Image Unavailable

- To view this video download Flash Player
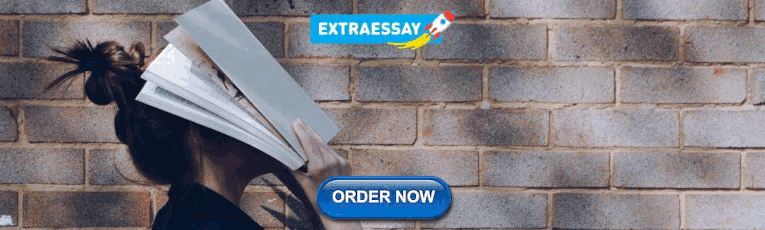
Artificial Intelligence in Education: Emerging Technologies, Models and Applications: Proceedings of 2021 2nd International Conference on Artificial ... and Communications Technologies, 104) 1st ed. 2022 Edition
This edited book is a collection of selected research papers presented at the 2021 2nd International Conference on Artificial Intelligence in Education Technology (AIET 2021), held in Wuhan, China on July 2-4, 2021. AIET establishes a platform for AI in education researchers to present research, exchange innovative ideas, propose new models, as well as demonstrate advanced methodologies and novel systems. Rapid developments in artificial intelligence (AI) and the disruptive potential of AI in educational use has drawn significant attention from the education community in recent years. For educators entering this uncharted territory, many theoretical and practical questions concerning AI in education are raised, and issues on AI’s technical, pedagogical, administrative and socio-cultural implications are being debated.
The book provides a comprehensive picture of the current status, emerging trends, innovations, theory, applications, challenges and opportunities of currentAI in education research. This timely publication is well-aligned with UNESCO’s Beijing Consensus on Artificial Intelligence (AI) and Education. It is committed to exploring how best to prepare our students and harness emerging technologies for achieving the Education 2030 Agenda as we move towards an era in which AI is transforming many aspects of our lives. Providing a broad coverage of recent technology-driven advances and addressing a number of learning-centric themes, the book is an informative and useful resource for researchers, practitioners, education leaders and policy-makers who are involved or interested in AI and education.
- ISBN-10 9811675260
- ISBN-13 978-9811675263
- Edition 1st ed. 2022
- Publisher Springer
- Publication date March 18, 2022
- Language English
- Dimensions 6.14 x 0.88 x 9.21 inches
- Print length 391 pages
- See all details

Editorial Reviews
From the back cover, about the author.
Dr. Eric Cheng is a specialist in educational management, knowledge management and Lesson Study. He is currently associate professor of the Department of Curriculum and Instruction of the Education University of Hong Kong. He is now serving as a school manager of Pentecostal Yu Leung Fat Primary School, C.C.C. Tam Lee Lai Fun Memorial Secondary School and E.L.C.H.K. Lutheran Secondary School. He is an Associate Editor of the International Journal of Educational Administration and Policy Studies (IJEAPS), a visiting scholar of Nagoya University, Aichi University of Education and Budapest Metropolitan University, an external examiner of The Open University of Hong Kong on the Master of Education programme and doctoral thesis examiner of Nottingham University on Doctor of Education program.
Dr. Rekha Koul is Dean International, Faculty of Humanities and Associate Professor at STEM Research Group, School of Education, Curtin University, Australia. She has nearly three decades of teaching and research experience. Her expertise lies in the development, refinement and validation of questionnaires; investigations of the effects of classroom environments on student outcomes; evaluation of educational programs; teacher-action research aimed at improving their environments and evaluation of curriculum. She has successfully secured many local, national and international research grants to the value of over one million dollars. Her publication record includes two books authored, seven books edited, eight book chapters and many journal articles published in peer-reviewed journals. She has delivered invited key note addresses and also conducts teacher professional learning workshops.
Rekha convenes International Conference on Science, Mathematics and Technology Education (icSTEM), a biannual conference in different parts of the world and has established a Learning Environment Research Centre in Indonesia.
She was the elected chair of Jumki Basu Scholarship (NARST 2016-18) and Programme Chair for Learning Environment SIG (AERA).
Dr. Tianchong Alex Wang is currently a Post-doctoral Fellow in the Department of Curriculum and Instruction at The Education University of Hong Kong. He received an EdD degree from the Chinese University of Hong Kong, an MSc degree from the University of Hong Kong, and a BA Hons degree from Hong Kong Baptist University. Dr Wang has a solid technical background in Information and Communication Technologies (ICTs); he is holding a number of professional qualifications such MCP, MCSA, MCSE, MCDBA, MCTS, MCITP, ACSP, ACTC, and THXCP. He is also a member of IEEE. As an advocate of using digital technologies to enhance education quality and inclusion., Dr Wang’s current research interests include AI in assessment, blended learning, mobile learning, flipped classrooms, ICT-enabled learning at scale, and digital learning for development. He has been participating research projects commissioned or supported by international organisations such as UNESCO, IDRC and The HEADFoundation as well as local research funding bodies such as Education Bureau (EDB) and The University Grants Committee (UGC) of Hong Kong. Dr Wang is a passionate teacher educator. He serves as a PT Lecturer at the University of Hong Kong who teaches in the MSc (IT in Education) programme as well as a resource person who conducts teacher capacity-building activities for international organisations and government agencies.
Product details
- Publisher : Springer; 1st ed. 2022 edition (March 18, 2022)
- Language : English
- Hardcover : 391 pages
- ISBN-10 : 9811675260
- ISBN-13 : 978-9811675263
- Item Weight : 1.66 pounds
- Dimensions : 6.14 x 0.88 x 9.21 inches
- #2,356 in Artificial Intelligence (Books)
- #2,810 in Database Storage & Design
- #6,346 in Statistics (Books)
Customer reviews
Customer Reviews, including Product Star Ratings help customers to learn more about the product and decide whether it is the right product for them.
To calculate the overall star rating and percentage breakdown by star, we don’t use a simple average. Instead, our system considers things like how recent a review is and if the reviewer bought the item on Amazon. It also analyzed reviews to verify trustworthiness.
No customer reviews
- Amazon Newsletter
- About Amazon
- Accessibility
- Sustainability
- Press Center
- Investor Relations
- Amazon Devices
- Amazon Science
- Sell on Amazon
- Sell apps on Amazon
- Supply to Amazon
- Protect & Build Your Brand
- Become an Affiliate
- Become a Delivery Driver
- Start a Package Delivery Business
- Advertise Your Products
- Self-Publish with Us
- Become an Amazon Hub Partner
- › See More Ways to Make Money
- Amazon Visa
- Amazon Store Card
- Amazon Secured Card
- Amazon Business Card
- Shop with Points
- Credit Card Marketplace
- Reload Your Balance
- Amazon Currency Converter
- Your Account
- Your Orders
- Shipping Rates & Policies
- Amazon Prime
- Returns & Replacements
- Manage Your Content and Devices
- Recalls and Product Safety Alerts
- Conditions of Use
- Privacy Notice
- Consumer Health Data Privacy Disclosure
- Your Ads Privacy Choices
- About the Repository
Artificial intelligence in education: Emerging technologies, models and applications
- Department of Curriculum and Instruction (C&I)
Research output : Book/Report › Books
- Artificial intelligence
- AI in education
- Education technology
- Smart learning
- Intelligent and interactive technologies
- Online education
- Machine learning
- Deep learning
- Flipped classroom
- Intelligent tutoring system
This output contributes to the following UN Sustainable Development Goals (SDGs)
Access to Document
- 10.1007/978-981-16-7527-0
Other files and links
- Find@EdUHK Library

Artificial intelligence in education
Artificial Intelligence (AI) has the potential to address some of the biggest challenges in education today, innovate teaching and learning practices, and accelerate progress towards SDG 4. However, rapid technological developments inevitably bring multiple risks and challenges, which have so far outpaced policy debates and regulatory frameworks. UNESCO is committed to supporting Member States to harness the potential of AI technologies for achieving the Education 2030 Agenda, while ensuring that its application in educational contexts is guided by the core principles of inclusion and equity. UNESCO’s mandate calls inherently for a human-centred approach to AI . It aims to shift the conversation to include AI’s role in addressing current inequalities regarding access to knowledge, research and the diversity of cultural expressions and to ensure AI does not widen the technological divides within and between countries. The promise of “AI for all” must be that everyone can take advantage of the technological revolution under way and access its fruits, notably in terms of innovation and knowledge.
Furthermore, UNESCO has developed within the framework of the Beijing Consensus a publication aimed at fostering the readiness of education policy-makers in artificial intelligence. This publication, Artificial Intelligence and Education: Guidance for Policy-makers , will be of interest to practitioners and professionals in the policy-making and education communities. It aims to generate a shared understanding of the opportunities and challenges that AI offers for education, as well as its implications for the core competencies needed in the AI era

The UNESCO Courier, October-December 2023

- Plurilingual
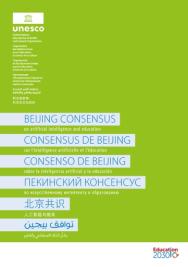
by Stefania Giannini, UNESCO Assistant Director-General for Education
International Forum on artificial intelligence and education
- More information
- Analytical report

Through its projects, UNESCO affirms that the deployment of AI technologies in education should be purposed to enhance human capacities and to protect human rights for effective human-machine collaboration in life, learning and work, and for sustainable development. Together with partners, international organizations, and the key values that UNESCO holds as pillars of their mandate, UNESCO hopes to strengthen their leading role in AI in education, as a global laboratory of ideas, standard setter, policy advisor and capacity builder. If you are interested in leveraging emerging technologies like AI to bolster the education sector, we look forward to partnering with you through financial, in-kind or technical advice contributions. 'We need to renew this commitment as we move towards an era in which artificial intelligence – a convergence of emerging technologies – is transforming every aspect of our lives (…),' said Ms Stefania Giannini, UNESCO Assistant Director-General for Education at the International Conference on Artificial Intelligence and Education held in Beijing in May 2019. 'We need to steer this revolution in the right direction, to improve livelihoods, to reduce inequalities and promote a fair and inclusive globalization.’'

Related items
- Artificial intelligence
- Open access
- Published: 19 September 2022
The application of AI technologies in STEM education: a systematic review from 2011 to 2021
- Weiqi Xu 1 &
- Fan Ouyang ORCID: orcid.org/0000-0002-4382-1381 1
International Journal of STEM Education volume 9 , Article number: 59 ( 2022 ) Cite this article
66k Accesses
54 Citations
12 Altmetric
Metrics details
The application of artificial intelligence (AI) in STEM education (AI-STEM), as an emerging field, is confronted with a challenge of integrating diverse AI techniques and complex educational elements to meet instructional and learning needs. To gain a comprehensive understanding of AI applications in STEM education, this study conducted a systematic review to examine 63 empirical AI-STEM research from 2011 to 2021, grounded upon a general system theory (GST) framework.
The results examined the major elements in the AI-STEM system as well as the effects of AI in STEM education. Six categories of AI applications were summarized and the results further showed the distribution relationships of the AI categories with other elements (i.e., information, subject, medium, environment) in AI-STEM. Moreover, the review revealed the educational and technological effects of AI in STEM education.
Conclusions
The application of AI technology in STEM education is confronted with the challenge of integrating diverse AI techniques in the complex STEM educational system. Grounded upon a GST framework, this research reviewed the empirical AI-STEM studies from 2011 to 2021 and proposed educational, technological, and theoretical implications to apply AI techniques in STEM education. Overall, the potential of AI technology for enhancing STEM education is fertile ground to be further explored together with studies aimed at investigating the integration of technology and educational system.
Introduction
Artificial intelligence in education (AIEd) is an emerging interdisciplinary field that applies AI technologies in education to transform and promote the instructional and learning design, process and assessment (Chen et al., 2020 ; Holmes et al., 2019 ; Hwang et al., 2020 ). The application of AI in STEM education (referred to AI-STEM in this paper), as a sub-branch of AIEd, focuses on the design and implementation of AI applications to support STEM education. Automated AI technologies, e.g., intelligence tutoring, automated assessment, data mining and learning analytics, have been used in STEM education to enhance the instruction and learning quality (Chen et al., 2020 ; Hwang et al., 2020 ; McLaren et al., 2010 ). STEM education is a complex system, from a system perspective, consisting of interdependent elements, including subject, information, medium, and environment (Rapoport, 1986 ; Von Bertalanffy, 1968 ). The application of AI, as a critical technology element, should take careful consideration of these complex factors, to achieve a high-quality STEM education (Byrne & Callaghan, 2014 ; Krasovskiy, 2020 ; Xu & Ouyang, 2022 ). This systematic review aims to examine the different elements, including AI technology, subject, information, medium, environment in the AI-STEM system to gain a holistic understanding of the application and integration of AI technologies in the STEM education contexts. Specifically, we collected and reviewed empirical AI-STEM research from 2011 to 2021, summarized the AI techniques and applications, the characteristics of other system elements (i.e., information, subject, medium, environment), the distribution of AI in these elements, and the effects of AI in STEM education. Based on the results, this systematic review provided educational and technological implications for the practice and research in the AI-STEM education.
Literature review
With the development of computer science and computational technologies, automatic, adaptive, and efficient AI technologies have been widely applied in various academic fields. Artificial Intelligence in Education (AIEd), as an interdisciplinary field, emphasizes applying AI to assist instructor’s instructional process, empower student’s learning process, and promote the transformation of educational system (Chen et al., 2020 ; Holmes et al., 2019 ; Hwang et al., 2020 ; Ouyang & Jiao, 2021 ). First, AIEd has potential to enhance instructional design and pedagogical development in the teaching processes, such as accessing students’ performance automatically (Wang et al., 2011 ; Zampirolli et al., 2021 ), monitoring and tracking students’ learning (Berland et al., 2015 ; Ji & Han, 2019 ), and predicting at-risk students (Hellings & Haelermans, 2020 ; Lamb et al., 2021 ). Second, AIEd is beneficial for improving student-centered learning, such as providing adaptive tutoring (Kose & Arslan, 2017 ; Myneni et al., 2013 ), recommending personalized learning resources (Ledesma & García, 2017 ; Zhang et al., 2020 ), and diagnosing students’ learning gaps (Liu et al., 2017 ). Third, AIEd also brings opportunities to transform the educational system by highlighting the essential role of technology (Hwang et al., 2020 ), enriching the mediums of knowledge delivery (Holstein et al., 2019 ; Yannier et al., 2020 ), and changing the instructor–student relationship (Xu & Ouyang, 2022 ). Overall, different AI technologies (e.g., machine learning, deep learning) have been deployed in the field of education to enhance instructional and learning process.
The development of AIEd also brought transformations to the field of science, technology, engineering and mathematics (STEM) education, as a sub-branch of AIEd named AI-STEM. STEM education aims to improve students’ interdisciplinary knowledge inquiry and application, as well as their higher-order thinking, critical thinking and problem-solving ability (Bybee, 2013 ; Pimthong & Williams, 2018 ). The application of AI in STEM education has advantages to provide adaptive and personalized learning environments or resources, and aid instructors to understand students’ learning behavioral patterns, and automatically assess STEM learning performances (Alabdulhadi & Faisal, 2021 ; Walker et al., 2014 ). However, STEM education is a complex system, consisting of interdependent elements, including subject (e.g., instructor, student), information, medium, and environment (Rapoport, 1986 ; Von Bertalanffy, 1968 ). Achieving a high quality of STEM education requires a careful consideration of the complex social, pedagogical, environmental factors, rather than merely applying AI technologies in education (Krasovskiy, 2020 ; Xu & Ouyang, 2022 ). Therefore, a major challenge in AI-STEM is how to appropriately select and apply AI techniques to adapt to the multiple elements (e.g., subject, information, environment) in STEM education with a goal of high-quality instruction and learning (Castañeda & Selwyn, 2018 ; Selwyn, 2016 ). To gain a holistic understanding of the integration of AI technologies in the STEM education contexts, it is crucial to systematically review and examine the complex elements in AI-STEM from a system perspective.
During the past decade, the emerging field of AIEd has gained great attention (Chen et al., 2020 ; Holmes et al., 2019 ; Hwang et al., 2020 ; Ouyang et al., 2022 ). But existing literature review of AIEd has mainly focused on the trends, applications, and effects of AIEd from a technological perspective (Chen et al., 2020 ; Tang et al., 2021 ; Zawacki-Richter et al., 2019 ). Specifically, we located 18 literature review articles of AIEd published from 2011 to 2021 (see Fig. 1 ). These AIEd reviews focused on different educational levels, fields, and contexts, including higher education (Zawacki-Richter et al., 2019 ), e-learning (Tang et al., 2021 ), mathematics education (Hwang & Tu, 2021 ), language education (Liang et al., 2021 ), medical education (Khandelwal et al., 2019 ; Lee et al., 2021 ), programming education (Le et al., 2013 ), and special education (Drigas & Ioannidou, 2012 ). For example, Zawacki-Richter et. al. ( 2019 ) reviewed AIEd in the higher education context and four AI technical applications were classified, namely intelligent tutoring systems, adaptive systems and personalization, profiling and prediction, and assessment and evaluation. Liang et. al. ( 2021 ) focused on the application of AI in language education and investigated the roles and research foci (e.g., research methods, research sample groups) of AI techniques in language education. Drigas and Ioannidou ( 2012 ) explored AIEd in special education and summarized AI applications based on the student’s disorders, including reading, writing and spelling difficulties, dyslexia, autistic spectrum disorder, etc.
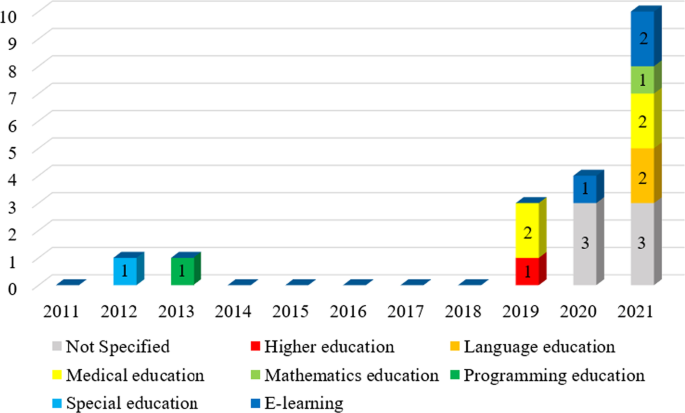
Existing literature review of AIEd articles, ranging from 2011 to 2021
Although various reviews were conducted to understand the field of AIEd, few of them focused on STEM education. Among these 18 literature review articles, we only located two works exploring the application of AI in STEM education. Le et. al. ( 2013 ) reviewed the AI-supported tutoring approaches in computer programming education and found that AI techniques were mainly applied to support feedback-based programming tutoring during the student’s individual learning. Hwang and Tu ( 2021 ) conducted a bibliometric mapping analysis to systematically review the roles of AI in mathematics education. The results clarified the role of AI in mathematics education into three main types, including intelligent tutoring systems, profiling and prediction, and adaptive systems and personalization. Although some review examined AI in computer science and mathematics education, there is a lack of literature review to investigate the application of AI in general STEM education context. More importantly, due to the complexity of AI-STEM, it is essential to systematically review multiple elements in AI-STEM as well as the effects of AI in the STEM education system.
To fill this gap, this systematic review aims to gain a comprehensive understanding of the integration of AI technologies in the STEM education contexts. Specifically, this review examined and summarized the applications and categories of AI element in the AI-STEM system, the characteristics of other system elements in AI-STEM except AI, the distribution of AI in these elements, and the effects of AI in STEM education. Three research questions (RQs) were proposed:
RQ1: What are the categories of the AI element in the AI-STEM system?
Rq2: what are the characteristics of other system elements (i.e., information, subject, medium, environment element) as well as the distribution of ai in these elements, rq3: what are the effects of ai in stem education.
In order to map the state-of-art of the application of AI techniques in STEM education, we conducted a systematic review from 2011 to 2021, following the Preferred Reporting Items for Systematic Reviews and Meta-Analyses (PRISMA) principles (Moher et al., 2009 ).
Database search
To locate the empirical studies of AI application in STEM education, the following major publisher databases were selected: Web of Science, Science Direct, Scopus, IEEE, EBSCO, ACM, Taylor & Francis, and Wiley (Guan et al., 2020 ). Filters were used to the empirical research and peer-reviewed articles in the field of education and educational research from January 2011 to December 2021. After the preliminary screening of articles, snowballing was conducted (Wohlin, 2014 ) to find the articles that were not extracted using the search strings.
Identification of search terms
Based on the specific requirements of bibliographic databases, we proposed the searching strategies. In terms of the research questions, three types of keywords were used as the search terms. First, keywords related to AIEd and specific AI applications were added (i.e., “artificial intelligence” OR “AI” OR “AIED” OR “machine learning” OR “intelligent tutoring system” OR “expert system” OR “recommended system” OR “recommendation system” OR “feedback system” OR “personalized learning” OR “adaptive learning” OR “prediction system” OR “student model” OR “learner model” OR “data mining” OR “learning analytics” OR “prediction model” OR “automated evaluation” OR “automated assessment” OR “robot” OR “virtual agent” OR “algorithm”). Second, keywords related to STEM were added (i.e., “STEM” OR “science” OR “technology” OR “math” OR “physics” OR “chemistry” OR “biology” OR “geography” OR “engineering” OR “programming” OR “lab”). Third, keywords related to education were added (i.e., “education” OR “learning” OR “course” OR “class” OR “teaching”).
Searching criteria
The search criteria were designed to locate the articles that focused on the applications of AI in STEM education. According to the research objectives, inclusion and exclusion criteria were adopted (see Table 1 ).
The screening process
The screening process involved the following procedures: (1) removing the duplicated articles; (2) reading the titles and abstracts and removing the articles according to the inclusion and exclusion criteria; (3) reading the full texts and removing the articles according to the inclusion and exclusion criteria; (4) using the snowballing to further locate the articles in Google Scholar, and (5) extracting data from the final filtered articles (see Fig. 2 ). All articles were imported into Mendeley software for screening.
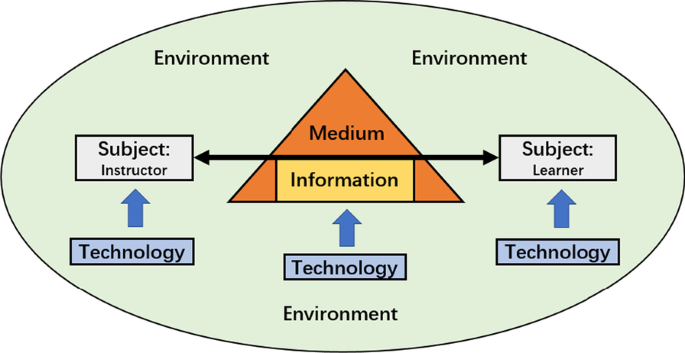
The selection flowchart used based on PRISMA (Moher et al., 2009 )
3373 articles were located as the result of the first round of searching. Among these records, 777 duplicates were removed and then 1879 records were excluded because they were not classified under Education & Educational research or journal article. By reviewing the titles and abstracts, the number of articles was reduced to 717 based on the criteria (see Table 1 ). The selected articles were examined by the first author to determine whether they were suitable for the purpose of this systematic review. The second author independently reviewed approximately 30% of the articles to confirm the reliability. The inter-rater agreement was 92%. Then, the full-text of articles were reviewed by the first author to verify that the articles met all the criteria for inclusion in the review. Finally, a total of 63 articles that met the criteria were identified for the systematic review.
Theoretical framework and analysis procedure
General system theory (GST) is a theoretical framework, arguing that the world is composed of different organic systems, which contain dynamically interacting elements and mutual relationships between them (Rapoport, 1986 ; Von Bertalanffy, 1950 ). The main principle of GST is that a system is not simply equal to the sum of its elements, but greater than the sum of its parts (Drack & Pouvreau, 2015 ; Von Bertalanffy, 1968 ). To deeply understand the complex nature and general rules of systems, GST highlights the system’s holistic principle to identify the internal elements, functional relationships of them, as well as the external influences upon a system (Crawford, 1974 ). The theoretical framework of GST has been widely applied in various fields to analyze different types of systems, such as physical, biological, social and educational systems (Drack & Pouvreau, 2015 ; Kitto, 2014 ). For example, Chen and Stroup ( 1993 ) suggested applying GST as an underpinned theoretical framework to guide the reform of science education and highlighted the integration of science curriculum to avoid the compartmentalized learning of physics, biology, and chemistry. Following this philosophy, we argue that GST can provide a new, holistic perspective to understand the integration of AI technologies and STEM education.
From the perspective of GST, AI-STEM can be viewed as an organic system, which mainly contains five basic elements, namely subject , information , medium , environment , and technology (Von Bertalanffy, 1968 ) (see Fig. 3 ). First, subject is defined as people in an educational system and different subjects of people (e.g., instructor, student) can take agency to interact with each other constantly and adaptively. Second, information refers to knowledge spread and constructed between subjects in an educational system, such as learning contents, course materials, knowledge artifacts, etc. Third, medium is the way or carrier to convey information and connect subjects in the system. Fourth, environment serves as an underlying context in an educational system, which influences the function of the whole educational system. Fifth, technology (e.g., AI techniques) is usually appeared as an external element to impact the functions of the educational system. Grounded upon GST, the integration of AI, as an external technology element, in an educational system (such as STEM) is a complex process, that has influences on other system elements (i.e., subject, information, medium, environment) and on the relationships between them. In summary, the framework of GST (see Fig. 3 ) highlights the multiple elements as well as their mutual relationships in AI-STEM system, which provides us a holistic view for applying AI technologies in STEM education.
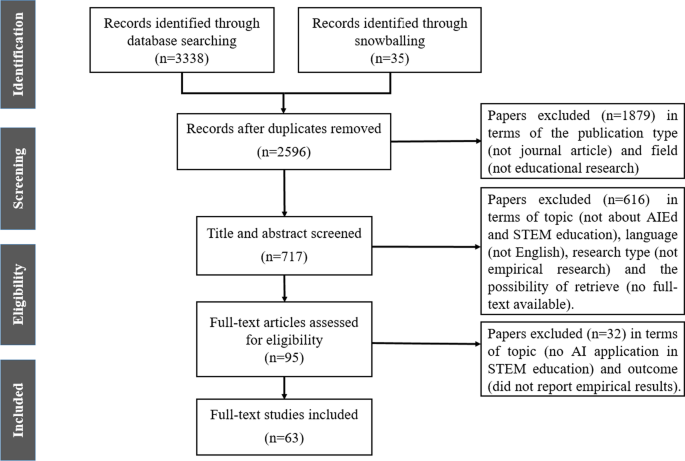
The integration of technology in an educational system from the GST perspective
We used content analysis method (Cohen et al., 2005 ; Zupic & Čater, 2015 ) to classify 63 AI-STEM articles in order to answer the research questions. Based on GST, a coding scheme of educational system elements was developed to systematically examine AI-STEM articles (see Table 2 ). This coding scheme included the subject of instructor (including instructor involvement and instructor strategy), the subject of learner (including educational level, sample size, and learning outcome), information (i.e., learning content), medium (i.e., educational medium), environment (i.e., educational context), and technology (i.e., AI technique).
63 articles were coded by two raters. The same article can be coded more than one code in one dimension. First, 20% of articles were coded by two coders independently in order to calculate coding reliability. Krippendorff’s ( 2004 ) alpha reliability was 0.91 among two raters at this phase. The remaining articles were coded independently by two raters after the reliability was ensured. Consensus was reached by two raters on conflicting coding results. We provided details and examples below to demonstrate how the coding results represented the review data (Graneheim & Lundman, 2004 ).
To answer the research questions, the results section presents the following three main topics: (1) the categories of the AI element in the AI-STEM system; (2) the characteristics of other system elements (i.e., information, subject, medium, and environment) as well as the distribution of AI in these elements, and (3) the effects and findings of the application of AI in STEM education.
Figure 4 demonstrates the trends of empirical studies by year. According to the distribution, the number of publication generally increased along the years. In addition, a majority of reviewed articles ( N = 42) were published in the last 4 years from 2018 to 2021. Only 9 of 63 reviewed articles were published in the first 4 years from 2011 to 2014.
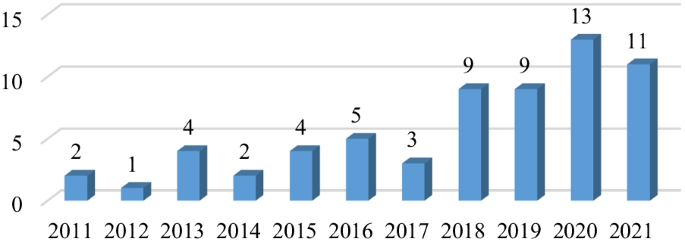
Distribution of the articles by year ( N = 63)
Regarding the element of AI technology in AI-STEM, six types of AI applications were identified, namely learning prediction ( N = 18, percentage = 29%), intelligent tutoring system ( N = 16, percentage = 25%), student behavior detection ( N = 13, percentage = 21%), automation ( N = 8, percentage = 13%), educational robots ( N = 6, percentage = 9%), and others ( N = 2, percentage = 3%) (see Fig. 5 and Table 3 ).
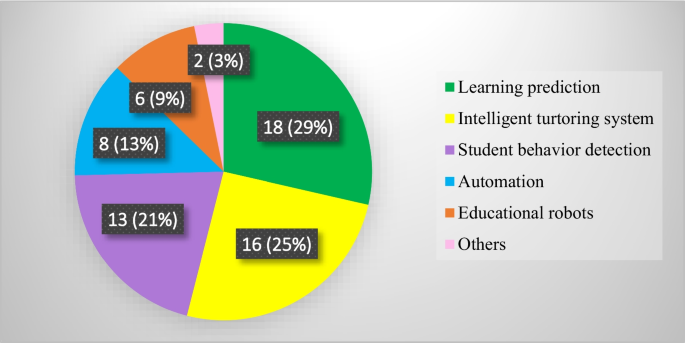
The application categories of AI techniques in STEM education ( N = 63)
Learning prediction
The first category of the AI applications in STEM education was learning prediction , illustrating should be something like systems which predict student learning performance or status in advance through AI algorithms and modeling approaches (Agrawal & Mavani, 2015 ; Lee et al., 2017 ). 18 of 63 reviewed articles (29%) focused on learning prediction in STEM education (see Fig. 5 ). Two sub-categories were summarized under learning prediction: learning performance prediction ( N = 14) and at-risk student prediction ( N = 4) (see Table 3 ). First, in the sub-category of learning performance prediction, AI algorithms and modeling techniques were employed in STEM education to help instructors adjust the instructional processes by predicting students’ learning performance (Deo et al., 2020 ; Hellings & Haelermans, 2020 ). For example, Buenaño-Fernández et. al. ( 2019 ) applied educational data mining and machine learning technique (i.e., decision tree) in computer engineering courses to predict students’ final performance based on their historical grades. Zabriskie et. al. ( 2019 ) utilized the random forest model and logistic regression model to predict the physics course outcomes. Another sub-category was predicting at-risk students and dropout factors in STEM education to help instructors intervene in student learning (Vyas et al., 2021 ; Yang et al., 2020 ). For example, Lacave et. al. ( 2018 ) used Bayesian networks techniques to investigate dropout factors of computer science students in higher education. Yang et. al. ( 2020 ) utilized random forest classification to create and examine the prediction models of identifying at-risk students in introductory physics classes. In summary, AI algorithms had been used in STEM education to help instructors or researchers predict students’ final academic performances and learning risks.
Intelligent tutoring system
The second category of the AI applications in STEM education was the intelligent tutoring system (ITS), defined as an AI-enabled system that was designed to provide customized instruction or feedback to students and promote personalized, adaptive learning (Chen et al., 2020 ; Hooshyar et al., 2015 ; Murray, 2003 ). Among the 63 reviewed articles, 16 articles (25%) focused on the applications of ITSs in promoting instruction and learning in STEM education (see Fig. 4 ). Three sub-categories were identified: instructional content delivery ( N = 9), recommendation of personalized learning path ( N = 4), and resource recommendation ( N = 3) (see Table 3 ). The first sub-category was using ITSs to deliver instructional content in STEM education. For example, Myneni et. al. ( 2013 ) introduced an interactive and intelligent learning system in physics education, where a virtual agent delivered physics concepts to students and decision algorithms were utilized to determine the support level of the virtual agent. Hooshyar et. al. ( 2018 ) proposed a novel flowchart-based ITS based on Bayesian networks techniques, which imitated a human instructor to conduct one-to-one instruction with students. The second sub-category of ITS was recommendations of personalized learning path based on student’s profile in STEM education. For example, De-Marcos et. al. ( 2015 ) combined genetic algorithm and parliamentary optimization algorithm to create personalized courseware sequencing paths in online STEM learning. Saito and Watanobe ( 2020 ) proposed an approach of recommending learning paths that applied recurrent neural network and sequential prediction model to create students’ ability charts and learning paths in programming learning based on their submission history. The third sub-category of ITS was recommending learning resource according to student’s needs in STEM education. For example, Ledesma and García ( 2017 ) introduced an expert system as a support tool to tackle mathematical topics, by recommending appropriate mathematical problems in accordance with a student’s learning style. Lin and Chen ( 2020 ) proposed a deep learning recommendation based system in programming learning that recommended learning tasks, learning missions and materials according to students’ learning processes and levels. In summary, AI technologies were widely applied in ITSs to enhance personalized and adaptive learning in STEM education through providing one-to-one tutoring and recommending personalized learning paths and resources.
Student behavior detection
The third category of the AI applications in STEM education was student behavior detection , which referred to systems to exploit and track students’ learning behaviors, patterns, and characteristics with AI-enabled data mining and learning analytics in the instructional and learning processes (Chrysafiadi & Virvou, 2013 ; Ji & Han, 2019 ; Zheng et al., 2020 ). Among the 63 reviewed articles, 13 articles (21%) focused on the applications of AI techniques to detect student behaviors in STEM education (see Fig. 5 ). Two sub-categories were summarized under student behavior detection: student behavior analysis ( N = 8) and student behavior monitoring ( N = 5) (see Table 3 ). First, the sub-category of student behavior detection, was applied in STEM education to analyze and reveal students’ latent behaviors. For example, Hsiao et. al. ( 2020 ) collected students’ learning data from programming learning platform and examined their learning behaviors through hidden Markov model, and the results revealed the reviewing patterns and reflecting strategies of students in learning programming. Pereira et. al. ( 2020 ) used data mining techniques including k-means and association rule algorithm to understand students’ behavior in introductory programming, to help novice programmers promote their learning. Another sub-category of student behavior monitoring was applied to help instructors track students’ learning in STEM education. For example, Balakrishnan ( 2018 ) helped instructors to motivate engineering students’ learning through monitoring their learning behaviors such as preferred learning materials and self-directed learning performance. Yannier et. al. ( 2020 ) introduced a mixed-reality AI system supported with computer vision algorithms to track children’s active learning behaviors in science education. In summary, student behavior detection had great potential to aid instructors and researchers to analyze, understand, and monitor students’ behaviors in STEM education.
The fourth category of the AI applications in STEM education was automation , which utilized AI technologies to automatically assess students’ performances and generate questions or tasks for instructors (Aldabe & Maritxalar, 2014 ; Wang et al., 2011 ; Zampirolli et al., 2021 ). Among 63 reviewed articles, 8 articles (13%) focused on the AI-supported automated techniques in STEM education (see Fig. 5 ). Two sub-categories were summarized under automation: automated assessment ( N = 7) and automated questions generation ( N = 1) (see Table 3 ). The first sub-category of automated assessment provided instructors and students with convenient assistance in STEM education. For example, Wang et. al. ( 2011 ) developed an automated assessment system, AutoLEP, to help novice programmers gain programming skills by providing syntactic and structural checking and immediate feedback automatically. García-Gorrostieta et. al. ( 2018 ) introduced a system for automatic argument assessment of computer engineering students’ final reports, to help them improve the abilities of statement and justification in science argumentation. Another sub-category of automated questions generation had potential to reduce instructors’ instructional burdens in STEM education. For example, Aldabe and Maritxalar ( 2014 ) proposed an approach to help instructors automatically create multiple-choice tests in science courses through the use of corpora and natural language processing techniques. In summary, AI techniques were used in STEM education to aid instructors and students through automatically generating questions and assessing academic performances.
Educational robots
The fifth category of the AI applications in STEM education was educational robots , which was the adoption of robots in STEM education to facilitate students’ learning experience as well as allow them to acquire knowledge in interactive ways (Atman Uslu et al., 2022 ; Cao et al., 2021 ; Yang & Zhang, 2019 ). It is worth noting that robots are applications that contain various techniques (e.g., mechanical manufacturing, electronic sensors, AI); therefore, considering the research topic, only AI-supported robots were included in this review. Among the 63 reviewed articles, 6 articles (9%) focused on the application of educational robots in STEM education (see Fig. 5 ). Two sub-categories were identified under educational robots: programming robots ( N = 3) and social robots ( N = 3) (see Table 3 ). The first sub-category, programming robots, were specifically designed as learning tools that engaged students to design and operate them with programming languages (Atman Uslu et al., 2022 ). For example, Rodríguez Corral et. al. ( 2016 ) applied a specific ball-shaped robot with sensing, wireless communication and output capabilities in computer courses to teach students object-oriented programming languages. Cao et. al. ( 2021 ) introduced an artificial intelligence robot called LEGO MINDSTORMS EV3, to implement instructional tasks in information technology courses to promote students’ innovation and operational ability. Another sub-category of social robots was a kind of intelligent humanoid robots, which could serve as tutors, tutees or learning companions to students and allow students to interact with them orally and physically (Belpaeme et al., 2018 ; Xu & Ouyang, 2022 ). For example, Verner et. al. ( 2020 ) employed RoboThespian, a life-size humanoid robot, as a tutor to convey science knowledge and concepts to elementary school students. In summary, AI-based educational robots were used in STEM education as instructional tools or educational subjects (e.g., tutor, tutee, companion) to convey knowledge, promote students’ operational skills, and enhance their learning experience.
Among 63 reviewed articles, 2 articles (3%) focused on other applications of AI techniques in STEM education, including AI textbook and group formation. Tehlan et. al. ( 2020 ) utilized a genetic algorithm‐based approach to form student groups in collaborative learning based on their skills and personality traits in a programming course. Koć-Januchta et. al. ( 2020 ) introduced AI-enriched textbook in biology course to improve students’ engagement by encouraging them to ask questions and receive suggested questions.
To further understand how AI techniques have been integrated in STEM education, we examined the other system elements, including information, subject (i.e., instructor, student), medium, and environment in AI-STEM research. In addition, we explored the distribution of AI categories in these elements, to reveal the relationships between AI techniques and these elements.
Information in AI-STEM research
Information (referred to learning content in this study) was described as the subject knowledge and learning contents conveyed in AI-STEM system. In the reviewed 63 studies, all of them mentioned learning content in STEM education, including science, technology, engineering, mathematics, and cross-disciplinary (i.e., more than one discipline) (see Table 4 ). Among 63 articles, 24 studies focused on technology, followed by articles that focused on science ( N = 22) and engineering ( N = 7). Mathematics ( N = 3) attracted the least attention. In addition, 7 studies contained interdisciplinary subjects, such as computer engineering (Buenaño-Fernández et al., 2019 ; Tehlan et al., 2020 ), engineering mathematics (Deo et al., 2020 ), and integrated STEM education (Suh et al., 2019 ; Wang, 2016 ).
Figure 6 shows the frequency of AI application categories in different learning contents. Among all the AI categories, student behavior detection was most frequently applied in the technology domain ( N = 8), followed by learning prediction in science ( N = 6), and learning prediction in engineering ( N = 5) (see Fig. 6 ).
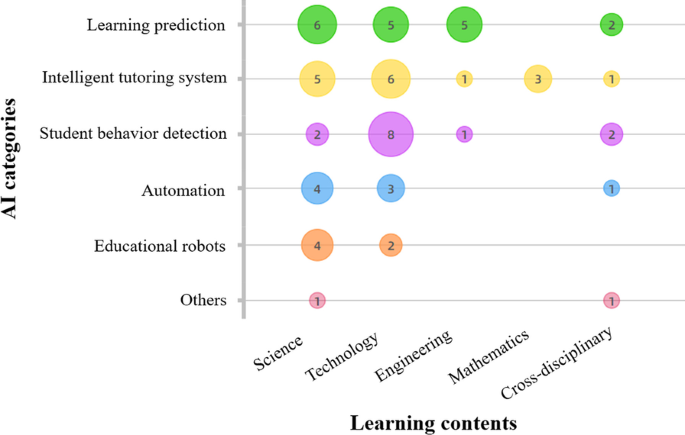
AI categories under learning content ( N = 63)
Instructor in AI-STEM research
Instructor , as a component of subject element in AI-STEM system, played a critical role in conducting instruction, conveying knowledge, and utilizing technologies. In the reviewed 63 studies, 50 of them mentioned the instructor involvement and 50 of them mentioned the instructional strategies, including traditional lecture, problem-based learning, project-based learning, game-based learning, self-learning, and collaborative learning (see Table 5 ). Regarding the instructor involvement, a majority of instructors would engage in the instructional and learning processes to support students ( N = 42), while some studies were conducted without instructors’ involvement and support ( N = 8). Additionally, among 50 articles, the traditional lecturing strategy was most frequently used by instructor ( N = 27), followed by problem-based learning ( N = 10). Also, some studies were carried out through project-based learning ( N = 5), self-learning ( N = 5), game-based learning ( N = 4), and collaborative learning ( N = 4).
All AI application categories were mainly applied with the instructor’s support, in which the most frequently used AI category were ITS ( N = 11) and learning prediction ( N = 11) (see Fig. 7 a). In addition, automation was only applied in lecture ( N = 8). Learning prediction was most frequently applied in lecture ( N = 8) and educational robots were most frequently applied in problem-based learning ( N = 4). Compared to other AI technologies, ITS and student behavior detection were integrated with more types of instructional strategies (see Fig. 7 b).
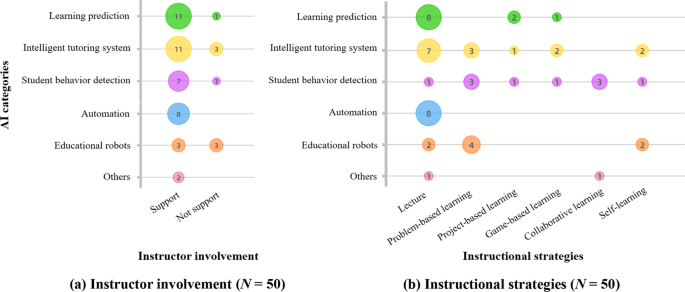
AI categories under instructor involvement and instructional strategies
Learner in AI-STEM research
Learner , as another component of subject element, could take agency to actively participate in the learning process as to influence the AI-STEM system. In the reviewed 63 studies, 59 of them mentioned the educational levels of learners, from kindergarten to higher education, and 55 of them mentioned sample sizes (see Table 6 ). Among all the educational levels, 43 focused on higher education ( N = 43), followed by elementary school ( N = 7), high school ( N = 5), and middle school ( N = 4). Only one study was conducted in kindergarten. In addition, the number of AI-STEM studies with the medium scale of learners ( N = 24) and the large scale of learners ( N = 21) were larger than the small-scale study ( N = 10).
AI application categories except educational robots were frequently applied in higher education (learning prediction: N = 13, ITS: N = 12, student behavior detection: N = 8, automation: N = 7). The educational robots were frequently applied in elementary school ( N = 3) (see Fig. 8 a). Moreover, regarding the sample size, learning prediction was most frequently used with a large scale ( N = 11), followed by ITS with a medium scale ( N = 9), and student behavior detection with a medium scale ( N = 7). Additionally, the categories of educational robots and others were not applied in large scale; the category of student behavior detection was not applied in small scale (see Fig. 8 b).
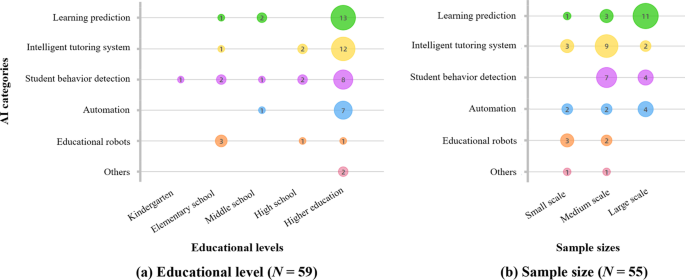
AI categories under educational levels and sample sizes
Medium in AI-STEM research
Medium (referred to educational medium in this study) was viewed as the way to convey information and connect subjects AI-STEM system. In the reviewed 63 studies, 50 of them mentioned the educational medium, including paper resource, entity resource (i.e., the material object in reality), computer system resource, web open resource, mobile phone resource and E-book resource (see Table 7 ). Among all educational mediums, computer system was the most frequently used in AI-STEM studies ( N = 28), followed by entity resource ( N = 10) and web open resource ( N = 9). Additionally, mobile phone resource ( N = 3), traditional paper resource ( N = 1), and E-book resource ( N = 1) was the infrequent medium to convey knowledge.
Among all the AI categories, ITS was most frequently used through computer system resource ( N = 15), followed by educational robots through entity resource ( N = 6), automation through computer system resource ( N = 5), and learning prediction through web open resource (see Fig. 9 ).
