by definition, a subset of a population. All sur- veys, regardless of sampling method, have some level of imprecision owing to variation in the sample. This is known as sampling error. A probabilistic sample is one where sam- pling theory provides the probability by which the member of the sample is selected from the total population. In traditional sampling methods, such as random-digit-dialing of house- holds within a geographic area, the total population of home telephone numbers is known. With address-based sampling, the total number of addresses in a specific area is known. Thus the total population is known and the probability of selecting any one phone number (or address) is known. This allows the data to be projected to the population as a whole. The difficulty with online sampling is that the population is unknown. Typically an e-mail address is used as the sampling unit (rather than a home telephone, as in the earlier example). The issues with e-mail addresses include duplication problems, in that one person may have more than one e-mail address; and clustering problems, where an e-mail address represents more than one person. As a result, online sampling differs from tra- ditional sampling in three significant ways: (1) the concept of a sampling frame is discarded and the focus is shifted to recruit- ing as large and diverse a group as possible; (2) instead of a representative sample of all households, a diverse group of persons with the attributes of interest for the panel is recruited; (3) the panel membership is rarely rotated, with panel mem- bers being retained as long as they keep completing surveys. Over time, this can lead to a very different panel membership than the initial profile of the panel. Coverage error occurs when persons, or groups of persons, have zero chance of being selected to participate in the sur- vey. Lack of access to the Internet creates significant cover- age bias. The AAPOR report includes data from 2008 stating that although 85% of the households in the continental United States have some level of Internet service, those without Inter- net access differ significantly from those who do. Those with- out access are more than twice as likely to be over the age of 65 as the general population. They are also more likely to be members of a minority group, to have incomes less than $25,000, to have a high school education (or less), to be unem- ployed, not to own a home, and to live in rural counties or the South Census Region. It can also be noted that having access to the Internet does not necessarily make for active users of the Internet. In 1970, household telephone coverage estimates of 88% led to the acceptability of using telephone surveys in place of in person interviewing. Coverage estimates of Inter- net usage are currently lower than 88%, indicating that it has not yet reached a level where it can be used to represent the general population. Commercial online access panels are even more problem- atic, in that a person has to have Internet access, receive an invitation to become a panel member, sign up for the panel,
17 and then participate in the surveys. Current estimates are that less than 5% of the population has signed up for an online panel, meaning that more than 95% of the population has a 0% chance of being selected. Non-response bias is when some of the persons in the sam- ple choose not to respond to the survey, or some of the ques- tions within the survey. Four stages of panel development are discussed, and how online panel survey development is affected by non-response bias: Stage 1: Recruitment of panel members. The previous discussion on coverage error points out issues with Internet access. In addition, there is bias regarding which Internet users are likely to join a panel. The report cites several studies that found online panels are more likely to be comprised of white, active Internet users with high education levels who are considerably more involved in civic and political activities; and who place less importance on religion and traditional gender roles and more importance on environmental issues. Stage 2: Joining and profiling the respondents. Most panels require a respondent to click through from the online ad to the companyâs website to register for the panel and complete some profile information, including an e-mail address. An e-mail is sent to the prospective panel mem- ber, who must respond in order to join the panel. A study by Alvarez et al. (2003) reported that just over 6% of those who clicked on the banner ad completed all of the steps to become a panel member. Stage 3: Completing the questionnaire. This is similar to random-digit dialing when a person refuses to par- ticipate in the survey or does not meet the eligibility requirements. Online surveys have an additional non- response bias from technical problems that can prevent delivery of the e-mail invitation or completion of the survey itself. Some panels will oversample groups that are known to have low response rates in order to have a representative sample after data collection is complete. Although this may result in a balanced sample on that particular dimension, it does not ensure that the sample is representative on other points. Stage 4: Panel maintenance. Attrition can be ânormal,â when people opt out for whatever reasons; or can be forced, when panel members are automatically dropped from the panel after a set period of time to keep the panel fresh. Many strategies are used to reduce panel attrition, but little research exists on reducing or determining the most âdesirableâ attrition rate to balance the costs of adding panel members with the potential concerns of long-term membership, such as panel conditioning. Measurement Error Measurement error is defined as the difference between an observed response and the underlying true response. This can be random error, as when a respondent picks an answer other than the true response, without any systematic direc- tion in the choice made. Systematic measurement error, or bias, occurs when the responses are more often skewed in one direction. Much of the literature regarding measurement error is related to the benefits and potential biases of personal interviewers and self-administered surveys, including paper and online surveys. Because this is an issue that is related to data collection methodology for any survey, not specific to panel surveys, it is beyond the scope of this project and will not be covered in this literature review. However, this is an important issue for all survey efforts, and researchers are encouraged to look at the issues related to both interviewers and self-administered surveys. One measurement issue directly related to panel surveys is that of panel conditioning. Repeatedly taking surveys on a par- ticular topic is known to make respondents more aware of that topic, pay more attention to it in their daily lives, and therefore have different responses on future surveys than if they had not been on the panel. The research on panel conditioning with online panels has mixed findings. Some studies have shown a marked bias towards an increased likelihood to purchase; other studies show that this effect can be mitigated by vary- ing topics from survey to survey. Other research studies have shown no difference in attitudinal responses between infre- quent and very experienced panel survey members. There are two theories on the effects of taking large numbers of surveys: Experienced survey-takers may be more likely to answer in a way that they believe is best for themselves (e.g., it will earn them more incentives, or get more surveys to complete; alter- natively, experienced survey takers will understand the pro- cess better, resulting in more accurate and complete responses. So far, there is no definitive research on the effects of panel members completing large numbers of surveys regarding the accuracy of the survey results. Sample Adjustments to Reduce Error and Bias It is agreed by most researchers that online panels are not representative of the general population, and that techniques are needed to correct for this if the results are used. Four tech- niques have been used to attempt to correct for the known biases with a goal of making the sample representative of the population: sampling to represent a population; modeling; post-survey adjustment; and propensity weighting. 1. The most common form of sampling to represent a certain population is quota sampling, with the quotas often being demographics to match the census. Other elements can be factored in by, for example, balancing members by political affiliation. There does not appear to be any research on the reliability or validity of this type of sampling applied to panel surveys. 2. Models are frequently used in the physical sciences and in epidemiological studies to reduce error and bias. Online panels are much more complex than epidemio- logical studies, however, making it more difficult to apply model-based techniques.
18 3. The most common post-survey adjustment is the weight- ing of survey data. The difference between the sample and sampling frame with probability samples is handled through probability theory principles. Because there is rarely a sampling frame in an online sample, the census and other sources are typically used to adjust the results for under-representation of certain groups of respon- dents. Work conducted by Dever et al. (2008) found that inclusion of enough variables could eliminate coverage bias, but did not address problems associated with being a non-probability sample. 4. To apply propensity weighting, a second âreferenceâ survey with a probability-based sample is conducted at the same time as the online panel survey, using the same questions. A model is built that can be used to weight future online surveys to better represent the target pop- ulation. Although this technique can be used success- fully, it can also increase other types of error, leading to erroneous conclusions from the resulting data. The AAPOR report (Baker 2010) provides an extensive discussion of and guidance on applying these techniques. The reader is encouraged to review the report before applying a sampling adjustment technique. Panel Data Quality Panel data cleaning is an important step in delivering results from respondents who are real, unique, and engaged in the sur- vey. Three areas of cleaning panel data are discussed: eliminat- ing fraudulent respondents, identifying duplicate respondents, and measuring engagement. Fraudulent respondents are those who sign up for a panel multiple times under false names and lie on the qualifying questionnaire to maximize their chances of participation. Duplicate responses occur when respondents answer the questionnaire more than once from the same invita- tion or when they are invited to complete the survey more than once because they belong to more than one panel. Measuring engagement is the most controversial technique. Four basic cleaning strategies are used to weed out respon- dents who may not be engaged with completing the survey, but are simply answering to earn the incentives: recognizing respondents with very short survey times (compared with all surveys); identifying respondents who answer all questions in a matrix format (usually scaled questions) the same way; recording an excessive selection of non-substantive answers, such as âdonât knowâ; and noting nonsense answers or identi- cal answers provided for all open-ended questions. Although there was no research at the time that demon- strated the effects of using cleaned data on the sample or final results, it is generally accepted that negative respondent behavior is detrimental to data quality. Industry Focus on Quality The market research industry has been focused on panel data quality, with virtually every national and international asso- ciation incorporating principles and guidelines for conducting online and panel research. Four key efforts are highlighted in the report: 1. The Council of American Survey Research Organiza- tion (CASRO) revised its Code of Standards and Eth- ics for Survey Research in 2007 to include specific clauses related to online panels. 2. ESOMAR developed comprehensive guidelines titled âConducting Market and Opinion Research Using the Internet.â This was supplemented by its â26 Questions to Help Research Buyers of Online Samples.â 3. The International Organization for Standardization technical committee that developed ISO 20252â Market, Opinion and Social Research also developed ISO 26362âAccess Panels in Market, Opinion and Social Research. The standard defines key terms and concepts in an attempt to create a common vocabu- lary for online panels, and details the specific kinds of information that a research panel is expected to make available to a client at the conclusion of every project. 4. The Advertising Research Foundation established the Online Research Quality Council, which in turn designed and executed the Foundations of Quality project. Work was in progress as of the writing of the AAPOR report, and as of the writing of this synthesis, results of the effort were just being made public. Recommendations The AAPOR Report on Online Panels makes the following recommendations to market researchers who are considering using online access panels: ⢠A non-probability online panel is appropriate when precise estimates of population values are not required, such as when testing the receptivity to product concepts and features. ⢠Avoid using non-probability online access panels when the research is to be used to estimate population values. There is no theoretical basis for making projections or estimates from this type of sample. ⢠The accuracy of self-administered computer surveys is undermined because it is a non-probability sample. A random-digit-dial telephone survey is more accurate than an online survey because it is a probability sam- ple, despite the coverage error arising from households without a landline phone. ⢠It has not yet been demonstrated that weighting the results from online access panel surveys is consistently effective and can be used to adjust for panel bias.
19 ⢠There are significant differences in the composition and practices of various online access panels, which can affect survey results. Different panels may yield significantly different results on the same questionnaire. Market Research by the Public Sector Poynterâs book devotes a section to issues specific to pub- lic sector research. Although most of the marketing research principles apply equally to the private and public sectors, there are a few areas where the public sector researcher needs to be particularly attentive, because public funds are being used to conduct the research and the results may determine how public funds are expended. Areas for particular attention are identified as: operating in the public eye, ârepresentativ- ity,â geographical limitations, social media and the public sector, and ethics. In the Public Eye Public sector research is subject to audit, inspection, and reporting in the media. Freedom of information laws ensure that the public has a right to see how public funds are being spent. Poorly conducted research could be brought to light in a public forum, creating public relations problems for a per- ceived waste of taxpayer money and jeopardizing the ability to conduct future research. As a result, care must be taken to ensure public sector research is conducted to the highest qual- ity and ethical standards. Representativity Having a representative sample is always important, but is of special concern for public agencies. Many public services, such as public transportation, target specific groups which may have multiple challenges. Much of the target population may not have Internet access, and those that do may not be typical of the market segment they are expected to represent. For each study, the researcher must carefully assess whether an online survey is appropriate for that market and research purpose, and whether the sampling and recruitment strategies provide survey results that can be defended in public. Geographical Limitations Public agencies have strict geographic boundaries from which a sample population can be drawn. Face-to-face or telephone surveys are often simplified by these restrictions. Surveys using an online access panel, however, can be problematic, as there may not be an adequate sample of persons from the target area. This is further exacerbated when the sample is also required to be representative of the population within a speci- fied geographical area. Social Media and the Public Sector There are several ways in which social media are being used for research in the public sector. Online communities engage in a range of activities, including information-sharing, research, community-building, and engagement. Online research com- munities are typically closed communities, operated by a ven- dor, with membership by invitation only as part of an overall sampling plan (see the MnDOT case example of an online research community). Twitter, blogs, and public discussions are resources for passive research, using data mining tools to monitor trends in what people are saying about the agency. Although useful information can be elicited from these sources, it should be noted that they do not provide a representative sample, and should be considered public comment rather than research. Social media is often used to reach out to groups that are otherwise hard to reach, such as young adults. It should be noted that using a variety of social media techniques, such as Facebook, YouTube, and Twitter, is likely to reach the same people multiple times. If multiple social media channels are used to recruit online survey participants, for example, the researcher must be prepared for the potential duplication of survey responses. Ethics There is an expectation that research will be reliable, and can be used by a decision-making body in a public forum. First and foremost, the researcher must provide unbiased market research. Often a vendor is used to conduct the research so as to provide a wall between the agency and the research and avoid the appearance of leading the respondents, or âspinningâ the results. The second concern is that quantitative research based on random probability sampling has been the standard method for achieving that level of reliability expected of a pub- lic agency. Since online research is typically not from a proba- bilistic sample, the researcher should recognize the potential lack of statistical reliability inherent in the research design and ensure that decision makers understand the limitations of the data. FUTURE OF MARKET RESEARCH Technology has fundamentally changed how society com- municates and how it does business. Whereas people used to communicate by means of the telephone at home, cell phones make communication possible virtually anywhere. Cell phone numbers do not represent a physical address; they have become a moving, real-time âpersonalâ address. The Internet provides instant access to information and communication through e-mail, websites and social media. The smart phone combines mobile communication with the Internet, creating
20 a completely new, technology-based world. Panels can now be developed online, quickly and easily. Household and per- sonal contact information is no longer tied to a home address, but exists outside of the personâs geographic location. This technology has led to a revolution in market research. Recruiting survey respondents is easier; developing a panel is faster; and surveys are online, resulting in automation of survey tabulation and reporting. As a result, recruiting and maintaining research panels is simpler, less expensive, and very attractive to decision makers who want results ânow.â But these changes have created a myriad of concerns, pri- marily related to using non-probabilistic sampling practices. The history of sampling theory provides some insights into what may occur in the future. In 1934, although sam- pling theory had not yet been developed, Anders Kaier con- vinced an international audience that representative samples could be used to represent a population. Morris Hansen of the U.S. Census Bureau greatly expanded the theory and prac- tice of sampling and helped convince the bureau to accept sampling and quality control methods in the 1940 Census. Through the leadership of these two important individuals, the practice was adopted. From there, sampling theory was developedâit followed the practice, rather than the theory creating the practice. Brick states that data collection costs will continue to put pressure on agencies to use non-probability samples from online recruitment. If this cannot be done within design-based probability sampling theory, he suggests two potential out- comes: A new paradigm that accommodates online surveys is introduced, which replaces or supplements traditional prob- ability sampling; or online surveys using non-probabilistic sampling are restricted to specific applications as a result of the weak theoretical basis. One potential solution is the use of multiple-frame sam- pling to reduce coverage error, a fundamental concern with online panel research. For example, to reach transit riders, an online survey could be placed on the agency website and be supplemented with paper surveys on board vehicles. Stat- isticians are working on establishing a theoretical basis for conducting sampling using the multiple-frame technique (Brick 2011). In addition to the changes in survey practice that led to the historical development of sampling theory, two additional factors are cited as creating the paradigm shift from popula- tion surveying to representative sampling in 1934. The first was the wealth of scientific development and statistical ideas, not necessarily related to survey sampling, which neverthe- less supported the growth and change in methods. The sec- ond factor was societyâs demand for information on a wide range of topics that made population sampling cumbersome and expensive. This desire for faster, cheaper research drove the development of probability sampling and our current market research paradigm. These characteristics are in place today, almost 80 years after probabilistic sampling made its debut. With the rapid changes in technology and societyâs insatiable thirst for more information, more quickly, and for less cost, a new research paradigm with a theoretical founda- tion to support non-probabilistic online surveying may be on the horizon.
TRB’s Transit Cooperative Research Program (TCRP) Synthesis 105: Use of Market Research Panels in Transit describes the various types of market research panels, identifies issues that researchers should be aware of when engaging in market research and panel surveys, and provides examples of successful market research panel programs.
The report also provides information about common pitfalls to be avoided and successful techniques that may help maximize research dollars without jeopardizing the quality of the data or validity of the results.
READ FREE ONLINE
Welcome to OpenBook!
You're looking at OpenBook, NAP.edu's online reading room since 1999. Based on feedback from you, our users, we've made some improvements that make it easier than ever to read thousands of publications on our website.
Do you want to take a quick tour of the OpenBook's features?
Show this book's table of contents , where you can jump to any chapter by name.
...or use these buttons to go back to the previous chapter or skip to the next one.
Jump up to the previous page or down to the next one. Also, you can type in a page number and press Enter to go directly to that page in the book.
To search the entire text of this book, type in your search term here and press Enter .
Share a link to this book page on your preferred social network or via email.
View our suggested citation for this chapter.
Ready to take your reading offline? Click here to buy this book in print or download it as a free PDF, if available.
Get Email Updates
Do you enjoy reading reports from the Academies online for free ? Sign up for email notifications and we'll let you know about new publications in your areas of interest when they're released.
Internet marketing: a content analysis of the research
- Special theme
- Open access
- Published: 31 January 2013
- Volume 23 , pages 177–204, ( 2013 )
Cite this article
You have full access to this open access article
- J. Ken Corley II 1 ,
- Zack Jourdan 2 &
- W. Rhea Ingram 2
49k Accesses
24 Citations
2 Altmetric
Explore all metrics
The amount of research related to Internet marketing has grown rapidly since the dawn of the Internet Age. A review of the literature base will help identify the topics that have been explored as well as identify topics for further research. This research project collects, synthesizes, and analyses both the research strategies (i.e., methodologies) and content (e.g., topics, focus, categories) of the current literature, and then discusses an agenda for future research efforts. We analyzed 411 articles published over the past eighteen years (1994-present) in thirty top Information Systems (IS) journals and 22 articles in the top 5 Marketing journals. The results indicate an increasing level of activity during the 18-year period, a biased distribution of Internet marketing articles focused on exploratory methodologies, and several research strategies that were either underrepresented or absent from the pool of Internet marketing research. We also identified several subject areas that need further exploration. The compilation of the methodologies used and Internet marketing topics being studied can serve to motivate researchers to strengthen current research and explore new areas of this research.
Similar content being viewed by others
A Backward Glance of who and what Marketing Scholars have Been Researching, 1977–2002
Marketing Research Directions in the 80’s and 90’s
Marketing Theory: The Present Stage Of Development
Avoid common mistakes on your manuscript.
Introduction
In the early years of the Internet Age, the potential of using the Internet as a distribution channel excited business managers who believed this tool would boost sales and increase organizational performance (Hansen 1995 ; Westland and Au 1997 ). These believers suspected an online presence could offer advantages to their customers, while providing a shopping experience similar to the traditional bricks-and-mortar store (Jarvenpaa and Todd 1996 ). The advantages included providing around the clock access for customers, reducing geographic boundaries to provide access to new markets, and enabling immediate communication with customers.
The prediction of an explosion of online shopping became a marriage between information technology experts and marketing professionals. Most would believe the information technology researchers were studying the Internet technology and its advantages, while the marketers were focused on the consumer’s use of the technology. As technology advanced, more marketing activities emerged to market goods and services via the Internet. Today, Internet marketing is defined as “the use of the Internet as a virtual storefront where products are sold directly to the customer” (Kiang et al. 2000 , p. 383), or another view includes “the strategic process of creating, distributing, promoting, and pricing products for targeted customers in the virtual environment of the Internet” (Pride et al. 2007 ). This research attempts to categorize the various Internet marketing activities in a broad context including strategies such as customer relationship management (Hwang 2009 ), electronic marketplaces (Novak and Schwabe 2009 ), online auctions (Loebbecke et al. 2010 ), and electronic branding (Otim and Grover 2010 ) in tandem with unique IS issues including web site evaluation (Chiou et al. 2010 ), piracy (Smith and Telang 2009 ), security (Ransbotham and Mitra 2009 ), and technology architecture (Du et al. 2008 ).
With concepts as varied as this in one research domain, a periodic review is necessary to discover and explore new technologies such as mobile banking (Sripalawat et al. 2011 ), virtual worlds (Sutanto et al. 2011 ), and social media (de Valck et al. 2009 ) as they emerge on the Internet marketing landscape. The following sections of the paper will examine the current literature to determine what is known about the concept of Internet marketing. First, a description of the methodology for the analysis of the Internet marketing research is presented. This is followed by the results including an analysis of a smaller sample of the Internet marketing research in the top Marketing journals. Finally, the research is summarized with a discussion of the limitations of this project and suggestions for future research.
Methodology
The approach to this analysis of the Internet marketing research is to first identify trends in the Information System (IS) literature. Specifically, we wished to capture the trends pertaining to (1) the number and distribution of Internet marketing articles published in the leading journals, (2) methodologies employed in Internet marketing research, and (3) the research topics being published in this area of research. During the analysis of the literature, we attempted to identify gaps and needs in the research and therefore discuss a research agenda which allows for the progression of research (Webster and Watson 2002 ). In short, we hope to paint a representative landscape of the current Internet marketing literature base in IS in order to influence the direction of future research efforts in this important area of study.
In order to examine the current state of research on Internet marketing, the authors conducted a literature review and analysis in three phases: Phase 1 accumulated a representative pool of articles; Phase 2 classified the articles by research method; and, Phase 3 classified the research by research topic. Each of the three phases is discussed in the following paragraphs.
Phase 1: accumulation of article pool
We used the Thomson Reuters Web of Science (WoS) citation database and Google Scholar to search for research articles with a focus on Internet marketing. The search parameters were constrained based on (a) a list of top ranked journals, (b) a specific time range, and (c) key search terms.
First, the researchers chose to use the top 30 journals from Peffers and Tang’s ( 2003 ) IS journals ranking (see Table 1 ). Peffers and Tang’s ( 2003 ) ranking of ‘pure’ IS journals was adopted for this study because it was based on the responses of IS researchers who were asked to rank journals by their “relative value to the researcher and the audience as an outlet for IS research.” In Peffers and Tang’s ( 2003 ) original ranking scheme two journals, ‘Communications of the Association of Information Systems’ and ‘Information and Management,’ tied for fifth place. Peffers and Tang resolved this issue by ranking both journals in the fifth position skipping the rank of the sixth position. As noted in Table 1 , 7 of the top 30 journals were not listed in the WoS database. Consequently, all 30 journals were searched using Google Scholar and only 23 journals were searched using the WoS database. The search parameters were further constrained to a specific timeframe.
Electronic commerce and Internet marketing did not exist prior to the widespread adoption and dissemination of the public Internet and the Worldwide Web (WWW). Therefore, the search parameters were further constrained based on the historical timeframe in which technologies capable of facilitating the development of e-commerce were first introduced. The graphical user interface based browser known as Netscape Navigator was launched as a free download for public use in 1994. Many experts identify the launch of Netscape Navigator as the historical event leading to the global public’s widespread adoption and use of the Internet and the World Wide Web (WWW) (Friedman 2006 ). Therefore, the search parameters for both WoS and Google Scholar were constrained to time period of 1994 through August of 2011.
The final constraint was based on the key search term “Internet Marketing.” In both WoS and Google Scholar the search engine scanned for the term ‘Internet Marketing’ and close variations of this term found in the title, abstract, and keywords of articles published in the top 30 IS journals between January of 1994 and August of 2011 when the search was executed. There was considerable overlap in the pool of articles returned from the two search engines (WoS and Google Scholar). Once duplicate entries and non-research articles (book reviews, editorials, commentary, etc.) were removed 453 articles remained in the composite data pool. The researchers then reviewed each article and identified 42 articles that were unrelated to the topic of Internet marketing. These 42 articles represented false positives returned from the WoS and Google Scholar search engines and were subsequently removed leaving 411 articles in the final composite article data pool for analysis.
Phase 2: classification by research strategy
Once the researchers identified the articles for the final data pool, each article was examined and categorized according to its research strategy. Due to the subjective nature of research strategy classification, content analysis methods were used for the categorization process. Figure 1 illustrates steps in the content analysis process adapted from Neuendorf ( 2002 ) and successfully employed by several similar research studies (Corley et al. 2011 ; Cumbie et al. 2005 ; Jourdan et al. 2008 ). First, the research categories were adopted from Scandura and Williams ( 2000 ) (see Table 2 ), who extended the research strategies initially described by McGrath ( 1982 ). Specifically, nine categories of research strategies were selected including: Formal theory/literature reviews, sample survey, laboratory experiment, experimental simulation, field study (primary data), field study (secondary data), field experiment, judgment task, and computer simulation.
Overview of literature analysis
Second, to guard against the threats to reliability (Neuendorf 2002 ), we performed a pilot test on articles meeting the search parameters from other top journals. That is, the articles used in the pilot test (a) were not part of the data set generated in Phase 1, and (b) the data generated from the pilot test were not included in the final data analysis for this study. Researchers independently categorized the articles in the pilot test based on the best fit among the nine research strategies. After all articles in the pilot test were categorized, the researchers compared their analyses. In instances where the independent categorizations did not match the researchers re-evaluated the article collaboratively by reviewing the research strategy definitions, discussing the disagreement thoroughly, and collaboratively assigning the article to a single category. This process allowed the researchers to develop a collaborative interpretation of the research strategy definitions. Simply stated, this pilot test served as a training session for accurately categorizing the articles for this study with respect to research strategy.
Each research strategy is defined by a specific design approach and each is also associated with certain tradeoffs that researchers must make when designing a study. These tradeoffs are inherent flaws that limit the conclusions that can be drawn from a particular research strategy. These tradeoffs refer to three aspects of a study that can vary depending on the research strategy employed. These variable aspects include: generalizability from the sample to the target population (external validity); precision in measurement and control of behavioural variables (internal and construct validity); and the issue of realism of context (Scandura and Williams 2000 ).
Cook and Campbell ( 1976 ) stated that a study has generalizability when the study has external validity across times, settings, and individuals. Formal theory/literature reviews and sample surveys have a high degree of generalizability by establishing the relationship between two constructs and illustrating that this relationship has external validity. A research strategy that has low external validity but high internal validity is the laboratory experiment. In the laboratory experiment, where the degree of measurement precision is high, cause and effect relationships may be determined, but these relationships may not be generalizable for other times, settings, and populations. While the formal theory/literature reviews and sample surveys have a high degree of generalizability and the laboratory experiment has a high degree of precision of measurement, these strategies have low degree of contextual realism. The only two strategies that maximize degree of contextual realism are field studies that use either primary or secondary data because the data is collected in an organizational setting (Scandura and Williams 2000 ).
The other four strategies maximize neither generalizability, nor degree of precision in measurement, nor degree of contextual realism. This point illustrates the futility of using only one strategy when conducting Internet marketing research. Because no single strategy can maximize all types of validity, it is best for researchers to use a variety of research strategies. Table 2 contains an overview of the nine strategies and their ranking on the three strategy tradeoffs (Scandura and Williams 2000 ).
Two coders independently reviewed and classified each article according to research strategy. Only a few articles were reviewed at one sitting to minimize coder fatigue and thus protect intercoder reliability (Neuendorf 2002 ). Upon completion of the independent classification, a tabulation of agreements and disagreements were computed, intercoder crude agreement (percent of agreement) was 91.8 % percent, and intercoder reliability using Cohen’s Kappa (Cohen 1960 ) was calculated ( k = 0.847). These two calculations were well within the acceptable ranges for intercoder crude agreement and intercoder reliability (Neuendorf 2002 ). The reliability measures were calculated prior to discussing disagreements as mandated by Weber ( 1990 ). If the original reviewers did not agree on how a particular article was coded, an additional reviewer arbitrated the discussion of how the disputed article was to be coded. This process resolved the disputes in all cases.
Phase 3: categorization by internet marketing research topic
Typically the process of categorizing research articles by a specific research topic involves an iterative cycle of brainstorming and discussion sessions among the researchers. This iterative process helps to identify common themes within the data pool of articles. Through the collaborative discussions during this process researchers can synthesize a hierarchical structure within the literature of overarching research topics and more granular level subtopics. The final outcome is a better understanding of the current state of a particular stream of research. This iterative process was modified for this specific study on the topic of Internet marketing.
During the initial stages of the current project the researchers began investigating tentative outlets for publishing a literature review on the topic of Internet marketing. A special call for papers (CFP) on the topic of Internet marketing from the journal ‘Electronic Marketing’ was identified as a potential target journal by one of the authors. Further investigation revealed that the editors had outlined six specific research topic categories for the special CFP including: Business Models of Online Marketing, The Future of Search Strategies, The Internet Advertising Landscape, Commercial Exploitation of Web 2.0 in Consumer Marketing and in an Organizational Context, Evaluation of Online Performance, and Other Topics. Each of these six research topics was accompanied by a general definition and a few examples. The researchers adopted these six research topics to categorize the articles in the data pool.
