Home Blog Design Understanding Data Presentations (Guide + Examples)
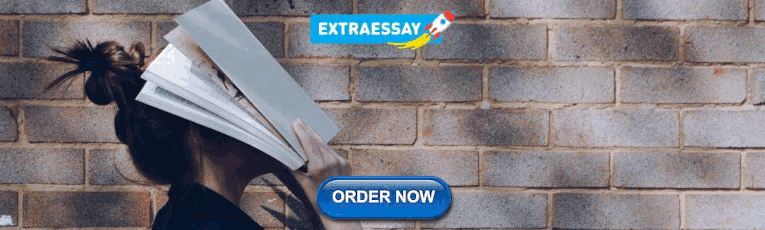
Understanding Data Presentations (Guide + Examples)
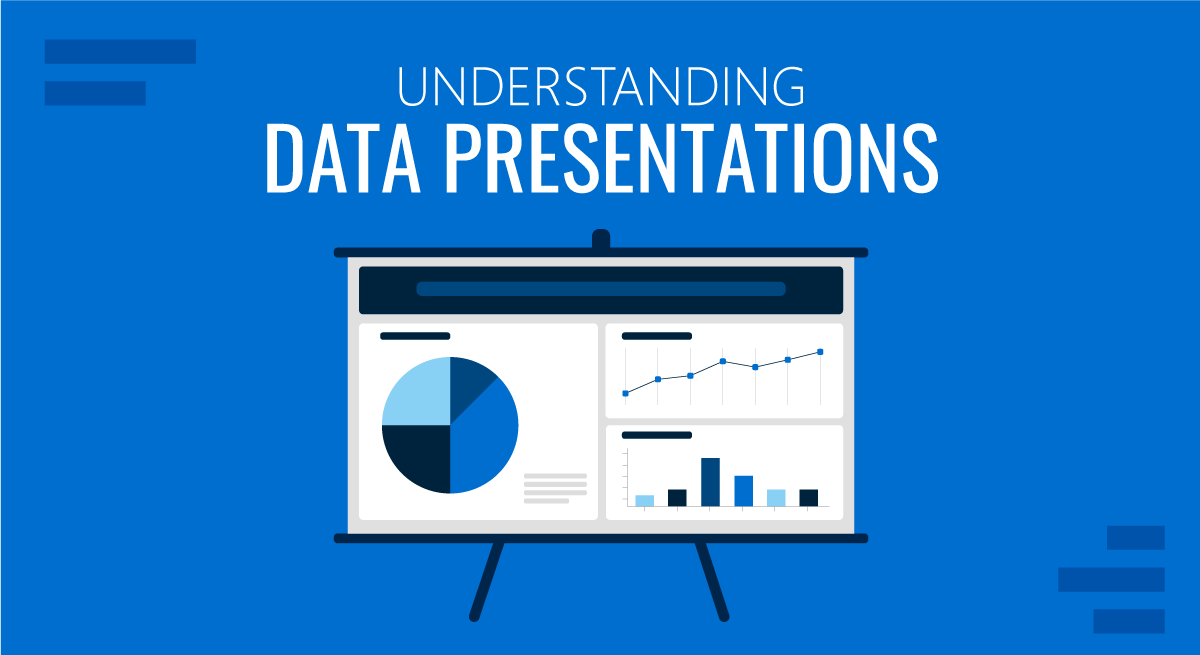
In this age of overwhelming information, the skill to effectively convey data has become extremely valuable. Initiating a discussion on data presentation types involves thoughtful consideration of the nature of your data and the message you aim to convey. Different types of visualizations serve distinct purposes. Whether you’re dealing with how to develop a report or simply trying to communicate complex information, how you present data influences how well your audience understands and engages with it. This extensive guide leads you through the different ways of data presentation.
Table of Contents
What is a Data Presentation?
What should a data presentation include, line graphs, treemap chart, scatter plot, how to choose a data presentation type, recommended data presentation templates, common mistakes done in data presentation.
A data presentation is a slide deck that aims to disclose quantitative information to an audience through the use of visual formats and narrative techniques derived from data analysis, making complex data understandable and actionable. This process requires a series of tools, such as charts, graphs, tables, infographics, dashboards, and so on, supported by concise textual explanations to improve understanding and boost retention rate.
Data presentations require us to cull data in a format that allows the presenter to highlight trends, patterns, and insights so that the audience can act upon the shared information. In a few words, the goal of data presentations is to enable viewers to grasp complicated concepts or trends quickly, facilitating informed decision-making or deeper analysis.
Data presentations go beyond the mere usage of graphical elements. Seasoned presenters encompass visuals with the art of storytelling with data, so the speech skillfully connects the points through a narrative that resonates with the audience. Depending on the purpose – inspire, persuade, inform, support decision-making processes, etc. – is the data presentation format that is better suited to help us in this journey.
To nail your upcoming data presentation, ensure to count with the following elements:
- Clear Objectives: Understand the intent of your presentation before selecting the graphical layout and metaphors to make content easier to grasp.
- Engaging introduction: Use a powerful hook from the get-go. For instance, you can ask a big question or present a problem that your data will answer. Take a look at our guide on how to start a presentation for tips & insights.
- Structured Narrative: Your data presentation must tell a coherent story. This means a beginning where you present the context, a middle section in which you present the data, and an ending that uses a call-to-action. Check our guide on presentation structure for further information.
- Visual Elements: These are the charts, graphs, and other elements of visual communication we ought to use to present data. This article will cover one by one the different types of data representation methods we can use, and provide further guidance on choosing between them.
- Insights and Analysis: This is not just showcasing a graph and letting people get an idea about it. A proper data presentation includes the interpretation of that data, the reason why it’s included, and why it matters to your research.
- Conclusion & CTA: Ending your presentation with a call to action is necessary. Whether you intend to wow your audience into acquiring your services, inspire them to change the world, or whatever the purpose of your presentation, there must be a stage in which you convey all that you shared and show the path to staying in touch. Plan ahead whether you want to use a thank-you slide, a video presentation, or which method is apt and tailored to the kind of presentation you deliver.
- Q&A Session: After your speech is concluded, allocate 3-5 minutes for the audience to raise any questions about the information you disclosed. This is an extra chance to establish your authority on the topic. Check our guide on questions and answer sessions in presentations here.
Bar charts are a graphical representation of data using rectangular bars to show quantities or frequencies in an established category. They make it easy for readers to spot patterns or trends. Bar charts can be horizontal or vertical, although the vertical format is commonly known as a column chart. They display categorical, discrete, or continuous variables grouped in class intervals [1] . They include an axis and a set of labeled bars horizontally or vertically. These bars represent the frequencies of variable values or the values themselves. Numbers on the y-axis of a vertical bar chart or the x-axis of a horizontal bar chart are called the scale.
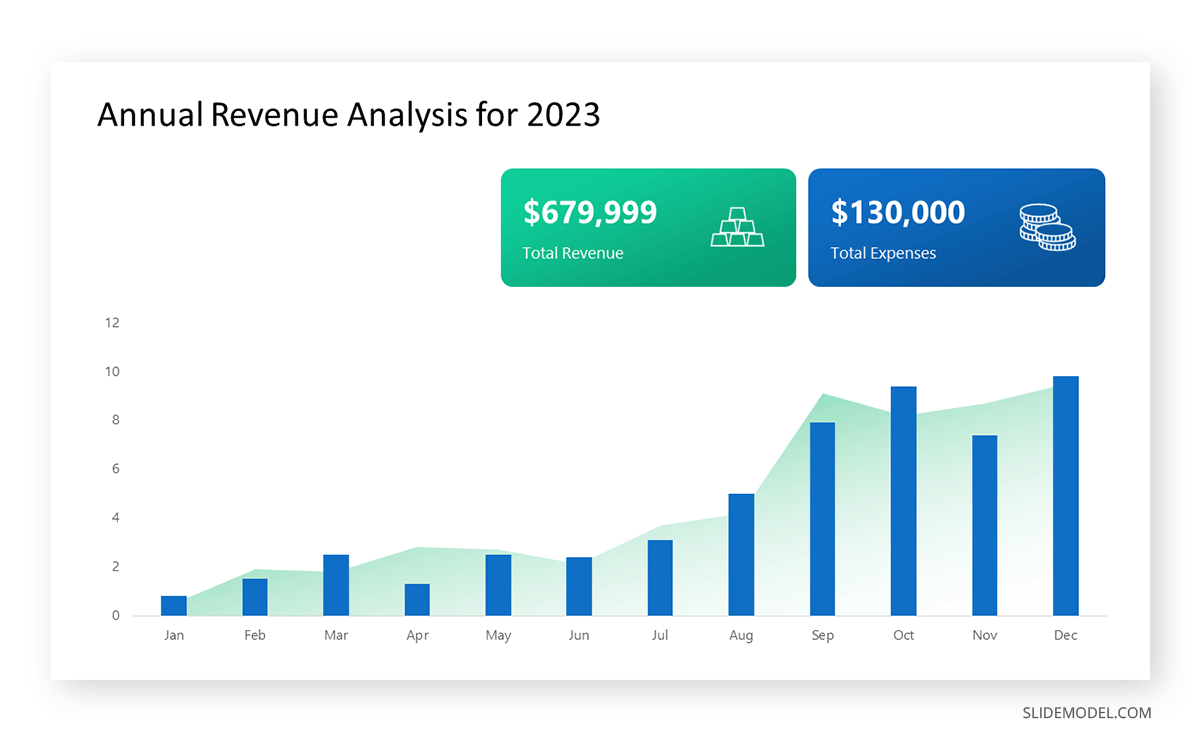
Real-Life Application of Bar Charts
Let’s say a sales manager is presenting sales to their audience. Using a bar chart, he follows these steps.
Step 1: Selecting Data
The first step is to identify the specific data you will present to your audience.
The sales manager has highlighted these products for the presentation.
- Product A: Men’s Shoes
- Product B: Women’s Apparel
- Product C: Electronics
- Product D: Home Decor
Step 2: Choosing Orientation
Opt for a vertical layout for simplicity. Vertical bar charts help compare different categories in case there are not too many categories [1] . They can also help show different trends. A vertical bar chart is used where each bar represents one of the four chosen products. After plotting the data, it is seen that the height of each bar directly represents the sales performance of the respective product.
It is visible that the tallest bar (Electronics – Product C) is showing the highest sales. However, the shorter bars (Women’s Apparel – Product B and Home Decor – Product D) need attention. It indicates areas that require further analysis or strategies for improvement.
Step 3: Colorful Insights
Different colors are used to differentiate each product. It is essential to show a color-coded chart where the audience can distinguish between products.
- Men’s Shoes (Product A): Yellow
- Women’s Apparel (Product B): Orange
- Electronics (Product C): Violet
- Home Decor (Product D): Blue
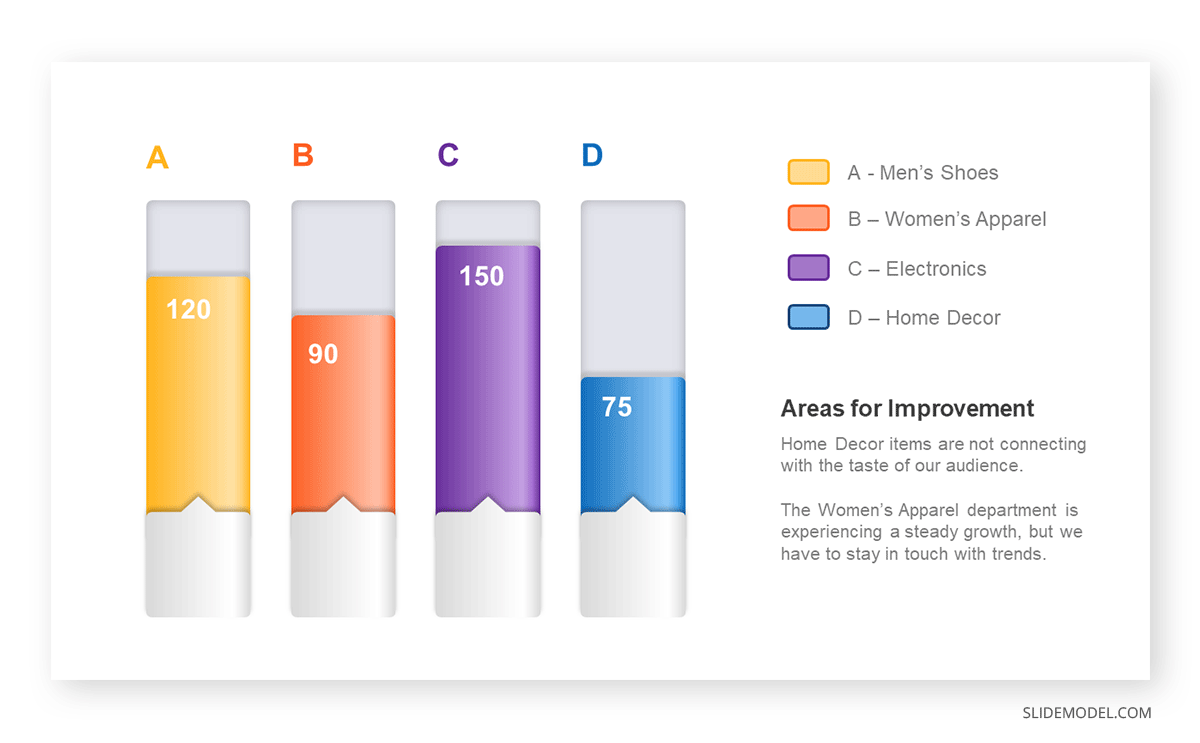
Bar charts are straightforward and easily understandable for presenting data. They are versatile when comparing products or any categorical data [2] . Bar charts adapt seamlessly to retail scenarios. Despite that, bar charts have a few shortcomings. They cannot illustrate data trends over time. Besides, overloading the chart with numerous products can lead to visual clutter, diminishing its effectiveness.
For more information, check our collection of bar chart templates for PowerPoint .