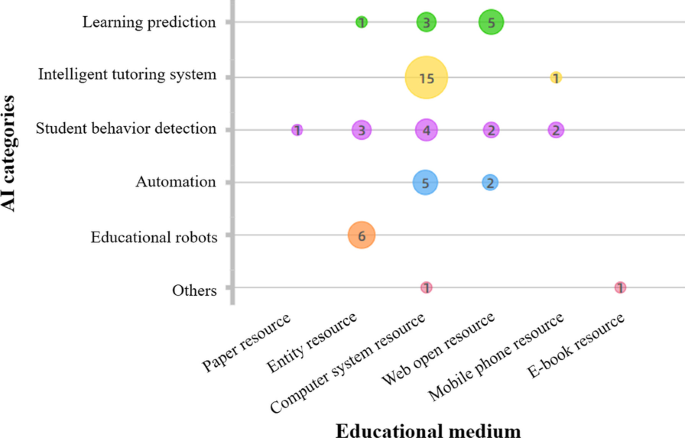
AI categories under educational medium ( N = 50)
Environment in AI-STEM research
Environment (referred to educational context in this study) served as an underlying context to influence the whole AI-STEM system. In the reviewed 63 studies, 51 of them mentioned the educational environment, including face-to-face environment, experimental learning environment, informal learning environment, web-based environment and augmented/virtual reality (see Table 8 ). Among the 51 studies, 33 studies were implemented in face-to-face environment, followed by web-based environment ( N = 11) and experimental environment ( N = 6). Two studies conducted in informal learning environment (McLurkin et al., 2013 ; Verner et al., 2020 ) and only one study conducted in augmented reality (Lin & Chen, 2020 ).
All categories of AI techniques were commonly applied in face-to-face environment, in which the most frequently used AI technology category was learning prediction ( N = 10), followed by automation ( N = 7), ITS ( N = 6), and educational robots ( N = 5). Moreover, compared to other AI categories, ITS was the most frequently used technique in the web-based environment ( N = 7) (see Fig. 10 ).
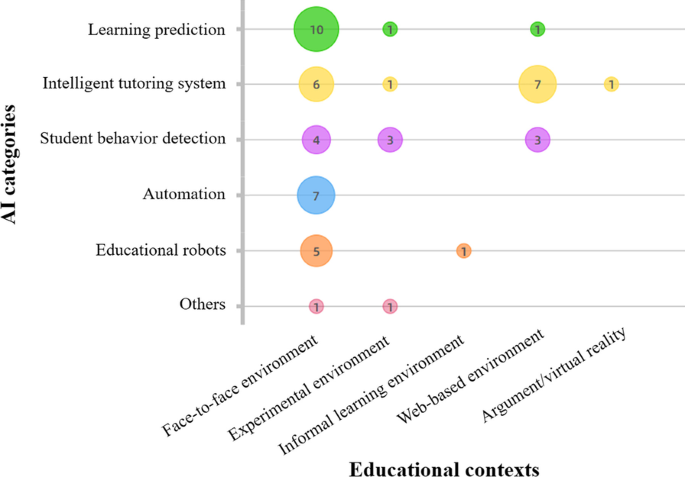
AI categories under educational contexts ( N = 51)
This review summarized the educational and technological effects of AI applications in AI-STEM research.
Educational effects and findings
From the educational perspective, 42 of the 63 reviewed articles reported the educational effects and findings when applying AI techniques in STEM education. Specifically, 30 out of the 42 articles reported the instruction and learning effects (e.g., learning performance, affective perception, higher-order thinking) of the application of AI techniques in STEM education. 12 articles out of 42 reported students’ learning behaviors and patterns by using AI-enabled data mining and learning analytics techniques.
The effect of learning performance
Among all the reviewed articles, 22 studies revealed the educational effects of AI technologies on students’ learning performance. Most of them showed significantly positive influence of AI techniques on the improvement of students’ learning performances ( N = 20). For example, Wu et. al. ( 2013 ) investigated the effect of a context-aware ubiquitous learning system in a geosciences course and the results showed that context-aware ubiquitous learning system had significantly positive effects on the learning achievements of students. Thai et. al. ( 2021 ) conducted a cluster randomized study to examine the effect of My Math Academy, a digital game-based learning environment that provided personalized content on kindergarten students; the results revealed the significant improvement of learning gains, especially for the moderate-level students. Tehlan et. al. ( 2020 ) used a quasi-experiment approach to examine the effects of genetic algorithm-supported pair programming in a programming course; the results found that the students’ learning performances were significantly higher in pair programming than individual programming. Two articles reported insignificant results of the learning performance effects. Koć-Januchta et. al. ( 2020 ) used a quasi-experiment to compare the effect of AI-enabled E-book and common E-book in students’ biology learning, and the results showed that there was no significant difference of students’ learning gains between these two types of books. Also, Hellings and Haelermans ( 2020 ) conducted a randomized experiment to examine the effect of a learning analytics dashboard with predictive function in a computer programming course, but no significant improvement was found on student performance in the final exam.
The effect of affective perception
Among all the reviewed articles, a majority of studies revealed the educational effects of AI technologies on students’ affective perception, such as attitude, interest, and motivation ( N = 17). On the one hand, students showed satisfaction and positive attitude towards the integration of AI technologies and STEM education. For example, Azcona et. al. ( 2019 ) used a questionnaire to find students’ positive feedbacks and attitudes towards the application of learning analytics in computer programming classes to detect and warn learning risks. Gavrilović et. al. ( 2018 ) evaluated student’s satisfaction of an AI-supported adaptive learning system in Java programming learning through the survey approach; the results revealed the positive feedbacks of students. On the other hand, the application of AI technologies also arouses students’ interests and motivation in STEM learning. For example, Balakrishnan ( 2018 ) used a mixed-method approach (i.e., questionnaire and interview) to examine the impact of a computer-based personalized learning environment (PLE) on engineering students’ motivation, and the results revealed the potential of PLE to engage students in learning with a strong sense of interest and motivation. Verner et. al. ( 2020 ) investigated students’ perceptions and attitudes towards an interactive robot tutor in science classes and found that the human–robot interaction fostered students’ active learning, maintain their attention and interest in the learning processes.
The effect of higher-order thinking
Among all the reviewed articles, some studies revealed the educational effects of AI technologies on students’ higher-order thinking ( N = 7), such as problem-solving ability, computational thinking, and self-regulated learning skills. For example, Hooshyar et. al. ( 2015 ) employed a quasi-experimental design to examine the impact of a flowchart-based intelligent tutoring system (FITS) on students’ programming learning and found better improvement of problem-solving abilities in the FITS group than the control group. Lin and Chen ( 2020 ) found that students who used a deep learning-based AR system performed significantly better in computational thinking than those using an AR system without deep learning recommendation. Jones and Castellano ( 2018 ) utilized adaptive robotic tutors to promote students’ self-regulated learning skills and found that when a robotic tutor provided scaffoldings adaptively, more self-regulated learning behaviors were observed from students over the control condition without scaffoldings. García-Gorrostieta et. al. ( 2018 ) used experimental evaluation to test the effect of the automatic argument assessment on students’ computer engineering writing, and the results revealed that the argument assessment system helped students improve argumentation ability in their writing.
The effect of student learning pattern and behavior
Among 42 reviewed articles that mentioned educational effects and findings, 12 articles revealed students’ learning patterns and behaviors in STEM education by using AI-enabled data mining and learning analytics approaches. For example, Sapounidis et. al. ( 2019 ) detected 48 children’s preference profiles on tangible and graphical programming through latent class modeling; results found that the graphical programming was preferred by a majority of children, especially children in younger ages. Pereira et. al. ( 2020 ) used learning analytics (i.e., k-means, association rule algorithms) in the Amazonas to understand students’ behavior in introductory programming courses and found high heterogeneity among them. Three clusters of novice programmers were detected to explain how student behaviors during programming influenced the learning outcomes. Wang ( 2016 ) utilized data mining and learning analytics techniques (i.e., association rule, decision tree) to investigate college students’ course-taking patterns in STEM learning; the results found that the most viable course-taking trajectories is taking mathematics courses after initial exposure to subject courses in STEM.
Technological effects and findings
From the technological perspective, 24 of the 63 reviewed articles reported the technological effect and findings (e.g., efficiency of technology, accuracy of algorithm) when applying AI techniques in STEM education. For example, Çınar et. al. ( 2020 ) utilized multiple machine learning algorithms, including Support Vector Machines (SVM), Gini, k-Nearest Neighbors (KNN), Breiman’s Bagging, Freund and Schapire’s Adaboost.M1 algorithms, to automatically grade open-ended physics questions; the results reported that AdaBoost.M1 had the best performance with the highest accuracy of prediction models among all machine learning algorithms. Nehm et. al. ( 2012 ) used a corpus of biology evolutionary explanations written by 565 undergraduates to test the efficacy of an automated assessment program, Summarization Integrated Development Environment (SIDE); the results showed that, compared to human expert scoring, SIDE had better performance when scoring models were built and tested at the individual item level, and the performance degraded when suites of items or entire instruments were used to build and test scoring models. Bertolini et. al. ( 2021 ) employed five machine learning methods to quantify predictive efficacy of predictive modeling in undergraduate students’ outcome in biology. Results found that individual machine learning methods, especially logistic regression achieved a poor prediction performance while ensemble machine learning methods, in particular the generalized linear model with elastic net (GLMNET), achieved the high accuracy. Deo et. al. ( 2020 ) adopted a computationally efficient AI model called extreme learning machines (ELM) to predict weighted score and the examination score in engineering mathematics courses; the results showed that ELM outperformed in prediction with respect to random forest and Volterra.
Discussion and implications
Addressing research questions.
Although AIEd has attracted wide attention in educational research and practice, few research works have investigated the applications of AI in STEM education context. To gain a comprehensive understanding of the integration of AI in STEM education, this study conducted a systematic review of AI-STEM empirical research from 2011 to 2021. Grounded upon GST, we examined the AI technologies and applications in STEM education, the characteristics of other system elements (i.e., information, subject, medium, environment), the distribution of AI in these elements, and the effects of AI applications in STEM education. To answer the first question, we found a gradually increasing trend of AI applications in STEM education in the past decade. Furthermore, six categories of AI applications were located, namely learning prediction, ITS, student behavior detection, automation, educational robots, and others (i.e., AI text book, group formation). Regarding the characteristics of elements and the distribution of AI in these elements, first, we found all categories of AI techniques, especially student behavior detection, ITS, and learning prediction, were frequently applied in the learning contents of science and technology. Second, instructors usually involved in STEM education to support students and they used lecturing strategy the most frequently, followed by problem-based learning. Automation was only applied in the lecturing instruction mode and educational robots were most frequently applied in the problem-based learning mode. Third, a majority of AI techniques (except educational robots) were applied in higher education with medium and large scale of learners. The most frequently used AI in higher education were learning prediction and ITSs. Fourth, computer system resource was the most frequently used medium to convey knowledge, particularly when it was applied in ITSs and automation, while paper, mobile phone, and E-book resources were seldom used in AI-STEM research. Fifth, the face-to-face environment was mainly utilized to support all categories of AI applications, and web-based environment was most frequently used supported with ITSs.
Regarding the third question, this review summarized the educational and technological effects and findings of AI applications in STEM. From the educational perspective, the results showed that most of the AI applications had positive effects on students’ academic performance. However, insignificant improvements of learning outcomes were also found in two empirical studies (Hellings & Haelermans, 2020 ; Koć-Januchta et al., 2020 ). Moreover, most students held positive attitudes towards the use of AI technology in STEM education, and AI technologies aroused their interest and motivation as well. In other words, the AI applications are beneficial for fostering student’s active learning in STEM education. Moreover, the applications of AI techniques also contributed to the development of students’ higher-order thinking, e.g., computational thinking, problem-solving ability. In addition, AI techniques have great potential to assist instructors by detecting students’ learning patterns and behaviors in STEM education. From the technological perspective, the reviewed articles mainly reported a good efficiency and algorithm accuracy when applying AI in STEM education. Specifically, AI algorithms, especially ensemble machine learning methods, performed well in learning prediction, automation, and personalized recommendation. Overall, underpinned by the GST framework, this review presented an overview of recent trends of the field of AI-STEM, which guided the following educational, technological, and theoretical implications.
Educational implications
The emergence of AI indirectly influences the subject elements (e.g., instructor, learner) in STEM education, which in turn would eventually influence the educational practices and effects. First, AI has potential to transform the instructor–student relationships in STEM education from the instructor-directed to student-centered learning (Cviko et al., 2014 ). When AI is applied in STEM education, the role of instructor is expected to shift from a leader to a collaborator or a facilitator under the AI-empowered, learner-as-leader paradigm (Ouyang & Jiao, 2021 ). However, this review found that the instructor-centered lecturing mode was the most frequently used instructional strategy in AI-STEM studies, while other student-centered instructional strategies (e.g., the project-based learning, collaborative learning, game-based learning) appeared infrequently. One of the reasons centers on the complexity of integrating technology and pedagogy in STEM education (Castañeda & Selwyn, 2018 ; Jiao et al., 2022 ; Loveless, 2011 ). For example, ITS and automation techniques are usually designed based on behaviorism (Skinner, 1953 ) to support instructor’s knowledge delivery and exam evaluation, which may be challenging for instructors to use when integrating it in the student-centered instructional strategies. Recent research has started to balance pedagogical design and technological application in educational practices in order to achieve the goal of AI–instructor collaboration and student-centered learning when AI is integrated (Baker & Smith, 2019 ; Holmes et al., 2019 ; Roll & Wylie, 2016 ). Furthermore, another critical question is: would AI replace instructor responsibilities and roles in STEM education (Segal, 2019 )? In this review, we found that the role of instructor was still irreplaceable, because the instructor’s involvement existed in most of the AI-STEM research. Even though AI can free instructors from redundant tasks in STEM education, it still lacks the human ability to convey social emotion, solve critical problems, and implement creative activities (Collinson, 1996 ; Gary, 2019 ; Muhisn et al., 2019 ). Therefore, although AI techniques can bring opportunities to develop STEM education (Hwang et al., 2020 ), we cannot overstate the role of technology and overlook the essential role of instructor (Selwyn, 2016 ). Overall, instructors, as important subjects in the educational system, need to take agency to promote the pedagogical designs and strategies when applying AI technologies, in order to achieve a high quality of AI-STEM education (Cantú-Ortiz et al., 2020 ).
Technological implications
Although AI has the potential to enhance the instruction and learning in STEM education (Chen et al., 2020 ; Holmes et al., 2019 ), the development of AI-STEM requires a better fit between AI technologies and other system elements in STEM education. First, regarding the relationships between AI and information element, the results showed that most of the AI applications were used in science and technology learning contents, and educational robots and automation were not applied in engineering and mathematics learning contents. Since STEM education contains interdisciplinary knowledge and learning contents from different subjects, AI is usually restricted in specific learning contents or courses (Douce et al., 2005 ). Therefore, one of the future directions is to expand the commonality and accessibility of AI techniques in different STEM subjects and courses. Second, the range of AI applications was mainly located in higher education, while few of AI techniques were applied in other educational levels, especially in kindergarten. To some extent, due to the complex function and feedback mechanisms, most of the AI techniques (e.g., ITS, learning prediction) might be appropriate for adult learners. Hence, some interactive AI techniques, e.g., social robots, AI-enabled games, can be designed and developed to support young children’s STEM learning (Belpaeme et al., 2018 ; Zapata-Cáceres & Martin-Barroso, 2021 ). Therefore, the ease of use is also one of the important considerations in future development of AI technologies (Law, 2019 ; Xu & Ouyang, 2022 ). Third, most of the AI-STEM research was conducted through the traditional mediums (e.g., computer system resource) and contexts (e.g., face-to-face learning environment). A future direction is to create AI-empowered STEM learning environment through combining the advanced educational mediums (e.g., E-book) and contexts (AR/VR), in order to better represent and convey knowledge (Mystakidis et al., 2021 ).
Theoretical implications
Due to the complexity of AI-STEM system, this research used a theoretical framework based on GST to examine the multiple elements (i.e., AI technology, information, subject, medium, environment) in AI-STEM research. Compared to previous AIEd reviews that mainly focused on the technological perspective, GST provides a holistic view for us to consider the complex human, pedagogical, environment factors when applying AI in STEM education (Kitto, 2014 ; Von Bertalanffy, 1968 ). For example, we found that sometimes instructors did not engage in STEM education to support students, especially when applying ITSs and educational robots. It might reveal a new trend that some AI technologies (e.g., social robot, virtual agent) might have the potential to replace the original role of instructor and work as a new subject to individually convey knowledge (Xu & Ouyang, 2022 ). Additionally, the results showed the different characteristics of learner’s sample size when applying different AI techniques. Learning prediction was more likely to applied with a large scale of students, and educational robots were inclined to be applied with a small scale of students. The features of AI technologies might explain this phenomenon. For example, a data training process is necessary before learning prediction, which requires the support of algorithmic modeling techniques and large data sets (Agrawal & Mavani, 2015 ; Lee et al., 2017 ), while educational robots, as human–machine interaction technologies, seem more suitable and practicable for small-scale STEM learning (Atman Uslu et al., 2022 ; Belpaeme et al., 2018 ). Overall, the current study utilized the GST framework to examine the multiple elements in the complex AI-STEM system; it is suggested that different stakeholders, e.g., educators, technical developers, and researchers, can adopt the GST framework as a guide to comprehensively consider the complex elements when applying AI techniques in STEM education (Kitto, 2014 ; Von Bertalanffy, 1950 ).
Conclusion, limitation, and future direction
The application of AI technology in STEM education is an emerging trend, which is confronted with the challenge of integrating diverse AI techniques in the complex STEM educational system. Grounded upon a GST framework, this research reviewed the empirical AI-STEM studies from 2011 to 2021. Specifically, this systematic review examined (1) the categories of the AI element in the AI-STEM system; (2) the characteristics of other system elements (i.e., information, subject, medium, and environment) as well as the distribution of AI in these elements, and (3) the effects of AI in STEM education. Based on the results, the current work proposed educational, technological, and theoretical implications for future AI-STEM research, to better aid the educators, researchers and technical developers to integrate the AI techniques and STEM education.
There are three limitations in this systematic review, which lead to future research directions. First, although we searched the best-known scholar databases with the keywords relevant to AI-STEM, some biases might exist in the searching and screening process. Since AI-STEM is a highly technology-dependent field, some studies might only highlight the technology rather than the education context. Therefore, future studies can adjust the searching criteria to solve these problems. Second, from a system perspective, we used a GST framework to examine the multiple elements in the complex AI-STEM system, but we did not investigate the mutual relationships between elements. Therefore, the complex relationships between different elements (e.g., instructor–learner, learner–learner relationship) in AI-STEM system need to be further explored in order to gain a deep understanding of the application of AI in STEM education (e.g., Xu & Ouyang, 2022 ). Third, the current study only implemented a systematic review, a meta-analysis could be conducted in the future to report the effect sizes of recent empirical studies to gain a deeper understanding of the effects of the AI-STEM integration in an educational system. Overall, the potential of AI technology for enhancing STEM education is fertile ground to be further explored together with studies aimed at investigating the integration of technology and educational system.
Availability of data and materials
The data are available upon request from the corresponding author.
*Reviewed articles ( N = 63)
Agrawal, H., & Mavani, H. (2015). Student performance prediction using machine learning. International Journal of Engineering Research and Technology, 4 (03), 111–113. https://doi.org/10.17577/IJERTV4IS030127
Article Google Scholar
Alabdulhadi, A., & Faisal, M. (2021). Systematic literature review of STEM self-study related ITSs. Education and Information Technologies, 26 , 1549–1588. https://doi.org/10.1007/s10639-020-10315-z
*Aldabe, I., & Maritxalar, M. (2014). Semantic similarity measures for the generation of science tests in Basque. IEEE Transactions on Learning Technologies, 7 (4), 375–387. https://doi.org/10.1109/TLT.2014.2355831
*Alemán, J. L. F. (2011). Automated assessment in a programming tools course. IEEE Transactions on Education, 54 (4), 576–581. https://doi.org/10.1109/TE.2010.2098442
Atman Uslu, N., Yavuz, G. Ö., & KoçakUsluel, Y. (2022). A systematic review study on educational robotics and robots. Interactive Learning Environments . https://doi.org/10.1080/10494820.2021.2023890
*Azcona, D., Hsiao, I. H., & Smeaton, A. F. (2019). Detecting students-at-risk in computer programming classes with learning analytics from students’ digital footprints. User Modeling and User-Adapted Interaction, 29 (4), 759–788. https://doi.org/10.1007/s11257-019-09234-7
Baker, T., & Smith, L. (2019). Educ-AI-tion rebooted? Exploring the future of artificial intelligence in schools and colleges. https://media.nesta.org.uk/documents/Future_of_AI_and_education_v5_WEB.Pdf
*Balakrishnan, B. (2018). Motivating engineering students learning via monitoring in personalized learning environment with tagging system. Computer Applications in Engineering Education, 26 (3), 700–710. https://doi.org/10.1002/cae.21924
Belpaeme, T., Kennedy, J., Ramachandran, A., Scassellati, B., & Tanaka, F. (2018). Social robots for education: A review. Science Robotics, 3 (21), eaat5954. https://doi.org/10.1126/scirobotics.aat5954
*Berland, M., Davis, D., & Smith, C. P. (2015). AMOEBA: Designing for collaboration in computer science classrooms through live learning analytics. International Journal of Computer-Supported Collaborative Learning, 10 (4), 425–447. https://doi.org/10.1007/s11412-015-9217-z
*Bertolini, R., Finch, S. J., & Nehm, R. H. (2021). Testing the impact of novel assessment sources and machine learning methods on predictive outcome modeling in undergraduate biology. Journal of Science Education and Technology, 30 (2), 193–209. https://doi.org/10.1007/s10956-020-09888-8
*Blikstein, P., Worsley, M., Piech, C., Sahami, M., Cooper, S., & Koller, D. (2014). Programming pluralism: Using learning analytics to detect patterns in the learning of computer programming. Journal of the Learning Sciences, 23 (4), 561–599. https://doi.org/10.1080/10508406.2014.954750
*Buenaño-Fernández, D., Gil, D., & Luján-Mora, S. (2019). Application of machine learning in predicting performance for computer engineering students: A case study. Sustainability, 11 (10), 1–18. https://doi.org/10.3390/su11102833
Bybee, R. W. (2013). The case for STEM education: Challenges and opportunities . NSTA Press.
Google Scholar
Byrne, D., & Callaghan, G. (2014). Complexity theory and the social sciences . Routledge.
Cantú-Ortiz, F. J., Galeano Sánchez, N., Garrido, L., Terashima-Marin, H., & Brena, R. F. (2020). An artificial intelligence educational strategy for the digital transformation. International Journal on Interactive Design and Manufacturing, 14 (4), 1195–1209. https://doi.org/10.1007/s12008-020-00702-8
*Cao, X., Li, Z., & Zhang, R. (2021). Analysis on academic benchmark design and teaching method improvement under artificial intelligence robot technology. International Journal of Emerging Technologies in Learning, 16 (5), 58–72. https://doi.org/10.3991/ijet.v16i05.20295
Castañeda, L., & Selwyn, N. (2018). More than tools? Making sense of the ongoing digitizations of higher education. International Journal of Educational Technology in Higher Education, 15 (1), 1–10. https://doi.org/10.1186/s41239-018-0109-y
Chen, D., & Stroup, W. (1993). General system theory: Toward a conceptual framework for science and technology education for all. Journal of Science Education and Technology, 2 (3), 447–459. https://doi.org/10.1007/BF00694427
Chen, L., Chen, P., & Lin, Z. (2020). Artificial intelligence in education: A review. IEEE Access, 8 , 75264–75278. https://doi.org/10.1109/ACCESS.2020.2988510
*Chrysafiadi, K., & Virvou, M. (2013). PeRSIVA: An empirical evaluation method of a student model of an intelligent e-learning environment for computer programming. Computers & Education, 68 , 322–333. https://doi.org/10.1016/j.compedu.2013.05.020
*Çınar, A., Ince, E., Gezer, M., & Yılmaz, Ö. (2020). Machine learning algorithm for grading open-ended physics questions in Turkish. Education and Information Technologies, 25 , 3821–3844. https://doi.org/10.1007/s10639-020-10128-0
Cohen, L., Manion, L., & Morrison, K. (2005). Research methods in education . Routledge Falmer.
Collinson, V. (1996). Reaching students: Teachers ways of knowing . Corwin Press.
Crawford, J. L. (1974). A systems approach model for the application of general systems theory principles to education [Doctoral dissertation, University of Houston]. The University of Houston Institutional Repository. https://hdl.handle.net/10657/10661
Cviko, A., McKenney, S., & Voogt, J. (2014). Teacher roles in designing technology-rich learning activities for early literacy: A cross-case analysis. Computers & Education, 72 , 68–79. https://doi.org/10.1016/j.compedu.2013.10.014
*De-Marcos, L., Garcia-Cabot, A., Garcia-Lopez, E., & Medina, J. A. (2015). Parliamentary optimization to build personalized learning paths: Case study in web engineering curriculum. International Journal of Engineering Education, 31 (4), 1092–1105.
*Deo, R. C., Yaseen, Z. M., Al-Ansari, N., Nguyen-Huy, T., Langlands, T. A. M., & Galligan, L. (2020). Modern artificial intelligence model development for undergraduate student performance prediction: An investigation on engineering mathematics courses. IEEE Access, 8 , 136697–136724. https://doi.org/10.1109/ACCESS.2020.3010938
Douce, C., Livingstone, D., & Orwell, J. (2005). Automatic test-based assessment of programming: A review. Journal on Educational Resources in Computing, 5 (3), 4-es. https://doi.org/10.1145/1163405.1163409
Drack, M., & Pouvreau, D. (2015). On the history of Ludwig von Bertalanffy’s “general systemology”, and on its relationship to cybernetics—Part III: Convergences and divergences. International Journal of General Systems, 44 (5), 523–571. https://doi.org/10.1080/03081079.2014.1000642
Drigas, A. S., & Ioannidou, R. E. (2012). Artificial intelligence in special education: A decade review. International Journal of Engineering Education, 28 (6), 1366. http://imm.demokritos.gr/publications/AI_IJEE.pdf
*Ferrarelli, P., & Iocchi, L. (2021). Learning Newtonian physics through programming robot experiments. Technology, Knowledge and Learning, 26 , 789–824. https://doi.org/10.1007/s10758-021-09508-3
*Figueiredo, M., Esteves, L., Neves, J., & Vicente, H. (2016). A data mining approach to study the impact of the methodology followed in chemistry lab classes on the weight attributed by the students to the lab work on learning and motivation. Chemistry Education Research and Practice, 17 (1), 156–171. https://doi.org/10.1039/c5rp00144g
*García-Gorrostieta, J. M., López-López, A., & González-López, S. (2018). Automatic argument assessment of final project reports of computer engineering students. Computer Applications in Engineering Education, 26 (5), 1217–1226. https://doi.org/10.1002/cae.21996
Gary, K. (2019). Pragmatic standards versus saturated phenomenon: Cultivating a love of learning. Journal of Philosophy of Education, 53 (3), 477–490. https://doi.org/10.1111/1467-9752.12377
*Gavrilović, N., Arsić, A., Domazet, D., & Mishra, A. (2018). Algorithm for adaptive learning process and improving learners’ skills in Java programming language. Computer Applications in Engineering Education, 26 (5), 1362–1382. https://doi.org/10.1002/cae.22043
Graneheim, U. H., & Lundman, B. (2004). Qualitative content analysis in nursing research: Concepts, procedures and measures to achieve trustworthiness. Nurse Education Today, 24 (2), 105–112. https://doi.org/10.1016/j.nedt.2003.10.001
Guan, C., Mou, J., & Jiang, Z. (2020). Artificial intelligence innovation in education: A twenty-year data-driven historical analysis. International Journal of Innovation Studies, 4 (4), 134–147. https://doi.org/10.1016/j.ijis.2020.09.001
*Hellings, J., & Haelermans, C. (2020). The effect of providing learning analytics on student behaviour and performance in programming: A randomised controlled experiment. Higher Education, 83 (1), 1–18. https://doi.org/10.1007/s10734-020-00560-z
Holmes, W., Bialik, M., & Fadel, C. (2019). Artificial intelligence in education: Promises and implications for teaching and learning . Center for Curriculum Redesign.
Holstein, K., McLaren, B. M., & Aleven, V. (2019). Co-designing a real-time classroom orchestration tool to support teacher—AI complementarity. Journal of Learning Analytics, 6 (2), 27–52. https://doi.org/10.18608/jla.2019.62.3
*Hooshyar, D., Ahmad, R. B., Yousefi, M., Yusop, F. D., & Horng, S. (2015). A flowchart-based intelligent tutoring system for improving problem-solving skills of novice programmers. Journal of Computer Assisted Learning, 31 , 345–361. https://doi.org/10.1111/jcal.12099
*Hooshyar, D., Binti Ahmad, R., Wang, M., Yousefi, M., Fathi, M., & Lim, H. (2018). Development and evaluation of a game-based Bayesian intelligent tutoring system for teaching programming. Journal of Educational Computing Research, 56 (6), 775–801. https://doi.org/10.1177/0735633117731872
*Hsiao, I. H., Huang, P. K., & Murphy, H. (2020). Integrating programming learning analytics across physical and digital space. IEEE Transactions on Emerging Topics in Computing, 8 (1), 206–217. https://doi.org/10.1109/TETC.2017.2701201
Hwang, G. J., & Tu, Y. F. (2021). Roles and research trends of artificial intelligence in mathematics education: A bibliometric mapping analysis and systematic review. Mathematics, 9 (6), 584. https://doi.org/10.3390/math9060584
Hwang, G. J., Xie, H., Wah, B. W., & Gašević, D. (2020). Vision, challenges, roles and research issues of artificial intelligence in education. Computers and Education: Artificial Intelligence, 1 , 100001. https://doi.org/10.1016/j.caeai.2020.100001
*Ji, Y., & Han, Y. (2019). Monitoring indicators of the flipped classroom learning process based on data mining—Taking the course of “virtual reality technology” as an example. International Journal of Emerging Technologies in Learning, 14 (3), 166–176. https://doi.org/10.3991/ijet.v14i03.10105
Jiao, P., Ouyang, F., Zhang, Q., & Alavi, A. H. (2022). Artificial intelligence-enabled prediction model of student academic performance in online engineering education. Artificial Intelligence Review . https://doi.org/10.1007/s10462-022-10155-y
*Jones, A., Bull, S., & Castellano, G. (2018). “I know that now, I’ m going to learn this next” Promoting self-regulated learning with a robotic tutor. International Journal of Social Robotics, 10 (4), 439–454. https://doi.org/10.1007/s12369-017-0430-y
*Jones, A., & Castellano, G. (2018). Adaptive robotic tutors that support self-regulated learning: A longer-term investigation with primary school children. International Journal of Social Robotics, 10 (3), 357–370. https://doi.org/10.1007/s12369-017-0458-z
*Khan, I., Ahmad, A. R., Jabeur, N., & Mahdi, M. N. (2021). Machine learning prediction and recommendation framework to support introductory programming course. International Journal of Emerging Technologies in Learning, 16 (17), 42–59. https://doi.org/10.3991/ijet.v16i17.18995
Khandelwal, P., Srinivasan, K., & Roy, S. S. (2019). Surgical education using artificial intelligence, augmented reality and machine learning: A review. In A. Sengupta & P. Eng (Eds.), 2019 IEEE international conference on consumer electronics—Taiwan (pp. 1–2). IEEE.
*Kinnebrew, J. S., Killingsworth, S. S., Clark, D. B., Biswas, G., Sengupta, P., Minstrell, J., Martinez-garza, M., & Krinks, K. (2017). Contextual markup and mining in digital games for science learning: Connecting player behaviors to learning goals. IEEE Transactions on Learning Technologies, 10 (1), 93–103. https://doi.org/10.1109/TLT.2016.2521372
Kitto, K. (2014). A contextualised general systems theory. Systems, 2 (4), 541–565. https://doi.org/10.3390/systems2040541
*Koć-Januchta, M. M., Schönborn, K. J., Tibell, L. A. E., Chaudhri, V. K., & Heller, H. C. (2020). Engaging with biology by asking questions: Investigating students’ interaction and learning with an artificial intelligence-enriched textbook. Journal of Educational Computing Research, 58 (6), 1190–1224. https://doi.org/10.1177/0735633120921581
*Kose, U., & Arslan, A. (2017). Optimization of self-learning in computer engineering courses: An intelligent software system supported by artificial neural network and vortex optimization algorithm. Computer Applications in Engineering Education, 25 (1), 142–156. https://doi.org/10.1002/cae.21787
*Krämer, N. C., Karacora, B., Lucas, G., Dehghani, M., Rüther, G., & Gratch, J. (2016). Closing the gender gap in STEM with friendly male instructors? On the effects of rapport behavior and gender of a virtual agent in an instructional interaction. Computers & Education, 99 , 1–13. https://doi.org/10.1016/j.compedu.2016.04.002
Krasovskiy, D. (2020). The challenges and benefits of adopting AI in STEM education. https://upjourney.com/the-challenges-and-benefits-of-adopting-ai-in-stem-education
Krippendorff, K. (2004). Reliability in content analysis: Some common misconceptions and recommendations. Human Communication Research, 30 (3), 411–433. https://doi.org/10.1093/hcr/30.3.411
*Lacave, C., Molina, A. I., & Cruz-Lemus, J. A. (2018). Learning analytics to identify dropout factors of computer science studies through Bayesian networks. Behaviour and Information Technology, 37 (10–11), 993–1007. https://doi.org/10.1080/0144929X.2018.1485053
*Lamb, R., Hand, B., & Kavner, A. (2021). Computational modeling of the effects of the science writing heuristic on student critical thinking in science using machine learning. Journal of Science Education and Technology, 30 (2), 283–297. https://doi.org/10.1007/s10956-020-09871-3
Law, N. W. Y. (2019). Human development and augmented intelligence. In The 20th international conference on artificial intelligence in education (AIED 2019), Chicago, IL, USA.
Le, N. T., Strickroth, S., Gross, S., & Pinkwart, N. (2013). A review of AI-supported tutoring approaches for learning programming. In N. Nguyen, T. Van Do, & H. Le Thi (Eds.), Advanced computational methods for knowledge engineering (pp. 267–279). Springer. https://doi.org/10.1007/978-3-319-00293-4_20
Chapter Google Scholar
*Ledesma, E. F. R., & García, J. J. G. (2017). Selection of mathematical problems in accordance with student’s learning style. International Journal of Advanced Computer Science and Applications, 8 (3), 101–105. https://doi.org/10.14569/IJACSA.2017.080316
Lee, J., Jang, D., & Park, S. (2017). Deep learning-based corporate performance prediction model considering technical capability. Sustainability, 9 (6), 899. https://doi.org/10.3390/su9060899
Lee, J., Wu, A. S., Li, D., & Kulasegaram, K. M. (2021). Artificial intelligence in undergraduate medical education: A scoping review. Academic Medicine, 96 (11S), S62–S70. https://doi.org/10.1097/ACM.0000000000004291
Liang, J. C., Hwang, G. J., Chen, M. R. A., & Darmawansah, D. (2021). Roles and research foci of artificial intelligence in language education: An integrated bibliographic analysis and systematic review approach. Interactive Learning Environments . https://doi.org/10.1080/10494820.2021.1958348
*Lin, P. H., & Chen, S. Y. (2020). Design and evaluation of a deep learning recommendation based augmented reality system for teaching programming and computational thinking. IEEE Access, 8 , 45689–45699. https://doi.org/10.1109/ACCESS.2020.2977679
Liu, M., Li, Y., Xu, W., & Liu, L. (2017). Automated essay feedback generation and its impact on revision. IEEE Transactions on Learning Technologies, 10 (4), 502–513. https://doi.org/10.1109/TLT.2016.2612659
Loveless, A. (2011). Technology, pedagogy and education: Reflections on the accomplishment of what teachers know, do and believe in a digital age. Technology, Pedagogy and Education, 20 (3), 301–316. https://doi.org/10.1080/1475939X.2011.610931
*Maestrales, S., Zhai, X., Touitou, I., Baker, Q., Schneider, B., & Krajcik, J. (2021). Using machine learning to score multi-dimensional assessments of chemistry and physics. Journal of Science Education and Technology, 30 (2), 239–254. https://doi.org/10.1007/s10956-020-09895-9
*Mahboob, K., Ali, S. A., & Laila, U. E. (2020). Investigating learning outcomes in engineering education with data mining. Computer Applications in Engineering Education, 28 (6), 1652–1670. https://doi.org/10.1002/cae.22345
*Matthew, F. T., Adepoju, A. I., Ayodele, O., Olumide, O., Olatayo, O., Adebimpe, E., Bolaji, O., & Funmilola, E. (2018). Development of mobile-interfaced machine learning-based predictive models for improving students’ performance in programming courses. International Journal of Advanced Computer Science and Applications, 9 (5), 105–115. https://doi.org/10.14569/IJACSA.2018.090514
McLaren, B. M., Scheuer, O., & Mikšátko, J. (2010). Supporting collaborative learning and e-discussions using artificial intelligence techniques. International Journal of Artificial Intelligence in Education, 20 (1), 1–46. https://doi.org/10.3233/JAI-2010-0001
McLurkin, J., Rykowski, J., John, M., Kaseman, Q., & Lynch, A. J. (2013). Using multi-robot systems for engineering education: Teaching and outreach with large numbers of an advanced, low-cost robot. IEEE Transactions on Education, 56 (1), 24-33. https://doi.org/10.1109/TE.2012.2222646
Moher, D., Liberati, A., Tetzlaff, J., Altman, D. G., Prisma Group. (2009). Preferred reporting items for systematic reviews and meta-analyses: The PRISMA statement. PLoS Medicine, 6 (7), e1000097. https://doi.org/10.1371/journal.pmed.1000097.t001
Muhisn, Z. A. A., Ahmad, M., Omar, M., & Muhisn, S. A. (2019). The Impact of socialization on collaborative learning method in e-learning management system (eLMS). International Journal of Emerging Technologies in Learning, 14 (20), 137–148.
Murray, T. (2003). An overview of intelligent tutoring system authoring tools: Updated analysis of the state of the art. In T. Murray, S. B. Blessing, & S. Ainsworth (Eds.), Authoring tools for advanced technology learning environments (pp. 491–544). Springer. https://doi.org/10.1007/978-94-017-0819-7_17
*Myneni, L. S., Narayanan, N. H., & Rebello, S. (2013). An interactive and intelligent learning system for physics education. IEEE Transactions on Learning Technologies, 6 (3), 228–239. https://doi.org/10.1109/TLT.2013.26
Mystakidis, S., Christopoulos, A., & Pellas, N. (2021). A systematic mapping review of augmented reality applications to support STEM learning in higher education. Education and Information Technologies, 27 , 1883–1927. https://doi.org/10.1007/S10639-021-10682-1/FIGURES/10
*Nehm, R. H., Ha, M., & Mayfield, E. (2012). Transforming biology assessment with machine learning: Automated scoring of written evolutionary explanations. Journal of Science Education and Technology, 21 (1), 183–196. https://doi.org/10.1007/s10956-011-9300-9
Ouyang, F., & Jiao, P. (2021). Artificial intelligence in education: The three paradigms. Computers and Education: Artificial Intelligence, 2 , 100020. https://doi.org/10.1016/j.caeai.2021.100020
Ouyang, F., Zheng, L., & Jiao, P. (2022). Artificial intelligence in online higher education: A systematic review of empirical research from 2011 to 2020. Education and Information Technologies . https://doi.org/10.1007/s10639-022-10925-9
*Pereira, F. D., Oliveira, E. H. T., Oliveira, D. B. F., Cristea, A. I., Carvalho, L. S. G., Fonseca, S. C., Toda, A., & Isotani, S. (2020). Using learning analytics in the Amazonas: Understanding students’ behaviour in introductory programming. British Journal of Educational Technology, 51 (4), 955–972. https://doi.org/10.1111/bjet.12953
Pimthong, P., & Williams, J. (2018). Preservice teachers’ understanding of STEM education. Kasetsart Journal of Social Sciences, 41 (2), 1–7. https://doi.org/10.1016/j.kjss.2018.07.017
Rapoport, A. (1986). General system theory: Essential concepts & applications . CRC Press.
*Rodríguez Corral, J. M., Morgado-Estévez, A., Cabrera Molina, D., Pérez-Peña, F., Amaya Rodríguez, C. A., & CivitBalcells, A. (2016). Application of robot programming to the teaching of object-oriented computer languages. International Journal of Engineering Education, 32 (4), 1823–1832.
Roll, I., & Wylie, R. (2016). Evolution and revolution in artificial intelligence in education. International Journal of Artificial Intelligence in Education, 26 (2), 582–599. https://doi.org/10.1007/s40593-016-0110-3
*Saito, T., & Watanobe, Y. (2020). Learning path recommendation system for programming education based on neural networks. International Journal of Distance Education Technologies, 18 (1), 36–64. https://doi.org/10.4018/IJDET.2020010103
*Sapounidis, T., Stamovlasis, D., & Demetriadis, S. (2019). Latent class modeling of children’s preference profiles on tangible and graphical robot programming. IEEE Transactions on Education, 62 (2), 127–133. https://doi.org/10.1109/TE.2018.2876363
Segal, M. (2019). A more human approach to artificial intelligence. Nature, 571 (7766), S18–S18. https://doi.org/10.1038/d41586-019-02213-3
Selwyn, N. (2016). Is technology good for education? Polity Press.
Skinner, B. F. (1953). Science and human behavior . Macmillan.
*Spikol, D., Ruffaldi, E., Dabisias, G., & Cukurova, M. (2018). Supervised machine learning in multimodal learning analytics for estimating success in project-based learning. Journal of Computer Assisted Learning, 34 (4), 366–377. https://doi.org/10.1111/jcal.12263
*Suh, S. C., Anusha Upadhyaya, B. N., & Ashwin Nadig, N. V. (2019). Analyzing personality traits and external factors for stem education awareness using machine learning. International Journal of Advanced Computer Science and Applications, 10 (5), 1–4. https://doi.org/10.14569/ijacsa.2019.0100501
Tang, K. Y., Chang, C. Y., & Hwang, G. J. (2021). Trends in artificial intelligence supported e-learning: A systematic review and co-citation network analysis (1998–2019). Interactive Learning Environments . https://doi.org/10.1080/10494820.2021.1875001
*Tehlan, K., Chakraverty, S., Chakraborty, P., & Khapra, S. (2020). A genetic algorithm-based approach for making pairs and assigning exercises in a programming course. Computer Applications in Engineering Education, 28 (6), 1708–1721. https://doi.org/10.1002/cae.22349
*Thai, K. P., Bang, H. J., & Li, L. (2021). Accelerating early math learning with research-based personalized learning games: A cluster randomized controlled trial. Journal of Research on Educational Effectiveness, 15 (1), 28–51. https://doi.org/10.1080/19345747.2021.1969710
*Troussas, C., Krouska, A., & Sgouropoulou, C. (2021). A novel teaching strategy through adaptive learning activities for computer programming. IEEE Transactions on Education, 64 (2), 103–109. https://doi.org/10.1109/TE.2020.3012744
*Tüfekçi, A., & Köse, U. (2013). Development of an artificial intelligence based software system on teaching computer programming and evaluation of the system. Hacettepe Üniversitesi Eğitim Fakültesi Dergisi, 28 (2), 469–481.
*Verner, I. M., Cuperman, D., Gamer, S., & Polishuk, A. (2020). Exploring affordances of robot manipulators in an introductory engineering course. International Journal of Engineering Education, 36 (5), 1691–1707.
Von Bertalanffy, L. (1950). An outline of general system theory. British Journal for the Philosophy of Science, 1 , 134–165. https://doi.org/10.1093/bjps/I.2.134
Von Bertalanffy, L. (1968). General system theory: Foundations, development, applications . George Braziller.
*Vyas, V. S., Kemp, B., & Reid, S. A. (2021). Zeroing in on the best early-course metrics to identify at-risk students in general chemistry: An adaptive learning pre-assessment vs. traditional diagnostic exam. International Journal of Science Education, 43 (4), 552–569. https://doi.org/10.1080/09500693.2021.1874071
Walker, E., Rummel, N., & Koedinger, K. R. (2014). Adaptive intelligent support to improve peer tutoring in algebra. International Journal of Artificial Intelligence in Education, 24 (1), 33–61. https://doi.org/10.1007/s40593-013-0001-9
*Wang, T., Su, X., Ma, P., Wang, Y., & Wang, K. (2011). Ability-training-oriented automated assessment in introductory programming course. Computers & Education, 56 (1), 220–226. https://doi.org/10.1016/j.compedu.2010.08.003
*Wang, X. (2016). Course-taking patterns of community college students beginning in STEM: Using data mining techniques to reveal viable STEM transfer pathways. Research in Higher Education, 57 (5), 544–569. https://doi.org/10.1007/s11162-015-9397-4
Wohlin, C. (2014). Guidelines for snowballing in systematic literature studies and a replication in software engineering. In C. Wohlin (Ed.), Proceedings of the 18th international conference on evaluation and assessment in software engineering (pp. 1–10). ACM Press. https://doi.org/10.1145/2601248.2601268
*Wu, P., Hwang, G., & Tsai, W. (2013). An expert system-based context-aware ubiquitous learning approach for conducting science learning activities. Journal of Educational Technology & Society, 16 (4), 217–230.