A second pilot study was performed mirroring the first pilot test as a means of training for categorizing articles by research topic. Researchers independently categorized the articles in the pilot test based on the best fit among the six research topics. After all articles in the pilot test were categorized, the researchers compared their analyses. In instances where the independent categorizations did not match, the researchers re-evaluated the article collaboratively by reviewing the research category definitions, discussing the disagreement thoroughly, and collaboratively assigning the article to a single category. This process allowed the researchers to develop a collaborative interpretation of the research topic definitions (see Table 3 ).
Once we established the category definitions, we independently placed each article in one Internet marketing category. As before, we categorized only a few articles at a time to minimize coder fatigue and thus protect intercoder reliability (Neuendorf 2002 ). Upon completion of the classification process, we tabulated agreements and disagreements, intercoder crude agreement (percent of agreement) was 86.2 %, and intercoder reliability using Cohen’s Kappa (Cohen 1960 ) for each category was calculated ( k = .08137). Again, the latter two calculations were well within the acceptable ranges (Neuendorf 2002 ). We again calculated the reliability measures prior to discussing disagreements as mandated by Weber ( 1990 ). If the original reviewers did not agree on how a particular article was coded, a third reviewer arbitrated the discussion of how the disputed article was to be coded. This process also resolved the disputes in all cases.
In order to identify gaps and needs in the research (Webster and Watson 2002 ), we hope to paint a representative landscape of the current Internet marketing literature base in order to influence the direction of future research efforts in this important area of study. In order to examine the current state of this research, the authors conducted a literature review and analysis in three phases. Phase 1 accumulated a representative pool of Internet marketing articles, and the articles were then analyzed with respect to year of publication and journal. Phase 2 contains a short discussion of the research strategies set forth by Scandura and Williams ( 2000 ) and the results of the classification of the articles by those research strategies. Phase 3 involved the creation and use of six Internet marketing research topics, a short discussion of each topic, and the results of the classification of each article within the research topics. These results are discussed in the following paragraphs.
Results of phase 1
Using the described search criteria within the selected journals, we collected a total of 411 articles (For the complete list of articles in our sample, see Appendix A .) In phase 1, we further analyzed the articles’ year of publication and journal. Figure 2 shows the number of articles per year in our sample. Please note that 2011 only represents articles acquired using WoS and Google Scholar search engines which were available at the time (August 2011) the search was conducted. There is a general increasing trend over the 18 year period, but no articles were found to be published in 1994 & 1996. The year 2010 shows the most activity with 52 articles (12.7 %). With Internet marketing issues becoming ever more important to researchers and practitioners, this comes as no surprise. Understanding 2011 was only a partial year in our sample, we were not concerned by the difference in quantity of publications over time.
Number of Internet Marketing Articles Published Per Year
In order to identify the research strategies used by Internet marketing research articles in the top 30 Information Systems (IS) journals in our sample, Table 4 was created to show the number of Internet marketing articles in each journal broken down by research strategy. This table illustrates the high level of Internet marketing publications that use the Formal Theory/Literature Review, Sample Survey, Field Study – Primary, and Field Study – Secondary research strategies. This indicates a body of research that is still in the exploratory stages. This table also illustrates the proclivity of some journals to accept certain research strategies over others. For example, the journals Decision Support Systems , International Journal of Electronic Commerce , and Journal of Management Information Systems had articles in this data set using seven of the nine research strategies. With this information, researchers that favour certain research strategies can target their research papers to journals that favour these strategies.
Number of Internet Marketing Articles Published in Each Research Strategy Category
Results of phase 2
The results of the categorization of the 411 articles according to the nine research strategies described by Scandura and Williams ( 2000 ) are summarized in Fig. 3 and Table 5 . Of the 411 articles, 110 articles (26.8 %) were classified as Formal Theory/Literature review making it the most prevalent research strategy. This was followed by Sample Survey with 94 articles (or 22.9 %), Field Study – Secondary Data with 91 articles (22.1 %), Field Study – Primary Data with 66 articles (16.1 %), and Computer Simulation with 25 articles (6.1 %). These five research strategies composed 94 % of the articles in the sample. No articles were classified as a Judgment Task. So, the remaining three research strategies represented the remaining six percent of the sample which included Lab Experiment with 11 articles (2.7 %), Field Experiment with 11 articles (2.7 %), and Experimental Simulation with 3 articles (0.7 %).
Further analysis showing the research strategies over the 18 year period from 1994 to August 2011 (Table 6 ) illustrates that Formal Theory/Literature Review, Sample Survey, Field Study – Secondary Data, and Field Study – Primary Data are represented in almost every year of the timeframe. No articles were found in the years 1994 & 1996, and only one article was found in 1995. These four strategies are exploratory in nature and indicate the beginnings of a body of research (Scandura and Williams 2000 ). Further categorization and analysis of the articles with respect to Internet marketing topic categories was conducted in the third phase of this research project.
Results of phase 3
Table 7 shows the number of articles per Internet marketing research topic category. These six categories provided a topic area classification for all of the 411 articles in our research sample. Of the 411 articles, 41.1 % were classified as ‘Business Models of Online Marketing’ making it the most prevalent Internet marketing topic category. This category was followed by ‘The Internet Advertising Landscape’ (22.4 %), ‘Evaluation of Online Performance’ (16.5 %), and ‘Other’ (10.0 %). These four research strategies accounted for 90 % of the articles in the sample. The topic categories titled ‘Commercial Exploitation of Web 2.0 in Consumer Marketing and in an Organizational Context’ and ‘The Future of Search Strategies’ represented the remaining six per cent (5.8 %) and four percent (4.1 %) of the articles. This illustration of the share of Internet marketing research that is represented by each category reveals the amount of attention topic categories of Internet marketing research have historically received among the top 30 IS journals.
By plotting Internet marketing research topics against research strategies (Table 8 ), many of the gaps in Internet marketing research are exposed. The gaps are at the intersection of less used methodologies (Judgement Task, Experimental Simulation, Lab Experiment) and less studied domains in Internet marketing (Search Strategies and Web 2.0). We believe these gaps exist for two reasons. First, some of these research strategies are not prevalent in IS research, and some top IS journals do not accept papers that use unusual research strategies. So, researchers avoid unorthodox strategies. The reason some of these categories have not been studied is because they represent relatively new phenomena, and the research has not caught up with the business reality. The great news for researchers interested in Internet marketing is that this domain should provide research opportunities for years to come. To better illustrate the categorization process, Table 9 presents a sample of articles noting their corresponding research strategy and research topic. These articles were randomly selected as typical examples and are not meant to serve as hallmarks of a particular research strategy or research topic within Internet marketing research.
About half (49 %) of the journal articles in this study use the Formal Theory/Literature Review and Sample Survey research strategies indicating the exploratory nature of the current research. We speculate the strategies used to study these topics were prevalent for several reasons. First, these strategies are the most appropriate for the early stages of research. In these exploratory years of Internet marketing research, formal theory/literature reviews are appropriate in order to determine what other strategies are being used in the research, define the topics under investigation, and find research in reference disciplines that are conducting similar research. Second, many researchers in business schools may prefer to administer sample surveys and field studies instead of laboratory experiment, experimental simulation, judgment task, and computer simulation because of the preferences for certain research strategies in the top journals in Information Systems and Marketing. Finally, organizations are less likely to commit to certain strategies (i.e. primary & secondary field studies and field experiments) because these strategies are more expensive for the organizations. These types of research strategies are very labour intensive to the organization being studied because records will need to be examined, personnel will need to be interviewed, and senior managers will be required to devote large amounts of their expensive time to help facilitate the research project. It is interesting to note that many of the articles coded as Field Study – Secondary and Computer Simulation used historical auction and pricing data freely available from the World Wide Web to avoid this issue.
Investigating the marketing literature
In order to investigate the Internet marketing research being conducted in the top Marketing Journals, we also performed a smaller literature review using the top five ranked marketing research journals following the same methodology previously described for the top 30 ranked IS journals. This list was compiled from three recent marketing journal rankings (Hofacker et al. 2009 ; Moussa and Touzani 2010 ; and Polonsky and Whitelaw 2006 ). The data pool included 24 articles, and after screening out irrelevant articles (book reviews, opinion pieces, etc.) the remaining 22 articles were categorized by research strategy and research topic (see Appendix B ). Upon completion of the categorization process, we tabulated agreements and disagreements. Intercoder crude agreement (percent of agreement) was 95.4 % for research strategy and 90.9 % for research topic. Cohen’s Kappa could not be calculated because the sample size was too small. These two calculations were well within the acceptable ranges (Neuendorf 2002 ). The results of the literature review of the top five marketing journals are displayed in Tables 10 and 11 .
The number of articles published on the topic of Internet marketing in each of the top five ranked marketing journals is presented in Table 10 . It is interesting to note that no articles were found in Journal of Consumer Research while 16 of the 22 (72.7 %) articles in the data pool were published in Marketing Science . This could indicate (a) Marketing Science is a top outlet for Internet marketing research or (b) the other Marketing journals use keywords other than “Internet marketing” to classify this area of research. The number of articles categorized based on both research strategy and research topic is presented in Table 11 . The three research strategies with the largest number of articles among the top five marketing journals were “Formal Theory / Lit Review” (45.5 %), “Field Study - Secondary” (27.3 %), and “Field Study – Primary” (18.2 %). This indicates, like the research published in the top IS journals, the Internet marketing research published in the top marketing journals is also still in the exploratory stages.
Fourteen of the twenty-two articles (63.6 %) were categorized within the research topic labelled “the Internet Advertising Landscape” while no articles were categorized within the research topics “Commercial Exploitation of Web 2.0” or “Evaluation of Online Performance.” In contrast to the analysis of the top thirty ranked IS journals in which the top three research topics were “Business Models of Online Marketing” (41.1 %), “the Internet Advertising Landscape” (22.4 %), and Evaluation of Online Performance (16.5 %); the top three research topics within the top five marketing journals were “the Internet marketing Landscape” (63.6 %), “Business Models of Online Marketing” (13.6 %), and “Other Topics” (13.6 %). Due to the small number of articles in the sample, it is difficult to make any statements regarding trends in the Internet marketing research in the top Marketing journals.
Limitations and directions for future research
The current analysis of the Internet marketing literature is not without limitations and should be offset with future efforts. In summary, this literature review highlights the upward trend of Internet marketing research but also the limitations of both the research strategies employed and the topics investigated. The authors would suggest future literature reviews should expand article searches to full article text searches, search a broader domain of research outlets, and include other Internet marketing related search terms. Our literature analysis is meant to serve as a representative sample of articles and not a comprehensive or exhaustive analysis of the entire population of articles published on the topic of ‘Internet marketing.’ To further investigate this body of research, future research studies could explore the diversity of the Internet marketing research domain (Lee et al. 2007 ) or revisit Ngai and Wat’s ( 2002 ) electronic commerce literature review to assess the progress of that research stream. Other studies could take a more in depth look at the various business models or Internet advertising strategies associated with Internet marketing by reviewing the literature in areas such as electronic auctions, search strategies, social media, e-tailing, and various other research domains.
As Internet marketing continues to grow, future studies should consider the role of research relative to generalizability, precision of measure, and realism of context. Future research efforts should adopt more precise measures of what is occurring in this domain. Much of the research in our sample reports the new technologies and issues in Internet marketing without attempting to explain the fundamental issues of IS research. This is to be expected as this research domain appears to still be in the exploratory stages. For researchers to continue to attempt to answer the important questions in Internet marketing, future studies need to employ a wider variety of research strategies to investigate these important issues. Scandura and Williams ( 2000 ) stated that looking at research strategies employed over time by triangulation in a given subject area can provide useful insights into how theories are developing. In addition to the lack of variety in research strategy, very little triangulation has occurred during the timeframe used to conduct this literature review. This absence of coordinated theory development causes the research in Internet marketing to appear haphazard and unfocused.
However, the good news is that many of the research strategies and topics in this research are available for future research efforts. Of particular interest to researchers and practitioners would be studies observing consumer behaviour in real time using lab and field experiments or measuring purchasing behaviour from using stored click stream data in a secondary field study. We encourage researchers in fields of IS and Marketing to continue developing the body of research on this important topic using cross-disciplinary teams composed of researchers from business and the behavioural sciences. In addition, future studies could consider the six Internet marketing categories with respect to the research strategies. More specifically, each ‘zero’ appearing in Tables 8 and 11 represent gaps in the literature which provide countless opportunities for researchers to build upon the current body of published research. With this in mind, we hope this research analysis lays a foundation for developing a more complete body of knowledge relative to Internet marketing research within the fields of Information Systems and Marketing.
Chiou, W. C., Lin, C. C., & Perng, C. (2010). A strategic framework for website evaluation based on a review of the literature from 1995–2006. Information Management, 47 (5–6), 282–290.
Article Google Scholar
Cohen, J. (1960). A coefficient of agreement for nominal scales. Educational and Psychological Measurement, 20 (1), 37–46.
Corley, J. K., Jourdan, S. Z., & Rainer, R. K. (2011). Privacy research: application of content analysis to assess a contemporary area of research. International Journal of Electronic Marketing and Retailing, 4 (2/3), 129–150.
Google Scholar
Cook, T. D., & Campbell, D. T. (1976). The design and conduct of quasi-experiments and true experiments in field settings. In M. D. Dunnette (Ed.), Handbook of industrial and organizational psychology . Chicago: Rand McNally.
Cumbie, B. A., Jourdan, S. Z., Peachey, T. A., Dugo, T. M., & Craighead, C. W. (2005). Enterprise resource planning research: where are we now and where should we go from here? Journal of Information Technology Theory & Application, 7 (2), 21–36.
de Valck, K., van Bruggen, G. H., & Wierenga, B. (2009). Virtual communities: a marketing perspective. Decision Support Systems, 47 (3), 185–203. doi: 10.1016/j.dss.2009.02.008 .
Du, A. Y., Geng, X. J., Gopal, R. D., Ramesh, R., & Whinston, A. B. (2008). Topographically discounted Internet infrastructure resources: a panel study and econometric analysis. Information Technology and Management, 9 (2), 135–146. doi: 10.1007/s10799-007-0034-6 .
Friedman, T. L. (2006). The world is flat, release 2.0 . New York: Fartar Straus, and Giroux.
Hansen, H. R. (1995). Conceptual-framework and guidelines for the implementation of mass information-systems. Information Management, 28 (2), 125–142. doi: 10.1016/0378-7206(95)94021-4 .
Hofacker, C. F., Gleim, M. R., & Lawson, S. J. (2009). Revealed reader preference for marketing journals. Journal of the Academy of Marketing Science, 37 (2), 238–247.
Hwang, Y. (2009). The impact of uncertainty avoidance, social norms and innovativeness on trust and ease of use in electronic customer relationship management. Electronic Markets, 19 (2–3), 89–98. doi: 10.1007/s12525-009-0007-1 .
Jarvenpaa, S. L., & Todd, P. A. (1996). Consumer reactions to electronic shopping on the World Wide Web. International Journal of Electronic Commerce, 1 (2), 59–88.
Jourdan, Z., Rainer, R. K., Jr., & Marshall, T. (2008). Business intelligence: a framework for research categorization. Information Systems Management, 25 (2), 121–131.
Kiang, M. Y., Raghu, T. S., & Shang, K. H. M. (2000). Marketing on the internet - who can benefit from an online marketing approach? Decision Support Systems, 27 (4), 383–393.
Lee, S. M., Hwang, T., & Kim, J. (2007). An analysis of diversity in electronic commerce research. International Journal of Electronic Commerce, 12 (1), 31–67.
Loebbecke, C., Powell, P., & Weiss, T. (2010). Repeated use of online auctions: investigating individual seller motivations. Electronic Markets, 20 (2), 105–117.
McGrath, J. (1982). Dilemmatics: the study of research choices and dilemmas. In J. E. McGrath, J. Martin, & R. A. Kilka (Eds.), Judgment calls in research . Beverly Hills: SAGE Publications.
Moussa, S., & Touzani, M. (2010). Ranking marketing journals using the Google scholar-based hg-index. Journal of Informetrics, 4 (1), 107–117.
Neuendorf, K. A. (2002). The content analysis guidebook . Thousand Oaks: SAGE Publications.
Ngai, E., & Wat, F. (2002). A literature review and classification of electronic commerce research. Information Management, 39 (5), 415–429.
Novak, J., & Schwabe, G. (2009). Designing for reintermediation in the brick-and-mortar world: towards the travel agency of the future. Electronic Markets, 19 (1), 15–29. doi: 10.1007/s12525-009-0003-5 .
Otim, S., & Grover, V. (2010). E-commerce: a brand name’s curse. Electronic Markets, 20 (2), 147–160. doi: 10.1007/s12525-010-0039-6 .
Peffers, K., & Tang, Y. (2003). Identifying and evaluating the universe of outlets for information systems research: ranking the journals. Journal of Information Technology Theory and Application, 5(1), 63–84.
Pride, W. M., & Ferrell, O. C. (2007). Foundations of Marketing (2nd ed.). Boston, MA: Houghton Mifflin.
Polonsky, M. J., & Whitelaw, P. (2006). Amulti-dimensional examination of marketing journals by North American academics. Marketing Education Review, 16 (3), 59–72.
Ransbotham, S., & Mitra, S. (2009). Choice and chance: a conceptual model of paths to information security compromise. Information Systems Research, 20 (1), 121–139.
Scandura, T. A., & Williams, E. A. (2000). Research methodology in management: current practices, trends, and implications for future research. Academy of Management Journal, 43 (6), 1248–1264.
Smith, M. D., & Telang, R. (2009). Competing with free: the impact of movie broadcasts on DVD sales and internet piracy. MIS Quarterly, 33 (2), 321–338.
Sripalawat, J., Thongmak, M., & Ngramyarn, A. (2011). M-banking in metropolitan Bangkok and a comparison with other countries. The Journal of Computer Information Systems, 51 (3), 67–76.
Sutanto, J., Phang, C. W., Tan, C. H., & Lu, X. (2011). Dr. Jekyll vis-a-vis Mr. Hyde: Personality variation between virtual and real worlds. Information & Management, 48 (1), 19–26.
Weber, R. H. (1990). Basic content analysis (2nd ed.). Thousand Oaks: Sage Publications.
Webster, J., & Watson, R. T. (2002). Analyzing the past to prepare for the future: writing a literature review. MIS Quarterly, 26 (2), xiii–xxiii.
Westland, J. C., & Au, G. (1997). A comparison of shopping experiences across three competing digital retailing interfaces. International Journal of Electronic Commerce, 2 (2), 57–69.
Download references
Author information
Authors and affiliations.
Walker College of Business, Computer Information Systems, Appalachian State University, 2113 Raley Hall, ASU Box 32049, Boone, NC, 28608-2049, USA
J. Ken Corley II
IS & DS, School of Business, Auburn University at Montgomery, 310H Clement Hall, PO Box 244023, Montgomery, AL, 36124-4023, USA
Zack Jourdan & W. Rhea Ingram
You can also search for this author in PubMed Google Scholar
Corresponding author
Correspondence to J. Ken Corley II .
Additional information
Responsible Editor: Christopher Patrick Holland
Appendix A – data sample (411 information systems articles)
Abbasi, A., Chen, H. C., & Nunamaker, J. F. (2008). Stylometric Identification in Electronic Markets: Scalability and Robustness. Journal of Management Information Systems, 25 (1), 49–78. doi: 10.2753/mis0742-1222250103
Adam, S. (2002). A model of Web use in direct and online marketing strategy. Electronic Markets, 12 (4), 262–269.
Albrecht, C. C., Dean, D. L., & Hansen, J. V. (2005). Marketplace and technology standards for B2B e-commerce: progress, challenges, and the state of the art. Information & Management, 42 (6), 865–875. doi: 10.1016/j.im.2004.09.003
Allen, G., & Wu, J. A. (2010). How well do shopbots represent online markets? A study of shopbots’ vendor coverage strategy. European Journal of Information Systems, 19 (3), 257–272. doi: 10.1057/ejis.2010.6
Amblee, N., & Bui, T. (2008). Can brand reputation improve the odds of being reviewed on-line? International Journal of Electronic Commerce, 12 (3), 11–28.
Amir, Y., Awerbuch, B., & Borgstrom, R. S. (2000). A cost-benefit framework for online management of a metacomputing system. Decision Support Systems, 28 (1–2), 155–164. doi: 10.1016/s0167-9236(99)00081-0
Anckar, B., & Walden, P. (2000). Destination Maui? An exploratory assessment of the efficacy of self-booking in travel. Electronic Markets, 10 (2), 110–119.
Animesh, A., Ramachandran, V., & Viswanathan, S. (2010). Quality Uncertainty and the Performance of Online Sponsored Search Markets: An Empirical Investigation. Information Systems Research, 21 (1), 190–201. doi: 10.1287/isre.1080.0222
Animesh, A., Viswanathan, S., & Agarwal, R. (2011). Competing “Creatively” in Sponsored Search Markets: The Effect of Rank, Differentiation Strategy, and Competition on Performance. Information Systems Research, 22 (1), 153–169.
Antony, S., Lin, Z. X., & Xu, B. (2006). Determinants of escrow service adoption in consumer-to-consumer online auction market: An experimental study. Decision Support Systems, 42 (3), 1889–1900. doi: 10.1016/j.dss.2006.04.012
Apigian, C. H., Ragu-Nathan, B. S., & Ragu-Nathan, T. (2006). Strategic profiles and Internet Performance: An empirical investigation into the development of a strategic Internet system. Information & Management, 43 (4), 455–468.
Aron, R., & Clemons, E. K. (2001). Achieving the optimal balance between investment in quality and investment in self-promotion for information products. Journal of Management Information Systems, 18 (2), 65–88.
Arunkundram, R., & Sundararajan, A. (1998). An economic analysis of electronic secondary markets: installed base, technology, durability and firm profitability. Decision Support Systems, 24 (1), 3–16. doi: 10.1016/s0167-9236(98)00059-1
Ayanso, A., & Yoogalingam, R. (2009). Profiling Retail Web Site Functionalities and Conversion Rates: A Cluster Analysis. International Journal of Electronic Commerce, 14 (1), 79–113. doi: 10.2753/jec1086-4415140103
Ba, S., Stallaert, J., Whinston, A. B., & Zhang, H. (2005). Choice of transaction channels: The effects of product characteristics on market evolution. Journal of Management Information Systems, 21 (4), 173–197.
Bai, X. (2011). Predicting consumer sentiments from online text. Decision Support Systems, 50 (4), 732–742. doi: 10.1016/j.dss.2010.08.024
Bakos, J. Y., & Nault, B. R. (1997). Ownership and investment in electronic networks. Information Systems Research, 8 (4), 321–341. doi: 10.1287/isre.8.4.321
Bakos, Y., & Katsamakas, E. (2008). Design and ownership of two-sided networks: Implications for Internet platforms. Journal of Management Information Systems, 25 (2), 171–202. doi: 10.2753/mis0742-1222250208
Bakos, Y., Lucas, H. C., Oh, W., Simon, G., Viswanathan, S., & Weber, B. W. (2005). The impact of e-commerce on competition in the retail brokerage industry. Information Systems Research, 16 (4), 352–371. doi: 10.1287/isre.1050.0064
Bampo, M., Ewing, M. T., Mather, D. R., Stewart, D., & Wallace, M. (2008). The effects of the social structure of digital networks on viral marketing performance. Information Systems Research, 19 (3), 273–290.
Bapna, R., Chang, S. A., Goes, P., & Gupta, A. (2009). Overlapping online auctions: empirical characterization of bidder strategies and auction prices. MIS Quarterly, 33 (4), 763–783.
Bapna, R., Goes, P., & Gupta, A. (2003). Replicating online Yankee auctions to analyze auctioneers’ and bidders’ strategies. Information Systems Research, 14 (3), 244–268. doi: 10.1287/isre.14.3.244.16562
Bapna, R., Jank, W., & Shmueli, G. (2008). Price formation and its dynamics in online auctions. Decision Support Systems, 44 (3), 641–656. doi: 10.1016/j.dss.2007.09.004
Barrot, C., Albers, S., Skiera, B., & Schafers, B. (2010). Vickrey vs. eBay: Why Second-Price Sealed-Bid Auctions Lead to More Realistic Price-Demand Functions. International Journal of Electronic Commerce, 14 (4), 7–38. doi: 10.2753/jec1086-4415140401
Basu, A., & Muylle, S. (2003). Online support for commerce processes by web retailers* 1. Decision Support Systems, 34 (4), 379–395.
Beech, J., Chadwick, S., & Tapp, A. (2000). Scoring with the Net-the Cybermarketing of English Football Clubs. Electronic Markets, 10 (3), 176–184.
Belanger, F., Hiller, J. S., & Smith, W. J. (2002). Trustworthiness in electronic commerce: the role of privacy, security, and site attributes. The Journal of Strategic Information Systems, 11 (3–4), 245–270.
Bell, D., de Cesare, S., Iacovelli, N., Lycett, M., & Merico, A. (2007). A framework for deriving semantic web services. Information Systems Frontiers, 9 (1), 69–84. doi: 10.1007/s10796-006-9018-z
Benbunan-Fich, R., & Fich, E. M. (2004). Effects of Web traffic announcements on firm value. International Journal of Electronic Commerce, 8 (4), 161–181.
Bergen, M. E., Kauffman, R. J., & Lee, D. (2005). Beyond the hype of frictionless markets: Evidence of heterogeneity in price rigidity on the Internet. Journal of Management Information Systems, 22 (2), 57–89.
Bhargava, H. K., & Choudhary, V. (2004). Economics of an information intermediary with aggregation benefits. Information Systems Research, 15 (1), 22–36. doi: 10.1287/isre.1040.0014
Bhatnagar, A., & Papatla, P. (2001). Identifying locations for targeted advertising on the Internet. International Journal of Electronic Commerce, 5 (3), 23–44.
Bhattacharjee, S., Gopal, R., Lertwachara, K., & Marsden, J. R. (2006). Whatever happened to payola? An empirical analysis of online music sharing. Decision Support Systems, 42 (1), 104–120.
Blount, Y. (2011). Employee management and service provision: a conceptual framework. Information Technology & People, 24 (2), 134–157. doi: 10.1108/09593841111137331
Bock, G. W., Lee, S. Y. T., & Li, H. Y. (2007). Price comparison and price dispersion: products and retailers at different Internet maturity stages. International Journal of Electronic Commerce, 11 (4), 101–124.
Bockstedt, J. C., Kauffman, R. J., & Riggins, F. J. (2006). The move to artist-led on-line music distribution: A theory-based assessment and prospects for structural changes in the digital music market. International Journal of Electronic Commerce, 10 (3), 7–38. doi: 10.2753/jec1086-4415100301
Bolton, G., Loebbecke, C., & Ockenfels, A. (2008). Does competition promote trust and trustworthiness in online trading? An experimental study. Journal of Management Information Systems, 25 (2), 145–169. doi: 10.2753/mis0742-1222250207
Browne, G. J., Durrett, J. R., & Wetherbe, J. C. (2004). Consumer reactions toward clicks and bricks: investigating buying behaviour on-line and at stores. Behaviour & Information Technology, 23 (4), 237–245. doi: 10.1080/01449290410001685411
Bunduchi, R. (2005). Business relationships in Internet-based electronic markets: the role of goodwill trust and transaction costs. Information Systems Journal, 15 (4), 321–341. doi: 10.1111/j.1365-2575.2005.00199.x
Burgess, S., Sellitto, C., Cox, C., & Buultjens, J. (2009). Trust perceptions of online travel information by different content creators: Some social and legal implications. Information Systems Frontiers , 1–15.
Byers, R. E., & Lederer, P. J. (2001). Retail bank services strategy: A model of traditional, electronic, and mixed distribution choices. Journal of Management Information Systems, 18 (2), 133–156.
Cao, Q., Duan, W., & Gan, Q. (2010). Exploring Determinants of Voting for the. Decision Support Systems .
Cao, Y., Gruca, T. S., & Klemz, B. R. (2003). Internet pricing, price satisfaction, and customer satisfaction. International Journal of Electronic Commerce, 8 (2), 31–50.