Line graphs help illustrate data trends, progressions, or fluctuations by connecting a series of data points called ‘markers’ with straight line segments. This provides a straightforward representation of how values change [5] . Their versatility makes them invaluable for scenarios requiring a visual understanding of continuous data. In addition, line graphs are also useful for comparing multiple datasets over the same timeline. Using multiple line graphs allows us to compare more than one data set. They simplify complex information so the audience can quickly grasp the ups and downs of values. From tracking stock prices to analyzing experimental results, you can use line graphs to show how data changes over a continuous timeline. They show trends with simplicity and clarity.
Real-life Application of Line Graphs
To understand line graphs thoroughly, we will use a real case. Imagine you’re a financial analyst presenting a tech company’s monthly sales for a licensed product over the past year. Investors want insights into sales behavior by month, how market trends may have influenced sales performance and reception to the new pricing strategy. To present data via a line graph, you will complete these steps.
First, you need to gather the data. In this case, your data will be the sales numbers. For example:
- January: $45,000
- February: $55,000
- March: $45,000
- April: $60,000
- May: $ 70,000
- June: $65,000
- July: $62,000
- August: $68,000
- September: $81,000
- October: $76,000
- November: $87,000
- December: $91,000
After choosing the data, the next step is to select the orientation. Like bar charts, you can use vertical or horizontal line graphs. However, we want to keep this simple, so we will keep the timeline (x-axis) horizontal while the sales numbers (y-axis) vertical.
Step 3: Connecting Trends
After adding the data to your preferred software, you will plot a line graph. In the graph, each month’s sales are represented by data points connected by a line.
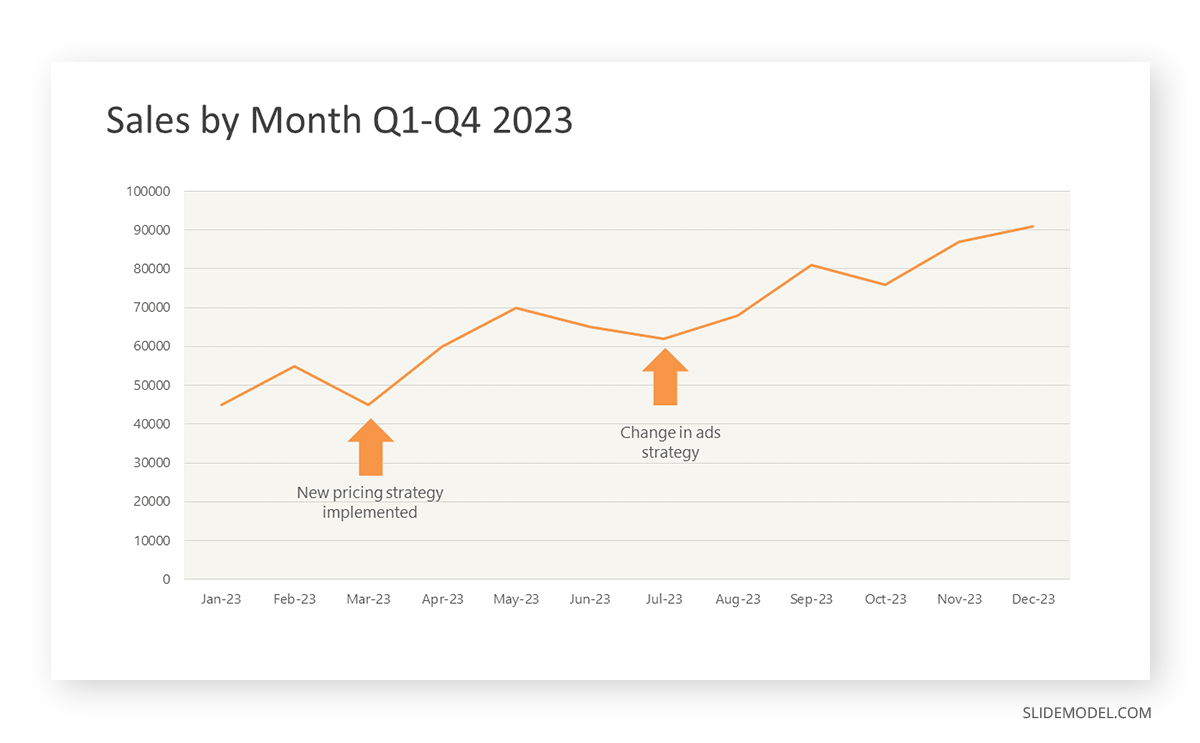
Step 4: Adding Clarity with Color
If there are multiple lines, you can also add colors to highlight each one, making it easier to follow.
Line graphs excel at visually presenting trends over time. These presentation aids identify patterns, like upward or downward trends. However, too many data points can clutter the graph, making it harder to interpret. Line graphs work best with continuous data but are not suitable for categories.
For more information, check our collection of line chart templates for PowerPoint and our article about how to make a presentation graph .
A data dashboard is a visual tool for analyzing information. Different graphs, charts, and tables are consolidated in a layout to showcase the information required to achieve one or more objectives. Dashboards help quickly see Key Performance Indicators (KPIs). You don’t make new visuals in the dashboard; instead, you use it to display visuals you’ve already made in worksheets [3] .
Keeping the number of visuals on a dashboard to three or four is recommended. Adding too many can make it hard to see the main points [4]. Dashboards can be used for business analytics to analyze sales, revenue, and marketing metrics at a time. They are also used in the manufacturing industry, as they allow users to grasp the entire production scenario at the moment while tracking the core KPIs for each line.
Real-Life Application of a Dashboard
Consider a project manager presenting a software development project’s progress to a tech company’s leadership team. He follows the following steps.
Step 1: Defining Key Metrics
To effectively communicate the project’s status, identify key metrics such as completion status, budget, and bug resolution rates. Then, choose measurable metrics aligned with project objectives.
Step 2: Choosing Visualization Widgets
After finalizing the data, presentation aids that align with each metric are selected. For this project, the project manager chooses a progress bar for the completion status and uses bar charts for budget allocation. Likewise, he implements line charts for bug resolution rates.
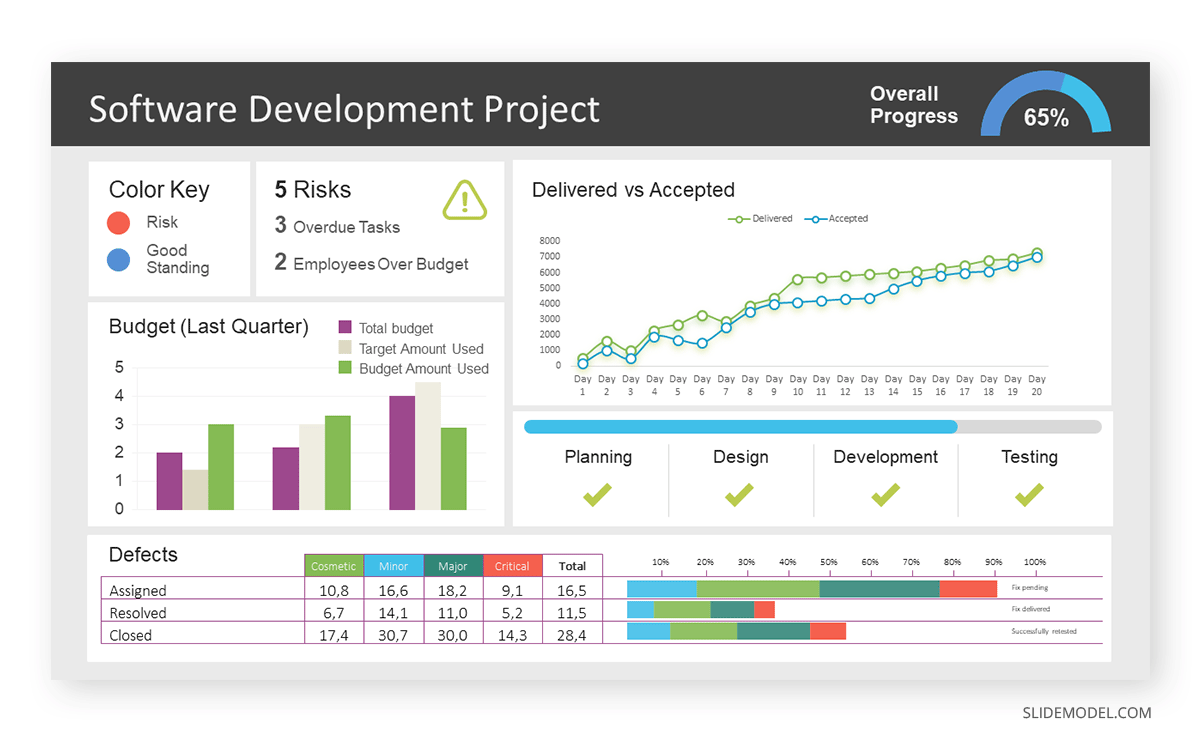
Step 3: Dashboard Layout
Key metrics are prominently placed in the dashboard for easy visibility, and the manager ensures that it appears clean and organized.
Dashboards provide a comprehensive view of key project metrics. Users can interact with data, customize views, and drill down for detailed analysis. However, creating an effective dashboard requires careful planning to avoid clutter. Besides, dashboards rely on the availability and accuracy of underlying data sources.
For more information, check our article on how to design a dashboard presentation , and discover our collection of dashboard PowerPoint templates .
Treemap charts represent hierarchical data structured in a series of nested rectangles [6] . As each branch of the ‘tree’ is given a rectangle, smaller tiles can be seen representing sub-branches, meaning elements on a lower hierarchical level than the parent rectangle. Each one of those rectangular nodes is built by representing an area proportional to the specified data dimension.
Treemaps are useful for visualizing large datasets in compact space. It is easy to identify patterns, such as which categories are dominant. Common applications of the treemap chart are seen in the IT industry, such as resource allocation, disk space management, website analytics, etc. Also, they can be used in multiple industries like healthcare data analysis, market share across different product categories, or even in finance to visualize portfolios.
Real-Life Application of a Treemap Chart
Let’s consider a financial scenario where a financial team wants to represent the budget allocation of a company. There is a hierarchy in the process, so it is helpful to use a treemap chart. In the chart, the top-level rectangle could represent the total budget, and it would be subdivided into smaller rectangles, each denoting a specific department. Further subdivisions within these smaller rectangles might represent individual projects or cost categories.
Step 1: Define Your Data Hierarchy
While presenting data on the budget allocation, start by outlining the hierarchical structure. The sequence will be like the overall budget at the top, followed by departments, projects within each department, and finally, individual cost categories for each project.
- Top-level rectangle: Total Budget
- Second-level rectangles: Departments (Engineering, Marketing, Sales)
- Third-level rectangles: Projects within each department
- Fourth-level rectangles: Cost categories for each project (Personnel, Marketing Expenses, Equipment)
Step 2: Choose a Suitable Tool
It’s time to select a data visualization tool supporting Treemaps. Popular choices include Tableau, Microsoft Power BI, PowerPoint, or even coding with libraries like D3.js. It is vital to ensure that the chosen tool provides customization options for colors, labels, and hierarchical structures.
Here, the team uses PowerPoint for this guide because of its user-friendly interface and robust Treemap capabilities.
Step 3: Make a Treemap Chart with PowerPoint
After opening the PowerPoint presentation, they chose “SmartArt” to form the chart. The SmartArt Graphic window has a “Hierarchy” category on the left. Here, you will see multiple options. You can choose any layout that resembles a Treemap. The “Table Hierarchy” or “Organization Chart” options can be adapted. The team selects the Table Hierarchy as it looks close to a Treemap.
Step 5: Input Your Data
After that, a new window will open with a basic structure. They add the data one by one by clicking on the text boxes. They start with the top-level rectangle, representing the total budget.
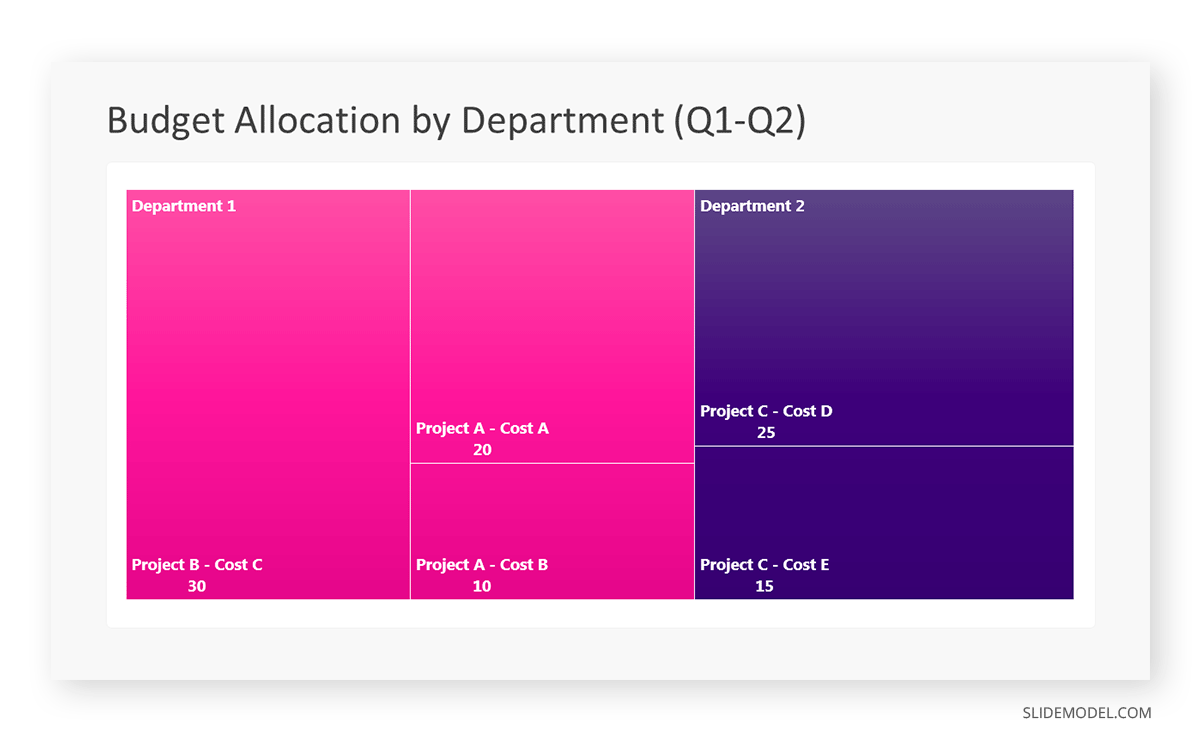
Step 6: Customize the Treemap
By clicking on each shape, they customize its color, size, and label. At the same time, they can adjust the font size, style, and color of labels by using the options in the “Format” tab in PowerPoint. Using different colors for each level enhances the visual difference.