*Xing, W., Pei, B., Li, S., Chen, G., & Xie, C. (2019). Using learning analytics to support students’ engineering design: The angle of prediction. Interactive Learning Environments . https://doi.org/10.1080/10494820.2019.1680391
Xu, W., & Ouyang, F. (2022). A systematic review of AI role in the educational system based on a proposed conceptual framework. Education and Information Technologies, 27 , 4195–4223. https://doi.org/10.1007/s10639-021-10774-y
*Yahya, A. A., & Osman, A. (2019). A data-mining-based approach to informed decision-making in engineering education. Computer Applications in Engineering Education, 27 (6), 1402–1418. https://doi.org/10.1002/cae.22158
*Yang, J., Devore, S., Hewagallage, D., Miller, P., Ryan, Q. X., & Stewart, J. (2020). Using machine learning to identify the most at-risk students in physics classes. Physical Review Physics Education Research, 16 (2), 20130. https://doi.org/10.1103/PhysRevPhysEducRes.16.020130
Yang, J., & Zhang, B. (2019). Artificial intelligence in intelligent tutoring robots: A systematic review and design guidelines. Applied Sciences, 9 (10), 2078. https://doi.org/10.3390/app9102078
*Yannier, N., Hudson, S. E., & Koedinger, K. R. (2020). Active learning is about more than hands-on: A mixed-reality AI system to support STEM education. International Journal of Artificial Intelligence in Education, 30 (1), 74–96. https://doi.org/10.1007/s40593-020-00194-3
*Yu, Y. (2017). Teaching with a dual-channel classroom feedback system in the digital classroom environment. IEEE Transactions on Learning Technologies, 10 (3), 391–402. https://doi.org/10.1109/TLT.2016.2598167
*Zabriskie, C., Yang, J., Devore, S., & Stewart, J. (2019). Using machine learning to predict physics course outcomes. Physical Review Physics Education Research, 15 (2), 20120. https://doi.org/10.1103/PhysRevPhysEducRes.15.020120
*Zampirolli, F. A., BorovinaJosko, J. M., Venero, M. L. F., Kobayashi, G., Fraga, F. J., Goya, D., & Savegnago, H. R. (2021). An experience of automated assessment in a large-scale introduction programming course. Computer Applications in Engineering Education, 29 (5), 1284–1299. https://doi.org/10.1002/cae.22385
*Zapata-Cáceres, M., & Martin-Barroso, E. (2021). Applying game learning analytics to a voluntary video game: Intrinsic motivation, persistence, and rewards in learning to program at an early age. IEEE Access, 9 , 123588–123602. https://doi.org/10.1109/ACCESS.2021.3110475
Zawacki-Richter, O., Marín, V. I., Bond, M., & Gouverneur, F. (2019). Systematic review of research on artificial intelligence applications in higher education—Where are the educators? International Journal of Educational Technology in Higher Education, 16 (39), 1–27. https://doi.org/10.1186/s41239-019-0171-0
*Zhang, Z., Liu, H., Shu, J., Nie, H., & Xiong, N. (2020). On automatic recommender algorithm with regularized convolutional neural network and IR technology in the self-regulated learning process. Infrared Physics and Technology, 105 , 103211. https://doi.org/10.1016/j.infrared.2020.103211
Zheng, R., Jiang, F., & Shen, R. (2020). Intelligent student behavior analysis system for real classrooms. In P. C. Center (Ed.), 2020 IEEE international conference on acoustics, speech and signal processing (pp. 9244–9248). IEEE.
*Zulfiani, Z., Suwarna, I. P., & Miranto, S. (2018). Science education adaptive learning system as a computer-based science learning with learning style variations. Journal of Baltic Science Education, 17 (4), 711–727. https://doi.org/10.33225/jbse/18.17.711
Zupic, I., & Čater, T. (2015). Bibliometric methods in management and organization. Organizational Research Methods, 18 (3), 429–472. https://doi.org/10.1177/1094428114562629
Download references
Acknowledgements
The authors would like to thank Luyi Zheng for her help on preliminary data analysis.
This work was supported by National Natural Science Foundation of China, No. 62177041.
Author information
Authors and affiliations.
College of Education, Zhejiang University, #866, Yuhangtang Rd., Hangzhou, 310058, Zhejiang, China
Weiqi Xu & Fan Ouyang
You can also search for this author in PubMed Google Scholar
Contributions
WX conducted data collection and analysis as well as writing of the first draft of this manuscript. FO designed the research, designed and facilitated data analysis and revised the manuscript. Both authors read and approved the final manuscript.
Corresponding author
Correspondence to Fan Ouyang .
Ethics declarations
Competing interests.
The authors declare that they have no competing interests.
Additional information
Publisher's note.
Springer Nature remains neutral with regard to jurisdictional claims in published maps and institutional affiliations.
Rights and permissions
Open Access This article is licensed under a Creative Commons Attribution 4.0 International License, which permits use, sharing, adaptation, distribution and reproduction in any medium or format, as long as you give appropriate credit to the original author(s) and the source, provide a link to the Creative Commons licence, and indicate if changes were made. The images or other third party material in this article are included in the article's Creative Commons licence, unless indicated otherwise in a credit line to the material. If material is not included in the article's Creative Commons licence and your intended use is not permitted by statutory regulation or exceeds the permitted use, you will need to obtain permission directly from the copyright holder. To view a copy of this licence, visit http://creativecommons.org/licenses/by/4.0/ .
Reprints and permissions
About this article
Cite this article.
Xu, W., Ouyang, F. The application of AI technologies in STEM education: a systematic review from 2011 to 2021. IJ STEM Ed 9 , 59 (2022). https://doi.org/10.1186/s40594-022-00377-5
Download citation
Received : 11 June 2022
Accepted : 09 September 2022
Published : 19 September 2022
DOI : https://doi.org/10.1186/s40594-022-00377-5
Share this article
Anyone you share the following link with will be able to read this content:
Sorry, a shareable link is not currently available for this article.
Provided by the Springer Nature SharedIt content-sharing initiative
- Artificial intelligence
- Artificial intelligence in education
- STEM education
- General system theory
- Educational system
- Research article
- Open access
- Published: 24 April 2023
Artificial intelligence in higher education: the state of the field
- Helen Crompton ORCID: orcid.org/0000-0002-1775-8219 1 , 3 &
- Diane Burke 2
International Journal of Educational Technology in Higher Education volume 20 , Article number: 22 ( 2023 ) Cite this article
95k Accesses
93 Citations
64 Altmetric
Metrics details
This systematic review provides unique findings with an up-to-date examination of artificial intelligence (AI) in higher education (HE) from 2016 to 2022. Using PRISMA principles and protocol, 138 articles were identified for a full examination. Using a priori, and grounded coding, the data from the 138 articles were extracted, analyzed, and coded. The findings of this study show that in 2021 and 2022, publications rose nearly two to three times the number of previous years. With this rapid rise in the number of AIEd HE publications, new trends have emerged. The findings show that research was conducted in six of the seven continents of the world. The trend has shifted from the US to China leading in the number of publications. Another new trend is in the researcher affiliation as prior studies showed a lack of researchers from departments of education. This has now changed to be the most dominant department. Undergraduate students were the most studied students at 72%. Similar to the findings of other studies, language learning was the most common subject domain. This included writing, reading, and vocabulary acquisition. In examination of who the AIEd was intended for 72% of the studies focused on students, 17% instructors, and 11% managers. In answering the overarching question of how AIEd was used in HE, grounded coding was used. Five usage codes emerged from the data: (1) Assessment/Evaluation, (2) Predicting, (3) AI Assistant, (4) Intelligent Tutoring System (ITS), and (5) Managing Student Learning. This systematic review revealed gaps in the literature to be used as a springboard for future researchers, including new tools, such as Chat GPT.
A systematic review examining AIEd in higher education (HE) up to the end of 2022.
Unique findings in the switch from US to China in the most studies published.
A two to threefold increase in studies published in 2021 and 2022 to prior years.
AIEd was used for: Assessment/Evaluation, Predicting, AI Assistant, Intelligent Tutoring System, and Managing Student Learning.
Introduction
The use of artificial intelligence (AI) in higher education (HE) has risen quickly in the last 5 years (Chu et al., 2022 ), with a concomitant proliferation of new AI tools available. Scholars (viz., Chen et al., 2020 ; Crompton et al., 2020 , 2021 ) report on the affordances of AI to both instructors and students in HE. These benefits include the use of AI in HE to adapt instruction to the needs of different types of learners (Verdú et al., 2017 ), in providing customized prompt feedback (Dever et al., 2020 ), in developing assessments (Baykasoğlu et al., 2018 ), and predict academic success (Çağataylı & Çelebi, 2022 ). These studies help to inform educators about how artificial intelligence in education (AIEd) can be used in higher education.
Nonetheless, a gap has been highlighted by scholars (viz., Hrastinski et al., 2019 ; Zawacki-Richter et al., 2019 ) regarding an understanding of the collective affordances provided through the use of AI in HE. Therefore, the purpose of this study is to examine extant research from 2016 to 2022 to provide an up-to-date systematic review of how AI is being used in the HE context.
Artificial intelligence has become pervasive in the lives of twenty-first century citizens and is being proclaimed as a tool that can be used to enhance and advance all sectors of our lives (Górriz et al., 2020 ). The application of AI has attracted great interest in HE which is highly influenced by the development of information and communication technologies (Alajmi et al., 2020 ). AI is a tool used across subject disciplines, including language education (Liang et al., 2021 ), engineering education (Shukla et al., 2019 ), mathematics education (Hwang & Tu, 2021 ) and medical education (Winkler-Schwartz et al., 2019 ),
Artificial intelligence
The term artificial intelligence is not new. It was coined in 1956 by McCarthy (Cristianini, 2016 ) who followed up on the work of Turing (e.g., Turing, 1937 , 1950 ). Turing described the existence of intelligent reasoning and thinking that could go into intelligent machines. The definition of AI has grown and changed since 1956, as there has been significant advancements in AI capabilities. A current definition of AI is “computing systems that are able to engage in human-like processes such as learning, adapting, synthesizing, self-correction and the use of data for complex processing tasks” (Popenici et al., 2017 , p. 2). The interdisciplinary interest from scholars from linguistics, psychology, education, and neuroscience who connect AI to nomenclature, perceptions and knowledge in their own disciplines could create a challenge when defining AI. This has created the need to create categories of AI within specific disciplinary areas. This paper focuses on the category of AI in Education (AIEd) and how AI is specifically used in higher educational contexts.
As the field of AIEd is growing and changing rapidly, there is a need to increase the academic understanding of AIEd. Scholars (viz., Hrastinski et al., 2019 ; Zawacki-Richter et al., 2019 ) have drawn attention to the need to increase the understanding of the power of AIEd in educational contexts. The following section provides a summary of the previous research regarding AIEd.
Extant systematic reviews
This growing interest in AIEd has led scholars to investigate the research on the use of artificial intelligence in education. Some scholars have conducted systematic reviews to focus on a specific subject domain. For example, Liang et. al. ( 2021 ) conducted a systematic review and bibliographic analysis the roles and research foci of AI in language education. Shukla et. al. ( 2019 ) focused their longitudinal bibliometric analysis on 30 years of using AI in Engineering. Hwang and Tu ( 2021 ) conducted a bibliometric mapping analysis on the roles and trends in the use of AI in mathematics education, and Winkler-Schwartz et. al. ( 2019 ) specifically examined the use of AI in medical education in looking for best practices in the use of machine learning to assess surgical expertise. These studies provide a specific focus on the use of AIEd in HE but do not provide an understanding of AI across HE.
On a broader view of AIEd in HE, Ouyang et. al. ( 2022 ) conducted a systematic review of AIEd in online higher education and investigated the literature regarding the use of AI from 2011 to 2020. The findings show that performance prediction, resource recommendation, automatic assessment, and improvement of learning experiences are the four main functions of AI applications in online higher education. Salas-Pilco and Yang ( 2022 ) focused on AI applications in Latin American higher education. The results revealed that the main AI applications in higher education in Latin America are: (1) predictive modeling, (2) intelligent analytics, (3) assistive technology, (4) automatic content analysis, and (5) image analytics. These studies provide valuable information for the online and Latin American context but not an overarching examination of AIEd in HE.
Studies have been conducted to examine HE. Hinojo-Lucena et. al. ( 2019 ) conducted a bibliometric study on the impact of AIEd in HE. They analyzed the scientific production of AIEd HE publications indexed in Web of Science and Scopus databases from 2007 to 2017. This study revealed that most of the published document types were proceedings papers. The United States had the highest number of publications, and the most cited articles were about implementing virtual tutoring to improve learning. Chu et. al. ( 2022 ) reviewed the top 50 most cited articles on AI in HE from 1996 to 2020, revealing that predictions of students’ learning status were most frequently discussed. AI technology was most frequently applied in engineering courses, and AI technologies most often had a role in profiling and prediction. Finally, Zawacki-Richter et. al. ( 2019 ) analyzed AIEd in HE from 2007 to 2018 to reveal four primary uses of AIEd: (1) profiling and prediction, (2) assessment and evaluation, (3) adaptive systems and personalization, and (4) intelligent tutoring systems. There do not appear to be any studies examining the last 2 years of AIEd in HE, and these authors describe the rapid speed of both AI development and the use of AIEd in HE and call for further research in this area.
Purpose of the study
The purpose of this study is in response to the appeal from scholars (viz., Chu et al., 2022 ; Hinojo-Lucena et al., 2019 ; Zawacki-Richter et al., 2019 ) to research to investigate the benefits and challenges of AIEd within HE settings. As the academic knowledge of AIEd HE finished with studies examining up to 2020, this study provides the most up-to-date analysis examining research through to the end of 2022.
The overarching question for this study is: what are the trends in HE research regarding the use of AIEd? The first two questions provide contextual information, such as where the studies occurred and the disciplines AI was used in. These contextual details are important for presenting the main findings of the third question of how AI is being used in HE.
In what geographical location was the AIEd research conducted, and how has the trend in the number of publications evolved across the years?
What departments were the first authors affiliated with, and what were the academic levels and subject domains in which AIEd research was being conducted?
Who are the intended users of the AI technologies and what are the applications of AI in higher education?
A PRISMA systematic review methodology was used to answer three questions guiding this study. PRISMA principles (Page et al., 2021 ) were used throughout the study. The PRISMA extension Preferred Reporting Items for Systematic Reviews and Meta-Analysis for Protocols (PRISMA-P; Moher et al., 2015 ) were utilized in this study to provide an a priori roadmap to conduct a rigorous systematic review. Furthermore, the Preferred Reporting Items for Systematic Reviews and Meta-Analysis (PRISMA principles; Page et al., 2021 ) were used to search, identify, and select articles to be included in the research were used for searching, identifying, and selecting articles, then in how to read, extract, and manage the secondary data gathered from those studies (Moher et al., 2015 , PRISMA Statement, 2021 ). This systematic review approach supports an unbiased synthesis of the data in an impartial way (Hemingway & Brereton, 2009 ). Within the systematic review methodology, extracted data were aggregated and presented as whole numbers and percentages. A qualitative deductive and inductive coding methodology was also used to analyze extant data and generate new theories on the use of AI in HE (Gough et al., 2017 ).
The research begins with the search for the research articles to be included in the study. Based on the research question, the study parameters are defined including the search years, quality and types of publications to be included. Next, databases and journals are selected. A Boolean search is created and used for the search of those databases and journals. Once a set of publications are located from those searches, they are then examined against an inclusion and exclusion criteria to determine which studies will be included in the final study. The relevant data to match the research questions is then extracted from the final set of studies and coded. This method section is organized to describe each of these methods with full details to ensure transparency.
Search strategy
Only peer-reviewed journal articles were selected for examination in this systematic review. This ensured a level of confidence in the quality of the studies selected (Gough et al., 2017 ). The search parameters narrowed the search focus to include studies published in 2016 to 2022. This timeframe was selected to ensure the research was up to date, which is especially important with the rapid change in technology and AIEd.
The data retrieval protocol employed an electronic and a hand search. The electronic search included educational databases within EBSCOhost. Then an additional electronic search was conducted of Wiley Online Library, JSTOR, Science Direct, and Web of Science. Within each of these databases a full text search was conducted. Aligned to the research topic and questions, the Boolean search included terms related to AI, higher education, and learning. The Boolean search is listed in Table 1 . In the initial test search, the terms “machine learning” OR “intelligent support” OR “intelligent virtual reality” OR “chatbot” OR “automated tutor” OR “intelligent agent” OR “expert system” OR “neural network” OR “natural language processing” were used. These were removed as they were subcategories of terms found in Part 1 of the search. Furthermore, inclusion of these specific AI terms resulted in a large number of computer science courses that were focused on learning about AI and not the use of AI in learning.
Part 2 of the search ensured that articles involved formal university education. The terms higher education and tertiary were both used to recognize the different terms used in different countries. The final Boolean search was “Artificial intelligence” OR AI OR “smart technologies” OR “intelligent technologies” AND “higher education” OR tertiary OR graduate OR undergraduate. Scholars (viz., Ouyang et al., 2022 ) who conducted a systematic review on AIEd in HE up to 2020 noted that they missed relevant articles from their study, and other relevant journals should intentionally be examined. Therefore, a hand search was also conducted to include an examination of other journals relevant to AIEd that may not be included in the databases. This is important as the field of AIEd is still relatively new, and journals focused on this field may not yet be indexed in databases. The hand search included: The International Journal of Learning Analytics and Artificial Intelligence in Education, the International Journal of Artificial Intelligence in Education, and Computers & Education: Artificial Intelligence.
Electronic and hand searches resulted in 371 articles for possible inclusion. The search parameters within the electronic database search narrowed the search to articles published from 2016 to 2022, per-reviewed journal articles, and duplicates. Further screening was conducted manually, as each of the 138 articles were reviewed in full by two researchers to examine a match against the inclusion and exclusion criteria found in Table 2 .
The inter-rater reliability was calculated by percentage agreement (Belur et al., 2018 ). The researchers reached a 95% agreement for the coding. Further discussion of misaligned articles resulted in a 100% agreement. This screening process against inclusion and exclusion criteria resulted in the exclusion of 237 articles. This included the duplicates and those removed as part of the inclusion and exclusion criteria, see Fig. 1 . Leaving 138 articles for inclusion in this systematic review.
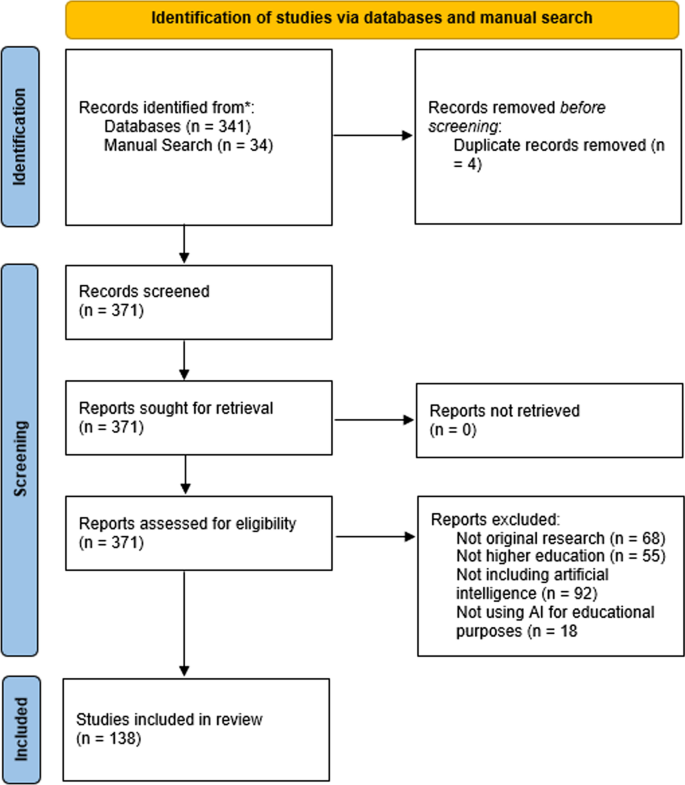
(From: Page et al., 2021 )
PRISMA flow chart of article identification and screening
The 138 articles were then coded to answer each of the research questions using deductive and inductive coding methods. Deductive coding involves examining data using a priori codes. A priori are pre-determined criteria and this process was used to code the countries, years, author affiliations, academic levels, and domains in the respective groups. Author affiliations were coded using the academic department of the first author of the study. First authors were chosen as that person is the primary researcher of the study and this follows past research practice (e.g., Zawacki-Richter et al., 2019 ). Who the AI was intended for was also coded using the a priori codes of Student, Instructor, Manager or Others. The Manager code was used for those who are involved in organizational tasks, e.g., tracking enrollment. Others was used for those not fitting the other three categories.
Inductive coding was used for the overarching question of this study in examining how the AI was being used in HE. Researchers of extant systematic reviews on AIEd in HE (viz., Chu et al., 2022 ; Zawacki-Richter et al., 2019 ) often used an a priori framework as researchers matched the use of AI to pre-existing frameworks. A grounded coding methodology (Strauss & Corbin, 1995 ) was selected for this study to allow findings of the trends on AIEd in HE to emerge from the data. This is important as it allows a direct understanding of how AI is being used rather than how researchers may think it is being used and fitting the data to pre-existing ideas.
Grounded coding process involved extracting how the AI was being used in HE from the articles. “In vivo” (Saldana, 2015 ) coding was also used alongside grounded coding. In vivo codes are when codes use language directly from the article to capture the primary authors’ language and ensure consistency with their findings. The grounded coding design used a constant comparative method. Researchers identified important text from articles related to the use of AI, and through an iterative process, initial codes led to axial codes with a constant comparison of uses of AI with uses of AI, then of uses of AI with codes, and codes with codes. Codes were deemed theoretically saturated when the majority of the data fit with one of the codes. For both the a priori and the grounded coding, two researchers coded and reached an inter-rater percentage agreement of 96%. After discussing misaligned articles, a 100% agreement was achieved.
Findings and discussion
The findings and discussion section are organized by the three questions guiding this study. The first two questions provide contextual information on the AIEd research, and the final question provides a rigorous investigation into how AI is being used in HE.
RQ1. In what geographical location was the AIEd research conducted, and how has the trend in the number of publications evolved across the years?
The 138 studies took place across 31 countries in six of seven continents of the world. Nonetheless, that distribution was not equal across continents. Asia had the largest number of AIEd studies in HE at 41%. Of the seven countries represented in Asia, 42 of the 58 studies were conducted in Taiwan and China. Europe, at 30%, was the second largest continent and had 15 countries ranging from one to eight studies a piece. North America, at 21% of the studies was the continent with the third largest number of studies, with the USA producing 21 of the 29 studies in that continent. The 21 studies from the USA places it second behind China. Only 1% of studies were conducted in South America and 2% in Africa. See Fig. 2 for a visual representation of study distribution across countries. Those continents with high numbers of studies are from high income countries and those with low numbers have a paucity of publications in low-income countries.
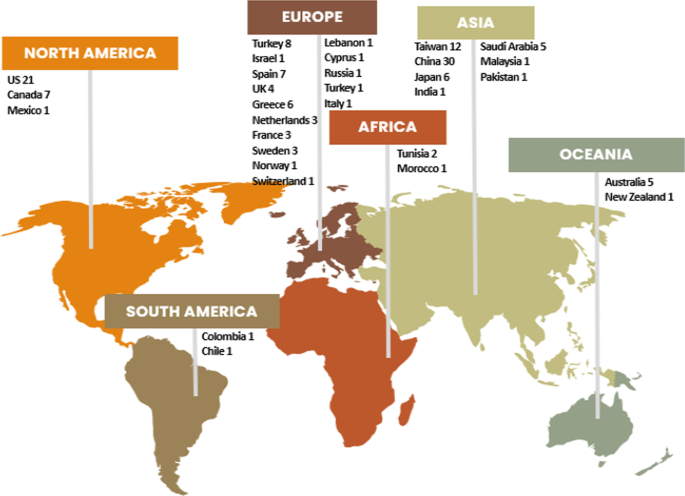
Geographical distribution of the AIEd HE studies
Data from Zawacki-Richter et. al.’s ( 2019 ) 2007–2018 systematic review examining countries found that the USA conducted the most studies across the globe at 43 out of 146, and China had the second largest at eleven of the 146 papers. Researchers have noted a rapid trend in Chinese researchers publishing more papers on AI and securing more patents than their US counterparts in a field that was originally led by the US (viz., Li et al., 2021 ). The data from this study corroborate this trend in China leading in the number of AIEd publications.
With the accelerated use of AI in society, gathering data to examine the use of AIEd in HE is useful in providing the scholarly community with specific information on that growth and if it is as prolific as anticipated by scholars (e.g., Chu et al., 2022 ). The analysis of data of the 138 studies shows that the trend towards the use of AIEd in HE has greatly increased. There is a drop in 2019, but then a great rise in 2021 and 2022; see Fig. 3 .
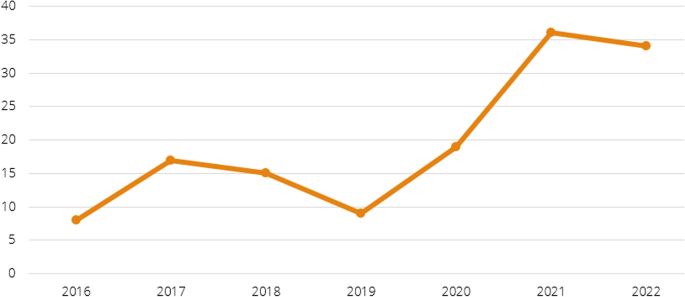
Chronological trend in AIEd in HE
Data on the rise in AIEd in HE is similar to the findings of Chu et. al. ( 2022 ) who noted an increase from 1996 to 2010 and 2011–2020. Nonetheless Chu’s parameters are across decades, and the rise is to be anticipated with a relatively new technology across a longitudinal review. Data from this study show a dramatic rise since 2020 with a 150% increase from the prior 2 years 2020–2019. The rise in 2021 and 2022 in HE could have been caused by the vast increase in HE faculty having to teach with technology during the pandemic lockdown. Faculty worldwide were using technologies, including AI, to explore how they could continue teaching and learning that was often face-to-face prior to lockdown. The disadvantage of this rapid adoption of technology is that there was little time to explore the possibilities of AI to transform learning, and AI may have been used to replicate past teaching practices, without considering new strategies previously inconceivable with the affordances of AI.
However, in a further examination of the research from 2021 to 2022, it appears that there are new strategies being considered. For example, Liu et. al.’s, 2022 study used AIEd to provide information on students’ interactions in an online environment and examine their cognitive effort. In Yao’s study in 2022, he examined the use of AI to determine student emotions while learning.
RQ2. What departments were the first authors affiliated with, and what were the academic levels and subject domains in which AIEd research was being conducted?
Department affiliations
Data from the AIEd HE studies show that of the first authors were most frequently from colleges of education (28%), followed by computer science (20%). Figure 4 presents the 15 academic affiliations of the authors found in the studies. The wide variety of affiliations demonstrate the variety of ways AI can be used in various educational disciplines, and how faculty in diverse areas, including tourism, music, and public affairs were interested in how AI can be used for educational purposes.
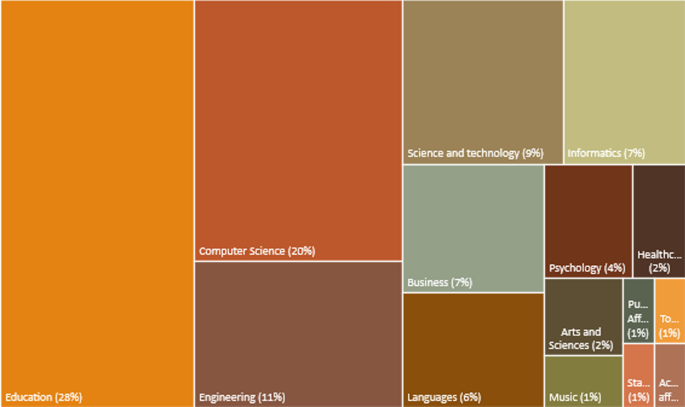
Research affiliations
In an extant AIED HE systematic review, Zawacki-Richter et. al.’s ( 2019 ) named their study Systematic review of research on artificial intelligence applications in higher education—where are the educators? In this study, the authors were keen to highlight that of the AIEd studies in HE, only six percent were written by researchers directly connected to the field of education, (i.e., from a college of education). The researchers found a great lack in pedagogical and ethical implications of implementing AI in HE and that there was a need for more educational perspectives on AI developments from educators conducting this work. It appears from our data that educators are now showing greater interest in leading these research endeavors, with the highest affiliated group belonging to education. This may again be due to the pandemic and those in the field of education needing to support faculty in other disciplines, and/or that they themselves needed to explore technologies for their own teaching during the lockdown. This may also be due to uptake in professors in education becoming familiar with AI tools also driven by a societal increased attention. As the focus of much research by education faculty is on teaching and learning, they are in an important position to be able to share their research with faculty in other disciplines regarding the potential affordances of AIEd.
Academic levels
The a priori coding of academic levels show that the majority of studies involved undergraduate students with 99 of the 138 (72%) focused on these students. This was in comparison to the 12 of 138 (9%) for graduate students. Some of the studies used AI for both academic levels: see Fig. 5
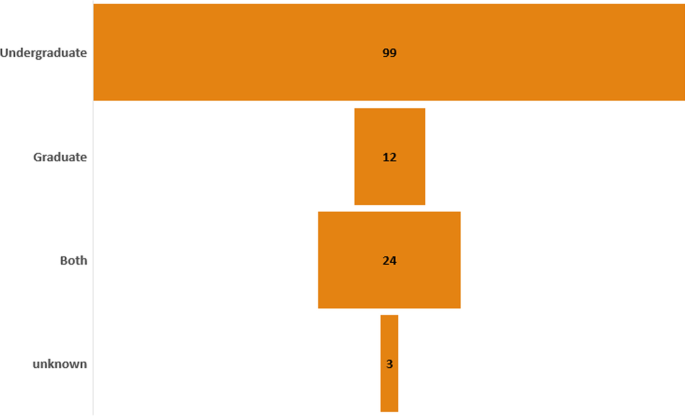
Academic level distribution by number of articles
This high percentage of studies focused on the undergraduate population was congruent with an earlier AIED HE systematic review (viz., Zawacki-Richter et al., 2019 ) who also reported student academic levels. This focus on undergraduate students may be due to the variety of affordances offered by AIEd, such as predictive analytics on dropouts and academic performance. These uses of AI may be less required for graduate students who already have a record of performance from their undergraduate years. Another reason for this demographic focus can also be convenience sampling, as researchers in HE typically has a much larger and accessible undergraduate population than graduates. This disparity between undergraduates and graduate populations is a concern, as AIEd has the potential to be valuable in both settings.
Subject domains
The studies were coded into 14 areas in HE; with 13 in a subject domain and one category of AIEd used in HE management of students; See Fig. 6 . There is not a wide difference in the percentages of top subject domains, with language learning at 17%, computer science at 16%, and engineering at 12%. The management of students category appeared third on the list at 14%. Prior studies have also found AIEd often used for language learning (viz., Crompton et al., 2021 ; Zawacki-Richter et al., 2019 ). These results are different, however, from Chu et. al.’s ( 2022 ) findings that show engineering dramatically leading with 20 of the 50 studies, with other subjects, such as language learning, appearing once or twice. This study appears to be an outlier that while the searches were conducted in similar databases, the studies only included 50 studies from 1996 to 2020.
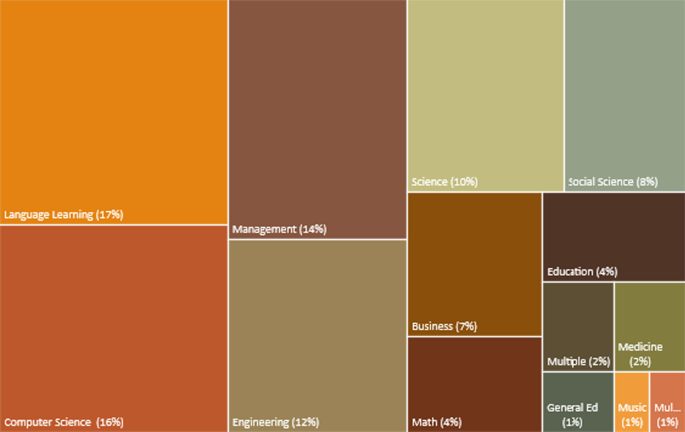
Subject domains of AIEd in HE
Previous scholars primarily focusing on language learning using AI for writing, reading, and vocabulary acquisition used the affordances of natural language processing and intelligent tutoring systems (e.g., Liang et al., 2021 ). This is similar to the findings in studies with AI used for automated feedback of writing in a foreign language (Ayse et al., 2022 ), and AI translation support (Al-Tuwayrish, 2016 ). The large use of AI for managerial activities in this systematic review focused on making predictions (12 studies) and then admissions (three studies). This is positive to see this use of AI to look across multiple databases to see trends emerging from data that may not have been anticipated and cross referenced before (Crompton et al., 2022 ). For example, to examine dropouts, researchers may consider examining class attendance, and may not examine other factors that appear unrelated. AI analysis can examine all factors and may find that dropping out is due to factors beyond class attendance.
RQ3. Who are the intended users of the AI technologies and what are the applications of AI in higher education?
Intended user of AI
Of the 138 articles, the a priori coding shows that 72% of the studies focused on Students, followed by a focus on Instructors at 17%, and Managers at 11%, see Fig. 7 . The studies provided examples of AI being used to provide support to students, such as access to learning materials for inclusive learning (Gupta & Chen, 2022 ), provide immediate answers to student questions, self-testing opportunities (Yao, 2022 ), and instant personalized feedback (Mousavi et al., 2020 ).
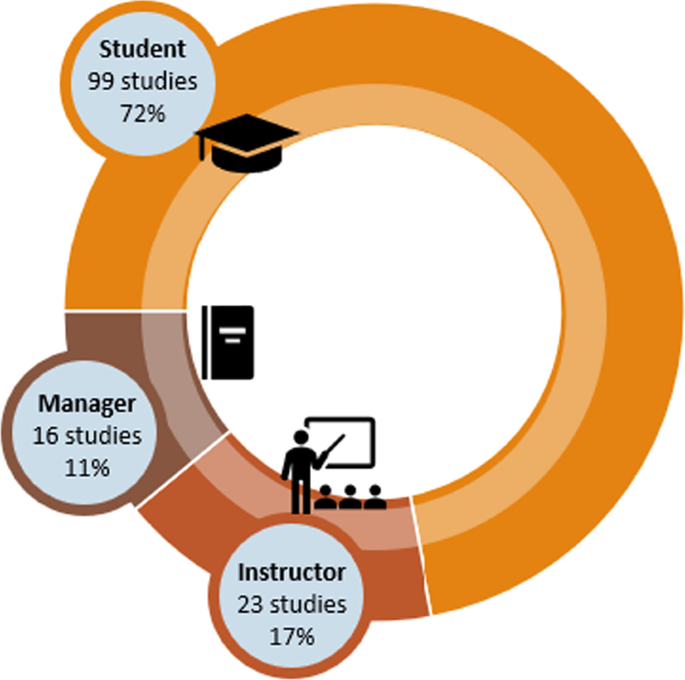
Intended user
The data revealed a large emphasis on students in the use of AIEd in HE. This user focus is different from a recent systematic review on AIEd in K-12 that found that AIEd studies in K-12 settings prioritized teachers (Crompton et al., 2022 ). This may appear that HE uses AI to focus more on students than in K-12. However, this large number of student studies in HE may be due to the student population being more easily accessibility to HE researchers who may study their own students. The ethical review process is also typically much shorter in HE than in K-12. Therefore, the data on the intended focus should be reviewed while keeping in mind these other explanations. It was interesting that Managers were the lowest focus in K-12 and also in this study in HE. AI has great potential to collect, cross reference and examine data across large datasets that can allow data to be used for actionable insight. More focus on the use of AI by managers would tap into this potential.
How is AI used in HE
Using grounded coding, the use of AIEd from each of the 138 articles was examined and six major codes emerged from the data. These codes provide insight into how AI was used in HE. The five codes are: (1) Assessment/Evaluation, (2) Predicting, (3) AI Assistant, (4) Intelligent Tutoring System (ITS), and (5) Managing Student Learning. For each of these codes there are also axial codes, which are secondary codes as subcategories from the main category. Each code is delineated below with a figure of the codes with further descriptive information and examples.
Assessment/evaluation
Assessment and Evaluation was the most common use of AIEd in HE. Within this code there were six axial codes broken down into further codes; see Fig. 8 . Automatic assessment was most common, seen in 26 of the studies. It was interesting to see that this involved assessment of academic achievement, but also other factors, such as affect.
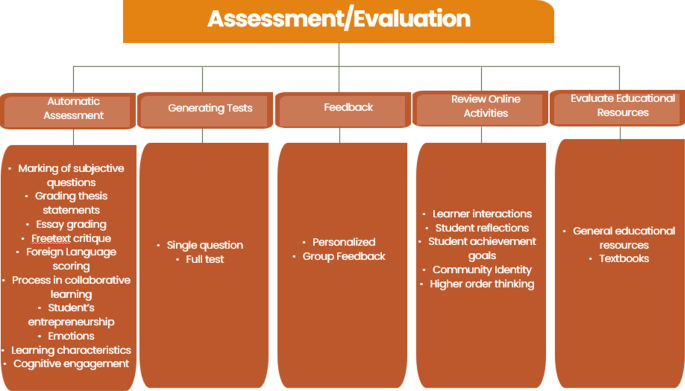
Codes and axial codes for assessment and evaluation
Automatic assessment was used to support a variety of learners in HE. As well as reducing the time it takes for instructors to grade (Rutner & Scott, 2022 ), automatic grading showed positive use for a variety of students with diverse needs. For example, Zhang and Xu ( 2022 ) used automatic assessment to improve academic writing skills of Uyghur ethnic minority students living in China. Writing has a variety of cultural nuances and in this study the students were shown to engage with the automatic assessment system behaviorally, cognitively, and affectively. This allowed the students to engage in self-regulated learning while improving their writing.
Feedback was a description often used in the studies, as students were given text and/or images as feedback as a formative evaluation. Mousavi et. al. ( 2020 ) developed a system to provide first year biology students with an automated personalized feedback system tailored to the students’ specific demographics, attributes, and academic status. With the unique feature of AIEd being able to analyze multiple data sets involving a variety of different students, AI was used to assess and provide feedback on students’ group work (viz., Ouatik et al., 2021 ).
AI also supports instructors in generating questions and creating multiple question tests (Yang et al., 2021 ). For example, (Lu et al., 2021 ) used natural language processing to create a system that automatically created tests. Following a Turing type test, researchers found that AI technologies can generate highly realistic short-answer questions. The ability for AI to develop multiple questions is a highly valuable affordance as tests can take a great deal of time to make. However, it would be important for instructors to always confirm questions provided by the AI to ensure they are correct and that they match the learning objectives for the class, especially in high value summative assessments.
The axial code within assessment and evaluation revealed that AI was used to review activities in the online space. This included evaluating student’s reflections, achievement goals, community identity, and higher order thinking (viz., Huang et al., 2021 ). Three studies used AIEd to evaluate educational materials. This included general resources and textbooks (viz., Koć‑Januchta et al., 2022 ). It is interesting to see the use of AI for the assessment of educational products, rather than educational artifacts developed by students. While this process may be very similar in nature, this shows researchers thinking beyond the traditional use of AI for assessment to provide other affordances.
Predicting was a common use of AIEd in HE with 21 studies focused specifically on the use of AI for forecasting trends in data. Ten axial codes emerged on the way AI was used to predict different topics, with nine focused on predictions regarding students and the other on predicting the future of higher education. See Fig. 9 .
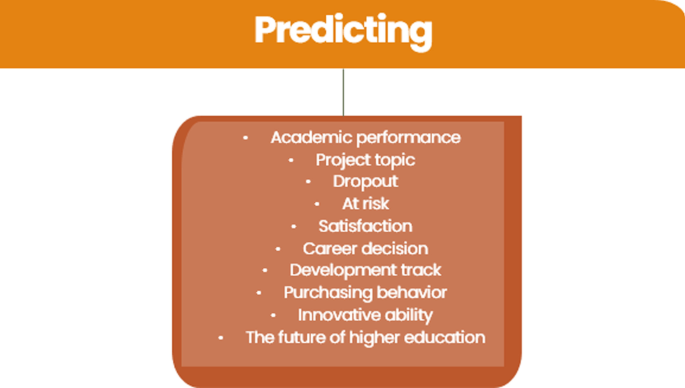
Predicting axial codes
Extant systematic reviews on HE highlighted the use of AIEd for prediction (viz., Chu et al., 2022 ; Hinojo-Lucena et al., 2019 ; Ouyang et al., 2022 ; Zawacki-Richter et al., 2019 ). Ten of the articles in this study used AI for predicting academic performance. Many of the axial codes were often overlapping, such as predicting at risk students, and predicting dropouts; however, each provided distinct affordances. An example of this is the study by Qian et. al. ( 2021 ). These researchers examined students taking a MOOC course. MOOCs can be challenging environments to determine information on individual students with the vast number of students taking the course (Krause & Lowe, 2014 ). However, Qian et al., used AIEd to predict students’ future grades by inputting 17 different learning features, including past grades, into an artificial neural network. The findings were able to predict students’ grades and highlight students at risk of dropping out of the course.
In a systematic review on AIEd within the K-12 context (viz., Crompton et al., 2022 ), prediction was less pronounced in the findings. In the K-12 setting, there was a brief mention of the use of AI in predicting student academic performance. One of the studies mentioned students at risk of dropping out, but this was immediately followed by questions about privacy concerns and describing this as “sensitive”. The use of prediction from the data in this HE systematic review cover a wide range of AI predictive affordances. students Sensitivity is still important in a HE setting, but it is positive to see the valuable insight it provides that can be used to avoid students failing in their goals.
AI assistant
The studies evaluated in this review indicated that the AI Assistant used to support learners had a variety of different names. This code included nomenclature such as, virtual assistant, virtual agent, intelligent agent, intelligent tutor, and intelligent helper. Crompton et. al. ( 2022 ), described the difference in the terms to delineate the way that the AI appeared to the user. For example, if there was an anthropomorphic presence to the AI, such as an avatar, or if the AI appeared to support via other means, such as text prompt. The findings of this systematic review align to Crompton et. al.’s ( 2022 ) descriptive differences of the AI Assistant. Furthermore, this code included studies that provide assistance to students, but may not have specifically used the word assistance. These include the use of chatbots for student outreach, answering questions, and providing other assistance. See Fig. 10 for the axial codes for AI Assistant.
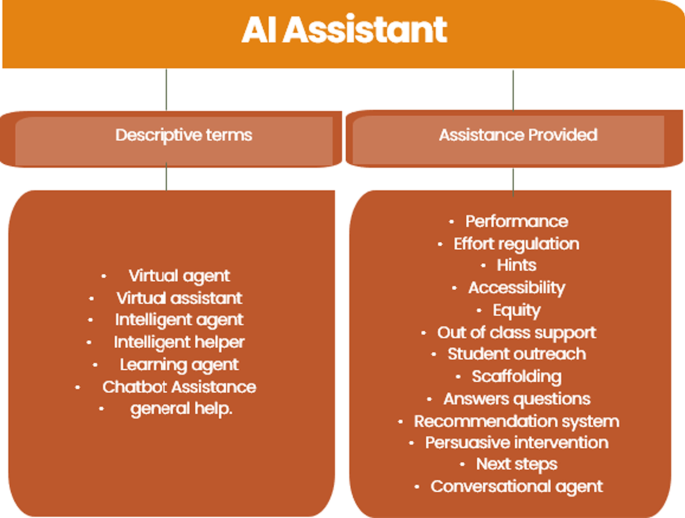
AI assistant axial codes
Many of these assistants offered multiple supports to students, such as Alex , the AI described as a virtual change agent in Kim and Bennekin’s ( 2016 ) study. Alex interacted with students in a college mathematics course by asking diagnostic questions and gave support depending on student needs. Alex’s support was organized into four stages: (1) goal initiation (“Want it”), (2) goal formation (“Plan for it”), (3) action control (“Do it”), and (4) emotion control (“Finish it”). Alex provided responses depending on which of these four areas students needed help. These messages supported students with the aim of encouraging persistence in pursuing their studies and degree programs and improving performance.
The role of AI in providing assistance connects back to the seminal work of Vygotsky ( 1978 ) and the Zone of Proximal Development (ZPD). ZPD highlights the degree to which students can rapidly develop when assisted. Vygotsky described this assistance often in the form of a person. However, with technological advancements, the use of AI assistants in these studies are providing that support for students. The affordances of AI can also ensure that the support is timely without waiting for a person to be available. Also, assistance can consider aspects on students’ academic ability, preferences, and best strategies for supporting. These features were evident in Kim and Bennekin’s ( 2016 ) study using Alex.
Intelligent tutoring system
The use of Intelligent Tutoring Systems (ITS) was revealed in the grounded coding. ITS systems are adaptive instructional systems that involve the use of AI techniques and educational methods. An ITS system customizes educational activities and strategies based on student’s characteristics and needs (Mousavinasab et al., 2021 ). While ITS may be an anticipated finding in AIED HE systematic reviews, it was interesting that extant reviews similar to this study did not always describe their use in HE. For example, Ouyang et. al. ( 2022 ), included “intelligent tutoring system” in search terms describing it as a common technique, yet ITS was not mentioned again in the paper. Zawacki-Richter et. al. ( 2019 ) on the other hand noted that ITS was in the four overarching findings of the use of AIEd in HE. Chu et. al. ( 2022 ) then used Zawacki-Richter’s four uses of AIEd for their recent systematic review.
In this systematic review, 18 studies specifically mentioned that they were using an ITS. The ITS code did not necessitate axial codes as they were performing the same type of function in HE, namely, in providing adaptive instruction to the students. For example, de Chiusole et. al. ( 2020 ) developed Stat-Knowlab, an ITS that provides the level of competence and best learning path for each student. Thus Stat-Knowlab personalizes students’ learning and provides only educational activities that the student is ready to learn. This ITS is able to monitor the evolution of the learning process as the student interacts with the system. In another study, Khalfallah and Slama ( 2018 ) built an ITS called LabTutor for engineering students. LabTutor served as an experienced instructor in enabling students to access and perform experiments on laboratory equipment while adapting to the profile of each student.
The student population in university classes can go into the hundreds and with the advent of MOOCS, class sizes can even go into the thousands. Even in small classes of 20 students, the instructor cannot physically provide immediate unique personalize questions to each student. Instructors need time to read and check answers and then take further time to provide feedback before determining what the next question should be. Working with the instructor, AIEd can provide that immediate instruction, guidance, feedback, and following questioning without delay or becoming tired. This appears to be an effective use of AIEd, especially within the HE context.
Managing student learning
Another code that emerged in the grounded coding was focused on the use of AI for managing student learning. AI is accessed to manage student learning by the administrator or instructor to provide information, organization, and data analysis. The axial codes reveal the trends in the use of AI in managing student learning; see Fig. 11 .