Castañeda, J. A., Muñoz-Leiva, F., & Luque, T. (2007). Web Acceptance Model (WAM): Moderating effects of user experience. Information & Management, 44 (4), 384–396.
Cazier, J. A., Shao, B. B. M., & Louis, R. D. S. (2007). Sharing information and building trust through value congruence. Information Systems Frontiers, 9 (5), 515–529.
Chang, H. H., & Chen, S. W. (2009). Consumer perception of interface quality, security, and loyalty in electronic commerce. Information & Management, 46 (7), 411–417.
Chang, M. K., Cheung, W. M., & Lai, V. S. (2005). Literature derived reference models for the adoption of online shopping. Information & Management, 42 (4), 543–559. doi: 10.1016/s0378-7206(04)00051-5
Changa, K. C., Jackson, J., & Grover, V. (2003). E-commerce and corporate strategy: an executive perspective. Information & Management, 40 (7), 663–675. doi: 10.1016/s0378-7206(02)00095-2
Chellappa, R. K., & Kumar, K. R. (2005). Examining the role of “Free” product-augmenting Online services in pricing and customer retention strategies. Journal of Management Information Systems, 22 (1), 355–377.
Chellappa, R. K., & Shivendu, S. (2003). Economic implications of variable technology standards for movie piracy in a global context. Journal of Management Information Systems, 20 (2), 137–168.
Chellappa, R. K., Sin, R. G., & Siddarth, S. (2011). Price Formats as a Source of Price Dispersion: A Study of Online and Offline Prices in the Domestic US Airline Markets. Information Systems Research, 22 (1), 83–98. doi: 10.1287/isre.1090.0264
Chen, C. C., Wu, C. S., & Wu, R. C. F. (2006). e-Service enhancement priority matrix: The case of an IC foundry company. Information & Management, 43 (5), 572–586. doi: 10.1016/j.im.2006.01.002
Chen, L. D., Gillenson, M. L., & Sherrell, D. L. (2002). Enticing online consumers: an extended technology acceptance perspective. Information & Management, 39 (8), 705–719. doi: 10.1016/s0378-7206(01)00127-6
Chen, P. Y., & Hitt, L. M. (2002). Measuring switching costs and the determinants of customer retention in Internet-enabled businesses: A study of the Online brokerage industry. Information Systems Research, 13 (3), 255–274. doi: 10.1287/isre.13.3.255.78
Cheng, F. F., & Wu, C. S. (2010). Debiasing the framing effect: The effect of warning and involvement. Decision Support Systems, 49 (3), 328–334.
Cheng, H. K., & Dogan, K. (2008). Customer-centric marketing with Internet coupons. Decision Support Systems, 44 (3), 606–620. doi: 10.1016/j.dss.2007.09.001
Cheng, T. C. E., Lam, D. Y. C., & Yeung, A. C. L. (2006). Adoption of Internet banking: An empirical study in Hong Kong. Decision Support Systems, 42 (3), 1558–1572. doi: 10.1016/j.dss.2006.01.002
Cheng, Z., & Nault, B. R. (2007). Internet channel entry: retail coverage and entry cost advantage. Information Technology & Management, 8 (2), 111–132. doi: 10.1007/s10799-007-0015-9
Cheung, K. W., Kwok, J. T., Law, M. H., & Tsui, K. C. (2003). Mining customer product rating for personalized marketing. Decision Support Systems, 35 (2), 231–243. doi: 10.1016/s0167-9236(02)00108-2
Chiou, W. C., Lin, C. C., & Perng, C. (2010). A strategic framework for website evaluation based on a review of the literature from 1995–2006. Information & Management, 47 (5–6), 282–290.
Chircu, A. M., & Kauffman, R. J. (2000a). Limits to value in electronic commerce-related IT investments. Journal of Management Information Systems, 17 (2), 59–80.
Chircu, A. M., & Kauffman, R. J. (2000b). Reintermediation strategies in business-to-business electronic commerce. International Journal of Electronic Commerce, 4 (4), 7–42.
Chircu, A. M., & Mahajan, V. (2006). Managing electronic commerce retail transaction costs for customer value. Decision Support Systems, 42 (2), 898–914. doi: 10.1016/j.dss.2005.07.011
Cho, V. (2006a). Factors in the adoption of third-party B2B portals in the textile industry. Journal of Computer Information Systems, 46 (3), 18–31.
Cho, V. (2006b). A study of the roles of trusts and risks in information-oriented online legal services using an integrated model. Information & Management, 43 (4), 502–520. doi: 10.1016/j.im.2005.12.002
Choi, J., Lee, S. M., & Soriano, D. R. (2009). An empirical study of user acceptance of fee-based online content. Journal of Computer Information Systems, 49 (3), 60–70.
Choudhary, V. (2010). Use of pricing schemes for differentiating information goods. Information Systems Research, 21 (1), 78.
Choudhury, V., & Karahanna, E. (2008). The relative advantage of electronic channels: A multidimensional view. MIS Quarterly, 32 (1), 179–200.
Christiaanse, E., Van Diepen, T., & Damsgaard, J. (2004). Proprietary versus Internet technologies and the adoption and impact of electronic marketplaces. Journal of Strategic Information Systems, 13 (2), 151–165. doi: 10.1016/j.jsis.2004.02.004
Chua, C. E. H., & Wareham, J. (2008). Parasitism and Internet auction fraud: An exploration. Information and Organization, 18 (4), 303–333. doi: 10.1016/j.infoandorg.2008.01.001
Chua, C. E. H., Wareham, J., & Robey, D. (2007). The role of online trading communities in managing Internet auction fraud. MIS Quarterly, 31 (4), 759–781.
Chun, S. H., & Kim, J. C. (2005). Pricing strategies in B2C electronic commerce: analytical and empirical approaches. Decision Support Systems, 40 (2), 375–388. doi: 10.1016/j.dss.2004.04.012
Clemons, E. K. (2009a). Business models for monetizing Internet applications and Web sites: Experience, theory, and predictions. Journal of Management Information Systems, 26 (2), 15–41.
Clemons, E. K. (2009b). The complex problem of monetizing virtual electronic social networks. Decision Support Systems, 48 (1), 46–56.
Crowston, K., & Myers, M. D. (2004). Information technology and the transformation of industries: three research perspectives. Journal of Strategic Information Systems, 13 (1), 5–28. doi: 10.1016/j.jsis.2004.02.001
Currie, W. L., & Parikh, M. A. (2006). Value creation in web services: An integrative model. Journal of Strategic Information Systems, 15 (2), 153–174. doi: 10.1016/j.jsis.2005.10.001
Cyr, D., Bonanni, C., Bowes, J., & Ilsever, J. (2005). Beyond trust: Web site design preferences across cultures. Journal of Global Information Management, 13 (4), 25.
Dai, Q. Z., & Kauffman, R. J. (2002). Business models for Internet-based B2B electronic markets. International Journal of Electronic Commerce, 6 (4), 41–72.
Datta, P. (2011). A preliminary study of ecommerce adoption in developing countries. Information Systems Journal, 21 (1), 3–32. doi: 10.1111/j.1365-2575.2009.00344.x
Datta, P., & Chatterjee, S. (2008). The economics and psychology of consumer trust in intermediaries in electronic markets: the EM-Trust Framework. European Journal of Information Systems, 17 (1), 12–28. doi: 10.1057/palgrave.ejis.3000729
Davis, A., & Khazanchi, D. (2008). An empirical study of online word of mouth as a predictor for multi product category e-Commerce Sales. Electronic Markets, 18 (2).
de Valck, K., van Bruggen, G. H., & Wierenga, B. (2009). Virtual communities: A marketing perspective. Decision Support Systems, 47 (3), 185–203. doi: 10.1016/j.dss.2009.02.008
De Wulf, K., Schillewaert, N., Muylle, S., & Rangarajan, D. (2006). The role of pleasure in web site success. Information & Management, 43 (4), 434–446.
Dehning, B., Richardson, V. J., Urbaczewski, A., & Wells, J. D. (2004). Reexamining the value relevance of e-commerce initiatives. Journal of Management Information Systems, 21 (1), 55–82.
Dellaert, B. G. C., & Dabholkar, P. A. (2009). Increasing the attractiveness of mass customization: The role of complementary on-line services and range of options. International Journal of Electronic Commerce, 13 (3), 43–70.
Dellarocas, C., Gao, G. D., & Narayan, R. (2010). Are consumers more likely to contribute online reviews for hit or niche products? Journal of Management Information Systems, 27 (2), 127–157. doi: 10.2753/mis0742-1222270204
Devaraj, S., Fan, M., & Kohli, R. (2006). Examination of online channel preference: Using the structure-conduct-outcome framework. Decision Support Systems, 42 (2), 1089–1103. doi: 10.1016/j.dss.2005.09.004
Dewan, R., Jing, B., & Seidmann, A. (2000). Adoption of Internet-based product customization and pricing strategies. Journal of Management Information Systems, 17 (2), 9–28.
Dewan, R. M., & Freimer, M. L. (2003). Consumers prefer bundled add-ins. Journal of Management Information Systems, 20 (2), 99–111.
Dewan, R. M., Freimer, M. L., Seidmann, A., & Zhang, J. (2004). Web portals: Evidence and analysis of media concentration. Journal of Management Information Systems, 21 (2), 181–199.
Dewan, S., & Ren, F. (2007). Risk and return of information technology initiatives: Evidence from electronic commerce announcements. Information Systems Research, 18 (4), 370–394. doi: 10.1287/isre.1070.0120
Dhar, V., & Ghose, A. (2010). Sponsored Search and Market Efficiency. Information Systems Research, 21 (4), 760–772. doi: 10.1287/isre.1100.0315
Dos Santos, B. L., & Peffers, K. (1998). Competitor and vendor influence on the adoption of innovative applications in electronic commerce. Information & Management, 34 (3), 175–184. doi: 10.1016/s0378-7206(98)00053-6
Dou, W. Y., Lim, K. H., Su, C. T., Zhou, N., & Cui, N. (2010). Brand positioning strategy using search engine marketing. MIS Quarterly, 34 (2), 261–279.
Du, A. Y., Geng, X. J., Gopal, R. D., Ramesh, R., & Whinston, A. B. (2008). Topographically discounted Internet infrastructure resources: a panel study and econometric analysis. Information Technology & Management, 9 (2), 135–146. doi: 10.1007/s10799-007-0034-6
Du, T. C., Li, E. Y., & Wei, E. (2005). Mobile agents for a brokering service in the electronic marketplace. Decision Support Systems, 39 (3), 371–383.
Duan, W., Gu, B., & Whinston, A. B. (2009). Informational cascades and software adoption on the internet: an empirical investigation. MIS Quarterly, 33 (1), 23–48.
Duan, W. J. (2010). Analyzing the impact of intermediaries in electronic markets: an empirical investigation of online consumer-to-consumer (C2C) auctions. Electronic Markets, 20 (2), 85–93. doi: 10.1007/s12525-010-0034-y
Dutta, A. (2001). Business planning for network services: A systems thinking approach. Information Systems Research, 12 (3), 260–285. doi: 10.1287/isre.12.3.260.9713
Dwivedi, Y. K., Papazafeiropoulou, A., Brinkman, W. P., & Lal, B. (2010). Examining the influence of service quality and secondary influence on the behavioural intention to change Internet service provider. Information Systems Frontiers, 12 (2), 207–217. doi: 10.1007/s10796-008-9074-7
Easley, R. F., Wood, C. A., & Barkataki, S. (2010). Bidding Patterns, Experience, and Avoiding the Winner’s Curse in Online Auctions. Journal of Management Information Systems, 27 (3), 241–268. doi: 10.2753/mis0742-1222270309
Edelman, B., & Ostrovsky, M. (2007). Strategic bidder behavior in sponsored search auctions. Decision Support Systems, 43 (1), 192–198. doi: 10.1016/j.dss.2006.08.008
El Sawy, O. A., Malhotra, A., Gosain, S., & Young, K. M. (1999). IT-intensive value innovation in the electronic economy: Insights from Marshall Industries. MIS Quarterly, 23 (3), 305–335.
Erat, P., Desouza, K. C., Schafer-Jugel, A., & Kurzawa, M. (2006). Business customer communities and knowledge sharing: exploratory study of critical issues. European Journal of Information Systems, 15 (5), 511–524. doi: 10.1057/palgrave.ejis.3000643
Even, A., Shankaranarayanan, G., & Berger, P. D. (2010). Evaluating a model for cost-effective data quality management in a real-world CRM setting. Decision Support Systems .
Flavián, C., Guinalíu, M., & Gurrea, R. (2006). The role played by perceived usability, satisfaction and consumer trust on website loyalty. Information & Management, 43 (1), 1–14.
Forman, C., Ghose, A., & Wiesenfeld, B. (2008). Examining the relationship between reviews and sales: The role of reviewer identity disclosure in electronic markets. Information Systems Research, 19 (3), 291–313. doi: 10.1287/isre.1080.0193
Gallaugher, J. M., Auger, P., & BarNir, A. (2001). Revenue streams and digital content providers: an empirical investigation. Information & Management, 38 (7), 473–485. doi: 10.1016/s0378-7206(00)00083-5
Gao, S. J., Wang, H. Q., Xu, D. M., & Wang, Y. F. (2007). An intelligent agent-assisted decision support system for family financial planning. Decision Support Systems, 44 (1), 60–78. doi: 10.1016/j.dss.2007.03.001
Garcia, R., & Gil, R. (2008). A web ontology for copyright contract management. International Journal of Electronic Commerce, 12 (4), 99–113. doi: 10.2753/jec1086-4415120404
Gauzente, C. (2009). Information search and paid results—proposition and test of a hierarchy-of-effect model. Electronic Markets, 19 (2), 163–177.
Gefen, D., Rose, G. M., Warkentin, M., & Pavlou, P. A. (2005). Cultural diversity and trust in IT adoption: A comparison of potential e-voters in the USA and South Africa. Journal of Global Information Management, 13 (1), 54–78. doi: 10.4018/jgim.2005010103
Ghose, A. (2009). Internet exchanges for used goods: an empirical analysis of trade patterns and adverse selection. MIS Quarterly, 33 (2), 263–291.
Ghose, A., Mukhopadhyay, T., & Rajan, U. (2007). The impact of Internet referral services on a supply chain. Information Systems Research, 18 (3), 300–319. doi: 10.1287/isre.1070.0130
Ghose, A., Smith, M. D., & Telang, R. (2006). Internet exchanges for used books: An empirical analysis of product cannibalization and welfare impact. Information Systems Research, 17 (1), 3–19. doi: 10.1287/isre.1050.0072
Ghose, A., & Yao, Y. L. (2011). Using Transaction Prices to Re-Examine Price Dispersion in Electronic Markets. Information Systems Research, 22 (2), 269–288. doi: 10.1287/isre.1090.0252
Glover, S., & Benbasat, I. (2010). A Comprehensive Model of Perceived Risk of E-Commerce Transactions. International Journal of Electronic Commerce, 15 (2), 47–78.
Gopal, R. D., Ramesh, R., & Whinston, A. B. (2003). Microproducts in a digital economy: Trading small, gaining large. International Journal of Electronic Commerce, 8 (2), 9–29.
Gopal, R. D., Tripathi, A. K., & Walter, Z. D. (2006). Economics of first-contact email advertising. Decision Support Systems, 42 (3), 1366–1382.
Gorman, M. F., Salisbury, W. D., & Brannon, I. (2009). Who wins when price information is more ubiquitous? An experiment to assess how infomediaries influence price. Electronic Markets, 19 (2–3), 151–162. doi: 10.1007/s12525-009-0009-z
Granados, N., Gupta, A., & Kauffman, R. J. (2008). Designing online selling mechanisms: Transparency levels and prices. Decision Support Systems, 45 (4), 729–745. doi: 10.1016/j.dss.2007.12.005
Granados, N., Gupta, A., & Kauffman, R. J. (2010). Information Transparency in Business-to-Consumer Markets: Concepts, Framework, and Research Agenda. Information Systems Research, 21 (2), 207–226. doi: 10.1287/isre.1090.0249
Granados, N. F., Gupta, A., & Kauffman, R. J. (2006). The impact of IT on market information and transparency: A unified theoretical framework. Journal of the Association for Information Systems, 7 (3), 148–178.
Granados, N. F., Kauffman, R. J., & King, B. (2008). How has electronic travel distribution been transformed? A test of the theory of newly vulnerable markets. Journal of Management Information Systems, 25 (2), 73–95. doi: 10.2753/mis0742-1222250204
Gregg, D. G., & Scott, J. E. (2006). The role of reputation systems in reducing on-line auction fraud. International Journal of Electronic Commerce, 10 (3), 95–120. doi: 10.2753/jec1086-4415100304
Gregor, S., & Jones, K. (1999). Beef producers online: Diffusion theory applied. Information Technology & People, 12 (1), 71–85.
Grenci, I. T. (2004). An adaptable customer decision support system for custom configurations. Journal of Computer Information Systems, 45 (2), 56–62.
Grover, V., & Saeed, K. A. (2004). Strategic orientation and performance of Internet-based businesses. Information Systems Journal, 14 (1), 23–42. doi: 10.1111/j.1365-2575.2004.00161.x
Gundepudi, P., Rudi, N., & Seidmann, A. (2001). Forward versus spot buying of information goods. Journal of Management Information Systems, 18 (2), 107–131.
Gupta, A., Su, B., & Walter, Z. (2004). Risk profile and consumer shopping behavior in electronic and traditional channels. Decision Support Systems, 38 (3), 347–367.
Gupta, A., Su, B. C., & Walter, Z. (2004). An empirical study of consumer switching from traditional to electronic channels: A purchase-decision process perspective. International Journal of Electronic Commerce, 8 (3), 131–161.
Gupta, S., & Kim, H. W. (2007). The moderating effect of transaction experience on the decision calculus in on-line repurchase. International Journal of Electronic Commerce, 12 (1), 127–158.
Hansen, H. R. (1995). Conceptual-framework and guidelines for the implementation of mass information-systems. Information & Management, 28 (2), 125–142. doi: 10.1016/0378-7206(95)94021-4
Harrison McKnight, D., Choudhury, V., & Kacmar, C. (2002). The impact of initial consumer trust on intentions to transact with a web site: a trust building model. The Journal of Strategic Information Systems, 11 (3–4), 297–323.
Harrison, T., & Waite, K. (2006). A time-based assessment of the influences, uses and benefits of intermediary website adoption. Information & Management, 43 (8), 1002–1013.
Hassanein, K., & Head, M. (2005). The impact of infusing social presence in the web interface: An investigation across product types. International Journal of Electronic Commerce, 10 (2), 31–55.
Hayne, S. C., Bugbee, B., & Wang, H. N. (2010). Bidder behaviours on eBay: collectibles and commodities. Electronic Markets, 20 (2), 95–104. doi: 10.1007/s12525-010-0036-9
Hempel, P. S., & Kwong, Y. K. (2001). B2B e-Commerce in emerging economies: i-metal.com’s non-ferrous metals exchange in China. Journal of Strategic Information Systems, 10 (4), 335–355. doi: 10.1016/s0963-8687(01)00058-0
Hennig-Thurau, T., & Walsh, G. (2003). Electronic word-of-mouth: Motives for and consequences of reading customer articulations on the Internet. International Journal of Electronic Commerce, 8 (2), 51–74.
Hinz, O., Hann, I. H., & Spann, M. (2011). Price discrimination in e-commerce? an examination of dynamic pricing in name-your-own price markets. MIS Quarterly, 35 (1), 81–98.
Hinz, O., & Spann, M. (2008). The impact of information diffusion on bidding behavior in secret reserve price auctions. Information Systems Research, 19 (3), 351–368.
Hinz, O., & Spann, M. (2010). Managing information diffusion in Name-Your-Own-Price auctions. Decision Support Systems, 49 (4), 474–485.
Ho, K. K. W., Yoo, B., Yu, S., & Tam, K. Y. (2007). The effect of culture and product categories on the level of use of buy-it-now (BIN) auctions by sellers. Journal of Global Information Management, 15 (4), 1–19. doi: 10.4018/jgim.2007100101
Holsapple, C. W., & Wu, J. (2008). Building effective online game websites with knowledge-based trust. Information Systems Frontiers, 10 (1), 47–60.
Hong, S. Y., & Kim, J. (2004). Architectural criteria for website evaluation - conceptual framework and empirical validation. Behaviour & Information Technology, 23 (5), 337–357. doi: 10.1080/01449290410001712753
Hou, H. P., Hu, M. Y., Chen, L., & Choi, J. Y. (2011). An enhanced model framework of personalized material flow services. Information Technology & Management, 12 (2), 149–159. doi: 10.1007/s10799-011-0099-0
Hou, J. W., & Blodgett, J. (2010). Market structure and quality uncertainty: a theoretical framework for online auction research. Electronic Markets, 20 (1), 21–32. doi: 10.1007/s12525-010-0026-y
Hsu, C. L., & Lin, J. C. C. (2008). Acceptance of blog usage: The roles of technology acceptance, social influence and knowledge sharing motivation. Information & Management, 45 (1), 65–74.
Hsu, C. L., & Lu, H. P. (2004). Why do people play on-line games? An extended TAM with social influences and flow experience. Information & Management, 41 (7), 853–868. doi: 10.1016/j.im.2003.08.014
Hu, N., Bose, I., Gao, Y., & Liu, L. (2010). Manipulation in digital word-of-mouth: A reality check for book reviews. Decision Support Systems .
Hu, X., Wu, G., Wu, Y., & Zhang, H. (2010). The effects of Web assurance seals on consumers’ initial trust in an online vendor: A functional perspective. Decision Support Systems, 48 (2), 407–418.
Hu, X. R., Lin, Z. X., Whinston, A. B., & Zhang, H. (2004). Hope or hype: On the viability of escrow services as trusted third parties in online auction environments. Information Systems Research, 15 (3), 236–249. doi: 10.1287/isre.1040.0027
Huang, J. H., Jiang, X. M., & Tang, Q. A. (2009). An e-commerce performance assessment model: Its development and an initial test on e-commerce applications in the retail sector of China. Information & Management, 46 (2), 100–108. doi: 10.1016/j.im.2008.12.003
Huang, M. H. (2003). Modeling virtual exploratory and shopping dynamics: an environmental psychology approach. Information & Management, 41 (1), 39–47.
Huang, M. H. (2005). Web performance scale. Information & Management, 42 (6), 841–852.
Hui, K. L., & Tam, K. Y. (2002). Software functionality: A game theoretic analysis. Journal of Management Information Systems, 19 (1), 151–184.
Hui, W., Yoo, B., & Tam, K. Y. (2008). Economics of shareware: How do uncertainty and piracy affect shareware quality and brand premium? Decision Support Systems, 44 (3), 580–594. doi: 10.1016/j.dss.2007.07.009
Huizingh, E. (2000). The content and design of web sites: an empirical study. Information & Management, 37 (3), 123–134.
Hwang, Y. (2009). The impact of uncertainty avoidance, social norms and innovativeness on trust and ease of use in electronic customer relationship management. Electronic Markets, 19 (2–3), 89–98. doi: 10.1007/s12525-009-0007-1
Jih, W. J. K., & Lee, S. F. (2003). An exploratory analysis of relationships between cellular phone uses shopping motivators and lifestyle indicators. Journal of Computer Information Systems, 44 (2), 65–73.
Joh, Y. H., & Lee, J. K. (2003). Buyer’s customized directory management over sellers’ e-catalogs: logic programming approach. Decision Support Systems, 34 (2), 197–212.
Joo, J. (2007). An empirical study on the relationship between customer value and repurchase intention in Korean Internet shopping malls. Journal of Computer Information Systems, 48 (1), 53–62.
Josang, A., Ismail, R., & Boyd, C. (2007). A survey of trust and reputation systems for online service provision. Decision Support Systems, 43 (2), 618–644. doi: 10.1016/j.dss.2005.05.019
Jukic, N., Jukic, B., Meamber, L., & Nezlek, G. (2002). Employing a multilevel secure approach in CRM systems. Journal of Information Technology Theory and Application, 4 (2), 4.
Junglas, I. A., Johnson, N. A., & Spitzmuller, C. (2008). Personality traits and concern for privacy: an empirical study in the context of location-based services. European Journal of Information Systems, 17 (4), 387–402. doi: 10.1057/ejis.2008.29
Juul, N. C., & Jorgensen, N. (2003). The security hole in WAP: An analysis of the network and business rationales underlying a failure. International Journal of Electronic Commerce, 7 (4), 73–92.
Kagie, M., van Wezel, M., & Groenen, P. J. F. (2008). A graphical shopping interface based on product attributes. Decision Support Systems, 46 (1), 265–276.
Kalanidhi, S. (2001). Value creation in a network: The role of pricing and revenue optimization and enterprise profit optimization (TM). Information Systems Frontiers, 3 (4), 465–470. doi: 10.1023/a:1012828921804
Kamssu, A. J., Reithel, B. J., & Ziegelmayer, J. L. (2003). Information technology and financial performance: The impact of being an Internet-dependent firm on stock returns. Information Systems Frontiers, 5 (3), 279–288. doi: 10.1023/a:1025649311259
Kannan, P., & Kopalle, P. K. (2001). Dynamic pricing on the Internet: Importance and implications for consumer behavior. International Journal of Electronic Commerce, 5 (3), 63–83.
Karageorgos, A., Thompson, S., & Mehandjiev, N. (2002). Agent-based system design for B2B electronic commerce. International Journal of Electronic Commerce, 7 (1), 59–90.
Karuga, G. G., Khraban, A. M., Nair, S. K., & Rice, D. O. (2001). AdPalette: an algorithm for customizing online advertisements on the fly. Decision Support Systems, 32 (2), 85–106.
Kassim, N. M., & Abdullah, N. A. (2008). Customer loyalty in e-Commerce settings: An empirical study. Electronic Markets, 18 (3), 275–290.
Kauffman, R. J., & Lee, D. (2010). A multi-Level theory approach to understanding price rigidity in Internet retailing. Journal of the Association for Information Systems, 11 (6), 303–338.
Kauffman, R. J., Lee, D., Lee, J., & Yoo, B. (2009). A hybrid firm’s pricing strategy in Electronic Commerce under channel migration. International Journal of Electronic Commerce, 14 (1), 11–54. doi: 10.2753/jec1086-4415140101
Kauffman, R. J., & Walden, E. A. (2001). Economics and electronic commerce: survey and research directions. International Journal of Electronic Commerce, 5 (4), 5–117.
Kauffman, R. J., & Wang, B. (2001). New buyers’ arrival under dynamic pricing market microstructure: The case of group-buying discounts on the Internet. Journal of Management Information Systems, 18 (2), 157–188.
Kavassalis, P., Bailey, J. P., & Lee, T. Y. (2000). Open-layered networks: the growing importance of market coordination. Decision Support Systems, 28 (1–2), 137–153. doi: 10.1016/s0167-9236(99)00080-9
Kavassalis, P., Spyropoulou, N., Drossos, D., Mitrokostas, E., Gikas, G., & Hatzistamatiou, A. (2003). Mobile permission marketing: framing the market inquiry. International Journal of Electronic Commerce, 8 (1), 55–79.
Kayhan, V. O., McCart, J. A., & Bhattacherjee, A. (2009). An empirical study of cross-listing in online auctions. Journal of Computer Information Systems, 49 (3), 76–80.
Kayhan, V. O., McCart, J. A., & Bhattacherjee, A. (2010). Cross-bidding in simultaneous online auctions: Antecedents and consequences. Information & Management, 47 (7–8), 325–332. doi: 10.1016/j.im.2010.07.001
Keating, B. W., Quazi, A. M., & Kriz, A. (2009). Financial risk and its impact on new purchasing behavior in the online retail setting. Electronic Markets, 19 (4), 237–250. doi: 10.1007/s12525-009-0021-3
Khouja, M., Hadzikadic, M., Rajagopalan, H. K., & Tsay, L. S. (2008). Application of complex adaptive systems to pricing of reproducible information goods. Decision Support Systems, 44 (3), 725–739. doi: 10.1016/j.dss.2007.10.005
Kiang, M. Y., Raghu, T. S., & Shang, K. H. M. (2000). Marketing on the Internet - who can benefit from an online marketing approach? Decision Support Systems, 27 (4), 383–393. doi: 10.1016/s0167-9236(99)00062-7
Kim, B., Barua, A., & Whinston, A. B. (2002). Virtual field experiments for a digital economy: A new research methodology for exploring an information economy. Decision Support Systems, 32 (3), 215–231.
