- Privacy Policy
Buy Me a Coffee

Home » What is a Hypothesis – Types, Examples and Writing Guide
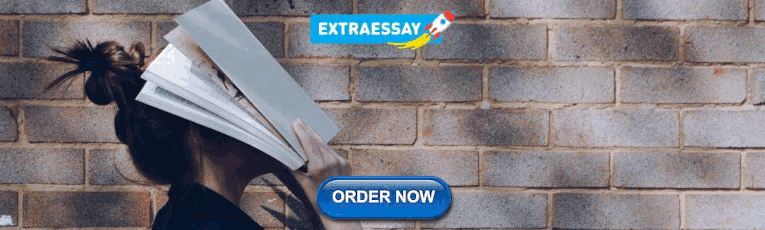
What is a Hypothesis – Types, Examples and Writing Guide
Table of Contents

Definition:
Hypothesis is an educated guess or proposed explanation for a phenomenon, based on some initial observations or data. It is a tentative statement that can be tested and potentially proven or disproven through further investigation and experimentation.
Hypothesis is often used in scientific research to guide the design of experiments and the collection and analysis of data. It is an essential element of the scientific method, as it allows researchers to make predictions about the outcome of their experiments and to test those predictions to determine their accuracy.
Types of Hypothesis
Types of Hypothesis are as follows:
Research Hypothesis
A research hypothesis is a statement that predicts a relationship between variables. It is usually formulated as a specific statement that can be tested through research, and it is often used in scientific research to guide the design of experiments.
Null Hypothesis
The null hypothesis is a statement that assumes there is no significant difference or relationship between variables. It is often used as a starting point for testing the research hypothesis, and if the results of the study reject the null hypothesis, it suggests that there is a significant difference or relationship between variables.
Alternative Hypothesis
An alternative hypothesis is a statement that assumes there is a significant difference or relationship between variables. It is often used as an alternative to the null hypothesis and is tested against the null hypothesis to determine which statement is more accurate.
Directional Hypothesis
A directional hypothesis is a statement that predicts the direction of the relationship between variables. For example, a researcher might predict that increasing the amount of exercise will result in a decrease in body weight.
Non-directional Hypothesis
A non-directional hypothesis is a statement that predicts the relationship between variables but does not specify the direction. For example, a researcher might predict that there is a relationship between the amount of exercise and body weight, but they do not specify whether increasing or decreasing exercise will affect body weight.
Statistical Hypothesis
A statistical hypothesis is a statement that assumes a particular statistical model or distribution for the data. It is often used in statistical analysis to test the significance of a particular result.
Composite Hypothesis
A composite hypothesis is a statement that assumes more than one condition or outcome. It can be divided into several sub-hypotheses, each of which represents a different possible outcome.
Empirical Hypothesis
An empirical hypothesis is a statement that is based on observed phenomena or data. It is often used in scientific research to develop theories or models that explain the observed phenomena.
Simple Hypothesis
A simple hypothesis is a statement that assumes only one outcome or condition. It is often used in scientific research to test a single variable or factor.
Complex Hypothesis
A complex hypothesis is a statement that assumes multiple outcomes or conditions. It is often used in scientific research to test the effects of multiple variables or factors on a particular outcome.
Applications of Hypothesis
Hypotheses are used in various fields to guide research and make predictions about the outcomes of experiments or observations. Here are some examples of how hypotheses are applied in different fields:
- Science : In scientific research, hypotheses are used to test the validity of theories and models that explain natural phenomena. For example, a hypothesis might be formulated to test the effects of a particular variable on a natural system, such as the effects of climate change on an ecosystem.
- Medicine : In medical research, hypotheses are used to test the effectiveness of treatments and therapies for specific conditions. For example, a hypothesis might be formulated to test the effects of a new drug on a particular disease.
- Psychology : In psychology, hypotheses are used to test theories and models of human behavior and cognition. For example, a hypothesis might be formulated to test the effects of a particular stimulus on the brain or behavior.
- Sociology : In sociology, hypotheses are used to test theories and models of social phenomena, such as the effects of social structures or institutions on human behavior. For example, a hypothesis might be formulated to test the effects of income inequality on crime rates.
- Business : In business research, hypotheses are used to test the validity of theories and models that explain business phenomena, such as consumer behavior or market trends. For example, a hypothesis might be formulated to test the effects of a new marketing campaign on consumer buying behavior.
- Engineering : In engineering, hypotheses are used to test the effectiveness of new technologies or designs. For example, a hypothesis might be formulated to test the efficiency of a new solar panel design.
How to write a Hypothesis
Here are the steps to follow when writing a hypothesis:
Identify the Research Question
The first step is to identify the research question that you want to answer through your study. This question should be clear, specific, and focused. It should be something that can be investigated empirically and that has some relevance or significance in the field.
Conduct a Literature Review
Before writing your hypothesis, it’s essential to conduct a thorough literature review to understand what is already known about the topic. This will help you to identify the research gap and formulate a hypothesis that builds on existing knowledge.
Determine the Variables
The next step is to identify the variables involved in the research question. A variable is any characteristic or factor that can vary or change. There are two types of variables: independent and dependent. The independent variable is the one that is manipulated or changed by the researcher, while the dependent variable is the one that is measured or observed as a result of the independent variable.
Formulate the Hypothesis
Based on the research question and the variables involved, you can now formulate your hypothesis. A hypothesis should be a clear and concise statement that predicts the relationship between the variables. It should be testable through empirical research and based on existing theory or evidence.
Write the Null Hypothesis
The null hypothesis is the opposite of the alternative hypothesis, which is the hypothesis that you are testing. The null hypothesis states that there is no significant difference or relationship between the variables. It is important to write the null hypothesis because it allows you to compare your results with what would be expected by chance.
Refine the Hypothesis
After formulating the hypothesis, it’s important to refine it and make it more precise. This may involve clarifying the variables, specifying the direction of the relationship, or making the hypothesis more testable.
Examples of Hypothesis
Here are a few examples of hypotheses in different fields:
- Psychology : “Increased exposure to violent video games leads to increased aggressive behavior in adolescents.”
- Biology : “Higher levels of carbon dioxide in the atmosphere will lead to increased plant growth.”
- Sociology : “Individuals who grow up in households with higher socioeconomic status will have higher levels of education and income as adults.”
- Education : “Implementing a new teaching method will result in higher student achievement scores.”
- Marketing : “Customers who receive a personalized email will be more likely to make a purchase than those who receive a generic email.”
- Physics : “An increase in temperature will cause an increase in the volume of a gas, assuming all other variables remain constant.”
- Medicine : “Consuming a diet high in saturated fats will increase the risk of developing heart disease.”
Purpose of Hypothesis
The purpose of a hypothesis is to provide a testable explanation for an observed phenomenon or a prediction of a future outcome based on existing knowledge or theories. A hypothesis is an essential part of the scientific method and helps to guide the research process by providing a clear focus for investigation. It enables scientists to design experiments or studies to gather evidence and data that can support or refute the proposed explanation or prediction.
The formulation of a hypothesis is based on existing knowledge, observations, and theories, and it should be specific, testable, and falsifiable. A specific hypothesis helps to define the research question, which is important in the research process as it guides the selection of an appropriate research design and methodology. Testability of the hypothesis means that it can be proven or disproven through empirical data collection and analysis. Falsifiability means that the hypothesis should be formulated in such a way that it can be proven wrong if it is incorrect.
In addition to guiding the research process, the testing of hypotheses can lead to new discoveries and advancements in scientific knowledge. When a hypothesis is supported by the data, it can be used to develop new theories or models to explain the observed phenomenon. When a hypothesis is not supported by the data, it can help to refine existing theories or prompt the development of new hypotheses to explain the phenomenon.
When to use Hypothesis
Here are some common situations in which hypotheses are used:
- In scientific research , hypotheses are used to guide the design of experiments and to help researchers make predictions about the outcomes of those experiments.
- In social science research , hypotheses are used to test theories about human behavior, social relationships, and other phenomena.
- I n business , hypotheses can be used to guide decisions about marketing, product development, and other areas. For example, a hypothesis might be that a new product will sell well in a particular market, and this hypothesis can be tested through market research.
Characteristics of Hypothesis
Here are some common characteristics of a hypothesis:
- Testable : A hypothesis must be able to be tested through observation or experimentation. This means that it must be possible to collect data that will either support or refute the hypothesis.
- Falsifiable : A hypothesis must be able to be proven false if it is not supported by the data. If a hypothesis cannot be falsified, then it is not a scientific hypothesis.
- Clear and concise : A hypothesis should be stated in a clear and concise manner so that it can be easily understood and tested.
- Based on existing knowledge : A hypothesis should be based on existing knowledge and research in the field. It should not be based on personal beliefs or opinions.
- Specific : A hypothesis should be specific in terms of the variables being tested and the predicted outcome. This will help to ensure that the research is focused and well-designed.
- Tentative: A hypothesis is a tentative statement or assumption that requires further testing and evidence to be confirmed or refuted. It is not a final conclusion or assertion.
- Relevant : A hypothesis should be relevant to the research question or problem being studied. It should address a gap in knowledge or provide a new perspective on the issue.
Advantages of Hypothesis
Hypotheses have several advantages in scientific research and experimentation:
- Guides research: A hypothesis provides a clear and specific direction for research. It helps to focus the research question, select appropriate methods and variables, and interpret the results.
- Predictive powe r: A hypothesis makes predictions about the outcome of research, which can be tested through experimentation. This allows researchers to evaluate the validity of the hypothesis and make new discoveries.
- Facilitates communication: A hypothesis provides a common language and framework for scientists to communicate with one another about their research. This helps to facilitate the exchange of ideas and promotes collaboration.
- Efficient use of resources: A hypothesis helps researchers to use their time, resources, and funding efficiently by directing them towards specific research questions and methods that are most likely to yield results.
- Provides a basis for further research: A hypothesis that is supported by data provides a basis for further research and exploration. It can lead to new hypotheses, theories, and discoveries.
- Increases objectivity: A hypothesis can help to increase objectivity in research by providing a clear and specific framework for testing and interpreting results. This can reduce bias and increase the reliability of research findings.
Limitations of Hypothesis
Some Limitations of the Hypothesis are as follows:
- Limited to observable phenomena: Hypotheses are limited to observable phenomena and cannot account for unobservable or intangible factors. This means that some research questions may not be amenable to hypothesis testing.
- May be inaccurate or incomplete: Hypotheses are based on existing knowledge and research, which may be incomplete or inaccurate. This can lead to flawed hypotheses and erroneous conclusions.
- May be biased: Hypotheses may be biased by the researcher’s own beliefs, values, or assumptions. This can lead to selective interpretation of data and a lack of objectivity in research.
- Cannot prove causation: A hypothesis can only show a correlation between variables, but it cannot prove causation. This requires further experimentation and analysis.
- Limited to specific contexts: Hypotheses are limited to specific contexts and may not be generalizable to other situations or populations. This means that results may not be applicable in other contexts or may require further testing.
- May be affected by chance : Hypotheses may be affected by chance or random variation, which can obscure or distort the true relationship between variables.
About the author
Muhammad Hassan
Researcher, Academic Writer, Web developer
You may also like

Data Collection – Methods Types and Examples

Delimitations in Research – Types, Examples and...

Research Process – Steps, Examples and Tips

Research Design – Types, Methods and Examples

Institutional Review Board – Application Sample...

Evaluating Research – Process, Examples and...
How to calculate contrasts from a fitted brms model
Answer more questions with your estimated parameters, without refitting the model.
Tilburg University
brms (Bayesian Regression Models using Stan) is an R package that allows fitting complex (multilevel, multivariate, mixture, …) statistical models with straightforward R modeling syntax, while using Stan for bayesian inference under the hood. You will find many uses of that package on this blog. I am particularly fond of brms’ helper functions for post-processing (visualizing, summarizing, etc) the fitted models. In this post, I will show how to calculate and visualize arbitrary contrasts (aka “(general linear) hypothesis tests”) with brms, with full uncertainty estimates.
Models and contrasts
Here, we will discuss linear models, which regress an outcome variable on a weighted combination of predictors, while allowing the weights to vary across individuals (hierarchical linear regression). After fitting the model, you will have estimates of the weights (“beta weights”, or simply regression parameters) that typically consist of an intercept (estimated level of outcome variable when all predictors are zero) and slopes, which indicate how the outcome variable changes as function of one-unit changes of the predictors, when other predictors are at 0.
However, we are often interested in further questions (contrasts, “general linear hypothesis tests”). For example, your model output may report one group’s change over time, and the difference of that slope between groups, but you are particularly interested in the other group’s slope. To find that slope, you’d need to calculate an additional contrast from your model. This is also commonly called “probing interactions” or sometimes “post hoc testing”.
Example data
To make this concrete, let’s consider a hypothetical example data set from Bolger and Laurenceau (2013) : Two groups’ ( treatment : 0/1) self-reported intimacy was tracked over 16 days ( time ). The dataset contains data from a total of 50 (simulated) individuals.
We might be interested in how the two groups’ feelings of intimacy developed over time, and how their temporal trajectories of intimacy differed. To be more specific, we have three questions:
Q1: How did intimacy develop over time for group 0? Q2: How did intimacy develop over time for group 1? Q3: How different were these two time-courses?
To answer, we model intimacy as a function of time, treatment, and their interactions. The hierarchical model includes varying intercepts and effects of time across participants.
Interpreting the model’s parameters
Let’s then answer our questions by looking at the model’s summary, and interpreting the estimated population-level parameters (the posterior means and standard deviations).
The first lesson is that most models are simply too complex to interpret by just looking at the numerical parameter estimates. Therefore, we always draw figures to help us interpret what the model thinks is going on. The figure below shows example participants’ data (left) and the model’s estimated effects on the right.

Then, we can begin interpreting the parameters. First, the intercept indicates estimated intimacy when time and treatment were at their respective baseline levels (0). It is always easiest to interpret the parameters by eyeballing the right panel of the figure above and trying to connect the numbers to the figure. This estimate is the left-most point of the red line.
The estimated time parameter describes the slope of the red line (Q1); treatment1 is the difference between the two lines at time zero (Q3). However, we cannot immediately answer Q2 from the parameters, although we can see that the slope of the blue line is about 0.05 + 0.06. To get the answer to Q2, or more generally, any contrast or “general linear hypothesis test” from a brms model, we can use the hypothesis() method.
hypothesis()
hypothesis() truly is an underappreciated method of the brms package. It can be very useful in probing complex models. It allows us to calculate, visualize, and summarize, with full uncertainty estimates, any transformation of the model’s parameters. These transformations are often called “contrasts” or “general linear hypothesis tests”. But really, they are just transformations of the joint posterior distribution of the model’s parameters.
To answer Q2, then, we encode our question into a combination of the models parameters:
The slope of group 1 is calculated from the model’s parameters by adding the slope of group 0 ( time ) and the interaction term time:treatment1 . = 0 indicates that we are interested in contrasting the resulting estimate the zero (“testing against zero” or even “testing the null hypothesis”). Then, we pass this named string to hypothesis() , and observe the results.
The output indicates that the estimated answer to Question 2 is 0.11 with a standard error of 0.02. I will return to Evid.Ratio and Post.Prob shortly.
The results can also be visualized.

That figure shows the (samples from the) posterior distribution of the answer to Question 2.
More contrasts
With hypothesis() you can answer many additional questions about your model, beyond the parameter estimates. To illustrate, say we are interested in the groups’ difference in intimacy at the end of the study (day 15; Question 4). (The difference at time 0 is reported by the group parameter.)
Directional hypotheses and posterior probabilities
We can also ask for directional questions. For example, what is the probability that group 0’s slope is greater than 0 (Q5)?

We can now return to Evid.Ratio and Post.Prob : The latter indicates the posterior probability that the parameter of interest is greater than zero ( > 0 ). (More accurately, the proportion of samples from the posterior that are greater than zero.) That should correspond to what you see in the figure above. The former is the ratio of the hypothesis and its complement (the ratio of time > 0 and time < 0 ). I find posterior probabilities more intuitive than evidence ratios, but they both return essentially the same information. Perhaps of interest, with uniform priors, posterior probabilities will exactly correspond (numerically, not conceptually) to frequentist one-sided p-values ( Marsman & Wagenmakers, 2017 ).
Multiple hypotheses
You can evaluate multiple hypotheses in one function call:
Hierarchical hypotheses
Up to this point, we have “tested” the model’s population level effects. (Parameters for the average person. “Fixed effects.”) Because we fit a hierarchical model with varying intercepts and slopes of time, we can also test the individual specific parameters. For example, we can look at every individual’s estimated intercept (intimacy at time 0):
In the above, we asked for the results of the hypothesis test, split by group id (which is the grouping factor in our hierarchical model), and indicated coef as the scope. The latter means that the estimates are the subject-specific deviations with the fixed effect added, as opposed to ranef , which are zero-centered.
The results of this question would be a bit too much information to print on screen, so instead we will draw a figure:

When you find that you have a brms model whose parameters don’t quite answer your questions, hypothesis() will probably give you the answer. For more advanced post-processing of your models, I recommend taking a look at the tidybayes package.
An R companion to Statistics: data analysis and modelling
Chapter 12 bayesian estimation with brms, 12.1 the brms package.
brms ( Bürkner 2023 ) stands for Bayesian Regression Models using Stan. ( https://mc-stan.org/ )[Stan] is general software for Bayesian model specification and estimation, implementing Hamiltonian Monte Carlo as the main MCMC algorithm. Due to its efficiency and flexibility, it has quickly become one of the most popular software packages for Bayesian modelling. Stan uses its own language to define Bayesian models, which may pose a learning curve. The brms package provides an easy-to-use interface to Stan for estimating generalized linear mixed-effects models. As this covers a large part of the models used for data analysis in psychology,
12.1.1 Model definition
Defining models in brms is relatively straightforward, as the package relies on a similar formula interface as lm , glm , and lme4 . The main workhorse is the brms::brm() function. Main arguments for brms::brm() are:
- formula : a model formula, using the lme4 syntax. Table 12.1 contains a reminder of this syntax.
- data : the data.frame containing the variables used in the model formula.
- family : similar to that used in glm() , a description of the response distribution (likelihood). By default, this is a Normal distribution.
- prior : definition of the prior distributions. If not specified, default priors are used for all parameters.
- sample_prior : logical, to indicate whether you also want to obtain draws from the prior distributions.
- chains : number of parallel MCMC chains to run (defaults to 4).
- iter : total number of MCMC iterations per chain (defaults to 2000).
- warmup : number of samples to discard as a warmup or burn-in sample (defaults to iter/2 ).
- thin : the so-called thinning rate, which can be used to only keep every \(k\) th sample.
- seed : may be used to set the random seed for replication of results.
- cores : specify the number of cores of the CPU to be used to run the chains in parallel. This defaults to 1 (each chain is run after the other). If you have a multicore CPU, it is better to set this higher (e.g. to 4) to speed-up computation time.
12.1.1.1 Brms family
The family argument in brms::brm() is used to define the random part of the model. The brms package extends the options of the family argument in the glm() function to allow for a much wider class of likelihoods. You can see the help file ( help("brmsfamily", package="brms") ) for a full list of the current options. Some examples of available options are:
- Normal distribution, for linear regression and the GLM: gaussian(link = "identity")
- Generalized Student \(t\) -distribution, useful for robust linear regression less influenced by outliers: student(link = "identity", link_sigma = "log", link_nu = "logm1")
- Bernoulli distribution, for logistic regression: bernoulli(link = "logit")
- Poisson distribution, useful for unbounded count data: poisson(link = "log")
- Categorical distribution, for multinomial logistic regression: categorical(link = "logit", refcat = NULL)
- Beta-distribution, for regression of a dependent variable bounded between 0 and 1 (e.g. proportions): Beta(link = "logit", link_phi = "log")
- Ex-Gaussian distribution, popular for modelling reaction times: exgaussian(link = "identity", link_sigma = "log", link_beta = "log")
And there are many more options. There is even a so-called Wiener diffusion distribution implemented, which can be used to estimate the drift-diffusion model ( wiener(link = "identity", link_bs = "log", link_ndt = "log", link_bias = "logit") ).
Note that many of the brmsfamily objects have multiple link functions, one for each parameter of the distribution. For example, in student(link = "identity", link_sigma = "log", link_nu = "logm1") , there is one link function for the mean ( link ), one for the standard deviation ( link_sigma ), and one for the degrees of freedom ( link_nu ). This family implements a generalized \(t\) -distribution, which, in contrast to the standard \(t\) -distribution, has a location and scale parameter like the Normal distribution. But the degrees of freedom allow this distribution to have wider tails than the Normal distribution, which can make it more robust against outliers.
As an example of estimating a model with brms , we can estimate a linear regression model as follows:
Because estimation with brms involves random sampling via stan , we use the seed argument to be able to reproduce the results.
You can obtain a summary of the model via the summary() function:
The output first shows details of the model (e.g. family and model formula), and MCMC estimation (e.g. number of chains and iterations). Under Population-Level Effects: you will find a summary of the approximate posterior distribution for the main parameters of interest ( Intercept , and slopes for hate_groups_per_million and percent_bachelors_degree_or_higher ). The Estimate is the posterior mean, the Est.Error is the posterior standard deviation, the l-95% CI and u-95% CI provide respectively the lower and upper bound of the 95% Credible Interval (CI) computed with the Equal-Tailed Interval (ETI) method. The width of the latter interval can be changed by supplying a prob argument to the summary function. For example, summary(mod, prob=.9) would return the 90% CI.
The Rhat the \(\hat{R}\) values, and Bulk_ESS an estimate of the Effective Sample Size (ESS). The Tail_ESS provides somewhat of a lower bound on the ESS estimate (see Vehtari et al. 2019 ) . Under Family Specific Parameters: , you will find the same information for the non-regression parameters (i.e. \(\sigma_\epsilon\) for a linear regression model).
You can obtain a quick plot showing a nonparametric density estimate for each parameter, and a plot of the sampled values per iteration and chain, via the plot() function:

If you want to create your own plots, there are several functions to extract the posterior samples of the parameters. Perhaps the most convenient is brms::as_draws_df() , which returns a data.frame with the posterior samples.
12.1.2 Priors
In the example above, we did not specify prior distributions. The brms::brm function uses default values for prior distributions if these are not explicitly set. You can view some detauls of these prior distributions via the prior_summary() function:
The output indicates that “flat” priors are used for the slopes of hate_groups_per_million and percent_bachelors_degree_or_higher . The “flat” priors are so-called improper priors , which indicate that any possible value is equally likely. These priors are therefore also uninformative priors . The prior for \(\sigma_\epsilon\) , the error SD ( sigma ), the prior is a (half) \(t\) -distribution with \(\text{df}=3\) , mean 0, and standard deviation 11.9. The SD is close to the SD of the dependent variable ( percent_Trump_votes ). This is reasonable in providing a weakly informative prior, as the error SD should be smaller than the SD of the dependent variable.
For the intercept, a \(t\) -distribution is used, with \(\text{df}=3\) , a mean of 49.3, and an SD of 11.9. The mean and SD of the prior are close to the mean and SD of percent_Trump_votes . So the prior for the intercept is based on characteristics of the dependent variable, which allows it to automatically have a reasonable scale. Note that the intercept in a brms::brm() model is computed separately, using centered predictors. It then corrects the sampled intercepts again after estimation, to provide an intercept for non-centered predictors. For more details, you can see the brms manual ( help("brmsformula", package="brms") ). If you need to define a prior on the scale of the usual intercept, this behaviour needs to be turned off first.
Default priors are generally fine. But if you want to define your own priors, this is possible with the prior argument. The prior argument expects a vector with prior specifications for each parameter for which you want to set a different prior than default. Each element in this vector needs to be an object of the brmsprior class. The easiest way to obtain such objects is by calling the brms::prior() function. This function has as important arguments: * prior : a specification of a distribution in the Stan language. There are many such distributions available (see the Stan Functions Reference for the full list). Common examples are normal(mean, sd) , student(df, mean, sd) . cauchy(mean, sd) , beta(alpha, beta) , where the values between the parentheses indicate so-called hyper-parameters (i.e. the parameters defining the distribution). I have used df , mean , and sd here, but the Stan manual uses nu , mu , and sigma for these. * class : the class of the parameter. This can for example be b for a regression coefficient (slope), sigma for an error SD, and Intercept for the intercept. This is what you will find under the class column in the output of brms::prior_summary() . Depending on the class, the prior may be restricted to respect the bounds for the parameter. For example, for a prior with class=sigma , the prior will always be truncated at 0, without you having to specify. * coef : the name of the coefficient in the parameter class. This is what you will find under the coef column in the output of brms::prior_summary() . * group : A grouping factor for group-level (random) effects.
For example, we can set Normal priors with a mean of 0 and a standard deviation depending on the parameters as follows. We use the c() function to create the vector with brmsprior objects. We need to define four such objects, each via a call to the prior() function. We assign the result to ab object called mod_priors , so that we can then use it in a call to brms::brm() :
We can now use our mod_priors object as the argument to prior , to estimate a regression model with non-default priors:
I have set sample_prior = TRUE so that we also get samples from the prior distributions. For example, we can get a histogram of the prior for \(\sigma_epsilon\) as:

12.2 Useful plots for MCMC samples
Besides the default plot() function, the brms::mcmc_plot() function can be used to obtain plots. Important arguments to this function are:
- object : a fitted brms::brm() model
- type : A specification of the type of plot. For example, hist (histogram), hist_by_chain (separate histograms for each MCMC chain), dens (nonparametric density plot), dens_overlay (overlaid density plots for each chain), violin (violin plots per chain), intervals (CI intervals for each parameter in the same plot), areas (densities for each parameter in the same plot, and trace (sampled parameters per iteration). For full details of all the options, see help("MCMC-overview", package="bayesplot") .
- variable : Names of the variables (parameters) to plot, as e.g. a character vector. The names of the variables correspond to those provided as the column names in brms::as_draws_df(mod) .
For example, we can get histograms of the posterior for each parameter as

12.3 An example of a Poisson mixed-effects regression model
As an example of estimating a more complex model, we will use the brms package to estimate a Poisson mixed-effects model. We will use the gestures data of the sdamr package for this example. We first load the data, set contrasts, and define an offset variable:
We now define the model in brms , using a random effects specification to indicate we want random intercepts for each participant ( (1|ID) ), and an addition term offset(log_d) which sets the offset (unlike in glm and glmer , in brms the offset needs to be part of the model formula).
Note that I’m specifying to use cores=4 CPU cores here. This will speed up the computation, but requires you to have sufficient cores.
We have relied on the default priors, which are the usual flat priors for the regression coefficients, a \(t\) -distribution for the Intercept, and half- \(t\) distributions for the random effects and errors:
The summary of the approximate posterior is:
Whilst the \(\hat{R}\) values look OK, the effective sample size is rather low for the Intercept , language1 , gender , and language1:gender1 effects. We first inspect the autocorrelation for these parameters:

Thinning may improve the issues with autocorrelation. We rerun the model with different settings for the number of iterations and thinning via the brms::update() function, providing the estimated model as the first argument, and then specifying the number of iterations and thinning required. For example, we can rerun the model again using 1000 iterations as warmup, but now running for an additional 4000 iterations, leading to a total iter=5000 . We furthermore set the thinning to thin=4 to only keep each fourth iteration, which should reduce the autocorrelation:
Checking the model summary shows that this had the desired effect:
Bayesian mixed effects (aka multi-level) ordinal regression models with brms
21 Feb 2017 | all notes
In the past two years I’ve found myself doing lots of statistical analyses on ordinal response data from a (Likert-scale) dialectology questionnaire. I’ve ended up with a good pipeline to run and compare many ordinal regression models with random effects in a Bayesian way using the handy R formula interface in the brms package.
In defense of ordinal regression
In (applied statistical) practice, ordinal data is often simply fit using linear regression (this seems to be particularly true in contemporary, quantitative grammaticality judgment-based syntax literature). While treating ordinal responses as continuous measures is in principle always wrong (because the scale is definitely not ratio), it can in practice be ok to apply linear regression to it, as long as it is reasonable to assume that the scale can be treated as interval data (i.e. the distances between individual response categories are meaningful/comparable). Situations that completely rule out the use of linear methods are:
- when there are edge effects in the data (i.e. many data points in the highest/lowest category)
- when one wants to derive predictions from the resulting statistical models – basing these on linear regression models will often lead to uninterpretable results
If you are happy with simply predicting something like a mean response which at best doesn’t match the actual response scale (whether they predicted response is actually outside the actual response scale or just between categories), and is at worst inaccurate due to its not taking into account any uneven distribution of responses across ordinal categories, that’s ok too. But the most reliable and insightful way of checking the quality/fit of a model is by visual inspection of the model predictions, which is why I always want to be able to produce meaningful (ordinal distribution) predictions to match them against the original data.
Ordinal regression a.k.a. cumulative link models
Ordinal regression methods are typically generalisations of methods used for modelling categorical (in the minimal case binary outcome) data. The most frequently used ordinal regression, ordered logistic (or more accurately ordered logit ) regression is an extension of logistic/logit regression: where in logistic regression you model one coefficient that captures the relative likelihood (in log-odds) of one outcome occurring over another (i.e. 2 outcomes captured by 1 coefficient), ordered logit regression models the relative likelihood of k different outcomes based on k-1 coefficients. The k-1 coefficients in the model capture the cumulative likelihood of responses falling into an expanding set of ordered response categories, e.g. in the case of 4 possible outcome categories ( o1 - o4 ) the three intercepts of the model, each of which will be at least as high as the preceding one, capture the baseline log-odds of observing:
As a consequence of this representation of the underlying probabilities, any log-odds coefficients that are added in the linear regression model actually lead to a multiplication of the odds in the model (i.e. the strength of the effect of a predictor on the modelled odds ratios is proportional to the original likelihood of those ratios, this is a consequence of the proportional odds assumption of logit regression).
The formulation and modelling in log-odds that is the result of the logit transformation are specific to ordered logit regression, however several other methods for modelling binary responses (such as probit models) also have ordinal counterparts (i.e. ordered probit ) where the coefficients and cumulative intercepts are interpreted in different ways.
Choosing a link function
So while the idea of representing the cumulative likelihood of an increasing pool of ordinal responses is a general one, there are several possible formats in which those cumulative probabilities can be represented and modelled, which is where link functions come into play. The role of a link function is simply to transform the probability or odds coefficients from the probability space [0,1] to the full linear space [-Inf,Inf] in such a way that the transformed coefficients can be modelled using linear regression in the best possible (read: most accurate/useful) way.
Different statistical packages support different link families, for example the ordinal package (which offers ordinal regression with one random effect) supports the cumulative links “logit”, “probit”, “cloglog”, “loglog” and “cauchit”, while brms (full-on Bayesian multi-level modelling) supports “logit”, “probit”, “probit_approx”, “cloglog” and “cauchit”. The choice of link function is typically not critical and most methods assume the “logit” function (the log-odds transformation that forms the basis of ordered logit regression) by default, but a different choice can be informed by your knowledge of the data.
The most appropriate or useful link function given a particular data set and model of it can be determined from the data by building models with several different link functions and selecting the one that yields the best (i.e. most predictive) fit, as measured by the models’ log-likelihood. While this is quite expensive to do with Bayesian models, here is a simple function making use of the clm function from the ordinal package to quickly fit several possible link functions and determine the one producing the best fit for a given model (note that this does not take any random effects into account; results in Bayesian models might vary even more):
The cumulative link function’s thresholds parameter can be used to apply additional constraints to the spacing of the model’s intercepts, but you’ll typically want to leave this at “flexible”, its most general default.
Before creating a full-on Bayesian model below, we can already create some simple models to inform our choice of link function for later:
Running a model in brms
While running Bayesian models using brms can be slightly more time-consuming than other R packages (because the STAN models have to be compiled first), its neat lmer() -like formula interface means it’s easy to create a large number of models with different sets of predictors which can then be compared. This maximally transparent way of presenting statistical model results is typical in fields such as sociolinguistics where you don’t just present your final model, but also dwell on insights gained along the way based on discovering that the addition of certain coefficients does not increase the predictiveness of the model.
When no priors over the model’s parameters are supplied explicitly via the prior argument, uninformative priors are assumed.
The link function fit above suggested that the logit link function with flexible thresholds is a good choice – both of these are the default for the cumulative() family, they’re only passed explicitly for the sake of clarity. After running the model, the resulting model fit can be inspected in usual ways using summary() , etc.
The trace of the Bayesian model fit as well as the posterior distribution of the coefficients can be visually inspected by calling plot(agemdl) .
Bayesian model comparison and hypothesis testing
brms models support comparison via the Watanabe-Akaike information criterion as well as Leave-One-Out cross-validation:
The model comparison suggests that neither adding the gender nor the age coefficients is particularly predictive (the standard errors of the improvements of the models is bigger than the improvements themselves), but we can still test hypotheses about specific parameters directly, such as whether the age coefficient is positive (where alpha specifies the size of the credible interval ):
Visual inspection, posteriors, random effects,…
brms provides many other useful functions, from ranef(agemdl) for estimating the relative size of the random effects per group to launch_shiny(agemdl) , which opens an interactive web interface that allows complete exploration of the model results and posterior distributions in your browser.
- brms website
- brms documentation
- introduction to cumulative link models
- stack exchange on mixed effects regression of centered/standardised ordinal responses vs. ordinal regression
- stack exchange on the proportional odds assumption and how to test it

An official website of the United States government
The .gov means it’s official. Federal government websites often end in .gov or .mil. Before sharing sensitive information, make sure you’re on a federal government site.
The site is secure. The https:// ensures that you are connecting to the official website and that any information you provide is encrypted and transmitted securely.
- Publications
- Account settings
Preview improvements coming to the PMC website in October 2024. Learn More or Try it out now .
- Advanced Search
- Journal List
- J Korean Med Sci
- v.36(50); 2021 Dec 27

Formulating Hypotheses for Different Study Designs
Durga prasanna misra.
1 Department of Clinical Immunology and Rheumatology, Sanjay Gandhi Postgraduate Institute of Medical Sciences, Lucknow, India.
Armen Yuri Gasparyan
2 Departments of Rheumatology and Research and Development, Dudley Group NHS Foundation Trust (Teaching Trust of the University of Birmingham, UK), Russells Hall Hospital, Dudley, UK.
Olena Zimba
3 Department of Internal Medicine #2, Danylo Halytsky Lviv National Medical University, Lviv, Ukraine.
Marlen Yessirkepov
4 Department of Biology and Biochemistry, South Kazakhstan Medical Academy, Shymkent, Kazakhstan.
Vikas Agarwal
George d. kitas.
5 Centre for Epidemiology versus Arthritis, University of Manchester, Manchester, UK.
Generating a testable working hypothesis is the first step towards conducting original research. Such research may prove or disprove the proposed hypothesis. Case reports, case series, online surveys and other observational studies, clinical trials, and narrative reviews help to generate hypotheses. Observational and interventional studies help to test hypotheses. A good hypothesis is usually based on previous evidence-based reports. Hypotheses without evidence-based justification and a priori ideas are not received favourably by the scientific community. Original research to test a hypothesis should be carefully planned to ensure appropriate methodology and adequate statistical power. While hypotheses can challenge conventional thinking and may be controversial, they should not be destructive. A hypothesis should be tested by ethically sound experiments with meaningful ethical and clinical implications. The coronavirus disease 2019 pandemic has brought into sharp focus numerous hypotheses, some of which were proven (e.g. effectiveness of corticosteroids in those with hypoxia) while others were disproven (e.g. ineffectiveness of hydroxychloroquine and ivermectin).
Graphical Abstract

DEFINING WORKING AND STANDALONE SCIENTIFIC HYPOTHESES
Science is the systematized description of natural truths and facts. Routine observations of existing life phenomena lead to the creative thinking and generation of ideas about mechanisms of such phenomena and related human interventions. Such ideas presented in a structured format can be viewed as hypotheses. After generating a hypothesis, it is necessary to test it to prove its validity. Thus, hypothesis can be defined as a proposed mechanism of a naturally occurring event or a proposed outcome of an intervention. 1 , 2
Hypothesis testing requires choosing the most appropriate methodology and adequately powering statistically the study to be able to “prove” or “disprove” it within predetermined and widely accepted levels of certainty. This entails sample size calculation that often takes into account previously published observations and pilot studies. 2 , 3 In the era of digitization, hypothesis generation and testing may benefit from the availability of numerous platforms for data dissemination, social networking, and expert validation. Related expert evaluations may reveal strengths and limitations of proposed ideas at early stages of post-publication promotion, preventing the implementation of unsupported controversial points. 4
Thus, hypothesis generation is an important initial step in the research workflow, reflecting accumulating evidence and experts' stance. In this article, we overview the genesis and importance of scientific hypotheses and their relevance in the era of the coronavirus disease 2019 (COVID-19) pandemic.
DO WE NEED HYPOTHESES FOR ALL STUDY DESIGNS?
Broadly, research can be categorized as primary or secondary. In the context of medicine, primary research may include real-life observations of disease presentations and outcomes. Single case descriptions, which often lead to new ideas and hypotheses, serve as important starting points or justifications for case series and cohort studies. The importance of case descriptions is particularly evident in the context of the COVID-19 pandemic when unique, educational case reports have heralded a new era in clinical medicine. 5
Case series serve similar purpose to single case reports, but are based on a slightly larger quantum of information. Observational studies, including online surveys, describe the existing phenomena at a larger scale, often involving various control groups. Observational studies include variable-scale epidemiological investigations at different time points. Interventional studies detail the results of therapeutic interventions.
Secondary research is based on already published literature and does not directly involve human or animal subjects. Review articles are generated by secondary research. These could be systematic reviews which follow methods akin to primary research but with the unit of study being published papers rather than humans or animals. Systematic reviews have a rigid structure with a mandatory search strategy encompassing multiple databases, systematic screening of search results against pre-defined inclusion and exclusion criteria, critical appraisal of study quality and an optional component of collating results across studies quantitatively to derive summary estimates (meta-analysis). 6 Narrative reviews, on the other hand, have a more flexible structure. Systematic literature searches to minimise bias in selection of articles are highly recommended but not mandatory. 7 Narrative reviews are influenced by the authors' viewpoint who may preferentially analyse selected sets of articles. 8
In relation to primary research, case studies and case series are generally not driven by a working hypothesis. Rather, they serve as a basis to generate a hypothesis. Observational or interventional studies should have a hypothesis for choosing research design and sample size. The results of observational and interventional studies further lead to the generation of new hypotheses, testing of which forms the basis of future studies. Review articles, on the other hand, may not be hypothesis-driven, but form fertile ground to generate future hypotheses for evaluation. Fig. 1 summarizes which type of studies are hypothesis-driven and which lead on to hypothesis generation.