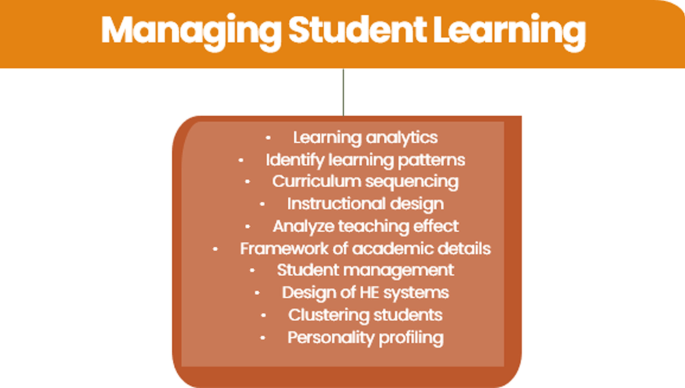
Learning analytics was an a priori term often found in studies which describes “the measurement, collection, analysis and reporting of data about learners and their contexts, for purposes of understanding and optimizing learning and the environments in which it occurs” (Long & Siemens, 2011 , p. 34). The studies investigated in this systematic review were across grades and subject areas and provided administrators and instructors different types of information to guide their work. One of those studies was conducted by Mavrikis et. al. ( 2019 ) who described learning analytics as teacher assistance tools. In their study, learning analytics were used in an exploratory learning environment with targeted visualizations supporting classroom orchestration. These visualizations, displayed as screenshots in the study, provided information such as the interactions between the students, goals achievements etc. These appear similar to infographics that are brightly colored and draw the eye quickly to pertinent information. AI is also used for other tasks, such as organizing the sequence of curriculum in pacing guides for future groups of students and also designing instruction. Zhang ( 2022 ) described how designing an AI teaching system of talent cultivation and using the digital affordances to establish a quality assurance system for practical teaching, provides new mechanisms for the design of university education systems. In developing such a system, Zhang found that the stability of the instructional design, overcame the drawbacks of traditional manual subjectivity in the instructional design.
Another trend that emerged from the studies was the use of AI to manage student big data to support learning. Ullah and Hafiz ( 2022 ) lament that using traditional methods, including non-AI digital techniques, asking the instructor to pay attention to every student’s learning progress is very difficult and that big data analysis techniques are needed. The ability to look across and within large data sets to inform instruction is a valuable affordance of AIEd in HE. While the use of AIEd to manage student learning emerged from the data, this study uncovered only 19 studies in 7 years (2016–2022) that focused on the use of AIEd to manage student data. This lack of the use was also noted in a recent study in the K-12 space (Crompton et al., 2022 ). In Chu et. al.’s ( 2022 ) study examining the top 50 most cited AIEd articles, they did not report the use of AIEd for managing student data in the top uses of AIEd HE. It would appear that more research should be conducted in this area to fully explore the possibilities of AI.
Gaps and future research
From this systematic review, six gaps emerged in the data providing opportunities for future studies to investigate and provide a fuller understanding of how AIEd can used in HE. (1) The majority of the research was conducted in high income countries revealing a paucity of research in developing countries. More research should be conducted in these developing countries to expand the level of understanding about how AI can enhance learning in under-resourced communities. (2) Almost 50% of the studies were conducted in the areas of language learning, computer science and engineering. Research conducted by members from multiple, different academic departments would help to advance the knowledge of the use of AI in more disciplines. (3) This study revealed that faculty affiliated with schools of education are taking an increasing role in researching the use of AIEd in HE. As this body of knowledge grows, faculty in Schools of Education should share their research regarding the pedagogical affordances of AI so that this knowledge can be applied by faculty across disciplines. (4) The vast majority of the research was conducted at the undergraduate level. More research needs to be done at the graduate student level, as AI provides many opportunities in this environment. (5) Little study was done regarding how AIEd can assist both instructors and managers in their roles in HE. The power of AI to assist both groups further research. (6) Finally, much of the research investigated in this systematic review revealed the use of AIEd in traditional ways that enhance or make more efficient current practices. More research needs to focus on the unexplored affordances of AIEd. As AI becomes more advanced and sophisticated, new opportunities will arise for AIEd. Researchers need to be on the forefront of these possible innovations.
In addition, empirical exploration is needed for new tools, such as ChatGPT that was available for public use at the end of 2022. With the time it takes for a peer review journal article to be published, ChatGPT did not appear in the articles for this study. What is interesting is that it could fit with a variety of the use codes found in this study, with students getting support in writing papers and instructors using Chat GPT to assess students work and with help writing emails or descriptions for students. It would be pertinent for researchers to explore Chat GPT.
Limitations
The findings of this study show a rapid increase in the number of AIEd studies published in HE. However, to ensure a level of credibility, this study only included peer review journal articles. These articles take months to publish. Therefore, conference proceedings and gray literature such as blogs and summaries may reveal further findings not explored in this study. In addition, the articles in this study were all published in English which excluded findings from research published in other languages.
In response to the call by Hinojo-Lucena et. al. ( 2019 ), Chu et. al. ( 2022 ), and Zawacki-Richter et. al. ( 2019 ), this study provides unique findings with an up-to-date examination of the use of AIEd in HE from 2016 to 2022. Past systematic reviews examined the research up to 2020. The findings of this study show that in 2021 and 2022, publications rose nearly two to three times the number of previous years. With this rapid rise in the number of AIEd HE publications, new trends have emerged.
The findings show that of the 138 studies examined, research was conducted in six of the seven continents of the world. In extant systematic reviews showed that the US led by a large margin in the number of studies published. This trend has now shifted to China. Another shift in AIEd HE is that while extant studies lamented the lack of focus on professors of education leading these studies, this systematic review found education to be the most common department affiliation with 28% and computer science coming in second at 20%. Undergraduate students were the most studied students at 72%. Similar to the findings of other studies, language learning was the most common subject domain. This included writing, reading, and vocabulary acquisition. In examination of who the AIEd was intended for, 72% of the studies focused on students, 17% instructors, and 11% managers.
Grounded coding was used to answer the overarching question of how AIEd was used in HE. Five usage codes emerged from the data: (1) Assessment/Evaluation, (2) Predicting, (3) AI Assistant, (4) Intelligent Tutoring System (ITS), and (5) Managing Student Learning. Assessment and evaluation had a wide variety of purposes, including assessing academic progress and student emotions towards learning, individual and group evaluations, and class based online community assessments. Predicting emerged as a code with ten axial codes, as AIEd predicted dropouts and at-risk students, innovative ability, and career decisions. AI Assistants were specific to supporting students in HE. These assistants included those with an anthropomorphic presence, such as virtual agents and persuasive intervention through digital programs. ITS systems were not always noted in extant systematic reviews but were specifically mentioned in 18 of the studies in this review. ITS systems in this study provided customized strategies and approaches to student’s characteristics and needs. The final code in this study highlighted the use of AI in managing student learning, including learning analytics, curriculum sequencing, instructional design, and clustering of students.
The findings of this study provide a springboard for future academics, practitioners, computer scientists, policymakers, and funders in understanding the state of the field in AIEd HE, how AI is used. It also provides actionable items to ameliorate gaps in the current understanding. As the use AIEd will only continue to grow this study can serve as a baseline for further research studies in the use of AIEd in HE.
Availability of data and materials
The datasets used and/or analysed during the current study are available from the corresponding author on reasonable request.
Alajmi, Q., Al-Sharafi, M. A., & Abuali, A. (2020). Smart learning gateways for Omani HEIs towards educational technology: Benefits, challenges and solutions. International Journal of Information Technology and Language Studies, 4 (1), 12–17.
Google Scholar
Al-Tuwayrish, R. K. (2016). An evaluative study of machine translation in the EFL scenario of Saudi Arabia. Advances in Language and Literary Studies, 7 (1), 5–10.
Ayse, T., & Nil, G. (2022). Automated feedback and teacher feedback: Writing achievement in learning English as a foreign language at a distance. The Turkish Online Journal of Distance Education, 23 (2), 120–139. https://doi.org/10.7575/aiac.alls.v.7n.1p.5
Article Google Scholar
Baykasoğlu, A., Özbel, B. K., Dudaklı, N., Subulan, K., & Şenol, M. E. (2018). Process mining based approach to performance evaluation in computer-aided examinations. Computer Applications in Engineering Education, 26 (5), 1841–1861. https://doi.org/10.1002/cae.21971
Belur, J., Tompson, L., Thornton, A., & Simon, M. (2018). Interrater reliability in systematic review methodology: Exploring variation in coder decision-making. Sociological Methods & Research, 13 (3), 004912411887999. https://doi.org/10.1177/0049124118799372
Çağataylı, M., & Çelebi, E. (2022). Estimating academic success in higher education using big five personality traits, a machine learning approach. Arab Journal Scientific Engineering, 47 , 1289–1298. https://doi.org/10.1007/s13369-021-05873-4
Chen, L., Chen, P., & Lin, Z. (2020). Artificial intelligence in education: A review. IEEE Access, 8 , 75264–75278. https://doi.org/10.1109/ACCESS.2020.2988510
Chu, H., Tu, Y., & Yang, K. (2022). Roles and research trends of artificial intelligence in higher education: A systematic review of the top 50 most-cited articles. Australasian Journal of Educational Technology, 38 (3), 22–42. https://doi.org/10.14742/ajet.7526
Cristianini, N. (2016). Intelligence reinvented. New Scientist, 232 (3097), 37–41. https://doi.org/10.1016/S0262-4079(16)31992-3
Crompton, H., Bernacki, M. L., & Greene, J. (2020). Psychological foundations of emerging technologies for teaching and learning in higher education. Current Opinion in Psychology, 36 , 101–105. https://doi.org/10.1016/j.copsyc.2020.04.011
Crompton, H., & Burke, D. (2022). Artificial intelligence in K-12 education. SN Social Sciences, 2 , 113. https://doi.org/10.1007/s43545-022-00425-5
Crompton, H., Jones, M., & Burke, D. (2022). Affordances and challenges of artificial intelligence in K-12 education: A systematic review. Journal of Research on Technology in Education . https://doi.org/10.1080/15391523.2022.2121344
Crompton, H., & Song, D. (2021). The potential of artificial intelligence in higher education. Revista Virtual Universidad Católica Del Norte, 62 , 1–4. https://doi.org/10.35575/rvuen.n62a1
de Chiusole, D., Stefanutti, L., Anselmi, P., & Robusto, E. (2020). Stat-Knowlab. Assessment and learning of statistics with competence-based knowledge space theory. International Journal of Artificial Intelligence in Education, 30 , 668–700. https://doi.org/10.1007/s40593-020-00223-1
Dever, D. A., Azevedo, R., Cloude, E. B., & Wiedbusch, M. (2020). The impact of autonomy and types of informational text presentations in game-based environments on learning: Converging multi-channel processes data and learning outcomes. International Journal of Artificial Intelligence in Education, 30 (4), 581–615. https://doi.org/10.1007/s40593-020-00215-1
Górriz, J. M., Ramírez, J., Ortíz, A., Martínez-Murcia, F. J., Segovia, F., Suckling, J., Leming, M., Zhang, Y. D., Álvarez-Sánchez, J. R., Bologna, G., Bonomini, P., Casado, F. E., Charte, D., Charte, F., Contreras, R., Cuesta-Infante, A., Duro, R. J., Fernández-Caballero, A., Fernández-Jover, E., … Ferrández, J. M. (2020). Artificial intelligence within the interplay between natural and artificial computation: Advances in data science, trends and applications. Neurocomputing, 410 , 237–270. https://doi.org/10.1016/j.neucom.2020.05.078
Gough, D., Oliver, S., & Thomas, J. (2017). An introduction to systematic reviews (2nd ed.). Sage.
Gupta, S., & Chen, Y. (2022). Supporting inclusive learning using chatbots? A chatbot-led interview study. Journal of Information Systems Education, 33 (1), 98–108.
Hemingway, P. & Brereton, N. (2009). In Hayward Medical Group (Ed.). What is a systematic review? Retrieved from http://www.medicine.ox.ac.uk/bandolier/painres/download/whatis/syst-review.pdf
Hinojo-Lucena, F., Arnaz-Diaz, I., Caceres-Reche, M., & Romero-Rodriguez, J. (2019). A bibliometric study on its impact the scientific literature. Education Science . https://doi.org/10.3390/educsci9010051
Hrastinski, S., Olofsson, A. D., Arkenback, C., Ekström, S., Ericsson, E., Fransson, G., Jaldemark, J., Ryberg, T., Öberg, L.-M., Fuentes, A., Gustafsson, U., Humble, N., Mozelius, P., Sundgren, M., & Utterberg, M. (2019). Critical imaginaries and reflections on artificial intelligence and robots in postdigital K-12 education. Postdigital Science and Education, 1 (2), 427–445. https://doi.org/10.1007/s42438-019-00046-x
Huang, C., Wu, X., Wang, X., He, T., Jiang, F., & Yu, J. (2021). Exploring the relationships between achievement goals, community identification and online collaborative reflection. Educational Technology & Society, 24 (3), 210–223.
Hwang, G. J., & Tu, Y. F. (2021). Roles and research trends of artificial intelligence in mathematics education: A bibliometric mapping analysis and systematic review. Mathematics, 9 (6), 584. https://doi.org/10.3390/math9060584
Khalfallah, J., & Slama, J. B. H. (2018). The effect of emotional analysis on the improvement of experimental e-learning systems. Computer Applications in Engineering Education, 27 (2), 303–318. https://doi.org/10.1002/cae.22075
Kim, C., & Bennekin, K. N. (2016). The effectiveness of volition support (VoS) in promoting students’ effort regulation and performance in an online mathematics course. Instructional Science, 44 , 359–377. https://doi.org/10.1007/s11251-015-9366-5
Koć-Januchta, M. M., Schönborn, K. J., Roehrig, C., Chaudhri, V. K., Tibell, L. A. E., & Heller, C. (2022). “Connecting concepts helps put main ideas together”: Cognitive load and usability in learning biology with an AI-enriched textbook. International Journal of Educational Technology in Higher Education, 19 (11), 11. https://doi.org/10.1186/s41239-021-00317-3
Krause, S. D., & Lowe, C. (2014). Invasion of the MOOCs: The promise and perils of massive open online courses . Parlor Press.
Li, D., Tong, T. W., & Xiao, Y. (2021). Is China emerging as the global leader in AI? Harvard Business Review. https://hbr.org/2021/02/is-china-emerging-as-the-global-leader-in-ai
Liang, J. C., Hwang, G. J., Chen, M. R. A., & Darmawansah, D. (2021). Roles and research foci of artificial intelligence in language education: An integrated bibliographic analysis and systematic review approach. Interactive Learning Environments . https://doi.org/10.1080/10494820.2021.1958348
Liu, S., Hu, T., Chai, H., Su, Z., & Peng, X. (2022). Learners’ interaction patterns in asynchronous online discussions: An integration of the social and cognitive interactions. British Journal of Educational Technology, 53 (1), 23–40. https://doi.org/10.1111/bjet.13147
Long, P., & Siemens, G. (2011). Penetrating the fog: Analytics in learning and education. Educause Review, 46 (5), 31–40.
Lu, O. H. T., Huang, A. Y. Q., Tsai, D. C. L., & Yang, S. J. H. (2021). Expert-authored and machine-generated short-answer questions for assessing students learning performance. Educational Technology & Society, 24 (3), 159–173.
Mavrikis, M., Geraniou, E., Santos, S. G., & Poulovassilis, A. (2019). Intelligent analysis and data visualization for teacher assistance tools: The case of exploratory learning. British Journal of Educational Technology, 50 (6), 2920–2942. https://doi.org/10.1111/bjet.12876
Moher, D., Shamseer, L., Clarke, M., Ghersi, D., Liberati, A., Petticrew, M., Shekelle, P., & Stewart, L. (2015). Preferred reporting items for systematic review and meta-analysis protocols (PRISMA-P) 2015 statement. Systematic Reviews, 4 (1), 1–9. https://doi.org/10.1186/2046-4053-4-1
Mousavi, A., Schmidt, M., Squires, V., & Wilson, K. (2020). Assessing the effectiveness of student advice recommender agent (SARA): The case of automated personalized feedback. International Journal of Artificial Intelligence in Education, 31 (2), 603–621. https://doi.org/10.1007/s40593-020-00210-6
Mousavinasab, E., Zarifsanaiey, N., Kalhori, S. R. N., Rakhshan, M., Keikha, L., & Saeedi, M. G. (2021). Intelligent tutoring systems: A systematic review of characteristics, applications, and evaluation methods. Interactive Learning Environments, 29 (1), 142–163. https://doi.org/10.1080/10494820.2018.1558257
Ouatik, F., Ouatikb, F., Fadlic, H., Elgoraria, A., Mohadabb, M. E. L., Raoufia, M., et al. (2021). E-Learning & decision making system for automate students assessment using remote laboratory and machine learning. Journal of E-Learning and Knowledge Society, 17 (1), 90–100. https://doi.org/10.20368/1971-8829/1135285
Ouyang, F., Zheng, L., & Jiao, P. (2022). Artificial intelligence in online higher education: A systematic review of empirical research from 2011–2020. Education and Information Technologies, 27 , 7893–7925. https://doi.org/10.1007/s10639-022-10925-9
Page, M. J., McKenzie, J. E., Bossuyt, P. M., Boutron, I., Hoffmann, T., Mulrow, C., Shamseer, L., Tetzlaff, J. M., Akl, E. A., Brennan, S. E., Chou, R., Glanville, J., Grimshaw, J. M., Hróbjartsson, A., Lalu, M. M., Li, T., Loder, E. W., Mayo-Wilson, E., McDonald, S., … Moher, D. (2021). The PRISMA 2020 statement: An updated guideline for reporting systematic reviews. British Medical Journal . https://doi.org/10.1136/bmj.n71
Popenici, S. A. D., & Kerr, S. (2017). Exploring the impact of artificial intelligence on teaching and learning in higher education. Research and Practice in Technology Enhanced Learning, 12 (22), 1–13. https://doi.org/10.1186/s41039-017-0062-8
PRISMA Statement. (2021). PRISMA endorsers. PRISMA statement website. http://www.prisma-statement.org/Endorsement/PRISMAEndorsers
Qian, Y., Li, C.-X., Zou, X.-G., Feng, X.-B., Xiao, M.-H., & Ding, Y.-Q. (2022). Research on predicting learning achievement in a flipped classroom based on MOOCs by big data analysis. Computer Applied Applications in Engineering Education, 30 , 222–234. https://doi.org/10.1002/cae.22452
Rutner, S. M., & Scott, R. A. (2022). Use of artificial intelligence to grade student discussion boards: An exploratory study. Information Systems Education Journal, 20 (4), 4–18.
Salas-Pilco, S., & Yang, Y. (2022). Artificial Intelligence application in Latin America higher education: A systematic review. International Journal of Educational Technology in Higher Education, 19 (21), 1–20. https://doi.org/10.1186/S41239-022-00326-w
Saldana, J. (2015). The coding manual for qualitative researchers (3rd ed.). Sage.
Shukla, A. K., Janmaijaya, M., Abraham, A., & Muhuri, P. K. (2019). Engineering applications of artificial intelligence: A bibliometric analysis of 30 years (1988–2018). Engineering Applications of Artificial Intelligence, 85 , 517–532. https://doi.org/10.1016/j.engappai.2019.06.010
Strauss, A., & Corbin, J. (1995). Grounded theory methodology: An overview. In N. K. Denzin & Y. S. Lincoln (Eds.), Handbook of qualitative research (pp. 273–285). Sage.
Turing, A. M. (1937). On computable numbers, with an application to the Entscheidungs problem. Proceedings of the London Mathematical Society, 2 (1), 230–265.
Turing, A. M. (1950). Computing machinery and intelligence. Mind, 59 , 443–460.
MathSciNet Google Scholar
Ullah, H., & Hafiz, M. A. (2022). Exploring effective classroom management strategies in secondary schools of Punjab. Journal of the Research Society of Pakistan, 59 (1), 76.
Verdú, E., Regueras, L. M., Gal, E., et al. (2017). Integration of an intelligent tutoring system in a course of computer network design. Educational Technology Research and Development, 65 , 653–677. https://doi.org/10.1007/s11423-016-9503-0
Vygotsky, L. S. (1978). Mind and society: The development of higher psychological processes . Harvard University Press.
Winkler-Schwartz, A., Bissonnette, V., Mirchi, N., Ponnudurai, N., Yilmaz, R., Ledwos, N., Siyar, S., Azarnoush, H., Karlik, B., & Del Maestro, R. F. (2019). Artificial intelligence in medical education: Best practices using machine learning to assess surgical expertise in virtual reality simulation. Journal of Surgical Education, 76 (6), 1681–1690. https://doi.org/10.1016/j.jsurg.2019.05.015
Yang, A. C. M., Chen, I. Y. L., Flanagan, B., & Ogata, H. (2021). Automatic generation of cloze items for repeated testing to improve reading comprehension. Educational Technology & Society, 24 (3), 147–158.
Yao, X. (2022). Design and research of artificial intelligence in multimedia intelligent question answering system and self-test system. Advances in Multimedia . https://doi.org/10.1155/2022/2156111
Zawacki-Richter, O., Marín, V. I., Bond, M., & Gouverneur, F. (2019). Systematic review of research on artificial intelligence applications in higher education—Where are the educators? International Journal of Educational Technology in Higher Education, 16 (1), 1–27. https://doi.org/10.1186/s41239-019-0171-0
Zhang, F. (2022). Design and application of artificial intelligence technology-driven education and teaching system in universities. Computational and Mathematical Methods in Medicine . https://doi.org/10.1155/2022/8503239
Zhang, Z., & Xu, L. (2022). Student engagement with automated feedback on academic writing: A study on Uyghur ethnic minority students in China. Journal of Multilingual and Multicultural Development . https://doi.org/10.1080/01434632.2022.2102175
Download references
Acknowledgements
The authors would like to thank Mildred Jones, Katherina Nako, Yaser Sendi, and Ricardo Randall for data gathering and organization.
Author information
Authors and affiliations.
Department of Teaching and Learning, Old Dominion University, Norfolk, USA
Helen Crompton
ODUGlobal, Norfolk, USA
Diane Burke
RIDIL, ODUGlobal, Norfolk, USA
You can also search for this author in PubMed Google Scholar
Contributions
HC: Conceptualization; Data curation; Project administration; Formal analysis; Methodology; Project administration; original draft; and review & editing. DB: Conceptualization; Data curation; Project administration; Formal analysis; Methodology; Project administration; original draft; and review & editing. Both authors read and approved this manuscript.
Corresponding author
Correspondence to Helen Crompton .
Ethics declarations
Competing interests.
The authors declare that they have no competing interests.
Additional information
Publisher's note.
Springer Nature remains neutral with regard to jurisdictional claims in published maps and institutional affiliations.
Rights and permissions
Open Access This article is licensed under a Creative Commons Attribution 4.0 International License, which permits use, sharing, adaptation, distribution and reproduction in any medium or format, as long as you give appropriate credit to the original author(s) and the source, provide a link to the Creative Commons licence, and indicate if changes were made. The images or other third party material in this article are included in the article's Creative Commons licence, unless indicated otherwise in a credit line to the material. If material is not included in the article's Creative Commons licence and your intended use is not permitted by statutory regulation or exceeds the permitted use, you will need to obtain permission directly from the copyright holder. To view a copy of this licence, visit http://creativecommons.org/licenses/by/4.0/ .
Reprints and permissions
About this article
Cite this article.
Crompton, H., Burke, D. Artificial intelligence in higher education: the state of the field. Int J Educ Technol High Educ 20 , 22 (2023). https://doi.org/10.1186/s41239-023-00392-8
Download citation
Received : 30 January 2023
Accepted : 23 March 2023
Published : 24 April 2023
DOI : https://doi.org/10.1186/s41239-023-00392-8
Share this article
Anyone you share the following link with will be able to read this content:
Sorry, a shareable link is not currently available for this article.
Provided by the Springer Nature SharedIt content-sharing initiative
- Artificial Intelligence
- Systematic review
- Higher education
Towards Integrating Emerging AI Applications in SE Education
Artificial Intelligence (AI) approaches have been incorporated into modern learning environments and software engineering (SE) courses and curricula for several years. However, with the significant rise in popularity of large language models (LLMs) in general, and OpenAI ’s LLM-powered chatbot ChatGPT in particular in the last year, educators are faced with rapidly changing classroom environments and disrupted teaching principles. Examples range from programming assignment solutions that are fully generated via ChatGPT, to various forms of cheating during exams. However, despite these negative aspects and emerging challenges, AI tools in general, and LLM applications in particular, can also provide significant opportunities in a wide variety of SE courses, supporting both students and educators in meaningful ways. In this early research paper, we present preliminary results of a systematic analysis of current trends in the area of AI, and how they can be integrated into university-level SE curricula, guidelines, and approaches to support both instructors and learners. We collected both teaching and research papers and analyzed their potential usage in SE education, using the ACM Computer Science Curriculum Guidelines CS2023. As an initial outcome, we discuss a series of opportunities for AI applications and further research areas.
1 Introduction
Artificial Intelligence (AI) in education, particularly at the university level, has been an active area for several years now, ranging from intelligent tutoring systems to learning analytics support [ 34 , 22 ] . However, the recent rise in popularity of large language models (LLMs) and chatbots has already disrupted teaching principles in many programming and software engineering (SE) courses. Solutions to programming exercises are automatically generated by students using, for example, ChatGPT and pose further challenges for educators when conducting online exams [ 31 ] . Despite the challenges, AI tools in general, and LLM applications in particular, can also be meaningfully employed in a wide variety of SE courses, commonly part of computer science curricula, supporting learners in tasks such as test case generation, architecture pattern creation, or bug detection [ 35 , 39 ] . Other application scenarios include providing individual feedback to students [ 1 ] , chatbots to increase learning experience [ 2 ] , or using AI-based tools as programming assistants [ 26 ] . However, so far, only a fraction of these aspects and novel application areas are covered in SE education and curricula. Moreover, not only learners can benefit from these new opportunities, but also teachers and educators may use them, for example, for generating assignment exercises, or as part of automated grading and assessment systems [ 14 ] . In either application scenario, however, the unreflected and careless use of these new tools can also impact the learning experience and outcomes in negative ways, posing new challenges to both students and educators [ 3 ] . To exacerbate the situation, existing didactic concepts in the context of SE education hardly take into account or take advantage of these new tools. Also, universities often lack guidelines for the ethical and responsible usage of AI tools in education. Apart from that, students need to be prepared for the ethical implications and societal impacts of AI technologies.
In this paper, we present a preliminary analysis of current trends in the area of AI and how they can potentially be integrated into SE curricula. As part of this, we specifically investigate how they can be leveraged in SE education for both students and educators and how didactic concepts can be adapted to appropriately deal with these new applications. For this purpose, we (1) collected current research on this topic from the past years, (2) performed further snowballing, and (3) grouped the extracted approaches and application areas with regard to their potential usage in SE education, using the ACM Computer Science Curriculum Guidelines version “Gamma” 1 1 1 Please visit csed.acm.org/cs2023-gamma for more information. , currently under review.
The main goal of our ongoing work is to gain a better understanding of how the current state of research in AI applications is reflected in SE education, identifying emerging challenges and opportunities for SE courses. As a result, in this short paper, we present an initial overview of techniques and exemplary use cases, alongside a roadmap for further research on and applications of AI tools in SE education.
2 Study Design & Related Work
In this section, we first provide a brief motivation for the need to systematically introduce AI in SE education, and further discuss existing related work in this area.
2.1 Identifying AI Trends in Research and Education
To gain better insights into current trends – particularly within the past year – we started a structured literature analysis for both research and educational venues in software engineering. We specifically chose to include both research and education venues, hoping this will provide a more comprehensive understanding of current research trends and the extent to which they have been integrated into SE education. Furthermore, we aim to document challenges educators need to be aware of and, as a result, potentially adapt didactic concepts and/or teaching materials. In this short paper, we report on our work in progress and the first results of this analysis. For this purpose, we discuss initial findings (cf. Section 3 ) and potential applications and opportunities (cf. Section 4 ).
To enable a more structured analysis, we further aim to categorize our findings alongside the different SE activities and areas. For SE, numerous taxonomies and classification schemes have been proposed, most notably the Software Engineering Body of Knowledge (SEBoK) [ 17 ] , with its 18 knowledge areas. As our primary focus, however, is on SE education, we have chosen to group our findings according to the ACM Computer Science Curriculum Guidelines version “Gamma” (CS2023), comprising 17 different knowledge areas, which also incorporate – to a very large extent – the areas proposed in the SEBoK. The ACM Computer Science Curriculum Guidelines are designed to assist educators in the area of computer science, describing the general structure, as well as listing important topics to cover, core and elective CS courses [ 32 ] . Most notably, the most recent version, CS2023, currently discussed by the ACM task force, will take into account competency-based education, incorporating a competency model, comprising required skills, and knowledge to be acquired by students. Introducing Learning Analytics and competency-based education in classes typically requires a significant effort and is also something we see huge potential for leveraging AI and alleviating the burden of, for example, manually assessing if all teaching materials correspond to specified competencies (cf. Section 4 ).
While CS2023 addresses a broad and comprehensive education, covering 17 different knowledge areas, ranging from computer science foundations, to AI, and parallel computing, we focus on two main areas: Software Development Fundamentals ( SDF ) that cover basic programming education, and second, Software Engineering ( SE ). A brief overview of the sub-categories for these two areas is shown in Table 1 . The goal of our work is to identify existing work and approaches for each sub-category of the two areas and collect different means of how AI can be incorporated, for example in teaching aspects of software architecture, such as design patterns, or architectural styles. For this purpose, as a starting point, to gain an initial understanding of current research and trends, we collected an initial set of research papers from 2023, of two prime software engineering conferences: the International Conf. of Software Engineering, and the International Conf. on the Foundations of Software Engineering, as well as four educational conferences/tracks: Software Engineering Education and Training Track@ICSE, the International Conf. on Software Engineering Education and Training, the Hawaii International Conf. on System Sciences, and the Conf. on Innovation and Technology in Computer Science Education.
The goal was to identify trends and hot topics and uncover areas that might be covered by research but not yet as part of the CS and SE education. In total, we started with a set of 51 publications (33 research-related and 18 education-related), which was extended to 71 after performing an extra round of snowballing. We focused on publications that presented AI applications or educational concepts in the area of SE and programming, but excluded any kind of study, analysis, or review that did not present a specific approach. After carefully reading the titles and abstracts, we excluded 17 papers that were deemed out of scope (e.g. tertiary studies), with a final set of 54 papers remaining.
After selecting our set of papers, we divided them randomly, with four researchers evaluating the papers independently. For the research papers, we focused on the purpose (e.g., test case generation), the technology used, and the specific application area. (Please note, that for the purpose of this paper, and for the sake of brevity, we mainly focus on the intent, and the relation to the CS2023 categories, but future work will broaden the scope of this analysis). For education papers, we also focused on the CS2023 categories, and tried to relate them to the research areas – and ultimately identify gaps and areas that are not yet considered in education. Based on this analysis, we then selected the respective ACM curriculum categories (i.e., Knowledge Units) where the proposed approach, tool, or research could be relevant or applied. After this step, we consolidated all assessments, discussed and resolved conflicts until mutual agreement was achieved, and tried to identify clusters of tools and approaches, as well as gaps. The final goal of the study is to derive a set of concrete actionable guidelines for different courses part of the CS curriculum, with a set of tools and approaches that could be used, and aspects that need to be considered. In the following sections, we discuss the initial assignment of approaches to the CS2023 Knowledge Units and potential application scenarios.
2.2 Related Work
Several systematic reviews have investigated and classified aspects of AI applications in education. Bittencourt et al. [ 4 ] conducted an analysis exploring the intersection of positive psychology and AI in education (P-AIED), identifying P-AIED as a new global movement focusing on positive emotion and engagement related to AI-supported teaching and learning. Kuhail et al. [ 21 ] and Okonkwo and Ade-Ibijola [ 29 ] performed studies related to the application of chatbots in education. Kuhail et al. analyzed work on educational chatbots and found that these are primarily used in computer science, language learning, general education, and to a lesser extent in fields like engineering and mathematics. Most chatbots were web-based and more than half functioned as teaching agents, while over a third acted as peer agents. Okonkwo and Ade-Ibijola analyze 53 primary studies and identify an increasing trend in the application of chatbots in education.
Zhai et al. [ 41 ] analyzed 100 papers focusing on the application of AI in the education sector from 2010 to 2020. They classified primary studies into a development layer (classification, matching, recommendation, and deep learning), an application layer (feedback, reasoning, and adaptive learning), and an integration layer (affection computing, role-playing, immersive learning, and gamification). Chen et al. [ 6 ] performed a study investigating the impact of AI on education, focusing on its application and effects in administrative, instructional, and learning contexts. Similarly, Zawacki-Richter et al. [ 40 ] conducted a systematic review of research on the application of AI in higher education. The majority of research comes from the fields of Computer Science and STEM with AI being mainly used in areas of student profiling and predictive analytics, as well as intelligent tutoring systems.
Several studies have focused on AI in computer science education. Denny et al. [ 9 ] explore the challenges and opportunities presented by recent advances in AI, particularly code generation models, and their potential impact on computing education. Kalles [ 19 ] reports on the experience of using AI systems as the basis of educating IT students. The application areas were mainly decision tree lifecycle management and board game learning mechanisms. Daun and Brings [ 8 ] discuss the role of generative AI technologies, such as ChatGPT, in SE education. They discuss potential risks associated with students’ use of generative AI but also identify several opportunities that these technologies can offer in enhancing educational practices such as individualization of education and personalized feedback.
Current work in this regard primarily addresses only parts of SE education, for example, programming. With our work, we aim to cover a broader spectrum of SE education, leveraging the ACM Computer Science Curriculum Guidelines.
3 Current AI Trends & Applications
The classification of the 54 recent AI papers provides several insights into potential areas where AI topics can be incorporated into computer science and SE courses. Table 1 provides an overview of the curriculum categories and number of papers relevant to this category, alongside some examples. In the following, we provide a brief summary 2 2 2 Please refer to doi.org/10.5281/zenodo.11316028 for the full classification. of potential applications in four distinct categories, which we identified by grouping the categories with the highest coverage.
Introductory Programming Education (cf. SDF 1 to SDF 4 )
The integration of AI into courses for novice programmers offers novel methods for enhancing the learning experience and addressing educational challenges. Recent works have studied the application of LLMs to block-based languages [ 15 ] which are often used in introductory programming courses. Incorporating AI-based code generation tools [ 33 ] can offer real-time coding assistance, thereby facilitating a deeper and more rapid comprehension of programming fundamentals. Furthermore, pair programming assistants [ 26 ] can provide on-demand, context-aware coding suggestions.
Design and Construction (cf. SDF 4 , SE 1 , SE 3 to SE 5 )
Given that software design focuses on the engineering-oriented design of internal software components, “machine-learning-based knowledge extraction techniques” [ 27 ] can be employed not only to discover, but also to better understand application programming interfaces (APIs). In the realm of software construction, recent studies have shed light on the robustness of code generation techniques, such as GitHub Copilot , which automatically generates code from natural language descriptions [ 25 ] . In addition, advances in so-called contrastive learning, exemplified by CoCoSoDa , have significantly enhanced the accuracy and performance of code search [ 37 ] . Moreover, the emergence of systems such as AlphaCode represent a breakthrough as they demonstrate competitive-level code generation capabilities, impacting problem-solving in programming competitions [ 24 ] .
Maintenance and Testing (cf. SE 2 , SE 7 )
The integration of AI into more advanced SE courses is currently only sparsely covered in education research, despite the existence of several research advances in this area. This includes, for example, automated test case generation or test augmentation support [ 28 ] . LLMs have also been employed in this context [ 20 ] fed with bug reports and tasked with generating a series of test candidates. Incorporating these tools in advanced software engineering, and software testing courses can foster awareness of these new tools and technologies. It is, however, also important to note, and convey the message, that these can only serve as an augmentation to existing, traditional, testing methods and are not meant to replace them.
V&V, Reliability, and Security (cf. SE 6 , SE 8 , SE 9 )
The integration of new tools using AI approaches for software verification and validation to recognize bugs or identify vulnerabilities has become an established research area. For example, models such as CodeBERT are used to recognize errors in source code [ 16 ] . In addition, the combination of ML and NLP has led to the development of novel AI approaches enabling code translation [ 42 ] , code repair, and inconsistency checking [ 5 ] to increase reliability and security of code.
4 Challenges & Opportunities in SE Education
Based on the analysis of trending topics and educational use, we have identified several areas of opportunities where AI could support both educators and students in various tasks. In the following section, we present two directions currently not – or only partially – covered in SE education research, where (1) AI tools should be considered, how AI tools and methods could be applied to (2) alleviate exercise creation as well as grading tasks, and (3) provide tailored support for students. Our literature search has shown that current work related to AI in SE education has a strong focus on exercise generation [ 38 ] , assessment [ 14 ] , and grading [ 1 ] using LLMs. However, the quality of the results varies and often does not yield satisfactory programming tasks or grading results (cf. Direction I ). Furthermore, going beyond introduction to programming courses, i.e., CS1, as well as basic data structures courses, i.e., CS2, we observed, that while being a very active research topic, only a few papers address more advanced areas in SE education (cf. Direction II ).
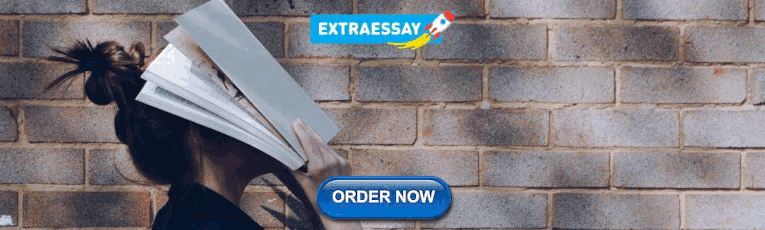
Direction I – Competency-focused Exercise Generation (Educators)
Following the trend of employing generative AI for a slew of different tasks, one potential application area could be the creation of more diverse and comprehensive exercises, e.g., for programming classes. While similar works have been proposed recently [ 38 , 13 ] , we think that this can go far beyond “simply” creating exercise sheets or programming tasks. Particularly taking into account competency-based education [ 30 ] , which is also reflected in the most recent 2023 ACM curriculum guidelines, with a dedicated competency model, LLMs could be used in this context. First, to create a set of tasks that covers certain competencies, e.g., specified in a competency model, but also to generate specific test cases that cover/check the achievement of certain competencies, and even provide support for assigning assignments and identifying gaps in missing competences or tasks that exceed what students should be able to accomplish as part of an assignment.
The challenge hereby resides in providing the LLM with the necessary information – e.g., a competency model – and the context and scope of the assignment and tasks.
The benefits are on the one hand a significant reduction in the manual tedious effort of creating variations of exercises (e.g., programming tasks) and on the other hand a move towards competency-based assessment and Learning Analytics support, without the need to manually incorporate these aspects in exercises and assignments.
Direction II – AI in SE (Educators and Students)
Various authors have stressed the application of AI tools in Software Development Fundamentals ( SDF 1 , SDF 2 , and SDF 3 ), both as an opportunity and a challenge (e.g., students generating assignments without critical reflection). However, the deeper application of AI tools in advanced SE classes does not seem to be widely considered [ 18 ] . In contrast, we discovered a wide variety of AI applications in research that intersect with basic and advanced software engineering education. Particularly software quality assurance, maintenance, and validation ( SE 6 and SE 8 ) are covered by several research papers, but are hardly present in the educational context.
The challenge from our perspective is striking a balance between those new technologies and tools that alleviate certain tasks and still teaching basic and advanced concepts and theories to students. For example, if code generation tools take over tasks, such as generating architectural patterns or unit tests, it is still crucial to convey knowledge about these foundational aspects so that students know how to apply them properly and understand the concepts behind them.
The benefits are that students are introduced to new tools and paradigms early on in their education that they are very likely to encounter later on during their professional careers. Moreover, it fosters understanding of AI capabilities and limitations, preparing students to understand ethical implications and societal impacts with a well-informed, critical perspective.
5 Outlook and Conclusion
With the release of ChatGPT, AI tools, and particularly LLMs have significantly impacted both educators and students, often being perceived as a boon and a bane at the same time. While providing a vast amount of opportunities for new and improved tools for generating code, analyzing bugs, or improving code quality, these aspects are only sparsely converted in current software engineering courses. In this early research paper, we present a preliminary analysis of current trends in the area of AI, and how they can be integrated into SE curricula. We further discuss several challenges and opportunities where AI-powered tools and techniques can support teachers and learners, but also need to be taken into account when designing exercises or course assignments.
We will further explore the development and evaluation of didactic methods that integrate AI tools into university-level courses. Additionally, there is a need to investigate the long-term impacts of AI integration on learning outcomes and employability of students.
- [1] R. Balse, B. Valaboju, S. Singhal, J. M. Warriem, and P. Prasad. Investigating the potential of GPT-3 in providing feedback for programming assessments. In Proc. of the 2023 Conf. on Innovation and Technology in Computer Science Education , page 292–298. ACM, 2023.
- [2] D. Benner, S. Schöbel, A. Janson, and J. M. Leimeister. Engaging minds - how gamified chatbots can support and motivate learners in digital education. In T. X. Bui, editor, Proc. of the 57th Hawaii Int’l Conf. on System Sciences , pages 54–63. ScholarSpace, 2024.
- [3] J. Bergström, V. Repo, and V. Tuunainen. Challenges to academic integrity from new tools-a survey of students’ perceptions and behaviors of employing chatgpt. 2024.
- [4] I. I. Bittencourt, G. Chalco, J. Santos, S. Fernandes, J. Silva, N. Batista, C. Hutz, and S. Isotani. Positive artificial intelligence in education (P-AIED): A roadmap. International Journal of Artificial Intelligence in Education , pages 1–61, 2023.
- [5] I. Bouzenia and M. Pradel. When to say what: Learning to find condition-message inconsistencies. In Proc. of the 45th Int’l Conf. on Software Engineering , pages 868–880. IEEE, 2023.
- [6] L. Chen, P. Chen, and Z. Lin. Artificial intelligence in education: A review. IEEE Access , 8:75264–75278, 2020.
- [7] B. P. Cipriano and P. Alves. Gpt-3 vs object oriented programming assignments: An experience report. In 2023 Conf. on Innovation and Technology in Computer Science Education V.1 , pages 61–67, 2023.
- [8] M. Daun and J. Brings. How chatgpt will change software engineering education. In Proc. of the 2023 Conf. on Innovation and Technology in Computer Science Education V.1 , pages 110–116, 2023.
- [9] P. Denny, J. Prather, B. A. Becker, J. Finnie-Ansley, A. Hellas, J. Leinonen, A. Luxton-Reilly, B. N. Reeves, E. A. Santos, and S. Sarsa. Computing education in the era of generative AI, 2023.
- [10] J. Dong, Y. Lou, D. Hao, and L. Tan. Revisiting learning-based commit message generation. In Proc. of the 45th Int’l Conference on Software Engineering , ICSE ’23, page 794–805. IEEE Press, 2023.
- [11] S. Ezzini, S. Abualhaija, C. Arora, and M. Sabetzadeh. AI-based question answering assistance for analyzing natural-language requirements. arXiv preprint arXiv:2302.04793 , 2023.
- [12] E. First, M. Rabe, T. Ringer, and Y. Brun. Baldur: Whole-proof generation and repair with large language models. In Proc. of the 31st ACM Joint European Software Engineering Conf. and Symposium on the Foundations of Software Engineering , pages 1229–1241, 2023.
- [13] T. C. Freitas, A. Costa Neto, M. J. V. Pereira, and P. R. Henriques. NLP/AI based techniques for programming exercises generation. In Proc. of the 4th Int’l Computer Programming Education Conf. Schloss Dagstuhl-Leibniz-Zentrum für Informatik, 2023.
- [14] V. González-Calatayud, P. Prendes-Espinosa, and R. Roig-Vila. Artificial intelligence for student assessment: A systematic review. Applied Sciences , 11(12):5467, 2021.
- [15] E. Griebl, B. Fein, F. Obermüller, G. Fraser, and R. Just. On the applicability of language models to block-based programs. In Proc. of the 45th Int’l Conf. on Software Engineering , pages 2374–2386, 2023.
- [16] A. Grishina, M. Hort, and L. Moonen. The earlybird catches the bug: On exploiting early layers of encoder models for more efficient code classification. arXiv preprint arXiv:2305.04940 , 2023.
- [17] IEEE Computer Society. SWEBOK Guide Version 4.0 beta. https://sebokwiki.org/wiki/SWEBOK_Guide_Version_4.0 , Last accessed 01-06-2024.
- [18] M. Johnson. Generative AI and CS education. Communications of the ACM , 67(4):23–24, Mar. 2024.
- [19] D. Kalles. Artificial intelligence meets software engineering in computing education. In Proc. of the 9th Hellenic Conf. on Artificial Intelligence , USA, 2016. ACM.
- [20] S. Kang, J. Yoon, and S. Yoo. Large language models are few-shot testers: Exploring LLM-based general bug reproduction. In Proc. of the 45th Int’l Conf. on Software Engineering , pages 2312–2323. IEEE, 2023.
- [21] M. A. Kuhail, N. Alturki, S. Alramlawi, and K. Alhejori. Interacting with educational chatbots: A systematic review. Education and Information Technologies , 28(1):973–1018, 2023.
- [22] N.-T. Le, S. Strickroth, S. Gross, and N. Pinkwart. A review of ai-supported tutoring approaches for learning programming. Advanced computational methods for knowledge engineering , pages 267–279, 2013.
- [23] J. Leinonen, P. Denny, S. MacNeil, S. Sarsa, S. Bernstein, J. Kim, A. Tran, and A. Hellas. Comparing code explanations created by students and large language models. In 2023 Conf. on Innovation and Technology in Computer Science Education V. 1 . ACM, 2023.