Kim, H. W., Chan, H. C., & Gupta, S. (2007). Value-based adoption of mobile Internet: An empirical investigation. Decision Support Systems, 43 (1), 111–126. doi: 10.1016/j.dss.2005.05.009
Kim, J., Jung, L., Han, K., & Lee, M. (2002). Businesses as buildings: Metrics for the architectural quality of Internet businesses. Information Systems Research, 13 (3), 239–254. doi: 10.1287/isre.13.3.239.79
Kim, J. W., Lee, B. H., Shaw, M. J., Chang, H. L., & Nelson, M. (2001). Application of decision-tree induction techniques to personalized advertisements on Internet storefronts. International Journal of Electronic Commerce, 5 (3), 45–62.
Kim, K., Kim, G. M., & Kil, E. S. (2009). Measuring the compatibility factors in mobile entertainment service adoption. Journal of Computer Information Systems, 50 (1), 141–148.
Kim, M. S., & Ahn, J. H. (2006). Comparison of trust sources of an online market-maker in the e-marketplace: Buyer’s and seller’s perspectives. Journal of Computer Information Systems, 47 (1), 84–94.
Kim, Y. (2005). The effects of buyer and product traits with seller reputation on price premiums in e-auction. Journal of Computer Information Systems, 46 (1), 79–91.
King, R. C., Sen, R., & Xia, M. (2004). Impact of Web-based e-commerce on channel strategy in retailing. International Journal of Electronic Commerce, 8 (3), 103–130.
Ko, I. S., & Leem, C. S. (2004). An Improvement of Response Speed for Electronic Commerce Systems. Information Systems Frontiers, 6 (4), 313–323.
Kocas, C. (2002). Evolution of prices in electronic markets under diffusion of price-comparison shopping. Journal of Management Information Systems, 19 (3), 99–119.
Kocas, C. (2005). A model of Internet pricing under price-comparison shopping. International Journal of Electronic Commerce, 10 (1), 111–134.
Koivumäki, T. (2002). Consumer attitudes and mobile travel portal. Electronic Markets, 12 (1), 47–57.
Komiak, P., Komiak, S. Y. X., & Imhof, M. (2008). Conducting international business at eBay: The Determinants of success of e Stores. Electronic Markets, 18 (2).
Koufaris, M. (2002). Applying the technology acceptance model and flow theory to online consumer behavior. Information Systems Research, 13 (2), 205–223. doi: 10.1287/isre.13.2.205.83
Kowtha, N. R., & Choon, T. W. I. (2001). Determinants of website development: a study of electronic commerce in Singapore. Information & Management, 39 (3), 227–242.
Kraemer, K. L., & Dedrick, J. (2002). Strategic use of the Internet and e-commerce: Cisco Systems. Journal of Strategic Information Systems, 11 (1), 5–29. doi: 10.1016/s0963-8687(01)00056-7
Kumar, C., Norris, J. B., & Sun, Y. (2009). Location and time do matter: A long tail study of website requests. Decision Support Systems, 47 (4), 500–507. doi: 10.1016/j.dss.2009.04.015
Kumar, K., & Becerra-Fernandez, I. (2007). Interaction technology: Speech act based information technology support for building collaborative relationships and trust. Decision Support Systems, 43 (2), 584–606. doi: 10.1016/j.dss.2005.05.017
Kwan, I. S. Y., Fong, J., & Wong, H. (2005). An e-customer behavior model with online analytical mining for Internet marketing planning. Decision Support Systems, 41 (1), 189–204.
Kwon, O. (2010). A pervasive P3P-based negotiation mechanism for privacy-aware pervasive e-commerce. Decision Support Systems .
Lagrosen, S. (2003). Online service marketing and delivery: the case of Swedish museums. Information Technology & People, 16 (2), 132–156.
Lahiri, A., Dewan, R. M., & Freimer, M. (2010). The disruptive effect of open platforms on markets for wireless services. Journal of Management Information Systems, 27 (3), 81–110.
Le, T. T. (2002). Pathways to leadership for business-to-business electronic marketplaces. Electronic Markets, 12 (2), 112–119.
Le, T. T., Rao, S. S., & Truong, D. (2004). Industry-sponsored marketplaces: a platform for supply chain integration or a vehicle for market aggregation? Electronic Markets, 14 (4), 295–307.
Lee, H. G., Westland, J. C., & Hong, S. (1999). The impact of electronic marketplaces on product prices: an empirical study of AUCNET. International Journal of Electronic Commerce, 4 (2) 45–60.
Lee, J., Podlaseck, M., Schonberg, E., Hoch, R., & Gomory, S. (2000). Understanding merchandising effectiveness of online stores. Electronic Markets, 10 (1), 20–28.
Lee, J. N., Pi, S. M., Kwok, R. C., & Huynh, M. Q. (2003). The contribution of commitment value in Internet commerce: An empirical investigation. Journal of the Association for Information systems, 4 (1), 2.
Lee, K. C., & Kwon, S. (2008). A cognitive map-driven avatar design recommendation DSS and its empirical validity. Decision Support Systems, 45 (3), 461–472. doi: 10.1016/j.dss.2007.06.008
Lee, K. C., & Kwon, S. J. (2006). The use of cognitive maps and case-based reasoning for B2B negotiation. Journal of Management Information Systems, 22 (4), 337–376.
Lee, M. K. O., & Turban, E. (2001). A trust model for consumer Internet shopping. International Journal of Electronic Commerce, 6 , 75–92.
Lee, O. (2002). An action research report on the Korean national digital library. Information & Management, 39 (4), 255–260.
Lee, S., Zufryden, F., & Dreze, X. (2003). A study of consumer switching behavior across Internet portal Web sites. International Journal of Electronic Commerce, 7 (3), 39–63.
Lei-da Chen, S. H., Pandzik, A., Spigarelli, J., & Jesseman, C. (2003). Small business Internet commerce: a case study. Information Resources Management Journal, 16 (3), 17–31.
Leidner, D. E. (1999). Virtual partnerships in support of electronic commerce: the case of TCIS. Journal of Strategic Information Systems, 8 (1), 105–117. doi: 10.1016/s0963-8687(99)00012-8
Levenburg, N. M. (2005). Does size matter? Small firms’ use of E Business tools in the supply chain. Electronic Markets, 15 (2), 94–105.
Levenburg, N. M., & Klein, H. A. (2006). Delivering customer services online: identifying best practices of medium sized enterprises. Information Systems Journal, 16 (2), 135–155.
Li, C. F. (2010). Buy-it now: one dollar auction attractive? Journal of Computer Information Systems, 51 (1), 99–106.
Li, D. H., Browne, G. J., & Wetherbe, J. C. (2006). Why do Internet users stick with a specific Web site? A relationship perspective. International Journal of Electronic Commerce, 10 (4), 105–141. doi: 10.2753/jec1086-4415100404
Li, E. Y., Du, T. C., & Wong, J. W. (2007). Access control in collaborative commerce. Decision Support Systems, 43 (2), 675–685.
Li, E. Y., McLeod, R., & Rogers, J. C. (2001). Marketing information systems in Fortune 500 companies: a longitudinal analysis of 1980, 1990, and 2000. Information & Management, 38 (5), 307–322. doi: 10.1016/s0378-7206(00)00073-2
Li, X., & Hitt, L. (2010). Price effects in online product reviews: An analytical model and empirical analysis. MIS Quarterly, 34 (4), 809–831.
Li, X., Hitt, L. M., & Zhang, Z. J. (2011). Product reviews and competition in markets for repeat purchase products. Journal of Management Information Systems, 27 (4), 9–42.
Li, X., Troutt, M. D., Brandyberry, A., & Wang, T. (2011). Decision factors for the adoption and continued use of Online direct sales channels among SMEs. Journal of the Association for Information systems, 12 (1), 4.
Li, Y. M. (2010). Pricing digital content distribution over heterogeneous channels. Decision Support Systems, 50 (1), 243–257. doi: 10.1016/j.dss.2010.08.027
Li, Y. M., & Lin, C. H. (2009). Pricing schemes for digital content with DRM mechanisms. Decision Support Systems, 47 (4), 528–539. doi: 10.1016/j.dss.2009.05.015
Liang, T. P., & Huang, J. S. (1998). An empirical study on consumer acceptance of products in electronic markets: a transaction cost model. Decision Support Systems, 24 (1), 29–43.
Liang, T. P., & Huang, J. S. (2000). A framework for applying intelligent agents to support electronic trading. Decision Support Systems, 28 (4), 305–317. doi: 10.1016/s0167-9236(99)00098-6
Liang, T. P., Yang, Y. F., Chen, D. N., & Ku, Y. C. (2008). A semantic-expansion approach to personalized knowledge recommendation. Decision Support Systems, 45 (3), 401–412.
Liao, Z. Q., & Cheung, M. T. (2001). Internet-based e-shopping and consumer attitudes: an empirical study. Information & Management, 38 (5), 299–306. doi: 10.1016/s0378-7206(00)00072-0
Liao, Z. Q., & Cheung, M. T. (2002). Internet-based e-banking and consumer attitudes: an empirical study. Information & Management, 39 (4), 283–295. doi: 10.1016/s0378-7206(01)00097-0
Lim, S. H., Lee, S., Hur, Y., & Koh, C. E. (2009). Role of trust in adoption of online auto insurance. Journal of Computer Information Systems, 50 (2), 151–159.
Lin, C. H., & Nguyen, C. (2011). Exploring e-payment adoption in vietnam and taiwan. Journal of Computer Information Systems, 51 (4), 41–52.
Liu, C., Arnett, K. P., Capella, L. M., & Beatty, R. C. (1997). Web sites of the Fortune 500 companies: Facing customers through home pages. Information & Management, 31 (6), 335–345. doi: 10.1016/s0378-7206(97)00001-3
Liu, C., Arnett, K. P., Capella, L. M., & Taylor, R. D. (2001). Key dimensions of Web design quality as related to consumer response. Journal of Computer Information Systems, 42 (1), 70–82.
Lohe, J., & Legner, C. (2010). SOA adoption in business networks: do service-oriented architectures really advance inter-organizational integration? Electronic Markets, 20 (3–4), 181–196. doi: 10.1007/s12525-010-0046-7
López-Nicolás, C., Molina-Castillo, F. J., & Bouwman, H. (2008). An assessment of advanced mobile services acceptance: Contributions from TAM and diffusion theory models. Information & Management, 45 (6), 359–364.
Lu, H., & Lin, J. C. C. (2002). Predicting customer behavior in the market-space: a study of Rayport and Sviokla’s framework. Information & Management, 40 (1), 1–10.
Lu, H. P., & Hsiao, K. L. (2010). The influence of extro/introversion on the intention to pay for social networking sites. Information & Management, 47 (3), 150–157.
Lu, M. T., & Chan, T. S. (1999). The current status of Internet commerce in Hong Kong. Journal of Computer Information Systems, 39 (4), 16–21.
Lu, Y. B., Deng, Z. H., & Wang, B. (2010). Exploring factors affecting Chinese consumers’ usage of short message service for personal communication. Information Systems Journal, 20 (2), 183–208. doi: 10.1111/j.1365-2575.2008.00312.x
Luo, X., & Seyedian, M. (2003). Contextual marketing and customer-orientation strategy for e-commerce: an empirical analysis. International Journal of Electronic Commerce, 8 (2), 95–118.
Ma, Z. M., Pant, G., & Sheng, O. R. L. (2010). Examining organic and sponsored search results: a vendor reliability perspective. Journal of Computer Information Systems, 50 (4), 30–38.
MacInnes, I., Li, Y. F., & Yurcik, W. (2005). Reputation and dispute in eBay transactions. International Journal of Electronic Commerce, 10 (1), 27–54.
Maditinos, D. I., & Theodoridis, K. (2010). Satisfaction determinants in the Greek online shopping context. Information Technology & People, 23 (4), 312–329. doi: 10.1108/09593841011087789
Mai, B., Menon, N. M., & Sarkar, S. (2010). No free lunch: Price premium for privacy seal-bearing vendors. Journal of Management Information Systems, 27 (2), 189–212. doi: 10.2753/mis0742-1222270206
Markopoulos, P. M., Aron, R., & Ungar, L. (2010). Information markets for product attributes: A game theoretic, dual pricing mechanism. Decision Support Systems, 49 (2), 187–199. doi: 10.1016/j.dss.2010.02.005
Martinsons, M. G. (2008). Relationship-based e-commerce: theory and evidence from China. Information Systems Journal, 18 (4), 331–356. doi: 10.1111/j.1365-2575.2008.00302.x
Massey, A. P., Montoya-Weiss, M. M., & Holcom, K. (2001). Re-engineering the customer relationship: leveraging knowledge assets at IBM. Decision Support Systems, 32 (2), 155–170. doi: 10.1016/s0167-9236(01)00108-7
McCarthy, R. V., & Aronson, J. E. (2000). Activating consumer response: A model for web site design strategy. Journal of Computer Information Systems, 41 (2), 2–8.
McKnight, D. H., & Chervany, N. L. (2001). What trust means in e-commerce customer relationships: an interdisciplinary conceptual typology. International Journal of Electronic Commerce, 6 (2), 35–59.
McKnight, D. H., Choudhury, V., & Kacmar, C. (2003). Developing and validating trust measures for e-commerce: An integrative typology. Information Systems Research, 13 (3), 334–359.
Miller, T. W., & Dickson, P. R. (2001). On-line market research. International Journal of Electronic Commerce, 5 (3), 139–167.
Mithas, S., Jones, J. L., & Mitchell, W. (2008). Buyer intention to use internet-enabled reverse auctions: the role of asset specificity, product specialization, and non-contractibility. MIS Quarterly, 32 (4), 705–724.
Möllenberg, A. (2004). Internet auctions in marketing: the consumer perspective. Electronic Markets, 14 (4), 360–371.
Montazemi, A. R., Siam, J. J., & Esfahanipour, A. (2008). Effect of network relations on the adoption of electronic trading systems. Journal of Management Information Systems, 25 (1), 233–266. doi: 10.2753/mis0742-1222250109
Mort, D. (1998). The impact of the Internet on the European online business information market. Database, 21 (4), 74–78.
Muller-Lankenau, C., Wehmeyer, K., & Klein, S. (2005). Multi-channel strategies: Capturing and exploring diversity in the European retail grocery industry. International Journal of Electronic Commerce, 10 (2), 85–122.
Mun, H. J., Yun, H., Kim, E. A., Hong, J. Y., & Lee, C. C. (2010). Research on factors influencing intention to use DMB using extended IS success model. Information Technology and Management , 1–13.
Murphy, J., Raffa, L., & Mizerski, R. (2003). The use of domain names in e-branding by the world’s top brands. Electronic Markets, 13 (3), 222–232.
Muylle, S., & Basu, A. (2004). Online support for commerce processes and survivability of web retailers. Decision Support Systems, 38 (1), 101–113.
Muylle, S., & Basu, A. (2008). Online support for business processes by electronic intermediaries. Decision Support Systems, 45 (4), 845–857. doi: 10.1016/j.dss.2008.02.005
Muylle, S., Moenaert, R., & Despontin, M. (2004). The conceptualization and empirical validation of web site user satisfaction. Information & Management, 41 (5), 543–560.
Nault, B. R., & Dexter, A. S. (2006). Agent-intermediated electronic markets in international freight transportation. Decision Support Systems, 41 (4), 787–802. doi: 10.1016/j.dss.2004.10.008
Ngai, E., & Wat, F. (2002). A literature review and classification of electronic commerce research. Information & Management, 39 (5), 415–429.
Nikolaeva, R. (2005). Strategic determinants of web site traffic in on-line retailing. International Journal of Electronic Commerce, 9 (4), 113–132.
Novak, J., & Schwabe, G. (2009). Designing for reintermediation in the brick-and-mortar world: Towards the travel agency of the future. Electronic Markets, 19 (1), 15–29. doi: 10.1007/s12525-009-0003-5
Nysveen, H., & Pedersen, P. E. (2004). An exploratory study of customers’ perception of company web sites offering various interactive applications: moderating effects of customers’ Internet experience. Decision Support Systems, 37 (1), 137–150.
O’Connor, G. C., & O’Keefe, B. (1997). Viewing the Web as a marketplace: the case of small companies. Decision Support Systems, 21 (3), 171–183. doi: 10.1016/s0167-9236(97)00027-4
Oh, W., & Lucas, H. C. (2006). Information technology and pricing decisions: Price adjustments in online computer markets. MIS Quarterly, 30 (3), 755–775.
Onur, I., & Tomak, K. (2006). Impact of ending rules in online auctions: The case of Yahoo.com. Decision Support Systems, 42 (3), 1835–1842. doi: 10.1016/j.dss.2006.03.010
Oorni, A. (2003). Consumer search in electronic markets: an experimental analysis of travel services. European Journal of Information Systems, 12 (1), 30–40. doi: 10.1057/palgrave.ejis.3000450
Otim, S., & Grover, V. (2010). E-commerce: a brand name’s curse. Electronic Markets, 20 (2), 147–160. doi: 10.1007/s12525-010-0039-6
Ozdemir, Z. D. (2007). Optimal multi-channel delivery of expertise: An economic analysis. International Journal of Electronic Commerce, 11 (3), 89–105. doi: 10.2753/jec1086-4415110303
Ozdemir, Z. D., Akcura, M. T., & Altinkemer, K. (2006). Second opinions and online consultations. Decision Support Systems, 42 (3), 1747–1758. doi: 10.1016/j.dss.2006.03.011
Ozdemir, Z. D., Altinkemer, K., De, P., & Ozcelik, Y. (2010). Donor-to-nonprofit online marketplace: An economic analysis of the effects on fund-raising. Journal of Management Information Systems, 27 (2), 213–242. doi: 10.2753/mis0742-1222270207
Pagell, R. A. (1997). Internet securities: An emerging service for an emerging niche market. Database, 20 (5), 56-&.
Pant, S., Sim, H. T., & Hsu, C. (2001). A framework for developing Web information systems plans: illustration with Samsung Heavy Industries Co., Ltd. Information & Management, 38 (6), 385–408. doi: 10.1016/s0378-7206(00)00078-1
Parameswaran, M., Stallaert, J., & Whinston, A. B. (2001). A market-based allocation mechanism for the DiffServ framework. Decision Support Systems, 31 (3), 351–361. doi: 10.1016/s0167-9236(00)00143-3
Park, D. H., Lee, J., & Han, I. (2007). The effect of on-line consumer reviews on consumer purchasing intention: The moderating role of involvement. International Journal of Electronic Commerce, 11 (4), 125–148.
Patel, N. (2002). Emergent forms of IT governance to support global Ebusiness models. Journal of Information Technology Theory and Application, 4 (2), 5.
Pavlou, P. A. (2003). Consumer acceptance of electronic commerce: Integrating trust and risk with the technology acceptance model. International Journal of Electronic Commerce, 7 (3), 101–134.
Pedersen, P. E. (2000). Behavioral effects of using software agents for product and merchant brokering. International Journal of Electronic Commerce, 5 (1), 125–141.
Peng, G., Fan, M., & Dey, D. (2011). Impact of network effects and diffusion channels on home computer adoption. Decision Support Systems, 51 (3), 384–393. doi: 10.1016/j.dss.2011.01.004
Phan, D. D. (2003). E-business development for competitive advantages: a case study. Information & Management, 40 (6), 581–590.
Phang, C. W., Kankanhalli, A., Ramakrishnan, K., & Raman, K. S. (2010). Customers preference of online store visit strategies: an investigation of demographic variables. European Journal of Information Systems, 19 (3), 344–358.
Piccoli, G., & Lloyd, R. (2010). Strategic impacts of IT-enabled consumer power: Insight from Internet distribution in the U.S. lodging industry. Information & Management, 47 (7–8), 333–340. doi: 10.1016/j.im.2010.07.002
Pihlstrom, M. (2007). Committed to content provider or mobile channel? Determinants of continuous mobile multimedia service use. Journal of Information Technology Theory and Application, 9 (1), 3.
Poon, S. (2000). Business environment and Internet commerce benefit-a small business perspective. European Journal of Information Systems, 9 (2), 72–81.
Poon, S., & Swatman, P. (1999a). An exploratory study of small business Internet commerce issues. Information & Management, 35 (1), 9–18.
Poon, S., & Swatman, P. (1999b). A longitudinal study of expectations in small business Internet commerce. International Journal of Electronic Commerce, 3 (3), 21–33.
Qu, Z., Zhang, H., & Li, H. Z. (2008). Determinants of online merchant rating: Content analysis of consumer comments about Yahoo merchants. Decision Support Systems, 46 (1), 440–449. doi: 10.1016/j.dss.2008.08.004
Rachlevsky-Reich, B., Ben-Shaul, I., Chan, N. T., Lo, A. W., & Poggio, T. (1999). GEM: A global electronic market system. Information Systems, 24 (6), 495–518. doi: 10.1016/s0306-4379(99)00029-0
Rafaeli, S., & Noy, A. (2002). Online auctions, messaging, communication and social facilitation: a simulation and experimental evidence. European Journal of Information Systems, 11 (3), 196–207. doi: 10.1057/palgrave.ejis.3000434
Raghu, T. S., Kannan, P. K., Rao, H. R., & Whinston, A. B. (2001). Dynamic profiling of consumers for customized offerings over the Internet: a model and analysis. Decision Support Systems, 32 (2), 117–134. doi: 10.1016/s0167-9236(01)00106-3
Ram, S., Park, J., & Lee, D. (1999). Digital libraries for the next millennium: challenges and research directions. Information Systems Frontiers, 1 (1), 75–94.
Ranchhod, A., Zhou, F., & Tinson, J. (2001). Factors influencing marketing effectiveness on the Web. Information Resources Management Journal, 14 (1), 4.
Ranganathan, C., & Ganapathy, S. (2002). Key dimensions of business-to-consumer web sites. Information & Management, 39 (6), 457–465.
Ransbotham, S., & Mitra, S. (2009). Choice and chance: A conceptual model of paths to information security compromise. Information Systems Research, 20 (1), 121–139. doi: 10.1287/isre.1080.0174
Rau, P. L. P., Chen, J. W., & Chen, D. Y. (2006). A study of presentations of mobile web banners for location-based information and entertainment information websites. Behaviour & Information Technology, 25 (3), 253–261. doi: 10.1080/01449290500222009
Regev, O., & Nisan, N. (2000). The POPCORN market. Online markets for computational resources. Decision Support Systems, 28 (1–2), 177–189. doi: 10.1016/s0167-9236(99)00067-6
Riedl, R., Hubert, M., & Kenning, P. (2010). Are there neural gender differences in online trust? an FMRI study on the perceived trustworthiness of ebay offers. MIS Quarterly, 34 (2), 397–428.
Riggins, F. J. (2004). A multichannel model of separating equilibrium in the face of the digital divide. Journal of Management Information Systems, 21 (2), 161–179.
Riquelme, H. (2001). An empirical review of price behaviour on the Internet. Electronic Markets, 11 (4), 263–272.
Robertson, G., Murphy, J., & Purchase, S. (2005). Distance to market: Propinquity across In store and Online food retailing. Electronic Markets, 15 (3), 235–245.
Rohm, A. W., & Pernul, G. (2000). COPS: a model and infrastructure for secure and fair electronic markets. Decision Support Systems, 29 (4), 343–355. doi: 10.1016/s0167-9236(00)00082-8
Romano Jr, N. C., & Fjermestad, J. (2001). Electronic commerce customer relationship management: An assessment of research. International Journal of Electronic Commerce, 6 (2), 61–113.
Rossignoli, C., Carugati, A., & Mola, L. (2009). The strategic mediator: a paradoxical role for a collaborative e-marketplace. Electronic Markets, 19 (1), 55–66. doi: 10.1007/s12525-009-0005-3
Roussos, G., Peterson, D., & Patel, U. (2003). Mobile identity management: An enacted view. International Journal of Electronic Commerce, 8 (1), 81–100.
Rust, R. T., & Lemon, K. N. (2001). E-service and the consumer. International Journal of Electronic Commerce, 5 (3), 85–101.
Ryan, G., & Valverde, M. (2006). Waiting in line for online services: a qualitative study of the user’s perspective. Information Systems Journal, 16 (2), 181–211.
Saban, K. A. (2001). Strategic preparedness: a critical requirement to maximize e-commerce investments. Electronic Markets, 11 (1), 26–36.
SAban, K. A., & Rau, S. E. (2005). The functionality of websites as export marketing channels for small and medium enterprises. Electronic Markets, 15 (2), 128–135.
Sagi, J., Carayannis, E., Dasgupta, S., & Thomas, G. (2004). ICT and business in the New Economy: Globalization and attitudes. Journal of Global Information Management, 12 (3), 44–64.
Sen, R., King, R. C., & Shaw, M. J. (2006). Buyers’ choice of online search strategy and its managerial implications. Journal of Management Information Systems, 23 (1), 211–238. doi: 10.2753/mis0742-1222230107
Shang, R. A., Chen, Y. C., & Shen, L. (2005). Extrinsic versus intrinsic motivations for consumers to shop on-line. Information & Management, 42 (3), 401–413.
Shim, J., Shin, Y. B., & Nottingham, L. (2002). Retailer web site influence on customer shopping: exploratory study on key factors of customer satisfaction. Journal of the Association for Information systems, 3 (1), 3.
Shin, B., & Lee, H. G. (2005). Ubiquitous computing driven business models: A Case of SK Telecom’s financial services. Electronic Markets, 15 (1), 4–12.
Shin, D. H. (2009). A cross-national study of mobile Internet services: A comparison of US and Korean mobile Internet users. Journal of Global Information Management, 17 (4), 29–54. doi: 10.4018/jgim.2009070902
Shmueli, G., Jank, W., Aris, A., Plaisant, C., & Shneiderman, B. (2006). Exploring auction databases through interactive visualization. Decision Support Systems, 42 (3), 1521–1538. doi: 10.1016/j.dss.2006.01.001
Sieber, S., & Sabatier, J. V. (2003). Market bundling strategies in the horizontal portal industry. International Journal of Electronic Commerce, 7 (4), 37–54.
Soh, C., Markus, M. L., & Goh, K. H. (2006). Electronic marketplaces and price transparency: Strategy, information technology, and success. MIS Quarterly, 30 (3), 705–723.
Son, J. Y., Kim, S. S., & Riggins, F. J. (2006). Consumer adoption of net-enabled infomediaries: theoretical explanations and an empirical test. Journal of the Association for Information systems, 7 (1), 18.
Song, J., & Zahedi, F. M. (2006). Internet market strategies: Antecedents and implications. Information & Management, 43 (2), 222–238.
Song, P., Zhang, C., Xu, Y. C., & Huang, L. (2010). Brand extension of online technology products: Evidence from search engine to virtual communities and online news. Decision Support Systems, 49 (1), 91–99.
Spiller, P., & Lohse, G. L. (1997). A classification of Internet retail stores. International Journal of Electronic Commerce, 2 (2), 29–56.
Sripalawat, J., Thongmak, M., & Ngramyarn, A. (2011). M-BANKING in metropolitan Bangkok and a comparison with other countries. Journal of Computer Information Systems, 51 (3), 67–76.
Stafford, M. R., & Stern, B. (2002). Consumer bidding behavior on Internet auction sites. International Journal of Electronic Commerce, 7 (1), 135–150.
Standifird, S. S., Roelofs, M. R., & Durham, Y. (2004). The impact of eBay’s buy-it-now function on bidder Behavior. International Journal of Electronic Commerce, 9 (2), 167–176.
Standing, S., Standing, C., & Love, P. E. D. (2010). A review of research on e-marketplaces 1997–2008. Decision Support Systems, 49 (1), 41–51. doi: 10.1016/j.dss.2009.12.008
Steyaert, J. C. (2004). Measuring the performance of electronic government services. Information & Management, 41 (3), 369–375. doi: 10.1016/s0378-7206(03)00025-9
Student, B. M. D., & Marketing, J. L. C. P. f. (2003). The impact of visiting a brand website on brand personality. Electronic Markets, 13 (3), 210–221.
Stylianou, A. C., Kumar, R. L., & Robbins, S. S. (2005). Pricing on the Internet and in conventional retail channels: A study of over-the-counter pharmaceutical products. International Journal of Electronic Commerce, 10 (1), 135–148.
Subramani, M., & Walden, E. (2001). The impact of e-commerce announcements on the market value of firms. Information Systems Research, 12 (2), 135–154.