Treemaps excel at illustrating hierarchical structures. These charts make it easy to understand relationships and dependencies. They efficiently use space, compactly displaying a large amount of data, reducing the need for excessive scrolling or navigation. Additionally, using colors enhances the understanding of data by representing different variables or categories.
In some cases, treemaps might become complex, especially with deep hierarchies. It becomes challenging for some users to interpret the chart. At the same time, displaying detailed information within each rectangle might be constrained by space. It potentially limits the amount of data that can be shown clearly. Without proper labeling and color coding, there’s a risk of misinterpretation.
A heatmap is a data visualization tool that uses color coding to represent values across a two-dimensional surface. In these, colors replace numbers to indicate the magnitude of each cell. This color-shaded matrix display is valuable for summarizing and understanding data sets with a glance [7] . The intensity of the color corresponds to the value it represents, making it easy to identify patterns, trends, and variations in the data.
As a tool, heatmaps help businesses analyze website interactions, revealing user behavior patterns and preferences to enhance overall user experience. In addition, companies use heatmaps to assess content engagement, identifying popular sections and areas of improvement for more effective communication. They excel at highlighting patterns and trends in large datasets, making it easy to identify areas of interest.
We can implement heatmaps to express multiple data types, such as numerical values, percentages, or even categorical data. Heatmaps help us easily spot areas with lots of activity, making them helpful in figuring out clusters [8] . When making these maps, it is important to pick colors carefully. The colors need to show the differences between groups or levels of something. And it is good to use colors that people with colorblindness can easily see.
Check our detailed guide on how to create a heatmap here. Also discover our collection of heatmap PowerPoint templates .
Pie charts are circular statistical graphics divided into slices to illustrate numerical proportions. Each slice represents a proportionate part of the whole, making it easy to visualize the contribution of each component to the total.
The size of the pie charts is influenced by the value of data points within each pie. The total of all data points in a pie determines its size. The pie with the highest data points appears as the largest, whereas the others are proportionally smaller. However, you can present all pies of the same size if proportional representation is not required [9] . Sometimes, pie charts are difficult to read, or additional information is required. A variation of this tool can be used instead, known as the donut chart , which has the same structure but a blank center, creating a ring shape. Presenters can add extra information, and the ring shape helps to declutter the graph.
Pie charts are used in business to show percentage distribution, compare relative sizes of categories, or present straightforward data sets where visualizing ratios is essential.
Real-Life Application of Pie Charts
Consider a scenario where you want to represent the distribution of the data. Each slice of the pie chart would represent a different category, and the size of each slice would indicate the percentage of the total portion allocated to that category.
Step 1: Define Your Data Structure
Imagine you are presenting the distribution of a project budget among different expense categories.
- Column A: Expense Categories (Personnel, Equipment, Marketing, Miscellaneous)
- Column B: Budget Amounts ($40,000, $30,000, $20,000, $10,000) Column B represents the values of your categories in Column A.
Step 2: Insert a Pie Chart
Using any of the accessible tools, you can create a pie chart. The most convenient tools for forming a pie chart in a presentation are presentation tools such as PowerPoint or Google Slides. You will notice that the pie chart assigns each expense category a percentage of the total budget by dividing it by the total budget.
For instance:
- Personnel: $40,000 / ($40,000 + $30,000 + $20,000 + $10,000) = 40%
- Equipment: $30,000 / ($40,000 + $30,000 + $20,000 + $10,000) = 30%
- Marketing: $20,000 / ($40,000 + $30,000 + $20,000 + $10,000) = 20%
- Miscellaneous: $10,000 / ($40,000 + $30,000 + $20,000 + $10,000) = 10%
You can make a chart out of this or just pull out the pie chart from the data.
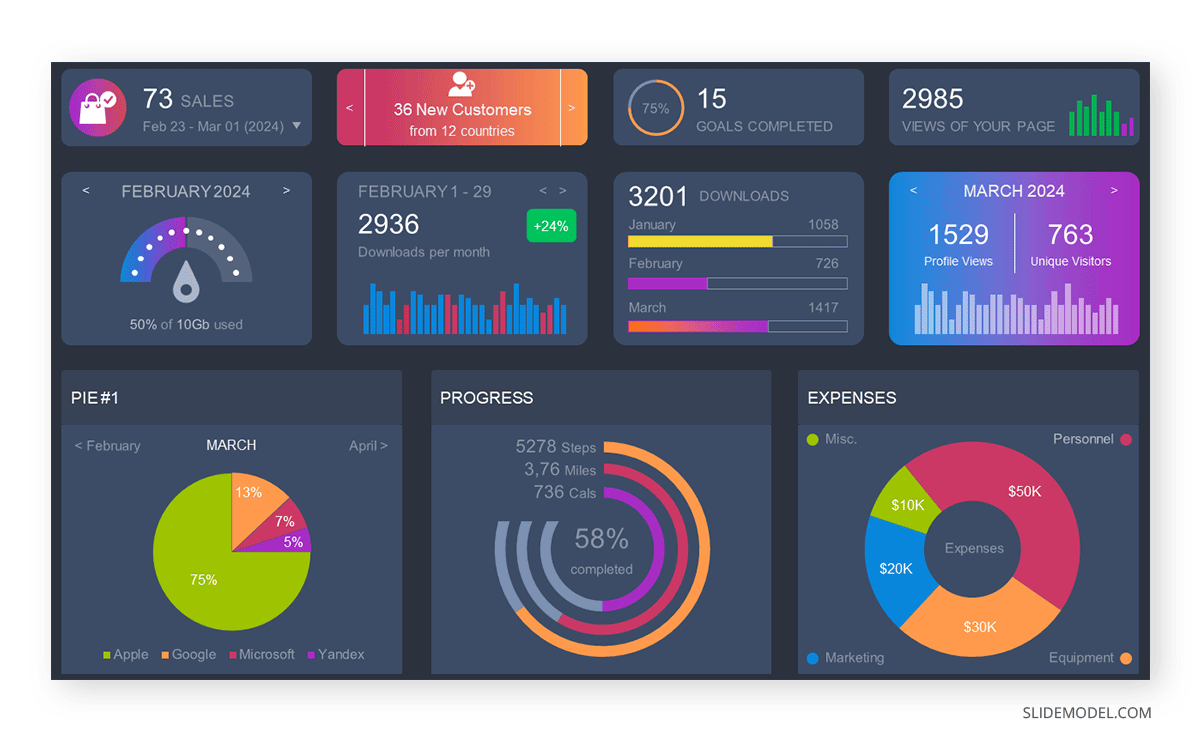
3D pie charts and 3D donut charts are quite popular among the audience. They stand out as visual elements in any presentation slide, so let’s take a look at how our pie chart example would look in 3D pie chart format.
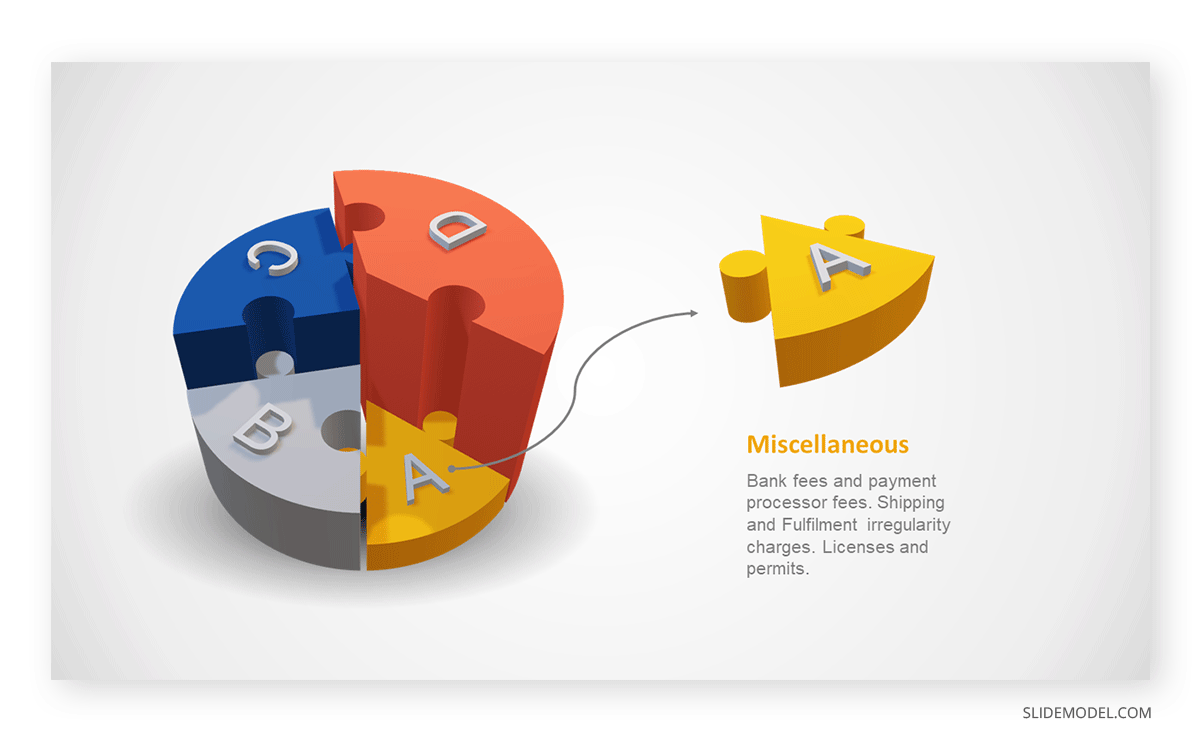
Step 03: Results Interpretation
The pie chart visually illustrates the distribution of the project budget among different expense categories. Personnel constitutes the largest portion at 40%, followed by equipment at 30%, marketing at 20%, and miscellaneous at 10%. This breakdown provides a clear overview of where the project funds are allocated, which helps in informed decision-making and resource management. It is evident that personnel are a significant investment, emphasizing their importance in the overall project budget.
Pie charts provide a straightforward way to represent proportions and percentages. They are easy to understand, even for individuals with limited data analysis experience. These charts work well for small datasets with a limited number of categories.
However, a pie chart can become cluttered and less effective in situations with many categories. Accurate interpretation may be challenging, especially when dealing with slight differences in slice sizes. In addition, these charts are static and do not effectively convey trends over time.
For more information, check our collection of pie chart templates for PowerPoint .
Histograms present the distribution of numerical variables. Unlike a bar chart that records each unique response separately, histograms organize numeric responses into bins and show the frequency of reactions within each bin [10] . The x-axis of a histogram shows the range of values for a numeric variable. At the same time, the y-axis indicates the relative frequencies (percentage of the total counts) for that range of values.
Whenever you want to understand the distribution of your data, check which values are more common, or identify outliers, histograms are your go-to. Think of them as a spotlight on the story your data is telling. A histogram can provide a quick and insightful overview if you’re curious about exam scores, sales figures, or any numerical data distribution.
Real-Life Application of a Histogram
In the histogram data analysis presentation example, imagine an instructor analyzing a class’s grades to identify the most common score range. A histogram could effectively display the distribution. It will show whether most students scored in the average range or if there are significant outliers.
Step 1: Gather Data
He begins by gathering the data. The scores of each student in class are gathered to analyze exam scores.
After arranging the scores in ascending order, bin ranges are set.
Step 2: Define Bins
Bins are like categories that group similar values. Think of them as buckets that organize your data. The presenter decides how wide each bin should be based on the range of the values. For instance, the instructor sets the bin ranges based on score intervals: 60-69, 70-79, 80-89, and 90-100.
Step 3: Count Frequency
Now, he counts how many data points fall into each bin. This step is crucial because it tells you how often specific ranges of values occur. The result is the frequency distribution, showing the occurrences of each group.
Here, the instructor counts the number of students in each category.
- 60-69: 1 student (Kate)
- 70-79: 4 students (David, Emma, Grace, Jack)
- 80-89: 7 students (Alice, Bob, Frank, Isabel, Liam, Mia, Noah)
- 90-100: 3 students (Clara, Henry, Olivia)
Step 4: Create the Histogram
It’s time to turn the data into a visual representation. Draw a bar for each bin on a graph. The width of the bar should correspond to the range of the bin, and the height should correspond to the frequency. To make your histogram understandable, label the X and Y axes.
In this case, the X-axis should represent the bins (e.g., test score ranges), and the Y-axis represents the frequency.