- [24] Y. Li, D. Choi, J. Chung, N. Kushman, J. Schrittwieser, R. Leblond, T. Eccles, J. Keeling, F. Gimeno, A. Dal Lago, et al. Competition-level code generation with alphacode. Science , 378(6624):1092–1097, 2022.
- [25] A. Mastropaolo, L. Pascarella, E. Guglielmi, M. Ciniselli, S. Scalabrino, R. Oliveto, and G. Bavota. On the robustness of code generation techniques: An empirical study on github copilot. In Proc. of the 45th Int’l Conf. on Software Engineering , pages 2149–2160, 2023.
- [26] A. Moradi Dakhel, V. Majdinasab, A. Nikanjam, F. Khomh, M. C. Desmarais, and Z. M. J. Jiang. GitHub copilot AI pair programmer: Asset or liability? Journal of Systems and Software , 203, 2023.
- [27] D. Nam, B. Myers, B. Vasilescu, and V. Hellendoorn. Improving API knowledge discovery with ML: A case study of comparable API methods. In 45th Int’l Conf. on Software Engineering , pages 1890–1906, 2023.
- [28] P. Nie, R. Banerjee, J. J. Li, R. J. Mooney, and M. Gligoric. Learning deep semantics for test completion. preprint - arXiv:2302.10166 , 2023.
- [29] C. W. Okonkwo and A. Ade-Ibijola. Chatbots applications in education: A systematic review. Computers and Education: Artificial Intelligence , 2:100033, 2021.
- [30] M. C. Pluff and V. Weiss. Competency-based education: The future of higher education. New Models of Higher Education: Unbundled, Rebundled, Customized, and DIY , pages 200–218, 2022.
- [31] M. M. Rahman and Y. Watanobe. ChatGPT for education and research: Opportunities, threats, and strategies. Applied Sciences , 13(9):5783, 2023.
- [32] R. K. Raj and A. N. Kumar. Toward computer science curricular guidelines 2023 (cs2023). Inroads , 13(4):22–25, 2022.
- [33] B. Reeves, S. Sarsa, J. Prather, P. Denny, B. A. Becker, A. Hellas, B. Kimmel, G. Powell, and J. Leinonen. Evaluating the performance of code generation models for solving parsons problems with small prompt variations. In Proc. of the 2023 Conf. on Innovation and Technology in Computer Science Education V.1 , page 299–305. ACM, 2023.
- [34] S. Z. Salas-Pilco, K. Xiao, and X. Hu. Artificial intelligence and learning analytics in teacher education: A systematic review. Education Sciences , 12(8):569, 2022.
- [35] M. Schäfer, S. Nadi, A. Eghbali, and F. Tip. An empirical evaluation of using large language models for automated unit test generation. IEEE Transactions on Software Engineering , 2023.
- [36] A. Shau, Y. Liang, W. Hsieh, X. Lin, and S. Ma. PSAbot: A chatbot system for the analysis of posts on stack overflow. In Proc. of the 35th Int’l Conf. on Software Engineering Education and Training , pages 137–141. IEEE, 2023.
- [37] E. Shi, Y. Wang, W. Gu, L. Du, H. Zhang, S. Han, D. Zhang, and H. Sun. CoCoSoDa: Effective contrastive learning for code search. In Proc. of the 45th Int’l Conf. on Software Engineering , pages 2198–2210, 2023.
- [38] S. Speth, N. Meißner, and S. Becker. Investigating the use of ai-generated exercises for beginner and intermediate programming courses: A ChatGPT case study. In Proc. of the 35th Int’l Conf. on Software Engineering Education and Training , pages 142–146. IEEE, 2023.
- [39] C. S. Xia, Y. Wei, and L. Zhang. Automated program repair in the era of large pre-trained language models. In Proc. of the 45th Int’l Conf. on Software Engineering , pages 1482–1494, 2023.
- [40] O. Zawacki-Richter, V. I. Marín, M. Bond, and F. Gouverneur. Systematic review of research on artificial intelligence applications in higher education–where are the educators? Int’l Journal of Educational Technology in Higher Education , 16(1):1–27, 2019.
- [41] X. Zhai, X. Chu, C. S. Chai, M. S. Y. Jong, A. Istenic, M. Spector, J.-B. Liu, J. Yuan, and Y. Li. A review of artificial intelligence (AI) in education from 2010 to 2020. Complexity , 2021:1–18, 2021.
- [42] J. Zhang, P. Nie, J. J. Li, and M. Gligoric. Multilingual code co-evolution using large language models. In Proc. of the 31st ACM Joint European Software Engineering Conf. and Symposium on the Foundations of Software Engineering , pages 695–707, 2023.
How AI can accelerate students’ holistic development and make teaching more fulfilling
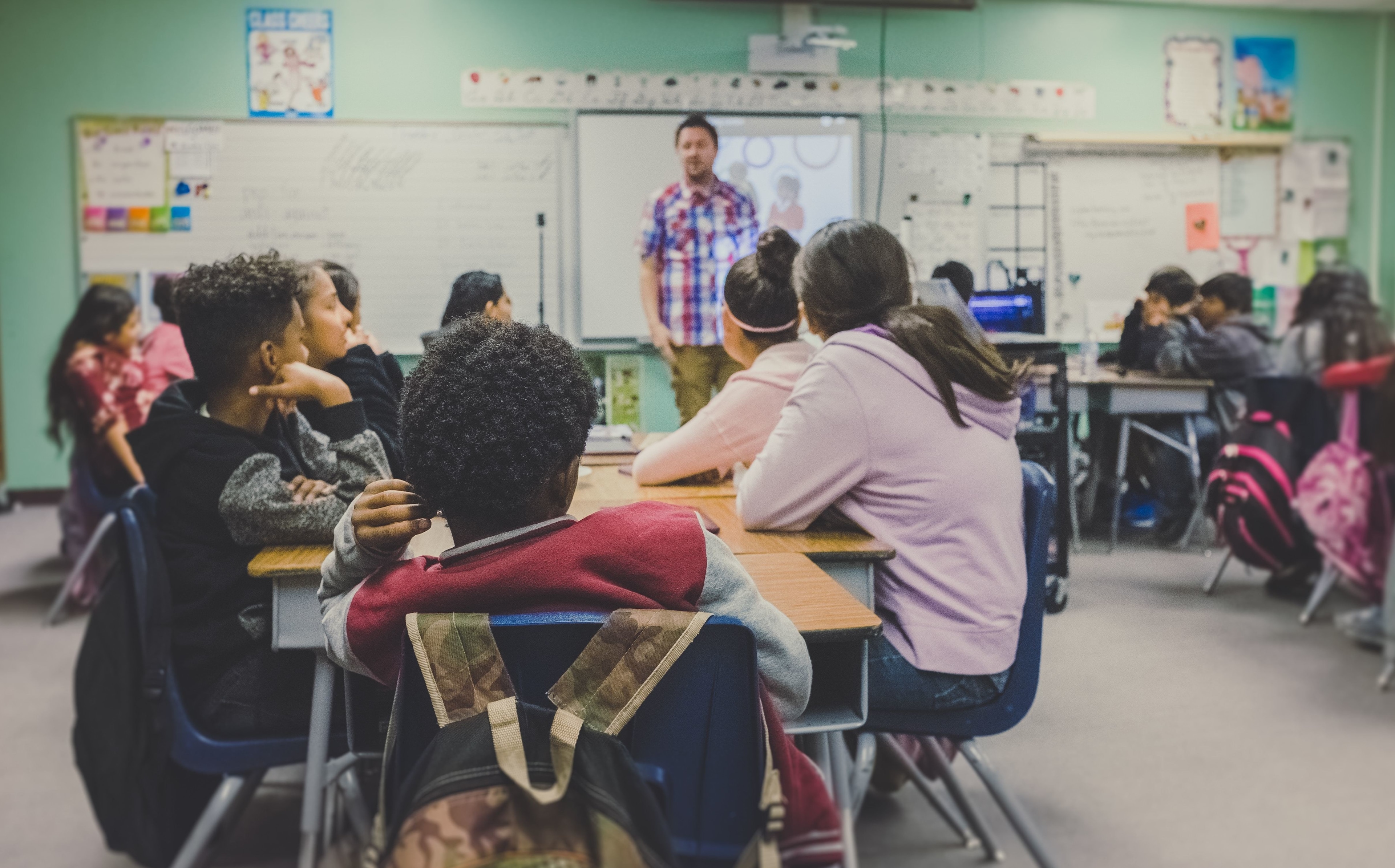
AI can free up time in the classroom. Image: Kenny Eliason on Unsplash
.chakra .wef-1c7l3mo{-webkit-transition:all 0.15s ease-out;transition:all 0.15s ease-out;cursor:pointer;-webkit-text-decoration:none;text-decoration:none;outline:none;color:inherit;}.chakra .wef-1c7l3mo:hover,.chakra .wef-1c7l3mo[data-hover]{-webkit-text-decoration:underline;text-decoration:underline;}.chakra .wef-1c7l3mo:focus,.chakra .wef-1c7l3mo[data-focus]{box-shadow:0 0 0 3px rgba(168,203,251,0.5);} Wendy Kopp
Bo stjerne thomsen.
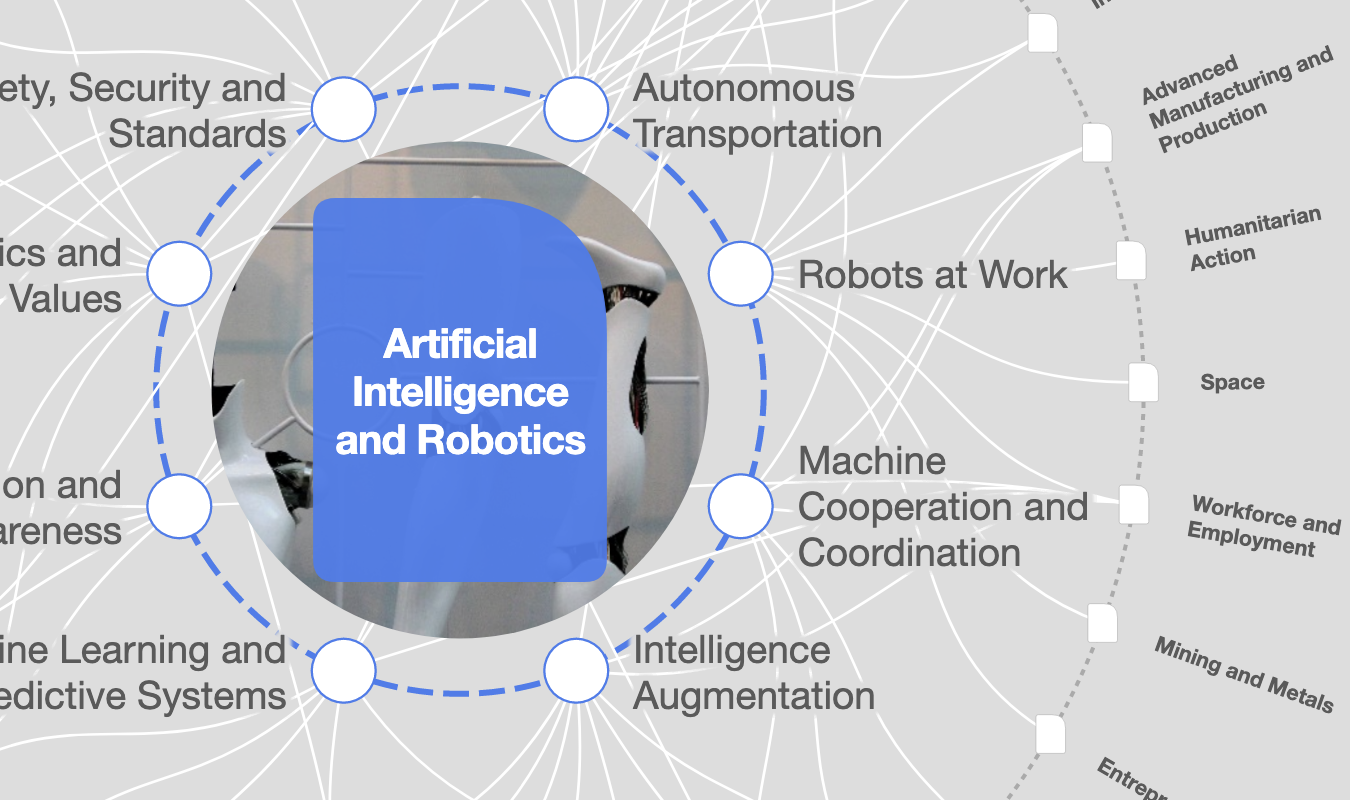
.chakra .wef-9dduvl{margin-top:16px;margin-bottom:16px;line-height:1.388;font-size:1.25rem;}@media screen and (min-width:56.5rem){.chakra .wef-9dduvl{font-size:1.125rem;}} Explore and monitor how .chakra .wef-15eoq1r{margin-top:16px;margin-bottom:16px;line-height:1.388;font-size:1.25rem;color:#F7DB5E;}@media screen and (min-width:56.5rem){.chakra .wef-15eoq1r{font-size:1.125rem;}} Artificial Intelligence is affecting economies, industries and global issues
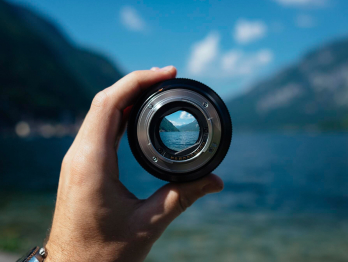
.chakra .wef-1nk5u5d{margin-top:16px;margin-bottom:16px;line-height:1.388;color:#2846F8;font-size:1.25rem;}@media screen and (min-width:56.5rem){.chakra .wef-1nk5u5d{font-size:1.125rem;}} Get involved with our crowdsourced digital platform to deliver impact at scale
Stay up to date:, society and equity.
Listen to the article
- Advances in artificial intelligence (AI) could transform education systems and make them more equitable.
- It can accelerate the long overdue transformation of education systems towards inclusive learning that will prepare young people to thrive and shape a better future.
- At the same time, teachers can use these technologies to enhance their teaching practice and professional experience.
With the rapidly accelerating integration of artificial intelligence (AI) in our work, life, and classrooms, educators all over the world are re-evaluating the purpose of education in light of these outsized implications. At Teach For All and the LEGO Foundation, we see the potential of AI to accelerate the long overdue transformation of education systems towards inclusive learning that will prepare young people to thrive and shape a better future.
At the same time, we see huge opportunities for teachers to use these technologies to enhance their own teaching practice and professional experience.
Have you read?
Our children are growing up with ai. here's what you need to know, chatgpt and cheating: 5 ways to change how students are graded, here's what americans think about generative ai like chatgpt and dall-e, the future of jobs report 2023.
Dialogue on the future of work and education has long emphasized the importance of developing skills and values that are uniquely human and less likely to be replaced by technology. The rise of ChatGPT is yet another proof point. Most students and teachers agree that ChatGPT is “just another example of why we can’t keep doing things the old way for schools in the modern world”. (Although please note that this blog was written in the "old way" as an interactive collaboration between humans, and not generated by AI.)
How AI tools can help educators and students
Our hope is that the advent of AI will spur educators, students, parents, and policy-makers to come together to consider what skills our students really need to navigate uncertainty, solve complex challenges and shape meaningful futures in a changing economy. This means embracing the challenge to provide learning that fosters agency, awareness, critical thinking and problem-solving skills, connectedness, and well-being. We already see that AI tools deployed by well-trained and well-supported teachers can be invaluable for accelerating progress towards this vision.
AI can help foster the skills students will need to navigate and shape the future. Tools like ChatGPT, as Dr. Kathy Hirsh-Pasek and Elias Blinkoff argue , can help promote students’ critical thinking when used in sophisticated ways for deeper, more engaged learning.
Vriti Saraf, CEO and founder of K20 Educators, in New York, agrees: “The less students need educators to be the main source of knowledge, the more educators can focus on developing the ability to curate, guide, critically assess learning, and help students gain skills that are so much more important than memorizing information.”
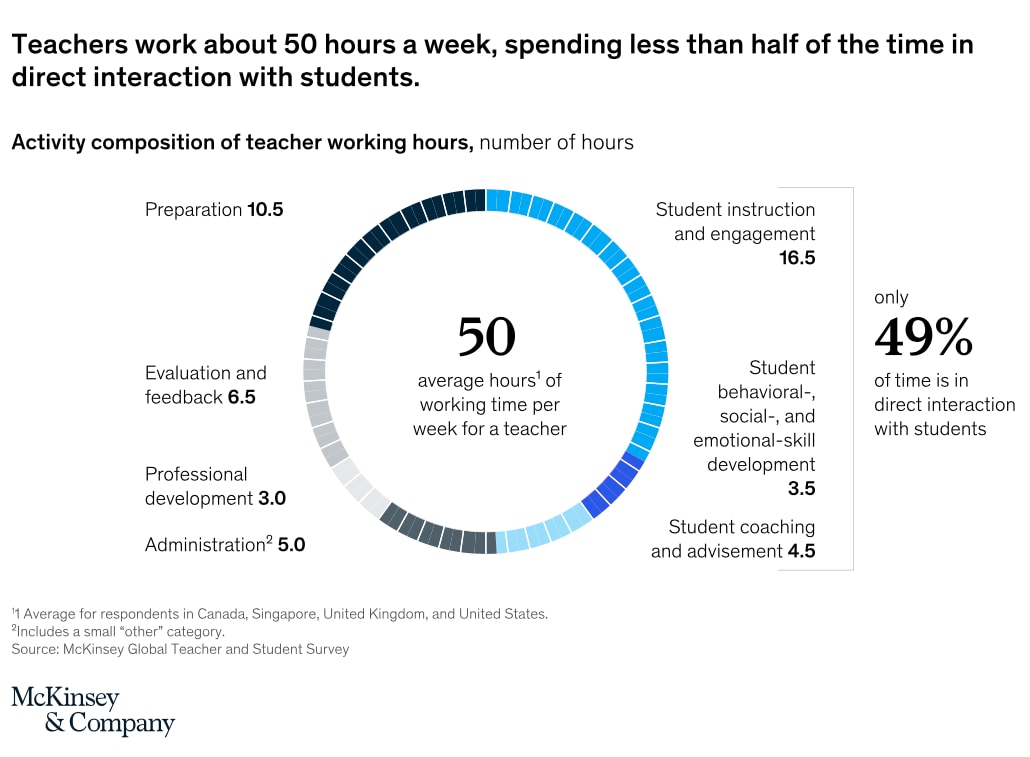
Noting the increased importance of social-emotional learning, Henry May, CEO of Co-School in Bogota, Colombia, notes that teachers have an essential role in shaping childhood experiences away from screens. So, "teachers must be trained on how to educate students on ethical principles; how to use AI tools appropriately; and how to mitigate the potential risk of AI to reduce human connection and belonging and increase loneliness."
Another potential benefit is that AI can free up time in the classroom. Teachers often cite unmanageable administrative tasks as their greatest source of exhaustion and burnout. By automating routine administrative tasks, AI could help streamline teacher workflows, giving them more time to build relationships with students and foster their learning and development.
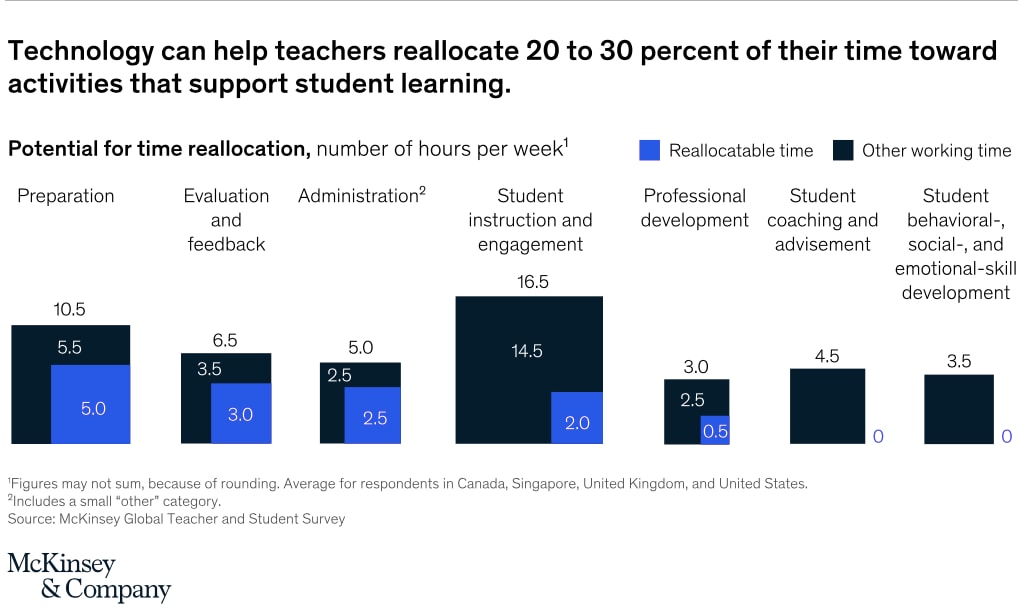
A classroom view of AI tools
Quim Sabría, a former teacher in Barcelona, Spain and co-founder of Edpuzzle, says AI could improve teacher productivity across areas like lesson planning and differentiation, grading and providing quality feedback, teacher-parent communication, and professional development.
In Lagos, Nigeria, teachers are beginning to see the efficiency and ease that AI brings to their work. Oluwaseun Kayode, who taught in Lagos and founded Schoolinka, is currently seeing an increasing number of teachers from across West Africa using AI to identify children’s literacy levels, uncover where students are struggling, and deepen personalized learning experiences.
In the US state of Illinois, a similar pattern is seen where Diego Marin , an 8th-grade math teacher, likens ChatGPT to “a personalized 1:1 tutor that is super valuable for students.”
The Top 10 Emerging Technologies of 2023 report outlined the technologies poised to positively impact society in the next few years, from health technology to AI to sustainable computing.
The World Economic Forum’s Centre for the Fourth Industrial Revolution is driving responsible technology governance, enabling industry transformation, addressing planetary health, and promoting equity and inclusion.
Learn more about our impact:
- Digital inclusion: Our EDISON Alliance is mobilizing leaders from across sectors to accelerate digital inclusion, having positively impacted the lives of 454 million people through the activation of 250 initiatives across 90 countries.
- AI in developing economies: Our Centre for the Fourth Industrial Revolution Rwanda is promoting the adoption of new technologies in the country, enabling over 4,000 daily health consultations using AI.
- Innovative healthcare: Our Medicine from the Sky initiative is using drones to deliver medicine to remote areas in India, completing over 950 successful drone flights.
- AI for agriculture: We are working with the Government of India to scale up agricultural technology in the country, helping more than 7,000 farmers monitor the health of their crops and soil using AI.
Want to know more about our centre’s impact or get involved? Contact us .
Equitable use of AI in education
We see tremendous promise for AI to spur educators around the world to reorient their energy away from routine administrative tasks towards accelerating students’ growth and learning, thus making teaching more fulfilling.
But we need to stay vigilant to ensure that AI is a force for equity and quality. We’ve all heard stories of technology being used to create shortcuts for lesson planning, and we must keep fine-tuning AI so that it does not replicate existing biases.
AI tools can be a catalyst for the transformation of our education systems but only with a commitment to a shared vision for equitable holistic education that gives all children the opportunity to thrive. To ensure AI benefits all students, including the most marginalized, we recommend being mindful of the following principles:
- Co-creation: Bring together ed-tech executives and equity-conscious educators from diverse communities to collaborate on applications of AI that reflect strong pedagogy, address local needs and cultural contexts, and overcome existing biases and inequities.
- Easy entry points: Support teachers to access and apply technologies to reduce administrative burdens and provide more personalized learning by providing open access resources and collaborative spaces to help them integrate AI into their work.
- Digital literacy: Invest in IT fundamentals and AI literacy to mitigate the growing digital divide, ensuring access to devices, bandwidth, and digital literacy development for teachers and students to overcome barriers.
- Best practice: Collect and shareinspiring examples of teachers using technologies to support student voice, curiosity, and agency for more active forms of learning to help inspire other teachers to leverage AI in these ways.
- Innovation and adaptation: Work with school leaders to support teacher professional development and foster a culture of innovation and adaptability. Recognize and reward teachers for new applications of AI and encourage peer-to-peer learning and specialized training.
Don't miss any update on this topic
Create a free account and access your personalized content collection with our latest publications and analyses.
License and Republishing
World Economic Forum articles may be republished in accordance with the Creative Commons Attribution-NonCommercial-NoDerivatives 4.0 International Public License, and in accordance with our Terms of Use.
The views expressed in this article are those of the author alone and not the World Economic Forum.
Related topics:
The agenda .chakra .wef-n7bacu{margin-top:16px;margin-bottom:16px;line-height:1.388;font-weight:400;} weekly.
A weekly update of the most important issues driving the global agenda
.chakra .wef-1dtnjt5{display:-webkit-box;display:-webkit-flex;display:-ms-flexbox;display:flex;-webkit-align-items:center;-webkit-box-align:center;-ms-flex-align:center;align-items:center;-webkit-flex-wrap:wrap;-ms-flex-wrap:wrap;flex-wrap:wrap;} More on Forum Institutional .chakra .wef-nr1rr4{display:-webkit-inline-box;display:-webkit-inline-flex;display:-ms-inline-flexbox;display:inline-flex;white-space:normal;vertical-align:middle;text-transform:uppercase;font-size:0.75rem;border-radius:0.25rem;font-weight:700;-webkit-align-items:center;-webkit-box-align:center;-ms-flex-align:center;align-items:center;line-height:1.2;-webkit-letter-spacing:1.25px;-moz-letter-spacing:1.25px;-ms-letter-spacing:1.25px;letter-spacing:1.25px;background:none;padding:0px;color:#B3B3B3;-webkit-box-decoration-break:clone;box-decoration-break:clone;-webkit-box-decoration-break:clone;}@media screen and (min-width:37.5rem){.chakra .wef-nr1rr4{font-size:0.875rem;}}@media screen and (min-width:56.5rem){.chakra .wef-nr1rr4{font-size:1rem;}} See all
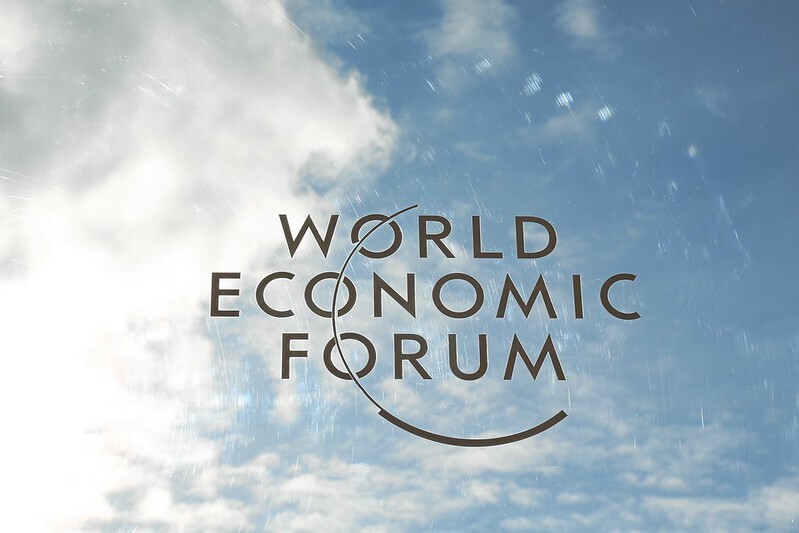
Institutional update
World Economic Forum
May 21, 2024
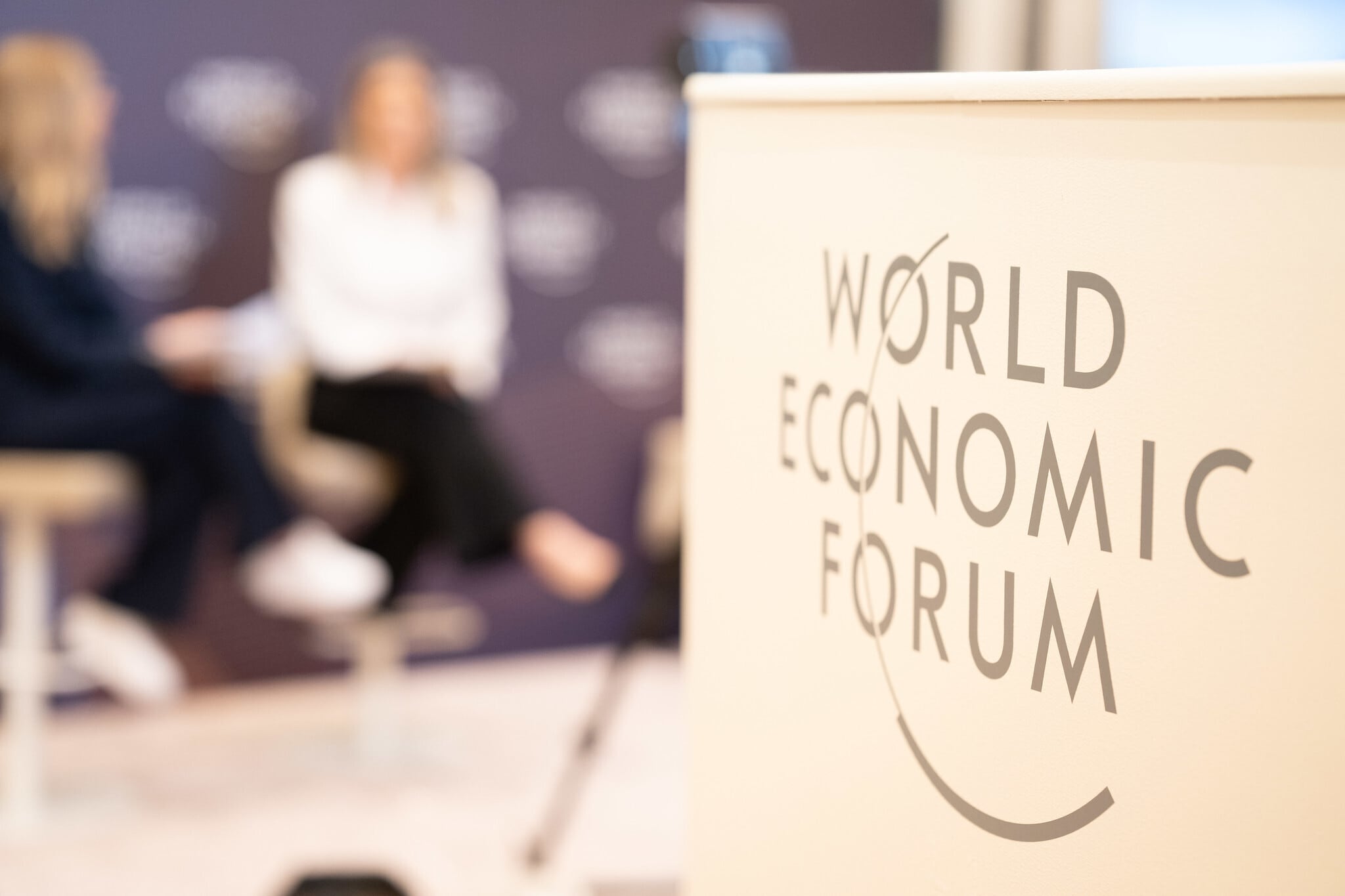
Reflections from MENA at the #SpecialMeeting24
Maroun Kairouz
May 3, 2024
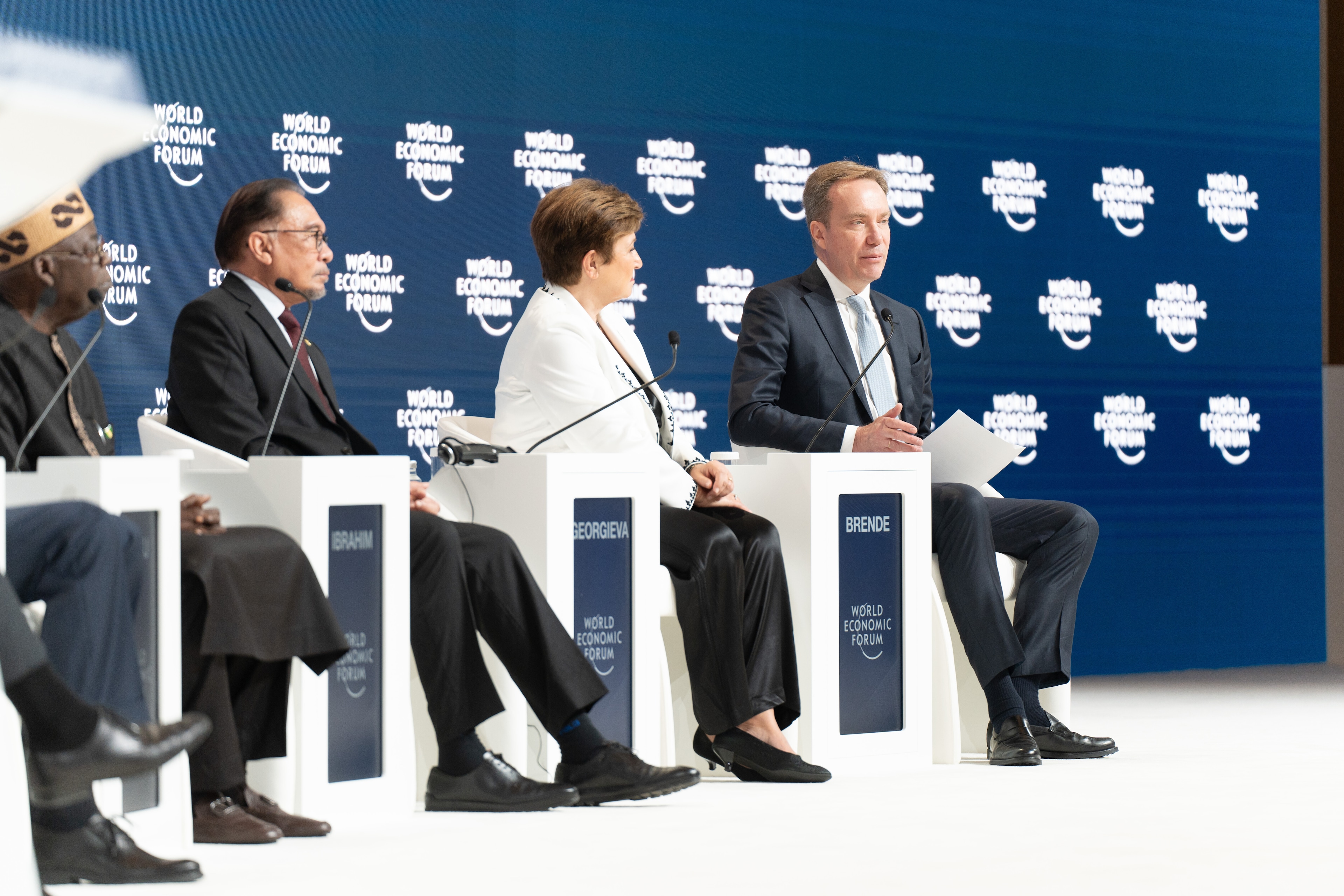
Day 2 #SpecialMeeting24: Key insights and what to know
Gayle Markovitz
April 28, 2024
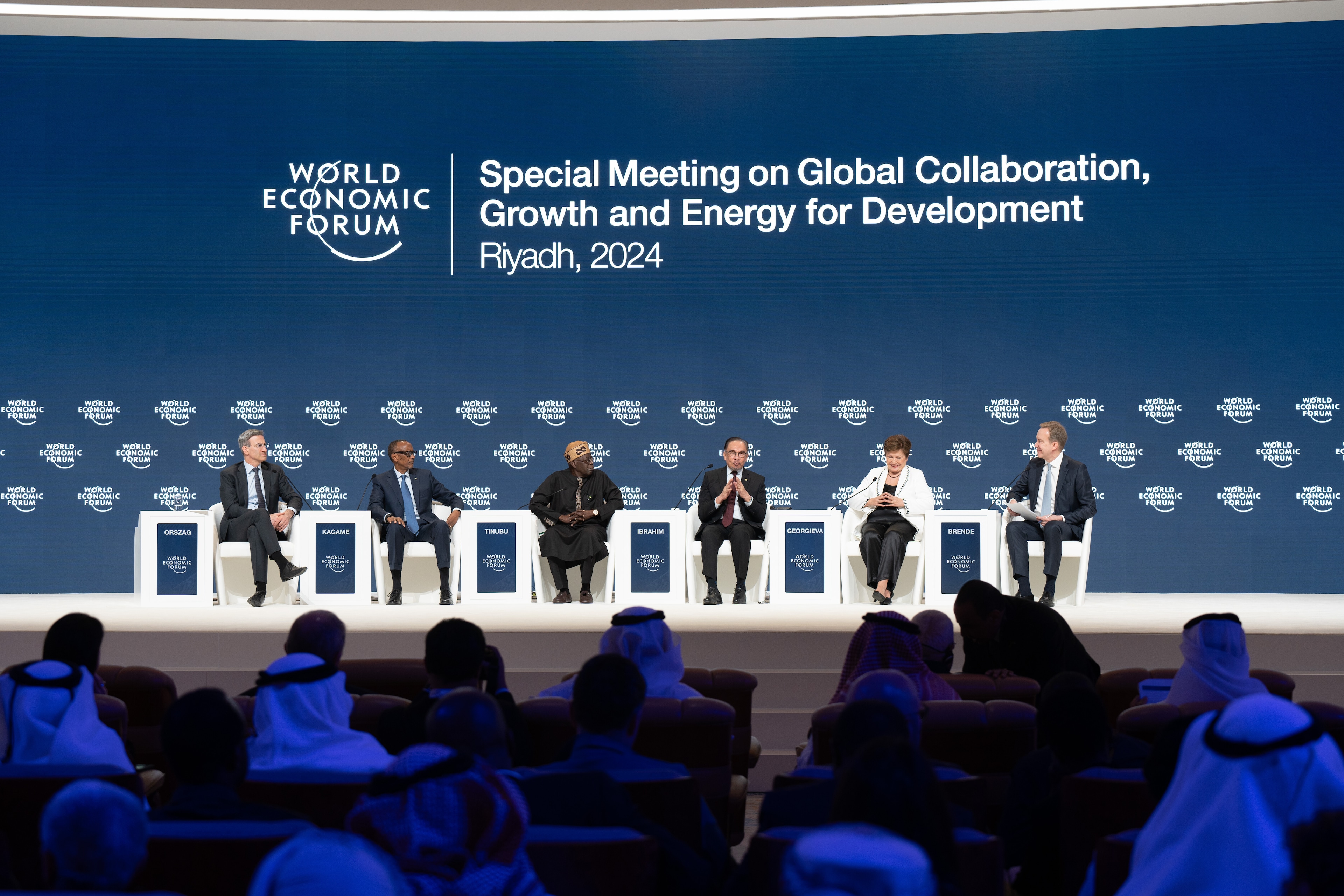
Day 1 #SpecialMeeting24: Key insights and what just happened
April 27, 2024
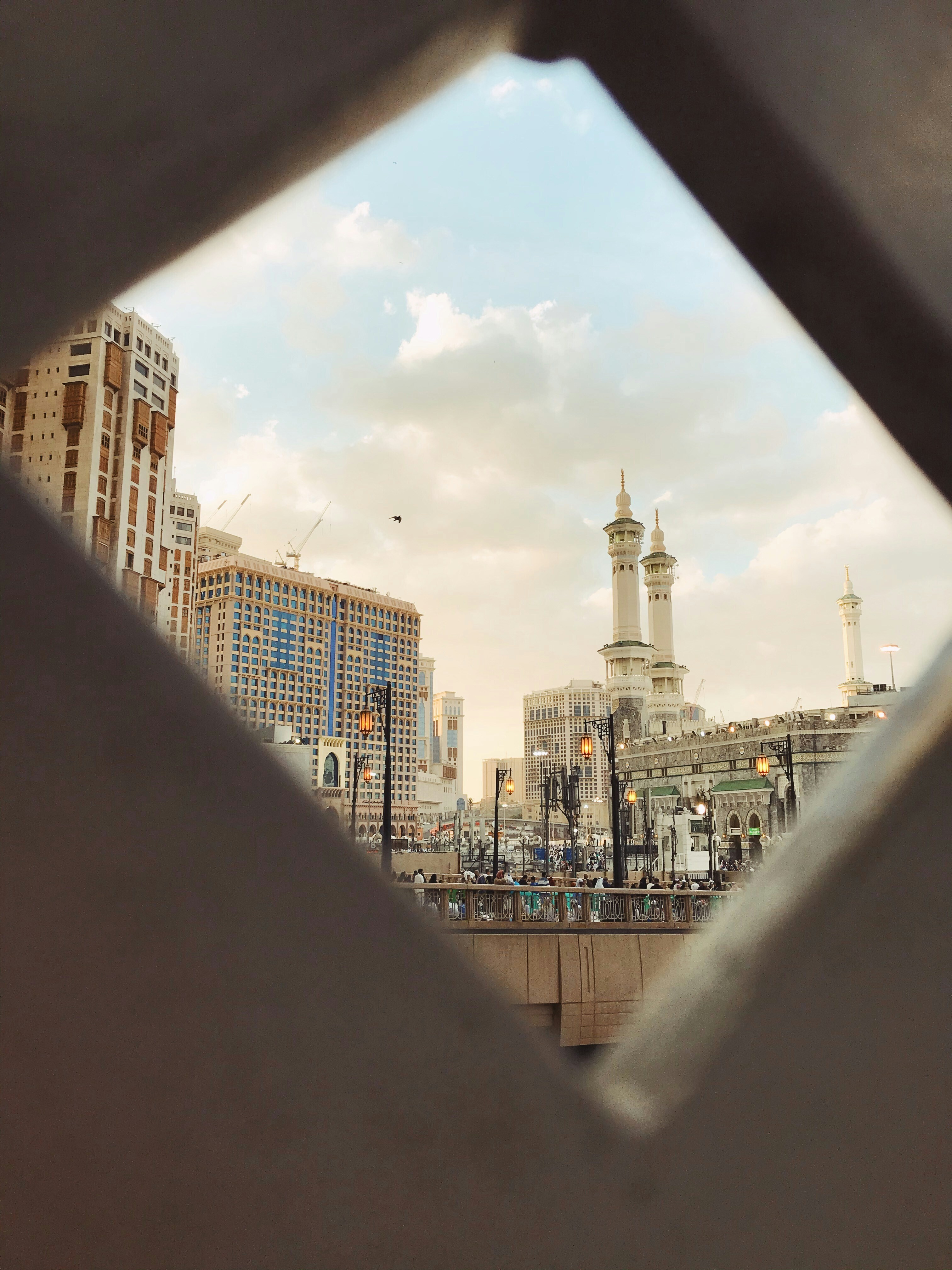
#SpecialMeeting24: What to know about the programme and who's coming
Mirek Dušek and Maroun Kairouz
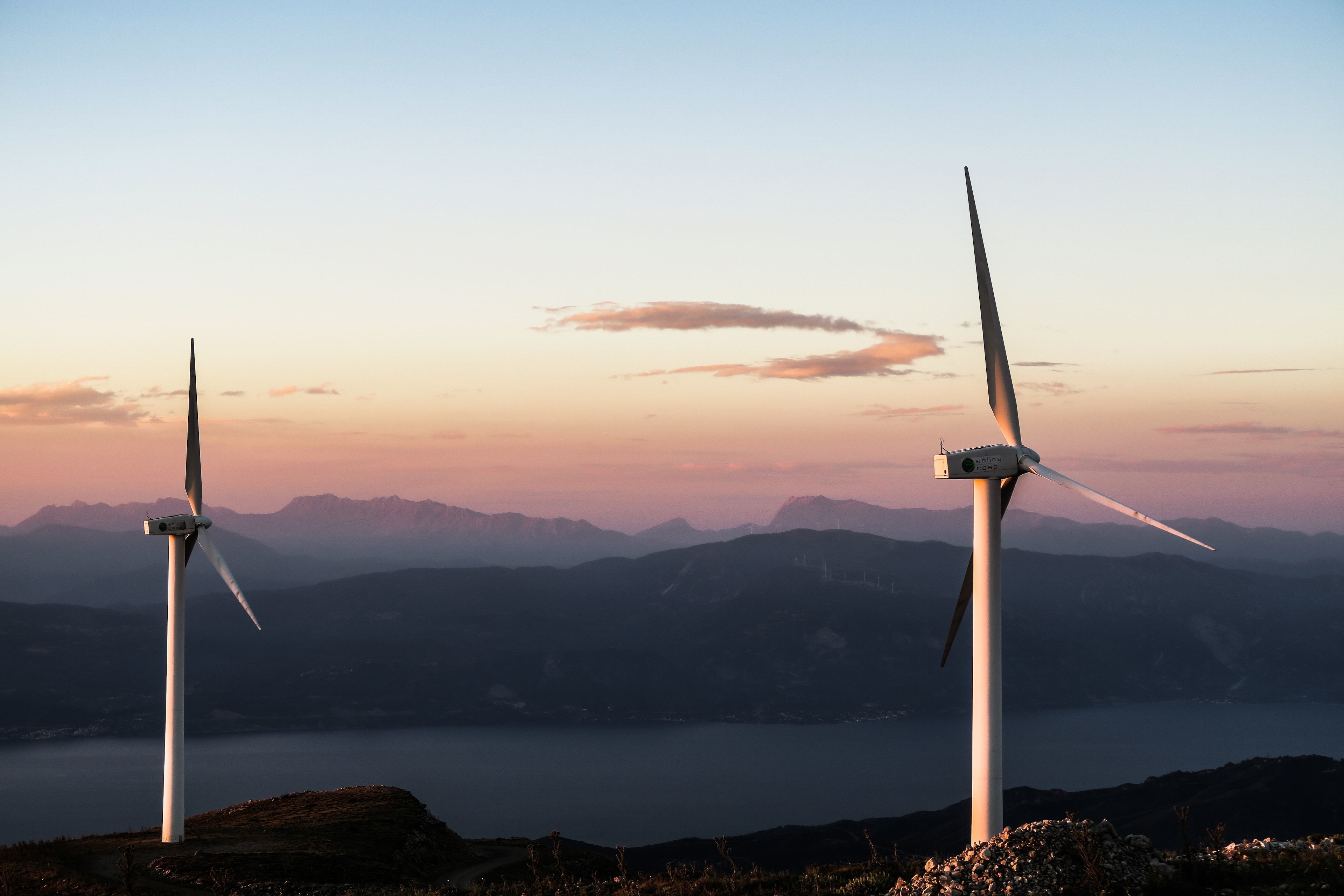
Climate finance: What are debt-for-nature swaps and how can they help countries?