Subramaniam, C., & Shaw, M. J. (2002). A study of the value and impact of B2B E-commerce: The case of web-based procurement. International Journal of Electronic Commerce, 6 (4), 19–40.
Susarla, A., & Barua, A. (2011). Contracting efficiency and new firm survival in markets enabled by information technology. Information Systems Research, 22 (2), 306–324. doi: 10.1287/isre.1090.0251
Sutanto, J., Phang, C. W., Tan, C. H., & Lu, X. (2010). Dr. Jekyll vis-a-vis Mr. Hyde: Personality variation between virtual and real worlds. Information & Management, 48 (1), 19–26.
Sutcliffe, A. G. (2000). Requirements analysis for socio-technical system design. Information Systems, 25 (3), 213–233. doi: 10.1016/s0306-4379(00)00016-8
Taha, K., & Elmasri, R. (2010). SPGProfile: Speak group profile. Information Systems, 35 (7), 774–790. doi: 10.1016/j.is.2010.04.001
Talukder, M., & Yeow, P. H. P. (2007). A comparative study of virtual communities in Bangladesh and the USA. Journal of Computer Information Systems, 47 (4), 82–90.
Tan, C. H., Teo, H. H., & Xu, H. (2010). Online auction: the effects of transaction probability and listing price on a seller’s decision-making behavior. Electronic Markets, 20 (1), 67–79. doi: 10.1007/s12525-010-0029-8
Tan, J., Cheng, W. N., & Rogers, W. J. (2002). From telemedicine to e-health: Uncovering new frontiers of biomedical research, clinical applications & public health services delivery. Journal of Computer Information Systems, 42 (5), 7–18.
Tan, M., & Teo, T. S. H. (1999). The diffusion of the Internet in a pro-IT cultural environment: A content analysis of the Singapore experience. Communications of the AIS, 2 (3), 6.
Tang, F. F., & Lu, D. (2001). Pricing patterns in the online CD market: an empirical study. Electronic Markets, 11 (3), 171–185.
Tang, K., Chen, Y. L., & Hu, H. W. (2008). Context-based market basket analysis in a multiple-store environment. Decision Support Systems, 45 (1), 150–163.
Tang, Q., & Cheng, H. (2006). Optimal strategies for a monopoly intermediary in the supply chain of complementary web services. Journal of Management Information Systems, 23 (3), 275–307.
Tang, Z. L., Hu, Y., & Smith, M. D. (2008). Gaining trust through online privacy protection: Self-regulation, mandatory standards, or Caveat Emptor. Journal of Management Information Systems, 24 (4), 153–173. doi: 10.2753/mis0742-1222240406
Teich, J., Wallenius, H., & Wallenius, J. (1999). Multiple-issue auction and market algorithms for the world wide web. Decision Support Systems, 26 (1), 49–66. doi: 10.1016/s0167-9236(99)00016-0
Telang, R., Rajan, U., & Mukhopadhyay, T. (2004). The market structure for Internet search engines. Journal of Management Information Systems, 21 (2), 137–160.
Teo, T. S. H. (2002). Attitudes toward online shopping and the Internet. Behaviour & Information Technology, 21 (4), 259–271. doi: 10.1080/0144929021000018342
Teo, T. S. H. (2006). To buy or not to buy online: adopters and non-adopters of online shopping in Singapore. Behaviour & Information Technology, 25 (6), 497–509.
Teo, T. S. H., & Pian, Y. J. (2003). A contingency perspective on Internet adoption and competitive advantage. European Journal of Information Systems, 12 (2), 78–92. doi: 10.1057/palgrave.ejis.3000448
Teo, T. S. H., & Too, B. L. (2000). Information systems orientation and business use of the Internet: An empirical study. International Journal of Electronic Commerce, 4 (4), 105–130.
Tewari, G., Youll, J., & Maes, P. (2003). Personalized location-based brokering using an agent-based intermediary architecture. Decision Support Systems, 34 (2), 127–137. doi: 10.1016/s0167-9236(02)00076-3
Tsang, M. M., Ho, S. C., & Liang, T. P. (2004). Consumer attitudes toward mobile advertising: An empirical study. International Journal of Electronic Commerce, 8 (3), 65–78.
Tu, Y., & Lu, M. (2006). An experimental and analytical study of on-line digital music sampling strategies. International Journal of Electronic Commerce, 10 (3), 39–70.
Usunier, J. C., Roulin, N., & Ivens, B. S. (2009). Cultural, National, and Industry-Level Differences in B2B Web Site Design and Content. International Journal of Electronic Commerce, 14 (2), 41–88.
Van de Kar, E. V., & den Hengst, M. (2009). Involving users early on in the design process: closing the gap between mobile information services and their users. Electronic Markets, 19 (1), 31–42. doi: 10.1007/s12525-008-0002-y
Van der Heijden, H. (2003). Factors influencing the usage of websites: the case of a generic portal in The Netherlands. Information & Management, 40 (6), 541–549.
Van Gigch, J. P. (2000). Do we need to impose more regulation upon the World Wide Web?-A metasystem analysis. Informing Science, 3 (3), 109–116.
Van Heijst, D., Potharst, R., & van Wezel, M. (2008). A support system for predicting eBay end prices. Decision Support Systems, 44 (4), 970–982. doi: 10.1016/j.dss.2007.11.004
Vatanasakdakul, S., D’Ambra, J., & Ramburuth, P. (2010). IT doesn’t fit! The influence of culture on B2B in Thailand. Journal of Global Information Technology Management, 13 (3), 10–38.
Vatanasombut, B., Igbaria, M., Stylianou, A. C., & Rodgers, W. (2008). Information systems continuance intention of web-based applications customers: The case of online banking. Information & Management, 45 (7), 419–428. doi: 10.1016/j.im.2008.03.005
Vedder, R. G., Guynes, C. S., & Vanecek, M. T. (1997). Electronic commerce on the WWW/Internet. Journal of Computer Information Systems, 38 (1), 20–25.
Vellido, A., Lisboa, P. J. G., & Meehan, K. (2000). Quantitative characterization and prediction of on-line purchasing behavior: A latent variable approach. International Journal of Electronic Commerce, 4 (4), 83–104.
Verhoef, P. C., Spring, P. N., Hoekstra, J. C., & Leeflang, P. S. H. (2003). The commercial use of segmentation and predictive modeling techniques for database marketing in the Netherlands. Decision Support Systems, 34 (4), 471–481.
Vijayasarathy, L. R., & Jones, J. M. (2000). Intentions to shop using Internet catalogues: exploring the effects of product types, shopping orientations, and attitudes towards computers. Electronic Markets, 10 (1), 29–38.
Vragov, R., Di Shang, R., & Lang, K. R. (2010). On-line auctions with buy-it-now pricing: A practical design model and experimental evaluation. International Journal of Electronic Commerce, 14 (4), 39–67. doi: 10.2753/jec1086-4415140402
Wakefield, R. L., Wakefield, K. L., Baker, J., & Wang, L. C. (2010). How website socialness leads to website use. European Journal of Information Systems, 20 (1), 118–132.
Walter, Z., Gupta, A., & Su, B. C. (2006). The sources of on-line price dispersion across product types: An integrative view of on-line search costs and price premiums. International Journal of Electronic Commerce, 11 (1), 37–62.
Wan, H. A. (2000). Opportunities to enhance a commercial website. Information & Management, 38 (1), 15–21.
Wang, H. C., & Doong, H. S. (2010). Online customers’ cognitive differences and their impact on the success of recommendation agents. Information & Management, 47 (2), 109–114.
Wang, H. Q. (1997). A conceptual model for virtual markets. Information & Management, 32 (3), 147–161. doi: 10.1016/s0378-7206(97)00017-7
Wang, J. C., & Chiang, M. J. (2009). Social interaction and continuance intention in online auctions: A social capital perspective. Decision Support Systems, 47 (4), 466–476.
Wang, Y. S., Lin, H. H., & Luarn, P. (2006). Predicting consumer intention to use mobile service. Information Systems Journal, 16 (2), 157–179.
Ward, S. G., & Clark, J. M. (2002). Bidding behavior in on-line auctions: an examination of the eBay Pokemon card market. International Journal of Electronic Commerce, 6 (4), 139–155.
Wattal, S., Telang, R., & Mukhpadhyay, T. (2009). Information personalization in a two-dimensional product differentiation model. Journal of Management Information Systems, 26 (2), 69–95. doi: 10.2753/mis0742-1222260204
Weber, T. A., & Zheng, Z. Q. (2007). A model of search intermediaries and paid referrals. Information Systems Research, 18 (4), 414–436. doi: 10.1287/isre.1070.0139
West, L. A. (2000). Private markets for public goods: Pricing strategies of online database vendors. Journal of Management Information Systems, 17 (1), 59–85.
Whitman, M. E., Perez, J., & Beise, C. (2001). A study of user attitudes toward persistent cookies. Journal of Computer Information Systems, 41 (3), 1–7.
Wilson III, E. J. (2000). Wiring the African economy. Electronic Markets, 10 (2), 80–86.
Wu, C. S., Cheng, F. F., & Yen, D. C. (2008). The atmospheric factors of online storefront environment design: An empirical experiment in Taiwan. Information & Management, 45 (7), 493–498.
Xu, B., Jones, D. R., & Shao, B. (2009). Volunteers’ involvement in online community based software development. Information & Management, 46 (3), 151–158.
Xu, D. J., Liao, S. S., & Li, Q. (2008). Combining empirical experimentation and modeling techniques: A design research approach for personalized mobile advertising applications. Decision Support Systems, 44 (3), 710–724.
Xu, H., Luo, X., Carroll, J. M., & Rosson, M. B. (2011). The personalization privacy paradox: An exploratory study of decision making process for location-aware marketing. Decision Support Systems, 51 (1), 42–52. doi: 10.1016/j.dss.2010.11.017
Xue, L., Ray, G., & Whinston, A. B. (2006). Strategic investment in switching cost: An integrated customer acquisition and retention perspective. International Journal of Electronic Commerce, 11 (1), 7–35. doi: 10.2753/jec1086-4415110101
Yang, H. L., & Chiu, H. K. (2002). Privacy disclosures of Web sites in Taiwan. Journal of Information Technology Theory and Application, 4 (3), 4.
Yang, J., Hu, X., & Zhang, H. (2007). Effects of a reputation feedback system on an online consumer-to-consumer auction market. Decision Support Systems, 44 (1), 93–105. doi: 10.1016/j.dss.2007.03.005
Yen, H. J. R., Hsu, S. H. Y., & Huang, C. Y. (2011). Good Soldiers on the Web: Understanding the Drivers of Participation in Online Communities of Consumption. International Journal of Electronic Commerce, 15 (4), 89–120.
Yen, H. J. R., Li, E. Y., & Niehoff, B. P. (2008). Do organizational citizenship behaviors lead to information system success?: Testing the mediation effects of integration climate and project management. Information & Management, 45 (6), 394–402.
Yeung, W. L., & Lu, M. (2004). Functional characteristics of commercial web sites: a longitudinal study in Hong Kong. Information & Management, 41 (4), 483–495.
Yeung, W. L., & Lu, M. T. (2004). Gaining competitive advantages through a functionality grid for website evaluation. Journal of Computer Information Systems, 44 (4), 67–77.
Yue, W. T., & Chaturvedi, A. (2000). The reward based online shopping community. Electronic Markets, 10 (4), 224–228.
Zhang, D. S. (2004). Web services composition for process management in E-business. Journal of Computer Information Systems, 45 (2), 83–91.
Zhang, H., & Li, H. Z. (2006). Factors affecting payment choices in online auctions: A study of eBay traders. Decision Support Systems, 42 (2), 1076–1088. doi: 10.1016/j.dss.2005.09.003
Zhang, J., Fang, X., & Sheng, O. R. L. (2006). Online consumer search depth: Theories and new findings. Journal of Management Information Systems, 23 (3), 71–95. doi: 10.2753/mis0742-1222230304
Zhang, K. Z. K., Lee, M. K. O., Cheung, C. M. K., & Chen, H. P. (2009). Understanding the role of gender in bloggers’ switching behavior. Decision Support Systems, 47 (4), 540–546. doi: 10.1016/j.dss.2009.05.013
Zhao, J., Wang, S., & Huang, W. V. (2008). A study of B2B e-market in China: E-commerce process perspective. Information & Management, 45 (4), 242–248.
Zhou, M., Dresner, M., & Windle, R. (2009). Revisiting feedback systems: Trust building in digital markets. Information & Management, 46 (5), 279–284. doi: 10.1016/j.im.2009.05.002
Zhu, K., Kraemer, K. L., Gurbaxani, V., & Xu, S. X. (2006). Migration to open-standard interorganizational systems: Network effects, switching costs, and path dependency. MIS Quarterly, 30 , 515–539.
Zhuang, Y. L., & Lederer, A. L. (2006). A resource-based view of electronic commerce. Information & Management, 43 (2), 251–261. doi: 10.1016/j.im.2005.06.006
Zimmerman, R. D., Thomas, R. J., Gan, D. Q., & Murillo-Sanchez, C. (1999). A web-based platform for experimental investigation of electric power auctions. Decision Support Systems, 24 (3–4), 193–205. doi: 10.1016/s0167-9236(98)00083-9
Zviran, M. (2008). User’s perspectives on privacy in web-based applications. Journal of Computer Information Systems, 48 (4), 97–105.
Zwass, V. (2010). Co-Creation: Toward a Taxonomy and an Integrated Research Perspective. International Journal of Electronic Commerce, 15 (1), 11–48. doi: 10.2753/jec1086-4415150101
Appendix B – data sample (22 marketing articles)
Acquisti, A., & Varian, H. R. (2005). Conditioning prices on purchase history. Marketing Science, 24(3), 367–381. doi: 10.1287/mksc.1040.0103
Ancarani, F., & Shankar, V. (2004). Price levels and price dispersion within and across multiple retailer types: Further evidence and extension. Journal of the Academy of Marketing Science, 32(2), 176–187. doi: 10.1177/0092070303261464
Ansari, A., Mela, C. F., & Neslin, S. A. (2008). Customer channel migration. Journal of Marketing Research, 45(1), 60–76. doi: 10.1509/jmkr.45.1.60
Balasubramanian, S. (1998). Mail versus mall: A strategic analysis of competition between direct marketers and conventional retailers. Marketing Science, 17(3), 181–195. doi: 10.1287/mksc.17.3.181
Bodapati, A. V. (2008). Recommendation systems with purchase data. Journal of Marketing Research, 45(1), 77–93. doi: 10.1509/jmkr.45.1.77
Danaher, P. J. (2007). Modeling page views across multiple websites with an application to Internet reach and frequency prediction. Marketing Science, 26(3), 422–437. doi: 10.1287/mksc.1060.0226
Danaher, P. J., Lee, J., & Kerbache, L. (2010). Optimal Internet Media Selection. Marketing Science, 29(2), 336–347. doi: 10.1287/mksc.1090.0507
Fitzsimons, G. J., & Lehmann, D. R. (2004). Reactance to recommendations: When unsolicited advice yields contrary responses. Marketing Science, 23(1), 82–94. doi: 10.1287/mksc.1030.0033
Godes, D., & Mayzlin, D. (2004). Using online conversations to study word-of-mouth communication. Marketing Science, 23(4), 545–560. doi: 10.1287/mksc.1040.0071
Hauser, J. R., Urban, G. L., Liberali, G., & Braun, M. (2009). Website morphing. Marketing Science, 28(2), 202–223. doi: 10.1287/mksc.1080.0459
He, C., & Chen, Y. X. (2006). Managing e-Marketplace: A strategic analysis of nonprice advertising. Marketing Science, 25(2), 175–187. doi: 10.1287/mksc.1050.0168
Katona, Z., & Sarvary, M. (2010). The race for sponsored links: Bidding patterns for search advertising. Marketing Science, 29(2), 199–215. doi: 10.1287/mksc.1090.0517
Kozinets, R. V., de Valck, K., Wojnicki, A. C., & Wilner, S. J. S. (2010). Networked Narratives: Understanding Word-of-Mouth Marketing in Online Communities. Journal of Marketing, 74(2), 71–89.
Mayzlin, D. (2006). Promotional chat on the Internet. Marketing Science, 25(2), 155–163. doi: 10.1287/mksc.1050.0137
Moe, W. W., & Trusov, M. (2011). The value of social dynamics in online product ratings forums. Journal of Marketing Research, 48(3), 444–456.
Ofek, E., Katona, Z., & Sarvary, M. (2011). “Bricks and clicks”: The impact of product returns on the strategies of multichannel retailers. Marketing Science, 30(1), 42–60. doi: 10.1287/mksc.1100.0588
Toubia, O., Simester, D. I., Hauser, J. R., & Dahan, E. (2003). Fast polyhedral adaptive conjoint estimation. Marketing Science, 22(3), 273–303. doi: 10.1287/mksc.22.3.273.17743
Wilbur, K. C., & Zhu, Y. (2009). Click fraud. Marketing Science, 28(2), 293–308. doi: 10.1287/mksc.1080.0397
Yoo, W. S., & Lee, E. (2011). Internet channel entry: A strategic analysis of mixed channel structures. Marketing Science, 30(1), 29–41. doi: 10.1287/mksc.1100.0586
Zhang, J., & Krishnamurthi, L. (2004). Customizing promotions in online stores. Marketing Science, 23(4), 561–578. doi: 10.1287/mksc.1040.0055
Zhu, F., & Zhang, X. Q. (2010). Impact of online consumer reviews on sales: The moderating role of product and consumer characteristics. Journal of Marketing, 74(2), 133–148.
Zhu, Y., & Wilbur, K. C. (2011). Hybrid advertising auctions. Marketing Science, 30(2), 249–273. doi: 10.1287/mksc.1100.0609
Rights and permissions
Open Access This article is distributed under the terms of the Creative Commons Attribution 2.0 International License ( https://creativecommons.org/licenses/by/2.0 ), which permits unrestricted use, distribution, and reproduction in any medium, provided the original work is properly cited.
Reprints and permissions
About this article
Corley, J.K., Jourdan, Z. & Ingram, W.R. Internet marketing: a content analysis of the research. Electron Markets 23 , 177–204 (2013). https://doi.org/10.1007/s12525-012-0118-y
Download citation
Received : 08 November 2011
Accepted : 14 September 2012
Published : 31 January 2013
Issue Date : September 2013
DOI : https://doi.org/10.1007/s12525-012-0118-y
Share this article
Anyone you share the following link with will be able to read this content:
Sorry, a shareable link is not currently available for this article.
Provided by the Springer Nature SharedIt content-sharing initiative
- Internet marketing
- Business models
- Internet advertising
- Literature review
- Content analysis
JEL classification
- Find a journal
- Publish with us
- Track your research
Thank you for visiting nature.com. You are using a browser version with limited support for CSS. To obtain the best experience, we recommend you use a more up to date browser (or turn off compatibility mode in Internet Explorer). In the meantime, to ensure continued support, we are displaying the site without styles and JavaScript.
- View all journals
- My Account Login
- Explore content
- About the journal
- Publish with us
- Sign up for alerts
- Open access
- Published: 08 May 2024
Exploring the dynamics of consumer engagement in social media influencer marketing: from the self-determination theory perspective
- Chenyu Gu ORCID: orcid.org/0000-0001-6059-0573 1 &
- Qiuting Duan 2
Humanities and Social Sciences Communications volume 11 , Article number: 587 ( 2024 ) Cite this article
404 Accesses
2 Altmetric
Metrics details
- Business and management
- Cultural and media studies
Influencer advertising has emerged as an integral part of social media marketing. Within this realm, consumer engagement is a critical indicator for gauging the impact of influencer advertisements, as it encompasses the proactive involvement of consumers in spreading advertisements and creating value. Therefore, investigating the mechanisms behind consumer engagement holds significant relevance for formulating effective influencer advertising strategies. The current study, grounded in self-determination theory and employing a stimulus-organism-response framework, constructs a general model to assess the impact of influencer factors, advertisement information, and social factors on consumer engagement. Analyzing data from 522 samples using structural equation modeling, the findings reveal: (1) Social media influencers are effective at generating initial online traffic but have limited influence on deeper levels of consumer engagement, cautioning advertisers against overestimating their impact; (2) The essence of higher-level engagement lies in the ad information factor, affirming that in the new media era, content remains ‘king’; (3) Interpersonal factors should also be given importance, as influencing the surrounding social groups of consumers is one of the effective ways to enhance the impact of advertising. Theoretically, current research broadens the scope of both social media and advertising effectiveness studies, forming a bridge between influencer marketing and consumer engagement. Practically, the findings offer macro-level strategic insights for influencer marketing.
Similar content being viewed by others
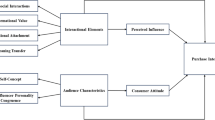
Exploring the effects of audience and strategies used by beauty vloggers on behavioural intention towards endorsed brands
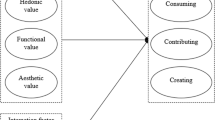
COBRAs and virality: viral campaign values on consumer behaviour
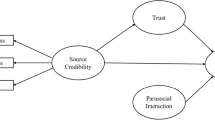
Exploring the impact of beauty vloggers’ credible attributes, parasocial interaction, and trust on consumer purchase intention in influencer marketing
Introduction.
Recent studies have highlighted an escalating aversion among audiences towards traditional online ads, leading to a diminishing effectiveness of traditional online advertising methods (Lou et al., 2019 ). In an effort to overcome these challenges, an increasing number of brands are turning to influencers as their spokespersons for advertising. Utilizing influencers not only capitalizes on their significant influence over their fan base but also allows for the dissemination of advertising messages in a more native and organic manner. Consequently, influencer-endorsed advertising has become a pivotal component and a growing trend in social media advertising (Gräve & Bartsch, 2022 ). Although the topic of influencer-endorsed advertising has garnered increasing attention from scholars, the field is still in its infancy, offering ample opportunities for in-depth research and exploration (Barta et al., 2023 ).
Presently, social media influencers—individuals with substantial follower bases—have emerged as the new vanguard in advertising (Hudders & Lou, 2023 ). Their tweets and videos possess the remarkable potential to sway the purchasing decisions of thousands if not millions. This influence largely hinges on consumer engagement behaviors, implying that the impact of advertising can proliferate throughout a consumer’s entire social network (Abbasi et al., 2023 ). Consequently, exploring ways to enhance consumer engagement is of paramount theoretical and practical significance for advertising effectiveness research (Xiao et al., 2023 ). This necessitates researchers to delve deeper into the exploration of the stimulating factors and psychological mechanisms influencing consumer engagement behaviors (Vander Schee et al., 2020 ), which is the gap this study seeks to address.
The Stimulus-Organism-Response (S-O-R) framework has been extensively applied in the study of consumer engagement behaviors (Tak & Gupta, 2021 ) and has been shown to integrate effectively with self-determination theory (Yang et al., 2019 ). Therefore, employing the S-O-R framework to investigate consumer engagement behaviors in the context of influencer advertising is considered a rational approach. The current study embarks on an in-depth analysis of the transformation process from three distinct dimensions. In the Stimulus (S) phase, we focus on how influencer factors, advertising message factors, and social influence factors act as external stimuli. This phase scrutinizes the external environment’s role in triggering consumer reactions. During the Organism (O) phase, the research explores the intrinsic psychological motivations affecting individual behavior as posited in self-determination theory. This includes the willingness for self-disclosure, the desire for innovation, and trust in advertising messages. The investigation in this phase aims to understand how these internal motivations shape consumer attitudes and perceptions in the context of influencer marketing. Finally, in the Response (R) phase, the study examines how these psychological factors influence consumer engagement behavior. This part of the research seeks to understand the transition from internal psychological states to actual consumer behavior, particularly how these states drive the consumers’ deep integration and interaction with the influencer content.
Despite the inherent limitations of cross-sectional analysis in capturing the full temporal dynamics of consumer engagement, this study seeks to unveil the dynamic interplay between consumers’ psychological needs—autonomy, competence, and relatedness—and their varying engagement levels in social media influencer marketing, grounded in self-determination theory. Through this lens, by analyzing factors related to influencers, content, and social context, we aim to infer potential dynamic shifts in engagement behaviors as psychological needs evolve. This approach allows us to offer a snapshot of the complex, multi-dimensional nature of consumer engagement dynamics, providing valuable insights for both theoretical exploration and practical application in the constantly evolving domain of social media marketing. Moreover, the current study underscores the significance of adapting to the dynamic digital environment and highlights the evolving nature of consumer engagement in the realm of digital marketing.
Literature review
Stimulus-organism-response (s-o-r) model.
The Stimulus-Response (S-R) model, originating from behaviorist psychology and introduced by psychologist Watson ( 1917 ), posits that individual behaviors are directly induced by external environmental stimuli. However, this model overlooks internal personal factors, complicating the explanation of psychological states. Mehrabian and Russell ( 1974 ) expanded this by incorporating the individual’s cognitive component (organism) into the model, creating the Stimulus-Organism-Response (S-O-R) framework. This model has become a crucial theoretical framework in consumer psychology as it interprets internal psychological cognitions as mediators between stimuli and responses. Integrating with psychological theories, the S-O-R model effectively analyzes and explains the significant impact of internal psychological factors on behavior (Koay et al., 2020 ; Zhang et al., 2021 ), and is extensively applied in investigating user behavior on social media platforms (Hewei & Youngsook, 2022 ). This study combines the S-O-R framework with self-determination theory to examine consumer engagement behaviors in the context of social media influencer advertising, a logic also supported by some studies (Yang et al., 2021 ).
Self-determination theory
Self-determination theory, proposed by Richard and Edward (2000), is a theoretical framework exploring human behavioral motivation and personality. The theory emphasizes motivational processes, positing that individual behaviors are developed based on factors satisfying their psychological needs. It suggests that individual behavioral tendencies are influenced by the needs for competence, relatedness, and autonomy. Furthermore, self-determination theory, along with organic integration theory, indicates that individual behavioral tendencies are also affected by internal psychological motivations and external situational factors.
Self-determination theory has been validated by scholars in the study of online user behaviors. For example, Sweet applied the theory to the investigation of community building in online networks, analyzing knowledge-sharing behaviors among online community members (Sweet et al., 2020 ). Further literature review reveals the applicability of self-determination theory to consumer engagement behaviors, particularly in the context of influencer marketing advertisements. Firstly, self-determination theory is widely applied in studying the psychological motivations behind online behaviors, suggesting that the internal and external motivations outlined within the theory might also apply to exploring consumer behaviors in influencer marketing scenarios (Itani et al., 2022 ). Secondly, although research on consumer engagement in the social media influencer advertising context is still in its early stages, some studies have utilized SDT to explore behaviors such as information sharing and electronic word-of-mouth dissemination (Astuti & Hariyawan, 2021 ). These behaviors, which are part of the content contribution and creation dimensions of consumer engagement, may share similarities in the underlying psychological motivational mechanisms. Thus, this study will build upon these foundations to construct the Organism (O) component of the S-O-R model, integrating insights from SDT to further understand consumer engagement in influencer marketing.
Consumer engagement
Although scholars generally agree at a macro level to define consumer engagement as the creation of additional value by consumers or customers beyond purchasing products, the specific categorization of consumer engagement varies in different studies. For instance, Simon and Tossan interpret consumer engagement as a psychological willingness to interact with influencers (Simon & Tossan, 2018 ). However, such a broad definition lacks precision in describing various levels of engagement. Other scholars directly use tangible metrics on social media platforms, such as likes, saves, comments, and shares, to represent consumer engagement (Lee et al., 2018 ). While this quantitative approach is not flawed and can be highly effective in practical applications, it overlooks the content aspect of engagement, contradicting the “content is king” principle of advertising and marketing. We advocate for combining consumer engagement with the content aspect, as content engagement not only generates more traces of consumer online behavior (Oestreicher-Singer & Zalmanson, 2013 ) but, more importantly, content contribution and creation are central to social media advertising and marketing, going beyond mere content consumption (Qiu & Kumar, 2017 ). Meanwhile, we also need to emphasize that engagement is not a fixed state but a fluctuating process influenced by ongoing interactions between consumers and influencers, mediated by the evolving nature of social media platforms and the shifting sands of consumer preferences (Pradhan et al., 2023 ). Consumer engagement in digital environments undergoes continuous change, reflecting a journey rather than a destination (Viswanathan et al., 2017 ).