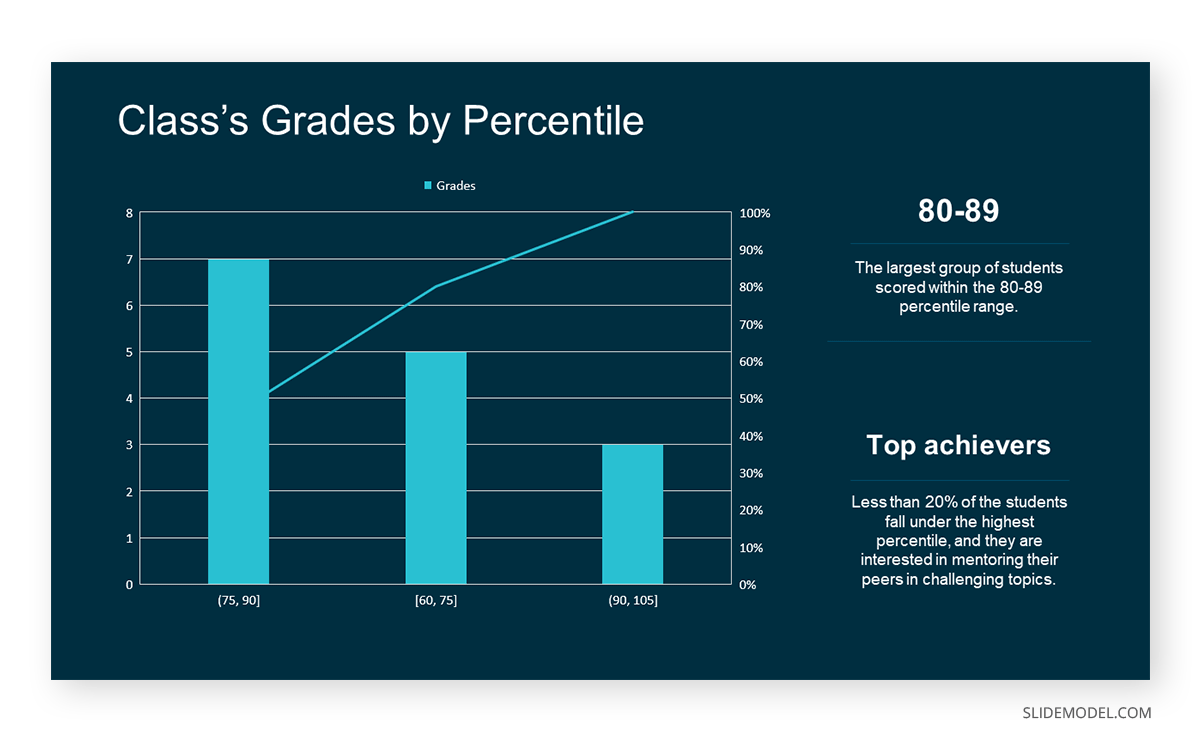
The histogram of the class grades reveals insightful patterns in the distribution. Most students, with seven students, fall within the 80-89 score range. The histogram provides a clear visualization of the class’s performance. It showcases a concentration of grades in the upper-middle range with few outliers at both ends. This analysis helps in understanding the overall academic standing of the class. It also identifies the areas for potential improvement or recognition.
Thus, histograms provide a clear visual representation of data distribution. They are easy to interpret, even for those without a statistical background. They apply to various types of data, including continuous and discrete variables. One weak point is that histograms do not capture detailed patterns in students’ data, with seven compared to other visualization methods.
A scatter plot is a graphical representation of the relationship between two variables. It consists of individual data points on a two-dimensional plane. This plane plots one variable on the x-axis and the other on the y-axis. Each point represents a unique observation. It visualizes patterns, trends, or correlations between the two variables.
Scatter plots are also effective in revealing the strength and direction of relationships. They identify outliers and assess the overall distribution of data points. The points’ dispersion and clustering reflect the relationship’s nature, whether it is positive, negative, or lacks a discernible pattern. In business, scatter plots assess relationships between variables such as marketing cost and sales revenue. They help present data correlations and decision-making.
Real-Life Application of Scatter Plot
A group of scientists is conducting a study on the relationship between daily hours of screen time and sleep quality. After reviewing the data, they managed to create this table to help them build a scatter plot graph:
In the provided example, the x-axis represents Daily Hours of Screen Time, and the y-axis represents the Sleep Quality Rating.
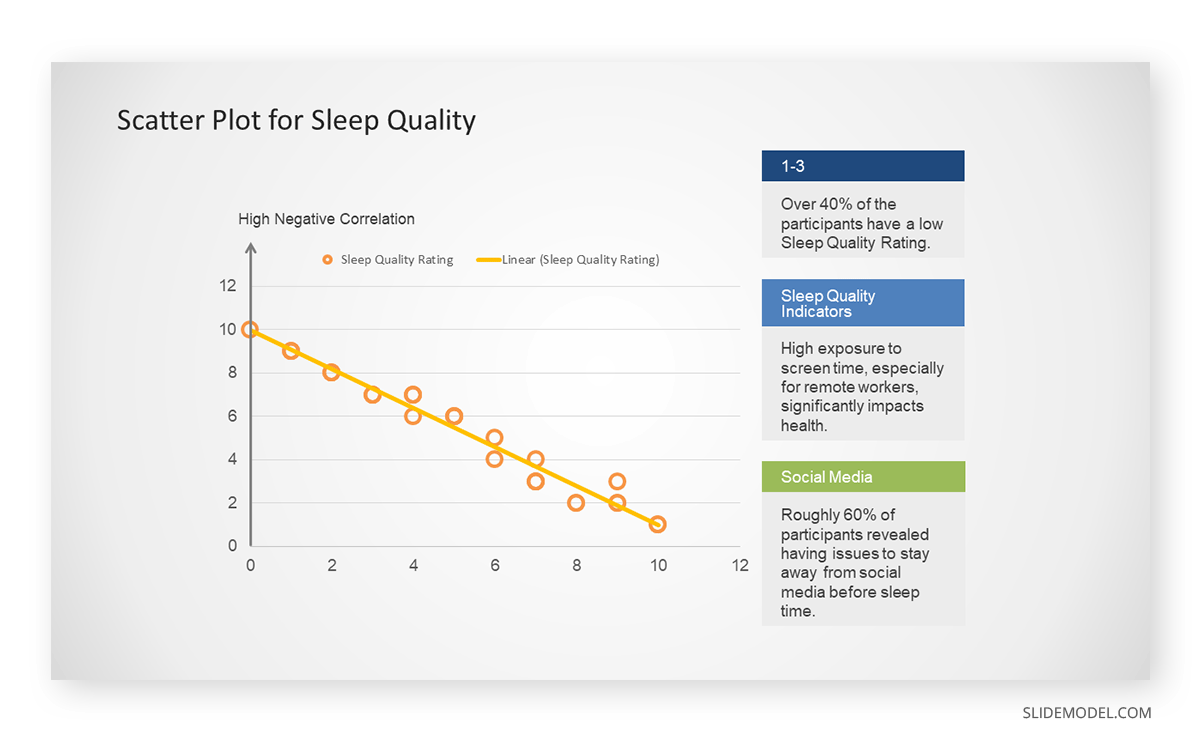
The scientists observe a negative correlation between the amount of screen time and the quality of sleep. This is consistent with their hypothesis that blue light, especially before bedtime, has a significant impact on sleep quality and metabolic processes.
There are a few things to remember when using a scatter plot. Even when a scatter diagram indicates a relationship, it doesn’t mean one variable affects the other. A third factor can influence both variables. The more the plot resembles a straight line, the stronger the relationship is perceived [11] . If it suggests no ties, the observed pattern might be due to random fluctuations in data. When the scatter diagram depicts no correlation, whether the data might be stratified is worth considering.
Choosing the appropriate data presentation type is crucial when making a presentation . Understanding the nature of your data and the message you intend to convey will guide this selection process. For instance, when showcasing quantitative relationships, scatter plots become instrumental in revealing correlations between variables. If the focus is on emphasizing parts of a whole, pie charts offer a concise display of proportions. Histograms, on the other hand, prove valuable for illustrating distributions and frequency patterns.
Bar charts provide a clear visual comparison of different categories. Likewise, line charts excel in showcasing trends over time, while tables are ideal for detailed data examination. Starting a presentation on data presentation types involves evaluating the specific information you want to communicate and selecting the format that aligns with your message. This ensures clarity and resonance with your audience from the beginning of your presentation.
1. Fact Sheet Dashboard for Data Presentation
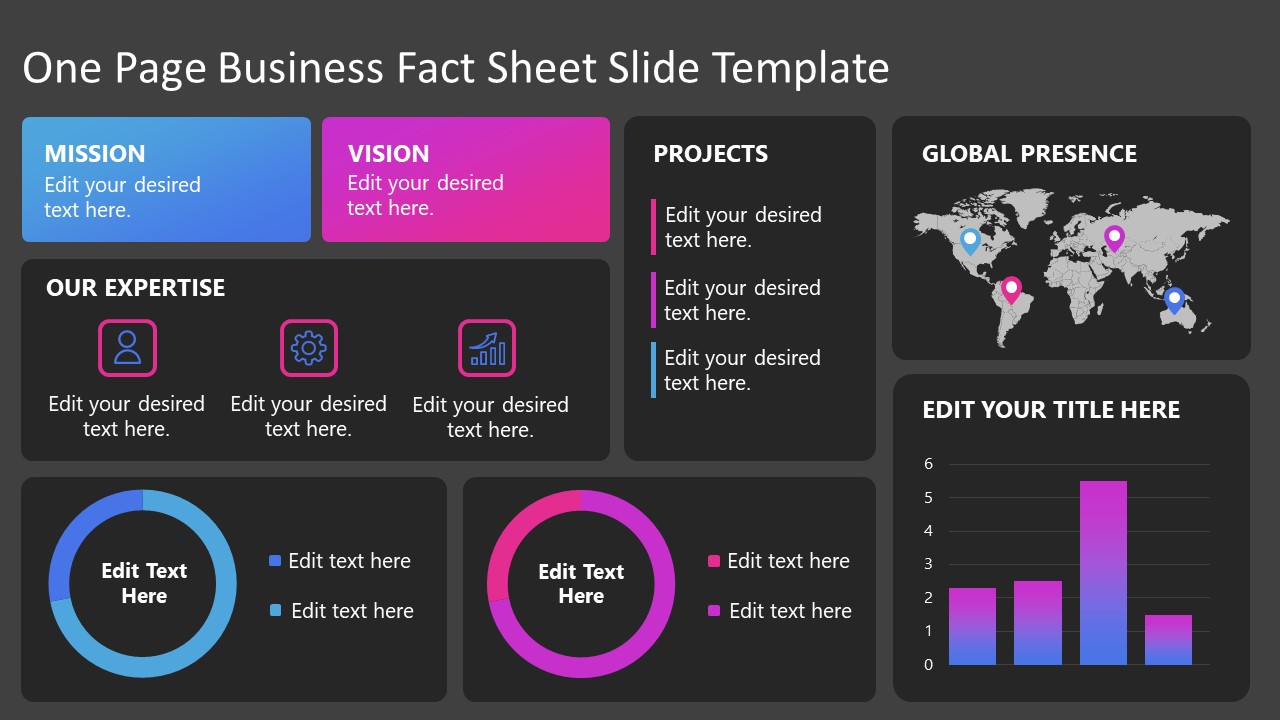
Convey all the data you need to present in this one-pager format, an ideal solution tailored for users looking for presentation aids. Global maps, donut chats, column graphs, and text neatly arranged in a clean layout presented in light and dark themes.
Use This Template
2. 3D Column Chart Infographic PPT Template
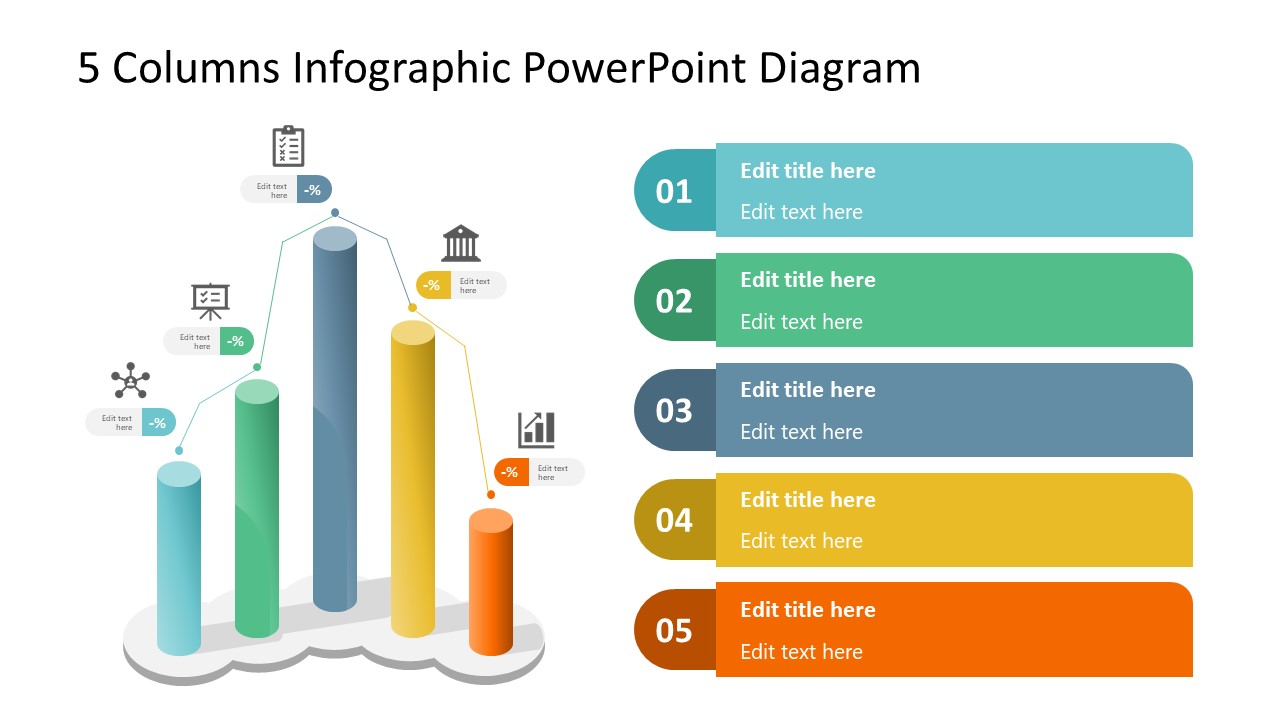
Represent column charts in a highly visual 3D format with this PPT template. A creative way to present data, this template is entirely editable, and we can craft either a one-page infographic or a series of slides explaining what we intend to disclose point by point.