STANDARDS OF WORKING AND SCIENTIFIC HYPOTHESES
A review of the published literature did not enable the identification of clearly defined standards for working and scientific hypotheses. It is essential to distinguish influential versus not influential hypotheses, evidence-based hypotheses versus a priori statements and ideas, ethical versus unethical, or potentially harmful ideas. The following points are proposed for consideration while generating working and scientific hypotheses. 1 , 2 Table 1 summarizes these points.
Evidence-based data
A scientific hypothesis should have a sound basis on previously published literature as well as the scientist's observations. Randomly generated (a priori) hypotheses are unlikely to be proven. A thorough literature search should form the basis of a hypothesis based on published evidence. 7
Unless a scientific hypothesis can be tested, it can neither be proven nor be disproven. Therefore, a scientific hypothesis should be amenable to testing with the available technologies and the present understanding of science.
Supported by pilot studies
If a hypothesis is based purely on a novel observation by the scientist in question, it should be grounded on some preliminary studies to support it. For example, if a drug that targets a specific cell population is hypothesized to be useful in a particular disease setting, then there must be some preliminary evidence that the specific cell population plays a role in driving that disease process.
Testable by ethical studies
The hypothesis should be testable by experiments that are ethically acceptable. 9 For example, a hypothesis that parachutes reduce mortality from falls from an airplane cannot be tested using a randomized controlled trial. 10 This is because it is obvious that all those jumping from a flying plane without a parachute would likely die. Similarly, the hypothesis that smoking tobacco causes lung cancer cannot be tested by a clinical trial that makes people take up smoking (since there is considerable evidence for the health hazards associated with smoking). Instead, long-term observational studies comparing outcomes in those who smoke and those who do not, as was performed in the landmark epidemiological case control study by Doll and Hill, 11 are more ethical and practical.
Balance between scientific temper and controversy
Novel findings, including novel hypotheses, particularly those that challenge established norms, are bound to face resistance for their wider acceptance. Such resistance is inevitable until the time such findings are proven with appropriate scientific rigor. However, hypotheses that generate controversy are generally unwelcome. For example, at the time the pandemic of human immunodeficiency virus (HIV) and AIDS was taking foot, there were numerous deniers that refused to believe that HIV caused AIDS. 12 , 13 Similarly, at a time when climate change is causing catastrophic changes to weather patterns worldwide, denial that climate change is occurring and consequent attempts to block climate change are certainly unwelcome. 14 The denialism and misinformation during the COVID-19 pandemic, including unfortunate examples of vaccine hesitancy, are more recent examples of controversial hypotheses not backed by science. 15 , 16 An example of a controversial hypothesis that was a revolutionary scientific breakthrough was the hypothesis put forth by Warren and Marshall that Helicobacter pylori causes peptic ulcers. Initially, the hypothesis that a microorganism could cause gastritis and gastric ulcers faced immense resistance. When the scientists that proposed the hypothesis themselves ingested H. pylori to induce gastritis in themselves, only then could they convince the wider world about their hypothesis. Such was the impact of the hypothesis was that Barry Marshall and Robin Warren were awarded the Nobel Prize in Physiology or Medicine in 2005 for this discovery. 17 , 18
DISTINGUISHING THE MOST INFLUENTIAL HYPOTHESES
Influential hypotheses are those that have stood the test of time. An archetype of an influential hypothesis is that proposed by Edward Jenner in the eighteenth century that cowpox infection protects against smallpox. While this observation had been reported for nearly a century before this time, it had not been suitably tested and publicised until Jenner conducted his experiments on a young boy by demonstrating protection against smallpox after inoculation with cowpox. 19 These experiments were the basis for widespread smallpox immunization strategies worldwide in the 20th century which resulted in the elimination of smallpox as a human disease today. 20
Other influential hypotheses are those which have been read and cited widely. An example of this is the hygiene hypothesis proposing an inverse relationship between infections in early life and allergies or autoimmunity in adulthood. An analysis reported that this hypothesis had been cited more than 3,000 times on Scopus. 1
LESSONS LEARNED FROM HYPOTHESES AMIDST THE COVID-19 PANDEMIC
The COVID-19 pandemic devastated the world like no other in recent memory. During this period, various hypotheses emerged, understandably so considering the public health emergency situation with innumerable deaths and suffering for humanity. Within weeks of the first reports of COVID-19, aberrant immune system activation was identified as a key driver of organ dysfunction and mortality in this disease. 21 Consequently, numerous drugs that suppress the immune system or abrogate the activation of the immune system were hypothesized to have a role in COVID-19. 22 One of the earliest drugs hypothesized to have a benefit was hydroxychloroquine. Hydroxychloroquine was proposed to interfere with Toll-like receptor activation and consequently ameliorate the aberrant immune system activation leading to pathology in COVID-19. 22 The drug was also hypothesized to have a prophylactic role in preventing infection or disease severity in COVID-19. It was also touted as a wonder drug for the disease by many prominent international figures. However, later studies which were well-designed randomized controlled trials failed to demonstrate any benefit of hydroxychloroquine in COVID-19. 23 , 24 , 25 , 26 Subsequently, azithromycin 27 , 28 and ivermectin 29 were hypothesized as potential therapies for COVID-19, but were not supported by evidence from randomized controlled trials. The role of vitamin D in preventing disease severity was also proposed, but has not been proven definitively until now. 30 , 31 On the other hand, randomized controlled trials identified the evidence supporting dexamethasone 32 and interleukin-6 pathway blockade with tocilizumab as effective therapies for COVID-19 in specific situations such as at the onset of hypoxia. 33 , 34 Clues towards the apparent effectiveness of various drugs against severe acute respiratory syndrome coronavirus 2 in vitro but their ineffectiveness in vivo have recently been identified. Many of these drugs are weak, lipophilic bases and some others induce phospholipidosis which results in apparent in vitro effectiveness due to non-specific off-target effects that are not replicated inside living systems. 35 , 36
Another hypothesis proposed was the association of the routine policy of vaccination with Bacillus Calmette-Guerin (BCG) with lower deaths due to COVID-19. This hypothesis emerged in the middle of 2020 when COVID-19 was still taking foot in many parts of the world. 37 , 38 Subsequently, many countries which had lower deaths at that time point went on to have higher numbers of mortality, comparable to other areas of the world. Furthermore, the hypothesis that BCG vaccination reduced COVID-19 mortality was a classic example of ecological fallacy. Associations between population level events (ecological studies; in this case, BCG vaccination and COVID-19 mortality) cannot be directly extrapolated to the individual level. Furthermore, such associations cannot per se be attributed as causal in nature, and can only serve to generate hypotheses that need to be tested at the individual level. 39
IS TRADITIONAL PEER REVIEW EFFICIENT FOR EVALUATION OF WORKING AND SCIENTIFIC HYPOTHESES?
Traditionally, publication after peer review has been considered the gold standard before any new idea finds acceptability amongst the scientific community. Getting a work (including a working or scientific hypothesis) reviewed by experts in the field before experiments are conducted to prove or disprove it helps to refine the idea further as well as improve the experiments planned to test the hypothesis. 40 A route towards this has been the emergence of journals dedicated to publishing hypotheses such as the Central Asian Journal of Medical Hypotheses and Ethics. 41 Another means of publishing hypotheses is through registered research protocols detailing the background, hypothesis, and methodology of a particular study. If such protocols are published after peer review, then the journal commits to publishing the completed study irrespective of whether the study hypothesis is proven or disproven. 42 In the post-pandemic world, online research methods such as online surveys powered via social media channels such as Twitter and Instagram might serve as critical tools to generate as well as to preliminarily test the appropriateness of hypotheses for further evaluation. 43 , 44
Some radical hypotheses might be difficult to publish after traditional peer review. These hypotheses might only be acceptable by the scientific community after they are tested in research studies. Preprints might be a way to disseminate such controversial and ground-breaking hypotheses. 45 However, scientists might prefer to keep their hypotheses confidential for the fear of plagiarism of ideas, avoiding online posting and publishing until they have tested the hypotheses.
SUGGESTIONS ON GENERATING AND PUBLISHING HYPOTHESES
Publication of hypotheses is important, however, a balance is required between scientific temper and controversy. Journal editors and reviewers might keep in mind these specific points, summarized in Table 2 and detailed hereafter, while judging the merit of hypotheses for publication. Keeping in mind the ethical principle of primum non nocere, a hypothesis should be published only if it is testable in a manner that is ethically appropriate. 46 Such hypotheses should be grounded in reality and lend themselves to further testing to either prove or disprove them. It must be considered that subsequent experiments to prove or disprove a hypothesis have an equal chance of failing or succeeding, akin to tossing a coin. A pre-conceived belief that a hypothesis is unlikely to be proven correct should not form the basis of rejection of such a hypothesis for publication. In this context, hypotheses generated after a thorough literature search to identify knowledge gaps or based on concrete clinical observations on a considerable number of patients (as opposed to random observations on a few patients) are more likely to be acceptable for publication by peer-reviewed journals. Also, hypotheses should be considered for publication or rejection based on their implications for science at large rather than whether the subsequent experiments to test them end up with results in favour of or against the original hypothesis.
Hypotheses form an important part of the scientific literature. The COVID-19 pandemic has reiterated the importance and relevance of hypotheses for dealing with public health emergencies and highlighted the need for evidence-based and ethical hypotheses. A good hypothesis is testable in a relevant study design, backed by preliminary evidence, and has positive ethical and clinical implications. General medical journals might consider publishing hypotheses as a specific article type to enable more rapid advancement of science.
Disclosure: The authors have no potential conflicts of interest to disclose.
Author Contributions:
- Data curation: Gasparyan AY, Misra DP, Zimba O, Yessirkepov M, Agarwal V, Kitas GD.
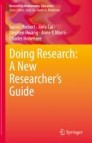
Doing Research: A New Researcher’s Guide pp 17–49 Cite as
How Do You Formulate (Important) Hypotheses?
- James Hiebert 6 ,
- Jinfa Cai 7 ,
- Stephen Hwang 7 ,
- Anne K Morris 6 &
- Charles Hohensee 6
- Open Access
- First Online: 03 December 2022
10k Accesses
Part of the book series: Research in Mathematics Education ((RME))
Building on the ideas in Chap. 1, we describe formulating, testing, and revising hypotheses as a continuing cycle of clarifying what you want to study, making predictions about what you might find together with developing your reasons for these predictions, imagining tests of these predictions, revising your predictions and rationales, and so on. Many resources feed this process, including reading what others have found about similar phenomena, talking with colleagues, conducting pilot studies, and writing drafts as you revise your thinking. Although you might think you cannot predict what you will find, it is always possible—with enough reading and conversations and pilot studies—to make some good guesses. And, once you guess what you will find and write out the reasons for these guesses you are on your way to scientific inquiry. As you refine your hypotheses, you can assess their research importance by asking how connected they are to problems your research community really wants to solve.
You have full access to this open access chapter, Download chapter PDF
Part I. Getting Started
We want to begin by addressing a question you might have had as you read the title of this chapter. You are likely to hear, or read in other sources, that the research process begins by asking research questions . For reasons we gave in Chap. 1 , and more we will describe in this and later chapters, we emphasize formulating, testing, and revising hypotheses. However, it is important to know that asking and answering research questions involve many of the same activities, so we are not describing a completely different process.
We acknowledge that many researchers do not actually begin by formulating hypotheses. In other words, researchers rarely get a researchable idea by writing out a well-formulated hypothesis. Instead, their initial ideas for what they study come from a variety of sources. Then, after they have the idea for a study, they do lots of background reading and thinking and talking before they are ready to formulate a hypothesis. So, for readers who are at the very beginning and do not yet have an idea for a study, let’s back up. Where do research ideas come from?
There are no formulas or algorithms that spawn a researchable idea. But as you begin the process, you can ask yourself some questions. Your answers to these questions can help you move forward.
What are you curious about? What are you passionate about? What have you wondered about as an educator? These are questions that look inward, questions about yourself.
What do you think are the most pressing educational problems? Which problems are you in the best position to address? What change(s) do you think would help all students learn more productively? These are questions that look outward, questions about phenomena you have observed.
What are the main areas of research in the field? What are the big questions that are being asked? These are questions about the general landscape of the field.
What have you read about in the research literature that caught your attention? What have you read that prompted you to think about extending the profession’s knowledge about this? What have you read that made you ask, “I wonder why this is true?” These are questions about how you can build on what is known in the field.
What are some research questions or testable hypotheses that have been identified by other researchers for future research? This, too, is a question about how you can build on what is known in the field. Taking up such questions or hypotheses can help by providing some existing scaffolding that others have constructed.
What research is being done by your immediate colleagues or your advisor that is of interest to you? These are questions about topics for which you will likely receive local support.
Exercise 2.1
Brainstorm some answers for each set of questions. Record them. Then step back and look at the places of intersection. Did you have similar answers across several questions? Write out, as clearly as you can, the topic that captures your primary interest, at least at this point. We will give you a chance to update your responses as you study this book.
Part II. Paths from a General Interest to an Informed Hypothesis
There are many different paths you might take from conceiving an idea for a study, maybe even a vague idea, to formulating a prediction that leads to an informed hypothesis that can be tested. We will explore some of the paths we recommend.
We will assume you have completed Exercise 2.1 in Part I and have some written answers to the six questions that preceded it as well as a statement that describes your topic of interest. This very first statement could take several different forms: a description of a problem you want to study, a question you want to address, or a hypothesis you want to test. We recommend that you begin with one of these three forms, the one that makes most sense to you. There is an advantage to using all three and flexibly choosing the one that is most meaningful at the time and for a particular study. You can then move from one to the other as you think more about your research study and you develop your initial idea. To get a sense of how the process might unfold, consider the following alternative paths.
Beginning with a Prediction If You Have One
Sometimes, when you notice an educational problem or have a question about an educational situation or phenomenon, you quickly have an idea that might help solve the problem or answer the question. Here are three examples.
You are a teacher, and you noticed a problem with the way the textbook presented two related concepts in two consecutive lessons. Almost as soon as you noticed the problem, it occurred to you that the two lessons could be taught more effectively in the reverse order. You predicted better outcomes if the order was reversed, and you even had a preliminary rationale for why this would be true.
You are a graduate student and you read that students often misunderstand a particular aspect of graphing linear functions. You predicted that, by listening to small groups of students working together, you could hear new details that would help you understand this misconception.
You are a curriculum supervisor and you observed sixth-grade classrooms where students were learning about decimal fractions. After talking with several experienced teachers, you predicted that beginning with percentages might be a good way to introduce students to decimal fractions.
We begin with the path of making predictions because we see the other two paths as leading into this one at some point in the process (see Fig. 2.1 ). Starting with this path does not mean you did not sense a problem you wanted to solve or a question you wanted to answer.
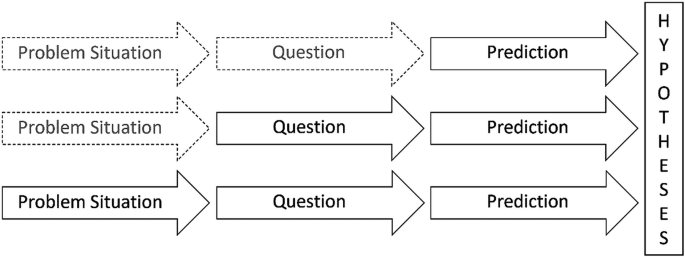
Three Pathways to Formulating Informed Hypotheses
Notice that your predictions can come from a variety of sources—your own experience, reading, and talking with colleagues. Most likely, as you write out your predictions you also think about the educational problem for which your prediction is a potential solution. Writing a clear description of the problem will be useful as you proceed. Notice also that it is easy to change each of your predictions into a question. When you formulate a prediction, you are actually answering a question, even though the question might be implicit. Making that implicit question explicit can generate a first draft of the research question that accompanies your prediction. For example, suppose you are the curriculum supervisor who predicts that teaching percentages first would be a good way to introduce decimal fractions. In an obvious shift in form, you could ask, “In what ways would teaching percentages benefit students’ initial learning of decimal fractions?”
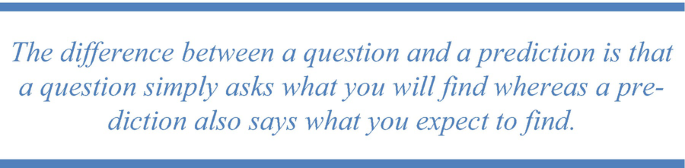
There are advantages to starting with the prediction form if you can make an educated guess about what you will find. Making a prediction forces you to think now about several things you will need to think about at some point anyway. It is better to think about them earlier rather than later. If you state your prediction clearly and explicitly, you can begin to ask yourself three questions about your prediction: Why do I expect to observe what I am predicting? Why did I make that prediction? (These two questions essentially ask what your rationale is for your prediction.) And, how can I test to see if it’s right? This is where the benefits of making predictions begin.
Asking yourself why you predicted what you did, and then asking yourself why you answered the first “why” question as you did, can be a powerful chain of thought that lays the groundwork for an increasingly accurate prediction and an increasingly well-reasoned rationale. For example, suppose you are the curriculum supervisor above who predicted that beginning by teaching percentages would be a good way to introduce students to decimal fractions. Why did you make this prediction? Maybe because students are familiar with percentages in everyday life so they could use what they know to anchor their thinking about hundredths. Why would that be helpful? Because if students could connect hundredths in percentage form with hundredths in decimal fraction form, they could bring their meaning of percentages into decimal fractions. But how would that help? If students understood that a decimal fraction like 0.35 meant 35 of 100, then they could use their understanding of hundredths to explore the meaning of tenths, thousandths, and so on. Why would that be useful? By continuing to ask yourself why you gave the previous answer, you can begin building your rationale and, as you build your rationale, you will find yourself revisiting your prediction, often making it more precise and explicit. If you were the curriculum supervisor and continued the reasoning in the previous sentences, you might elaborate your prediction by specifying the way in which percentages should be taught in order to have a positive effect on particular aspects of students’ understanding of decimal fractions.
Developing a Rationale for Your Predictions
Keeping your initial predictions in mind, you can read what others already know about the phenomenon. Your reading can now become targeted with a clear purpose.
By reading and talking with colleagues, you can develop more complete reasons for your predictions. It is likely that you will also decide to revise your predictions based on what you learn from your reading. As you develop sound reasons for your predictions, you are creating your rationales, and your predictions together with your rationales become your hypotheses. The more you learn about what is already known about your research topic, the more refined will be your predictions and the clearer and more complete your rationales. We will use the term more informed hypotheses to describe this evolution of your hypotheses.
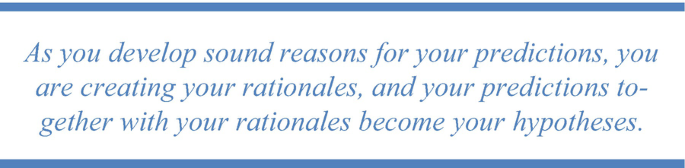
Developing more informed hypotheses is a good thing because it means: (1) you understand the reasons for your predictions; (2) you will be able to imagine how you can test your hypotheses; (3) you can more easily convince your colleagues that they are important hypotheses—they are hypotheses worth testing; and (4) at the end of your study, you will be able to more easily interpret the results of your test and to revise your hypotheses to demonstrate what you have learned by conducting the study.
Imagining Testing Your Hypotheses
Because we have tied together predictions and rationales to constitute hypotheses, testing hypotheses means testing predictions and rationales. Testing predictions means comparing empirical observations, or findings, with the predictions. Testing rationales means using these comparisons to evaluate the adequacy or soundness of the rationales.
Imagining how you might test your hypotheses does not mean working out the details for exactly how you would test them. Rather, it means thinking ahead about how you could do this. Recall the descriptor of scientific inquiry: “experience carefully planned in advance” (Fisher, 1935). Asking whether predictions are testable and whether rationales can be evaluated is simply planning in advance.
You might read that testing hypotheses means simply assessing whether predictions are correct or incorrect. In our view, it is more useful to think of testing as a means of gathering enough information to compare your findings with your predictions, revise your rationales, and propose more accurate predictions. So, asking yourself whether hypotheses can be tested means asking whether information could be collected to assess the accuracy of your predictions and whether the information will show you how to revise your rationales to sharpen your predictions.
Cycles of Building Rationales and Planning to Test Your Predictions
Scientific reasoning is a dialogue between the possible and the actual, an interplay between hypotheses and the logical expectations they give rise to: there is a restless to-and-fro motion of thought, the formulation and rectification of hypotheses (Medawar, 1982 , p.72).
As you ask yourself about how you could test your predictions, you will inevitably revise your rationales and sharpen your predictions. Your hypotheses will become more informed, more targeted, and more explicit. They will make clearer to you and others what, exactly, you plan to study.
When will you know that your hypotheses are clear and precise enough? Because of the way we define hypotheses, this question asks about both rationales and predictions. If a rationale you are building lets you make a number of quite different predictions that are equally plausible rather than a single, primary prediction, then your hypothesis needs further refinement by building a more complete and precise rationale. Also, if you cannot briefly describe to your colleagues a believable way to test your prediction, then you need to phrase it more clearly and precisely.
Each time you strengthen your rationales, you might need to adjust your predictions. And, each time you clarify your predictions, you might need to adjust your rationales. The cycle of going back and forth to keep your predictions and rationales tightly aligned has many payoffs down the road. Every decision you make from this point on will be in the interests of providing a transparent and convincing test of your hypotheses and explaining how the results of your test dictate specific revisions to your hypotheses. As you make these decisions (described in the succeeding chapters), you will probably return to clarify your hypotheses even further. But, you will be in a much better position, at each point, if you begin with well-informed hypotheses.
Beginning by Asking Questions to Clarify Your Interests
Instead of starting with predictions, a second path you might take devotes more time at the beginning to asking questions as you zero in on what you want to study. Some researchers suggest you start this way (e.g., Gournelos et al., 2019 ). Specifically, with this second path, the first statement you write to express your research interest would be a question. For example, you might ask, “Why do ninth-grade students change the way they think about linear equations after studying quadratic equations?” or “How do first graders solve simple arithmetic problems before they have been taught to add and subtract?”
The first phrasing of your question might be quite general or vague. As you think about your question and what you really want to know, you are likely to ask follow-up questions. These questions will almost always be more specific than your first question. The questions will also express more clearly what you want to know. So, the question “How do first graders solve simple arithmetic problems before they have been taught to add and subtract” might evolve into “Before first graders have been taught to solve arithmetic problems, what strategies do they use to solve arithmetic problems with sums and products below 20?” As you read and learn about what others already know about your questions, you will continually revise your questions toward clearer and more explicit and more precise versions that zero in on what you really want to know. The question above might become, “Before they are taught to solve arithmetic problems, what strategies do beginning first graders use to solve arithmetic problems with sums and products below 20 if they are read story problems and given physical counters to help them keep track of the quantities?”
Imagining Answers to Your Questions
If you monitor your own thinking as you ask questions, you are likely to begin forming some guesses about answers, even to the early versions of the questions. What do students learn about quadratic functions that influences changes in their proportional reasoning when dealing with linear functions? It could be that if you analyze the moments during instruction on quadratic equations that are extensions of the proportional reasoning involved in solving linear equations, there are times when students receive further experience reasoning proportionally. You might predict that these are the experiences that have a “backward transfer” effect (Hohensee, 2014 ).
These initial guesses about answers to your questions are your first predictions. The first predicted answers are likely to be hunches or fuzzy, vague guesses. This simply means you do not know very much yet about the question you are asking. Your first predictions, no matter how unfocused or tentative, represent the most you know at the time about the question you are asking. They help you gauge where you are in your thinking.
Shifting to the Hypothesis Formulation and Testing Path
Research questions can play an important role in the research process. They provide a succinct way of capturing your research interests and communicating them to others. When colleagues want to know about your work, they will often ask “What are your research questions?” It is good to have a ready answer.
However, research questions have limitations. They do not capture the three images of scientific inquiry presented in Chap. 1 . Due, in part, to this less expansive depiction of the process, research questions do not take you very far. They do not provide a guide that leads you through the phases of conducting a study.
Consequently, when you can imagine an answer to your research question, we recommend that you move onto the hypothesis formulation and testing path. Imagining an answer to your question means you can make plausible predictions. You can now begin clarifying the reasons for your predictions and transform your early predictions into hypotheses (predictions along with rationales). We recommend you do this as soon as you have guesses about the answers to your questions because formulating, testing, and revising hypotheses offers a tool that puts you squarely on the path of scientific inquiry. It is a tool that can guide you through the entire process of conducting a research study.
This does not mean you are finished asking questions. Predictions are often created as answers to questions. So, we encourage you to continue asking questions to clarify what you want to know. But your target shifts from only asking questions to also proposing predictions for the answers and developing reasons the answers will be accurate predictions. It is by predicting answers, and explaining why you made those predictions, that you become engaged in scientific inquiry.
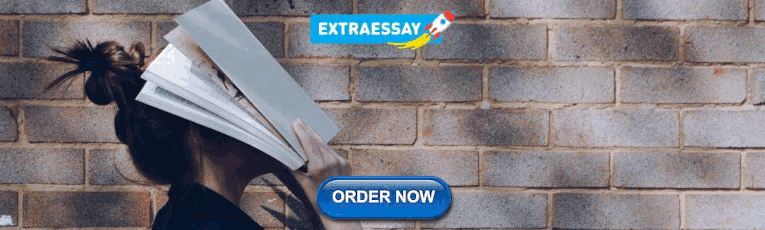
Cycles of Refining Questions and Predicting Answers
An example might provide a sense of how this process plays out. Suppose you are reading about Vygotsky’s ( 1987 ) zone of proximal development (ZPD), and you realize this concept might help you understand why your high school students had trouble learning exponential functions. Maybe they were outside this zone when you tried to teach exponential functions. In order to recognize students who would benefit from instruction, you might ask, “How can I identify students who are within the ZPD around exponential functions?” What would you predict? Maybe students in this ZPD are those who already had knowledge of related functions. You could write out some reasons for this prediction, like “students who understand linear and quadratic functions are more likely to extend their knowledge to exponential functions.” But what kind of data would you need to test this? What would count as “understanding”? Are linear and quadratic the functions you should assess? Even if they are, how could you tell whether students who scored well on tests of linear and quadratic functions were within the ZPD of exponential functions? How, in the end, would you measure what it means to be in this ZPD? So, asking a series of reasonable questions raised some red flags about the way your initial question was phrased, and you decide to revise it.
You set the stage for revising your question by defining ZPD as the zone within which students can solve an exponential function problem by making only one additional conceptual connection between what they already know and exponential functions. Your revised question is, “Based on students’ knowledge of linear and quadratic functions, which students are within the ZPD of exponential functions?” This time you know what kind of data you need: the number of conceptual connections students need to bridge from their knowledge of related functions to exponential functions. How can you collect these data? Would you need to see into the minds of the students? Or, are there ways to test the number of conceptual connections someone makes to move from one topic to another? Do methods exist for gathering these data? You decide this is not realistic, so you now have a choice: revise the question further or move your research in a different direction.
Notice that we do not use the term research question for all these early versions of questions that begin clarifying for yourself what you want to study. These early versions are too vague and general to be called research questions. In this book, we save the term research question for a question that comes near the end of the work and captures exactly what you want to study . By the time you are ready to specify a research question, you will be thinking about your study in terms of hypotheses and tests. When your hypotheses are in final form and include clear predictions about what you will find, it will be easy to state the research questions that accompany your predictions.
To reiterate one of the key points of this chapter: hypotheses carry much more information than research questions. Using our definition, hypotheses include predictions about what the answer might be to the question plus reasons for why you think so. Unlike research questions, hypotheses capture all three images of scientific inquiry presented in Chap. 1 (planning, observing and explaining, and revising one’s thinking). Your hypotheses represent the most you know, at the moment, about your research topic. The same cannot be said for research questions.
Beginning with a Research Problem
When you wrote answers to the six questions at the end of Part I of this chapter, you might have identified a research interest by stating it as a problem. This is the third path you might take to begin your research. Perhaps your description of your problem might look something like this: “When I tried to teach my middle school students by presenting them with a challenging problem without showing them how to solve similar problems, they didn’t exert much effort trying to find a solution but instead waited for me to show them how to solve the problem.” You do not have a specific question in mind, and you do not have an idea for why the problem exists, so you do not have a prediction about how to solve it. Writing a statement of this problem as clearly as possible could be the first step in your research journey.
As you think more about this problem, it will feel natural to ask questions about it. For example, why did some students show more initiative than others? What could I have done to get them started? How could I have encouraged the students to keep trying without giving away the solution? You are now on the path of asking questions—not research questions yet, but questions that are helping you focus your interest.
As you continue to think about these questions, reflect on your own experience, and read what others know about this problem, you will likely develop some guesses about the answers to the questions. They might be somewhat vague answers, and you might not have lots of confidence they are correct, but they are guesses that you can turn into predictions. Now you are on the hypothesis-formulation-and-testing path. This means you are on the path of asking yourself why you believe the predictions are correct, developing rationales for the predictions, asking what kinds of empirical observations would test your predictions, and refining your rationales and predictions as you read the literature and talk with colleagues.
A simple diagram that summarizes the three paths we have described is shown in Fig. 2.1 . Each row of arrows represents one pathway for formulating an informed hypothesis. The dotted arrows in the first two rows represent parts of the pathways that a researcher may have implicitly travelled through already (without an intent to form a prediction) but that ultimately inform the researcher’s development of a question or prediction.
Part III. One Researcher’s Experience Launching a Scientific Inquiry
Martha was in her third year of her doctoral program and beginning to identify a topic for her dissertation. Based on (a) her experience as a high school mathematics teacher and a curriculum supervisor, (b) the reading she has done to this point, and (c) her conversations with her colleagues, she has developed an interest in what kinds of professional development experiences (let’s call them learning opportunities [LOs] for teachers) are most effective. Where does she go from here?
Exercise 2.2
Before you continue reading, please write down some suggestions for Martha about where she should start.
A natural thing for Martha to do at this point is to ask herself some additional questions, questions that specify further what she wants to learn: What kinds of LOs do most teachers experience? How do these experiences change teachers’ practices and beliefs? Are some LOs more effective than others? What makes them more effective?
To focus her questions and decide what she really wants to know, she continues reading but now targets her reading toward everything she can find that suggests possible answers to these questions. She also talks with her colleagues to get more ideas about possible answers to these or related questions. Over several weeks or months, she finds herself being drawn to questions about what makes LOs effective, especially for helping teachers teach more conceptually. She zeroes in on the question, “What makes LOs for teachers effective for improving their teaching for conceptual understanding?”
This question is more focused than her first questions, but it is still too general for Martha to define a research study. How does she know it is too general? She uses two criteria. First, she notices that the predictions she makes about the answers to the question are all over the place; they are not constrained by the reasons she has assembled for her predictions. One prediction is that LOs are more effective when they help teachers learn content. Martha makes this guess because previous research suggests that effective LOs for teachers include attention to content. But this rationale allows lots of different predictions. For example, LOs are more effective when they focus on the content teachers will teach; LOs are more effective when they focus on content beyond what teachers will teach so teachers see how their instruction fits with what their students will encounter later; and LOs are more effective when they are tailored to the level of content knowledge participants have when they begin the LOs. The rationale she can provide at this point does not point to a particular prediction.
A second measure Martha uses to decide her question is too general is that the predictions she can make regarding the answers seem very difficult to test. How could she test, for example, whether LOs should focus on content beyond what teachers will teach? What does “content beyond what teachers teach” mean? How could you tell whether teachers use their new knowledge of later content to inform their teaching?
Before anticipating what Martha’s next question might be, it is important to pause and recognize how predicting the answers to her questions moved Martha into a new phase in the research process. As she makes predictions, works out the reasons for them, and imagines how she might test them, she is immersed in scientific inquiry. This intellectual work is the main engine that drives the research process. Also notice that revisions in the questions asked, the predictions made, and the rationales built represent the updated thinking (Chap. 1 ) that occurs as Martha continues to define her study.
Based on all these considerations and her continued reading, Martha revises the question again. The question now reads, “Do LOs that engage middle school mathematics teachers in studying mathematics content help teachers teach this same content with more of a conceptual emphasis?” Although she feels like the question is more specific, she realizes that the answer to the question is either “yes” or “no.” This, by itself, is a red flag. Answers of “yes” or “no” would not contribute much to understanding the relationships between these LOs for teachers and changes in their teaching. Recall from Chap. 1 that understanding how things work, explaining why things work, is the goal of scientific inquiry.
Martha continues by trying to understand why she believes the answer is “yes.” When she tries to write out reasons for predicting “yes,” she realizes that her prediction depends on a variety of factors. If teachers already have deep knowledge of the content, the LOs might not affect them as much as other teachers. If the LOs do not help teachers develop their own conceptual understanding, they are not likely to change their teaching. By trying to build the rationale for her prediction—thus formulating a hypothesis—Martha realizes that the question still is not precise and clear enough.
Martha uses what she learned when developing the rationale and rephrases the question as follows: “ Under what conditions do LOs that engage middle school mathematics teachers in studying mathematics content help teachers teach this same content with more of a conceptual emphasis?” Through several additional cycles of thinking through the rationale for her predictions and how she might test them, Martha specifies her question even further: “Under what conditions do middle school teachers who lack conceptual knowledge of linear functions benefit from LOs that engage them in conceptual learning of linear functions as assessed by changes in their teaching toward a more conceptual emphasis on linear functions?”
Each version of Martha’s question has become more specific. This has occurred as she has (a) identified a starting condition for the teachers—they lack conceptual knowledge of linear functions, (b) specified the mathematics content as linear functions, and (c) included a condition or purpose of the LO—it is aimed at conceptual learning.
Because of the way Martha’s question is now phrased, her predictions will require thinking about the conditions that could influence what teachers learn from the LOs and how this learning could affect their teaching. She might predict that if teachers engaged in LOs that extended over multiple sessions, they would develop deeper understanding which would, in turn, prompt changes in their teaching. Or she might predict that if the LOs included examples of how their conceptual learning could translate into different instructional activities for their students, teachers would be more likely to change their teaching. Reasons for these predictions would likely come from research about the effects of professional development on teachers’ practice.
As Martha thinks about testing her predictions, she realizes it will probably be easier to measure the conditions under which teachers are learning than the changes in the conceptual emphasis in their instruction. She makes a note to continue searching the literature for ways to measure the “conceptualness” of teaching.
As she refines her predictions and expresses her reasons for the predictions, she formulates a hypothesis (in this case several hypotheses) that will guide her research. As she makes predictions and develops the rationales for these predictions, she will probably continue revising her question. She might decide, for example, that she is not interested in studying the condition of different numbers of LO sessions and so decides to remove this condition from consideration by including in her question something like “. . . over five 2-hour sessions . . .”
At this point, Martha has developed a research question, articulated a number of predictions, and developed rationales for them. Her current question is: “Under what conditions do middle school teachers who lack conceptual knowledge of linear functions benefit from five 2-hour LO sessions that engage them in conceptual learning of linear functions as assessed by changes in their teaching toward a more conceptual emphasis on linear functions?” Her hypothesis is:
Prediction: Participating teachers will show changes in their teaching with a greater emphasis on conceptual understanding, with larger changes on linear function topics directly addressed in the LOs than on other topics.
Brief Description of Rationale: (1) Past research has shown correlations between teachers’ specific mathematics knowledge of a topic and the quality of their teaching of that topic. This does not mean an increase in knowledge causes higher quality teaching but it allows for that possibility. (2) Transfer is usually difficult for teachers, but the examples developed during the LO sessions will help them use what they learned to teach for conceptual understanding. This is because the examples developed during the LO sessions are much like those that will be used by the teachers. So larger changes will be found when teachers are teaching the linear function topics addressed in the LOs.
Notice it is more straightforward to imagine how Martha could test this prediction because it is more precise than previous predictions. Notice also that by asking how to test a particular prediction, Martha will be faced with a decision about whether testing this prediction will tell her something she wants to learn. If not, she can return to the research question and consider how to specify it further and, perhaps, constrain further the conditions that could affect the data.
As Martha formulates her hypotheses and goes through multiple cycles of refining her question(s), articulating her predictions, and developing her rationales, she is constantly building the theoretical framework for her study. Because the theoretical framework is the topic for Chap. 3 , we will pause here and pick up Martha’s story in the next chapter. Spoiler alert: Martha’s experience contains some surprising twists and turns.