The current study adopts a widely accepted definition of consumer engagement from existing research, offering operational feasibility and aligning well with the research objectives of this paper. Consumer engagement behaviors in the context of this study encompass three dimensions: content consumption, content contribution, and content creation (Muntinga et al., 2011 ). These dimensions reflect a spectrum of digital engagement behaviors ranging from low to high levels (Schivinski et al., 2016 ). Specifically, content consumption on social media platforms represents a lower level of engagement, where consumers merely click and read the information but do not actively contribute or create user-generated content. Some studies consider this level of engagement as less significant for in-depth exploration because content consumption, compared to other forms, generates fewer visible traces of consumer behavior (Brodie et al., 2013 ). Even in a study by Qiu and Kumar, it was noted that the conversion rate of content consumption is low, contributing minimally to the success of social media marketing (Qiu & Kumar, 2017 ).
On the other hand, content contribution, especially content creation, is central to social media marketing. When consumers comment on influencer content or share information with their network nodes, it is termed content contribution, representing a medium level of online consumer engagement (Piehler et al., 2019 ). Furthermore, when consumers actively upload and post brand-related content on social media, this higher level of behavior is referred to as content creation. Content creation represents the highest level of consumer engagement (Cheung et al., 2021 ). Although medium and high levels of consumer engagement are more valuable for social media advertising and marketing, this exploratory study still retains the content consumption dimension of consumer engagement behaviors.
Theoretical framework
Internal organism factors: self-disclosure willingness, innovativeness, and information trust.
In existing research based on self-determination theory that focuses on online behavior, competence, relatedness, and autonomy are commonly considered as internal factors influencing users’ online behaviors. However, this approach sometimes strays from the context of online consumption. Therefore, in studies related to online consumption, scholars often use self-disclosure willingness as an overt representation of autonomy, innovativeness as a representation of competence, and trust as a representation of relatedness (Mahmood et al., 2019 ).
The use of these overt variables can be logically explained as follows: According to self-determination theory, individuals with a higher level of self-determination are more likely to adopt compensatory mechanisms to facilitate behavior compared to those with lower self-determination (Wehmeyer, 1999 ). Self-disclosure, a voluntary act of sharing personal information with others, is considered a key behavior in the development of interpersonal relationships. In social environments, self-disclosure can effectively alleviate stress and build social connections, while also seeking societal validation of personal ideas (Altman & Taylor, 1973 ). Social networks, as para-social entities, possess the interactive attributes of real societies and are likely to exhibit similar mechanisms. In consumer contexts, personal disclosures can include voluntary sharing of product interests, consumption experiences, and future purchase intentions (Robertshaw & Marr, 2006 ). While material incentives can prompt personal information disclosure, many consumers disclose personal information online voluntarily, which can be traced back to an intrinsic need for autonomy (Stutzman et al., 2011 ). Thus, in this study, we consider the self-disclosure willingness as a representation of high autonomy.
Innovativeness refers to an individual’s internal level of seeking novelty and represents their personality and tendency for novelty (Okazaki, 2009 ). Often used in consumer research, innovative consumers are inclined to try new technologies and possess an intrinsic motivation to use new products. Previous studies have shown that consumers with high innovativeness are more likely to search for information on new products and share their experiences and expertise with others, reflecting a recognition of their own competence (Kaushik & Rahman, 2014 ). Therefore, in consumer contexts, innovativeness is often regarded as the competence dimension within the intrinsic factors of self-determination (Wang et al., 2016 ), with external motivations like information novelty enhancing this intrinsic motivation (Lee et al., 2015 ).
Trust refers to an individual’s willingness to rely on the opinions of others they believe in. From a social psychological perspective, trust indicates the willingness to assume the risk of being harmed by another party (McAllister, 1995 ). Widely applied in social media contexts for relational marketing, information trust has been proven to positively influence the exchange and dissemination of consumer information, representing a close and advanced relationship between consumers and businesses, brands, or advertising endorsers (Steinhoff et al., 2019 ). Consumers who trust brands or social media influencers are more willing to share information without fear of exploitation (Pop et al., 2022 ), making trust a commonly used representation of the relatedness dimension in self-determination within consumer contexts.
Construction of the path from organism to response: self-determination internal factors and consumer engagement behavior
Following the logic outlined above, the current study represents the internal factors of self-determination theory through three variables: self-disclosure willingness, innovativeness, and information trust. Next, the study explores the association between these self-determination internal factors and consumer engagement behavior, thereby constructing the link between Organism (O) and Response (R).
Self-disclosure willingness and consumer engagement behavior
In the realm of social sciences, the concept of self-disclosure willingness has been thoroughly examined from diverse disciplinary perspectives, encompassing communication studies, sociology, and psychology. Viewing from the lens of social interaction dynamics, self-disclosure is acknowledged as a fundamental precondition for the initiation and development of online social relationships and interactive engagements (Luo & Hancock, 2020 ). It constitutes an indispensable component within the spectrum of interactive behaviors and the evolution of interpersonal connections. Voluntary self-disclosure is characterized by individuals divulging information about themselves, which typically remains unknown to others and is inaccessible through alternative sources. This concept aligns with the tenets of uncertainty reduction theory, which argues that during interpersonal engagements, individuals seek information about their counterparts as a means to mitigate uncertainties inherent in social interactions (Lee et al., 2008 ). Self-disclosure allows others to gain more personal information, thereby helping to reduce the uncertainty in interpersonal relationships. Such disclosure is voluntary rather than coerced, and this sharing of information can facilitate the development of relationships between individuals (Towner et al., 2022 ). Furthermore, individuals who actively engage in social media interactions (such as liking, sharing, and commenting on others’ content) often exhibit higher levels of self-disclosure (Chu et al., 2023 ); additional research indicates a positive correlation between self-disclosure and online engagement behaviors (Lee et al., 2023 ). Taking the context of the current study, the autonomous self-disclosure willingness can incline social media users to read advertising content more attentively and share information with others, and even create evaluative content. Therefore, this paper proposes the following research hypothesis:
H1a: The self-disclosure willingness is positively correlated with content consumption in consumer engagement behavior.
H1b: The self-disclosure willingness is positively correlated with content contribution in consumer engagement behavior.
H1c: The self-disclosure willingness is positively correlated with content creation in consumer engagement behavior.
Innovativeness and consumer engagement behavior
Innovativeness represents an individual’s propensity to favor new technologies and the motivation to use new products, associated with the cognitive perception of one’s self-competence. Individuals with a need for self-competence recognition often exhibit higher innovativeness (Kelley & Alden, 2016 ). Existing research indicates that users with higher levels of innovativeness are more inclined to accept new product information and share their experiences and discoveries with others in their social networks (Yusuf & Busalim, 2018 ). Similarly, in the context of this study, individuals, as followers of influencers, signify an endorsement of the influencer. Driven by innovativeness, they may be more eager to actively receive information from influencers. If they find the information valuable, they are likely to share it and even engage in active content re-creation to meet their expectations of self-image. Therefore, this paper proposes the following research hypotheses:
H2a: The innovativeness of social media users is positively correlated with content consumption in consumer engagement behavior.
H2b: The innovativeness of social media users is positively correlated with content contribution in consumer engagement behavior.
H2c: The innovativeness of social media users is positively correlated with content creation in consumer engagement behavior.
Information trust and consumer engagement
Trust refers to an individual’s willingness to rely on the statements and opinions of a target object (Moorman et al., 1993 ). Extensive research indicates that trust positively impacts information dissemination and content sharing in interpersonal communication environments (Majerczak & Strzelecki, 2022 ); when trust is established, individuals are more willing to share their resources and less suspicious of being exploited. Trust has also been shown to influence consumers’ participation in community building and content sharing on social media, demonstrating cross-cultural universality (Anaya-Sánchez et al., 2020 ).
Trust in influencer advertising information is also a key predictor of consumers’ information exchange online. With many social media users now operating under real-name policies, there is an increased inclination to trust information shared on social media over that posted by corporate accounts or anonymously. Additionally, as users’ social networks partially overlap with their real-life interpersonal networks, extensive research shows that more consumers increasingly rely on information posted and shared on social networks when making purchase decisions (Wang et al., 2016 ). This aligns with the effectiveness goals of influencer marketing advertisements and the characteristics of consumer engagement. Trust in the content posted by influencers is considered a manifestation of a strong relationship between fans and influencers, central to relationship marketing (Kim & Kim, 2021 ). Based on trust in the influencer, which then extends to trust in their content, people are more inclined to browse information posted by influencers, share this information with others, and even create their own content without fear of exploitation or negative consequences. Therefore, this paper proposes the following research hypotheses:
H3a: Information trust is positively correlated with content consumption in consumer engagement behavior.
H3b: Information trust is positively correlated with content contribution in consumer engagement behavior.
H3c: Information trust is positively correlated with content creation in consumer engagement behavior.
Construction of the path from stimulus to organism: influencer factors, advertising information factors, social factors, and self-determination internal factors
Having established the logical connection from Organism (O) to Response (R), we further construct the influence path from Stimulus (S) to Organism (O). Revisiting the definition of influencer advertising in social media, companies, and brands leverage influencers on social media platforms to disseminate advertising content, utilizing the influencers’ relationships and influence over consumers for marketing purposes. In addition to consumer’s internal factors, elements such as companies, brands, influencers, and the advertisements themselves also impact consumer behavior. Although factors like the brand image perception of companies may influence consumer behavior, considering that in influencer marketing, companies and brands do not directly interact with consumers, this study prioritizes the dimensions of influencers and advertisements. Furthermore, the impact of social factors on individual cognition and behavior is significant, thus, the current study integrates influencers, advertisements, and social dimensions as the Stimulus (S) component.
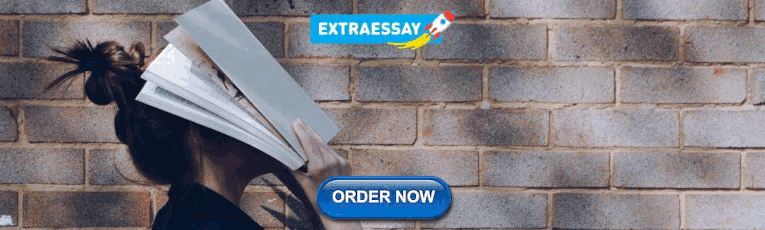
Influencer factors: parasocial identification
Self-determination theory posits that relationships are one of the key motivators influencing individual behavior. In the context of social media research, users anticipate establishing a parasocial relationship with influencers, resembling real-life relationships. Hence, we consider the parasocial identification arising from users’ parasocial interactions with influencers as the relational motivator. Parasocial interaction refers to the one-sided personal relationship that individuals develop with media characters (Donald & Richard, 1956 ). During this process, individuals believe that the media character is directly communicating with them, creating a sense of positive intimacy (Giles, 2002 ). Over time, through repeated unilateral interactions with media characters, individuals develop a parasocial relationship, leading to parasocial identification. However, parasocial identification should not be directly equated with the concept of social identification in social identity theory. Social identification occurs when individuals psychologically de-individualize themselves, perceiving the characteristics of their social group as their own, upon identifying themselves as part of that group. In contrast, parasocial identification refers to the one-sided interactional identification with media characters (such as celebrities or influencers) over time (Chen et al., 2021 ). Particularly when individuals’ needs for interpersonal interaction are not met in their daily lives, they turn to parasocial interactions to fulfill these needs (Shan et al., 2020 ). Especially on social media, which is characterized by its high visibility and interactivity, users can easily develop a strong parasocial identification with the influencers they follow (Wei et al., 2022 ).
Parasocial identification and self-disclosure willingness
Theories like uncertainty reduction, personal construct, and social exchange are often applied to explain the emergence of parasocial identification. Social media, with its convenient and interactive modes of information dissemination, enables consumers to easily follow influencers on media platforms. They can perceive the personality of influencers through their online content, viewing them as familiar individuals or even friends. Once parasocial identification develops, this pleasurable experience can significantly influence consumers’ cognitions and thus their behavioral responses. Research has explored the impact of parasocial identification on consumer behavior. For instance, Bond et al. found that on Twitter, the intensity of users’ parasocial identification with influencers positively correlates with their continuous monitoring of these influencers’ activities (Bond, 2016 ). Analogous to real life, where we tend to pay more attention to our friends in our social networks, a similar phenomenon occurs in the relationship between consumers and brands. This type of parasocial identification not only makes consumers willing to follow brand pages but also more inclined to voluntarily provide personal information (Chen et al., 2021 ). Based on this logic, we speculate that a similar relationship may exist between social media influencers and their fans. Fans develop parasocial identification with influencers through social media interactions, making them more willing to disclose their information, opinions, and views in the comment sections of the influencers they follow, engaging in more frequent social interactions (Chung & Cho, 2017 ), even if the content at times may be brand or company-embedded marketing advertisements. In other words, in the presence of influencers with whom they have established parasocial relationships, they are more inclined to disclose personal information, thereby promoting consumer engagement behavior. Therefore, we propose the following research hypotheses:
H4: Parasocial identification is positively correlated with consumer self-disclosure willingness.
H4a: Self-disclosure willingness mediates the impact of parasocial identification on content consumption in consumer engagement behavior.
H4b: Self-disclosure willingness mediates the impact of parasocial identification on content contribution in consumer engagement behavior.
H4c: Self-disclosure willingness mediates the impact of parasocial identification on content creation in consumer engagement behavior.
Parasocial identification and information trust
Information Trust refers to consumers’ willingness to trust the information contained in advertisements and to place themselves at risk. These risks include purchasing products inconsistent with the advertised information and the negative social consequences of erroneously spreading this information to others, leading to unpleasant consumption experiences (Minton, 2015 ). In advertising marketing, gaining consumers’ trust in advertising information is crucial. In the context of influencer marketing on social media, companies, and brands leverage the social connection between influencers and their fans. According to cognitive empathy theory, consumers project their trust in influencers onto the products endorsed, explaining the phenomenon of ‘loving the house for the crow on its roof.’ Research indicates that parasocial identification with influencers is a necessary condition for trust development. Consumers engage in parasocial interactions with influencers on social media, leading to parasocial identification (Jin et al., 2021 ). Consumers tend to reduce their cognitive load and simplify their decision-making processes, thus naturally adopting a positive attitude and trust towards advertising information disseminated by influencers with whom they have established parasocial identification. This forms the core logic behind the success of influencer marketing advertisements (Breves et al., 2021 ); furthermore, as mentioned earlier, because consumers trust these advertisements, they are also willing to share this information with friends and family and even engage in content re-creation. Therefore, we propose the following research hypotheses:
H5: Parasocial identification is positively correlated with information trust.
H5a: Information trust mediates the impact of parasocial identification on content consumption in consumer engagement behavior.
H5b: Information trust mediates the impact of parasocial identification on content contribution in consumer engagement behavior.
H5c: Information trust mediates the impact of parasocial identification on content creation in consumer engagement behavior.
Influencer factors: source credibility
Source credibility refers to the degree of trust consumers place in the influencer as a source, based on the influencer’s reliability and expertise. Numerous studies have validated the effectiveness of the endorsement effect in advertising (Schouten et al., 2021 ). The Source Credibility Model, proposed by the renowned American communication scholar Hovland and the “Yale School,” posits that in the process of information dissemination, the credibility of the source can influence the audience’s decision to accept the information. The credibility of the information is determined by two aspects of the source: reliability and expertise. Reliability refers to the audience’s trust in the “communicator’s objective and honest approach to providing information,” while expertise refers to the audience’s trust in the “communicator being perceived as an effective source of information” (Hovland et al., 1953 ). Hovland’s definitions reveal that the interpretation of source credibility is not about the inherent traits of the source itself but rather the audience’s perception of the source (Jang et al., 2021 ). This differs from trust and serves as a precursor to the development of trust. Specifically, reliability and expertise are based on the audience’s perception; thus, this aligns closely with the audience’s perception of influencers (Kim & Kim, 2021 ). This credibility is a cognitive statement about the source of information.
Source credibility and self-disclosure willingness
Some studies have confirmed the positive impact of an influencer’s self-disclosure on their credibility as a source (Leite & Baptista, 2022 ). However, few have explored the impact of an influencer’s credibility, as a source, on consumers’ self-disclosure willingness. Undoubtedly, an impact exists; self-disclosure is considered a method to attempt to increase intimacy with others (Leite et al., 2022 ). According to social exchange theory, people promote relationships through the exchange of information in interpersonal communication to gain benefits (Cropanzano & Mitchell, 2005 ). Credibility, deriving from an influencer’s expertise and reliability, means that a highly credible influencer may provide more valuable information to consumers. Therefore, based on the social exchange theory’s logic of reciprocal benefits, consumers might be more willing to disclose their information to trustworthy influencers, potentially even expanding social interactions through further consumer engagement behaviors. Thus, we propose the following research hypotheses:
H6: Source credibility is positively correlated with self-disclosure willingness.
H6a: Self-disclosure willingness mediates the impact of Source credibility on content consumption in consumer engagement behavior.
H6b: Self-disclosure willingness mediates the impact of Source credibility on content contribution in consumer engagement behavior.
H6c: Self-disclosure willingness mediates the impact of Source credibility on content creation in consumer engagement behavior.
Source credibility and information trust
Based on the Source Credibility Model, the credibility of an endorser as an information source can significantly influence consumers’ acceptance of the information (Shan et al., 2020 ). Existing research has demonstrated the positive impact of source credibility on consumers. Djafarova, in a study based on Instagram, noted through in-depth interviews with 18 users that an influencer’s credibility significantly affects respondents’ trust in the information they post. This credibility is composed of expertise and relevance to consumers, and influencers on social media are considered more trustworthy than traditional celebrities (Djafarova & Rushworth, 2017 ). Subsequently, Bao and colleagues validated in the Chinese consumer context, based on the ELM model and commitment-trust theory, that the credibility of brand pages on Weibo effectively fosters consumer trust in the brand, encouraging participation in marketing activities (Bao & Wang, 2021 ). Moreover, Hsieh et al. found that in e-commerce contexts, the credibility of the source is a significant factor influencing consumers’ trust in advertising information (Hsieh & Li, 2020 ). In summary, existing research has proven that the credibility of the source can promote consumer trust. Influencer credibility is a significant antecedent affecting consumers’ trust in the advertised content they publish. In brand communities, trust can foster consumer engagement behaviors (Habibi et al., 2014 ). Specifically, consumers are more likely to trust the advertising content published by influencers with higher credibility (more expertise and reliability), and as previously mentioned, consumer engagement behavior is more likely to occur. Based on this, the study proposes the following research hypotheses:
H7: Source credibility is positively correlated with information trust.
H7a: Information trust mediates the impact of source credibility on content consumption in consumer engagement behavior.
H7b: Information trust mediates the impact of source credibility on content contribution in consumer engagement behavior.
H7c: Information trust mediates the impact of source credibility on content creation in consumer engagement behavior.
Advertising information factors: informative value
Advertising value refers to “the relative utility value of advertising information to consumers and is a subjective evaluation by consumers.” In his research, Ducoffe pointed out that in the context of online advertising, the informative value of advertising is a significant component of advertising value (Ducoffe, 1995 ). Subsequent studies have proven that consumers’ perception of advertising value can effectively promote their behavioral response to advertisements (Van-Tien Dao et al., 2014 ). Informative value of advertising refers to “the information about products needed by consumers provided by the advertisement and its ability to enhance consumer purchase satisfaction.” From the perspective of information dissemination, valuable advertising information should help consumers make better purchasing decisions and reduce the effort spent searching for product information. The informational aspect of advertising has been proven to effectively influence consumers’ cognition and, in turn, their behavior (Haida & Rahim, 2015 ).
Informative value and innovativeness
As previously discussed, consumers’ innovativeness refers to their psychological trait of favoring new things. Studies have shown that consumers with high innovativeness prefer novel and valuable product information, as it satisfies their need for newness and information about new products, making it an important factor in social media advertising engagement (Shi, 2018 ). This paper also hypothesizes that advertisements with high informative value can activate consumers’ innovativeness, as the novelty of information is one of the measures of informative value (León et al., 2009 ). Acquiring valuable information can make individuals feel good about themselves and fulfill their perception of a “novel image.” According to social exchange theory, consumers can gain social capital in interpersonal interactions (such as social recognition) by sharing information about these new products they perceive as valuable. Therefore, the current study proposes the following research hypothesis:
H8: Informative value is positively correlated with innovativeness.
H8a: Innovativeness mediates the impact of informative value on content consumption in consumer engagement behavior.
H8b: Innovativeness mediates the impact of informative value on content contribution in consumer engagement behavior.
H8c: Innovativeness mediates the impact of informative value on content creation in consumer engagement behavior.
Informative value and information trust
Trust is a multi-layered concept explored across various disciplines, including communication, marketing, sociology, and psychology. For the purposes of this paper, a deep analysis of different levels of trust is not undertaken. Here, trust specifically refers to the trust in influencer advertising information within the context of social media marketing, denoting consumers’ belief in and reliance on the advertising information endorsed by influencers. Racherla et al. investigated the factors influencing consumers’ trust in online reviews, suggesting that information quality and value contribute to increasing trust (Racherla et al., 2012 ). Similarly, Luo and Yuan, in a study based on social media marketing, also confirmed that the value of advertising information posted on brand pages can foster consumer trust in the content (Lou & Yuan, 2019 ). Therefore, by analogy, this paper posits that the informative value of influencer-endorsed advertising can also promote consumer trust in that advertising information. The relationship between trust in advertising information and consumer engagement behavior has been discussed earlier. Thus, the current study proposes the following research hypotheses:
H9: Informative value is positively correlated with information trust.
H9a: Information trust mediates the impact of informative value on content consumption in consumer engagement behavior.
H9b: Information trust mediates the impact of informative value on content contribution in consumer engagement behavior.
H9c: Information trust mediates the impact of informative value on content creation in consumer engagement behavior.
Advertising information factors: ad targeting accuracy
Ad targeting accuracy refers to the degree of match between the substantive information contained in advertising content and consumer needs. Advertisements containing precise information often yield good advertising outcomes. In marketing practice, advertisers frequently use information technology to analyze the characteristics of different consumer groups in the target market and then target their advertisements accordingly to achieve precise dissemination and, consequently, effective advertising results. The utility of ad targeting accuracy has been confirmed by many studies. For instance, in the research by Qiu and Chen, using a modified UTAUT model, it was demonstrated that the accuracy of advertising effectively promotes consumer acceptance of advertisements in WeChat Moments (Qiu & Chen, 2018 ). Although some studies on targeted advertising also indicate that overly precise ads may raise concerns about personal privacy (Zhang et al., 2019 ), overall, the accuracy of advertising information is effective in enhancing advertising outcomes and is a key element in the success of targeted advertising.
Ad targeting accuracy and information trust
In influencer marketing advertisements, due to the special relationship recognition between consumers and influencers, the privacy concerns associated with ad targeting accuracy are alleviated (Vrontis et al., 2021 ). Meanwhile, the informative value brought by targeting accuracy is highlighted. More precise advertising content implies higher informative value and also signifies that the advertising content is more worthy of consumer trust (Della Vigna, Gentzkow, 2010 ). As previously discussed, people are more inclined to read and engage with advertising content they trust and recognize. Therefore, the current study proposes the following research hypotheses:
H10: Ad targeting accuracy is positively correlated with information trust.
H10a: Information trust mediates the impact of ad targeting accuracy on content consumption in consumer engagement behavior.
H10b: Information trust mediates the impact of ad targeting accuracy on content contribution in consumer engagement behavior.
H10c: Information trust mediates the impact of ad targeting accuracy on content creation in consumer engagement behavior.
Social factors: subjective norm
The Theory of Planned Behavior, proposed by Ajzen ( 1991 ), suggests that individuals’ actions are preceded by conscious choices and are underlain by plans. TPB has been widely used by scholars in studying personal online behaviors, these studies collectively validate the applicability of TPB in the context of social media for researching online behaviors (Huang, 2023 ). Additionally, the self-determination theory, which underpins this chapter’s research, also supports the notion that individuals’ behavioral decisions are based on internal cognitions, aligning with TPB’s assertions. Therefore, this paper intends to select subjective norms from TPB as a factor of social influence. Subjective norm refers to an individual’s perception of the expectations of significant others in their social relationships regarding their behavior. Empirical research in the consumption field has demonstrated the significant impact of subjective norms on individual psychological cognition (Yang & Jolly, 2009 ). A meta-analysis by Hagger, Chatzisarantis ( 2009 ) even highlighted the statistically significant association between subjective norms and self-determination factors. Consequently, this study further explores its application in the context of influencer marketing advertisements on social media.
Subjective norm and self-disclosure willingness
In numerous studies on social media privacy, subjective norms significantly influence an individual’s self-disclosure willingness. Wirth et al. ( 2019 ) based on the privacy calculus theory, surveyed 1,466 participants and found that personal self-disclosure on social media is influenced by the behavioral expectations of other significant reference groups around them. Their research confirmed that subjective norms positively influence self-disclosure of information and highlighted that individuals’ cognitions and behaviors cannot ignore social and environmental factors. Heirman et al. ( 2013 ) in an experiment with Instagram users, also noted that subjective norms could promote positive consumer behavioral responses. Specifically, when important family members and friends highly regard social media influencers as trustworthy, we may also be more inclined to disclose our information to influencers and share this information with our surrounding family and friends without fear of disapproval. In our subjective norms, this is considered a positive and valuable interactive behavior, leading us to exhibit engagement behaviors. Based on this logic, we propose the following research hypotheses:
H11: Subjective norms are positively correlated with self-disclosure willingness.
H11a: Self-disclosure willingness mediates the impact of subjective norms on content consumption in consumer engagement behavior.
H11b: Self-disclosure willingness mediates the impact of subjective norms on content contribution in consumer engagement behavior.
H11c: Self-disclosure willingness mediates the impact of subjective norms on content creation in consumer engagement behavior.
Subjective norm and information trust
Numerous studies have indicated that subjective norms significantly influence trust (Roh et al., 2022 ). This can be explained by reference group theory, suggesting people tend to minimize the effort expended in decision-making processes, often looking to the behaviors or attitudes of others as a point of reference; for instance, subjective norms can foster acceptance of technology by enhancing trust (Gupta et al., 2021 ). Analogously, if a consumer’s social network generally holds positive attitudes toward influencer advertising, they are also more likely to trust the endorsed advertisement information, as it conserves the extensive effort required in gathering product information (Chetioui et al., 2020 ). Therefore, this paper proposes the following research hypotheses:
H12: Subjective norms are positively correlated with information trust.
H12a: Information trust mediates the impact of subjective norms on content consumption in consumer engagement behavior.
H12b: Information trust mediates the impact of subjective norms on content contribution in consumer engagement behavior.
H12c: Information trust mediates the impact of subjective norms on content creation in consumer engagement behavior.
Conceptual model
In summary, based on the Stimulus (S)-Organism (O)-Response (R) framework, this study constructs the external stimulus factors (S) from three dimensions: influencer factors (parasocial identification, source credibility), advertising information factors (informative value, Ad targeting accuracy), and social influence factors (subjective norms). This is grounded in social capital theory and the theory of planned behavior. drawing on self-determination theory, the current study constructs the individual psychological factors (O) using self-disclosure willingness, innovativeness, and information trust. Finally, the behavioral response (R) is constructed using consumer engagement, which includes content consumption, content contribution, and content creation, as illustrated in Fig. 1 .
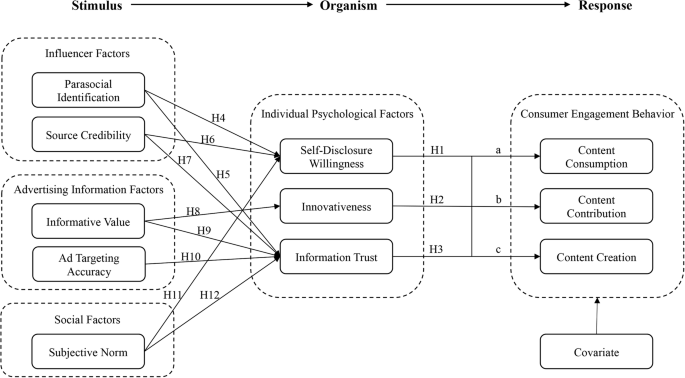
Consumer engagement behavior impact model based on SOR framework.
Materials and methods
Participants and procedures.