3. Data Circles Infographic PowerPoint Template
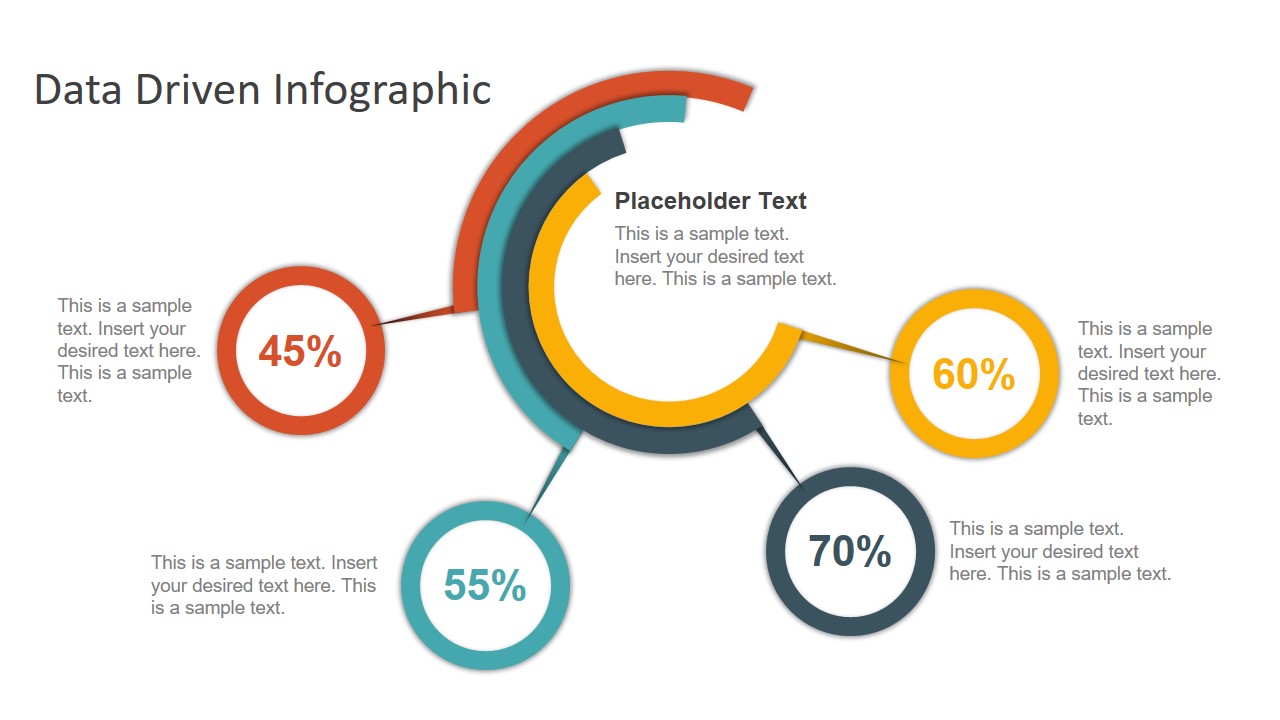
An alternative to the pie chart and donut chart diagrams, this template features a series of curved shapes with bubble callouts as ways of presenting data. Expand the information for each arch in the text placeholder areas.
4. Colorful Metrics Dashboard for Data Presentation
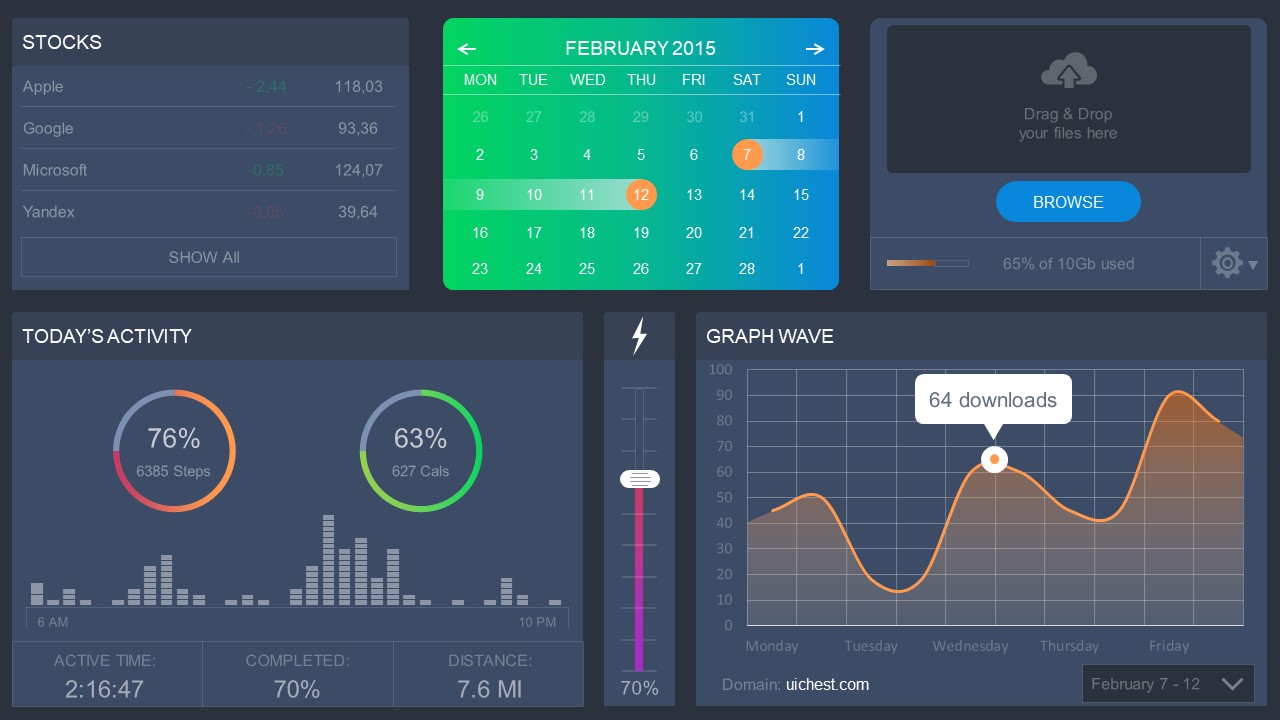
This versatile dashboard template helps us in the presentation of the data by offering several graphs and methods to convert numbers into graphics. Implement it for e-commerce projects, financial projections, project development, and more.
5. Animated Data Presentation Tools for PowerPoint & Google Slides
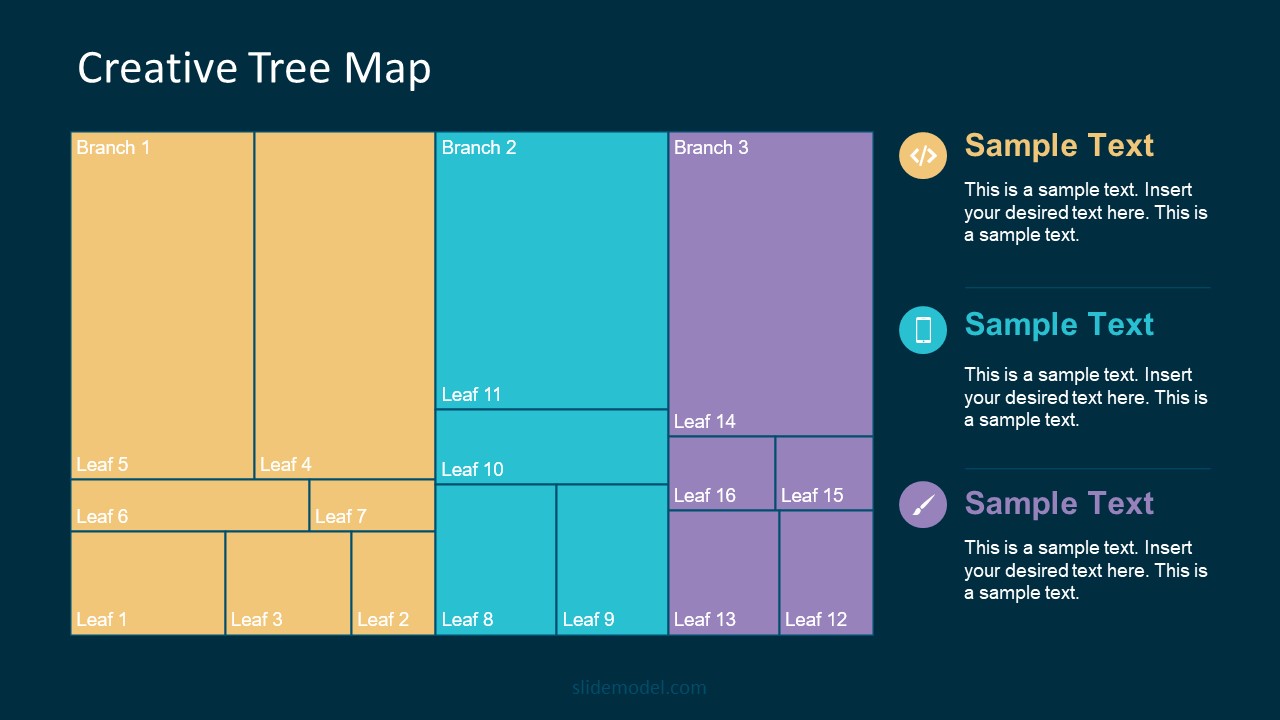
A slide deck filled with most of the tools mentioned in this article, from bar charts, column charts, treemap graphs, pie charts, histogram, etc. Animated effects make each slide look dynamic when sharing data with stakeholders.
6. Statistics Waffle Charts PPT Template for Data Presentations
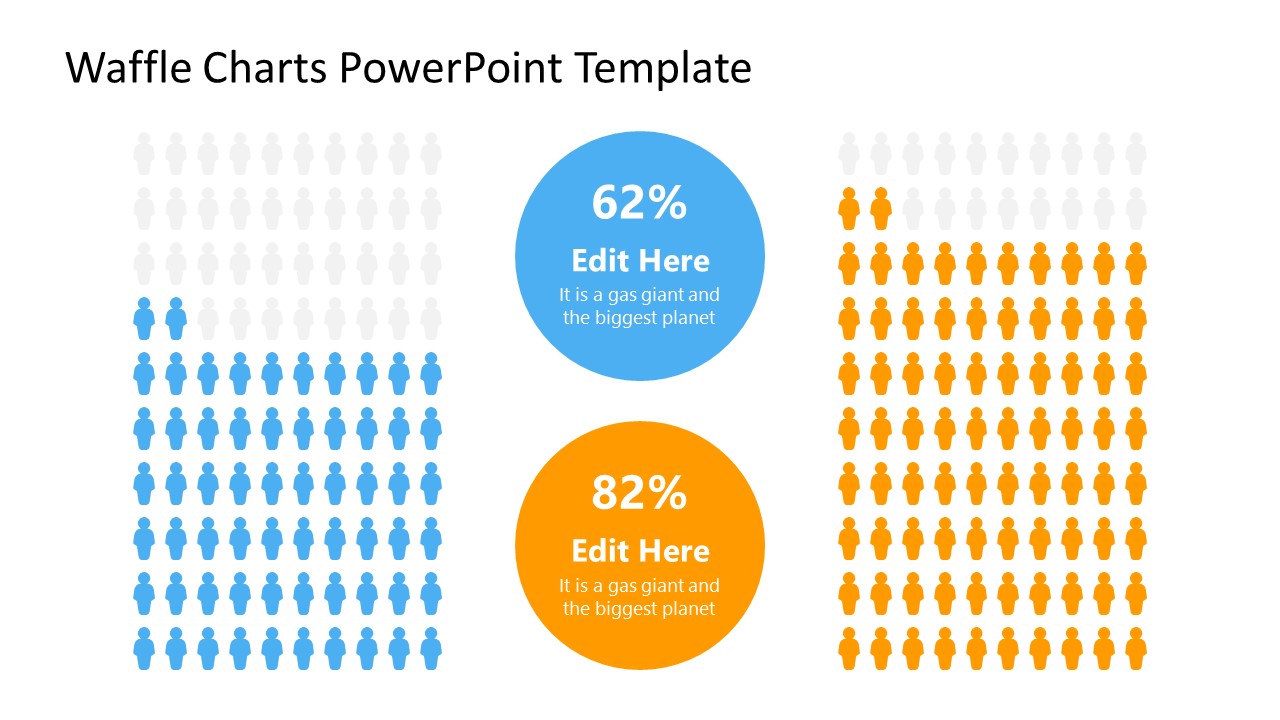
This PPT template helps us how to present data beyond the typical pie chart representation. It is widely used for demographics, so it’s a great fit for marketing teams, data science professionals, HR personnel, and more.