Before leaving Martha, however, we point out two aspects of the process in which she has been engaged. First, it can be useful to think about the process as identifying (1) the variables targeted in her predictions, (2) the mechanisms she believes explain the relationships among the variables, and (3) the definitions of all the terms that are special to her educational problem. By variables, we mean things that can be measured and, when measured, can take on different values. In Martha’s case, the variables are the conceptualness of teaching and the content topics addressed in the LOs. The mechanisms are cognitive processes that enable teachers to see the relevance of what they learn in PD to their own teaching and that enable the transfer of learning from one setting to another. Definitions are the precise descriptions of how the important ideas relevant to the research are conceptualized. In Martha’s case, definitions must be provided for terms like conceptual understanding, linear functions, LOs, each of the topics related to linear functions, instructional setting, and knowledge transfer.
A second aspect of the process is a practice that Martha acquired as part of her graduate program, a practice that can go unnoticed. Martha writes out, in full sentences, her thinking as she wrestles with her research question, her predictions of the answers, and the rationales for her predictions. Writing is a tool for organizing thinking and we recommend you use it throughout the scientific inquiry process. We say more about this at the end of the chapter.
Here are the questions Martha wrote as she developed a clearer sense of what question she wanted to answer and what answer she predicted. The list shows the increasing refinement that occurred as she continued to read, think, talk, and write.
Early questions: What kinds of LOs do most teachers experience? How do these experiences change teachers’ practices and beliefs? Are some LOs more effective than others? What makes them more effective?
First focused question: What makes LOs for teachers effective for improving their teaching for conceptual understanding?
Question after trying to predict the answer and imagining how to test the prediction: Do LOs that engage middle school mathematics teachers in studying mathematics content help teachers teach this same content with more of a conceptual emphasis?
Question after developing an initial rationale for her prediction: Under what conditions do LOs that engage middle school mathematics teachers in studying mathematics content help teachers teach this same content with more of a conceptual emphasis?
Question after developing a more precise prediction and richer rationale: Under what conditions do middle school teachers who lack conceptual knowledge of linear functions benefit from five 2-hour LO sessions that engage them in conceptual learning of linear functions as assessed by changes in their teaching toward a more conceptual emphasis on linear functions?
Part IV. An Illustrative Dialogue
The story of Martha described the major steps she took to refine her thinking. However, there is a lot of work that went on behind the scenes that wasn’t part of the story. For example, Martha had conversations with fellow students and professors that sharpened her thinking. What do these conversations look like? Because they are such an important part of the inquiry process, it will be helpful to “listen in” on the kinds of conversations that students might have with their advisors.
Here is a dialogue between a beginning student, Sam (S), and their advisor, Dr. Avery (A). They are meeting to discuss data Sam collected for a course project. The dialogue below is happening very early on in Sam’s conceptualization of the study, prior even to systematic reading of the literature.
Thanks for meeting with me today. As you know, I was able to collect some data for a course project a few weeks ago, but I’m having trouble analyzing the data, so I need your help. Let me try to explain the problem. As you know, I wanted to understand what middle-school teachers do to promote girls’ achievement in a mathematics class. I conducted four observations in each of three teachers’ classrooms. I also interviewed each teacher once about the four lessons I observed, and I interviewed two girls from each of the teachers’ classes. Obviously, I have a ton of data. But when I look at all these data, I don’t really know what I learned about my topic. When I was observing the teachers, I thought I might have observed some ways the teachers were promoting girls’ achievement, but then I wasn’t sure how to interpret my data. I didn’t know if the things I was observing were actually promoting girls’ achievement.
What were some of your observations?
Well, in a couple of my classroom observations, teachers called on girls to give an answer, even when the girls didn’t have their hands up. I thought that this might be a way that teachers were promoting the girls’ achievement. But then the girls didn’t say anything about that when I interviewed them and also the teachers didn’t do it in every class. So, it’s hard to know what effect, if any, this might have had on their learning or their motivation to learn. I didn’t want to ask the girls during the interview specifically about the teacher calling on them, and without the girls bringing it up themselves, I didn’t know if it had any effect.
Well, why didn’t you want to ask the girls about being called on?
Because I wanted to leave it as open as possible; I didn’t want to influence what they were going to say. I didn’t want to put words in their mouths. I wanted to know what they thought the teacher was doing that promoted their mathematical achievement and so I only asked the girls general questions, like “Do you think the teacher does things to promote girls’ mathematical achievement?” and “Can you describe specific experiences you have had that you believe do and do not promote your mathematical achievement?”
So then, how did they answer those general questions?
Well, with very general answers, such as that the teacher knows their names, offers review sessions, grades their homework fairly, gives them opportunities to earn extra credit, lets them ask questions, and always answers their questions. Nothing specific that helps me know what teaching actions specifically target girls’ mathematics achievement.
OK. Any ideas about what you might do next?
Well, I remember that when I was planning this data collection for my course, you suggested I might want to be more targeted and specific about what I was looking for. I can see now that more targeted questions would have made my data more interpretable in terms of connecting teaching actions to the mathematical achievement of girls. But I just didn’t want to influence what the girls would say.
Yes, I remember when you were planning your course project, you wanted to keep it open. You didn’t want to miss out on discovering something new and interesting. What do you think now about this issue?
Well, I still don’t want to put words in their mouths. I want to know what they think. But I see that if I ask really open questions, I have no guarantee they will talk about what I want them to talk about. I guess I still like the idea of an open study, but I see that it’s a risky approach. Leaving the questions too open meant I didn’t constrain their responses and there were too many ways they could interpret and answer the questions. And there are too many ways I could interpret their responses.
By this point in the dialogue, Sam has realized that open data (i.e., data not testing a specific prediction) is difficult to interpret. In the next part, Dr. Avery explains why collecting open data was not helping Sam achieve goals for her study that had motivated collecting open data in the first place.
Yes, I totally agree. Even for an experienced researcher, it can be difficult to make sense of this kind of open, messy data. However, if you design a study with a more specific focus, you can create questions for participants that are more targeted because you will be interested in their answers to these specific questions. Let’s reflect back on your data collection. What can you learn from it for the future?
When I think about it now, I realize that I didn’t think about the distinction between all the different constructs at play in my study, and I didn’t choose which one I was focusing on. One construct was the teaching moves that teachers think could be promoting achievement. Another is what teachers deliberately do to promote girls’ mathematics achievement, if anything. Another was the teaching moves that actually do support girls’ mathematics achievement. Another was what teachers were doing that supported girls’ mathematics achievement versus the mathematics achievement of all students. Another was students’ perception of what their teacher was doing to promote girls’ mathematics achievement. I now see that any one of these constructs could have been the focus of a study and that I didn’t really decide which of these was the focus of my course project prior to collecting data.
So, since you told me that the topic of this course project is probably what you’ll eventually want to study for your dissertation, which of these constructs are you most interested in?
I think I’m more interested in the teacher moves that teachers deliberately do to promote girls’ achievement. But I’m still worried about asking teachers directly and getting too specific about what they do because I don’t want to bias what they will say. And I chose qualitative methods and an exploratory design because I thought it would allow for a more open approach, an approach that helps me see what’s going on and that doesn’t bias or predetermine the results.
Well, it seems to me you are conflating three issues. One issue is how to conduct an unbiased study. Another issue is how specific to make your study. And the third issue is whether or not to choose an exploratory or qualitative study design. Those three issues are not the same. For example, designing a study that’s more open or more exploratory is not how researchers make studies fair and unbiased. In fact, it would be quite easy to create an open study that is biased. For example, you could ask very open questions and then interpret the responses in a way that unintentionally, and even unknowingly, aligns with what you were hoping the findings would say. Actually, you could argue that by adding more specificity and narrowing your focus, you’re creating constraints that prevent bias. The same goes for an exploratory or qualitative study; they can be biased or unbiased. So, let’s talk about what is meant by getting more specific. Within your new focus on what teachers deliberately do, there are many things that would be interesting to look at, such as teacher moves that address math anxiety, moves that allow girls to answer questions more frequently, moves that are specifically fitted to student thinking about specific mathematical content, and so on. What are one or two things that are most interesting to you? One way to answer this question is by thinking back to where your interest in this topic began.
In the preceding part of the dialogue, Dr. Avery explained how the goals Sam had for their study were not being met with open data. In the next part, Sam begins to articulate a prediction, which Sam and Dr. Avery then sharpen.
Actually, I became interested in this topic because of an experience I had in college when I was in a class of mostly girls. During whole class discussions, we were supposed to critically evaluate each other’s mathematical thinking, but we were too polite to do that. Instead, we just praised each other’s work. But it was so different in our small groups. It seemed easier to critique each other’s thinking and to push each other to better solutions in small groups. I began wondering how to get girls to be more critical of each other’s thinking in a whole class discussion in order to push everyone’s thinking.
Okay, this is great information. Why not use this idea to zoom-in on a more manageable and interpretable study? You could look specifically at how teachers support girls in critically evaluating each other’s thinking during whole class discussions. That would be a much more targeted and specific topic. Do you have predictions about what teachers could do in that situation, keeping in mind that you are looking specifically at girls’ mathematical achievement, not students in general?
Well, what I noticed was that small groups provided more social and emotional support for girls, whereas the whole class discussion did not provide that same support. The girls felt more comfortable critiquing each other’s thinking in small groups. So, I guess I predict that when the social and emotional supports that are present in small groups are extended to the whole class discussion, girls would be more willing to evaluate each other’s mathematical thinking critically during whole class discussion . I guess ultimately, I’d like to know how the whole class discussion could be used to enhance, rather than undermine, the social and emotional support that is present in the small groups.
Okay, then where would you start? Would you start with a study of what the teachers say they will do during whole class discussion and then observe if that happens during whole class discussion?
But part of my prediction also involves the small groups. So, I’d also like to include small groups in my study if possible. If I focus on whole groups, I won’t be exploring what I am interested in. My interest is broader than just the whole class discussion.
That makes sense, but there are many different things you could look at as part of your prediction, more than you can do in one study. For instance, if your prediction is that when the social and emotional supports that are present in small groups are extended to whole class discussions, girls would be more willing to evaluate each other’s mathematical thinking critically during whole class discussions , then you could ask the following questions: What are the social and emotional supports that are present in small groups?; In which small groups do they exist?; Is it groups that are made up only of girls?; Does every small group do this, and for groups that do this, when do these supports get created?; What kinds of small group activities that teachers ask them to work on are associated with these supports?; Do the same social and emotional supports that apply to small groups even apply to whole group discussion?
All your questions make me realize that my prediction about extending social and emotional supports to whole class discussions first requires me to have a better understanding of the social and emotional supports that exist in small groups. In fact, I first need to find out whether those supports commonly exist in small groups or is that just my experience working in small groups. So, I think I will first have to figure out what small groups do to support each other and then, in a later study, I could ask a teacher to implement those supports during whole class discussions and find out how you can do that. Yeah, now I’m seeing that.
The previous part of the dialogue illustrates how continuing to ask questions about one’s initial prediction is a good way to make it more and more precise (and researchable). In the next part, we see how developing a precise prediction has the added benefit of setting the researcher up for future studies.
Yes, I agree that for your first study, you should probably look at small groups. In other words, you should focus on only a part of your prediction for now, namely the part that says there are social and emotional supports in small groups that support girls in critiquing each other’s thinking . That begins to sharpen the focus of your prediction, but you’ll want to continue to refine it. For example, right now, the question that this prediction leads to is a question with a yes or no answer, but what you’ve said so far suggests to me that you are looking for more than that.
Yes, I want to know more than just whether there are supports. I’d like to know what kinds. That’s why I wanted to do a qualitative study.
Okay, this aligns more with my thinking about research as being prediction driven. It’s about collecting data that would help you revise your existing predictions into better ones. What I mean is that you would focus on collecting data that would allow you to refine your prediction, make it more nuanced, and go beyond what is already known. Does that make sense, and if so, what would that look like for your prediction?
Oh yes, I like that. I guess that would mean that, based on the data I collect for this next study, I could develop a more refined prediction that, for example, more specifically identifies and differentiates between different kinds of social and emotional supports that are present in small groups, or maybe that identifies the kinds of small groups that they occur in, or that predicts when and how frequently or infrequently they occur, or about the features of the small group tasks in which they occur, etc. I now realize that, although I chose qualitative research to make my study be more open, really the reason qualitative research fits my purposes is because it will allow me to explore fine-grained aspects of social and emotional supports that may exist for girls in small groups.
Yes, exactly! And then, based on the data you collect, you can include in your revised prediction those new fine-grained aspects. Furthermore, you will have a story to tell about your study in your written report, namely the story about your evolving prediction. In other words, your written report can largely tell how you filled out and refined your prediction as you learned more from carrying out the study. And even though you might not use them right away, you are also going to be able to develop new predictions that you would not have even thought of about social and emotional supports in small groups and your aim of extending them to whole-class discussions, had you not done this study. That will set you up to follow up on those new predictions in future studies. For example, you might have more refined ideas after you collect the data about the goals for critiquing student thinking in small groups versus the goals for critiquing student thinking during whole class discussion. You might even begin to think that some of the social and emotional supports you observe are not even replicable or even applicable to or appropriate for whole-class discussions, because the supports play different roles in different contexts. So, to summarize what I’m saying, what you look at in this study, even though it will be very focused, sets you up for a research program that will allow you to more fully investigate your broader interest in this topic, where each new study builds on your prior body of work. That’s why it is so important to be explicit about the best place to start this research, so that you can build on it.
I see what you are saying. We started this conversation talking about my course project data. What I think I should have done was figure out explicitly what I needed to learn with that study with the intention of then taking what I learned and using it as the basis for the next study. I didn’t do that, and so I didn’t collect data that pushed forward my thinking in ways that would guide my next study. It would be as if I was starting over with my next study.
Sam and Dr. Avery have just explored how specifying a prediction reveals additional complexities that could become fodder for developing a systematic research program. Next, we watch Sam beginning to recognize the level of specificity required for a prediction to be testable.
One thing that would have really helped would have been if you had had a specific prediction going into your data collection for your course project.
Well, I didn’t really have much of an explicit prediction in mind when I designed my methods.
Think back, you must have had some kind of prediction, even if it was implicit.
Well, yes, I guess I was predicting that teachers would enact moves that supported girls’ mathematical achievement. And I observed classrooms to identify those teacher moves, I interviewed teachers to ask them about the moves I observed, and I interviewed students to see if they mentioned those moves as promoting their mathematical achievement. The goal of my course project was to identify teacher moves that support girls’ mathematical achievement. And my specific research question was: What teacher moves support girls’ mathematical achievement?
So, really you were asking the teacher and students to show and tell you what those moves are and the effects of those moves, as a result putting the onus on your participants to provide the answers to your research question for you. I have an idea, let’s try a thought experiment. You come up with data collection methods for testing the prediction that there are social and emotional supports in small groups that support girls in critiquing each other’s thinking that still puts the onus on the participants. And then I’ll see if I can think of data collection methods that would not put the onus on the participants.
Hmm, well. .. I guess I could simply interview girls who participated in small groups and ask them “are there social and emotional supports that you use in small groups that support your group in critiquing each other’s thinking and if so, what are they?” In that case, I would be putting the onus on them to be aware of the social dynamics of small groups and to have thought about these constructs as much as I have. Okay now can you continue the thought experiment? What might the data collection methods look like if I didn’t put the onus on the participants?
First, I would pick a setting in which it was only girls at this point to reduce the number of variables. Then, personally I would want to observe a lot of groups of girls interacting in groups around tasks. I would be looking for instances when the conversation about students’ ideas was shut down and instances when the conversation about students’ ideas involved critiquing of ideas and building on each other’s thinking. I would also look at what happened just before and during those instances, such as: did the student continue to talk after their thinking was critiqued, did other students do anything to encourage the student to build on their own thinking (i.e., constructive criticism) or how did they support or shut down continued participation. In fact, now that I think about it, “critiquing each other’s thinking” can be defined in a number of different ways. I could mean just commenting on someone’s thinking, judging correctness and incorrectness, constructive criticism that moves the thinking forward, etc. If you put the onus on the participants to answer your research question, you are stuck with their definition, and they won’t have thought about this very much, if at all.
I think that what you are also saying is that my definitions would affect my data collection. If I think that critiquing each other’s thinking means that the group moves their thinking forward toward more valid and complete mathematical solutions, then I’m going to focus on different moves than if I define it another way, such as just making a comment on each other’s thinking and making each other feel comfortable enough to keep participating. In fact, am I going to look at individual instances of critiquing or look at entire sequences in which the critiquing leads to a goal? This seems like a unit of analysis question, and I would need to develop a more nuanced prediction that would make explicit what that unit of analysis is.
I agree, your definition of “critiquing each other’s thinking” could entirely change what you are predicting. One prediction could be based on defining critiquing as a one-shot event in which someone makes one comment on another person’s thinking. In this case the prediction would be that there are social and emotional supports in small groups that support girls in making an evaluative comment on another student’s thinking. Another prediction could be based on defining critiquing as a back-and-forth process in which the thinking gets built on and refined. In that case, the prediction would be something like that there are social and emotional supports in small groups that support girls in critiquing each other’s thinking in ways that do not shut down the conversation but that lead to sustained conversations that move each other toward more valid and complete solutions.
Well, I think I am more interested in the second prediction because it is more compatible with my long-term interests, which are that I’m interested in extending small group supports to whole class discussions. The second prediction is more appropriate for eventually looking at girls in whole class discussion. During whole class discussion, the teacher tries to get a sustained conversation going that moves the students’ thinking forward. So, if I learn about small group supports that lead to sustained conversations that move each other toward more valid and complete solutions , those supports might transfer to whole class discussions.
In the previous part of the dialogue, Dr. Avery and Sam showed how narrowing down a prediction to one that is testable requires making numerous important decisions, including how to define the constructs referred to in the prediction. In the final part of the dialogue, Dr. Avery and Sam begin to outline the reading Sam will have to do to develop a rationale for the specific prediction.
Do you see how your prediction and definitions are getting more and more specific? You now need to read extensively to further refine your prediction.
Well, I should probably read about micro dynamics of small group interactions, anything about interactions in small groups, and what is already known about small group interactions that support sustained conversations that move students’ thinking toward more valid and complete solutions. I guess I could also look at research on whole-class discussion methods that support sustained conversations that move the class to more mathematically valid and complete solutions, because it might give me ideas for what to look for in the small groups. I might also need to focus on research about how learners develop understandings about a particular subject matter so that I know what “more valid and complete solutions” look like. I also need to read about social and emotional supports but focus on how they support students cognitively, rather than in other ways.
Sounds good, let’s get together after you have processed some of this literature and we can talk about refining your prediction based on what you read and also the methods that will best suit testing that prediction.
Great! Thanks for meeting with me. I feel like I have a much better set of tools that push my own thinking forward and allow me to target something specific that will lead to more interpretable data.
Part V. Is It Always Possible to Formulate Hypotheses?
In Chap. 1 , we noted you are likely to read that research does not require formulating hypotheses. Some sources describe doing research without making predictions and developing rationales for these predictions. Some researchers say you cannot always make predictions—you do not know enough about the situation. In fact, some argue for the value of not making predictions (e.g., Glaser & Holton, 2004 ; Merton, 1968 ; Nemirovsky, 2011 ). These are important points of view, so we will devote this section to discussing them.
Can You Always Predict What You Will Find?
One reason some researchers say you do not need to make predictions is that it can be difficult to imagine what you will find. This argument comes up most often for descriptive studies. Suppose you want to describe the nature of a situation you do not know much about. Can you still make a prediction about what you will find? We believe that, although you do not know exactly what you will find, you probably have a hunch or, at a minimum, a very fuzzy idea. It would be unusual to ask a question about a situation you want to know about without at least a fuzzy inkling of what you might find. The original question just would not occur to you. We acknowledge you might have only a vague idea of what you will find and you might not have much confidence in your prediction. However, we expect if you monitor your own thinking you will discover you have developed a suspicion along the way, regardless how vague the suspicion might be. Through the cyclic process we discussed above, that suspicion or hunch gradually evolves and turns into a prediction.
The Benefits of Making Predictions Even When They Are Wrong: An Example from the 1970s
One of us was a graduate student at the University of Wisconsin in the late 1970s, assigned as a research assistant to a project that was investigating young children’s thinking about simple arithmetic. A new curriculum was being written, and the developers wanted to know how to introduce the earliest concepts and skills to kindergarten and first-grade children. The directors of the project did not know what to expect because, at the time, there was little research on five- and six-year-olds’ pre-instruction strategies for adding and subtracting.
After consulting what literature was available, talking with teachers, analyzing the nature of different types of addition and subtraction problems, and debating with each other, the research team formulated some hypotheses about children’s performance. Following the usual assumptions at the time and recognizing the new curriculum would introduce the concepts, the researchers predicted that, before instruction, most children would not be able to solve the problems. Based on the rationale that some young children did not yet recognize the simple form for written problems (e.g., 5 + 3 = ___), the researchers predicted that the best chance for success would be to read problems as stories (e.g., Jesse had 5 apples and then found 3 more. How many does she have now?). They reasoned that, even though children would have difficulty on all the problems, some story problems would be easier because the semantic structure is easier to follow. For example, they predicted the above story about adding 3 apples to 5 would be easier than a problem like, “Jesse had some apples in the refrigerator. She put in 2 more and now has 6. How many were in the refrigerator at the beginning?” Based on the rationale that children would need to count to solve the problems and that it can be difficult to keep track of the numbers, they predicted children would be more successful if they were given counters. Finally, accepting the common reasoning that larger numbers are more difficult than smaller numbers, they predicted children would be more successful if all the numbers in a problem were below 10.
Although these predictions were not very precise and the rationales were not strongly convincing, these hypotheses prompted the researchers to design the study to test their predictions. This meant they would collect data by presenting a variety of problems under a variety of conditions. Because the goal was to describe children’s thinking, problems were presented to students in individual interviews. Problems with different semantic structures were included, counters were available for some problems but not others, and some problems had sums to 9 whereas others had sums to 20 or more.
The punchline of this story is that gathering data under these conditions, prompted by the predictions, made all the difference in what the researchers learned. Contrary to predictions, children could solve addition and subtraction problems before instruction. Counters were important because almost all the solution strategies were based on counting which meant that memory was an issue because many strategies require counting in two ways simultaneously. For example, subtracting 4 from 7 was usually solved by counting down from 7 while counting up from 1 to 4 to keep track of counting down. Because children acted out the stories with their counters, the semantic structure of the story was also important. Stories that were easier to read and write were also easier to solve.
To make a very long story very short, other researchers were, at about the same time, reporting similar results about children’s pre-instruction arithmetic capabilities. A clear pattern emerged regarding the relative difficulty of different problem types (semantic structures) and the strategies children used to solve each type. As the data were replicated, the researchers recognized that kindergarten and first-grade teachers could make good use of this information when they introduced simple arithmetic. This is how Cognitively Guided Instruction (CGI) was born (Carpenter et al., 1989 ; Fennema et al., 1996 ).
To reiterate, the point of this example is that the study conducted to describe children’s thinking would have looked quite different if the researchers had made no predictions. They would have had no reason to choose the particular problems and present them under different conditions. The fact that some of the predictions were completely wrong is not the point. The predictions created the conditions under which the predictions were tested which, in turn, created learning opportunities for the researchers that would not have existed without the predictions. The lesson is that even research that aims to simply describe a phenomenon can benefit from hypotheses. As signaled in Chap. 1 , this also serves as another example of “failing productively.”
Suggestions for What to Do When You Do Not Have Predictions
There likely are exceptions to our claim about being able to make a prediction about what you will find. For example, there could be rare cases where researchers truly have no idea what they will find and can come up with no predictions and even no hunches. And, no research has been reported on related phenomena that would offer some guidance. If you find yourself in this position, we suggest one of three approaches: revise your question, conduct a pilot study, or choose another question.
Because there are many advantages to making predictions explicit and then writing out the reasons for these predictions, one approach is to adjust your question just enough to allow you to make a prediction. Perhaps you can build on descriptions that other researchers have provided for related situations and consider how you can extend this work. Building on previous descriptions will enable you to make predictions about the situation you want to describe.
A second approach is to conduct a small pilot study or, better, a series of small pilot studies to develop some preliminary ideas of what you might find. If you can identify a small sample of participants who are similar to those in your study, you can try out at least some of your research plans to help make and refine your predictions. As we detail later, you can also use pilot studies to check whether key aspects of your methods (e.g., tasks, interview questions, data collection methods) work as you expect.
A third approach is to return to your list of interests and choose one that has been studied previously. Sometimes this is the wisest choice. It is very difficult for beginning researchers to conduct research in brand-new areas where no hunches or predictions are possible. In addition, the contributions of this research can be limited. Recall the earlier story about one of us “failing productively” by completing a dissertation in a somewhat new area. If, after an exhaustive search, you find that no one has investigated the phenomenon in which you are interested or even related phenomena, it can be best to move in a different direction. You will read recommendations in other sources to find a “gap” in the research and develop a study to “fill the gap.” This can be helpful advice if the gap is very small. However, if the gap is large, too large to predict what you might find, the study will present severe challenges. It will be more productive to extend work that has already been done than to launch into an entirely new area.
Should You Always Try to Predict What You Will Find?
In short, our answer to the question in the heading is “yes.” But this calls for further explanation.
Suppose you want to observe a second-grade classroom in order to investigate how students talk about adding and subtracting whole numbers. You might think, “I don’t want to bias my thinking; I want to be completely open to what I see in the classroom.” Sam shared a similar point of view at the beginning of the dialogue: “I wanted to leave it as open as possible; I didn’t want to influence what they were going to say.” Some researchers say that beginning your research study by making predictions is inappropriate precisely because it will bias your observations and results. The argument is that by bringing a set of preconceptions, you will confirm what you expected to find and be blind to other observations and outcomes. The following quote illustrates this view: “The first step in gaining theoretical sensitivity is to enter the research setting with as few predetermined ideas as possible—especially logically deducted, a priori hypotheses. In this posture, the analyst is able to remain sensitive to the data by being able to record events and detect happenings without first having them filtered through and squared with pre-existing hypotheses and biases” (Glaser, 1978, pp. 2–3).
We take a different point of view. In fact, we believe there are several compelling reasons for making your predictions explicit.
Making Your Predictions Explicit Increases Your Chances of Productive Observations
Because your predictions are an extension of what is already known, they prepare you to identify more nuanced relationships that can advance our understanding of a phenomenon. For example, rather than simply noticing, in a general sense, that students talking about addition and subtraction leads them to better understandings, you might, based on your prediction, make the specific observation that talking about addition and subtraction in a particular way helps students to think more deeply about a particular concept related to addition and subtraction. Going into a study without predictions can bring less sensitivity rather than more to the study of a phenomenon. Drawing on knowledge about related phenomena by reading the literature and conducting pilot studies allows you to be much more sensitive and your observations to be more productive.
Making Your Predictions Explicit Allows You to Guard Against Biases
Some genres and methods of educational research are, in fact, rooted in philosophical traditions (e.g., Husserl, 1929/ 1973 ) that explicitly call for researchers to temporarily “bracket” or set aside existing theory as well as their prior knowledge and experience to better enter into the experience of the participants in the research. However, this does not mean ignoring one’s own knowledge and experience or turning a blind eye to what has been learned by others. Much more than the simplistic image of emptying one’s mind of preconceptions and implicit biases (arguably an impossible feat to begin with), the goal is to be as reflective as possible about one’s prior knowledge and conceptions and as transparent as possible about how they may guide observations and shape interpretations (Levitt et al., 2018 ).
We believe it is better to be honest about the predictions you are almost sure to have because then you can deliberately plan to minimize the chances they will influence what you find and how you interpret your results. For starters, it is important to recognize that acknowledging you have some guesses about what you will find does not make them more influential. Because you are likely to have them anyway, we recommend being explicit about what they are. It is easier to deal with biases that are explicit than those that lurk in the background and are not acknowledged.
What do we mean by “deal with biases”? Some journals require you to include a statement about your “positionality” with respect to the participants in your study and the observations you are making to gather data. Formulating clear hypotheses is, in our view, a direct response to this request. The reasons for your predictions are your explicit statements about your positionality. Often there are methodological strategies you can use to protect the study from undue influences of bias. In other words, making your vague predictions explicit can help you design your study so you minimize the bias of your findings.
Making Your Predictions Explicit Can Help You See What You Did Not Predict
Making your predictions explicit does not need to blind you to what is different than expected. It does not need to force you to see only what you want to see. Instead, it can actually increase your sensitivity to noticing features of the situation that are surprising, features you did not predict. Results can stand out when you did not expect to see them.
In contrast, not bringing your biases to consciousness might subtly shift your attention away from these unexpected results in ways that you are not aware of. This path can lead to claiming no biases and no unexpected findings without being conscious of them. You cannot observe everything, and some things inevitably will be overlooked. If you have predicted what you will see, you can design your study so that the unexpected results become more salient rather than less.
Returning to the example of observing a second-grade classroom, we note that the field already knows a great deal about how students talk about addition and subtraction. Being cognizant of what others have observed allows you to enter the classroom with some clear predictions about what will happen. The rationales for these predictions are based on all the related knowledge you have before stepping into the classroom, and the predictions and rationales help you to better deal with what you see. This is partly because you are likely to be surprised by the things you did not anticipate. There is almost always something that will surprise you because your predictions will almost always be incomplete or too general. This sensitivity to the unanticipated—the sense of surprise that sparks your curiosity—is an indication of your openness to the phenomenon you are studying.
Making Your Predictions Explicit Allows You to Plan in Advance
Recall from Chap. 1 the descriptor of scientific inquiry: “Experience carefully planned in advance.” If you make no predictions about what might happen, it is very difficult, if not impossible, to plan your study in advance. Again, you cannot observe everything, so you must make decisions about what you will observe. What kind of data will you plan to collect? Why would you collect these data instead of others? If you have no idea what to expect, on what basis will you make these consequential decisions? Even if your predictions are vague and your rationales for the predictions are a bit shaky, at least they provide a direction for your plan. They allow you to explain why you are planning this study and collecting these data. They allow you to “carefully plan in advance.”
Making Your Predictions Explicit Allows You to Put Your Rationales in Harm’s Way
Rationales are developed to justify the predictions. Rationales represent your best reasoning about the research problem you are studying. How can you tell whether your reasoning is sound? You can try it out with colleagues. However, the best way to test it is to put it in “harm’s way” (Cobb, Confrey, diSessa, Lehrer, & Schauble, 2003 p. 10). And the best approach to putting your reasoning in harm’s way is to test the predictions it generates. Regardless if you are conducting a qualitative or quantitative study, rationales can be improved only if they generate testable predictions. This is possible only if predictions are explicit and precise. As we described earlier, rationales are evaluated for their soundness and refined in light of the specific differences between predictions and empirical observations.
Making Your Predictions Explicit Forces You to Organize and Extend Your (and the Field’s) Thinking
By writing out your predictions (even hunches or fuzzy guesses) and by reflecting on why you have these predictions and making these reasons explicit for yourself, you are advancing your thinking about the questions you really want to answer. This means you are making progress toward formulating your research questions and your final hypotheses. Making more progress in your own thinking before you conduct your study increases the chances your study will be of higher quality and will be exactly the study you intended. Making predictions, developing rationales, and imagining tests are tools you can use to push your thinking forward before you even collect data.
Suppose you wonder how preservice teachers in your university’s teacher preparation program will solve particular kinds of math problems. You are interested in this question because you have noticed several PSTs solve them in unexpected ways. As you ask the question you want to answer, you make predictions about what you expect to see. When you reflect on why you made these predictions, you realize that some PSTs might use particular solution strategies because they were taught to use some of them in an earlier course, and they might believe you expect them to solve the problems in these ways. By being explicit about why you are making particular predictions, you realize that you might be answering a different question than you intend (“How much do PSTs remember from previous courses?” or even “To what extent do PSTs believe different instructors have similar expectations?”). Now you can either change your question or change the design of your study (i.e., the sample of students you will use) or both. You are advancing your thinking by being explicit about your predictions and why you are making them.
The Costs of Not Making Predictions
Avoiding making predictions, for whatever reason, comes with significant costs. It prevents you from learning very much about your research topic. It would require not reading related research, not talking with your colleagues, and not conducting pilot studies because, if you do, you are likely to find a prediction creeping into your thinking. Not doing these things would forego the benefits of advancing your thinking before you collect data. It would amount to conducting the study with as little forethought as possible.
Part VI. How Do You Formulate Important Hypotheses?
We provided a partial answer in Chap. 1 to the question of a hypothesis’ importance when we encouraged considering the ultimate goal to which a study’s findings might contribute. You might want to reread Part III of Chap. 1 where we offered our opinions about the purposes of doing research. We also recommend reading the March 2019 editorial in the Journal for Research in Mathematics Education (Cai et al., 2019b ) in which we address what constitutes important educational research.
As we argued in Chap. 1 and in the March 2019 editorial, a worthy ultimate goal for educational research is to improve the learning opportunities for all students. However, arguments can be made for other ultimate goals as well. To gauge the importance of your hypotheses, think about how clearly you can connect them to a goal the educational community considers important. In addition, given the descriptors of scientific inquiry proposed in Chap. 1 , think about how testing your hypotheses will help you (and the community) understand what you are studying. Will you have a better explanation for the phenomenon after your study than before?
Although we address the question of importance again, and in more detail, in Chap. 5 , it is useful to know here that you can determine the significance or importance of your hypotheses when you formulate them. The importance need not depend on the data you collect or the results you report. The importance can come from the fact that, based on the results of your study, you will be able to offer revised hypotheses that help the field better understand an important issue. In large part, it is these revised hypotheses rather than the data that determine a study’s importance.
A critical caveat to this discussion is that few hypotheses are self-evidently important. They are important only if you make the case for their importance. Even if you follow closely the guidelines we suggest for formulating an important hypothesis, you must develop an argument that convinces others. This argument will be presented in the research paper you write.