The current study conducted a survey through the Wenjuanxing platform to collect data. Participants were recruited through social media platforms such as WeChat, Douyin, Weibo et al., as samples drawn from social media users better align with the research purpose of our research and ensure the validity of the sample. Before the survey commenced, all participants were explicitly informed about the purpose of this study, and it was made clear that volunteers could withdraw from the survey at any time. Initially, 600 questionnaires were collected, with 78 invalid responses excluded. The criteria for valid questionnaires were as follows: (1) Respondents must have answered “Yes” to the question, “Do you follow any influencers (internet celebrities) on social media platforms?” as samples not using social media or not following influencers do not meet the study’s objective, making this question a prerequisite for continuing the survey; (2) Respondents had to correctly answer two hidden screening questions within the questionnaire to ensure that they did not randomly select scores; (3) The total time taken to complete the questionnaire had to exceed one minute, ensuring that respondents had sufficient time to understand and thoughtfully answer each question; (4) Respondents were not allowed to choose the same score for eight consecutive questions. Ultimately, 522 valid questionnaires were obtained, with an effective rate of 87.00%, meeting the basic sample size requirements for research models (Gefen et al., 2011 ). Detailed demographic information of the study participants is presented in Table 1 .
Measurements
To ensure the validity and reliability of the data analysis results in this study, the measurement tools and scales used in this chapter were designed with reference to existing established research. The main variables in the survey questionnaire include parasocial identification, source credibility, informative value, ad targeting accuracy, subjective norms, self-disclosure willingness, innovativeness, information trust, content consumption, content contribution, and content creation. The measurement scale for parasocial identification was adapted from the research of Schramm and Hartmann, comprising 6 items (Schramm & Hartmann, 2008 ). The source credibility scale was combined from the studies of Cheung et al. and Luo & Yuan’s research in the context of social media influencer marketing, including 4 items (Cheung et al., 2009 ; Lou & Yuan, 2019 ). The scale for informative value was modified based on Voss et al.‘s research, consisting of 4 items (Voss et al., 2003 ). The ad targeting accuracy scale was derived from the research by Qiu Aimei et al., 2018 ) including 3 items. The subjective norm scale was adapted from Ajzen’s original scale, comprising 3 items (Ajzen, 2002 ). The self-disclosure willingness scale was developed based on Chu and Kim’s research, including 3 items (Chu & Kim, 2011 ). The innovativeness scale was formulated following the study by Sun et al., comprising 4 items (Sun et al., 2006 ). The information trust scale was created in reference to Chu and Choi’s research, including 3 items (Chu & Choi, 2011 ). The scales for the three components of social media consumer engagement—content consumption, content contribution, and content creation—were sourced from the research by Buzeta et al., encompassing 8 items in total (Buzeta et al., 2020 ).
All scales were appropriately revised for the context of social media influencer marketing. To avoid issues with scoring neutral attitudes, a uniform Likert seven-point scale was used for each measurement item (ranging from 1 to 7, representing a spectrum from ‘strongly disagree’ to ‘strongly agree’). After the overall design of the questionnaire was completed, a pre-test was conducted with 30 social media users to ensure that potential respondents could clearly understand the meaning of each question and that there were no obstacles to answering. This pre-test aimed to prevent any difficulties or misunderstandings in the questionnaire items. The final version of the questionnaire is presented in Table 2 .
Data analysis
Since the model framework of the current study is derived from theoretical deductions of existing research and, while logically constructed, does not originate from an existing research model, this study still falls under the category of exploratory research. According to the analysis suggestions of Hair and other scholars, in cases of exploratory research model frameworks, it is more appropriate to choose Smart PLS for Partial Least Squares Path Analysis (PLS) to conduct data analysis and testing of the research model (Hair et al., 2012 ).
Measurement of model
In this study, careful data collection and management resulted in no missing values in the dataset. This ensured the integrity and reliability of the subsequent data analysis. As shown in Table 3 , after deleting measurement items with factor loadings below 0.5, the final factor loadings of the measurement items in this study range from 0.730 to 0.964. This indicates that all measurement items meet the retention criteria. Additionally, the Cronbach’s α values of the latent variables range from 0.805 to 0.924, and all latent variables have Composite Reliability (CR) values greater than the acceptable value of 0.7, demonstrating that the scales of this study have passed the reliability test requirements (Hair et al., 2019 ). All latent variables in this study have Average Variance Extracted (AVE) values greater than the standard acceptance value of 0.5, indicating that the convergent validity of the variables also meets the standard (Fornell & Larcker, 1981 ). Furthermore, the results show that the Variance Inflation Factor (VIF) values for each factor are below 10, indicating that there are no multicollinearity issues with the scales in this study (Hair, 2009 ).
The current study then further verified the discriminant validity of the variables, with specific results shown in Table 4 . The square roots of the average variance extracted (AVE) values for all variables (bolded on the diagonal) are greater than the Pearson correlation coefficients between the variables, indicating that the discriminant validity of the scales in this study meets the required standards (Fornell & Larcker, 1981 ). Additionally, a single-factor test method was employed to examine common method bias in the data. The first unrotated factor accounted for 29.71% of the variance, which is less than the critical threshold of 40%. Therefore, the study passed the test and did not exhibit serious common method bias (Podsakoff et al., 2003 ).
To ensure the robustness and appropriateness of our structural equation model, we also conducted a thorough evaluation of the model fit. Initially, through PLS Algorithm calculations, the R 2 values of each variable were greater than the standard acceptance value of 0.1, indicating good predictive accuracy of the model. Subsequently, Blindfolding calculations were performed, and the results showed that the Stone-Geisser Q 2 values of each variable were greater than 0, demonstrating that the model of this study effectively predicts the relationships between variables (Dijkstra & Henseler, 2015 ). In addition, through CFA, we also obtained some indicator values, specifically, χ 2 /df = 2.528 < 0.3, RMSEA = 0.059 < 0.06, SRMR = 0.055 < 0.08. Given its sensitivity to sample size, we primarily focused on the CFI, TLI, and NFI values, CFI = 0.953 > 0.9, TLI = 0.942 > 0.9, and NFI = 0.923 > 0.9 indicating a good fit. Additionally, RMSEA values below 0.06 and SRMR values below 0.08 were considered indicative of a good model fit. These indices collectively suggested that our model demonstrates a satisfactory fit with the data, thereby reinforcing the validity of our findings.
Research hypothesis testing
The current study employed a Bootstrapping test with a sample size of 5000 on the collected raw data to explore the coefficients and significance of the paths in the research model. The final test data results of this study’s model are presented in Table 5 .
The current study employs S-O-R model as the framework, grounded in theories such as self-determination theory and theory of planned behavior, to construct an influence model of consumer engagement behavior in the context of social media influencer marketing. It examines how influencer factors, advertisement information factors, and social influence factors affect consumer engagement behavior by impacting consumers’ psychological cognitions. Using structural equation modeling to analyze collected data ( N = 522), it was found that self-disclosure willingness, innovativeness, and information trust positively influence consumer engagement behavior, with innovativeness having the largest impact on higher levels of engagement. Influencer factors, advertisement information factors, and social factors serve as effective external stimuli, influencing psychological motivators and, consequently, consumer engagement behavior. The specific research results are illustrated in Fig. 2 .
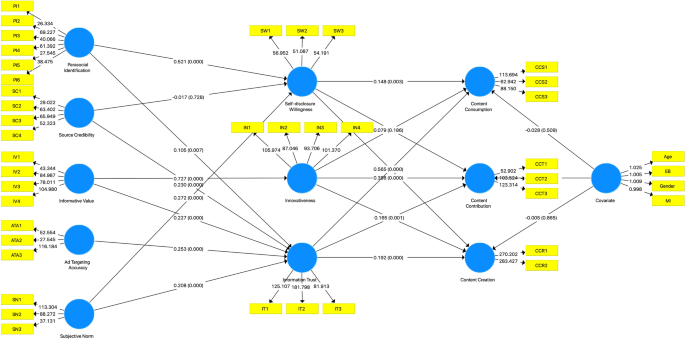
Tested structural model of consumer engagement behavior.
The impact of psychological motivators on different levels of consumer engagement: self-disclosure willingness, innovativeness, and information trust
The research analysis indicates that self-disclosure willingness and information trust are key drivers for content consumption (H1a, H2a validated). This aligns with previous findings that individuals with a higher willingness to disclose themselves show greater levels of engagement behavior (Chu et al., 2023 ); likewise, individuals who trust advertisement information are more inclined to engage with advertisement content (Kim, Kim, 2021 ). Moreover, our study finds that information trust has a stronger impact on content consumption, underscoring the importance of trust in the dissemination of advertisement information. However, no significant association was found between individual innovativeness and content consumption (H3a not validated).
Regarding the dimension of content contribution in consumer engagement, self-disclosure willingness, information trust, and innovativeness all positively impact it (H1b, H2b, and H3b all validated). This is consistent with earlier research findings that individuals with higher self-disclosure willingness are more likely to like, comment on, or share content posted by influencers on social media platforms (Towner et al., 2022 ); the conclusions of this paper also support that innovativeness is an important psychological driver for active participation in social media interactions (Kamboj & Sharma, 2023 ). However, at the level of consumer engagement in content contribution, while information trust also exerts a positive effect, its impact is the weakest, although information trust has the strongest impact on content consumption.
In social media advertising, the ideal outcome is the highest level of consumer engagement, i.e., content creation, meaning consumers actively join in brand content creation, seeing themselves as co-creators with the brand (Nadeem et al., 2021 ). Our findings reveal that self-disclosure willingness, innovativeness, and information trust all positively influence content creation (H1c, H2c, and H3c all validated). The analysis found that similar to the impact on content contribution, innovativeness has the most significant effect on encouraging individual content creation, followed by self-disclosure willingness, with information trust having the least impact.
In summary, while some previous studies have shown that self-disclosure willingness, innovativeness, and information trust are important factors in promoting consumer engagement (Chu et al., 2023 ; Nadeem et al., 2021 ; Geng et al., 2021 ), this study goes further by integrating and comparing all three within the same research framework. It was found that to trigger higher levels of consumer engagement behavior, trust is not the most crucial psychological motivator; rather, the most effective method is to stimulate consumers’ innovativeness, thus complementing previous research. Subsequently, this study further explores the impact of different stimulus factors on various psychological motivators.
The influence of external stimulus factors on psychological motivators: influencer factors, advertisement information factors, and social factors
The current findings indicate that influencer factors, such as parasocial identification and source credibility, effectively enhance consumer engagement by influencing self-disclosure willingness and information trust. This aligns with prior research highlighting the significance of parasocial identification (Shan et al., 2020 ). Studies suggest parasocial identification positively impacts consumer engagement by boosting self-disclosure willingness and information trust (validated H4a, H4b, H4c, and H5a), but not content contribution or creation through information trust (H5b, H5c not validated). Source credibility’s influence on self-disclosure willingness was not significant (H6 not validated), thus negating the mediating effect of self-disclosure willingness (H6a, H6b, H6c not validated). Influencer credibility mainly affects engagement through information trust (H7a, H7b, H7c validated), supporting previous findings (Shan et al., 2020 ).
Advertisement factors (informative value and ad targeting accuracy) promote engagement through innovativeness and information trust. Informative value significantly impacts higher-level content contribution and creation through innovativeness (H8b, H8c validated), while ad targeting accuracy influences consumer engagement at all levels mainly through information trust (H10a, H10b, H10c validated).
Social factors (subjective norms) enhance self-disclosure willingness and information trust, consistent with previous research (Wirth et al., 2019 ; Gupta et al., 2021 ), and further promote consumer engagement across all levels (H11a, H11b, H11c, H12a, H12b, and H12c all validated).
In summary, influencer, advertisement, and social factors impact consumer engagement behavior by influencing psychological motivators, with influencer factors having the greatest effect on content consumption, advertisement content factors significantly raising higher-level consumer engagement through innovativeness, and social factors also influencing engagement through self-disclosure willingness and information trust.
Implication
From a theoretical perspective, current research presents a comprehensive model of consumer engagement within the context of influencer advertising on social media. This model not only expands the research horizon in the fields of social media influencer advertising and consumer engagement but also serves as a bridge between two crucial themes in new media advertising studies. Influencer advertising has become an integral part of social media advertising, and the construction of a macro model aids researchers in understanding consumer psychological processes and behavioral patterns. It also assists advertisers in formulating more effective strategies. Consumer engagement, focusing on the active role of consumers in disseminating information and the long-term impact on advertising effectiveness, aligns more closely with the advertising effectiveness measures in the new media context than traditional advertising metrics. However, the intersection of these two vital themes lacks comprehensive research and a universal model. This study constructs a model that elucidates the effects of various stimuli on consumer psychology and engagement behaviors, exploring the connections and mechanisms through different mediating pathways. By differentiating levels of engagement, the study offers more nuanced conclusions for diverse advertising objectives. Furthermore, this research validates the applicability of self-determination theory in the context of influencer advertising effectiveness. While this psychological theory has been utilized in communication behavior research, its effectiveness in the field of advertising requires further exploration. The current study introduces self-determination theory into the realm of influencer advertising and consumer engagement, thereby expanding its application in the field of advertising communication. It also responds to the call from the advertising and marketing academic community to incorporate more psychological theories to explain the ‘black box’ of consumer psychology. The inclusion of this theory re-emphasizes the people-centric approach of this research and highlights the primary role of individuals in advertising communication studies.
From a practical perspective, this study provides significant insights for adapting marketing strategies to the evolving media landscape and the empowered role of audiences. Firstly, in the face of changes in the communication environment and the empowerment of audience communication capabilities, traditional marketing approaches are becoming inadequate for new media advertising needs. Traditional advertising focuses on direct, point-to-point effects, whereas social media advertising aims for broader, point-to-mass communication, leveraging audience proactivity to facilitate the viral spread of content across online social networks. Secondly, for brands, the general influence model proposed in this study offers guidance for influencer advertising strategy. If the goal is to maximize reach and brand recognition with a substantial advertising budget, partnering with top influencers who have a large following can be an effective strategy. However, if the objective is to maximize cost-effectiveness with a limited budget by leveraging consumer initiative for secondary spread, the focus should be on designing advertising content that stimulates consumer creativity and willingness to innovate. Thirdly, influencers are advised to remain true to their followers. In influencer marketing, influencers attract advertisers through their influence over followers, converting this influence into commercial gain. This influence stems from the trust followers place in the influencer, thus influencers should maintain professional integrity and prioritize the quality of information they share, even when presented with advertising opportunities. Lastly, influencers should assert more control over their relationships with advertisers. In traditional advertising, companies and brands often exert significant control over the content. However, in the social media era, influencers should negotiate more creative freedom in their advertising partnerships, asserting a more equal relationship with advertisers. This approach ensures that content quality remains high, maintaining the trust influencers have built with their followers.
Limitations and future directions
while this study offers valuable insights into the dynamics of influencer marketing and consumer engagement on social media, several limitations should be acknowledged: Firstly, constrained by the research objectives and scope, this study’s proposed general impact model covers three dimensions: influencers, advertisement information, and social factors. However, these dimensions are not limited to the five variables discussed in this paper. Therefore, we call for future research to supplement and explore more crucial factors. Secondly, in the actual communication environment, there may be differences in the impact of communication effectiveness across various social media platforms. Thus, future research could also involve comparative studies and explorations between different social media platforms. Thirdly, the current study primarily examines the direct effects of various factors on consumer engagement. However, the potential interaction effects between these variables (e.g., how influencers’ credibility might interact with advertisement information quality) are not extensively explored. Future research could investigate these complex interrelationships for a more holistic understanding. Lastly, our study, being cross-sectional, offers preliminary insights into the complex and dynamic nature of engagement between social media influencers and consumers, yet it does not incorporate the temporal dimension. The diverse impacts of psychological needs on engagement behaviors hint at an underlying dynamism that merits further investigation. Future research should consider employing longitudinal designs to directly observe how these dynamics evolve over time.
The findings of the current study not only theoretically validate the applicability of self-determination theory in the field of social media influencer marketing advertising research but also broaden the scope of advertising effectiveness research from the perspective of consumer engagement. Moreover, the research framework offers strategic guidance and reference for influencer marketing strategies. The main conclusions of this study can be summarized as follows.
Innovativeness is the key factor in high-level consumer engagement behavior. Content contribution represents a higher level of consumer engagement compared to content consumption, as it not only requires consumers to dedicate attention to viewing advertising content but also to share this information across adjacent nodes within their social networks. This dissemination of information is a pivotal factor in the success of influencer marketing advertisements. Hence, companies and brands prioritize consumers’ content contribution over mere viewing of advertising content (Qiu & Kumar, 2017 ). Compared to content consumption and contribution, content creation is considered the highest level of consumer engagement, where consumers actively create and upload brand-related content, and it represents the most advanced outcome sought by enterprises and brands in advertising campaigns (Cheung et al., 2021 ). The current study posits that to pursue better outcomes in social media influencer advertising marketing, enhancing consumers’ willingness for self-disclosure, innovativeness, and trust in advertising information are effective strategies. However, the crux lies in leveraging the consumer’s subjective initiative, particularly in boosting their innovativeness. If the goal is simply to achieve content consumption rather than higher levels of consumer engagement, the focus should be on fostering trust in advertising information. There is no hierarchy in the efficacy of different strategies; they should align with varying marketing contexts and advertising objectives.
The greatest role of social media influencers lies in attracting online traffic. information trust is the core element driving content consumption, and influencer factors mainly affect consumer engagement behaviors through information trust. Therefore, this study suggests that the primary role of influencers in social media advertising is to attract online traffic, i.e., increase consumer behavior regarding ad content consumption (reducing avoidance of ad content), and help brands achieve the initial goal of making consumers “see and complete ads.” However, their impact on further high-level consumer engagement behaviors is limited. This mechanism serves as a reminder to advertisers not to overestimate the effects of influencers in marketing. Currently, top influencers command a significant portion of the ad budget, which could squeeze the budget for other aspects of advertising, potentially affecting the overall effectiveness of the campaign. Businesses and brands should consider deeper strategic implications when planning their advertising campaigns.
Valuing Advertising Information Factors, Content Remains King. Our study posits that in the social media influencer marketing context, the key to enhancing consumer contribution and creation of advertising content lies primarily in the advertising information factors. In other words, while content consumption is important, advertisers should objectively assess the role influencers play in advertising. In the era of social media, content remains ‘king’ in advertising. This view indirectly echoes the points made in the previous paragraph: influencers effectively perform initial ‘online traffic generation’ tasks in social media, but this role should not be overly romanticized or exaggerated. Whether it’s companies, brands, or influencers, providing consumers with advertisements rich in informational value is crucial to achieving better advertising outcomes and potentially converting consumers into stakeholders.
Subjective norm is an unignorable social influence factor. Social media is characterized by its network structure of information dissemination, where a node’s information is visible to adjacent nodes. For instance, if user A likes a piece of content C from influencer I, A’s follower B, who may not follow influencer I, can still see content C via user A’s page. The aim of marketing in the social media era is to influence a node and then spread the information to adjacent nodes, either secondarily or multiple times (Kumar & Panda, 2020 ). According to the Theory of Planned Behavior, an individual’s actions are influenced by significant others in their lives, such as family and friends. Previous studies have proven the effectiveness of the Theory of Planned Behavior in influencing attitudes toward social media advertising (Ranjbarian et al., 2012 ). Current research further confirms that subjective norms also influence consumer engagement behaviors in influencer marketing on social media. Therefore, in advertising practice, brands should not only focus on individual consumers but also invest efforts in groups that can influence consumer decisions. Changing consumer behavior in the era of social media marketing doesn’t solely rely on the company’s efforts.
As communication technology advances, media platforms will further empower individual communicative capabilities, moving beyond the era of the “magic bullet” theory. The distinction between being a recipient and a transmitter of information is increasingly blurred. In an era where everyone is both an audience and an influencer, research confined to the role of the ‘recipient’ falls short of addressing the dynamics of ‘transmission’. Future research in marketing and advertising should thus focus more on the power of individual transmission. Furthermore, as Marshall McLuhan famously said, “the medium is the extension of man.” The evolution of media technology remains human-centric. Accordingly, future marketing research, while paying heed to media transformations, should emphasize the centrality of the ‘human’ element.
Data availability
The datasets generated and/or analyzed during the current study are not publicly available due to privacy issues. Making the full data set publicly available could potentially breach the privacy that was promised to participants when they agreed to take part, and may breach the ethics approval for the study. The data are available from the corresponding author on reasonable request.