7. Data Presentation Dashboard Template for Google Slides
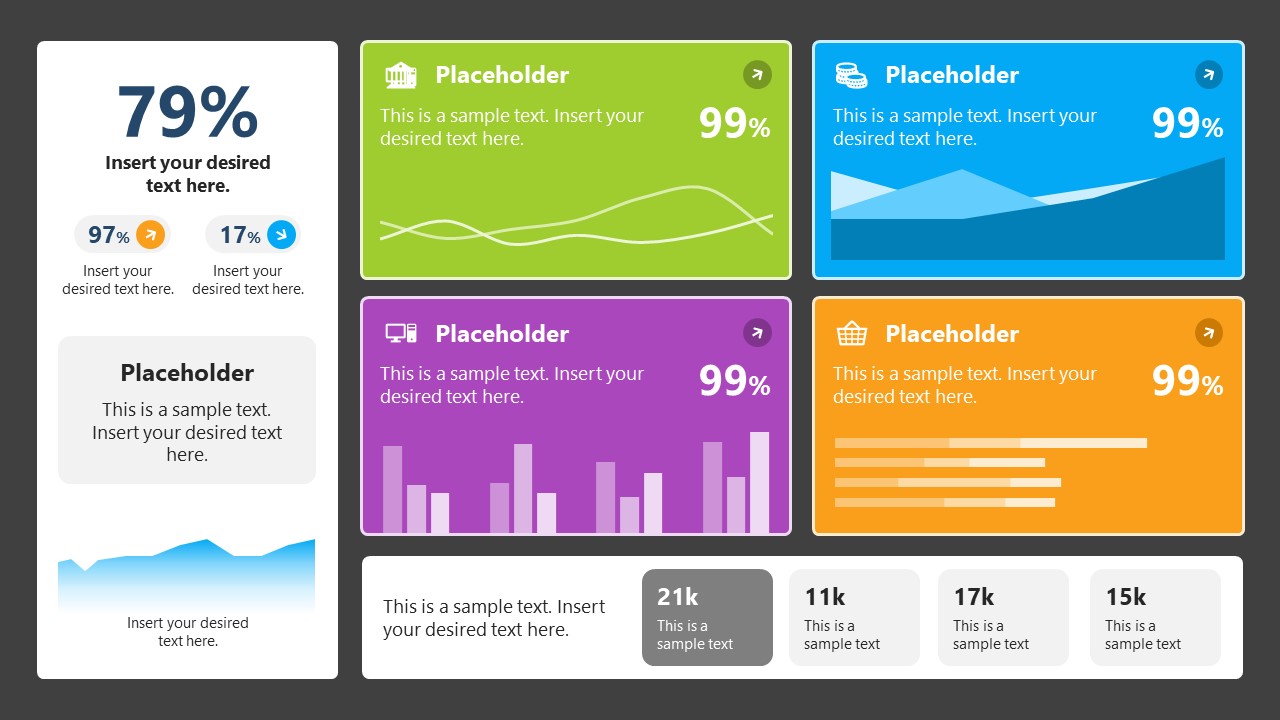
A compendium of tools in dashboard format featuring line graphs, bar charts, column charts, and neatly arranged placeholder text areas.
8. Weather Dashboard for Data Presentation
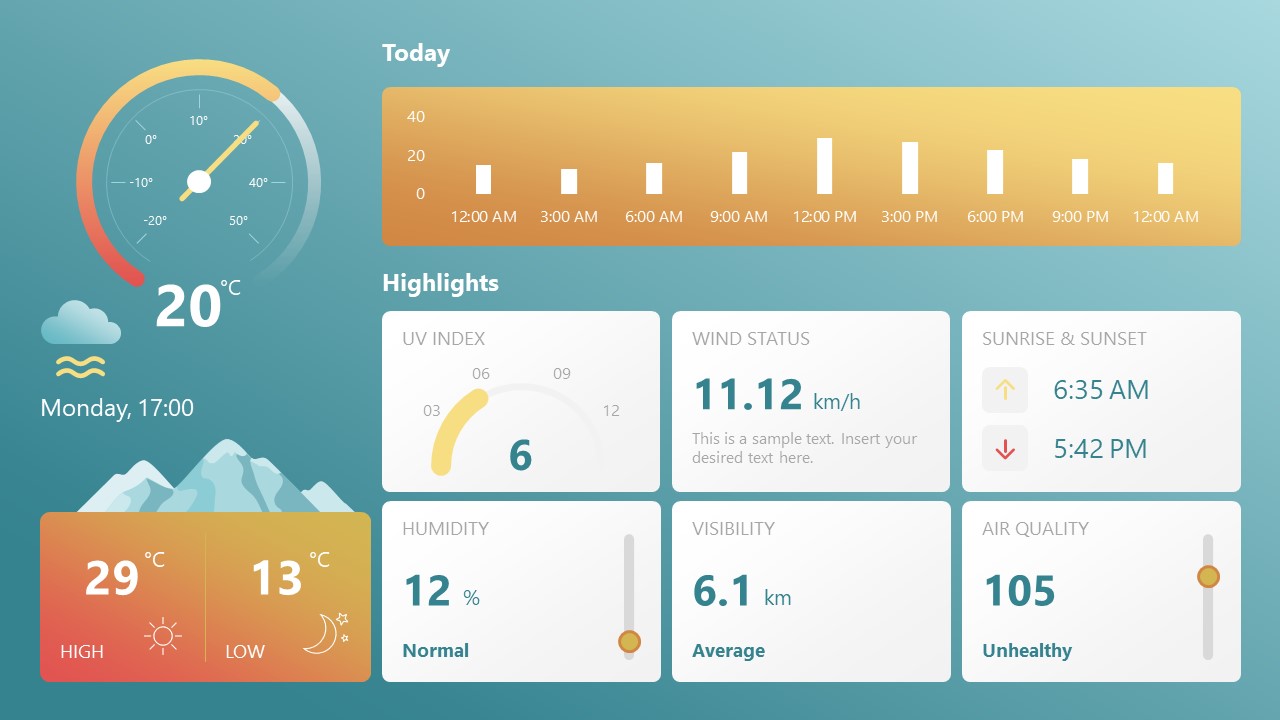
Share weather data for agricultural presentation topics, environmental studies, or any kind of presentation that requires a highly visual layout for weather forecasting on a single day. Two color themes are available.
9. Social Media Marketing Dashboard Data Presentation Template
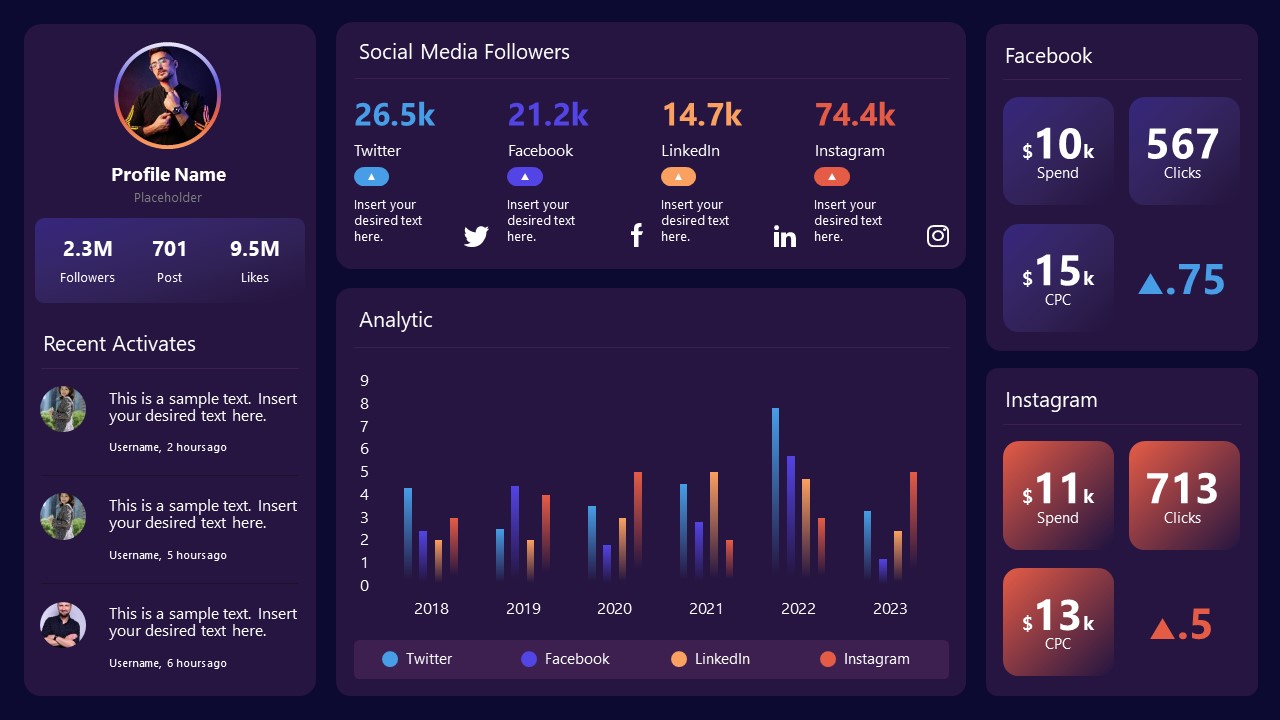
Intended for marketing professionals, this dashboard template for data presentation is a tool for presenting data analytics from social media channels. Two slide layouts featuring line graphs and column charts.
10. Project Management Summary Dashboard Template
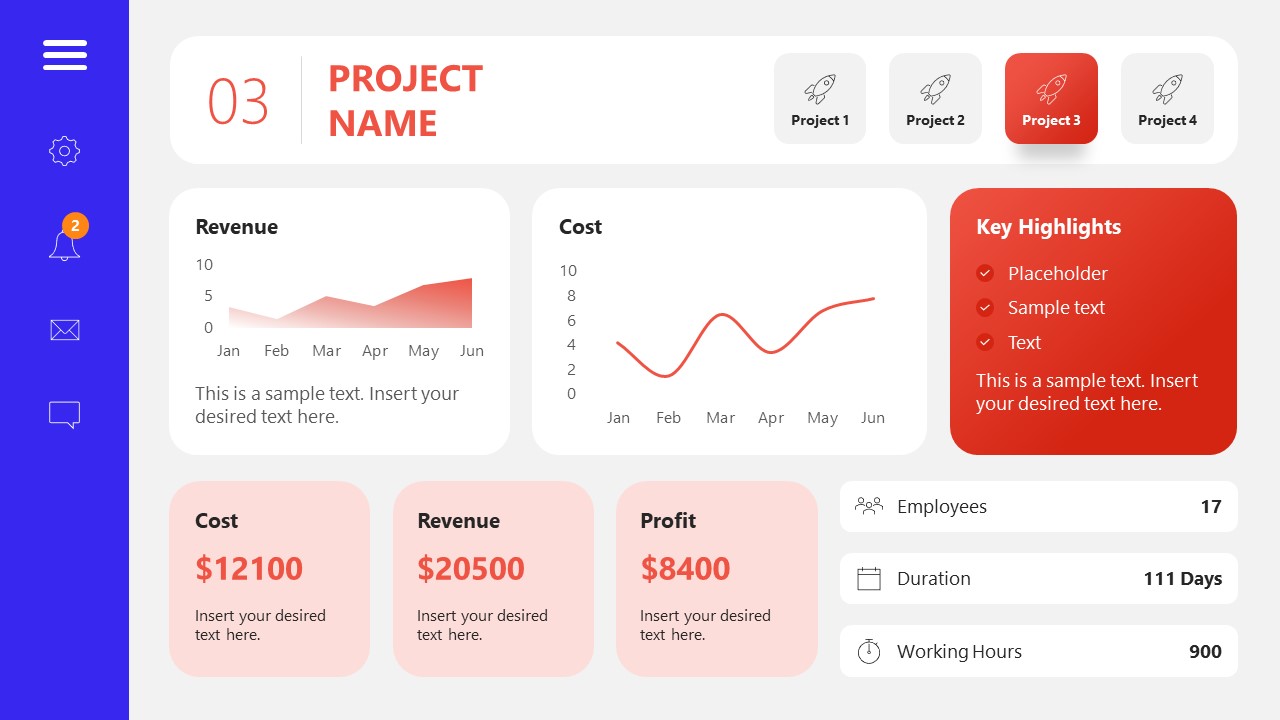
A tool crafted for project managers to deliver highly visual reports on a project’s completion, the profits it delivered for the company, and expenses/time required to execute it. 4 different color layouts are available.
11. Profit & Loss Dashboard for PowerPoint and Google Slides
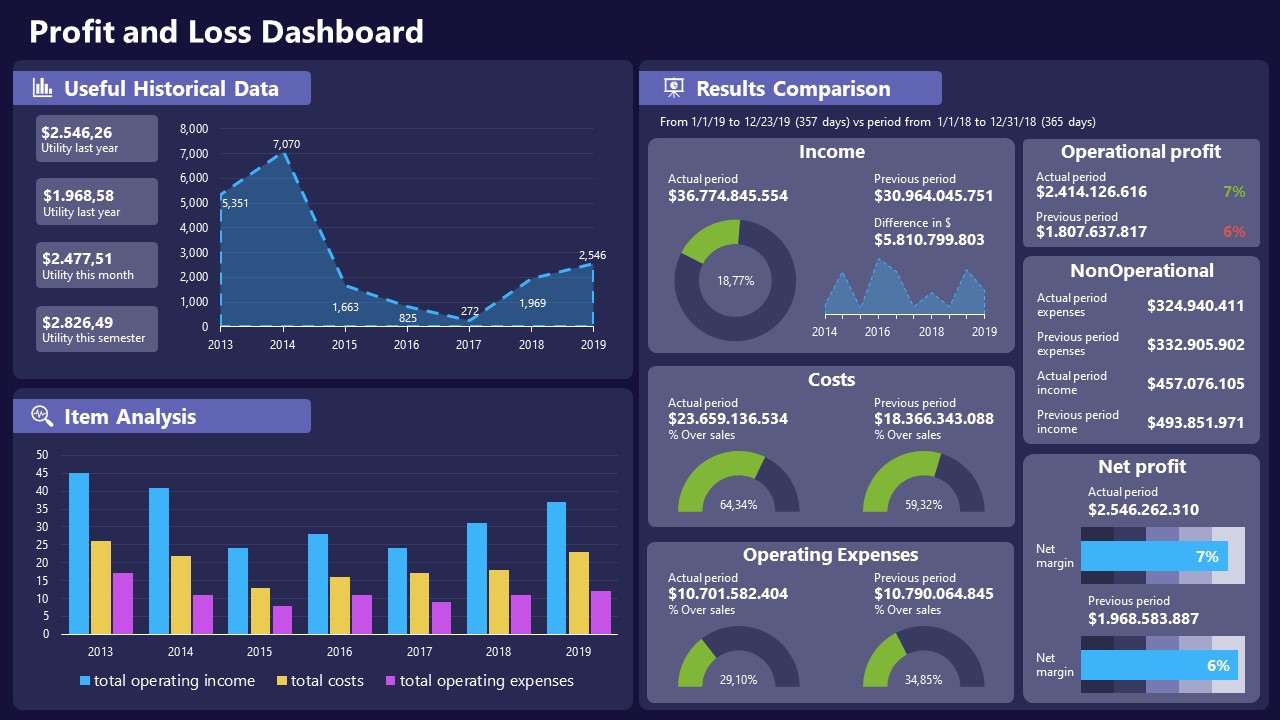
A must-have for finance professionals. This typical profit & loss dashboard includes progress bars, donut charts, column charts, line graphs, and everything that’s required to deliver a comprehensive report about a company’s financial situation.