Consider Martha’s hypothesis presented earlier. When we left Martha, she predicted that “Participating teachers will show changes in their teaching with a greater emphasis on conceptual understanding with larger changes on linear function topics directly addressed in the LOs than on other topics.” For researchers and educators not intimately familiar with this area of research, it is not apparent why someone should spend a year or more conducting a dissertation to test this prediction. Her rationale, summarized earlier, begins to describe why this could be an important hypothesis. But it is by writing a clear argument that explains her rationale to readers that she will convince them of its importance.
How Martha fills in her rationale so she can create a clear written argument for its importance is taken up in Chap. 3 . As we indicated, Martha’s work in this regard led her to make some interesting decisions, in part due to her own assessment of what was important.
Part VII. Beginning to Write the Research Paper for Your Study
It is common to think that researchers conduct a study and then, after the data are collected and analyzed, begin writing the paper about the study. We recommend an alternative, especially for beginning researchers. We believe it is better to write drafts of the paper at the same time you are planning and conducting your study. The paper will gradually evolve as you work through successive phases of the scientific inquiry process. Consequently, we will call this paper your evolving research paper .

You will use your evolving research paper to communicate your study, but you can also use writing as a tool for thinking and organizing your thinking while planning and conducting the study. Used as a tool for thinking, you can write drafts of your ideas to check on the clarity of your thinking, and then you can step back and reflect on how to clarify it further. Be sure to avoid jargon and general terms that are not well defined. Ask yourself whether someone not in your field, maybe a sibling, a parent, or a friend, would be able to understand what you mean. You are likely to write multiple drafts with lots of scribbling, crossing out, and revising.
Used as a tool for communicating, writing the best version of what you know before moving to the next phase will help you record your decisions and the reasons for them before you forget important details. This best-version-for-now paper also provides the basis for your thinking about the next phase of your scientific inquiry.
At this point in the process, you will be writing your (research) questions, the answers you predict, and the rationales for your predictions. The predictions you make should be direct answers to your research questions and should flow logically from (or be directly supported by) the rationales you present. In addition, you will have a written statement of the study’s purpose or, said another way, an argument for the importance of the hypotheses you will be testing. It is in the early sections of your paper that you will convince your audience about the importance of your hypotheses.
In our experience, presenting research questions is a more common form of stating the goal of a research study than presenting well-formulated hypotheses. Authors sometimes present a hypothesis, often as a simple prediction of what they might find. The hypothesis is then forgotten and not used to guide the analysis or interpretations of the findings. In other words, authors seldom use hypotheses to do the kind of work we describe. This means that many research articles you read will not treat hypotheses as we suggest. We believe these are missed opportunities to present research in a more compelling and informative way. We intend to provide enough guidance in the remaining chapters for you to feel comfortable organizing your evolving research paper around formulating, testing, and revising hypotheses.
While we were editing one of the leading research journals in mathematics education ( JRME ), we conducted a study of reviewers’ critiques of papers submitted to the journal. Two of the five most common concerns were: (1) the research questions were unclear, and (2) the answers to the questions did not make a substantial contribution to the field. These are likely to be major concerns for the reviewers of all research journals. We hope the knowledge and skills you have acquired working through this chapter will allow you to write the opening to your evolving research paper in a way that addresses these concerns. Much of the chapter should help make your research questions clear, and the prior section on formulating “important hypotheses” will help you convey the contribution of your study.
Exercise 2.3
Look back at your answers to the sets of questions before part II of this chapter.
Think about how you would argue for the importance of your current interest.
Write your interest in the form of (1) a research problem, (2) a research question, and (3) a prediction with the beginnings of a rationale. You will update these as you read the remaining chapters.
Part VIII. The Heart of Scientific Inquiry
In this chapter, we have described the process of formulating hypotheses. This process is at the heart of scientific inquiry. It is where doing research begins. Conducting research always involves formulating, testing, and revising hypotheses. This is true regardless of your research questions and whether you are using qualitative, quantitative, or mixed methods. Without engaging in this process in a deliberate, intense, relentless way, your study will reveal less than it could. By engaging in this process, you are maximizing what you, and others, can learn from conducting your study.
In the next chapter, we build on the ideas we have developed in the first two chapters to describe the purpose and nature of theoretical frameworks . The term theoretical framework, along with closely related terms like conceptual framework, can be somewhat mysterious for beginning researchers and can seem like a requirement for writing a paper rather than an aid for conducting research. We will show how theoretical frameworks grow from formulating hypotheses—from developing rationales for the predicted answers to your research questions. We will propose some practical suggestions for building theoretical frameworks and show how useful they can be. In addition, we will continue Martha’s story from the point at which we paused earlier—developing her theoretical framework.
Cai, J., Morris, A., Hohensee, C., Hwang, S., Robison, V., Cirillo, M., Kramer, S. L., & Hiebert, J. (2019b). Posing significant research questions. Journal for Research in Mathematics Education, 50 (2), 114–120. https://doi.org/10.5951/jresematheduc.50.2.0114
Article Google Scholar
Carpenter, T. P., Fennema, E., Peterson, P. L., Chiang, C. P., & Loef, M. (1989). Using knowledge of children’s mathematics thinking in classroom teaching: An experimental study. American Educational Research Journal, 26 (4), 385–531.
Fennema, E., Carpenter, T. P., Franke, M. L., Levi, L., Jacobs, V. R., & Empson, S. B. (1996). A longitudinal study of learning to use children’s thinking in mathematics instruction. Journal for Research in Mathematics Education, 27 (4), 403–434.
Glaser, B. G., & Holton, J. (2004). Remodeling grounded theory. Forum: Qualitative Social Research, 5(2). https://www.qualitative-research.net/index.php/fqs/article/view/607/1316
Gournelos, T., Hammonds, J. R., & Wilson, M. A. (2019). Doing academic research: A practical guide to research methods and analysis . Routledge.
Book Google Scholar
Hohensee, C. (2014). Backward transfer: An investigation of the influence of quadratic functions instruction on students’ prior ways of reasoning about linear functions. Mathematical Thinking and Learning, 16 (2), 135–174.
Husserl, E. (1973). Cartesian meditations: An introduction to phenomenology (D. Cairns, Trans.). Martinus Nijhoff. (Original work published 1929).
Google Scholar
Levitt, H. M., Bamberg, M., Creswell, J. W., Frost, D. M., Josselson, R., & Suárez-Orozco, C. (2018). Journal article reporting standards for qualitative primary, qualitative meta-analytic, and mixed methods research in psychology: The APA Publications and Communications Board Task Force report. American Psychologist, 73 (1), 26–46.
Medawar, P. (1982). Pluto’s republic [no typo]. Oxford University Press.
Merton, R. K. (1968). Social theory and social structure (Enlarged edition). Free Press.
Nemirovsky, R. (2011). Episodic feelings and transfer of learning. Journal of the Learning Sciences, 20 (2), 308–337. https://doi.org/10.1080/10508406.2011.528316
Vygotsky, L. (1987). The development of scientific concepts in childhood: The design of a working hypothesis. In A. Kozulin (Ed.), Thought and language (pp. 146–209). The MIT Press.
Download references
Author information
Authors and affiliations.
School of Education, University of Delaware, Newark, DE, USA
James Hiebert, Anne K Morris & Charles Hohensee
Department of Mathematical Sciences, University of Delaware, Newark, DE, USA
Jinfa Cai & Stephen Hwang
You can also search for this author in PubMed Google Scholar
Rights and permissions
Open Access This chapter is licensed under the terms of the Creative Commons Attribution 4.0 International License ( http://creativecommons.org/licenses/by/4.0/ ), which permits use, sharing, adaptation, distribution and reproduction in any medium or format, as long as you give appropriate credit to the original author(s) and the source, provide a link to the Creative Commons license and indicate if changes were made.
The images or other third party material in this chapter are included in the chapter's Creative Commons license, unless indicated otherwise in a credit line to the material. If material is not included in the chapter's Creative Commons license and your intended use is not permitted by statutory regulation or exceeds the permitted use, you will need to obtain permission directly from the copyright holder.
Reprints and permissions
Copyright information
© 2023 The Author(s)
About this chapter
Cite this chapter.
Hiebert, J., Cai, J., Hwang, S., Morris, A.K., Hohensee, C. (2023). How Do You Formulate (Important) Hypotheses?. In: Doing Research: A New Researcher’s Guide. Research in Mathematics Education. Springer, Cham. https://doi.org/10.1007/978-3-031-19078-0_2
Download citation
DOI : https://doi.org/10.1007/978-3-031-19078-0_2
Published : 03 December 2022
Publisher Name : Springer, Cham
Print ISBN : 978-3-031-19077-3
Online ISBN : 978-3-031-19078-0
eBook Packages : Education Education (R0)
Share this chapter
Anyone you share the following link with will be able to read this content:
Sorry, a shareable link is not currently available for this article.
Provided by the Springer Nature SharedIt content-sharing initiative
- Publish with us
Policies and ethics
- Find a journal
- Track your research
Have a language expert improve your writing
Run a free plagiarism check in 10 minutes, generate accurate citations for free.
- Knowledge Base
Methodology
- How to Write a Strong Hypothesis | Steps & Examples
How to Write a Strong Hypothesis | Steps & Examples
Published on May 6, 2022 by Shona McCombes . Revised on November 20, 2023.
A hypothesis is a statement that can be tested by scientific research. If you want to test a relationship between two or more variables, you need to write hypotheses before you start your experiment or data collection .
Example: Hypothesis
Daily apple consumption leads to fewer doctor’s visits.
Table of contents
What is a hypothesis, developing a hypothesis (with example), hypothesis examples, other interesting articles, frequently asked questions about writing hypotheses.
A hypothesis states your predictions about what your research will find. It is a tentative answer to your research question that has not yet been tested. For some research projects, you might have to write several hypotheses that address different aspects of your research question.
A hypothesis is not just a guess – it should be based on existing theories and knowledge. It also has to be testable, which means you can support or refute it through scientific research methods (such as experiments, observations and statistical analysis of data).
Variables in hypotheses
Hypotheses propose a relationship between two or more types of variables .
- An independent variable is something the researcher changes or controls.
- A dependent variable is something the researcher observes and measures.
If there are any control variables , extraneous variables , or confounding variables , be sure to jot those down as you go to minimize the chances that research bias will affect your results.
In this example, the independent variable is exposure to the sun – the assumed cause . The dependent variable is the level of happiness – the assumed effect .
Prevent plagiarism. Run a free check.
Step 1. ask a question.
Writing a hypothesis begins with a research question that you want to answer. The question should be focused, specific, and researchable within the constraints of your project.
Step 2. Do some preliminary research
Your initial answer to the question should be based on what is already known about the topic. Look for theories and previous studies to help you form educated assumptions about what your research will find.
At this stage, you might construct a conceptual framework to ensure that you’re embarking on a relevant topic . This can also help you identify which variables you will study and what you think the relationships are between them. Sometimes, you’ll have to operationalize more complex constructs.
Step 3. Formulate your hypothesis
Now you should have some idea of what you expect to find. Write your initial answer to the question in a clear, concise sentence.
4. Refine your hypothesis
You need to make sure your hypothesis is specific and testable. There are various ways of phrasing a hypothesis, but all the terms you use should have clear definitions, and the hypothesis should contain:
- The relevant variables
- The specific group being studied
- The predicted outcome of the experiment or analysis
5. Phrase your hypothesis in three ways
To identify the variables, you can write a simple prediction in if…then form. The first part of the sentence states the independent variable and the second part states the dependent variable.
In academic research, hypotheses are more commonly phrased in terms of correlations or effects, where you directly state the predicted relationship between variables.
If you are comparing two groups, the hypothesis can state what difference you expect to find between them.
6. Write a null hypothesis
If your research involves statistical hypothesis testing , you will also have to write a null hypothesis . The null hypothesis is the default position that there is no association between the variables. The null hypothesis is written as H 0 , while the alternative hypothesis is H 1 or H a .
- H 0 : The number of lectures attended by first-year students has no effect on their final exam scores.
- H 1 : The number of lectures attended by first-year students has a positive effect on their final exam scores.
If you want to know more about the research process , methodology , research bias , or statistics , make sure to check out some of our other articles with explanations and examples.
- Sampling methods
- Simple random sampling
- Stratified sampling
- Cluster sampling
- Likert scales
- Reproducibility
Statistics
- Null hypothesis
- Statistical power
- Probability distribution
- Effect size
- Poisson distribution
Research bias
- Optimism bias
- Cognitive bias
- Implicit bias
- Hawthorne effect
- Anchoring bias
- Explicit bias
Here's why students love Scribbr's proofreading services
Discover proofreading & editing
A hypothesis is not just a guess — it should be based on existing theories and knowledge. It also has to be testable, which means you can support or refute it through scientific research methods (such as experiments, observations and statistical analysis of data).
Null and alternative hypotheses are used in statistical hypothesis testing . The null hypothesis of a test always predicts no effect or no relationship between variables, while the alternative hypothesis states your research prediction of an effect or relationship.
Hypothesis testing is a formal procedure for investigating our ideas about the world using statistics. It is used by scientists to test specific predictions, called hypotheses , by calculating how likely it is that a pattern or relationship between variables could have arisen by chance.
Cite this Scribbr article
If you want to cite this source, you can copy and paste the citation or click the “Cite this Scribbr article” button to automatically add the citation to our free Citation Generator.
McCombes, S. (2023, November 20). How to Write a Strong Hypothesis | Steps & Examples. Scribbr. Retrieved April 11, 2024, from https://www.scribbr.com/methodology/hypothesis/
Is this article helpful?
Shona McCombes
Other students also liked, construct validity | definition, types, & examples, what is a conceptual framework | tips & examples, operationalization | a guide with examples, pros & cons, unlimited academic ai-proofreading.
✔ Document error-free in 5minutes ✔ Unlimited document corrections ✔ Specialized in correcting academic texts
- Resources Home 🏠
- Try SciSpace Copilot
- Search research papers
- Add Copilot Extension
- Try AI Detector
- Try Paraphraser
- Try Citation Generator
- April Papers
- June Papers
- July Papers