Kate Whiting
April 26, 2024
- Open access
- Published: 06 February 2023
A critical evaluation, challenges, and future perspectives of using artificial intelligence and emerging technologies in smart classrooms
- Eleni Dimitriadou ORCID: orcid.org/0000-0001-9257-0999 1 , 2 &
- Andreas Lanitis 1 , 2
Smart Learning Environments volume 10 , Article number: 12 ( 2023 ) Cite this article
22k Accesses
39 Citations
4 Altmetric
Metrics details
The term "Smart Classroom" has evolved over time and nowadays reflects the technological advancements incorporated in educational spaces. The rapid advances in technology, and the need to create more efficient and creative classes that support both in-class and remote activities, have led to the integration of Artificial Intelligence and smart technologies in smart classes. In this paper we discuss the concept of Artificial Intelligence in Education and present a literature review related to smart classroom technology, with an emphasis on emerging technologies such as AI-related technologies. As part of this survey key technologies related to smart classes used for effective class management that enhance the convenience of classroom environments, the use of different types of smart teaching aids during the educational process and the use of automated performance assessment technologies are presented. Apart from discussing a variety of technological accomplishments in each of the aforementioned areas, the role of AI is discussed, allowing the readers to comprehend the importance of AI in key technologies related to smart classes. Furthermore, through a SWOT analysis, the Strengths, Weaknesses, Opportunities, and Threats of adopting AI in smart classes are presented, while the future perspectives and challenges in utilizing AI-based techniques in smart classes are discussed. This survey targets educators and AI professionals so that the former get informed about the potential, and limitations of AI in education, while the latter can get inspiration from the challenges and peculiarities of educational AI-based systems.
Introduction
The term “S.M.A.R.T” Classroom stands for Showing, Manageable, Accessible, Real-time Interactive, and Testing (Huang et al., 2019 ), and refers to a setting where the physical space is infused with carefully constructed digital tools and resources to encourage student connection on various social levels, enhance face-to-face interaction in real-time, and record the collective knowledge of the entire class (Lui & Slotta, 2014 ). A smart classroom is defined as a combination of several high-end technologies that aim to assist educators and students in optimising their overall leaning experience (Micrea et al., 2021 ). A Smart classroom combines school education and technology (Li et al., 2019 ) such as mobile technologies, automatic communication and learning tools, video projectors, cameras, sensors, facial recognition software, and other modules that keep track of a variety of environmental factors (Mircea et al., 2021 ). The role of teachers in the smart classroom is to enhance students' performance, creative and thinking skills (Palanisamy et al., 2020 ) while also using new teaching methodologies such as social learning, mobile learning, ubiquitous learning (Chen et al., 2016 ). Although a smart class combines technology with other elements, such as teaching strategies and classroom models, in this paper we focus our attention on the technological dimension of a smart class.
The introduction of Artificial Intelligence (AI) combined with emerging technologies having the form of interactive, remote, and mobile computing in physical and/ or virtual environments constitutes an evident trend in the development of the concept of smart classroom. Most of the technologies employed in a smart class rely on Artificial Intelligence (AI) that empowers the interactive, adaptive, and smart usage of those technologies during the learning process. In the work presented in this paper, a smart classroom is defined as physical or remote space which integrates emerging technologies (Have et al., 2021 ) and AI to provide an enhanced learning experience (see Fig. 1 ).
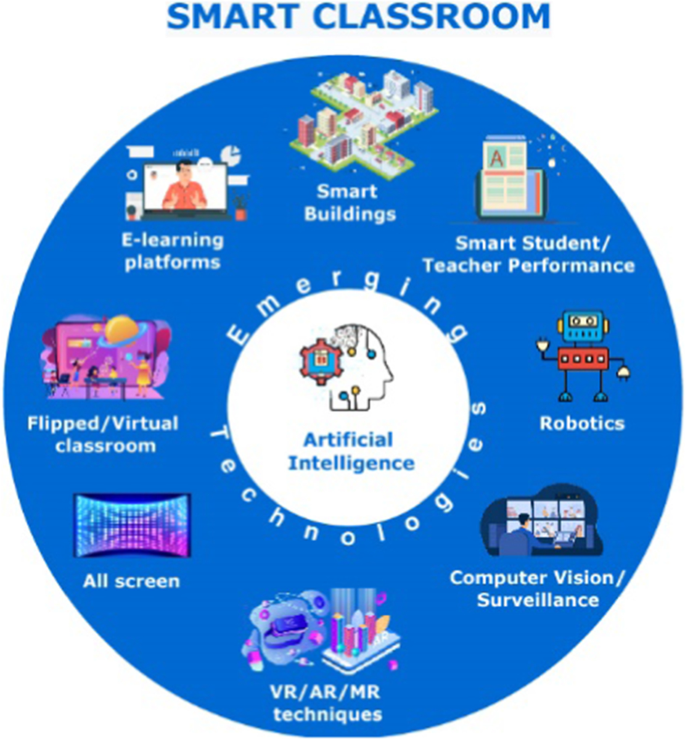
The main technologies encountered in a smart classroom
The term “ Artificial Intelligence ” (AI) was first mentioned by John McCarthyin in 1956 and refers to the ability of computer systems to undertake human tasks (like learning and thinking) that frequently can only be attained through human intelligence (Sadiku et al., 2021 ). Since the 1970s, the specific field of Artificial Intelligence in Education (AIED) has begun to influence the application of technology to instruction and learning, to improve the learning process, and promote student achievements (Southgate et al., 2019 ). The aim of AIED is to establish AI-powered systems such as virtual pedagogical agents, AI robots and intelligent systems which allow flexible, engaging and personalised learning as well as to automate daily tasks of teaching (e.g. feedback and assessment) (AlFarsi et al., 2021 ). The last few years, the topic of AI has been empowered by the groundbreaking technology of deep learning (Sejnowski, 2020 ) that allowed the successful application of AI to several complex machine learning tasks.
Several surveys related to smart classes appear in the literature. Saini and Goel ( 2019 ) focus on technologies related to smart content preparation and distribution, smart student engagement, smart assessment, and smart physical environment. For each pillar Saini and Goel ( 2019 ) provide a review of different technologies and techniques used in a smart classroom and provide recommendations for future research directions. While this survey has some similarities to our approach towards the presentation of concepts related to smart classes, in our case we focus our attention on the use of emerging technologies in conjunction with artificial intelligence in smart classes. Furthermore, when compared to the article by Saini and Goel ( 2019 ), a wider range of smart technologies are presented. Chen et al. ( 2020 ) focus their attention on the use of AI in education. They state that ΑΙ has been extensively used in education in different forms such as computer programs, humanoid robots, web-based chatbots, and online platforms. Despite the wide range of technologies presented by Chen et al. ( 2020 ), this survey also includes an extended range of educational technologies coupled with a comprehensive discussion of the advantages and disadvantages of AI in education, that also includes a SWOT analysis. Chen et al. ( 2022 ) indicate the usefulness of AI in education, which may be used in the form of intelligent tutoring systems for special education, natural language processing, educational robots, performance prediction, discourse analysis, teaching evaluation, learner emotion detection and personalized learning. Their survey is mainly focused on presenting statistical figures related to the use of AI in education, such as the size of research community per subject, and the most frequent AI terms in literature. More recently, Dimitriadou and Lanitis ( 2022a ) presented a short survey on the topic of the use of AI in smart classes. When compared to this work the current paper provides a more extensive coverage of several key technologies related to smart classes, and in addition it provides a comprehensive discussion of advantages and limitations of using AI in smart classes.
In relation to previous surveys reported in the literature, the main contributions of this survey include the review of the latest technologies and discussion of future directions that could support the creation of a next-generation smart classroom, and the understanding of the use of AI in connection to the technologies used in a smart classroom, allowing the readers to get acquainted with the potential of using AI in smart classes, and the main advantages, disadvantages and potential dangers of using this technology. In the remainder of the survey, we present a literature review for studies related to smart classes and AI in education, and present key smart class technologies related to classroom management, teaching aids, and performance assessment. In Sect. " Impact of smart classroom ", we describe the advantages, disadvantages of key technologies related to smart classes. In Sect. " Discussion-future directions ", we elaborate on the role of AI in smart classes, followed by a discussion and possible future research directions.
Key technologies related to smart classes
In this section the key technologies related to smart classes are presented while emphasis is given to the role of AI in the technologies described. The main topics presented are separated in three main categories that refer to class management technologies, teaching aids and performance assessment technologies (see Table 1 ).
Classroom management
The term classroom management refers to the way or approach that a teacher uses to control / manage his / her classroom. Within this scope the management aims to maintain a comfortable and safe teaching environment that contributes to the efficient class delivery. In relation to class management technologies, in this survey we focus on the issues of Computer Vision-based surveillance/security and Smart Environment.
Computer vision-based surveillance
Computer Vision techniques in smart classroom are often used for the tasks of Attendance Recognition or Action (Behaviours) Recognition.
Attendance recognition
In an attempt to reduce the time need for keeping student attendance records, the process of attendance recognition is often automated based on facial identification technologies (Kawaguchi et al. 2005 ). For example Chowdhury et al ( 2020 ), use a suggested a Convolutional Neural networks (CNN’s) based facial identification system to identify students in a real-time video stream captured by a static camera. Several variations to this technique involve systems that can utilize images captured by moving cameras (Mery et al., 2019 ), systems that utilize cameras fixed in the entrance of a class (Chintalapati & Raghunadh, 2013 ), or systems that utilize rotating cameras (Gupta et al., 2018 ).
Action (behaviour) recognition
Human action recognition is a vision-based technique that can identify a complete action performed by a human in a video sequence (Kong & Fu, 2022 ). The ability to recognize human behavior can be extremely important inside a smart classroom (Wang, 2021 ) as it enables the recognition of student behavior and emotions allowing the detection of uncomfortable situations for students, such as high anxiety or reduced concertation levels. Smart classrooms with developed AI-powered surveillance system can detect students who are not paying attention in class and alert the teacher (Parambil et al., 2022 ). In addition, action recognition systems can analyse students’ behaviour during the course and estimate their engagement (Thomas & Jayagopi, 2017 ). Furthermore, automated action recognition also helps students with special needs by monitoring them and warning teachers for potential episodes, for example in case they have an epilepsy episode (Lau et al., 2014 ).
Recently, automated methods for the behaviour analysis of the students and their engagement estimation are widely utilized in a classroom. Ngoc Anh et al. ( 2019 ), presented a system to monitor the behaviour of students in the classroom. Similarly, Thomas and Jayagopi ( 2017 ), used a machine learning algorithm to analyze the students’ engagement in a classroom by analyzing students’ head position, eye gaze direction and facial expressions. Furthermore, Yang and Chen ( 2011 ), presented an automatic smart class system which was focused on eye and face detection to determine if the students were active or not.
Previous studies related to recognizing students’ actions in smart classroom include the work of Li et al. ( 2019 ) who proposed a new spontaneous actions database that show 15 different student actions. The smart classroom, in this study, included round-tables for students and four cameras, which were fixed on the wall (front and back of classroom), to record the students’ actions from various viewpoints. Recently, Dimitriadou and Lanitis ( 2022a , 2022b ) proposed an action recognition system that recognizes seven actions performed by students attending online courses, which are recognized using CNN architectures. In this case both the images captured and the action recognition process is performed on the personal computer of each student, allowing in that way the application of this method to remote teaching activities.
Ashwin and Guddeti ( 2020 ), demonstrated a Hybrid Convolutional Neural Network to analyze students’ body postures, gestures and facial expressions to investigate engagement. Three states of student’s engagement were examined: boredom, engaged and neutral. Rashmi et al. ( 2021 ), proposed an automatic system, that monitors the students’ activities, such as the actions of sleeping, eating, using phone, discussion and being engage. The aim of this study is to localize and recognize multiple actions of the students in an image frame.
Similar technologies can be used for recognizing student actions in school yards. For example, suspicious actions, such as student fights, drug delivery, bullying incidents could be detected automatically allowing the prevention of mental and physical health injuries of students. Ye et al. ( 2018 ) suggested a strategy to identify occurrences of abuse in the environment of school utilizing motion and audio sensors to evaluate activities and verbal expression. Gutierrez and Troyer ( 2014 ) describe a simulator named SimBully to illustrate the impact of public belief and attitudes on abuse occurrences by classmates. Ali et al. ( 2020 ) use the YOLOv3 network to recognize student behaviours such as calling, napping, or reading a book indoors or outdoors with the goal to discover any undesirable behaviours.
- Smart environment
Smart buildings are described as whole structures that use existing technology resources and AI to produce a secure, functioning and friendly setting that utilizes resources wisely and economically (Dryjanski et al., 2020 ). Sensor technology (Abbasy & Quesada, 2017 ), the Internet of things (IoT) (Abdel-Basset et al., 2019 ), external telecommunications and smartphone software technology are commonly used to power advanced technologies in smart classrooms (Wu et al., 2020 ).
AI plays an important role in the creation and implementation of computer applications in order to successfully manage the administrative issues of a school and assist workers in their everyday tasks. Data collected instantly from sensors and cameras can be utilized for the surveillance of students and in collaboration with AI create a safer environment for students. The use of AI in smart environments and smart classes can increase efficiency and result in high performance of students and teachers as well (Augusto et al., 2009 ).
Teaching aids
In a modern smart-classroom, the teaching process is assisted by a plethora of technological means, to maximize the engagement and interaction of students. In this section we provide an overview of these technologies.
Educational Robots can be ‘Real’ devices (Shiomi et al., 2015 ; Weibel et al., 2020 ), or they can be software agents in the form of chatbots (Kollia & Siolas, 2016 ; Pereira, 2016 ).
‘Real’ robots
A real robot is a device that can perform actions usually undertaken by humans. Since the introduction of the first robot back in 1980 (Johal et al., 2018 ) several educational animal-like or human-like robots have been presented to suit different levels of education. Educational robots constitute a subgroup of educational technology, as they are employed to make learning easier, enhance the educational performance of students (Mubin et al., 2013 ) and assist the students in their active participation in the process of problem solving. The main driving force in introducing robots in the learning process is for creating systems that offer more social interaction and support learning (Timms, 2016 ). Examples of educational robots used over the years are summarized in Table 2 .
Initially, robots were constructed to perform repetitive tasks, without any AI. However, the importance of having intelligent machines that may perform advanced tasks eventually led to the use of a series of sensors that provide information about the environment along with the integration of AI for processing and making decisions based on the information received by the sensors (Brady et al., 2012 ; West, 2018 ). Typical sensors used in robotics include microphones, Time-of-flight (ToF) optic sensors and motion detectors (Ben-Ari & Mondada, 2018 ) used in conjunction with AI algorithms for sensing an environment (Poppinga & Laue, 2019 ). In addition, ToF cameras on robots can be enhanced by utilizing CCD cameras, and infrared depth cameras. All of the data received by sensors is usually used to train neural network models and educate robots to execute all of their duties, from comprehending a user to effectively reacting (Vega & Cañas, 2019 ). AI-based functionality incorporated in robots include speech recognition, motion control, computer vision, natural language processing, smart agent technology, movement control, and control for grasping objects.
Students accept and form relationships with robots far more effortlessly because of their interaction, which has been shown to improve psychosocial and physical development (Feil-Seifer & Matarić, 2009 ), as well as their capacity to interact, which improves the process of learning, makes it more exciting and help learners acquire more knowledge (Han et al., 2005 ). Social robots, specifically, have been successful in assisting kids with autism in comprehending concepts such as boundaries between individuals and emotional intimacy and in improving independent learning skills (Woo et al., 2021 ). Robots can become familiar with the personal needs of each student and respond accordingly (Jones & Castellano, 2018 ). Another important feature of robots is their ability to record students’ expressions and mood changes. Robots not only assist students during their courses, but they are also in advance evaluating their behavior and any emotional disturbances that may suggest despair or stress (Werner-Seidler, 2017 ). For example, Researchers at MIT have developed a robot called "Teacher bot" that can detect and respond to student emotions to provide personalized feedback and support (Bourguet et al., 2020 ).
While educational robotics can be extremely useful within a class environment, their use can also extend to teaching activities for students who cannot be physically present be in class supporting in that way remote teaching activities. For example, children that are obliged to stay home or being treated at hospitals may face serious consequences regarding their social development. Thus, missing out long periods of school and social interactions with their peers, due to factors that are beyond the control of children, may result to social isolation and feeling lonely (Helms et al., 2016 ). To combat the above negative situation, robots may ensure that no classes and time with friends are missed, by enabling children have a continuous connection with their teacher and peers (Soares et al., 2017 ).”
The notion chatbot is a combination of two words: “chat” demonstrating conversation and “bot” standing for robot (Chocarro et al., 2021 ). Chatbots simulate conversations with human users via the use of instant messaging services. Chatbots, demonstrate high potential as a learning teaching tool for remote students and can offer personal assistance, educational content support (Colace et al., 2018 ), while they can be used as tutors accompanying the process of learning (Chocarro et al., 2021 ). Examples of educational chatbots used over the years are summarized in Table 3 .
The operation of chatbots is a mix of artificial intelligence and Natural Language Processing (NLP). Natural Language Processing is a branch of artificial intelligence concerned with computers' capacity to grasp written and auditory speech in the same way that humans do (Chowdhury et al., 2003 ). NLP is consisted of three primary elements strongly correlated with AI, speech recognition and speech generation. All three topics are based on AI approaches, like GAN deep neural networks, in order to enhance the quality of the generated speech (Hsu et al., 2019 ) and reasoning, that helps bots make predictions and draw conclusions with the aim to respond appropriately in every interaction with a human. Voice interactive interfaces arose primarily as a result of breakthroughs in computer and speech recognition technology (Guttormsen et al., 2011 ). For example the Amazon Echo is an instance of a voice-interaction-based technology (Teja, 2020 ). It uses DNN to process any given dataset and translate any language for example and Recurrent Neural Networks (RNN) as a controller.
Virtual/augmented/mixed reality
Smart classrooms often incorporate virtual, augmented, and mixed reality as a means of introducing immersive learning experiences. Virtual Reality (VR) concerns the 3D simulation of an imaginary or real environment, that the user can visualize, explore and interact with it (Górski et al., 2016 ). On the other hand, Augmented Reality (AR) offers an interactive experience to users, by adding virtual information to the physical environment of the students and enabling them to use their whole body as a means of interacting with both virtual and real content (Billinghurst et al., 2015 ). Mixed Reality (MR) refers to a blending of real-world and virtual/digital world objects which are visualized together on only one display in a coherent space (Kasapakis et al., 2019 ) (see Fig. 2 ).
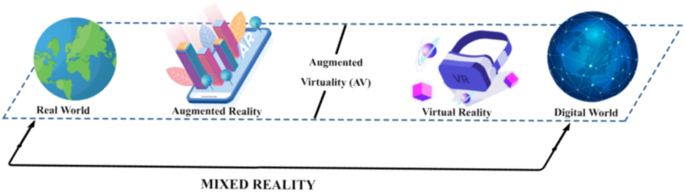
The differences between virtual, augmented and mixed reality
The last few years, the idea of metaverse has risen and is expected to be part of our reality the next few decades. The concept of the metaverse has been around since the 1990s and has gained renewed attention in recent years due to the increasing prevalence of virtual and augmented reality technologies. Metaverse is a parallel digital universe which allows multiple users to emerge into environments that combine both the physical and digital world (Mystakides et al., 2022 ). Metaverse employs technologies like virtual reality, augmented reality and blockchain to achieve the immersion, as these technologies can achieve multisensory interactions. It provides genuine, physical user interaction and complex interrelationships with virtual objects. In education, Metaverse is not a new concept as several researchers and educators have discussed its implications for learning. Metaverse can be the online space where individuals can meet and socially interact for educational and not only, reasons. So, in the field of education, the metaverse has the potential to revolutionize the way that students learn and interact with educational content. By using virtual reality and other immersive technologies, students can experience educational content in a more interactive and engaging way, which can help to improve retention and understanding. Although, there are some challenges concerning the use of Metaverse in education. Some of them that we are addressing here are data privacy, technology implementation, efficiency, cost, lack of standardization and addiction.
Virtual reality in education
VR has been used as an educational tool for numerous subjects (see Table 4 ). Sobota et al. ( 2017 ), states that the two techniques widely used to offer immersive and semi-immersive experience regarding virtual reality in smart classroom are: (a) CAVE (Cave automatic virtual environment) and HMDs (Head-mounted displays) and (b) Interactive school desk. CAVE constitutes a room sized pace including several projection walls where the user is able to move freely in the space and experience their body in immediate interaction with virtual scene and HMDs are suitable devices that offer virtual environment to one user every time. Furthermore, there are different VR accessories which can combine with HMDs and CAVEs, such as gloves, suits or controllers which can offer more exciting experience.
The application of VR in education changed some of the previous teaching ideas, but also some of the already existing teaching models (Chen & Tsai, 2012 ; Gu, 2017 ). Several studies concluded that VR technologies are more likely to influence the motivation and academic performance of students in a positive way (Ibáñez et al., 2014 ; Martín-Gutiérrez et al., 2017 ). Furthermore, Hampel and Dancsházy ( 2014 ) argues that the creation of an environment of virtual learning is quite helpful for students, since students are able to acquire knowledge by themselves. Additionally, there is evidence that VR technologies enhance students’ collaborative and communicative skills along with their cognitive and psychomotor skills (Kaufmann & Schmalstieg, 2002 ; Martín-Gutiérrez et al., 2017 ; Zhou et al., 2008 ) whereas VR technologies can be used for the training of the educators as well (Stavroulia et al., 2016 ).
Augmented reality in education
There are different types of augmented display devices in a smart class that include tablets, smartphones, smartboards and different software which enables the creation of augmented scenarios such as Aurasma, Footnote 1 Layar, Footnote 2 Augment Footnote 3 and Aumentaty Footnote 4 (Chamba-Eras & Aguilar, 2017 ). Oculus Quest, Microsoft HoloLens and Windows Mixed Reality are AR headsets/glasses utilized as augmented display devices. According to Torres et al. ( 2011 ), AR in smart classrooms can be used in the following forms: Enlarged book, Virtual models of specific complicated structures, Educational games for the classroom, Virtual models which produce sounds, Magic eyeglasses, Magic mirrors, Magic doors and windows, Navigation support and Cooperative space. Chamba-Eras and Aguilar ( 2017 ) state that AR is recommended to compensate various deficiencies that might occur in a smart classroom such as difficulties in doing complicated and dangerous experiments, carrying out actual experiments due to equipment costs, and unavailability of appropriate facilities. Furthermore, the study from Stanford University has shown that students who learn with AR technology demonstrate greater knowledge retention and improved problem-solving skills (Queiroz et al., 2022 ). The importance of Augmented Reality technology in education has led to its implementation in various topics as shown in Table 5 :
Mixed reality in education
MR applications in real and smart classroom have many benefits from the perspective of the students in learning and the procedure of obtaining knowledge or skills. According to Dascalu et al. ( 2014 ), some benefits by MR to educational uses are: (a) students remain focused on the task at-hand, (b) it is fostered the affective side of learning, (c) computer-based learning gets more human-oriented, and (d) students’ interest and motivation towards learning is enhanced. Furthermore, MR offers immersive and engaging experiences via creative problem solving. MR worlds achieve high levels of immersion through Head Mounted Displays (HDMs), such as Microsoft HoloLens, HTC Vive, Oculus Rift and Magic Leap One, or AjnaLens. Different educational tools were developed to enhance the efficiency of teaching-process such as Virtual Toolkit (Mateu et al., 2015 ), SMALLable (Tolentino et al., 2009 ), TIWE Linguistico (Fiore et al., 2014 ) and Robostage (Chang et al., 2010 ).
The role of AI for VR, AR and MR
The integration of AI in VR/AR applications has the potential to improve its effectiveness, enabling programmers to develop more engaging and fascinating applications (Kaviyaraj & Uma, 2021 ). The key areas where AI is used in conjunction with VR/AR/MR include the generation of 3D assets, Interaction, Reasoning, Visualization. In the case of AR and MR computer vision capabilities such as pose estimation, object detection, scene labeling and semantic segmentation are used to control content, project an object in the scene, and trigger a spot or occlude objects from the scene (Sahu et al., 2021 ). Furthermore, AI-based techniques are utilized to generate avatars, digital humanoid characters or complementing users that interact and take decisions immediately according to the gamers' choices, resulting in more engaging experiences (El Beheiry et al., 2019 ).
E-learning platforms
Beetham and Sharpe ( 2013 ) state that E-learning platforms (see Table 6 ) are on-line systems that aim to support synchronous, asynchronous or hybrid learning activities. Within this context, synchronous learning is done in real time, asynchronous learning, is done at a convenient time for the student (Potode & Manjare, 2015 ), and hybrid learning constitutes of a combination of synchronous and asynchronous learning activities.
It has been demonstrated that kids who spend a lot of time on e-learning platforms are more engaged and obtain greater grades because they modify their perception of their schoolwork (Benta et al., 2015 ). Students seem to be more activated by the way these platforms work and managed to do the assignments they had and create a sense of responsibility to their submissions, as well as to complete some difficult activities (Benta et al., 2014 ). Students also claimed that this type of environment intrigued them to participate in extra lectures and seminars.
Quite often AI is introduced in E-learning platforms in order to maximize the learning experience of students through the use of adaptive educational systems. The latter can adapt to individual needs and offer support that is tailored to each student, aiming to help students meet their individual goals in the best possible way that fits their personalities and characteristics (Colchester et al., 2017 ). To this end, adaptive educational systems use the learner profile to diagnose individual characteristics and abilities, the taught model to present the learning material and the instructional model to formulate the content in a dynamic and adaptive way. Recently, a new AI-based e-learning platform called "Edu4AI" has been developed to personalize the curriculum for each student based on their learning style, ability and progress (Geramani et al., 2022 ). The efficiency of adaptive educational systems proves the capability of AI to assist leaning in multiple ways (Durlach & Lesgold, 2012 ). Apart from supporting adaptive learning AI is also utilized in other aspects of the learning process. For example, natural language processing algorithms (Chowdhary, 2020 ) are often used to identify plagiarism and avoid transcribing in assignments submitted by students (Chong et al., 2010 ). Furthermore, E-learning platforms are used in different classroom models such as Flipped classroom and Virtual classroom.
Flipped classroom is an innovative educational method where tasks usually performed in the classroom, like presenting the lecture, are conducted at home, while homework is discussed and performed in class (Akçayır et al., 2018 ) whereas a Virtual classroom is defined as a new educational environment that allows students to attend courses online while also facilitating interaction and collaboration by using the artificial intelligence tools and abilities the platform offers (Rufai et al., 2015 ). Lo et al. ( 2017 ) believe that AI has great potential in the flipped classroom approach, because it may enable the personalization and adaptation of the learning process to the students’ needs. Shan and Liu ( 2021 ) suggest a model of Hybrid Teaching of Artificial Intelligence and Flipped Classroom, which combines big data, cloud and online applications to implement comprehensive and individualized learning.
All Screen refers to the ability to project multimedia including audio, photographs, and movies on many screens such as TVs and smartphones. Screen mirroring allows users to access and view the same image or video in two or more screens (Brudy et al., 2019 ). Screen mirroring is valuable since it might improve the connectivity among a cellphone and a different device, such as smart TVs (Ouyang & Zhou, 2019 ). Thus, touch gesture input, with virtual buttons on the screen, is a frequent technique for interacting with screens (Ouyang et al., 2021 ). There are several advantages that all screens may provide to a smart class. To begin with, only wireless screen mirroring makes the connection between instructors' and students' devices straightforward and reliable (Ellern & Buchanan, 2018 ). Furthermore, practical issues related to the use of projectors, such as connectivity issues, requirement for low-light conditions, and projector noise, are mitigated through the adoption of all screen technology (Sahlström et al., 2019 ).
Performance assessment
Student and educator performance assessment and feedback is a highly important education task. Although traditionally performance assessment/prediction has been a quite complex and time-question process, it has been extremely facilitated through automated assessment in a smart classroom (Balfour, 2013 ).
Smart student performance assessment/prediction
Student performance assessment aims on the one hand to inform the teacher about the degree to which students have learnt the content of the lesson and how well they are expected to perform in the future, and on the other, to grade students and provide feedback to them about their performance during the learning process (Saini & Goel, 2019 ). Traditionally, performance assessment was carried out in a paper or oral format. However, the aforementioned method has many disadvantages, since it is a time-consuming and tiring process, while it results to piles of wasted paper and writing material (Vimal & Kumbharana, 2016 ).
In a smart classroom dedicated tools can facilitate the performance assessment/prediction through the automation of the assessment. The easiest tool to assess students in a smart classroom is the employment of multiple-choice questions, which allow automated evaluation and feedback, with the aid of an online web server that compares students’ answers with the configured correct answer (Balfour, 2013 ). An important application of AI in student assessment is plagiarism checking, with Turnitin as a frequently used tool (Ahmed, 2015 ). Bhatia and Kaur ( 2021 ) add an innovative performance assessment/prediction tool based on quantum game theoretic (QGT) decision making. This tool incorporates IoT to gather information and data about students, which are evaluated over a computing platform, aiming to analyze performance and determine the academic enhancement of students. The above methods give the ability to teachers to offer continuous assessment to students, avoiding all the tedious work, while students receive feedback constantly.
Numerous machine learning techniques have been proposed for predicting student performance. Amra and Maghari ( 2017 ) propose a system giving predictions regarding the future performance of secondary students based on several attributes. They compared two distinct machine learning algorithms: K-Nearest Neighbors (KNN) and the Naïve Bayes classifier by feeding them with educational data set of secondary schools, collected from the ministry of education in Gaza Strip. Waheed et al. ( 2020 ), propose a system to predict the students’ academic performance in a virtual learning environment. Their system used artificial neural networks to classify students in two classes: failure and success, receiving as input data of the assessment performance of 32,593 students provided from an open dataset. Authors made a comparison of the results using baseline methods: logistic regression, support vector machines and Artificial Neural Networks (ANN). The ANN method had the best performance out of the tested models. Warschauer and Grimes ( 2008 ), propose the automatic assessment of writing essay assignments with the use of artificial intelligence, using interview data and observations notes as inputs. Students and teachers had positive approach (i.e., student motivation rising, proposing autonomous student activity, constituting a saver of time for teachers).
Educator performance assessment
Traditional assessment methods are usually based on the observation of teachers from experts during course time something that can be expensive, not accurate and usually the feedback provided is infrequent and is related to the performance and not on how teachers can enhance their techniques (Archer et al., 2016 ). To overcome this crucial impediment in teacher development, new technologies are used to produce high quality and meaningful, and continuous automatic feedback for the educators.
Bhatia and Kaur ( 2021 ) use IoT systems are used in classes to collect information regarding students and educators to identify their progress. For this purpose, they utilize a Bayesian model and the collected data are assessed through a fog-cloud computing device over time for both students’ and educators’ performance. Jensen et al. ( 2020 ) devised a method for teachers to effortlessly audiotape the conversations and lectures in a classroom. They utilized voice recognition and Machine Learning (ML) algorithms to provide generalized estimations of essential aspects of educator speech. Therefore, they state that actual instructor conversation can be captured and evaluated for automated feedback. Jensen et al. ( 2021 ) address the issue of designing a framework for automatic educator feedback, that necessitates several considerations about data harvesting processes, automatic assessment and the way feedback is displayed. For this purpose, they employ machine learning techniques, such as Random Forest classifiers, and use transfer learning techniques from BERT algorithm for NLP.
Impact of smart classroom
In this section, based on information derived from the literature, in combination with critical assessment, the impact of smart classroom on the learning process is analyzed while disadvantages of using key technologies related to smart classes and the impact of AI are also discussed.
The integration of AI systems that process data collected by Internet of Things (IoT) and other sensors, can help monitor the circumstances of the classroom, offering a safe and eco-friendly environment while they can also monitor students and inform teachers in the case of a student misconduct or potential accidents. AI algorithms can be used to optimize lighting and temperature in classrooms based on occupancy, ambient temperature, and other factors. All these tools and systems can contribute to the establishment of a better and safer learning environment. Furthermore, the interaction with the learning material offered by key technologies related to smart classes can help boost students’ learning, information may be retained more easily and self-efficiency may increase (León et al., 2016 ). The enhanced interactively offered in smart classes help students have an active role in the class delivery process rather than having a passive role that causes loss of concentration and interest. However, the use of technology for teaching and learning may be related to disconnectedness, which is usually expressed as feelings of separation from learning, the curriculum, the peers and the teachers and the learning devices. Disconnection of students may jeopardize the learning outcomes of students because it results in disengagement, decreased student ownership and absence of student agency. For this reason, teachers in smart classrooms should find ways to remove the barriers to meaningful student involvement and encourage their engagement with the school and the learning process (Blessinger & Wankel, 2013 ).
Lin et al. ( 2019 ) suggest that an adjustable smart system can assist students, improve the learning process and foster a considerable quantity of intellectual learners. Smart classrooms and emerging technologies may overcome the problems related to the provision of timely and individualized support to students, using smart applications that respond to students and provide automated feedback immediately, by comparing current and previous student performance and by motivating students (Vimal & Kumbharana, 2016 ). In addition, Smart classes support the provision of synchronous and asynchronous education while they support both teacher-led and student-centered activities (Beetham & Sharpe, 2013 ). Moreover, the above combination allows to enrich learning with the provision of extra material, improve and retain knowledge through the students’ longer interaction with the learning subject, the teachers and their peers. At the same time, the style of the traditional classroom that is offered on scheduled dates and time is retained, resulting to keeping students aware and alert. Having this in mind, and knowing the fact that many teachers are not familiar with technology, while some of them focus on the use of usual software such as Word, PowerPoint, etc., the teachers may face difficulties, or they may need technical support for the full utilization of the emerging and artificial intelligence tools. These teachers need appropriate education to support the successful implementation of a smart classroom with new technologies.
E-learning involves the use of the internet as a platform for educational activities, that can include three-dimensional environments or real-time virtual interactions between students and teachers. The e-learning environment is seen as a crucial tool for supporting conventional learning formats and is changing how higher education is provided. E-learning platforms have several benefits, including improving student-centered learning, helping students become more independent while educators take on innovative roles, encouraging educators to be more reflective and methodical in creating better e-learning resources, and ultimately giving students the skills to adapt to a constantly changing technology-driven environment. AI-powered personalization and adaptive learning can be used to adjust the pace and difficulty level of content for individual students. E-learning enables students to receive learning activities from educators at a distance, allows for larger classes, and makes it easier to identify and record behaviors and errors to improve learning activities. Even though face-to-face learning is better for daily discussions and contributes to an active environment (Valdez et al., 2015 ), this generation of adolescents dislikes traditional classroom education. Although they like to learn at their own pace, they are curious. Because of their addiction to technology, they rely increasingly on online teaching and learning resources. Through collaborative learning, they enjoy sharing their knowledge with their peers and benefiting from the strengths of their competitors (Agarwal et al., 2019 ).
Virtual spaces in a smart classroom resemble real places allowing students to have an immersive experience and create real memories. Moreover, seeing, ‘touching’ and hearing involve more senses in the learning process and link the learning subjects in multiple ways. Therefore, enriched presentation of the learning material and better visualization, which resembles reality and involves more senses, enhances students’ experience and learning becomes sustainable (Lui & Slotta, 2014 ). Furthermore, the student’s motivation is triggered, situated scaffolding is provided and learning is connected with the student’s everyday life (Bower et al., 2014 ) through an experiential learning process. AI can be used to create more immersive and interactive learning experiences by tracking student movements and adjusting the virtual environment accordingly. Since a smart classroom is equipped with contemporary visualization technologies, which include interactive whiteboards, projectors, all-screen technology, virtual/augmented reality headsets, cameras, and sensors, students can better visualize the content they are taught, enhancing in that way the learning experience. In a smart classroom, students may be immersed in online virtual environments using headsets; as a result, distractions are removed, and the student’s attention is captured. Furthermore, perspective-changing in virtual reality visualizations allows students to become actors rather than just observers, transforming the learning process into a highly experiential experience (Krüger et al., 2019 ).
Computer vision based surveillance
Smart classrooms provide real-time video analysis to educators that want to recognize the behavioural participation and behavioural disaffection of their students (Michalsky, 2021 ). AI-enabled cameras can be used to monitor the classroom for safety and security, as well as to track student attendance and participation . The employment of smart tools and applications, such as cameras, plagiarism checking, and recording, combined with the continuous gathering of data, allows teachers to control student attendance and supervise them both in class and during online assessments (Saini & Goel, 2019 ).
A challenge concerning the use of advanced technological systems is bias in AI. More specifically, there is a concern regarding how fair can AI systems be to every single student despite the personal attributes of a student (i.e., race and gender) (Li et al., 2021 ). Groups that face discrimination in the community of technology, like female students, might face more severe inequalities if the creation of AI systems doesn’t consider how to mitigate such biases. Technologically advanced key technologies related to smart classes incorporating AI capabilities are threatened by security and privacy issues because they store and process data that contain personal and sensitive information that may be exposed to potential invaders (Manca et al., 2016 ). Furthermore, sensors used in classes (i.e. camera sensors or microphones) often used as part of smart-class technological tools, are associated with privacy issues.
One of the greatest challenges in introducing key technologies related to smart classes in schools is the overall cost of equipment required for a comprehensive smart-class deployment is high (Saini & Goel, 2019 ), preventing in that way the widespread use of smart-class technologies. Bearing in mind that the cost does not refer only to the equipment purchase and initial installation, but also to the continuous upgrade and maintenance, the use of smart class technology involves significant running expenses. Also, because all components of a smart class are somehow disconnected from each other, it is not seamless to integrate all technologies under a common framework, and as a result, the task of setting up the equipment can be a lengthy and time-consuming procedure.
Learning about computers, electronics, mechanical engineering, and languages may be interesting thanks to robots. It has been demonstrated that when language acquisition was facilitated by a robot as opposed to audiotapes and books, young children did better on post-learning assessments and displayed greater enthusiasm (Mubin et al., 2013 ). AI can be used to control and program educational robots that can interact with students and enhance their learning experience. The teacher in smart classroom takes on the role of a facilitator if the robot is the focal point of the learning activity (i.e., used as a teaching tool, as in the case of teaching about robotics). If the robot plays a passive role, the teacher must provide fundamental knowledge (e.g., by using the robot in language classes). In such cases, robotics curriculum implementation and teacher training are crucial. Looking ahead, it is evident that more has to be done to secure teacher support before robots can be completely included into our schools. Teachers were rated less favorably than parents and children in a survey regarding school robots. Teachers need to be reassured that the objective is not to replace them with robots but to give them a teaching tool or aid that can enhance the educational process and inspire students.
AI in smart classes: A SWOT analysis
Based on the analysis presented in the previous subsections, the advantages of using AI and emerging technologies may also involve risks that may jeopardise the learning efficiency and experience. In this subsection a SWOT analysis of using AI in smart classes is presented as a means of summarizing the potential of using AI in smart classes, along with possible drawbacks. These observations are presented in a SWOT analysis presented in Table 7 .
Discussion-future directions
In this section we outline the main issues in the dimensions of technology infrastructure, personnel, and data handling that need to be addressed by the research community in order to maximize the impact of AI in enhancing smart classes.
Technology infrastructure
To enhance the capabilities of a smart classroom it is necessary to integrate all technologies, hence a combination of emerging technologies and AI is essential. A central AI system that can manage the use of different technologies, suggest optimum ways of integrating each technology in specific classes, and provide a comprehensive evaluation of students and the educational process will be a highly desirable feature of future smart classes.
Since the teaching process is a highly dynamic process where educators need to adapt to the changes in student attitudes and overall class requirements, it is important to deploy AI-based systems that continuously monitor the student requirements and adjust to respond to all changes. While this can take the form of reinforcement learning (Liu et al., 2018 ) dedicated techniques for AI systems that deal with in-class scenarios, need to be devised.
The integration of special technical equipment is usually an issue due to the expensive cost. As a result, it is critical to adopt new low-cost technical equipment that students may use anywhere, at any time. Experts should develop techniques and technologies that can run on personal equipment rather than dedicated machines, for example using smart phones or low-end personal computers. When it comes to AI-based systems that need to be re-trained continuously, efficient training methods that allow the training process to be completed using ordinary computer systems, need to be employed, so that costs associated with the purchase of dedicated equipment or the purchase of computational time, are decreased.
The need to adopt in practice appropriate teacher training programs regarding the use of technology in education has become urgent. Apart from training for using emerging technologies, educators should also receive adequate training for AI related issues, so that they learn how to harness the power of AI systems for the benefit of the education process. Thus, it is imperative that dedicated AI courses for educators are created, so that smart class teachers are well aware of the potential and risks of using AI empowered emerging technologies. Furthermore, dedicated user-friendly tools that will allow educators to train and use Machine Learning modules should be developed.
Classroom overlays for teachers that incorporate grades, special arrangements, and medical and social information are probably among the educational applications of the future. As technology advances, it will be able to alert teachers to students' learning needs and behavioral issues in real-time and provide solutions. Teachers are sometimes untrained to handle the technical challenges that may occur when a device does not function as planned. As a result, for teachers to succeed, there may be a significant amount of assistance required. To prevent the design of learning from being largely the responsibility of computer scientists who have a limited understanding of successful pedagogy, it is essential that educators learn how to integrate technology into their teaching (Krüger et al., 2019 ). With the recognition that students' abilities can be impacted by their cognitive, motor, and spatial capabilities, technology also makes it easier for teachers to teach content and learning objectives. Students can become more actively involved in the learning process as they develop their motivation and foundational knowledge (Liono et al., 2021 ).
Empathy is the ability to recognize somebody's emotional reactions and motives, care for them and their sentiments (Srinivasan & González, 2022 ). It is vital to develop specialized AI systems that take into consideration the unique characteristics of each student, through an empathetic nature. The topic of producing “empathetic” AI systems can open up several research directions.
Data handling
One of the most crucial issues of the future of smart classes is ethics in the use of data in AI systems (Borenstein & Howard, 2021 ). It is vital to address the way data are collected and used by those systems in order to avoid the violation of privacy. Regulations regarding the collection of data must be established and adopted by the scientific community. Data can also be encrypted and anonymised, so in case a hack occurs it won’t be feasible to find correlations between provided data and individuals. Within this scope, new methods that guarantee data security, but at the same time allows the access to the necessary information by different stakeholders, within a smart-class needs to be developed.
Bias in AI is an issue that needs be addressed while using advanced technological systems. More precisely, there is worry about how fair AI systems can be to all students, regardless the attributes of each student such as ability level, race, religion, appearance, or gender (Li et al., 2021 ). Developers must consider all the biases that may rise due to their personal beliefs and eliminate them. In this way, any form of discrimination towards minorities will be alleviated and students will be able to attend education and receive fair feedback compared to their peers. Furthermore, since supervised AI systems often rely on annotated data, techniques that ensure that any form of bias in the annotation process is eliminated, so that the resulting AI systems are not subjected to any kind of discrimination. AI systems should include machine learning techniques with explainable AI to analyze the educational factors that lead to more fair and effective decision making for students since the ML-based black box model is more understandable to educators (Guleria & Sood, 2022 ).
Using data collection, user profiling, and adaptive learning can be useful in creating a more personalized and effective learning experience, and artificial intelligence can play a role in supporting these efforts. However, it is important to carefully consider the ethical implications of collecting and using data, and to ensure that students' privacy is protected. The use of AI in education can also raise questions about the role of technology in learning and the potential for it to replace human teachers. While AI can certainly be a useful tool for supporting and enhancing education, it is important to consider the limitations of technology and the value of human interaction and guidance in the learning process. Overall, it is necessary to carefully evaluate the potential benefits and risks of using AI and other technology in education, and to strike a balance between the use of technology and more traditional teaching methods.
Conclusions
A range of AI-assisted emerging technologies, that include technologies related to class management, teaching aids and performance assessment have been presented. For each smart class technology presented the role of AI was discussed, allowing the in that way the determination of the role of AI in smart classes. Furthermore, through the analysis of advantages and disadvantages of smart classes, along with a SWOT analysis, the prospects, and trends related to the use of AI on smart classes have been discussed, allowing in that way the definition of several future research directions. The future directions presented can provide motivation to the AI, and educational technology research communities to engage in research activities that aim to deal with the identified challenges. Since the new era of technological advancement and the proliferation of digital devices and applications that are routinely used in everyday life has been integrated in education, there is a continuous need to invest in improving the services offered to students and the further development of AI-based smart classes definitely leads those efforts in the right direction.
Since the concept of smart-classes is continuously enriched through the introduction of requirements and new technologies, in the future we plan to monitor this area and produce updated surveys to reflect future developments and conduct investigations in the area of intelligent learning environment. In addition, in the future we plan to provide specific comparative evaluations of different technologies, so that to quantify the effect of existing technologies and highlight the need for future improvements.
https://www.aurasma.com .
https://www.layar.com/ .
http://www.augment.com/es/ .
http://www.aumentaty.com/ .
Abbreviations
ARTIFICIAL intelligence
Artificial intelligence in education
Artificial neural networks
Augmented reality
Cave automatic virtual environment
Head-mounted displays
Internet of things
Machine learning
Mixed reality
Virtual reality
Abbasy, M. B., & Quesada, E. V. (2017). Predictable influence of IoT in the higher education. International Journal of Information and Education Technology .