Overwhelming visuals
One of the mistakes related to using data-presenting methods is including too much data or using overly complex visualizations. They can confuse the audience and dilute the key message.
Inappropriate chart types
Choosing the wrong type of chart for the data at hand can lead to misinterpretation. For example, using a pie chart for data that doesn’t represent parts of a whole is not right.
Lack of context
Failing to provide context or sufficient labeling can make it challenging for the audience to understand the significance of the presented data.
Inconsistency in design
Using inconsistent design elements and color schemes across different visualizations can create confusion and visual disarray.
Failure to provide details
Simply presenting raw data without offering clear insights or takeaways can leave the audience without a meaningful conclusion.
Lack of focus
Not having a clear focus on the key message or main takeaway can result in a presentation that lacks a central theme.
Visual accessibility issues
Overlooking the visual accessibility of charts and graphs can exclude certain audience members who may have difficulty interpreting visual information.
In order to avoid these mistakes in data presentation, presenters can benefit from using presentation templates . These templates provide a structured framework. They ensure consistency, clarity, and an aesthetically pleasing design, enhancing data communication’s overall impact.
Understanding and choosing data presentation types are pivotal in effective communication. Each method serves a unique purpose, so selecting the appropriate one depends on the nature of the data and the message to be conveyed. The diverse array of presentation types offers versatility in visually representing information, from bar charts showing values to pie charts illustrating proportions.
Using the proper method enhances clarity, engages the audience, and ensures that data sets are not just presented but comprehensively understood. By appreciating the strengths and limitations of different presentation types, communicators can tailor their approach to convey information accurately, developing a deeper connection between data and audience understanding.
[1] Government of Canada, S.C. (2021) 5 Data Visualization 5.2 Bar Chart , 5.2 Bar chart . https://www150.statcan.gc.ca/n1/edu/power-pouvoir/ch9/bargraph-diagrammeabarres/5214818-eng.htm
[2] Kosslyn, S.M., 1989. Understanding charts and graphs. Applied cognitive psychology, 3(3), pp.185-225. https://apps.dtic.mil/sti/pdfs/ADA183409.pdf
[3] Creating a Dashboard . https://it.tufts.edu/book/export/html/1870
[4] https://www.goldenwestcollege.edu/research/data-and-more/data-dashboards/index.html
[5] https://www.mit.edu/course/21/21.guide/grf-line.htm
[6] Jadeja, M. and Shah, K., 2015, January. Tree-Map: A Visualization Tool for Large Data. In GSB@ SIGIR (pp. 9-13). https://ceur-ws.org/Vol-1393/gsb15proceedings.pdf#page=15
[7] Heat Maps and Quilt Plots. https://www.publichealth.columbia.edu/research/population-health-methods/heat-maps-and-quilt-plots
[8] EIU QGIS WORKSHOP. https://www.eiu.edu/qgisworkshop/heatmaps.php
[9] About Pie Charts. https://www.mit.edu/~mbarker/formula1/f1help/11-ch-c8.htm
[10] Histograms. https://sites.utexas.edu/sos/guided/descriptive/numericaldd/descriptiven2/histogram/ [11] https://asq.org/quality-resources/scatter-diagram
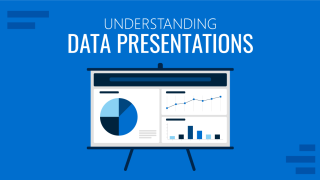
Like this article? Please share
Data Analysis, Data Science, Data Visualization Filed under Design
Related Articles
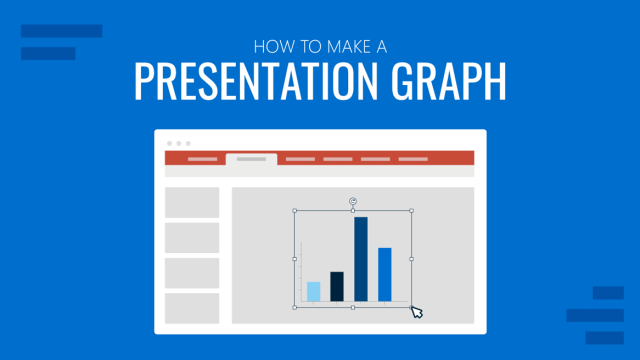
Filed under Design • March 27th, 2024
How to Make a Presentation Graph
Detailed step-by-step instructions to master the art of how to make a presentation graph in PowerPoint and Google Slides. Check it out!
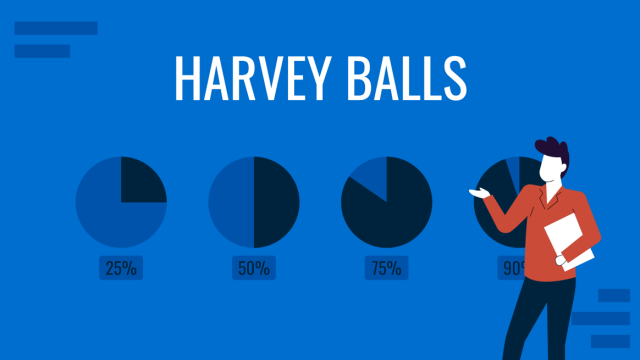
Filed under Presentation Ideas • January 6th, 2024
All About Using Harvey Balls
Among the many tools in the arsenal of the modern presenter, Harvey Balls have a special place. In this article we will tell you all about using Harvey Balls.
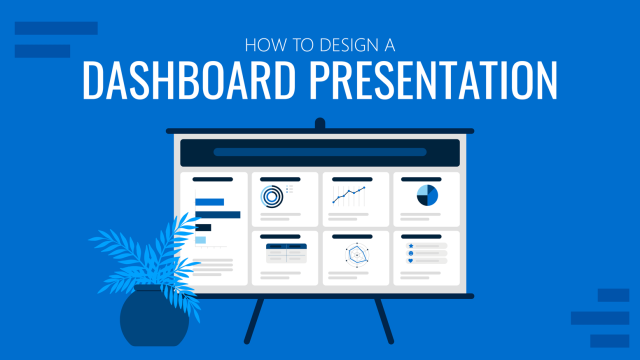
Filed under Business • December 8th, 2023
How to Design a Dashboard Presentation: A Step-by-Step Guide
Take a step further in your professional presentation skills by learning what a dashboard presentation is and how to properly design one in PowerPoint. A detailed step-by-step guide is here!
Leave a Reply
- 13 min read
What is Data Interpretation? Methods, Examples & Tools

What is Data Interpretation?
- Importance of Data Interpretation in Today's World
Types of Data Interpretation
Quantitative data interpretation, qualitative data interpretation, mixed methods data interpretation, methods of data interpretation, descriptive statistics, inferential statistics, visualization techniques, benefits of data interpretation, data interpretation process, data interpretation use cases, data interpretation tools, data interpretation challenges and solutions, overcoming bias in data, dealing with missing data, addressing data privacy concerns, data interpretation examples, sales trend analysis, customer segmentation, predictive maintenance, fraud detection, data interpretation best practices, maintaining data quality, choosing the right tools, effective communication of results, ongoing learning and development, data interpretation tips.
Data interpretation is the process of making sense of data and turning it into actionable insights. With the rise of big data and advanced technologies, it has become more important than ever to be able to effectively interpret and understand data.
In today's fast-paced business environment, companies rely on data to make informed decisions and drive growth. However, with the sheer volume of data available, it can be challenging to know where to start and how to make the most of it.
This guide provides a comprehensive overview of data interpretation, covering everything from the basics of what it is to the benefits and best practices.
Data interpretation refers to the process of taking raw data and transforming it into useful information. This involves analyzing the data to identify patterns, trends, and relationships, and then presenting the results in a meaningful way. Data interpretation is an essential part of data analysis, and it is used in a wide range of fields, including business, marketing, healthcare, and many more.
Importance of Data Interpretation in Today's World
Data interpretation is critical to making informed decisions and driving growth in today's data-driven world. With the increasing availability of data, companies can now gain valuable insights into their operations, customer behavior, and market trends. Data interpretation allows businesses to make informed decisions, identify new opportunities, and improve overall efficiency.
There are three main types of data interpretation: quantitative, qualitative, and mixed methods.
Quantitative data interpretation refers to the process of analyzing numerical data. This type of data is often used to measure and quantify specific characteristics, such as sales figures, customer satisfaction ratings, and employee productivity.
Qualitative data interpretation refers to the process of analyzing non-numerical data, such as text, images, and audio. This data type is often used to gain a deeper understanding of customer attitudes and opinions and to identify patterns and trends.
Mixed methods data interpretation combines both quantitative and qualitative data to provide a more comprehensive understanding of a particular subject. This approach is particularly useful when analyzing data that has both numerical and non-numerical components, such as customer feedback data.
There are several data interpretation methods, including descriptive statistics, inferential statistics, and visualization techniques.
Descriptive statistics involve summarizing and presenting data in a way that makes it easy to understand. This can include calculating measures such as mean, median, mode, and standard deviation.
Inferential statistics involves making inferences and predictions about a population based on a sample of data. This type of data interpretation involves the use of statistical models and algorithms to identify patterns and relationships in the data.
Visualization techniques involve creating visual representations of data, such as graphs, charts, and maps. These techniques are particularly useful for communicating complex data in an easy-to-understand manner and identifying data patterns and trends.
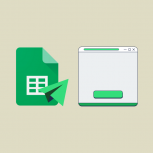
When sharing a Google Sheets spreadsheet Google usually tries to share the entire document. Here’s how to share only one tab instead.
Data interpretation plays a crucial role in decision-making and helps organizations make informed choices. There are numerous benefits of data interpretation, including:
- Improved decision-making: Data interpretation provides organizations with the information they need to make informed decisions. By analyzing data, organizations can identify trends, patterns, and relationships that they may not have been able to see otherwise.
- Increased efficiency: By automating the data interpretation process, organizations can save time and improve their overall efficiency. With the right tools and methods, data interpretation can be completed quickly and accurately, providing organizations with the information they need to make decisions more efficiently.
- Better collaboration: Data interpretation can help organizations work more effectively with others, such as stakeholders, partners, and clients. By providing a common understanding of the data and its implications, organizations can collaborate more effectively and make better decisions.
- Increased accuracy: Data interpretation helps to ensure that data is accurate and consistent, reducing the risk of errors and miscommunication. By using data interpretation techniques, organizations can identify errors and inconsistencies in their data, making it possible to correct them and ensure the accuracy of their information.
- Enhanced transparency: Data interpretation can also increase transparency, helping organizations demonstrate their commitment to ethical and responsible data management. By providing clear and concise information, organizations can build trust and credibility with their stakeholders.
- Better resource allocation: Data interpretation can help organizations make better decisions about resource allocation. By analyzing data, organizations can identify areas where they are spending too much time or money and make adjustments to optimize their resources.
- Improved planning and forecasting: Data interpretation can also help organizations plan for the future. By analyzing historical data, organizations can identify trends and patterns that inform their forecasting and planning efforts.
Data interpretation is a process that involves several steps, including:
- Data collection: The first step in data interpretation is to collect data from various sources, such as surveys, databases, and websites. This data should be relevant to the issue or problem the organization is trying to solve.
- Data preparation: Once data is collected, it needs to be prepared for analysis. This may involve cleaning the data to remove errors, missing values, or outliers. It may also include transforming the data into a more suitable format for analysis.
- Data analysis: The next step is to analyze the data using various techniques, such as statistical analysis, visualization, and modeling. This analysis should be focused on uncovering trends, patterns, and relationships in the data.
- Data interpretation: Once the data has been analyzed, it needs to be interpreted to determine what the results mean. This may involve identifying key insights, drawing conclusions, and making recommendations.
- Data communication: The final step in the data interpretation process is to communicate the results and insights to others. This may involve creating visualizations, reports, or presentations to share the results with stakeholders.
Data interpretation can be applied in a variety of settings and industries. Here are a few examples of how data interpretation can be used:
- Marketing: Marketers use data interpretation to analyze customer behavior, preferences, and trends to inform marketing strategies and campaigns.
- Healthcare: Healthcare professionals use data interpretation to analyze patient data, including medical histories and test results, to diagnose and treat illnesses.
- Financial Services: Financial services companies use data interpretation to analyze financial data, such as investment performance, to inform investment decisions and strategies.
- Retail: Retail companies use data interpretation to analyze sales data, customer behavior, and market trends to inform merchandising and pricing strategies.
- Manufacturing: Manufacturers use data interpretation to analyze production data, such as machine performance and inventory levels, to inform production and inventory management decisions.
These are just a few examples of how data interpretation can be applied in various settings. The possibilities are endless, and data interpretation can provide valuable insights in any industry where data is collected and analyzed.
Data interpretation is a crucial step in the data analysis process, and the right tools can make a significant difference in accuracy and efficiency. Here are a few tools that can help you with data interpretation:
- Share parts of your spreadsheet, including sheets or even cell ranges, with different collaborators or stakeholders.
- Review and approve edits by collaborators to their respective sheets before merging them back with your master spreadsheet.
- Integrate popular tools and connect your tech stack to sync data from different sources, giving you a timely, holistic view of your data.
- Google Sheets: Google Sheets is a free, web-based spreadsheet application that allows users to create, edit, and format spreadsheets. It provides a range of features for data interpretation, including functions, charts, and pivot tables.
- Microsoft Excel: Microsoft Excel is a spreadsheet software widely used for data interpretation. It provides various functions and features to help you analyze and interpret data, including sorting, filtering, pivot tables, and charts.
- Tableau: Tableau is a data visualization tool that helps you see and understand your data. It allows you to connect to various data sources and create interactive dashboards and visualizations to communicate insights.
- Power BI: Power BI is a business analytics service that provides interactive visualizations and business intelligence capabilities with an easy interface for end users to create their own reports and dashboards.