The Craft of Writing a Strong Hypothesis

Table of Contents
Writing a hypothesis is one of the essential elements of a scientific research paper. It needs to be to the point, clearly communicating what your research is trying to accomplish. A blurry, drawn-out, or complexly-structured hypothesis can confuse your readers. Or worse, the editor and peer reviewers.
A captivating hypothesis is not too intricate. This blog will take you through the process so that, by the end of it, you have a better idea of how to convey your research paper's intent in just one sentence.
What is a Hypothesis?
The first step in your scientific endeavor, a hypothesis, is a strong, concise statement that forms the basis of your research. It is not the same as a thesis statement , which is a brief summary of your research paper .
The sole purpose of a hypothesis is to predict your paper's findings, data, and conclusion. It comes from a place of curiosity and intuition . When you write a hypothesis, you're essentially making an educated guess based on scientific prejudices and evidence, which is further proven or disproven through the scientific method.
The reason for undertaking research is to observe a specific phenomenon. A hypothesis, therefore, lays out what the said phenomenon is. And it does so through two variables, an independent and dependent variable.
The independent variable is the cause behind the observation, while the dependent variable is the effect of the cause. A good example of this is “mixing red and blue forms purple.” In this hypothesis, mixing red and blue is the independent variable as you're combining the two colors at your own will. The formation of purple is the dependent variable as, in this case, it is conditional to the independent variable.
Different Types of Hypotheses