Abd-Elaal, E. S., Gamage, S. H., & Mills, J. E. (2019). Artificial intelligence is a tool for cheating academic integrity. In 30th Annual Conference for the Australasian Association for Engineering Education, (pp. 397–403).
Abdel-Basset, M., Manogaran, G., Mohamed, M., & Rushdy, E. (2019). Internet of things in smart education environment: Supportive framework in the decision-making process. Concurrency and Computation: Practice and Experience, 31 (10), e4515.
Article Google Scholar
Adams, J. C., & Hotrop, J. (2008). Building an economical VR system for CS education. ACM SIGCSE Bulletin, 40 (3), 148–152.
Agarwal, A., Naidu, V. R., & Al Mamari, R. (2019). A framework to enhance learning experience in flipped classroom based on student accountability towards active participation. EDULEARN19 Proceedings , 1 , 1569-1577.
Ahmed, R. A. (2015). Overview of different plagiarism detection tools. International Journal of Futuristic Trends in Engineering and Technology, 2 (10), 1–3.
Google Scholar
Akçayır, G., & Akçayır, M. (2018). The flipped classroom: A review of its advantages and challenges. Computers & Education, 126 , 334–345.
Alakärppä, I., Jaakkola, E., Väyrynen, J., & Häkkilä, J. (2017). Using nature elements in mobile AR for education with children. In Proceedings of the 19th International Conference on Human-Computer Interaction with Mobile Devices and Services (pp. 1–13).
Alfalah, S. F., Falah, J. F., Alfalah, T., Elfalah, M., Muhaidat, N., & Falah, O. (2019). A comparative study between a virtual reality heart anatomy system and traditional medical teaching modalities. Virtual Reality, 23 (3), 229–234.
AlFarsi, G., Tawafak, R. M., ElDow, A., Malik, S. I., Jabbar, J., & Al Sideiri, A. (2021). Smart classroom technology in artificial intelligence: A review paper. In International Conference on Culture Heritage, Education, Sustainable Tourism, and Innovation Technologies (pp. 229–235).
Ali, M. Y., Zhang, X. D., & Harun-Ar-Rashid, M. (2020). Student activities detection of SUST using YOLOv3 on deep learning. Indonesian Journal of Electrical Engineering and Informatics, 8 (4), 757–769.
Amra, I. A. A., & Maghari, A. Y. (2017). Students performance prediction using KNN and Naïve Bayesian. In 2017 8th International Conference on Information Technology (pp. 909–913).
Archer, J., Cantrell, S., Holtzman, S. L., Joe, J. N., Tocci, C. M., & Wood, J. (2016). Better feedback for better teaching: A practical guide to improving classroom observations .
Augusto, J. C. (2009). Ambient intelligence: Opportunities and consequences of its use in smart classrooms. Innovation in Teaching and Learning in Information and Computer Sciences, 8 (2), 53–63.
Balfour, S. P. (2013). Assessing writing in MOOCs: automated essay scoring and calibrated peer review™. Research & Practice in Assessment, 8 , 40–48.
Beetham, H., & Sharpe, H. (2013). Rethinking pedagogy for a digital age: Designing for 21st century learning Abingdon . Routledge.
Book Google Scholar
Ben-Ari, M., & Mondada, F. (2018). Robots and their applications. In Elements of Robotics .
Benta, D., Bologa, G., & Dzitac, I. (2014). E-learning platforms in higher education. Case Study. Procedia Computer Science, 31 , 1170–1176.
Benta, D., Bologa, G., Dzitac, S., & Dzitac, I. (2015). University level learning and teaching via e-learning platforms. Procedia Computer Science, 55 , 1366–1373.
Bhatia, M., & Kaur, A. (2021). Quantum computing inspired framework of student performance assessment in smart classroom. Transactions on Emerging Telecommunications Technologies , 32 (9).
Billinghurst, M., Clark, A., & Lee, G. (2015). A survey of augmented reality. Foundations and Trends® in Human–Computer Interaction , 8 (2–3), 73–272.
Blessinger, P., & Wankel, C. (Eds.). (2013). Increasing student engagement and retention using classroom technologies: Classroom response systems and mediated discourse technologies .
Blyth, C. (2018). Immersive technologies and language learning. Foreign Language Annals .
Borenstein, J., & Howard, A. (2021). Emerging challenges in AI and the need for AI ethics education. AI and Ethics, 1 (1), 61–65.
Bourguet, M.-L., Jin, Y., Shi, Y., Chen, Y., Rincon-Ardila, L., & Venture, G. (2020). Social robots that can sense and improve student engagement. In 2020 IEEE International Conference on Teaching, Assessment, and Learning for Engineering (TALE) , 127–134.
Bower, M., Howe, C., McCredie, N., Robinson, A., & Grover, D. (2014). Augmented Reality in education–cases, places and potentials. Educational Media International, 51 (1), 1–15.
Brady, M., Gerhardt, L. A., & Davidson, H. F. (2012). Robotics and artificial intelligence.
Brudy, F., Holz, C., Rädle, R., Wu, C. J., Houben, S., Klokmose, C. N., & Marquardt, N. (2019). Cross-device taxonomy: Survey, opportunities and challenges of interactions spanning across multiple devices. In Proceedings of the 2019 chi conference on human factors in computing systems (pp. 1–28).
Burrack, F., & Thompson, D. (2021). Canvas (LMS) as a means for effective student learning assessment across an institution of higher education. Journal of Assessment in Higher Education, 2 (1), 1–19.
Cai, S., Liu, E., Yang, Y., & Liang, J. C. (2019). Tablet-based AR technology: Impacts on students’ conceptions and approaches to learning mathematics according to their self-efficacy. British Journal of Educational Technology, 50 (1), 248–263.
Causo, A., Vo, G. T., Chen, I., & Yeo, S. H. (2016). Design of robots used as education companion and tutor. In Robotics and mechatronics (pp. 75–84).
Chamba-Eras, L., & Aguilar, J. (2017). Augmented reality in a smart classroom—Case study: SaCI. IEEE Revista Iberoamericana De Tecnologias Del Aprendizaje, 12 (4), 165–172.
Chang, C. W., Lee, J. H., Wang, C. Y., & Chen, G. D. (2010). Improving the authentic learning experience by integrating robots into the mixed-reality environment. Computers & Education, 55 (4), 1572–1578.
Chen, L., Chen, P., & Lin, Z. (2020). Artificial intelligence in education: A review. Ieee Access .
Chen, C. M., & Tsai, Y. N. (2012). Interactive augmented reality system for enhancing library instruction in elementary schools. Computers & Education, 59 (2), 638–652.
Chen, N. S., Cheng, I. L., & Chew, S. W. (2016). Evolution is not enough: Revolutionizing current learning environments to smart learning environments. International Journal of Artificial Intelligence in Education, 26 (2), 561–581.
Chen, X., Zou, D., Xie, H., Cheng, G., & Liu, C. (2022). Two decades of artificial intelligence in education. Educational Technology & Society, 25 (1), 28–47.
Chintalapati, S., & Raghunadh, M. V. (2013). Automated attendance management system based on face recognition algorithms. In 2013 IEEE International conference on computational intelligence and computing research (pp. 1–5).
Chocarro, R., Cortiñas, M., & Marcos-Matás, G. (2021). Teachers’ attitudes towards chatbots in education: a technology acceptance model approach considering the effect of social language, bot proactiveness, and users’ characteristics. Educational Studies (pp. 1–19).
Chong, M., Specia, L., & Mitkov, R. (2010). Using natural language processing for automatic detection of plagiarism. In Proceedings of the 4th International Plagiarism Conference .
Chowdhary, K. (2020). Natural language processing. Fundamentals of artificial intelligence .
Chowdhury, G.G. (2003). Natural Language Processing. Annual Review of Information Science and Technology.
Chowdhury, S., Nath, S., Dey, A., & Das, A. (2020). Development of an automatic class attendance system using cnn-based face recognition. In 2020 Emerging Technology in Computing, Communication and Electronics (pp. 1–5).
Colace, F., De Santo, M., Lombardi, M., Pascale, F., Pietrosanto, A., & Lemma, S. (2018). Chatbot for e-learning: A case of study. International Journal of Mechanical Engineering and Robotics Research, 7 (5), 528–533.
Colchester, K., Hagras, H., Alghazzawi, D., & Aldabbagh, G. (2017). A survey of artificial intelligence techniques employed for adaptive educational systems within e-learning platforms. Journal of Artificial Intelligence and Soft Computing Research, 7 (1), 47–64.
Dascalu, M. I., Moldoveanu, A., & Shudayfat, E. A. (2014). Mixed reality to support new learning paradigms. In 2014 18th International Conferences on System Theory, Control and Computing (pp. 692–697).
Demitriadou, E., Stavroulia, K. E., & Lanitis, A. (2020). Comparative evaluation of virtual and augmented reality for teaching mathematics in primary education. Education and Information Technologies, 25 , 381–401.
Díaz León, C. A., Hincapié Montoya, E. M., Guirales Arredondo, E. A., & Moreno López, G. A. (2016). Design and development of an interaction system in order to be implemented in a smart classroom. Revista EIA, 26 , 95–109.
Dimitriadou, E., & Lanitis, A. (2022b). Using student action recognition to enhance the efficiency of tele-education. In Proceedings of the 17th International Joint Conference on Computer Vision, Imaging and Computer Graphics Theory and Applications (pp. 543–549).
Dimitriadou, E., & Lanitis, A. (2022a). The role of artificial intelligence in smart classes: A survey. In 2022a IEEE 21st Mediterranean Electrotechnical Conference (pp. 642–647).
Dobre, I. (2015). Learning management systems for higher education-an overview of available options for higher education organizations. Procedia-Social and Behavioral Sciences, 180 , 313–320.
Dryjanski, M., Buczkowski, M., Ould-Cheikh-Mouhamedou, Y., & Kliks, A. (2020). Adoption of smart cities with a practical smart building implementation. IEEE Internet of Things Magazine, 3 (1), 58–63.
Dünser, A., & Hornecker, E. (2007). Lessons from an AR book study. In Proceedings of the 1st international conference on Tangible and embedded interaction (pp. 179–182).
Durlach, P. J., & Lesgold, A. M. (2012). Adaptive technologies for training and education .
El Beheiry, M., Doutreligne, S., Caporal, C., Ostertag, C., Dahan, M., & Masson, J. B. (2019). Virtual reality: Beyond visualization. Journal of Molecular Biology, 431 (7), 1315–1321.
Ellern, G. J. D., & Buchanan, H. E. (2018). No strings attached? Challenges and successes in creating a flexible, wire-free active learning classroom. Library Hi Tech .
Feil-Seifer, D., & Matarić, M. J. (2009). Toward socially assistive robotics for augmenting interventions for children with autism spectrum disorders. In Experimental robotics , (pp. 201–210).
Fiore, A., Mainetti, L., & Vergallo, R. (2014). An innovative educational format based on a mixed reality environment: A case study and benefit evaluation. In International Conference on E-Learning, E-Education, and Online Training (pp. 93–100).
Geramani, K., Mazzucato, A., Gaudiello, I., & Ioannidis, G. (2022). Artificial Intelligence in the secondary education: The paradigm of Edu4AI. In E Third Workshop on Technology Enhanced Learning Environments .
Górski, F., Buń, P., Wichniarek, R., Zawadzki, P., & Hamrol, A. (2016). Effective design of educational virtual reality applications for medicine using knowledge-engineering techniques. EURASIA Journal of Mathematics, Science and Technology Education.
GU, W. (2017). The Application of Virtual Reality in Education. DEStech Transactions on Computer Science and Engineering .
Guleria, P., & Sood, M. (2022). Explainable AI and machine learning: performance evaluation and explainability of classifiers on educational data mining inspired career counseling. Education and Information Technologies , 1–36.
Gupta, S. K., Ashwin, T. S., & Guddeti, R. M. R. (2018). Cvucams: Computer vision based unobtrusive classroom attendance management system. In 2018 IEEE 18th International Conference on Advanced Learning Technologies (pp. 101–102).
Gutierrez, E. O. C., & De Troyer, O. (2014). SimBully: A'bullying in schools' simulation.
Guttormsen, M., Bürger, A., Hansen, T. E., & Lietaer, N. (2011). The SiRi particle-telescope system. Nuclear Instruments and Methods in Physics Research Section a: Accelerators, Spectrometers, Detectors and Associated Equipment, 648 (1), 168–173.
Hampel, G., & Dancsházy, K. (2014). Creating a virtual learning environment. Agrárinformatika/journal of Agricultural Informatics, 5 (1), 46–55.
Han, J., Jo, M., Park, S., & Kim, S. (2005). The educational use of home robots for children. In ROMAN 2005. IEEE International Workshop on Robot and Human Interactive Communication (pp. 378–383).
Han, J., & Kim, D. (2009). r-Learning services for elementary school students with a teaching assistant robot. In 4th ACM/IEEE Intl Conf. on Human-Robot Interaction (pp. 255–256).
Hashimoto, T., Kato, N., & Kobayashi, H. (2011). Development of educational system with the android robot SAYA and evaluation. Intl. Journal of Advanced Robotic Systems, 8 (3), 28.
Have, H. & Neves, M. d. C. P. (2021). Emerging technologies. Dictionary of Global Bioethics .
Helms, A. S., Schmiegelow, K., Brok, J., Johansen, C., Thorsteinsson, T., Simovska, V., & Larsen, H. B. (2016). Facilitation of school re-entry and peer acceptance of children with cancer: A review and meta-analysis of intervention studies. European Journal of Cancer Care, 25 (1), 170–179.
Hsu, P. C., Wang, C. H., Liu, A. T., & Lee, H. Y. (2019). Towards robust neural vocoding for speech generation: A survey .
Huang, L. S., Su, J. Y., & Pao, T. L. (2019). A context aware smart classroom architecture for smart campuses. Applied Sciences, 9 (9), 1837.
Ibáñez, M. B., Di Serio, Á., Villarán, D., & Kloos, C. D. (2014). Experimenting with electromagnetism using augmented reality: Impact on flow student experience and educational effectiveness. Computers & Education, 71 , 1–13.
Ip, H. H., Wong, S. W., Chan, D. F., Byrne, J., Li, C., Yuan, V. S., Lau, K. S., & Wong, J. Y. (2018). Enhance emotional and social adaptation skills for children with autism spectrum disorder: A virtual reality enabled approach. Computers & Education, 117 , 1–15.
Ishiguro, H., Ono, T., Imai, M., Maeda, T., Kanda, T., & Nakatsu, R. (2001). Robovie: An interactive humanoid robot. Industrial Robot: An International Journal, 28 (6), 498–504.
Jensen, E., Dale, M., Donnelly, P. J., Stone, C., Kelly, S., Godley, A., & D'Mello, S. K. (2020). Toward automated feedback on teacher discourse to enhance teacher learning. In Proceedings of the 2020 CHI Conference on Human Factors in Computing Systems .
Jensen, E., L. Pugh, S., & K. D'Mello, S. (2021). A deep transfer learning approach to modeling teacher discourse in the classroom. In LAK21: 11th International Learning Analytics and Knowledge Conference (pp. 302–312).
Johal, W., Castellano, G., Tanaka, F., & Okita, S. (2018). Robots for learning. International Journal of Social Robotics, 10 (3), 293–294.
Jones, A., & Castellano, G. (2018). Adaptive robotic tutors that support self-regulated learning: A longer-term investigation with primary school children. International Journal of Social Robotics, 10 (3), 357–370.
Karri, S. P. R., & Kumar, B. S. (2020). Deep learning techniques for implementation of chatbots. In 2020 International Conference on Computer Communication and Informatics (pp. 1–5).
Kasapakis, V., Gavalas, D., & Dzardanova, E. (2019). Mixed Reality .
Kaufmann, H., & Schmalstieg, D. (2002). Mathematics and geometry education with collaborative augmented reality. In ACM SIGGRAPH 2002 conference abstracts and applications.
Kaviyaraj, R., & Uma, M. (2021). A Survey on Future of Augmented Reality with AI in Education. In 2021 International Conference on Artificial Intelligence and Smart Systems (pp. 47–52).
Kawaguchi, Y., Shoji, T., Lin, W., Kakusho, K., & Minoh, M. (2005). Face recognition-based lecture attendance system. In The 3rd AEARU workshop on network education (pp. 70–75).
Kc, D. (2017). Evaluation of moodle features at kajaani university of applied sciences–case study. Procedia Computer Science, 116 , 121–128.
Kennedy, J., Baxter, P., & Belpaeme, T. (2015). Comparing robot embodiments in a guided discovery learning interaction with children. International Journal of Social Robotics, 7 (2), 293–308.
Kerr, J., & Lawson, G. (2020). Augmented reality in design education: Landscape architecture studies as AR experience. International Journal of Art & Design Education, 39 (1), 6–21.
Kim, J., & Shim, J. (2022). Development of an AR-based AI education app for non-majors. IEEE Access, 10 , 14149–14156.
Knudsen, C. J., & Naeve, A. (2002). Presence production in a distributed shared virtual environment for exploring mathematics. In Advanced Computer Systems (pp. 149–159).
Kollia, I., & Siolas, G. (2016). Using the IBM Watson cognitive system in educational contexts. In 2016 IEEE Symposium Series on Computational Intelligence (pp. 1–8).
Kong, Y., & Fu, Y. (2022). Human action recognition and prediction: A survey. International Journal of Computer Vision, 130 (5), 1366–1401.
Krüger, J. M., Buchholz, A., & Bodemer, D. (2019). Augmented reality in education: three unique characteristics from a user’s perspective. In Proc. 27th Int. Conf. on Comput. in Educ .
Lau, T. B., Ong, A. C., & Putra, F. A. (2014). Non-invasive monitoring of people with disabilities via motion detection. International Journal of Signal Processing Systems, 2 (1), 37–41.
Lee, J. H., Yang, H., Shin, D., & Kim, H. (2020). Chatbots .
Li, X., Wang, M., Zeng, W., & Lu, W. (2019). A students’ action recognition database in smart classroom. In 2019 14th International Conference on Computer Science & Education.
Li, C., Xing, W., & Leite, W. (2021). Using fair AI with debiased network embeddings to support help seeking in an online math learning platform. In International Conference on Artificial Intelligence in Education (pp. 245–250).
Lin, H., Xie, S., Xiao, Z., Deng, X., & Cai, K. (2019). Adaptive Recommender System for an Intelligent Classroom Teaching Model. International journal of emerging technologies in learning , 14 (5).
Liono, R. A., Amanda, N., Pratiwi, A., & Gunawan, A. A. (2021). A systematic literature review: Learning with visual by the help of augmented reality helps students learn better. Procedia Computer Science, 179 , 144–152.
Liu, S., Chen, Y., Huang, H., Xiao, L., & Hei, X. (2018). Towards smart educational recommendations with reinforcement learning in classroom. In 2018 IEEE International Conference on Teaching, Assessment, and Learning for Engineering (pp. 1079–1084).
Lo, C. K., Hew, K. F., & Chen, G. (2017). Toward a set of design principles for mathematics flipped classrooms: A synthesis of research in mathematics education. Educational Research Review, 22 , 50–73.
Loos, R. (2015). CoWriter: Children using robots to learn writing. Robotics Today, March , 8 .
Lu, S. J., & Liu, Y. C. (2015). Integrating augmented reality technology to enhance children’s learning in marine education. Environmental Education Research, 21 (4), 525–541.
Lui, M., & Slotta, J. D. (2014). Immersive simulations for smart classrooms: exploring evolutionary concepts in secondary science. Technology, Pedagogy and Education .
Manca, S., Caviglione, L., & Raffaghelli, J. (2016). Big data for social media learning analytics: potentials and challenges. Journal of e-Learning and Knowledge Society , 12 (2).
Martín-Gutiérrez, J., Mora, C. E., Añorbe-Díaz, B., & González-Marrero, A. (2017). Virtual technologies trends in education. Eurasia Journal of Mathematics, Science and Technology Education, 13 (2), 469–486.
Mateu, J., Lasala, M. J., & Alamán, X. (2015). Developing mixed reality educational applications: The virtual touch toolkit. Sensors, 15 (9), 21760–21784.
Mery, D., Mackenney, I., & Villalobos, E. (2019). Student attendance system in crowded classrooms using a smartphone camera. In 2019 IEEE Winter Conference on Applications of Computer Vision (pp. 857–866)
Michalsky, T. (2021). Integrating video analysis of teacher and student behaviors to promote Preservice teachers’ teaching meta-strategic knowledge. Metacognition and Learning, 16 (3), 595–622.
Mintz, R., Litvak, S., & Yair, Y. (2001). 3D-virtual reality in science education: An implication for astronomy teaching. Journal of Computers in Mathematics and Science Teaching, 20 (3), 293–305.
Mircea, M., Stoica, M., & Ghilic-Micu, B. (2021). Investigating the impact of the internet of things in higher education environment. IEEE Access, 9 , 33396–33409.
Morrissey, K., & Kirakowski, J. (2013). ‘Realness’ in chatbots: establishing quantifiable criteria. In International conference on human-computer interaction (pp. 87–96).
Moseley, S., & Ajani, T. (2015). USERS'PERCEPTIONS ON THE BRIGHTSPACE LEARNING MANAGEMENT SYSTEM. Issues in Information Systems , 16 (4).
Mubin, O., Stevens, C. J., Shahid, S., Al Mahmud, A., & Dong, J. J. (2013). A review of the applicability of robots in education. Journal of Technology in Education and Learning, 1 (209–0015), 13.
Mystakidis, S. (2022). Metaverse. Encyclopedia, 2 (1), 486–497.
Ngoc Anh, B., Tung Son, N., Truong Lam, P., Phuong Chi, L., Huu Tuan, N., Cong Dat, N., Huu Trung, N., Umar Aftab, M., & Van Dinh, T. (2019). A computer-vision based application for student behavior monitoring in classroom. Applied Sciences, 9 (22), 4729.
Okita, S. Y., Ng-Thow-Hing, V., & Sarvadevabhatla, R. (2009). Learning together: ASIMO developing an interactive learning partnership with children. In RO-MAN 2009-The 18th IEEE International Symposium on Robot and Human Interactive Communication .
Osada, J., Ohnaka, S., & Sato, M. (2006). The scenario and design process of childcare robot, PaPeRo. In Proceedings of the 2006 ACM SIGCHI international conference on Advances in computer entertainment technology (pp. 80-es).
Ouyang, X., & Zhou, J. (2019). How to help older adults move the focus on a smart TV? Exploring the effects of arrow hints and element size consistency. International Journal of Human-Computer Interaction, 35 (15), 1420–1436.
Ouyang, X., Zhou, J., & Xiang, H. (2021). Screen mirroring is not as easy as it seems: A closer look at older adults’ cross-device experience through touch gestures. International Journal of Human-Computer Interaction, 37 (12), 1173–1189.
Pakanati, D., Thanner, G., & Reddy, R. R. (2020). Design of College Chatbot using Amazon Web Services .
Palanisamy, P., Paavizhi, K., & Saravanakumar, A. R. (2020). Techno pedagogical skills for teaching-learning process in smart class. Talent Development & Excellence, 12 (1), 4984–4994.
Parambil, M. M. A., Ali, L., Alnajjar, F., & Gochoo, M. (2022). Smart classroom: A deep learning approach towards attention assessment through class behavior detection. Advances in Science and Engineering Technology International Conferences (ASET), 2022 , 1–6. https://doi.org/10.1109/ASET53988.2022.9735018
Parmar, D., Isaac, J., Babu, S. V., D'Souza, N., Leonard, A. E., Jörg, S., Gundersen, K. & Daily, S. B. (2016). Programming moves: Design and evaluation of applying embodied interaction in virtual environments to enhance computational thinking in middle school students. In 2016 IEEE Virtual Reality (VR) (pp. 131–140).
Pereira, J. (2016). Leveraging chatbots to improve self-guided learning through conversational quizzes. In Proceedings of the fourth international conference on technological ecosystems for enhancing multiculturality (pp. 911–918).
Pham, T. V., Nguyen, A. T. T., Ngo, T. D., Le, D. H., Le, K. C., Nguyen, T. H., & Le, H. Q. (2020, November). Proposed smart university model as a sustainable living lab for university digital transformation. In 2020 5th International Conference on Green Technology and Sustainable Development (pp. 472–479)
Poppinga, B., & Laue, T. (2019). JET-Net: Real-time object detection for mobile robots. In Robot World Cup (pp. 227–240).
Potode, A., & Manjare, P. (2015). E-learning using artificial intelligence. International Journal of Computer Science and Information Technology Research, 3 (1), 78–82.
Queiroz, A. C. M., Fauville, G., Herrera, F., Da Leme, M. I. S., & Bailenson, J. N. (2022). Do students learn better with immersive virtual reality videos than conventional videos? A comparison of media effects with middle school girls. Technology, Mind, and Behavior . https://doi.org/10.1037/tmb0000082
Rashmi, M., Ashwin, T. S., & Guddeti, R. M. R. (2021). Surveillance video analysis for student action recognition and localization inside computer laboratories of a smart campus. Multimedia Tools and Applications, 80 (2), 2907–2929.
Reeves, L. E., Bolton, E., Bulpitt, M., Scott, A., Tomey, I., Gates, M., & Baldock, R. A. (2021). Use of augmented reality (AR) to aid bioscience education and enrich student experience. Research in Learning Technology, 29 , 2572.
Rufai, M. M., Alebiosu, S. O., & Adeakin, O. A. S. (2015). A conceptual model for virtual classroom management. International Journal of Computer Science, Engineering and Information Technology, 5 (1), 27–32.
Sadiku, M. N., Ashaolu, T. J., Ajayi-Majebi, A., & Musa, S. M. (2021). Artificial intelligence in education. International Journal of Scientific Advances, 2 (1), 5–11.
Sahlström, F., Tanner, M., & Valasmo, V. (2019). Connected youth, connected classrooms. Smartphone use and student and teacher participation during plenary teaching. Learning, Culture and Social Interaction, 21 , 311–331.
Sahu, C. K., Young, C., & Rai, R. (2021). Artificial intelligence (AI) in augmented reality (AR)-assisted manufacturing applications: A review. International Journal of Production Research, 59 (16), 4903–4959.
Saini, M. K., & Goel, N. (2019). How smart are smart classrooms? A review of smart classroom technologies. ACM Computing Surveys, 52 (6), 1–28.
Salichs, M. A., Barber, R., Khamis, A. M., Malfaz, M., Gorostiza, J. F., Pacheco, R., Rivas, R., Corrales, A., Delgado, E., & Garcia, D. (2006). Maggie: A robotic platform for human-robot social interaction. In 2006 IEEE conference on robotics, automation and mechatronics (pp. 1–7)
Sampaio, A. Z., Ferreira, M. M., Rosário, D. P., & Martins, O. P. (2010). 3D and VR models in civil engineering education: Construction, rehabilitation and maintenance. Automation in Construction, 19 (7), 819–828.
Sejnowski, T. J. (2020). The unreasonable effectiveness of deep learning in artificial intelligence. Proceedings of the National Academy of Sciences, 117 (48), 30033–30038.
Shan, S., & Liu, Y. (2021). Blended teaching design of college students’ mental health education course based on artificial intelligence flipped class. Mathematical Problems in Engineering , 2021 .
Shiomi, M., Kanda, T., Howley, I., Hayashi, K., & Hagita, N. (2015). Can a social robot stimulate science curiosity in classrooms? International Journal of Social Robotics, 7 (5), 641–652.
Singh, J., Joesph, M. H., & Jabbar, K. B. A. (2019). Rule-based chatbot for student enquiries. In Journal of Physics: Conference Series, 1228 (1), 012060.
Soares, N., Kay, J. C., & Craven, G. (2017). Mobile robotic telepresence solutions for the education of hospitalized children. Perspectives in Health Information Management , 14 .
Sobota, B., Korečko, Š., Jacho, L., Pastornický, P., Hudák, M., & Sivý, M. (2017). Virtual-reality technologies and smart environments in the process of disabled people education. In 2017 15th International Conference on Emerging eLearning Technologies and Applications.
Southgate, E., Blackmore, K., Pieschl, S., Grimes, S., McGuire, J., & Smithers, K. (2019). Artificial intelligence and emerging technologies in schools .
Srinivasan, R., & González, B. S. M. (2022). The role of empathy for artificial intelligence accountability. Journal of Responsible Technology, 9 , 100021.
Stavroulia, K. E., Ruiz-Harisiou, A., Manouchou, E., Georgiou, K., Sella, F., & Lanitis, A. (2016). A 3D virtual environment for training teachers to identify bullying. In 2016 18th Mediterranean Electrotechnical Conference (pp. 1–6).
Teja, S. V. (2020). Chatbot using deep learning. Academic Leadership-Online Journal .
Thomas, C., & Jayagopi, D. B. (2017). Predicting student engagement in classrooms using facial behavioral cues. In Proceedings of the 1st ACM SIGCHI International Workshop on Multimodal Interaction for education (pp. 33–40).
Tian, X., Risha, Z., Ahmed, I., Lekshmi Narayanan, A. B., & Biehl, J. (2021). Let's talk it out: A chatbot for effective study habit behavioral change. In Proceedings of the ACM on Human-Computer Interaction (pp.1–32).
Timms, M. J. (2016). Letting artificial intelligence in education out of the box: educational cobots and smart classrooms. International Journal of Artificial Intelligence in Education .
Tolentino, L., Birchfield, D., & Kelliher, A. (2009). SMALLab for special needs: Using a mixed-reality platform to explore learning for children with autism. In Proceedings of the NSF Media Arts, Science and Technology Conference, Santa Barbara, CA, USA (pp. 29–30).
Torres, D. R. (2011). Realidad Aumentada, educación y museos. Revista ICONO 14. Revista científica de Comunicación y Tecnologías emergentes , 9 (2), 212–226.
TS, A., & Guddeti, R. M. R. (2020). Automatic detection of students’ affective states in classroom environment using hybrid convolutional neural networks. Education and Information Technologies, 25 (2), 1387–1415.
Valdez, M. T., Ferreira, C. M., Martins, M. J. M., & Barbosa, F. M. (2015). 3D virtual reality experiments to promote electrical engineering education. In 2015 International Conference on Information Technology Based Higher Education and Training (pp. 1–4).
Vega, J., & Cañas, J. M. (2019). Open vision system for low-cost robotics education. Electronics, 8 (11), 1295.
Vimal, P. P., & Kumbharana, C. K. (2016). Analysis of different examination patterns having question answer formulation, evaluation techniques and comparison of MCQ type with one word answer for automated online examination. International Journal of Scientific and Research Publications , 6 (3).
Waheed, H., Hassan, S. U., Aljohani, N. R., Hardman, J., Alelyani, S., & Nawaz, R. (2020). Predicting academic performance of students from VLE big data using deep learning models. Computers in Human Behavior, 104 , 106189.
Wang, R., Zhang, G., Zhang, F., Dong, Z., & Qi, M. (2021). Student Behavior Recognition in Remote Video Classrooms. In Advances in Intelligent Information Hiding and Multimedia Signal Processing (pp. 496–504).
Warschauer, M., & Grimes, D. (2008). Automated writing assessment in the classroom. Pedagogies: an International Journal, 3 (1), 22–36.
Weibel, M., Nielsen, M. K. F., Topperzer, M. K., Hammer, N. M., Møller, S. W., Schmiegelow, K., & Bækgaard Larsen, H. (2020). Back to school with telepresence robot technology: A qualitative pilot study about how telepresence robots help school-aged children and adolescents with cancer to remain socially and academically connected with their school classes during treatment. Nursing Open, 7 (4), 988–997.
Werner-Seidler, A., Perry, Y., Calear, A. L., Newby, J. M., & Christensen, H. (2017). School-based depression and anxiety prevention programs for young people: A systematic review and meta-analysis. Clinical Psychology Review, 51 , 30–47.
West, D. M. (2018). The future of work: Robots, AI, and automation . Brookings Institution Press.
Woo, H., LeTendre, G. K., Pham-Shouse, T., & Xiong, Y. (2021). The use of social robots in classrooms: A review of field-based studies. Educational Research Review, 33 , 100388.
Wu, X., Yang, Y., Yu, X., & Zheng, C. (2020). Intelligent Classroom System Based on Internet of Things Technology. In International Conferences on Big Data Analytics for Cyber-Physical-Systems (pp. 610–616).
Yang, S., & Chen, L. (2011). A face and eye detection based feedback system for smart classroom. In Proceedings of 2011 International Conference on Electronic & Mechanical Engineering and Information Technology (Vol. 2, pp. 571–574).
Ye, L., Wang, P., Wang, L., Ferdinando, H., Seppänen, T., & Alasaarela, E. (2018). A combined motion-audio school bullying detection algorithm. Intlernational Journal of Pattern Recognition and Artificial Intelligence, 32 (12), 1850046.
Yoon, S. A., Elinich, K., Wang, J., Steinmeier, C., & Tucker, S. (2012). Using augmented reality and knowledge-building scaffolds to improve learning in a science museum. International Journal of Computer-Supported Collaborative Learning, 7 (4), 519–541.
Zhou, F., Duh, H. B. L., & Billinghurst, M. (2008). Trends in augmented reality tracking, interaction and display: A review of ten years of ISMAR. In 2008 7th IEEE/ACM Intl. Symposium on Mixed and Augmented Reality (pp. 193–202).
Zulkifli, N. A., & Rozimela, Y. (2021). Online applications to support remote classroom dialogue and assessment. Journal of Physics: Conference Series, 1779 (1), 012039.
Download references
Acknowledgements
This project has received funding from the European Union’s Horizon 2020 Research and Innovation Programme under Grant Agreement No 739578 and the Government of the Republic of Cyprus through the Directorate General for European Programmes, Coordination and Development.
This project has received partial funding from the European Union’s Horizon 2020 Research and Innovation Programme under Grant Agreement No 739578 and the Government of the Republic of Cyprus through the Directorate General for European Programmes, Coordination and Development.
Author information
Authors and affiliations.
Visual Media Computing Lab, Department of Multimedia and Graphic Arts, Cyprus University of Technology, Limassol, Cyprus
Eleni Dimitriadou & Andreas Lanitis
CYENS Centre of Excellence, Nicosia, Cyprus
You can also search for this author in PubMed Google Scholar
Contributions
ED carried out the literature review and authored the paper under the supervision of AL. Both authors read and approved the final manuscript
Corresponding author
Correspondence to Eleni Dimitriadou .
Ethics declarations
Ethics approval and consent to participate.
Not applicable–This is a survey paper that does not require ethics approval.
Consent for publication
All data generated or analysed during this study are included in this published article.
Competing interests
The authors have no financial or proprietary interests in any material discussed in this article.
Additional information
Publisher's note.
Springer Nature remains neutral with regard to jurisdictional claims in published maps and institutional affiliations.
Rights and permissions
Open Access This article is licensed under a Creative Commons Attribution 4.0 International License, which permits use, sharing, adaptation, distribution and reproduction in any medium or format, as long as you give appropriate credit to the original author(s) and the source, provide a link to the Creative Commons licence, and indicate if changes were made. The images or other third party material in this article are included in the article's Creative Commons licence, unless indicated otherwise in a credit line to the material. If material is not included in the article's Creative Commons licence and your intended use is not permitted by statutory regulation or exceeds the permitted use, you will need to obtain permission directly from the copyright holder. To view a copy of this licence, visit http://creativecommons.org/licenses/by/4.0/ .
Reprints and permissions
About this article
Cite this article.
Dimitriadou, E., Lanitis, A. A critical evaluation, challenges, and future perspectives of using artificial intelligence and emerging technologies in smart classrooms. Smart Learn. Environ. 10 , 12 (2023). https://doi.org/10.1186/s40561-023-00231-3
Download citation
Received : 29 August 2022
Accepted : 31 January 2023
Published : 06 February 2023
DOI : https://doi.org/10.1186/s40561-023-00231-3
Share this article
Anyone you share the following link with will be able to read this content:
Sorry, a shareable link is not currently available for this article.
Provided by the Springer Nature SharedIt content-sharing initiative
- Educational technology
- Artificial intelligence
- Emerging technologies
- Smart classroom
Numbers, Facts and Trends Shaping Your World
Read our research on:
Full Topic List
Regions & Countries
Publications
- Our Methods
- Short Reads
- Tools & Resources
Read Our Research On:
Internet & Technology
6 facts about americans and tiktok.
62% of U.S. adults under 30 say they use TikTok, compared with 39% of those ages 30 to 49, 24% of those 50 to 64, and 10% of those 65 and older.
Many Americans think generative AI programs should credit the sources they rely on
Americans’ use of chatgpt is ticking up, but few trust its election information, whatsapp and facebook dominate the social media landscape in middle-income nations, sign up for our internet, science, and tech newsletter.
New findings, delivered monthly
Electric Vehicle Charging Infrastructure in the U.S.
64% of Americans live within 2 miles of a public electric vehicle charging station, and those who live closest to chargers view EVs more positively.
When Online Content Disappears
A quarter of all webpages that existed at one point between 2013 and 2023 are no longer accessible.
A quarter of U.S. teachers say AI tools do more harm than good in K-12 education
High school teachers are more likely than elementary and middle school teachers to hold negative views about AI tools in education.
Teens and Video Games Today
85% of U.S. teens say they play video games. They see both positive and negative sides, from making friends to harassment and sleep loss.
Americans’ Views of Technology Companies
Most Americans are wary of social media’s role in politics and its overall impact on the country, and these concerns are ticking up among Democrats. Still, Republicans stand out on several measures, with a majority believing major technology companies are biased toward liberals.
22% of Americans say they interact with artificial intelligence almost constantly or several times a day. 27% say they do this about once a day or several times a week.
About one-in-five U.S. adults have used ChatGPT to learn something new (17%) or for entertainment (17%).
Across eight countries surveyed in Latin America, Africa and South Asia, a median of 73% of adults say they use WhatsApp and 62% say they use Facebook.
5 facts about Americans and sports
About half of Americans (48%) say they took part in organized, competitive sports in high school or college.
REFINE YOUR SELECTION
Research teams, signature reports.
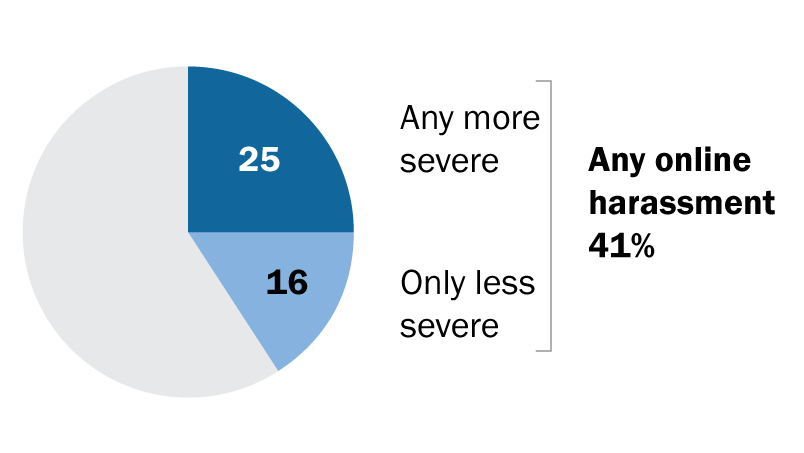
The State of Online Harassment
Roughly four-in-ten Americans have experienced online harassment, with half of this group citing politics as the reason they think they were targeted. Growing shares face more severe online abuse such as sexual harassment or stalking
Parenting Children in the Age of Screens
Two-thirds of parents in the U.S. say parenting is harder today than it was 20 years ago, with many citing technologies – like social media or smartphones – as a reason.
Dating and Relationships in the Digital Age
From distractions to jealousy, how Americans navigate cellphones and social media in their romantic relationships.
Americans and Privacy: Concerned, Confused and Feeling Lack of Control Over Their Personal Information
Majorities of U.S. adults believe their personal data is less secure now, that data collection poses more risks than benefits, and that it is not possible to go through daily life without being tracked.
Americans and ‘Cancel Culture’: Where Some See Calls for Accountability, Others See Censorship, Punishment
Social media fact sheet, digital knowledge quiz, video: how do americans define online harassment.
1615 L St. NW, Suite 800 Washington, DC 20036 USA (+1) 202-419-4300 | Main (+1) 202-857-8562 | Fax (+1) 202-419-4372 | Media Inquiries
Research Topics
- Email Newsletters
ABOUT PEW RESEARCH CENTER Pew Research Center is a nonpartisan fact tank that informs the public about the issues, attitudes and trends shaping the world. It conducts public opinion polling, demographic research, media content analysis and other empirical social science research. Pew Research Center does not take policy positions. It is a subsidiary of The Pew Charitable Trusts .
© 2024 Pew Research Center
Applying the 6E learning by design model to support student teachers to integrate artificial intelligence applications in their classroom
- Open access
- Published: 04 June 2024
Cite this article
You have full access to this open access article
- Musa Saimon ORCID: orcid.org/0000-0003-4758-8270 1 ,
- Fredrick Mtenzi 2 ,
- Zsolt Lavicza 1 ,
- Kristóf Fenyvesi 3 ,
- Maik Arnold 4 &
- José Manuel Diego-Mantecón 5
423 Accesses
Explore all metrics
The 6E Learning by Design (LbD) model can enhance student teachers’ development of competence for integrating technologies in the classrooms including Artificial Intelligence (AI). However, teacher educators rarely use the 6E LbD model in supporting and encouraging student teachers to integrate AI applications in their classrooms effectively. To attract teacher educators to use the 6E LbD model, in the present study, we modeled learning activities for each phase. We also examined the impact of the 6E LbD model in supporting student teachers to integrate AI in the classroom. We adopted a Participatory Action Research approach implemented in two cycles. We collected data from 35 student teachers who were selected purposively. We collected data through observation, reflective journals and document analysis. We observed student teachers during the classroom activities and analysed their lesson plans and reflective journals, and interpreted data based on a thematic analysis. Findings show that there are various activities facilitating learning in different phases of the 6E LbD model. Also, findings show that the 6E LbD enables student teachers to develop skills and competence for integrating AI in their classrooms. The findings show that an evidence-based approach will motivate teacher educators to use the 6E LbD model.
Similar content being viewed by others
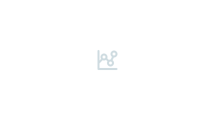
Students’ voices on generative AI: perceptions, benefits, and challenges in higher education
Ai and education: the importance of teacher and student relations.
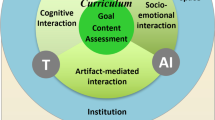
Learning design to support student-AI collaboration: perspectives of leading teachers for AI in education
Avoid common mistakes on your manuscript.
1 Introduction
Artificial intelligence (AI) technology has the potential to transform the education industry. Automated Scoring, Tutoring Systems, Adaptive Learning and Automatic Writing Systems are just a few examples of forms of AI that can enhance assessment, and personalized learning opportunities among others (Celik, 2023 ; Zhai et al., 2021 ). Al Darayseh ( 2023 , p. 1) posits,
It is necessary to emphasize the great potential offered by AI for use in education through the Internet and the accompanying vast developments that have created ease of access for students and teachers to the information they need and want to obtain.
This indicates the extent to which integrating AI in the teaching and learning process is unavoidably necessary.
Despite the potential of AI to transform the teaching and learning process, previous studies have shown that education, so far, is one of the fields that most negligible benefit from AI (Celik, 2023 ; Salas-Pilco et al., 2022 ; Shum & Luckin, 2019 ). For instance, Salas-Pilco et al. ( 2022 ) argue that the application of AI in data analytics in education is very low compared to finance, industry and medicine. Lack of teachers’ skills to integrate AI in education is cited to be among the causes of failure to harness the potential of AI in education (Franzoni et al., 2020 ; Kim et al., 2022 ; Kohnke et al., 2023 ; Lee & Perret, 2022 ; Nazaretsky et al., 2022 ; Utterberg Modén et al., 2021 ). For instance, Kohnke et al. ( 2023 ) observed that the majority of language teachers are unconsciously familiar with AI applications and they lack confidence to integrate AI int their classroom. These authors observed that teachers embrace AI only if they are capable of resolving challenges related to AI technology. Therefore, this shows how important it is for teacher educators to train teachers to integrate AI in their classrooms. It should be noted that training teachers to integrate technology in the classroom is a complex task that requires teacher educators to be informed of what specific aspects should be addressed to help teachers develop the needed competences. The Technological Pedagogical Content Knowledge (TPCK) framework shows that teachers can effectively integrate technology in the classroom only if they have developed Technological Knowledge TK, Pedagogical Knowledge (PK) and Content Knowledge (CK) (Mishra & Koehler, 2006 ). This framework indicates that skills for integrating technology are beyond teachers’ ability to operate technological tools. As Mishra and Koehra (2005, p.94–95) underscore,
There is more to teacher preparation than training teachers how to use tools—it requires appreciation of the complex set of interrelationships between artifacts, users, tools, and practices. What is needed, we argue, is an approach that helps teachers develop deeper understandings of the nuances and complexities of technology integration.
Furthermore, Celik ( 2023 ) developed Intelligent-TPACK by adding the four ethical aspects, namely Transparency, accountability, inclusiveness and fairness, to the TPACK framework as shown in Fig. 1 . The transparency aspect requires the teacher to justify AI-based decisions. Accountability entails the awareness of the teacher of who are the AI developers. Inclusiveness means the ability of teachers to consider AI accessibility to all students. Fairness means the teachers’ ability to enhance equity in the AI-based learning environment. The Intelligent-TPACK offers insights into how training teachers to integrate AI could be more complex compared to preparing them for integrating other technologies. Yet the framework does not answer the question of how successfully teacher educators can prepare teachers to cope with this complex task.