Abbasi AZ, Tsiotsou RH, Hussain K, Rather RA, Ting DH (2023) Investigating the impact of social media images’ value, consumer engagement, and involvement on eWOM of a tourism destination: a transmittal mediation approach. J Retail Consum Serv 71:103231. https://doi.org/10.1016/j.jretconser.2022.103231
Article Google Scholar
Ajzen I (2002) Perceived behavioral control, self‐efficacy, locus of control, and the theory of planned behavior 1. J Appl Soc Psychol 32(4):665–683. https://doi.org/10.1111/j.1559-1816.2002.tb00236.x
Ajzen I (1991) The theory of planned behavior. Organ Behav Hum Decis Process 50(2):179–211. https://doi.org/10.1016/0749-5978(91)90020-T
Altman I, Taylor DA (1973) Social penetration: the development of interpersonal relationships. Holt, Rinehart & Winston
Anaya-Sánchez R, Aguilar-Illescas R, Molinillo S, Martínez-López FJ (2020) Trust and loyalty in online brand communities. Span J Mark ESIC 24(2):177–191. https://doi.org/10.1108/SJME-01-2020-0004
Astuti BA, Hariyawan A (2021) Perspectives of social capital and self-determination on e-WOM at millennial generation in Yogyakarta. Integr J Bus Econ 5(1):399475. https://doi.org/10.33019/ijbe.v5i1.338
Bao Z, Wang D (2021) Examining consumer participation on brand microblogs in China: perspectives from elaboration likelihood model, commitment–trust theory and social presence. J Res Interact Mark 15(1):10–29. https://doi.org/10.1108/JRIM-02-2019-0027
Barta S, Belanche D, Fernández A, Flavián M (2023) Influencer marketing on TikTok: the effectiveness of humor and followers’ hedonic experience. J Retail Consum Serv 70:103149. https://doi.org/10.1016/j.jretconser.2022.103149
Bond BJ (2016) Following your “friend”: social media and the strength of adolescents’ parasocial relationships with media personae. Cyberpsych Behav Soc Netw 19(11):656–660. https://doi.org/10.1089/cyber.2016.0355
Breves P, Amrehn J, Heidenreich A, Liebers N, Schramm H (2021) Blind trust? The importance and interplay of parasocial relationships and advertising disclosures in explaining influencers’ persuasive effects on their followers. Int J Advert 40(7):1209–1229. https://doi.org/10.1080/02650487.2021.1881237
Brodie RJ, Ilic A, Juric B, Hollebeek L (2013) Consumer engagement in a virtual brand community: an exploratory analysis. J Bus Res 66(1):105–114. https://doi.org/10.1016/j.jbusres.2011.07.029
Buzeta C, De Pelsmacker P, Dens N (2020) Motivations to use different social media types and their impact on consumers’ online brand-related activities (COBRAs). J Interact Mark 52(1):79–98. https://doi.org/10.1016/j.intmar.2020.04.0
Chen KJ, Lin JS, Shan Y (2021) Influencer marketing in China: The roles of parasocial identification, consumer engagement, and inferences of manipulative intent. J Consum Behav 20(6):1436–1448. https://doi.org/10.1002/cb.1945
Chetioui Y, Benlafqih H, Lebdaoui H (2020) How fashion influencers contribute to consumers’ purchase intention. J Fash Mark Manag 24(3):361–380. https://doi.org/10.1108/JFMM-08-2019-0157
Cheung ML, Pires GD, Rosenberger III PJ, De Oliveira MJ (2021) Driving COBRAs: the power of social media marketing. Mark Intell Plan 39(3):361–376. https://doi.org/10.1108/MIP-11-2019-0583
Cheung MY, Luo C, Sia CL, Chen H (2009) Credibility of electronic word-of-mouth: Informational and normative determinants of on-line consumer recommendations. Int J Electron Comm 13(4):9–38. https://doi.org/10.2753/JEC1086-4415130402
Chung S, Cho H (2017) Fostering parasocial relationships with celebrities on social media: Implications for celebrity endorsement. Psychol Mark 34(4):481–495. https://doi.org/10.1002/mar.21001
Chu SC, Choi SM (2011) Electronic word-of-mouth in social networking sites: a cross-cultural study of the United States and China. J Glob Mark 24(3):263–281. https://doi.org/10.1080/08911762.2011.592461
Chu SC, Kim Y (2011) Determinants of consumer engagement in electronic word-of-mouth (eWOM) in social networking sites. Int J Advert 30(1):47–75. https://doi.org/10.2501/IJA-30-1-047-075
Chu TH, Sun M, Crystal Jiang L (2023) Self-disclosure in social media and psychologicalwell-being: a meta-analysis. J Soc Pers Relat 40(2):576–599. https://doi.org/10.1177/02654075221119429
Cropanzano R, Mitchell MS (2005) Social exchange theory: an interdisciplinary review. J Manag 31(6):874–900. https://doi.org/10.1177/0149206305279602
Della Vigna S, Gentzkow M (2010) Persuasion: empirical evidence. Annu Rev Econ 2(1):643–669. https://doi.org/10.1146/annurev.economics.102308.124309
Dijkstra TK, Henseler J (2015) Consistent and asymptotically normal PLS estimators for linear structural equations. Comput Stat Data 81:10–23. https://doi.org/10.1016/j.csda.2014.07.008
Article MathSciNet Google Scholar
Djafarova E, Rushworth C (2017) Exploring the credibility of online celebrities’ Instagram profiles in influencing the purchase decisions of young female users. Comput Hum Behav 68:1–7. https://doi.org/10.1016/j.chb.2016.11.009
D Horton D, Richard Wohl R (1956) Mass communication and para-social interaction: Observations on intimacy at a distance. Psychiatry 19(3):215–229. https://doi.org/10.1080/00332747.1956.11023049
Ducoffe RH (1995) How consumers assess the value of advertising. J Curr Issues Res Adver 17(1):1–18. https://doi.org/10.1080/10641734.1995.10505022
Fornell C, Larcker DF (1981) Structural equation models with unobservable variables and measurement error: Algebra and statistics. J Mark Res 18(3):382–388. https://doi.org/10.1177/002224378101800313
Gefen D, Straub DW, Rigdon EE (2011) An update and extension to SEM guidelines for administrative and social science research. Mis Quart 35(2):iii–xiv. https://doi.org/10.2307/23044042
Geng S, Yang P, Gao Y, Tan Y, Yang C (2021) The effects of ad social and personal relevance on consumer ad engagement on social media: the moderating role of platform trust. Comput Hum Behav 122:106834. https://doi.org/10.1016/j.chb.2021.106834
Giles DC (2002) Parasocial interaction: a review of the literature and a model for future research. Media Psychol 4(3):279–305. https://doi.org/10.1207/S1532785XMEP0403_04
Gräve JF, Bartsch F (2022) # Instafame: exploring the endorsement effectiveness of influencers compared to celebrities. Int J Advert 41(4):591–622. https://doi.org/10.1080/02650487.2021.1987041
Gupta R, Ranjan S, Gupta A (2021) Consumer’s perceived trust and subjective norms as antecedents of mobile wallets adoption and continuance intention: a technology acceptance approach. Recent Adv Technol Accept Models Theor 211–224. https://doi.org/10.1007/978-3-030-64987-6_13
Habibi MR, Laroche M, Richard MO (2014) The roles of brand community and community engagement in building brand trust on social media. Comput Hum Behav 37:152–161. https://doi.org/10.1016/j.chb.2014.04.016
Hagger MS, Chatzisarantis NL (2009) Integrating the theory of planned behaviour and self‐determination theory in health behaviour: a meta‐analysis. Brit J Health Psych 14(2):275–302. https://doi.org/10.1348/135910708X373959
Haida A, Rahim HL (2015) Social media advertising value: A Study on consumer’s perception. Int Acad Res J Bus Technol 1(1):1–8. https://www.researchgate.net/publication/280325676_Social_Media_Advertising_Value_A_Study_on_Consumer%27s_Perception
Google Scholar
Hair JF (2009) Multivariate data analysis. Prentice Hall, Upper Saddle River
Hair JF, Ringle CM, Gudergan SP, Fischer A, Nitzl C, Menictas C (2019) Partial least squares structural equation modeling-based discrete choice modeling: an illustration in modeling retailer choice. Bus Res 12(1):115–142. https://doi.org/10.1007/s40685-018-0072-4
Hair JF, Sarstedt M, Ringle CM, Mena JA (2012) An assessment of the use of partial least squares structural equation modeling in marketing research. Acad Mark Sci 40:414–433. https://doi.org/10.1007/s11747-011-0261-6
Heirman W, Walrave M, Ponnet K (2013) Predicting adolescents’ disclosure of personal information in exchange for commercial incentives: An application of an extended theory of planned behavior. Cyberpsych Behav Soc Netw16(2):81–87. https://doi.org/10.1089/cyber.2012.0041
Hewei T, Youngsook L (2022) Factors affecting continuous purchase intention of fashion products on social E-commerce: SOR model and the mediating effect. Entertain Comput 41:100474. https://doi.org/10.1016/j.entcom.2021.100474
Hovland CI, Janis IL, Kelley HH (1953) Communication and persuasion. Yale University Press
Hsieh JK, Li YJ (2020) Will you ever trust the review website again? The importance of source credibility. Int J Electron Commerce 24(2):255–275. https://doi.org/10.1080/10864415.2020.1715528
Huang YC (2023) Integrated concepts of the UTAUT and TPB in virtual reality behavioral intention. J Retail Consum Serv 70:103127. https://doi.org/10.1016/j.jretconser.2022.103127
Hudders L, Lou C (2023) The rosy world of influencer marketing? Its bright and dark sides, and future research recommendations. Int J Advert 42(1):151–161. https://doi.org/10.1080/02650487.2022.2137318
Itani OS, Kalra A, Riley J (2022) Complementary effects of CRM and social media on customer co-creation and sales performance in B2B firms: The role of salesperson self-determination needs. Inf Manag 59(3):103621. https://doi.org/10.1016/j.im.2022.103621
Jang W, Kim J, Kim S, Chun JW (2021) The role of engagement in travel influencer marketing: the perspectives of dual process theory and the source credibility model. Curr Issues Tour 24(17):2416–2420. https://doi.org/10.1080/13683500.2020.1845126
Jin SV, Ryu E, Muqaddam A (2021) I trust what she’s# endorsing on Instagram: moderating effects of parasocial interaction and social presence in fashion influencer marketing. J Fash Mark Manag 25(4):665–681. https://doi.org/10.1108/JFMM-04-2020-0059
Kamboj S, Sharma M (2023) Social media adoption behaviour: consumer innovativeness and participation intention. Int J Consum Stud 47(2):523–544. https://doi.org/10.1111/ijcs.12848
Kaushik AK, Rahman Z (2014) Perspectives and dimensions of consumer innovativeness: a literature review and future agenda. J Int Consum Mark 26(3):239–263. https://doi.org/10.1080/08961530.2014.893150
Kelley JB, Alden DL (2016) Online brand community: through the eyes of self-determination theory. Internet Res 26(4):790–808. https://doi.org/10.1108/IntR-01-2015-0017
K Kim DY, Kim HY (2021) Trust me, trust me not: A nuanced view of influencer marketing on social media. J Bus Res 134:223–232. https://doi.org/10.1016/j.jbusres.2021.05.024
Koay KY, Ong DLT, Khoo KL, Yeoh HJ (2020) Perceived social media marketing activities and consumer-based brand equity: Testing a moderated mediation model. Asia Pac J Mark Logist 33(1):53–72. https://doi.org/10.1108/APJML-07-2019-0453
Kumar S, Panda BS (2020) Identifying influential nodes in Social Networks: Neighborhood Coreness based voting approach. Phys A: Stat Mech Appl 553:124215. https://doi.org/10.1016/j.physa.2020.124215
Lee D, Hosanagar K, Nair HS (2018) Advertising content and consumer engagement on social media: evidence from Facebook. Manag Sci 64(11):5105–5131. https://doi.org/10.1287/mnsc.2017.2902
Lee DH, Im S, Taylor CR (2008) Voluntary self‐disclosure of information on the Internet: a multimethod study of the motivations and consequences of disclosing information on blogs. Psychol Mark 25(7):692–710. https://doi.org/10.1002/mar.20232
Lee J, Rajtmajer S, Srivatsavaya E, Wilson S (2023) Online self-disclosure, social support, and user engagement during the COVID-19 pandemic. ACM Trans Soc Comput 6(3-4):1–31. https://doi.org/10.1145/3617654
Lee Y, Lee J, Hwang Y (2015) Relating motivation to information and communication technology acceptance: self-determination theory perspective. Comput Hum Behav 51:418–428. https://doi.org/10.1016/j.chb.2015.05.021
Leite FP, Baptista PDP (2022) The effects of social media influencers’ self-disclosure on behavioral intentions: The role of source credibility, parasocial relationships, and brand trust. J Mark Theory Pr 30(3):295–311. https://doi.org/10.1080/10696679.2021.1935275
Leite FP, Pontes N, de Paula Baptista P (2022) Oops, I’ve overshared! When social media influencers’ self-disclosure damage perceptions of source credibility. Comput Hum Behav 133:107274. https://doi.org/10.1016/j.chb.2022.107274
León SP, Abad MJ, Rosas JM (2009) Giving contexts informative value makes information context-specific. Exp Psychol. https://doi.org/10.1027/1618-3169/a000006
Lou C, Tan SS, Chen X (2019) Investigating consumer engagement with influencer-vs. brand-promoted ads: The roles of source and disclosure. J Interact Advert 19(3):169–186. https://doi.org/10.1080/15252019.2019.1667928
Lou C, Yuan S (2019) Influencer marketing: how message value and credibility affect consumer trust of branded content on social media. J Interact Advert 19(1):58–73. https://doi.org/10.1080/15252019.2018.1533501
Luo M, Hancock JT (2020) Self-disclosure and social media: motivations, mechanisms and psychological well-being. Curr Opin Psychol 31:110–115. https://doi.org/10.1016/j.copsyc.2019.08.019
Article PubMed Google Scholar
Mahmood S, Khwaja MG, Jusoh A (2019) Electronic word of mouth on social media websites: role of social capital theory, self-determination theory, and altruism. Int J Space-Based Situat Comput 9(2):74–89. https://doi.org/10.1504/IJSSC.2019.104217
Majerczak P, Strzelecki A (2022) Trust, media credibility, social ties, and the intention to share towards information verification in an age of fake news. Behav Sci 12(2):51. https://doi.org/10.3390/bs12020051
Article PubMed PubMed Central Google Scholar
McAllister DJ (1995) Affect-and cognition-based trust as foundations for interpersonal cooperation in organizations. Acad Manag J 38(1):24–59. https://doi.org/10.5465/256727
Mehrabian A, Russell JA (1974). An approach to environmental psychology. The MIT Press
Minton EA (2015) In advertising we trust: Religiosity’s influence on marketplace and relational trust. J Advert 44(4):403–414. https://doi.org/10.1080/00913367.2015.1033572
Moorman C, Deshpande R, Zaltman G (1993) Factors affecting trust in market research relationships. J Mark 57(1):81–101. https://doi.org/10.1177/002224299305700106
Muntinga DG, Moorman M, Smit EG (2011) Introducing COBRAs: Exploring motivations for brand-related social media use. Int J Advert 30(1):13–46. https://doi.org/10.2501/IJA-30-1-013-046
Nadeem W, Tan TM, Tajvidi M, Hajli N (2021) How do experiences enhance brand relationship performance and value co-creation in social commerce? The role of consumer engagement and self brand-connection. Technol Forecast Soc 171:120952. https://doi.org/10.1016/j.techfore.2021.120952
Oestreicher-Singer G, Zalmanson L (2013) Content or community? A digital business strategy for content providers in the social age. MIS Quart 37(2):591–616. https://www.jstor.org/stable/43825924
Okazaki S (2009) Social influence model and electronic word of mouth: PC versus mobile internet. Int J Advert 28(3):439–472. https://doi.org/10.2501/S0265048709200692
Piehler R, Schade M, Kleine-Kalmer B, Burmann C (2019) Consumers’ online brand-related activities (COBRAs) on SNS brand pages: an investigation of consuming, contributing and creating behaviours of SNS brand page followers. Eur J Mark 53(9):1833–1853. https://doi.org/10.1108/EJM-10-2017-0722
Podsakoff PM, MacKenzie SB, Lee JY, Podsakoff NP (2003) Common method biases in behavioral research: a critical review of the literature and recommended remedies. J Appl Psychol 88(5):879. https://doi.org/10.1037/0021-9010.88.5.879
Pop RA, Săplăcan Z, Dabija DC, Alt MA (2022) The impact of social media influencers on travel decisions: The role of trust in consumer decision journey. Curr Issues Tour 25(5):823–843. https://doi.org/10.1080/13683500.2021.1895729
Pradhan B, Kishore K, Gokhale N (2023) Social media influencers and consumer engagement: a review and future research agenda. Int J Consum Stud 47(6):2106–2130. https://doi.org/10.1111/ijcs.12901
Qiu A, Chen M (2018) 基于UTAUT修正模型的微信朋友圈广告接受意愿分析 [Analysis of WeChat moments advertising acceptance intention based on a modified UTAUT model]. Stat Decis 34(12):99–102. https://doi.org/10.13546/j.cnki.tjyjc.2018.12.024
Qiu L, Kumar S (2017) Understanding voluntary knowledge provision and content contribution through a social-media-based prediction market: a field experiment. Inf Syst Res 28(3):529–546. https://doi.org/10.1287/isre.2016.0679
Racherla P, Mandviwalla M, Connolly DJ (2012) Factors affecting consumers’ trust in online product reviews. J Consum Behav 11(2):94–104. https://doi.org/10.1002/cb.385
Ranjbarian B, Gharibpoor M, Lari A (2012) Attitude toward SMS advertising and derived behavioral intension, an empirical study using TPB (SEM method). J Am Sci 8(7):297–307. https://www.ceeol.com/search/article-detail?id=466212
Robertshaw GS, Marr NE (2006) The implications of incomplete and spurious personal information disclosures for direct marketing practice. J Database Mark Custom Strategy Manag. 13:186–197. https://doi.org/10.1057/palgrave.dbm.3240296
Roh T, Seok J, Kim Y (2022) Unveiling ways to reach organic purchase: Green perceived value, perceived knowledge, attitude, subjective norm, and trust. J Retail Consum Serv 67:102988. https://doi.org/10.1016/j.jretconser.2022.102988
Schivinski B, Christodoulides G, Dabrowski D (2016) Measuring consumers’ engagement with brand-related social-media content: Development and validation of a scale that identifies levels of social-media engagement with brands. J Advert Res 56(1):64–80. https://doi.org/10.2501/JAR-2016-004
Schouten AP, Janssen L, Verspaget M (2021) Celebrity vs. Influencer endorsements in advertising: the role of identification, credibility, and product-endorser fit. Leveraged marketing communications, Routledge. pp. 208–231
Schramm H, Hartmann T (2008) The PSI-Process Scales. A new measure to assess the intensity and breadth of parasocial processes. Communications. https://doi.org/10.1515/COMM.2008.025
Shan Y, Chen KJ, Lin JS (2020) When social media influencers endorse brands: the effects of self-influencer congruence, parasocial identification, and perceived endorser motive. Int J Advert 39(5):590–610. https://doi.org/10.1080/02650487.2019.1678322
Shi Y (2018) The impact of consumer innovativeness on the intention of clicking on SNS advertising. Mod Econ 9(2):278–285. https://doi.org/10.4236/me.2018.92018
Article CAS Google Scholar
Simon F, Tossan V (2018) Does brand-consumer social sharing matter? A relational framework of customer engagement to brand-hosted social media. J Bus Res 85:175–184. https://doi.org/10.1016/j.jbusres.2017.12.050
Steinhoff L, Arli D, Weaven S, Kozlenkova IV (2019) Online relationship marketing. J Acad Mark Sci 47:369–393. https://doi.org/10.1007/s11747-018-0621-6
Stutzman F, Capra R, Thompson J (2011) Factors mediating disclosure in social network sites. Comput Hum Behav 27(1):590–598. https://doi.org/10.1016/j.chb.2010.10.017
Sun T, Youn S, Wu G, Kuntaraporn M (2006) Online word-of-mouth (or mouse): An exploration of its antecedents and consequences. J Comput-Mediat Comm 11(4):1104–1127. https://doi.org/10.1111/j.1083-6101.2006.00310.x
Sweet KS, LeBlanc JK, Stough LM, Sweany NW (2020) Community building and knowledge sharing by individuals with disabilities using social media. J Comput Assist Lear 36(1):1–11. https://doi.org/10.1111/jcal.12377
Tak P, Gupta M (2021) Examining travel mobile app attributes and its impact on consumer engagement: An application of SOR framework. J Internet Commer 20(3):293–318. https://doi.org/10.1080/15332861.2021.1891517
Towner E, Grint J, Levy T, Blakemore SJ, Tomova L (2022) Revealing the self in a digital world: a systematic review of adolescent online and offline self-disclosure. Curr Opin Psychol 45:101309. https://doi.org/10.1016/j.copsyc.2022.101309
Vander Schee BA, Peltier J, Dahl AJ (2020) Antecedent consumer factors, consequential branding outcomes and measures of online consumer engagement: current research and future directions. J Res Interact Mark 14(2):239–268. https://doi.org/10.1108/JRIM-01-2020-0010
Van-Tien Dao W, Nhat Hanh Le A, Ming-Sung Cheng J, Chao Chen D (2014) Social media advertising value: The case of transitional economies in Southeast Asia. Int J Advert 33(2):271–294. https://doi.org/10.2501/IJA-33-2-271-294
Viswanathan V, Hollebeek LD, Malthouse EC, Maslowska E, Jung Kim S, Xie W (2017) The dynamics of consumer engagement with mobile technologies. Serv Sci 9(1):36–49. https://doi.org/10.1287/serv.2016.0161
Voss KE, Spangenberg ER, Grohmann B (2003) Measuring the hedonic and utilitarian dimensions of consumer attitude. J Mark Res 40(3):310–320. https://doi.org/10.1509/jmkr.40.3.310.19238
Vrontis D, Makrides A, Christofi M, Thrassou A (2021) Social media influencer marketing: A systematic review, integrative framework and future research agenda. Int J Consum Stud 45(4):617–644. https://doi.org/10.1111/ijcs.12647
Wang T, Yeh RKJ, Chen C, Tsydypov Z (2016) What drives electronic word-of-mouth on social networking sites? Perspectives of social capital and self-determination. Telemat Inf 33(4):1034–1047. https://doi.org/10.1016/j.tele.2016.03.005
Watson JB (1917) An Attempted formulation of the scope of behavior psychology. Psychol Rev 24(5):329. https://doi.org/10.1037/h0073044
Wehmeyer ML (1999) A functional model of self-determination: Describing development and implementing instruction. Focus Autism Dev Dis 14(1):53–61. https://www.imdetermined.org/wp-content/uploads/2018/06/SD5_A-Functional-Model-of.pdf
Wei X, Chen H, Ramirez A, Jeon Y, Sun Y (2022) Influencers as endorsers and followers as consumers: exploring the role of parasocial relationship, congruence, and followers’ identifications on consumer–brand engagement. J Interact Advert 22(3):269–288. https://doi.org/10.1080/15252019.2022.2116963
Wirth J, Maier C, Laumer S (2019) Subjective norm and the privacy calculus: explaining self-disclosure on social networking sites. Paper presented at the 27th European Conference on Information Systems (ECIS). Stockholm & Uppsala, Sweden, 8–14, June 2019 https://aisel.aisnet.org/ecis2019_rp
Xiao L, Li X, Zhang Y (2023) Exploring the factors influencing consumer engagement behavior regarding short-form video advertising: a big data perspective. J Retail Consum Serv 70:103170. https://doi.org/10.1016/j.jretconser.2022.103170
Yang J, Peng MYP, Wong S, Chong W (2021) How E-learning environmental stimuli influence determinates of learning engagement in the context of COVID-19? SOR model perspective. Front Psychol 12:584976. https://doi.org/10.3389/fpsyg.2021.584976
Yang K, Jolly LD (2009) The effects of consumer perceived value and subjective norm on mobile data service adoption between American and Korean consumers. J Retail Consum Serv 16(6):502–508. https://doi.org/10.1016/j.jretconser.2009.08.005
Yang S, Zhou S, Cheng X (2019) Why do college students continue to use mobile learning? Learning involvement and self‐determination theory. Brit J Educ Technol 50(2):626–637. https://doi.org/10.1111/bjet.12634
Yusuf AS, Busalim AH (2018) Influence of e-WOM engagement on consumer purchase intention in social commerce. J Serv Mark 32(4):493–504. https://doi.org/10.1108/JSM-01-2017-0031
Zhang G, Yue X, Ye Y, Peng MYP (2021) Understanding the impact of the psychological cognitive process on student learning satisfaction: combination of the social cognitive career theory and SOR model. Front Psychol 12:712323. https://doi.org/10.3389/fpsyg.2021.712323
Zhang J, Liu J, Zhong W (2019) 广告精准度与广告效果:基于隐私关注的现场实验 [Ad targeting accuracy and advertising effectiveness: a field experiment based on privacy concerns]. Manag Sci 32(06):123–132
CAS Google Scholar
Download references
Acknowledgements
The authors thank all the participants of this study. The participants were all informed about the purpose and content of the study and voluntarily agreed to participate. The participants were able to stop participating at any time without penalty. Funding for this study was provided by Minjiang University Research Start-up Funds (No. 324-32404314).
Author information
Authors and affiliations.
School of Journalism and Communication, Minjiang University, Fuzhou, China
School of Journalism and Communication, Shanghai University, Shanghai, China
Qiuting Duan
You can also search for this author in PubMed Google Scholar
Contributions
Conceptualization: CG; methodology: CG and QD; software: CG and QD; validation: CG; formal analysis: CG and QD; investigation: CG and QD; resources: CG; data curation: CG and QD; writing—original draft preparation: CG; writing—review and editing: CG; visualization: CG; project administration: CG. All authors have read and agreed to the published version of the manuscript.
Corresponding author
Correspondence to Chenyu Gu .
Ethics declarations
Competing interests.
The authors declare no competing interests.
Ethical approval
The questionnaire and methodology for this study were approved by the School of Journalism and Communication, Minjiang University, Committee on Ethical Research (No. MJUCER20230621). The procedures used in this study adhere to the tenets of the Declaration of Helsinki.
Informed consent
Informed consent was obtained from all participants and/or their legal guardians.
Additional information
Publisher’s note Springer Nature remains neutral with regard to jurisdictional claims in published maps and institutional affiliations.
Supplementary information
Rights and permissions.
Open Access This article is licensed under a Creative Commons Attribution 4.0 International License, which permits use, sharing, adaptation, distribution and reproduction in any medium or format, as long as you give appropriate credit to the original author(s) and the source, provide a link to the Creative Commons licence, and indicate if changes were made. The images or other third party material in this article are included in the article’s Creative Commons licence, unless indicated otherwise in a credit line to the material. If material is not included in the article’s Creative Commons licence and your intended use is not permitted by statutory regulation or exceeds the permitted use, you will need to obtain permission directly from the copyright holder. To view a copy of this licence, visit http://creativecommons.org/licenses/by/4.0/ .
Reprints and permissions
About this article
Cite this article.
Gu, C., Duan, Q. Exploring the dynamics of consumer engagement in social media influencer marketing: from the self-determination theory perspective. Humanit Soc Sci Commun 11 , 587 (2024). https://doi.org/10.1057/s41599-024-03127-w
Download citation
Received : 17 December 2023
Accepted : 23 April 2024
Published : 08 May 2024
DOI : https://doi.org/10.1057/s41599-024-03127-w
Share this article
Anyone you share the following link with will be able to read this content:
Sorry, a shareable link is not currently available for this article.
Provided by the Springer Nature SharedIt content-sharing initiative
Quick links
- Explore articles by subject
- Guide to authors
- Editorial policies

Review of Marketing Research: Volume 6
Table of contents, review of marketing research, ad hoc reviewers, a review of prior classifications of purchase behavior and a proposal for a new typology, measuring customer lifetime value, learning models, customer co-creation, challenges in measuring return on marketing investment, service-dominant logic, marketing in a world with costs of price adjustment, about the editor and contributors.
- Naresh K. Malhotra
We’re listening — tell us what you think
Something didn’t work….
Report bugs here
All feedback is valuable
Please share your general feedback
Join us on our journey
Platform update page.
Visit emeraldpublishing.com/platformupdate to discover the latest news and updates
Questions & More Information
Answers to the most commonly asked questions here
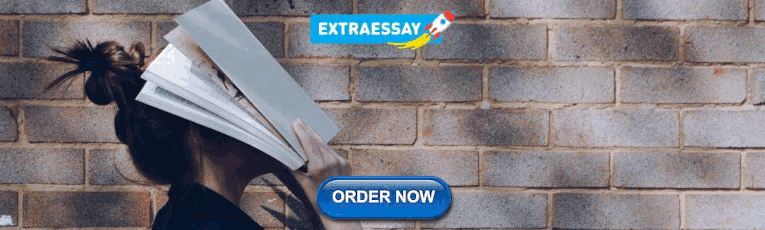
COMMENTS
A more careful analysis of the conceptualizations in Table 1 helps us discern several recurring themes that reflect the three central elements of market innovation. First, most conceptualizations employ a structural notion of market. For example, they refer to product-market structures (Darroch & Miles, 2011), exchange structures (Giesler, 2012), market norms and market representations ...
This is why the literature review as a research method is more relevant than ever. Traditional literature reviews often lack thoroughness and rigor and are conducted ad hoc, rather than following a specific methodology. Therefore, questions can be raised about the quality and trustworthiness of these types of reviews.
Specifically, a review of the recent academic and practitioner marketing literature (e.g., Cuevas, 2018; ... Based on what they consider the key benefits of review papers, we identify four major contributions of our literature review to the SMI research domain. First, we critically evaluated the methodological approaches and theories commonly ...
In this article, we reviewed the market innovation literature, iden-. ti ed six research clusters, discussed their interrelations, identi ed. major shifts in the literature, and proposed new ...
The purpose of this article is to contribute to ongoing discussions in academic marketing aimed at fostering greater attention to conceptual and theoretical research (e.g. Hunt, 2018; Lehmann, 2005; MacInnis, 2011; Vargo & Koskela-Huotari, 2020; Yadav, 2010).Unlike other approaches to the validation of theory in academic marketing, whereby empirical and mathematical proofs are seen as the main ...
Review of Marketing Research, now in its 10th volume, is a fairly recent publication covering the important areas of marketing research with a more comprehensive state-of-the-art orientation.The chapters in this publication review the literature in a particular area, offer a critical commentary, develop an innovative framework, and discuss future developments, as well as present specific ...
Review of Marketing Research All Books; Recent Chapters; All books in this series (20 titles) Artificial Intelligence in Marketing, Volume 20. Measurement in Marketing, Volume 19. Marketing Accountability for Marketing and Non-marketing Outcomes, Volume 18 ...
Review of Marketing Research covers the important areas of marketing research with a more comprehensive state-of-the-art orientation. Each of the articles in this publication will review the literature in a particular area, offer a critical commentary, develop an innovative framework, and discuss the future developments, in addition to ...
Review of Marketing Research, now in its seventh volume, is a fairly recent publication covering the important areas of marketing research with a more comprehensive state-of-the-art orientation.The chapters in this publication review the literature in a particular area, offer a critical commentary, develop an innovative framework, and discuss future developments, as well as present specific ...
Lamberton C, Stephen A T. A thematic exploration of digital, SM, and mobile marketing: research evolution from 2000 to 2015 and an agenda for future inquiry. Journal of Marketing 2016 ... Fernandes C, Raposo M. Social media marketing: A literature review and implications. Psychology and Marketing 2016; 33: 1029-1038. Crossref. Google Scholar ...
By way of a systematic literature review (SLR), the article evaluates 57 qualifying publications in the context of AI-powered marketing and qualitatively and quantitatively ranks them based on their coverage, impact, relevance, and contributed guidance, and elucidates the findings across various sectors, research contexts, and scenarios.
Examples of literature reviews. Step 1 - Search for relevant literature. Step 2 - Evaluate and select sources. Step 3 - Identify themes, debates, and gaps. Step 4 - Outline your literature review's structure. Step 5 - Write your literature review.
Marketing is the process of promoting and selling products and services, including market research and advertising. Today, marketing is an essential part of any organization's growth strategy. Many firms use marketing methods unknowingly to promote themselves and increase sales of their products and services. Marketing is one of the most ...
Without it, quantitative market This literature review consists of four sections: â ¢ The first section provides a brief history of survey sam- pling and the theoretical basis for market research anal- ysis, providing context for what became the standard procedures and expectations of market research. ... Because this is an emerging area of ...
A Literature Review on Digital Marketing: The Evolution of a. Revolution. Marina Basimakopoulou 1*, Kostas Theologou 1 and Panagiotis Tzavaras 2. 1 National Technical University of Athens, Greece ...
The contribution of the present paper is to extend and update these earlier reviews by providing the first-ever systematic literature review of research into the effects of marketing by schools. A key initial aim of our review was to map and scope the breadth of existing literature on the types of marketing practices employed by schools within ...
The amount of research related to Internet marketing has grown rapidly since the dawn of the Internet Age. A review of the literature base will help identify the topics that have been explored as well as identify topics for further research. This research project collects, synthesizes, and analyses both the research strategies (i.e., methodologies) and content (e.g., topics, focus, categories ...
Further literature review reveals the applicability of self-determination theory to consumer engagement behaviors, particularly in the context of influencer marketing advertisements ...
MAHATMA GANDHI COLLEGE OF PHARMACEUTICAL SCIENCES 1. The Role of Marketing Research: A L iterature Review. Saurav Kumar, Dr. Dilip Agarwal, Dr. Gaurav Bhaduka, Bhanu Pratap Singh 4. 1 Student, B ...
In general, literature reviews are structured in a similar way to a standard essay, with an introduction, a body and a conclusion. These are key structural elements. Additionally, a stand-alone extended literature review has an abstract. Throughout, headings and subheadings are used to divide up the literature review into meaningful sections.
The basis of the article is a literature review of positioning research published in academic journals between 1969 and 2017. These articles range across such disciplines as marketing, strategic marketing and management. ... International Journal of Research in Marketing: 1: 11: Journal of Advertising: 1: 12: Journal of Advertising Research: 1: ...
Linked Open Literature Review using the Neuro-symbolic Open Research Knowledge Graph. Pages 1015-1018. ... Comparing Research Contributions in a Scholarly Knowledge Graph. In Proceedings of the Third International Workshop on Capturing Scientific Knowledge co-located with the 10th International Conference on Knowledge Capture (K-CAP 2019 ...
The literature review highlights uncertainty about whether time, market size, and number of competing firms constrain or facilitate market concentration. The sector-dependent nature of market concentration encourages studies in diverse industries, such as elite international sport where there is a death of market concentration studies.
CSR decoupling refers to the misalignment between a company's stated CSR policies and its actual practices, resulting in issues like diminished financial performance and heightened risk. While initially explored in developed economies such as the US, recent research has shifted focus towards developing nations like China. However, a comprehensive review of CSR decoupling literature in the ...
Abstract. Stock markets are without any doubt, an integral and indispensable part of a country's economy. But the impact of stock markets on the country's economy can be different from how the ...
Alexander Ljungqvist. Share this: Private equity is a $3 trillion asset class, attracting many investors. This literature review synthesizes the main insights of the academic literature on private equity, focusing on its performance, value creation, and key aspects for investors. The Economics of Private Equity: A Critical Review Read PDF.
Review of Marketing Research | Editors: Naresh K. Malhotra. Citation: Baumgartner, H. (2010), "A Review of Prior Classifications of Purchase Behavior and a Proposal for a New Typology", Malhotra, N.K. (Ed.) Review of Marketing Research (Review of Marketing Research, Vol. 6), Emerald Group Publishing Limited, Leeds, pp. 3-36.
The Journal of Housing Economics has recently published a paper entitled "Rent control effects through the lens of empirical research: An almost complete review of the literature", written by Dr Konstantin A. Kholodilin from the German Institute for Economic Research (DIW). It is a meta-study which summarises the empirical literature of the various effects of rent controls.
Due to their extended p-orbital delocalization, conjugated polymers absorb light in the range of visible-NIR frequencies. We attempt to exploit this property to create materials that compete with inorganic semiconductors in photovoltaic and light-emitting materials. Beyond competing for applications in photonic devices, organic conjugated compounds, polymers, and small molecules have also ...
Marketing Literature Review. April 1986. Journal of Marketing 50 (2):100-115. DOI: 10.1177/002224298605000209. Authors: Paul Surgi Speck. University of Missouri - St. Louis. References (76 ...