Types of hypotheses
Some would stand by the notion that there are only two types of hypotheses: a Null hypothesis and an Alternative hypothesis. While that may have some truth to it, it would be better to fully distinguish the most common forms as these terms come up so often, which might leave you out of context.
Apart from Null and Alternative, there are Complex, Simple, Directional, Non-Directional, Statistical, and Associative and casual hypotheses. They don't necessarily have to be exclusive, as one hypothesis can tick many boxes, but knowing the distinctions between them will make it easier for you to construct your own.
1. Null hypothesis
A null hypothesis proposes no relationship between two variables. Denoted by H 0 , it is a negative statement like “Attending physiotherapy sessions does not affect athletes' on-field performance.” Here, the author claims physiotherapy sessions have no effect on on-field performances. Even if there is, it's only a coincidence.
2. Alternative hypothesis
Considered to be the opposite of a null hypothesis, an alternative hypothesis is donated as H1 or Ha. It explicitly states that the dependent variable affects the independent variable. A good alternative hypothesis example is “Attending physiotherapy sessions improves athletes' on-field performance.” or “Water evaporates at 100 °C. ” The alternative hypothesis further branches into directional and non-directional.
- Directional hypothesis: A hypothesis that states the result would be either positive or negative is called directional hypothesis. It accompanies H1 with either the ‘<' or ‘>' sign.
- Non-directional hypothesis: A non-directional hypothesis only claims an effect on the dependent variable. It does not clarify whether the result would be positive or negative. The sign for a non-directional hypothesis is ‘≠.'
3. Simple hypothesis
A simple hypothesis is a statement made to reflect the relation between exactly two variables. One independent and one dependent. Consider the example, “Smoking is a prominent cause of lung cancer." The dependent variable, lung cancer, is dependent on the independent variable, smoking.
4. Complex hypothesis
In contrast to a simple hypothesis, a complex hypothesis implies the relationship between multiple independent and dependent variables. For instance, “Individuals who eat more fruits tend to have higher immunity, lesser cholesterol, and high metabolism.” The independent variable is eating more fruits, while the dependent variables are higher immunity, lesser cholesterol, and high metabolism.
5. Associative and casual hypothesis
Associative and casual hypotheses don't exhibit how many variables there will be. They define the relationship between the variables. In an associative hypothesis, changing any one variable, dependent or independent, affects others. In a casual hypothesis, the independent variable directly affects the dependent.
6. Empirical hypothesis
Also referred to as the working hypothesis, an empirical hypothesis claims a theory's validation via experiments and observation. This way, the statement appears justifiable and different from a wild guess.
Say, the hypothesis is “Women who take iron tablets face a lesser risk of anemia than those who take vitamin B12.” This is an example of an empirical hypothesis where the researcher the statement after assessing a group of women who take iron tablets and charting the findings.
7. Statistical hypothesis
The point of a statistical hypothesis is to test an already existing hypothesis by studying a population sample. Hypothesis like “44% of the Indian population belong in the age group of 22-27.” leverage evidence to prove or disprove a particular statement.
Characteristics of a Good Hypothesis
Writing a hypothesis is essential as it can make or break your research for you. That includes your chances of getting published in a journal. So when you're designing one, keep an eye out for these pointers:
- A research hypothesis has to be simple yet clear to look justifiable enough.
- It has to be testable — your research would be rendered pointless if too far-fetched into reality or limited by technology.
- It has to be precise about the results —what you are trying to do and achieve through it should come out in your hypothesis.
- A research hypothesis should be self-explanatory, leaving no doubt in the reader's mind.
- If you are developing a relational hypothesis, you need to include the variables and establish an appropriate relationship among them.
- A hypothesis must keep and reflect the scope for further investigations and experiments.
Separating a Hypothesis from a Prediction
Outside of academia, hypothesis and prediction are often used interchangeably. In research writing, this is not only confusing but also incorrect. And although a hypothesis and prediction are guesses at their core, there are many differences between them.
A hypothesis is an educated guess or even a testable prediction validated through research. It aims to analyze the gathered evidence and facts to define a relationship between variables and put forth a logical explanation behind the nature of events.
Predictions are assumptions or expected outcomes made without any backing evidence. They are more fictionally inclined regardless of where they originate from.
For this reason, a hypothesis holds much more weight than a prediction. It sticks to the scientific method rather than pure guesswork. "Planets revolve around the Sun." is an example of a hypothesis as it is previous knowledge and observed trends. Additionally, we can test it through the scientific method.
Whereas "COVID-19 will be eradicated by 2030." is a prediction. Even though it results from past trends, we can't prove or disprove it. So, the only way this gets validated is to wait and watch if COVID-19 cases end by 2030.
Finally, How to Write a Hypothesis
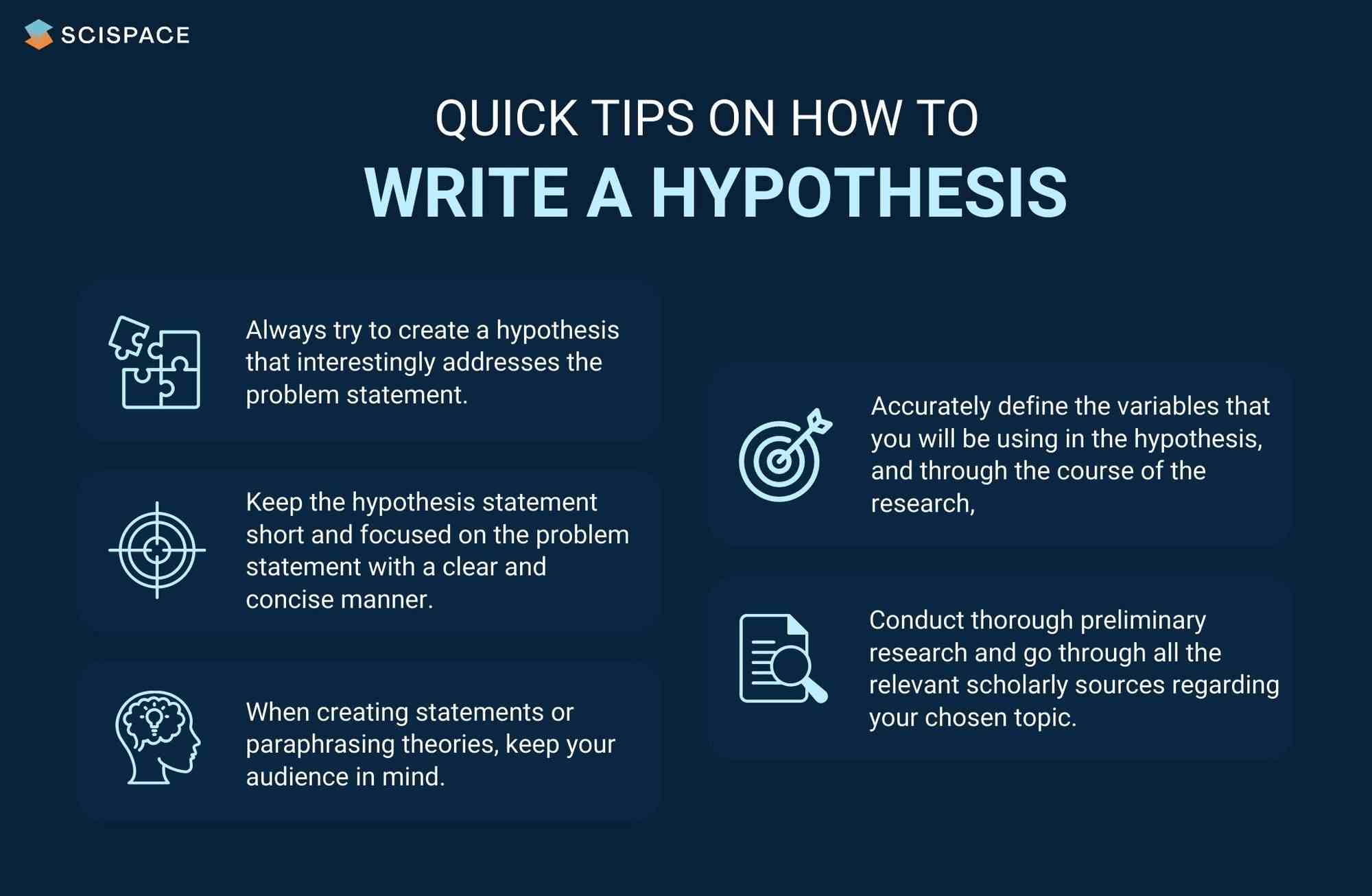
Quick tips on writing a hypothesis
1. Be clear about your research question
A hypothesis should instantly address the research question or the problem statement. To do so, you need to ask a question. Understand the constraints of your undertaken research topic and then formulate a simple and topic-centric problem. Only after that can you develop a hypothesis and further test for evidence.
2. Carry out a recce
Once you have your research's foundation laid out, it would be best to conduct preliminary research. Go through previous theories, academic papers, data, and experiments before you start curating your research hypothesis. It will give you an idea of your hypothesis's viability or originality.
Making use of references from relevant research papers helps draft a good research hypothesis. SciSpace Discover offers a repository of over 270 million research papers to browse through and gain a deeper understanding of related studies on a particular topic. Additionally, you can use SciSpace Copilot , your AI research assistant, for reading any lengthy research paper and getting a more summarized context of it. A hypothesis can be formed after evaluating many such summarized research papers. Copilot also offers explanations for theories and equations, explains paper in simplified version, allows you to highlight any text in the paper or clip math equations and tables and provides a deeper, clear understanding of what is being said. This can improve the hypothesis by helping you identify potential research gaps.
3. Create a 3-dimensional hypothesis
Variables are an essential part of any reasonable hypothesis. So, identify your independent and dependent variable(s) and form a correlation between them. The ideal way to do this is to write the hypothetical assumption in the ‘if-then' form. If you use this form, make sure that you state the predefined relationship between the variables.
In another way, you can choose to present your hypothesis as a comparison between two variables. Here, you must specify the difference you expect to observe in the results.
4. Write the first draft
Now that everything is in place, it's time to write your hypothesis. For starters, create the first draft. In this version, write what you expect to find from your research.
Clearly separate your independent and dependent variables and the link between them. Don't fixate on syntax at this stage. The goal is to ensure your hypothesis addresses the issue.
5. Proof your hypothesis
After preparing the first draft of your hypothesis, you need to inspect it thoroughly. It should tick all the boxes, like being concise, straightforward, relevant, and accurate. Your final hypothesis has to be well-structured as well.
Research projects are an exciting and crucial part of being a scholar. And once you have your research question, you need a great hypothesis to begin conducting research. Thus, knowing how to write a hypothesis is very important.
Now that you have a firmer grasp on what a good hypothesis constitutes, the different kinds there are, and what process to follow, you will find it much easier to write your hypothesis, which ultimately helps your research.
Now it's easier than ever to streamline your research workflow with SciSpace Discover . Its integrated, comprehensive end-to-end platform for research allows scholars to easily discover, write and publish their research and fosters collaboration.
It includes everything you need, including a repository of over 270 million research papers across disciplines, SEO-optimized summaries and public profiles to show your expertise and experience.
If you found these tips on writing a research hypothesis useful, head over to our blog on Statistical Hypothesis Testing to learn about the top researchers, papers, and institutions in this domain.
Frequently Asked Questions (FAQs)
1. what is the definition of hypothesis.
According to the Oxford dictionary, a hypothesis is defined as “An idea or explanation of something that is based on a few known facts, but that has not yet been proved to be true or correct”.
2. What is an example of hypothesis?
The hypothesis is a statement that proposes a relationship between two or more variables. An example: "If we increase the number of new users who join our platform by 25%, then we will see an increase in revenue."
3. What is an example of null hypothesis?
A null hypothesis is a statement that there is no relationship between two variables. The null hypothesis is written as H0. The null hypothesis states that there is no effect. For example, if you're studying whether or not a particular type of exercise increases strength, your null hypothesis will be "there is no difference in strength between people who exercise and people who don't."
4. What are the types of research?
• Fundamental research
• Applied research
• Qualitative research
• Quantitative research
• Mixed research
• Exploratory research
• Longitudinal research
• Cross-sectional research
• Field research
• Laboratory research
• Fixed research
• Flexible research
• Action research
• Policy research
• Classification research
• Comparative research
• Causal research
• Inductive research
• Deductive research
5. How to write a hypothesis?
• Your hypothesis should be able to predict the relationship and outcome.
• Avoid wordiness by keeping it simple and brief.
• Your hypothesis should contain observable and testable outcomes.
• Your hypothesis should be relevant to the research question.
6. What are the 2 types of hypothesis?
• Null hypotheses are used to test the claim that "there is no difference between two groups of data".
• Alternative hypotheses test the claim that "there is a difference between two data groups".
7. Difference between research question and research hypothesis?
A research question is a broad, open-ended question you will try to answer through your research. A hypothesis is a statement based on prior research or theory that you expect to be true due to your study. Example - Research question: What are the factors that influence the adoption of the new technology? Research hypothesis: There is a positive relationship between age, education and income level with the adoption of the new technology.
8. What is plural for hypothesis?
The plural of hypothesis is hypotheses. Here's an example of how it would be used in a statement, "Numerous well-considered hypotheses are presented in this part, and they are supported by tables and figures that are well-illustrated."
9. What is the red queen hypothesis?
The red queen hypothesis in evolutionary biology states that species must constantly evolve to avoid extinction because if they don't, they will be outcompeted by other species that are evolving. Leigh Van Valen first proposed it in 1973; since then, it has been tested and substantiated many times.
10. Who is known as the father of null hypothesis?
The father of the null hypothesis is Sir Ronald Fisher. He published a paper in 1925 that introduced the concept of null hypothesis testing, and he was also the first to use the term itself.
11. When to reject null hypothesis?
You need to find a significant difference between your two populations to reject the null hypothesis. You can determine that by running statistical tests such as an independent sample t-test or a dependent sample t-test. You should reject the null hypothesis if the p-value is less than 0.05.

You might also like

Consensus GPT vs. SciSpace GPT: Choose the Best GPT for Research
Literature Review and Theoretical Framework: Understanding the Differences

Types of Essays in Academic Writing - Quick Guide (2024)