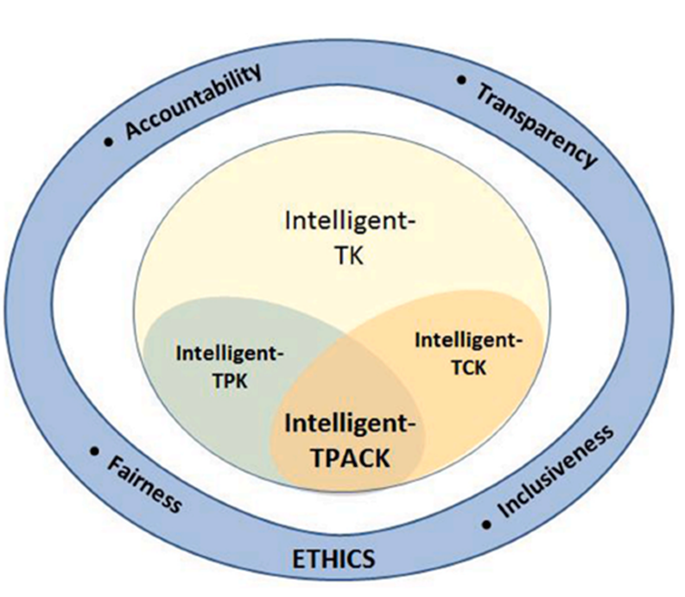
Intelligent-TPACK
Source: (Cellik, 2023, p.8)
Literature shows that Learning by Design is one of the best approaches to training teachers to use technology in the classroom (An et al., 2022 ; Celik, 2023 ; Koehler & Mishra, 2005 ). Learning by design allows teachers to practice what they learn and thus internalize skills and knowledge (Koehler & Mishra, 2005 ). Furthermore, it helps them develop real-life problem-solving skills (An et al., 2022 ; Koehler & Mishra, 2005 ; Utterberg Modén et al., 2021 ). This implies the potential of this learning approach to help teachers customize solutions they develop in the classroom for any problem they may encounter. Therefore, there is a need for teacher education to adopt LbD models in preparing teachers to integrate AI in the classroom.
The 6E Learning by Design (LbD) is one of the models used in coping with complex teaching learning demands in various contexts including in teacher education (Gholam, 2019 ; Khaeroningtyas et al., 2016 ; Lin et al., 2020 ). Footnote 1 However, there is a lack of evidence on the application of this model in preparing teachers to integrate AI in their classrooms. Teacher educators may not be convinced of the impact of this model and/or are not conversant with the application of the model in preparing teachers to integrate AI in their classrooms. We believe that hesitation to apply this model in teacher preparation courses may exacerbate the existing gap in harnessing the potential of AI in the education industry. Therefore, to rescue the situation, the present study shows how the 6E LbD model can be applied in preparing teachers to integrate AI in the classroom. Specifically, the present study intends to: (a) model learning activities for each phase of the 6E LbD model in preparing teachers to integrate AI in their classroom; (b) examine the impact of the model on teachers’ preparedness to integrate AI in their classroom.
The paper is organized as follows. Section 2 covers a theoretical framework. In Sect. 3 , we conceptualize the 6E LbD model. In Sect. 4 , we discuss empirical studies related to adopting the 6E LbD model in teacher preparations. In Sect. 5 , we present the methodology for the study. We present findings in Sect. 6 . In Sect. 7 , we discuss the study’s findings, and in Sect. 8 we present conclusions and recommendations for the further development of teacher training.
2 Theoretical framework
The proposed study is informed by the social theory of Community of Practice (CoP) as advocated by Wenger ( 2008 ). The main assumption of the learning theory of community practice is that whether in a formal context or informal human beings tend to develop similar interests to pursue certain goals from which they learn from each other collaboratively to meet such a goal. As Farnsworth et al. ( 2016 ) restate, “In other words, learning takes place through our participation in multiple social practices, practices which are formed through pursuing any kind of enterprise overtime.” (p. 2) According to Wenger ( 2008 ), the participation of an individual in a particular activity as a member of the group that engages in such activity creates the sense of belonging to the group in a way that it motivates him or her to invest his/her effort to maintain the bond regardless of the experienced obstacles. This means that learning in this perspective involves attaching the value of gaining potential competence as a social contract for one to remain a respected member of the learning community.
Since the present study aims at sharing successful practices for using the 6E LbD model to prepare teachers to integrate AI in the classroom, it fits in the framework of CoP. We believe that sharing the successful practices for the 6E LbD model will help other educators in implementing teacher preparation courses using the model. Hence, this will improve teacher educators’ community skills and knowledge for adopting the 6E LbD model to prepare teachers to integrate AI into education.
3 6E learning by design model
The 6E LbD model was developed by the International Technology and Engineering Educators Association (ITEEA) (Lin et al., 2020 ; Yazıcı et al., 2022 ). This model is an extension of the 5E instructional model that was developed by the Biological Science Curriculum Study (BSCS) (Lin et al., 2020 ). The 6E model comprises six components including engineering which do not exist in the 5E model. According to Burke ( 2014 ), the 6E LbD model is comprehensive in the sense that it combines the elements of engineering and inquiry, which makes them integrate various disciplines (Technology and Engineering). As Burke ( 2014 , n.p) posits, “6E model provides a student-centred framework that deliberately and purposefully uses the Technology (T) and Engineering (E) dimensions of STEM.” To make it clearer, Burke ( 2014 ) described the six components of the 6E LbD model alongside Engineering process as shown in Table 1 .
Table 1 shows that various phases of this model provide learners with opportunities to understand the problem, find the solution and evaluate it. Based on this, one may argue that the model helps learners to learn about how to solve problems rather than what is the solution to the specific problem, something that enhances learners’ ability to deal with any problem that they may face in the future. Conceiving the 6E LbD model through the lens of Burke ( 2014 ), Yazici et al. ( 2022 , para. 5) posit,
Students are provided with opportunities to realize a deeper understanding of the core problem through the application of concepts during the engineering phase students question and integrate inquiry with engineering concepts to make design decisions in problem solving. While they apply what they have learned to new situations and new problems in the enrichment phase, they understand and use the concepts of design, modelling, resources, systems and ethical values.
In addition, Gholam ( 2019 ) cautions that the elements of the 6E LbD model should be viewed as interactive. This shows how 6E Learning by Design model is relevant to preparing teachers to integrate AI as AI integration is a complex task that requires one to engage in solving problems day by day.
4 The application of learning by design models in technological related teacher preparations
Findings from previous studies show that LbD models enable both pre-service and in-service teachers to develop needed knowledge skills and knowledge for integrating technology in the classroom. Lee and Perret ( 2022 ) worked collaboratively with teachers in the US to design a curriculum for STEM learning. They report that teachers highly rated the project as valuable to their learning. Also, Anabousy and Daher ( 2022 ) observed an improvement in skills for designing STEM learning units among preservice teachers who were involved in LbD training in Israel. In addition, Haas et al. ( 2021 ) reported that hands-on activities helped pre-service teachers develop skills for facilitating Outdoor STEAM activities in the classroom. Although these studies are not focused on training about employing technology, they partly show the usefulness of Learning by Design in preparing teachers to use technology because technology is part of the STEM classroom. In addition, these studies show how the Learning by Desing approach enhances the development of interdisciplinary skills. This is because the ability to facilitate STEM learning implies teachers’ competence in transdisciplinary/interdisciplinary teaching approaches. However, evidence on how the specific model of Learning by Design in the context of preparing teachers to integrate AI is missing. This implies the need for more studies that can fill this gap.
In contrast, Lu ( 2014 ), Makri et al. ( 2014 ) and Lu et al. ( 2011 ) observed positive impacts of Learning by Design in teacher preparation courses based on specific models of Learning by Design. For instance, Lu ( 2014 ) adopted Kolodnen (2003)’s model of Learning by Design in training pre-service teachers. Lu ( 2014 ) reports that the training approach led to improved teachers’ skills in using technology in the classroom. Some of the learning tasks reported in this class include reflection, designing and solving real-life problems. Besides, Lu et al. ( 2011 ) reported that the application of Kolodner (2003)’s model of Learning by Design improved technological-pedagogical knowledge and pedagogical knowledge more than content knowledge among teachers who were being trained in using Microsoft Word, Excel and PowerPoint. On the other hand, Makri et al. ( 2014 ) revealed that the application of Kalantizis and Cope (2012)’s framework in teacher training courses in Greece impacted teachers positively. The activities that were used in this study include exploration and discussions. Despite providing insights into the impacts of specific models of the LbD approach, these studies report on limited models excluding the 6E LbD model. Also, the application of the mentioned model was not on training teachers in the context of integrating. Therefore, since the present study focuses on the 6E LbD model in the context of preparing teachers to embrace AIs in their classrooms, there is no way we can rely on the findings from these studies.
Above all, An et al. ( 2022 ) employed the Learning by Design approach to training teachers to use Robotics AI in the US where they noted improved teachers’ skills in teaching with robotics AI. They reported that teachers developed knowledge and skills related to the content knowledge about robotics technology, constraints of using robotics AI and teaching with robotics. They also reported that their project was accompanied by various learning activities such as Robotics building, Reflective essays, open-ended questions and presentations. In another study from the field of engineering education, Guzmán-Ramírez et al. ( 2019 , p.1237) developed a “field programmable gate array-based educational system” focused on the modeling, implementation, and evaluation of hardware architectures in artificial neural networks based on a LbD approach. The authors conclude that encouraging students and academics to expand their capabilities through designing new modules, especially a flexible course structure supports the effectiveness of learning at the undergraduate level through a learning-by-design approach applied to real-world problems like the iris-plant issue.
These studies show that Learning by Design leads to the successful training of teachers on integrating AI technology in the classroom. However, the study focused on a limited number of AI technologies and said nothing about the 6E LbD model. This implies the need for more studies that focus on 6E LbD in the context of a large number of AIs. Hence the present study is significant in filling this gap.
5 Methodology
5.1 study context.
The study was conducted at one of the higher learning institutions in East Africa involving student teachers who were pursuing master’s degrees in Education specializing in mathematics, Language and Literacy, Education Leadership and Management, Early Childhood Education and Science. The student teachers in this class come from the three East Africa countries namely Uganda, Kenya and Tanzania. These teachers are teaching pre-primary, primary and secondary school. One of the admission requirements in this master’s degree was a minimum of three years of teaching experience. Therefore, all the student teachers regardless of their counry of origin and specialization, they had worked as teachers for at least three years. Furthermore, there is low technology integration in the classroom contexts (Uganda, Kenya and Tanzania) where these student teachers work, despite having educational policies for integrating technology in teaching. Aga Khan university offers the ICT in Education course as a way to support student teachers who join master program to develop competence to integrate technology in their respective countries. This study was part of the intervention in the ICT in education course that these students take in their second semester. The intervention project aimed familiarizing student teachers with AI and developing competence for integrating AI in their classroom. The project covered the whole semester in which there were three contact hours per week.
5.2 Research approach
We adopted the qualitative Participatory Action Research (PAR) approach. Action research intends to modify existing satisfying situations through collaborative initiatives with the community in the relevant setting (Berg & Lune, 2017 ; Burns, 2009 ; James et al., 2008 ; Kemmis et al., 2014 ; Koshy, 2005 ). Laurillard ( 2008 ) argue that teachers as researchers have the responsibility to assess their classroom situation and collaborate with students to improve the teaching and learning process. Our approach aligns with the principles of the PAR since one of the researchers is responsible for facilitating the ICT in Education Course at this university. In this regard, he took the role of ‘the teacher as a researcher’ and collaborated with other researchers and students to improve the teaching and learning process. During the implementation of PAR, we adopted the spiral model of PAR as presented by Kemmis et al. ( 2014 ). This model has four phases, namely planning/reconnaissance, action/intervention, observation, and reflection. The planning stage involve identification of major concerns and developing possible solutions. Intervention phase involves implementing the solution developed from the reconnaissance phase. The observation and reflection phase involves assessing the intervention to draw lessons for possible improvement of the next cycles. These phases are interactive in a way that it allows the researcher to go back and forth within a certain cycle setting. Figure 2 illustrates the interactive nature of these phases. Moreover, PAR can go through several cycles; however, given the limited time, we implemented PAR with two cycles only.
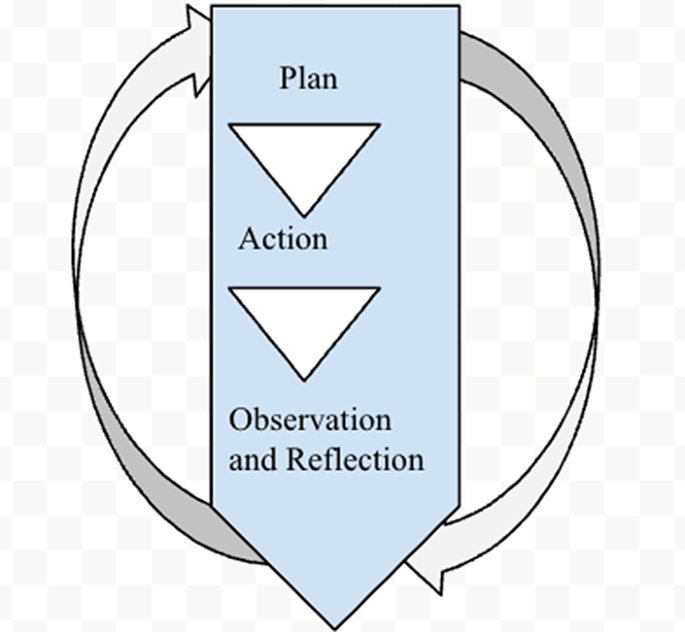
Phases of PAR
5.3 Participants, data collection methods, and analysis
We collected data through observation, document analysis and reflective journals from students. Data collection was conducted within 12 weeks of the second semester starting from May, 2023 to July 2023. We observed students when they were working in the classroom. We analyzed student teachers’ lesson plans and reflections. Only students who took part qualified to be part of the study. Thus, we used a purposive sampling technique based on the involvement criteria. The class has a total number of 69 student teachers. However, we collected data for this study from 35 student teachers only. The number of student teachers to participate in this study was determined by the data saturation point. The data saturation point is when the researcher finds no new emerging themes from the respondents (Hennink & Kaiser, 2022 ). We renamed the participants as STUDENT TEACHERS I-XXXV to hide their identity.
Finally, we analyzed data thematically based on the framework proposed by Auerbach and Silverstein ( 2003 ), Miles et al. ( 2014 ) and Miles and Huberman ( 1994 ). Based on this framework, we coded, sorted, consolidated data and developed themes. Finally, these themes were verified through relating them to theories and views of participants. In the coding stage, we coded data collected through document analysis, and reflective journals manually by assigning different labels to data. In contrast, data from observation of student teachers’ interaction with peers and Ais were coded separately because we used a checklist, which enabled us to collect and code them simultaneously throughout the project course. We classified the coded data from document analysis, observation, and reflective journals based on their relationship by using tables. We finally developed themes for each of the classifications of the data and related these themes to theories that informed the study. To ensure the rigor of the data analysis, we analysed data in two separate groups and later exchanged the analysis results for peer review. In case of differences, we discussed until we found consensus.
5.4 The PAR implementation
The implementation of the PAR is presented in the Tables 2 and 3 for the two cycles.
The present study was guided by two research objectives: (a) modeling the teaching-learning activities for each phase of the 6E LbD model and (b) examining the impact of the 6E LbD model in supporting student teachers to integrate AI in their classrooms. In this section, we present findings thematically based on the research objectives.
6.1 Activities for each phase of the 6E LbD
Sharing real-life narratives about the impacts of embracing or neglecting AI attracts the interest of student teachers. The narrative about the researcher’s personal experience with the introduction of cryptocurrency, and the experience of the Italian government on ChatGPT (McCallum, 2023 ) control made student teachers curious to learn about the integration of AI in the classroom. This was evident from their reflections as STUDENT TEACHER I reflected, “In summary, the session was an eye opener since from it, it was discovered that the use of AI in education cannot be ignored. If the educationists will, other sectors will use it alongside other digital hardware and software.” This shows the extent to which such narratives awakened student teachers. In addition, providing opportunities for student teachers to practice technologies in contexts where they use traditional means was identified as a catalyst for student teachers’ interest in the lesson. It was observed during the exercise that student teachers developed more questions and they looked excited to learn more practices with technologies, especially in their classroom. It was also evident from their reflections, as STUDENT TEACHER V reflected, “The transition from written responses to audio or video submissions was a significant shift for many of us. It required embracing new technologies and overcoming the initial discomfort of speaking or appearing on camera.” This shows that the exercise enhanced student teachers’ change in attitude towards using technologies.
Moreover, allowing student teachers to interact with AI for their interests, and exposing student teachers to various AI are useful activities for the Exploration phase. Data analysis indicates that these activities allow student teachers to experiment with AIs. As STUDENT TEACHER XX reflected, “ I learned how a variety of AIs can be utilized in the spheres of academic and research writing. The use of ChatGPT, perplexity, Elicit, and Connected papers to search and consolidate relevant data was amazing” Based on this reflection, it is evident that these activities were helpful for student teachers to determine the strengths and weaknesses of each AI appropriately.
On the other hand, providing face-to-face and online opportunities for student teachers to share their reflections is useful for the Explaining phase. Data analysis indicates that both face-to-face and online channels allow student teachers to explain their questions, discoveries and answers to questions they developed before. For instance, from the online channel, STUDENT TEACHER XXV explained,
In my presentation, I discussed how I integrated ChatGPT into my lesson preparation, coupled with the use of Tome AI—an application also built upon ChatGPT—to generate accompanying slides…. As an educator, the input we provide to these AI systems plays a pivotal role in shaping the outcomes they produce. It’s important to recognize that these AI tools are not infallible. Hence, the responsibility rests with the teacher to customize and tailor the materials to best suit the needs of their learners.
Therefore, this shows how the provision of a reflection channel enhances learning in the Explain phase where student teachers are required to share their discoveries.
Besides, providing micro-teaching sessions and peer learning sessions among student teachers enhances learning among teachers in the Engineering phase. Data analysis indicates that these activities engage student teachers in solving problems through engineering processes such as planning, designing and evaluating. It was observed that students had to go through various stages such as decision-making and evaluation to develop the AI-integrated lesson for the micro-teaching sessions. Similarly, peer teaching requires engaging in various engineering-related aspects before producing the lesson plan for the session. The influence of micro-teaching sessions was evident from student teachers’ reflections. As STUDENT TEACHER XXI reflected,
One experiencing moment was an assignment where we went off as a group, because of not understanding it clearly. It was a reflecting moment whereby any teacher who wants to integrate technology into his/her teaching, has to ask himself/herself several questions before integration to enhance the teaching and learning practices.
Therefore, this reflection shows how valuable the micro-teaching exercise challenged student teachers in a way that they needed to make informed judgments about the choice of their AI.
Furthermore, data analysis indicates that developing lesson plans is useful for the Enriching phase. Through this exercise student teachers deepen their understanding of integrating AI in the classroom. The lesson development was enriching to student teachers because it required them to synthesize theoretical knowledge from teaching and technological frameworks. The lesson plans developed showed the coherence between the choice of AI, learning content, pedagogy and expected competence. Also, student teachers’ reflections showed that the exercise influenced student teachers to deepen their knowledge. For instance, STUDENT TEACHER II reflected, “ It was found out that pedagogical considerations should be taken into account when using the AIs in education since their wrong use can be detrimental.” Therefore, it is clear that the development of lesson plans enables student teachers to recognise issues that one should consider to ensure the successful integration of AI in the classroom.
Above all, providing channels for peer feedback is identified as one of the most valuable activities for enhancing learning in the Evaluation phase. Data analysis indicates that peer feedback enabled student teachers to evaluate their lesson plans. During the feedback session, we observed student teachers taking notes and others requesting more clarifications for feedback given by their peers that they can use for improving their lesson plans. Also, this was evident from the reflections, as STUDENT TEACHER XXXII reflected, “ This message [the peer feedback] led to personal reflection, prompting me to utilize AIs more effectively in future teaching scenarios for enhanced learner engagement and ethical use.” Therefore, this shows that providing student teachers with access to peer feedback enables them to evaluate their approaches to integrating AIs in their classrooms.
6.2 The impact of the 6E LbD model on Student teachers to integrate AI in the classrooms
Data analysis indicates that the 6E LbD model facilitates student teachers to develop competence for integrating AI in their classrooms. It was noted that student teachers developed lesson plans that align with theoretical frameworks for integrating AI in the classrooms. Also, some student teachers’ reflections showed they have developed the competence to integrate AI in the classrooms. For instance, STUDENT TEACHER IX stated,
While AI tools can be useful, there are some potential ethical concerns. AI models can have issues with fairness, bias, and transparency which could impact the information they provide. As with any information source, it is important to verify AI output and exercise good judgment.
STUDENT TEACHER XVIII also reflected, “ Overall, the use of all these applications must be tailored to the teacher’s pedagogical content.” This shows how competent these student teachers are in integrating AIs into the classrooms. Therefore, one of the impacts of the 6E LbD model is to develop competence for integrating AI in the classroom.
Another impact of the 6E LbD model in supporting student teachers to integrate AI in the classroom was the familiarization with various AI. Data analysis indicates that the use of this model enabled student teachers to be familiar with various AIs. It was observed during preparations for micro-teaching that student teachers used a variety of AIs. Also, this was alluded to in their reflections as STUDENT TEACHER XIX posted,
I learned about the AI tools: ChatGPT, Perplexity, Elicit, and Connected papers. What I learned is that every AI has specific functions and that AI tools give answers to the right questions, sometimes AI tools may provide mismatch responses. What we have to do is to monitor the AI tools, for example, ChatGPT.
This shows that the student teacher is familiar with various AIs and knows how each of them functions.
The development of skills to use AIs among student teachers is another impact of employing the 6E LbD model in supporting student teachers to integrate AI in the classroom. Data analysis indicates that student teachers developed skills on how to interact to optimize its output. Analysis of lesson plans shows that student teachers used AI to achieve different purposes such as creating images and accessing notes and they have a good plan for how to use it to engage learners. Therefore, it is evident that student teachers developed skills to interact with AIs successfully.
In addition, the 6E LbD model impacted student teachers by motivating them to employ AIs in their classrooms. Reflections from student teachers showed that they developed a curiosity to adopt AIs in their working stations. For instance, STUDENT TEACHER XXIII stated,
And I reflected deeply about it and found that I had been underutilizing the AIs. I shall use the AIs in future for learner engagement in activities that I would not have done without the AI. For example, in the absence of a resource for teaching and the AI can generate it.
This shows the determination of the student teacher to integrate AI in his/her workplace as a result of learning through the 6E LbD model.
The development of problem-solving skills such as critical thinking, communication and collaboration is one of the impacts of learning through the 6E LbD model. Data analysis indicates that student teachers developed problem-solving skills as they engaged in various learning activities and applied the information gathering and exploration methods previously acquired to the AI-related topics in the course. It was observed during the presentation that student teachers were able to share feedback constructively. This indicates the development of collaboration and communication skills. Apart from that, data from lesson plans and reflections show that student teachers were making informed decisions on the types of AI to integrate to serve specific purposes. STUDENT TEACHER XXXI stated, “ As I pondered these matters, I realized that the incorporation of AI into education should be guided by a strong ethical framework.” This shows that student teachers employed critical thinking in determining the solution for integrating AI in the classroom successfully.
7 Discussion
The findings show that the modelled activities can enhance learning in various phases of the 6E LbD model among student teachers and that this model provides an effective guideline to the integration of AI into the classroom. This implies the possibility of attracting other teacher educators to use the model, especially those who had been hesitant to use it because of unfamiliarity with the relevant activities to employ in the phases of the model. Based on the theory of CoP, people will always strive to participate in certain activities to maintain a bond with the community they belong to (Wenger, 2008 ; Farnsworth et al., 2016 ). Therefore, the modeled activities may act as the catalyst for teacher educators who wish to use the 6E LbD model in supporting student teachers’ integrated AI in their classrooms.
However, the modeled activities should be regarded as interactive in a way that one cannot draw the line between them. The interactivity of the phases (Gholam, 2019 ) might be the main reason for the inseparability of the activities. The interactive nature of the activities makes it possible for one activity to be influential in more than one phase. For instance, allowing student teachers to share their reflections can influence the exploration, explanation and evaluation phases. This is because student teachers are likely to make some exploration from which their reflection will come. In addition, once student teachers share their reflection (Explaining phase) they may get some feedback from colleagues that can help them refine their previous understanding, which brings in the aspects of the Evaluation phase. Therefore, this indicates the extent to which the activities for various phases are inseparable.
The findings on the modeled activities for the phases of the 6E LbD model make the present study slightly similar to the previous studies such as (Lu, 2014 ; Makri et al., 2014 , An, 2022 ). The learning activities reported in the previous studies such as exploration and discussion (Makri et al., 2014 ), robotics building and reflections (An et al., 2022 ), and reflection and designing (Lu, 2014 ) correspond to learning activities reported in the present study. This similarity may be caused by the fact that both studies come from student teachers’ learning contexts that adopted LbD models.
On the other hand, these findings differentiate the present study from the previous ones due to the fact that previous studies involved only models of LbD that are different from the 6E LbD model. For instance, the study conducted by Lu ( 2014 ) reported learning activities related to Kolodnen (2003)’s model and the one conducted by Makri et al. ( 2014 ) adopted the Kalantizis and Cope (2012)’s framework. This difference is attributed to the difference in focus of the studies on models of LbD, especially regarding the various dimensions of the student teacher learning process.
Apart from the findings on the modeled activities for phases of the 6E LbD model, this study also shows that the impacts of the 6E LbD model include the development of competence for integrating AI, problem-solving skills and skills for interacting with AI among student teachers. This means that the model is useful for supporting student teachers to integrate AI in the classrooms. This aligns with the consensus among various scholars (Koehler & Mishra, 2005 ; An et al., 2022 ; Utterberg Modern, 202) that LbD is the most useful for developing teachers’ competence to integrate technology in the classroom. LbD models are useful because they enable teachers to deal with complex teaching contexts (Khaeroningtyas et al., 2016 ; Lin, 2020 ; Gholam, 2019 ). Therefore, teacher educators should consider employing the 6E LbD model to cater to the complexities that student teachers may face in integrating AI in their classrooms.
These findings are similar to those from previous studies (Lee & Perret, 2022 ; Anabousy & Daher, 2022 ) in that they all report LbD as helpful in training teachers to integrate technology in the classrooms. However, unlike the previous studies, the present studies focused on the 6E LbD model for supporting teachers to integrate AI in the classrooms. The difference could be attributed to the difference in the focus of the studies. While the previous studies focused on developing teachers’ competence in integrating technologies through LbD models, the present studies were confined to the specific model (6E). Therefore, the findings from the present study are similar and different to the previous study in various aspects.
Overall, we argue that the present study aligns with previous studies in the sense that it also shows the significant contribution of LbD models in training teachers to integrate technologies in the classrooms. However, its uniqueness lies in the fact that the study focused on the impact of the 6E LbD model on Artificial Intelligence in Education (AIED), which to our knowledge has not yet thoroughly been addressed in the previous research. In addition, despite 6E LbD being reported in the previous studies, none of the previous studies modeled the learning activities in each phase of the model, especially concerning training teachers to integrate AI in the classrooms. Therefore, the unique contribution of the present study is not only advocating the use LbD model but also showing the specific LbD model (the 6E model) and the potential tools (modeled activities) for using the model in supporting the integration of AI in teaching and learning.
8 Conclusions and recommendations
The present study modeled activities for each phase of the 6E LbD model and examined the impact of the model in supporting teachers to integrate AI in the classroom. Sharing real-life narratives, providing reflection opportunities and practising micro-teaching are some of the modeled activities for the different phases of the model. It has also been observed that the model enables student teachers to develop competence for integrating AI, familiarity with AIs, problem-solving skills and skills to interact with AIs. Based on these findings, teacher educators may use modeled activities as their starting point to adopt the 6E LbD model in supporting their student teachers in integrating AIs in the classrooms. Additionally, these findings are potential evidence for the usefulness of the 6E LbD model in supporting student teachers to integrate AIs in their classrooms. Therefore, we urge teacher educators to employ the model in their training to support student teachers in developing desirable competence for integrating AI in their classrooms. Further, we call for further research on the use of LbD models to support teachers in integrating AI in the classrooms to broaden teacher educators’ chances to support student teachers such as based on comparisons to other models, longitudinal studies, or adaptations to other learning and teaching contexts.
Data availability
The datasets used in the current study are available from the authors upon reasonable request.
As LbD is a project-based inquiry in learning, there is a family resemblance to other design-based teaching and research approaches such as, for example, that of The Design-based Research Collective ( 2003 ).
Al Darayseh, A. (2023). Acceptance of artificial intelligence in teaching science: Science teachers’ perspective. Computers and Education: Artificial Intelligence , 4 , 100132.
Google Scholar
An, H., Sung, W., & Yoon, S. Y. (2022). Implementation of learning by design in a synchronized online environment to teach educational robotics to inservice teachers. Educational Technology Research and Development , 70 (4), 1473–1496.
Article Google Scholar
Anabousy, A., & Daher, W. (2022). Prospective teachers’ design of Steam learning units: Steam capabilities’ analysis. Jotse , 12 (2), 529–546.
Auerbach, C. F., & Silverstein, L. B. (2003). Qualitative data: An introduction to coding and analysis . New York University.
Berg, B. L., & Lune, H. (2017). Qualitative research methods for the social sciences (Ninth edition) . Pearson.
Burke, D. T. E. (2014). The ITEEA 6E learning bydesign (TM) model maximizing informed design and inquiry in the integrative STEM classroom. Technology and Engineering Teacher , 73 (6), 14–19.
Burns, A. (2009). Doing Action Research in English Language Teaching: A Guide for Practitioners (1st ed.). Routledge. https://doi.org/10.4324/9780203863466 .
Celik, I. (2023). Towards Intelligent-TPACK: An empirical study on teachers’ professional knowledge to ethically integrate artificial intelligence (AI)-based tools into education. Computers in Human Behavior , 138 , 107468.
Farnsworth, V., Kleanthous, I., & Wenger-Trayner, E. (2016). Communities of practice as a social theory of learning: A conversation with Etienne Wenger. British Journal of Educational Studies , 64 (2), 139–160.
Franzoni, V., Milani, A., Mengoni, P., & Piccinato, F. (2020). Artificial intelligence visual metaphors in e-learning interfaces for learning analytics. Applied Sciences , 10 (20), 7195.
Gholam, A. P. (2019). Inquiry-based learning: Student teachers’ challenges and perceptions. Journal of Inquiry and Action in Education , 10 (2), 6.
Guzmán-Ramírez, E., Garcia, I., & García‐Juárez, M. (2019). A learning by design application for modeling, implementing, and evaluating hardware architectures for artificial neural networks at undergraduate level. Computer Applications in Engineering Education , 27 (5), 1236–1252. https://doi.org/10.1002/cae.22148 .
Haas, B., Kreis, Y., & Lavicza, Z. (2021). Integrated STEAM Approach in Outdoor Trails with Elementary School Pre-service Teachers. Educational Technology & Society , 24 (4), 205–219.
Hennink, M., & Kaiser, B. N. (2022). Sample sizes for saturation in qualitative research: A systematic review of empirical tests. Social Science & Medicine , 292 , 114523. https://doi.org/10.1016/j.socscimed.2021.114523 .
James, E. A., Milenkiewicz, M. T., & Bucknam, A. (2008). Participatory action research for educational leadership: Using data-driven decision making to improve schools . Sage.
Kemmis, S., McTaggart, R., & Nixon, R. (2014). The Action Research Planner. Springer Singapore. https://doi.org/10.1007/978-981-4560-67-2 .
Khaeroningtyas, N., Permanasari, A., & Hamidah, I. (2016). Stem learning in material of temperature and its change to improve scientific literacy of junior high school. Jurnal Pendidikan IPA Indonesia , 5 (1), 94–100.
Kim, J., Lee, H., & Cho, Y. H. (2022). Learning design to support student-AI collaboration: Perspectives of leading teachers for AI in education. Education and Information Technologies , 27 (5), 6069–6104.
Koehler, M. J., & Mishra, P. (2005). Teachers learning technology by design. Journal of Computing in Teacher Education , 21 (3), 94–102.
Kohnke, L., Moorhouse, B. L., & Zou, D. (2023). Exploring generative artificial intelligence preparedness among university language instructors: A case study. Computers and Education: Artificial Intelligence , 5 , 100156.
Koshy, V. (2005). Action research for improving practice: A practical guide . PCP/Sage.
Laurillard, D. (2008). The teacher as action researcher: Using technology to capture pedagogic form. Studies in Higher Education , 33 (2), 139–154.
Lee, I., & Perret, B. (2022). Preparing High School Teachers to Integrate AI Methods into STEM Classrooms. Proceedings of the AAAI Conference on Artificial Intelligence, 36(11), 12783–12791.
Lin, K. Y., Hsiao, H. S., Williams, P. J., & Chen, Y. H. (2020). Effects of 6E-oriented STEM practical activities in cultivating middle school students’ attitudes toward technology and technological inquiry ability. Research in Science & Technological Education , 38 (1), 1–18.
Lu, L. (2014). Learning by design: Technology preparation for digital native preservice teachers [PhD Thesis]. Syracuse University.
Lu, L., Johnson, L., Tolley, L., Gilliard-Cook, T., & Lei, J. (2011). Learning by design: TPACK in action. Society for Information Technology & Teacher Education International Conference, 4388–4395.
Makri, K., Papanikolaou, K., & Tsakiri, A. (2014). Blending the community of inquiry framework with learning by design: Towards a synthesis for blended learning in teacher training. Electronic Journal of E-Learning , 12 (2), 183–194.
McCallum, S. (2023, April 1). CHATGPT banned in Italy over privacy concerns . BBC News. https://www.bbc.com/news/technology-65139406 .
Miles, M. B., & Huberman, A. M. (1994). Qualitative data analysis: An expanded sourcebook (2nd ed.). Sage.
Miles, M. B., Huberman, A. M., & Saldaña, J. (2014). Qualitative data analysis: A methods Sourcebook (Third Edition) . SAGE Publications, Inc.
Mishra, P., & Koehler, M. J. (2006). Technological Pedagogical Content Knowledge: A Framework for teacher knowledge. Teachers College Record , 108 (6), 1017–1054. https://doi.org/10.1111/j.1467-9620.2006.00684.x .
Nazaretsky, T., Cukurova, M., & Alexandron, G. (2022). An instrument for measuring teachers’ trust in AI-based educational technology. LAK22: 12th International Learning Analytics and Knowledge Conference, 56–66.
Salas-Pilco, S. Z., Xiao, K., & Hu, X. (2022). Artificial intelligence and learning analytics in teacher education: A systematic review. Education Sciences , 12 (8), 569.
Shum, S. J. B., & Luckin, R. (2019). Learning analytics and AI: Politics, pedagogy and practices. British Journal of Educational Technology , 50 (6), 2785–2793.
The Design-Based Research Collective. (2003). Design-based research: An emerging paradigm for Educational Inquiry. Educational Researcher , 32 (1), 5–8. https://doi.org/10.3102/0013189X032001005 .
Utterberg Modén, M., Tallvid, M., Lundin, J., & Lindström, B. (2021). Intelligent tutoring systems: Why teachers abandoned a technology aimed at automating teaching processes .
Wenger, E. (2008). Communities of practice: Learning, meaning, and identity . Cambridge Univ. Press.
Yazıcı, Y. Y., Hacıoğlu, Y., & Sarı, U. (2022). Entrepreneurship, STEM attitude, and career interest development through 6E learning byDeSIGN™ model based STEM education. International Journal of Technology and Design Education , 1–21.
Zhai, X., Chu, X., Chai, C. S., Jong, M. S. Y., Istenic, A., Spector, M., Liu, J. B., Yuan, J., & Li, Y. (2021). A review of Artificial Intelligence (AI) in education from 2010 to 2020. Complexity , 2021 , 1–18.
Download references
Open access funding provided by Johannes Kepler University Linz.
Author information
Authors and affiliations.
Johannes Kepler Universität Linz, Linz, Austria
Musa Saimon & Zsolt Lavicza
Institute for Educational Development, Aga Khan University, Dares Salaam, Tanzania
Fredrick Mtenzi
University of Jyväskylä, Jyväskylä, Finland
Kristóf Fenyvesi
Fachhochschule Dresden, Dresden, Germany
Maik Arnold
University of Cantabria, Santander, Spain
José Manuel Diego-Mantecón
You can also search for this author in PubMed Google Scholar
Corresponding author
Correspondence to Musa Saimon .
Ethics declarations
Conflict of interest.
The authors have no competing interests to declare that are relevant to the content of this article.
Additional information
Publisher’s note.
Springer Nature remains neutral with regard to jurisdictional claims in published maps and institutional affiliations.
Rights and permissions
Open Access This article is licensed under a Creative Commons Attribution 4.0 International License, which permits use, sharing, adaptation, distribution and reproduction in any medium or format, as long as you give appropriate credit to the original author(s) and the source, provide a link to the Creative Commons licence, and indicate if changes were made. The images or other third party material in this article are included in the article’s Creative Commons licence, unless indicated otherwise in a credit line to the material. If material is not included in the article’s Creative Commons licence and your intended use is not permitted by statutory regulation or exceeds the permitted use, you will need to obtain permission directly from the copyright holder. To view a copy of this licence, visit http://creativecommons.org/licenses/by/4.0/ .
Reprints and permissions
About this article
Saimon, M., Mtenzi, F., Lavicza, Z. et al. Applying the 6E learning by design model to support student teachers to integrate artificial intelligence applications in their classroom. Educ Inf Technol (2024). https://doi.org/10.1007/s10639-024-12795-9
Download citation
Received : 13 February 2024
Accepted : 15 May 2024
Published : 04 June 2024
DOI : https://doi.org/10.1007/s10639-024-12795-9
Share this article
Anyone you share the following link with will be able to read this content:
Sorry, a shareable link is not currently available for this article.
Provided by the Springer Nature SharedIt content-sharing initiative
- Artificial intelligence
- 6E learning by desing model
- Student teachers
- Teacher educators
- Find a journal
- Publish with us
- Track your research
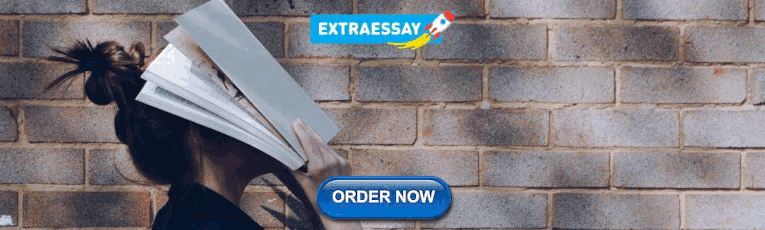
IMAGES
VIDEO
COMMENTS
Book Title: Artificial Intelligence in Education: Emerging Technologies, Models and Applications. Book Subtitle: Proceedings of 2021 2nd International Conference on Artificial Intelligence in Education Technology. Editors: Eric C. K. Cheng, Rekha B. Koul, Tianchong Wang, Xinguo Yu
Request PDF | Artificial Intelligence in Education: Emerging Technologies, Models and Applications | This edited book is a collection of selected research papers presented at the 2021 2nd ...
This edited book is a collection of selected research papers presented at the 2021 2nd International Conference on Artificial Intelligence in Education Technology (AIET 2021), held in Wuhan, China on July 2-4, 2021. AIET establishes a platform for AI in education researchers to present research, exchange innovative ideas, propose new models, as well as demonstrate advanced methodologies and ...
This timely publication is well-aligned with UNESCO's Beijing Consensus on Artificial Intelligence (AI) and Education. It is committed to exploring how best to prepare our students and harness emerging technologies for achieving the Education 2030 Agenda as we move towards an era in which AI is transforming many aspects of our lives.
1. Introduction. Information technologies, particularly artificial intelligence (AI), are revolutionizing modern education. AI algorithms and educational robots are now integral to learning management and training systems, providing support for a wide array of teaching and learning activities (Costa et al., 2017, García et al., 2007).Numerous applications of AI in education (AIED) have emerged.
Artificial intelligence in education: Emerging technologies, models and applications Chi Keung Eric CHENG (Editor), Rekha B. KOUL (Editor), Tianchong WANG (Editor), Xinguo YU (Editor) Department of Curriculum and Instruction (C&I)
The Digital and eTextbook ISBNs for Artificial Intelligence in Education: Emerging Technologies, Models and Applications are 9789811675270, 9811675279 and the print ISBNs are 9789811675263, 9811675260. Save up to 80% versus print by going digital with VitalSource.
2.1 Prolific countries. Artificial intelligence in education (AIEd) research has been conducted in many countries around the world. The 40 articles reported AIEd research studies in 16 countries (See Table 1).USA was so far the most prolific, with nine articles meeting all criteria applied in this study, and noticeably seven of them were conducted in K-12.
Addressing varied unfinished learning of students due to the pandemic is a policy priority, and AI may improve the adaptivity of learning resources to students' strengths and needs. Improving teaching jobs is a priority, and via automated assistants or other tools, AI may provide teachers greater support.
UNESCO is committed to supporting Member States to harness the potential of AI technologies for achieving the Education 2030 Agenda, while ensuring that its application in educational contexts is guided by the core principles of inclusion and equity. UNESCO's mandate calls inherently for a human-centred approach to AI.
Artificial intelligence (AI) applications in education are on the rise and have received a lot of attention in the last couple of years. AI and adaptive learning technologies are prominently featured as important developments in educational technology in the 2018 Horizon report (Educause, 2018), with a time to adoption of 2 or 3 years.According to the report, experts anticipate AI in education ...
Artificial Intelligence Applications and Technologies. AI-directed learning applies techniques such as fuzzy logic, decision trees, neural networks, Bayesian networks, and hidden Markova models to support electronic learning (e-learning) and various forms of education and training (Colchester et al. Citation 2017).The constructivist learning paradigm supports learner-centered and learner ...
Artificial intelligence and generative models such as ChatGPT accounts for the new requirements and convenience of learning and at least in theory, can embody a learner's socio-cultural background. However, this AI transition has magnified many pedagogical issues related to quality including the digital divide between those who have access to ...
The application of artificial intelligence (AI) in STEM education (AI-STEM), as an emerging field, is confronted with a challenge of integrating diverse AI techniques and complex educational elements to meet instructional and learning needs. To gain a comprehensive understanding of AI applications in STEM education, this study conducted a systematic review to examine 63 empirical AI-STEM ...
The use of artificial intelligence (AI) in higher education (HE) has risen quickly in the last 5 years (Chu et al., 2022), with a concomitant proliferation of new AI tools available.Scholars (viz., Chen et al., 2020; Crompton et al., 2020, 2021) report on the affordances of AI to both instructors and students in HE.These benefits include the use of AI in HE to adapt instruction to the needs of ...
This paper reports on a scoping review of research studies on artificial intelligence in education (AIED) published over the last two decades (2001-2021). ... Emerging Technologies, Models and Applications. ... R.B., Wang, T., Yu, X. (eds) Artificial Intelligence in Education: Emerging Technologies, Models and Applications. AIET 2021. Lecture ...
Artificial Intelligence (AI) in education, particularly at the university level, has been an active area for several years now, ranging from intelligent tutoring systems to learning analytics support [34, 22].However, the recent rise in popularity of large language models (LLMs) and chatbots has already disrupted teaching principles in many programming and software engineering (SE) courses.
Artificial Intelligence (AI) approaches have been incorporated into modern learning environments and software engineering (SE) courses and curricula for several years. However, with the significant rise in popularity of large language models (LLMs) in general, and OpenAI's LLM-powered chatbot ChatGPT in particular in the last year, educators are faced with rapidly changing classroom ...
Advances in artificial intelligence (AI) could transform education systems and make them more equitable. It can accelerate the long overdue transformation of education systems towards inclusive learning that will prepare young people to thrive and shape a better future.; At the same time, teachers can use these technologies to enhance their teaching practice and professional experience.
Artificial Intelligence. The Office of Educational Technology is working to develop policies and supports focused on the effective, safe, and fair use of AI-enabled educational technology. Today, many priorities for improvements to teaching and learning are unmet. Educators seek technology-enhanced approaches addressing these priorities that ...
In this paper we discuss the concept of Artificial Intelligence in Education and present a literature review related to smart classroom technology, with an emphasis on emerging technologies such as AI-related technologies. ... has begun to influence the application of technology to instruction and learning, to improve the learning process, and ...
Theoretically, this meta-analysis provided a panoramic picture of two leading technology acceptance models regarding the acceptance/adoption of AI and AI-based technology in education sector. This meta-analysis provided a way forward for researchers to extend research on AI-based applications including ChatGPT, intelligent tutoring, AI-based ...
Artificial Intelligence in Education has very well-developed basic themes. Artificial Intelligence is the emerging motor theme. The trend topics are also evolving at a very fast pace, given the rate of development in AI. The current trend topics include - Chat GPT, Generative Artificial Intelligence, and Ethics. Finally, the study develops a ...
Three paradigms are proposed for Artificial Intelligence in Education (AIED). • Three paradigms are AI-directed, AI-supported, and AI-empowered. • AI is used to represent knowledge models and direct cognitive learning in Paradigm One. • AI is used to support learning while learners work as collaborators with AI in Paradigm Two. •
The 6E Learning by Design (LbD) model can enhance student teachers' development of competence for integrating technologies in the classrooms including Artificial Intelligence (AI).
Incorporating artificial intelligence (AI) into learning platforms has the potential to transform teaching and learning in the future generation of education. Using tailored learning paths ...
Machine learning definition. Machine learning is a subfield of artificial intelligence (AI) that uses algorithms trained on data sets to create self-learning models that are capable of predicting outcomes and classifying information without human intervention. Machine learning is used today for a wide range of commercial purposes, including ...
Emerging Technology; User Demographics; Mobile + More; short read Apr 3, 2024. ... A quarter of U.S. teachers say AI tools do more harm than good in K-12 education. ... 22% of Americans say they interact with artificial intelligence almost constantly or several times a day. 27% say they do this about once a day or several times a week.
The 6E Learning by Design (LbD) model can enhance student teachers' development of competence for integrating technologies in the classrooms including Artificial Intelligence (AI). However, teacher educators rarely use the 6E LbD model in supporting and encouraging student teachers to integrate AI applications in their classrooms effectively. To attract teacher educators to use the 6E LbD ...