- 中文
- Business Research Methods
/rc_header2020.tmb-hi-sml.jpg?sfvrsn=81a6f1dd_0)
Why Business Research Methods?
Business Research Methods (BRM) is part of your Master's degree. This module will help you make better decisions in situations where a solution to a problem is not immediately obvious because you are missing some of the information.
This module will not just help you in your role as a manager, but also in your personal life, where you will often use research to solve problems or issues. Business Research Methods is a mandatory graduation module at TIAS, and it will certainly prove its worth in your professional or personal career.
According to previous participants, BRM has been invaluable in helping them deal with problems in a structured manner. This module offers an excellent combination of theory and practice, making it highly applicable to real-life situations.
With the Business Research Methods modules, you will:
- Learn when applied research is useful and how to set it up
- Develop the knowledge and skills to conduct applied research
- Learn to value your research reports.
For students doing an entire Master's program at TIAS, both BRM modules are mandatory. The BRM I module takes place at the start of your program and BRM II at the end, just before graduation.
Business Research Methods is for participants who wish to study one of the following Master's programs in full:
- Executive Master of Health Administration (MHA)
- Executive Master in Management and Organization (MMO)
- Executive Master in Information Management (MIM)
- Executive Master of Public and Non-profit Management (MPM)
- Executive Master of Management in Education (MME)
- Executive Master of Operations & Supply Chain Management (MOS)
- Executive Master of Real Estate (MRE)
BRM I - Contents
After a general introduction on conducting research, you will get started on the actual research process and problem analysis.
This process includes the following steps:
- Identifying the organizational problem
- Conducting preliminary research, consisting of exploration (and in many cases a diagnosis)
- Defining the organizational problem
- Drawing up the preconditions for the solution
The organizational problem will form the starting point for the problem definition (including the research objective and research questions). Next, you will practice developing a research proposal and conducting a literature study.
BRM II - Contents
The second module takes place before graduation. You will learn what reviewing literature entails and how to develop a theoretical framework. You will also go over collecting, analyzing, and interpreting both qualitative and quantitative data that are aimed at solving the organizational problem. Based on the data you have collected, you will draw your conclusions and present your recommendations on how to solve the organizational problem. A key part of the module is dedicated to developing the ability to reflect critically on your own and others' research.
Dates and locations
More information, programs at tias school for business & society.
Are you ready for the next step in your career and do you want to create more impact in business and society? TIAS has developed a wide and varied range of courses and learning paths for this purpose. Including MBA courses, Executive Masters, Advanced Programs, InCompany, Modules and Masterclasses. We offer courses and learning paths that match your ambitions, learning goals and career phase.
Our programs
An Introduction to Data Analysis
13.6 testing hypotheses.
The brms package also contains a useful function to address hypotheses about model parameters. The function brms::hypothesis can compute Bayes factors for point-valued hypotheses using the Savage-Dickey method. It also computes a binary test of whether a point-valued hypothesis is credible based on inclusion in a Bayesian credible interval. For interval-valued hypotheses \(\theta \in [a;b]\) , the function brms::hypothesis computes the posterior odds (called evidence ratio in the context of this function): 63 \[ \frac{P(\theta \in [a;b] \mid D)}{P(\theta \not \in [a;b] \mid D)} \]
Computing Bayes factors for point-valued hypotheses with brms::hypothesis requires proper priors for all parameters that are part of the hypothesis. It also requires taking samples from the priors of parameters. 64 So, here is a function call of brms:brm which (i) specifies a reasonably unconstrained but proper parameter for the slope coefficient for year and (ii) also collects samples from the prior (by setting the option sample_prior = "yes" ):
Before addressing hypotheses about the slope parameter for year , let’s remind ourselves of the summary statistics for the posterior:
The main “research hypothesis” of interest is whether the slope for year is credibly positive. This is an interval-valued hypothesis and we can test it like so:
The table shows the estimate for the slope of year , together with an estimated error, lower and upper bounds of a credible interval (95% by default). All of these numbers are rounded. It also shows the “Evidence ratio” which, for an interval-valued hypothesis is not the Bayes factor, but the posterior odds (see above). In the present case, an evidence ratio of Inf means that all posterior samples for the slope coefficient were positive. This is also expressed in the posterior probability (“Post.Prod” in the table) for the proposition that the interval-valued hypothesis is true (given data and model).
The following tests a point-valued hypothesis:
For this point-valued hypothesis, the estimate (and associated error and credible interval) are calculated as a comparison against 0, as shown in the “Hypothesis” column. The evidence ratio given in the results table is the Bayes factor of the point-valued hypothesis against the embedding model (the full regression model with the prior we specified), as calculated by the Savage-Dickey method. As before, the posterior probability is also shown. The “Star” in this table indicates that the point-valued hypothesis is excluded from the computed credible interval, so that - if we adopted the (controversial) binary decision logic discussed in Chapter 11 - we would reject the tested hypothesis.
Notice that for priors where \(P(\theta \in [a;b]) = 0.5\) , the posterior odds equal the Bayes factor. For other priors, we’d need to correct the posterior odds by the priors to obtain Bayes factors, something that the brms package does not (presently seem to) do, unfortunately. ↩︎
It may seem unnecessary to take prior samples for parameters, because, after all, couldn’t we just look at the (closed-form) definition of the prior for that parameter? Well, that only works for top-level parameters, but not parameters in a hierarchical model which depend on the value of other parameters and which therefore have no (easily accessible) closed-form prior to look up. ↩︎
brm: Fit Bayesian Generalized (Non-)Linear Multivariate Multilevel Models
Description.
Fit Bayesian generalized (non-)linear multivariate multilevel models using Stan for full Bayesian inference. A wide range of distributions and link functions are supported, allowing users to fit -- among others -- linear, robust linear, count data, survival, response times, ordinal, zero-inflated, hurdle, and even self-defined mixture models all in a multilevel context. Further modeling options include non-linear and smooth terms, auto-correlation structures, censored data, meta-analytic standard errors, and quite a few more. In addition, all parameters of the response distributions can be predicted in order to perform distributional regression. Prior specifications are flexible and explicitly encourage users to apply prior distributions that actually reflect their beliefs. In addition, model fit can easily be assessed and compared with posterior predictive checks and leave-one-out cross-validation.
An object of class brmsfit , which contains the posterior draws along with many other useful information about the model. Use
methods(class = "brmsfit") for an overview on available methods.
An object of class formula , brmsformula , or mvbrmsformula (or one that can be coerced to that classes): A symbolic description of the model to be fitted. The details of model specification are explained in brmsformula .
An object of class data.frame (or one that can be coerced to that class) containing data of all variables used in the model.
A description of the response distribution and link function to be used in the model. This can be a family function, a call to a family function or a character string naming the family. Every family function has a link argument allowing to specify the link function to be applied on the response variable. If not specified, default links are used. For details of supported families see brmsfamily . By default, a linear gaussian model is applied. In multivariate models, family might also be a list of families.
One or more brmsprior objects created by set_prior or related functions and combined using the c method or the + operator. See also default_prior for more help.
(Deprecated) An optional cor_brms object describing the correlation structure within the response variable (i.e., the 'autocorrelation'). See the documentation of cor_brms for a description of the available correlation structures. Defaults to NULL , corresponding to no correlations. In multivariate models, autocor might also be a list of autocorrelation structures. It is now recommend to specify autocorrelation terms directly within formula . See brmsformula for more details.
A named list of objects containing data, which cannot be passed via argument data . Required for some objects used in autocorrelation structures to specify dependency structures as well as for within-group covariance matrices.
(Deprecated) A list of matrices that are proportional to the (within) covariance structure of the group-level effects. The names of the matrices should correspond to columns in data that are used as grouping factors. All levels of the grouping factor should appear as rownames of the corresponding matrix. This argument can be used, among others to model pedigrees and phylogenetic effects. It is now recommended to specify those matrices in the formula interface using the gr and related functions. See vignette("brms_phylogenetics") for more details.
Indicate if draws from priors should be drawn additionally to the posterior draws. Options are "no" (the default), "yes" , and "only" . Among others, these draws can be used to calculate Bayes factors for point hypotheses via hypothesis . Please note that improper priors are not sampled, including the default improper priors used by brm . See set_prior on how to set (proper) priors. Please also note that prior draws for the overall intercept are not obtained by default for technical reasons. See brmsformula how to obtain prior draws for the intercept. If sample_prior is set to "only" , draws are drawn solely from the priors ignoring the likelihood, which allows among others to generate draws from the prior predictive distribution. In this case, all parameters must have proper priors.
(Deprecated) Logical; indicates whether the population-level design matrices should be treated as sparse (defaults to FALSE ). For design matrices with many zeros, this can considerably reduce required memory. Sampling speed is currently not improved or even slightly decreased. It is now recommended to use the sparse argument of brmsformula and related functions.
Optional list containing user specified knot values to be used for basis construction of smoothing terms. See gamm for more details.
Should unused factors levels in the data be dropped? Defaults to TRUE .
An optional stanvars object generated by function stanvar to define additional variables for use in Stan 's program blocks.
(Deprecated) An optional character string containing self-defined Stan functions, which will be included in the functions block of the generated Stan code. It is now recommended to use the stanvars argument for this purpose instead.
An instance of S3 class brmsfit derived from a previous fit; defaults to NA . If fit is of class brmsfit , the compiled model associated with the fitted result is re-used and all arguments modifying the model code or data are ignored. It is not recommended to use this argument directly, but to call the update method, instead.
An object generated by save_pars controlling which parameters should be saved in the model. The argument has no impact on the model fitting itself.
(Deprecated) A flag to indicate if group-level effects for each level of the grouping factor(s) should be saved (default is TRUE ). Set to FALSE to save memory. The argument has no impact on the model fitting itself.
(Deprecated) A flag to indicate if draws of latent noise-free variables obtained by using me and mi terms should be saved (default is FALSE ). Saving these draws allows to better use methods such as predict with the latent variables but leads to very large R objects even for models of moderate size and complexity.
(Deprecated) A flag to indicate if draws from all variables defined in Stan's parameters block should be saved (default is FALSE ). Saving these draws is required in order to apply the methods bridge_sampler , bayes_factor , and post_prob . Can be set globally for the current R session via the "brms.save_pars" option (see options ).
Initial values for the sampler. If NULL (the default) or "random" , Stan will randomly generate initial values for parameters in a reasonable range. If 0 , all parameters are initialized to zero on the unconstrained space. This option is sometimes useful for certain families, as it happens that default random initial values cause draws to be essentially constant. Generally, setting init = 0 is worth a try, if chains do not initialize or behave well. Alternatively, init can be a list of lists containing the initial values, or a function (or function name) generating initial values. The latter options are mainly implemented for internal testing but are available to users if necessary. If specifying initial values using a list or a function then currently the parameter names must correspond to the names used in the generated Stan code (not the names used in R ). For more details on specifying initial values you can consult the documentation of the selected backend .
(Deprecated) Alias of init .
Number of Markov chains (defaults to 4).
Number of total iterations per chain (including warmup; defaults to 2000).
A positive integer specifying number of warmup (aka burnin) iterations. This also specifies the number of iterations used for stepsize adaptation, so warmup draws should not be used for inference. The number of warmup should not be larger than iter and the default is iter/2 .
Thinning rate. Must be a positive integer. Set thin > 1 to save memory and computation time if iter is large.
Number of cores to use when executing the chains in parallel, which defaults to 1 but we recommend setting the mc.cores option to be as many processors as the hardware and RAM allow (up to the number of chains). For non-Windows OS in non-interactive R sessions, forking is used instead of PSOCK clusters.
Number of threads to use in within-chain parallelization. For more control over the threading process, threads may also be a brmsthreads object created by threading . Within-chain parallelization is experimental! We recommend its use only if you are experienced with Stan's reduce_sum function and have a slow running model that cannot be sped up by any other means. Can be set globally for the current R session via the "brms.threads" option (see options ).
The platform and device IDs of the OpenCL device to use for fitting using GPU support. If you don't know the IDs of your OpenCL device, c(0,0) is most likely what you need. For more details, see opencl . Can be set globally for the current R session via the "brms.opencl" option
Logical. Indicates whether normalization constants should be included in the Stan code (defaults to TRUE ). Setting it to FALSE requires Stan version >= 2.25 to work. If FALSE , sampling efficiency may be increased but some post processing functions such as bridge_sampler will not be available. Can be controlled globally for the current R session via the `brms.normalize` option.
A named list of parameters to control the sampler's behavior. It defaults to NULL so all the default values are used. The most important control parameters are discussed in the 'Details' section below. For a comprehensive overview see stan .
Character string naming the estimation approach to use. Options are "sampling" for MCMC (the default), "meanfield" for variational inference with independent normal distributions, "fullrank" for variational inference with a multivariate normal distribution, or "fixed_param" for sampling from fixed parameter values. Can be set globally for the current R session via the "brms.algorithm" option (see options ).
Character string naming the package to use as the backend for fitting the Stan model. Options are "rstan" (the default) or "cmdstanr" . Can be set globally for the current R session via the "brms.backend" option (see options ). Details on the rstan and cmdstanr packages are available at https://mc-stan.org/rstan/ and https://mc-stan.org/cmdstanr/ , respectively. Additionally a "mock" backend is available to make testing brms and packages that depend on it easier. The "mock" backend does not actually do any fitting, it only checks the generated Stan code for correctness and then returns whatever is passed in an additional mock_fit argument as the result of the fit.
Logical; If TRUE , the future package is used for parallel execution of the chains and argument cores will be ignored. Can be set globally for the current R session via the "future" option. The execution type is controlled via plan (see the examples section below).
Verbosity level between 0 and 2 . If 1 (the default), most of the informational messages of compiler and sampler are suppressed. If 2 , even more messages are suppressed. The actual sampling progress is still printed. Set refresh = 0 to turn this off as well. If using backend = "rstan" you can also set open_progress = FALSE to prevent opening additional progress bars.
The seed for random number generation to make results reproducible. If NA (the default), Stan will set the seed randomly.
Either NULL or a character string. In the latter case, the model's Stan code is saved via cat in a text file named after the string supplied in save_model .
A list of further arguments passed to rstan::stan_model for backend = "rstan" or to cmdstanr::cmdstan_model for backend = "cmdstanr" , which allows to change how models are compiled.
Either NULL or a character string. In the latter case, the fitted model object is saved via saveRDS in a file named after the string supplied in file . The .rds extension is added automatically. If the file already exists, brm will load and return the saved model object instead of refitting the model. Unless you specify the file_refit argument as well, the existing files won't be overwritten, you have to manually remove the file in order to refit and save the model under an existing file name. The file name is stored in the brmsfit object for later usage.
Logical or a character string, specifying one of the compression algorithms supported by saveRDS . If the file argument is provided, this compression will be used when saving the fitted model object.
Modifies when the fit stored via the file argument is re-used. Can be set globally for the current R session via the "brms.file_refit" option (see options ). For "never" (default) the fit is always loaded if it exists and fitting is skipped. For "always" the model is always refitted. If set to "on_change" , brms will refit the model if model, data or algorithm as passed to Stan differ from what is stored in the file. This also covers changes in priors, sample_prior , stanvars , covariance structure, etc. If you believe there was a false positive, you can use brmsfit_needs_refit to see why refit is deemed necessary. Refit will not be triggered for changes in additional parameters of the fit (e.g., initial values, number of iterations, control arguments, ...). A known limitation is that a refit will be triggered if within-chain parallelization is switched on/off.
Logical. If TRUE , the Stan model is not created and compiled and the corresponding 'fit' slot of the brmsfit object will be empty. This is useful if you have estimated a brms-created Stan model outside of brms and want to feed it back into the package.
For internal use only.
Further arguments passed to Stan. For backend = "rstan" the arguments are passed to sampling or vb . For backend = "cmdstanr" the arguments are passed to the cmdstanr::sample or cmdstanr::variational method.
Paul-Christian Buerkner [email protected]
Fit a generalized (non-)linear multivariate multilevel model via full Bayesian inference using Stan. A general overview is provided in the vignettes vignette("brms_overview") and vignette("brms_multilevel") . For a full list of available vignettes see vignette(package = "brms") .
Formula syntax of brms models
Details of the formula syntax applied in brms can be found in brmsformula .
Families and link functions
Details of families supported by brms can be found in brmsfamily .
Prior distributions
Priors should be specified using the set_prior function. Its documentation contains detailed information on how to correctly specify priors. To find out on which parameters or parameter classes priors can be defined, use default_prior . Default priors are chosen to be non or very weakly informative so that their influence on the results will be negligible and you usually don't have to worry about them. However, after getting more familiar with Bayesian statistics, I recommend you to start thinking about reasonable informative priors for your model parameters: Nearly always, there is at least some prior information available that can be used to improve your inference.
Adjusting the sampling behavior of Stan
In addition to choosing the number of iterations, warmup draws, and chains, users can control the behavior of the NUTS sampler, by using the control argument. The most important reason to use control is to decrease (or eliminate at best) the number of divergent transitions that cause a bias in the obtained posterior draws. Whenever you see the warning "There were x divergent transitions after warmup." you should really think about increasing adapt_delta . To do this, write control = list(adapt_delta = <x>) , where <x> should usually be value between 0.8 (current default) and 1 . Increasing adapt_delta will slow down the sampler but will decrease the number of divergent transitions threatening the validity of your posterior draws.
Another problem arises when the depth of the tree being evaluated in each iteration is exceeded. This is less common than having divergent transitions, but may also bias the posterior draws. When it happens, Stan will throw out a warning suggesting to increase max_treedepth , which can be accomplished by writing control = list(max_treedepth = <x>) with a positive integer <x> that should usually be larger than the current default of 10 . For more details on the control argument see stan .
Paul-Christian Buerkner (2017). brms: An R Package for Bayesian Multilevel Models Using Stan. Journal of Statistical Software , 80(1), 1-28. doi:10.18637/jss.v080.i01
Paul-Christian Buerkner (2018). Advanced Bayesian Multilevel Modeling with the R Package brms. The R Journal . 10(1), 395–411. doi:10.32614/RJ-2018-017
brms , brmsformula , brmsfamily , brmsfit
Run the code above in your browser using DataCamp Workspace
Estimating Distributional Models with brms
Paul bürkner, introduction.
This vignette provides an introduction on how to fit distributional regression models with brms . We use the term distributional model to refer to a model, in which we can specify predictor terms for all parameters of the assumed response distribution. In the vast majority of regression model implementations, only the location parameter (usually the mean) of the response distribution depends on the predictors and corresponding regression parameters. Other parameters (e.g., scale or shape parameters) are estimated as auxiliary parameters assuming them to be constant across observations. This assumption is so common that most researchers applying regression models are often (in my experience) not aware of the possibility of relaxing it. This is understandable insofar as relaxing this assumption drastically increase model complexity and thus makes models hard to fit. Fortunately, brms uses Stan on the backend, which is an incredibly flexible and powerful tool for estimating Bayesian models so that model complexity is much less of an issue.
Suppose we have a normally distributed response variable. Then, in basic linear regression, we specify a predictor term \(\eta_{\mu}\) for the mean parameter \(\mu\) of the normal distribution. The second parameter of the normal distribution – the residual standard deviation \(\sigma\) – is assumed to be constant across observations. We estimate \(\sigma\) but do not try to predict it. In a distributional model, however, we do exactly this by specifying a predictor term \(\eta_{\sigma}\) for \(\sigma\) in addition to the predictor term \(\eta_{\mu}\) . Ignoring group-level effects for the moment, the linear predictor of a parameter \(\theta\) for observation \(n\) has the form
\[\eta_{\theta n} = \sum_{i = 1}^{K_{\theta}} b_{\theta i} x_{\theta i n}\] where \(x_{\theta i n}\) denotes the value of the \(i\) th predictor of parameter \(\theta\) for observation \(n\) and \(b_{\theta i}\) is the \(i\) th regression coefficient of parameter \(\theta\) . A distributional normal model with response variable \(y\) can then be written as
\[y_n \sim \mathcal{N}\left(\eta_{\mu n}, \, \exp(\eta_{\sigma n}) \right)\] We used the exponential function around \(\eta_{\sigma}\) to reflect that \(\sigma\) constitutes a standard deviation and thus only takes on positive values, while a linear predictor can be any real number.
A simple distributional model
Unequal variance models are possibly the most simple, but nevertheless very important application of distributional models. Suppose we have two groups of patients: One group receives a treatment (e.g., an antidepressive drug) and another group receives placebo. Since the treatment may not work equally well for all patients, the symptom variance of the treatment group may be larger than the symptom variance of the placebo group after some weeks of treatment. For simplicity, assume that we only investigate the post-treatment values.
The following model estimates the effect of group on both the mean and the residual standard deviation of the normal response distribution.
Useful summary statistics and plots can be obtained via

The population-level effect sigma_grouptreat , which is the contrast of the two residual standard deviations on the log-scale, reveals that the variances of both groups are indeed different. This impression is confirmed when looking at the conditional_effects of group . Going one step further, we can compute the residual standard deviations on the original scale using the hypothesis method.
We may also directly compare them and plot the posterior distribution of their difference.

Indeed, the residual standard deviation of the treatment group seems to larger than that of the placebo group. Moreover the magnitude of this difference is pretty similar to what we expected due to the values we put into the data simulations.
Zero-Inflated Models
Another important application of the distributional regression framework are so called zero-inflated models. These models are helpful whenever there are more zeros in the response variable than one would naturally expect. For example, if one seeks to predict the number of cigarettes people smoke per day and also includes non-smokers, there will be a huge amount of zeros which, when not modeled appropriately, can seriously distort parameter estimates. Here, we consider an example dealing with the number of fish caught by various groups of people. On the UCLA website (), the data are described as follows: “The state wildlife biologists want to model how many fish are being caught by fishermen at a state park. Visitors are asked how long they stayed, how many people were in the group, were there children in the group and how many fish were caught. Some visitors do not fish, but there is no data on whether a person fished or not. Some visitors who did fish did not catch any fish so there are excess zeros in the data because of the people that did not fish.”
As predictors we choose the number of people per group, the number of children, as well as whether the group consists of campers. Many groups may not even try catching any fish at all (thus leading to many zero responses) and so we fit a zero-inflated Poisson model to the data. For now, we assume a constant zero-inflation probability across observations.
Again, we summarize the results using the usual methods.

According to the parameter estimates, larger groups catch more fish, campers catch more fish than non-campers, and groups with more children catch less fish. The zero-inflation probability zi is pretty large with a mean of 41%. Please note that the probability of catching no fish is actually higher than 41%, but parts of this probability are already modeled by the Poisson distribution itself (hence the name zero- inflation ). If you want to treat all zeros as originating from a separate process, you can use hurdle models instead (not shown here).
Now, we try to additionally predict the zero-inflation probability by the number of children. The underlying reasoning is that we expect groups with more children to not even try catching fish. Most children are just terribly bad at waiting for hours until something happens. From a purely statistical perspective, zero-inflated (and hurdle) distributions are a mixture of two processes and predicting both parts of the model is natural and often very reasonable to make full use of the data.

To transform the linear predictor of zi into a probability, brms applies the logit-link:
\[logit(zi) = \log\left(\frac{zi}{1-zi}\right) = \eta_{zi}\]
The logit-link takes values within \([0, 1]\) and returns values on the real line. Thus, it allows the transition between probabilities and linear predictors.
According to the model, trying to fish with children not only decreases the overall number fish caught (as implied by the Poisson part of the model) but also drastically increases your change of catching no fish at all (as implied by the zero-inflation part) most likely because groups with more children are not even trying.
Additive Distributional Models
In the examples so far, we did not have multilevel data and thus did not fully use the capabilities of the distributional regression framework of brms . In the example presented below, we will not only show how to deal with multilevel data in distributional models, but also how to incorporate smooth terms (i.e., splines) into the model. In many applications, we have no or only a very vague idea how the relationship between a predictor and the response looks like. A very flexible approach to tackle this problems is to use splines and let them figure out the form of the relationship. For illustration purposes, we simulate some data with the mgcv package, which is also used in brms to prepare smooth terms.
The data contains the predictors x0 to x3 as well as the grouping factor fac indicating the nested structure of the data. We predict the response variable y using smooth terms of x1 and x2 and a varying intercept of fac . In addition, we assume the residual standard deviation sigma to vary by a smoothing term of x0 and a varying intercept of fac .

This model is likely an overkill for the data at hand, but nicely demonstrates the ease with which one can specify complex models with brms and to fit them using Stan on the backend.
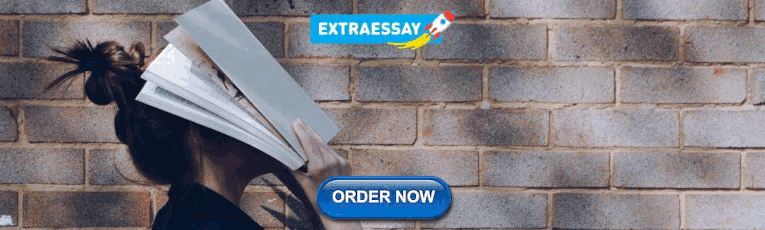
IMAGES
VIDEO
COMMENTS
The formulation of a hypothesis is based on existing knowledge, observations, and theories, and it should be specific, testable, and falsifiable. A specific hypothesis helps to define the research question, which is important in the research process as it guides the selection of an appropriate research design and methodology. Testability of the ...
These transformations are often called "contrasts" or "general linear hypothesis tests". But really, they are just transformations of the joint posterior distribution of the model's parameters. To answer Q2, then, we encode our question into a combination of the models parameters: q2 <- c(q2 = "time + time:treatment1 = 0") The slope ...
The researcher states a hypothesis to be tested, formulates an analysis plan, analyzes sample data. according to the plan, and accepts or rejects the null hypothesis, based on r esults of the ...
12.1.1.1 Brms family. The family argument in brms::brm() is used to define the random part of the model. The brms package extends the options of the family argument in the glm() function to allow for a much wider class of likelihoods. You can see the help file (help("brmsfamily", package="brms")) for a full list of the current options.Some examples of available options are:
The formulation and modelling in log-odds that is the result of the logit transformation are specific to ordered logit regression, however several other methods for modelling binary responses (such as probit models) also have ordinal counterparts (i.e. ordered probit) where the coefficients and cumulative intercepts are interpreted in different ...
7. Different Types of Hypothesis Null Hypothesis: A type of hypothesis used in statistics that proposes that no statistical significance exists in a set of given observations is called a null hypothesis. It is denoted by 'H0'. Alternative Hypothesis: The alternate hypothesis is just an alternative to the null. When we reject the null hypothesis, we accept another hypothesis known as ...
BRM CH 02 FORMULATION OF RESEARCH PROBLEM - Free download as PDF File (.pdf), Text File (.txt) or read online for free.
Formulating Hypotheses for Different Study Designs. Generating a testable working hypothesis is the first step towards conducting original research. Such research may prove or disprove the proposed hypothesis. Case reports, case series, online surveys and other observational studies, clinical trials, and narrative reviews help to generate ...
Building on the ideas in Chap. 1, we describe formulating, testing, and revising hypotheses as a continuing cycle of clarifying what you want to study, making predictions about what you might find together with developing your reasons for these predictions, imagining tests of these predictions, revising your predictions and rationales, and so ...
5. Phrase your hypothesis in three ways. To identify the variables, you can write a simple prediction in if…then form. The first part of the sentence states the independent variable and the second part states the dependent variable. If a first-year student starts attending more lectures, then their exam scores will improve.
Brm lecture9 hypothesis testing - Download as a PDF or view online for free. ... Functions of a Hypothesis • The formulation of a hypothesis provides a study with focus. It tells you what specific aspects of a research problem to investigate; • It tells you what data to collect and what not to collect, thereby providing focus to the study; ...
3. Simple hypothesis. A simple hypothesis is a statement made to reflect the relation between exactly two variables. One independent and one dependent. Consider the example, "Smoking is a prominent cause of lung cancer." The dependent variable, lung cancer, is dependent on the independent variable, smoking. 4.
Extreme values are tails, marginal values are the tails of the bimodals nearest the trough, and modes are modes. During the training phase the dimensions are uncorrelated.) I've come to realize that a cumulative ordered logistic regression is probably the best way to analyze this data. Using the brms package in R, I've specified my model using ...
With the Business Research Methods modules, you will: Learn when applied research is useful and how to set it up. Develop the knowledge and skills to conduct applied research. Learn to value your research reports. For students doing an entire Master's program at TIAS, both BRM modules are mandatory. The BRM I module takes place at the start of ...
An R object typically of class brmsfit. A character vector specifying one or more non-linear hypothesis concerning parameters of the model. A string specifying the class of parameters being tested. Default is "b" for fixed effects. Other typical options are "sd" or "cor".
13.6 Testing hypotheses. The brms package also contains a useful function to address hypotheses about model parameters. The function brms::hypothesis can compute Bayes factors for point-valued hypotheses using the Savage-Dickey method. It also computes a binary test of whether a point-valued hypothesis is credible based on inclusion in a Bayesian credible interval.
Either NULL or a character string. In the latter case, the fitted model object is saved via saveRDS in a file named after the string supplied in file. The .rds extension is added automatically. If the file already exists, brm will load and return the saved model object instead of refitting the model.
It provides a basis for a clear and easily comprehended report at the completion of the study. Example of Hypothesis, Political participation Increases With education; Variable one (dependent variable.) relationship Variable two (independent variable) The result of the hypothesis test is the substance of our conclusion and expressed as ...
An Open Access Journal from The Law Brigade (Publishing) Group 148 JOURNAL OF LEGAL STUDIES AND RESEARCH Volume 6 Issue 6 - ISSN 2455 2437 December 2020 www.thelawbrigade.com MEANING The word hypothesis is made up of two Greek roots which mean that it is some sort of 'sub- statements', for it is the presumptive statement of a proposition, which the investigation seeks
Unit-I BRM - Free download as PDF File (.pdf), Text File (.txt) or read online for free. This document discusses research methodology, including the definition of research, the research process, and types of research. It describes the key steps in the research process as defining the problem, literature review, hypothesis formulation, research design, sampling, data collection, analysis ...
• Hypothesis is considered as an intelligent guess or prediction, that gives directional to the researcher to answer the research question. • Hypothesis or Hypotheses are defined as the formal statement of the tentative or expected prediction or explanation of the relationship between two or more variables in a specified population.
Fortunately, brms uses Stan on the backend, which is an incredibly flexible and powerful tool for estimating Bayesian models so that model complexity is much less of an issue. Suppose we have a normally distributed response variable. Then, in basic linear regression, we specify a predictor term ημ η μ for the mean parameter μ μ of the ...
There are several Hypermarkets - using the word 'selective' (hypermarkets) would frame the RO better Finding Fault in the R.O. BRM Formulation of Research Problem & Hypothesis ' To study a few specific aspects of buying behaviour of young women such as knowledge, attitude and perception towards certain cosmetic products such as Skin ...