- R: R is a programming language and software environment for statistical computing and graphics. It is widely used by statisticians, data scientists, and researchers to analyze and interpret data.
Each of these tools has its strengths and weaknesses, and the right tool for you will depend on your specific needs and requirements. Consider the size and complexity of your data, the analysis methods you need to use, and the level of customization you require, before making a decision.

If you work with important data in Google Sheets, you probably want an extra layer of protection. Here's how you can password protect a Google Sheet
Data interpretation can be a complex and challenging process, but there are several solutions that can help overcome some of the most common difficulties.
Data interpretation can often be biased based on the data sources and the people who interpret it. It is important to eliminate these biases to get a clear and accurate understanding of the data. This can be achieved by diversifying the data sources, involving multiple stakeholders in the data interpretation process, and regularly reviewing the data interpretation methodology.
Missing data can often result in inaccuracies in the data interpretation process. To overcome this challenge, data scientists can use imputation methods to fill in missing data or use statistical models that can account for missing data.
Data privacy is a crucial concern in today's data-driven world. To address this, organizations should ensure that their data interpretation processes align with data privacy regulations and that the data being analyzed is adequately secured.
Data interpretation is used in a variety of industries and for a range of purposes. Here are a few examples:
Sales trend analysis is a common use of data interpretation in the business world. This type of analysis involves looking at sales data over time to identify trends and patterns, which can then be used to make informed business decisions.
Customer segmentation is a data interpretation technique that categorizes customers into segments based on common characteristics. This can be used to create more targeted marketing campaigns and to improve customer engagement.
Predictive maintenance is a data interpretation technique that uses machine learning algorithms to predict when equipment is likely to fail. This can help organizations proactively address potential issues and reduce downtime.
Fraud detection is a use case for data interpretation involving data and machine learning algorithms to identify patterns and anomalies that may indicate fraudulent activity.
To ensure that data interpretation processes are as effective and accurate as possible, it is recommended to follow some best practices.
Data quality is critical to the accuracy of data interpretation. To maintain data quality, organizations should regularly review and validate their data, eliminate data biases, and address missing data.
Choosing the right data interpretation tools is crucial to the success of the data interpretation process. Organizations should consider factors such as cost, compatibility with existing tools and processes, and the complexity of the data to be analyzed when choosing the right data interpretation tool. Layer, an add-on that equips teams with the tools to increase efficiency and data quality in their processes on top of Google Sheets, is an excellent choice for organizations looking to optimize their data interpretation process.
Data interpretation results need to be communicated effectively to stakeholders in a way they can understand. This can be achieved by using visual aids such as charts and graphs and presenting the results clearly and concisely.
The world of data interpretation is constantly evolving, and organizations must stay up to date with the latest developments and best practices. Ongoing learning and development initiatives, such as attending workshops and conferences, can help organizations stay ahead of the curve.
Regardless of the data interpretation method used, following best practices can help ensure accurate and reliable results. These best practices include:
- Validate data sources: It is essential to validate the data sources used to ensure they are accurate, up-to-date, and relevant. This helps to minimize the potential for errors in the data interpretation process.
- Use appropriate statistical techniques: The choice of statistical methods used for data interpretation should be suitable for the type of data being analyzed. For example, regression analysis is often used for analyzing trends in large data sets, while chi-square tests are used for categorical data.
- Graph and visualize data: Graphical representations of data can help to quickly identify patterns and trends. Visualization tools like histograms, scatter plots, and bar graphs can make the data more understandable and easier to interpret.
- Document and explain results: Results from data interpretation should be documented and presented in a clear and concise manner. This includes providing context for the results and explaining how they were obtained.
- Use a robust data interpretation tool: Data interpretation tools can help to automate the process and minimize the risk of errors. However, choosing a reliable, user-friendly tool that provides the features and functionalities needed to support the data interpretation process is vital.
Data interpretation is a crucial aspect of data analysis and enables organizations to turn large amounts of data into actionable insights. The guide covered the definition, importance, types, methods, benefits, process, analysis, tools, use cases, and best practices of data interpretation.
As technology continues to advance, the methods and tools used in data interpretation will also evolve. Predictive analytics and artificial intelligence will play an increasingly important role in data interpretation as organizations strive to automate and streamline their data analysis processes. In addition, big data and the Internet of Things (IoT) will lead to the generation of vast amounts of data that will need to be analyzed and interpreted effectively.
Data interpretation is a critical skill that enables organizations to make informed decisions based on data. It is essential that organizations invest in data interpretation and the development of their in-house data interpretation skills, whether through training programs or the use of specialized tools like Layer. By staying up-to-date with the latest trends and best practices in data interpretation, organizations can maximize the value of their data and drive growth and success.
Hady has a passion for tech, marketing, and spreadsheets. Besides his Computer Science degree, he has vast experience in developing, launching, and scaling content marketing processes at SaaS startups.
Layer is now Sheetgo
Automate your procesess on top of spreadsheets.

- SUGGESTED TOPICS
- The Magazine
- Newsletters
- Managing Yourself
- Managing Teams
- Work-life Balance
- The Big Idea
- Data & Visuals
- Reading Lists
- Case Selections
- HBR Learning
- Topic Feeds
- Account Settings
- Email Preferences
Present Your Data Like a Pro
- Joel Schwartzberg

Demystify the numbers. Your audience will thank you.
While a good presentation has data, data alone doesn’t guarantee a good presentation. It’s all about how that data is presented. The quickest way to confuse your audience is by sharing too many details at once. The only data points you should share are those that significantly support your point — and ideally, one point per chart. To avoid the debacle of sheepishly translating hard-to-see numbers and labels, rehearse your presentation with colleagues sitting as far away as the actual audience would. While you’ve been working with the same chart for weeks or months, your audience will be exposed to it for mere seconds. Give them the best chance of comprehending your data by using simple, clear, and complete language to identify X and Y axes, pie pieces, bars, and other diagrammatic elements. Try to avoid abbreviations that aren’t obvious, and don’t assume labeled components on one slide will be remembered on subsequent slides. Every valuable chart or pie graph has an “Aha!” zone — a number or range of data that reveals something crucial to your point. Make sure you visually highlight the “Aha!” zone, reinforcing the moment by explaining it to your audience.
With so many ways to spin and distort information these days, a presentation needs to do more than simply share great ideas — it needs to support those ideas with credible data. That’s true whether you’re an executive pitching new business clients, a vendor selling her services, or a CEO making a case for change.

- JS Joel Schwartzberg oversees executive communications for a major national nonprofit, is a professional presentation coach, and is the author of Get to the Point! Sharpen Your Message and Make Your Words Matter and The Language of Leadership: How to Engage and Inspire Your Team . You can find him on LinkedIn and X. TheJoelTruth
Partner Center
A Step-by-Step Guide to the Data Analysis Process
Like any scientific discipline, data analysis follows a rigorous step-by-step process. Each stage requires different skills and know-how. To get meaningful insights, though, it’s important to understand the process as a whole. An underlying framework is invaluable for producing results that stand up to scrutiny.
In this post, we’ll explore the main steps in the data analysis process. This will cover how to define your goal, collect data, and carry out an analysis. Where applicable, we’ll also use examples and highlight a few tools to make the journey easier. When you’re done, you’ll have a much better understanding of the basics. This will help you tweak the process to fit your own needs.
Here are the steps we’ll take you through:
- Defining the question
- Collecting the data
- Cleaning the data
- Analyzing the data
- Sharing your results
- Embracing failure
On popular request, we’ve also developed a video based on this article. Scroll further along this article to watch that.
Ready? Let’s get started with step one.
1. Step one: Defining the question
The first step in any data analysis process is to define your objective. In data analytics jargon, this is sometimes called the ‘problem statement’.
Defining your objective means coming up with a hypothesis and figuring how to test it. Start by asking: What business problem am I trying to solve? While this might sound straightforward, it can be trickier than it seems. For instance, your organization’s senior management might pose an issue, such as: “Why are we losing customers?” It’s possible, though, that this doesn’t get to the core of the problem. A data analyst’s job is to understand the business and its goals in enough depth that they can frame the problem the right way.
Let’s say you work for a fictional company called TopNotch Learning. TopNotch creates custom training software for its clients. While it is excellent at securing new clients, it has much lower repeat business. As such, your question might not be, “Why are we losing customers?” but, “Which factors are negatively impacting the customer experience?” or better yet: “How can we boost customer retention while minimizing costs?”
Now you’ve defined a problem, you need to determine which sources of data will best help you solve it. This is where your business acumen comes in again. For instance, perhaps you’ve noticed that the sales process for new clients is very slick, but that the production team is inefficient. Knowing this, you could hypothesize that the sales process wins lots of new clients, but the subsequent customer experience is lacking. Could this be why customers don’t come back? Which sources of data will help you answer this question?
Tools to help define your objective
Defining your objective is mostly about soft skills, business knowledge, and lateral thinking. But you’ll also need to keep track of business metrics and key performance indicators (KPIs). Monthly reports can allow you to track problem points in the business. Some KPI dashboards come with a fee, like Databox and DashThis . However, you’ll also find open-source software like Grafana , Freeboard , and Dashbuilder . These are great for producing simple dashboards, both at the beginning and the end of the data analysis process.
2. Step two: Collecting the data
Once you’ve established your objective, you’ll need to create a strategy for collecting and aggregating the appropriate data. A key part of this is determining which data you need. This might be quantitative (numeric) data, e.g. sales figures, or qualitative (descriptive) data, such as customer reviews. All data fit into one of three categories: first-party, second-party, and third-party data. Let’s explore each one.
What is first-party data?
First-party data are data that you, or your company, have directly collected from customers. It might come in the form of transactional tracking data or information from your company’s customer relationship management (CRM) system. Whatever its source, first-party data is usually structured and organized in a clear, defined way. Other sources of first-party data might include customer satisfaction surveys, focus groups, interviews, or direct observation.
What is second-party data?
To enrich your analysis, you might want to secure a secondary data source. Second-party data is the first-party data of other organizations. This might be available directly from the company or through a private marketplace. The main benefit of second-party data is that they are usually structured, and although they will be less relevant than first-party data, they also tend to be quite reliable. Examples of second-party data include website, app or social media activity, like online purchase histories, or shipping data.
What is third-party data?
Third-party data is data that has been collected and aggregated from numerous sources by a third-party organization. Often (though not always) third-party data contains a vast amount of unstructured data points (big data). Many organizations collect big data to create industry reports or to conduct market research. The research and advisory firm Gartner is a good real-world example of an organization that collects big data and sells it on to other companies. Open data repositories and government portals are also sources of third-party data .
Tools to help you collect data
Once you’ve devised a data strategy (i.e. you’ve identified which data you need, and how best to go about collecting them) there are many tools you can use to help you. One thing you’ll need, regardless of industry or area of expertise, is a data management platform (DMP). A DMP is a piece of software that allows you to identify and aggregate data from numerous sources, before manipulating them, segmenting them, and so on. There are many DMPs available. Some well-known enterprise DMPs include Salesforce DMP , SAS , and the data integration platform, Xplenty . If you want to play around, you can also try some open-source platforms like Pimcore or D:Swarm .
Want to learn more about what data analytics is and the process a data analyst follows? We cover this topic (and more) in our free introductory short course for beginners. Check out tutorial one: An introduction to data analytics .
3. Step three: Cleaning the data
Once you’ve collected your data, the next step is to get it ready for analysis. This means cleaning, or ‘scrubbing’ it, and is crucial in making sure that you’re working with high-quality data . Key data cleaning tasks include:
- Removing major errors, duplicates, and outliers —all of which are inevitable problems when aggregating data from numerous sources.
- Removing unwanted data points —extracting irrelevant observations that have no bearing on your intended analysis.
- Bringing structure to your data —general ‘housekeeping’, i.e. fixing typos or layout issues, which will help you map and manipulate your data more easily.
- Filling in major gaps —as you’re tidying up, you might notice that important data are missing. Once you’ve identified gaps, you can go about filling them.
A good data analyst will spend around 70-90% of their time cleaning their data. This might sound excessive. But focusing on the wrong data points (or analyzing erroneous data) will severely impact your results. It might even send you back to square one…so don’t rush it! You’ll find a step-by-step guide to data cleaning here . You may be interested in this introductory tutorial to data cleaning, hosted by Dr. Humera Noor Minhas.
Carrying out an exploratory analysis
Another thing many data analysts do (alongside cleaning data) is to carry out an exploratory analysis. This helps identify initial trends and characteristics, and can even refine your hypothesis. Let’s use our fictional learning company as an example again. Carrying out an exploratory analysis, perhaps you notice a correlation between how much TopNotch Learning’s clients pay and how quickly they move on to new suppliers. This might suggest that a low-quality customer experience (the assumption in your initial hypothesis) is actually less of an issue than cost. You might, therefore, take this into account.
Tools to help you clean your data
Cleaning datasets manually—especially large ones—can be daunting. Luckily, there are many tools available to streamline the process. Open-source tools, such as OpenRefine , are excellent for basic data cleaning, as well as high-level exploration. However, free tools offer limited functionality for very large datasets. Python libraries (e.g. Pandas) and some R packages are better suited for heavy data scrubbing. You will, of course, need to be familiar with the languages. Alternatively, enterprise tools are also available. For example, Data Ladder , which is one of the highest-rated data-matching tools in the industry. There are many more. Why not see which free data cleaning tools you can find to play around with?
4. Step four: Analyzing the data
Finally, you’ve cleaned your data. Now comes the fun bit—analyzing it! The type of data analysis you carry out largely depends on what your goal is. But there are many techniques available. Univariate or bivariate analysis, time-series analysis, and regression analysis are just a few you might have heard of. More important than the different types, though, is how you apply them. This depends on what insights you’re hoping to gain. Broadly speaking, all types of data analysis fit into one of the following four categories.
Descriptive analysis
Descriptive analysis identifies what has already happened . It is a common first step that companies carry out before proceeding with deeper explorations. As an example, let’s refer back to our fictional learning provider once more. TopNotch Learning might use descriptive analytics to analyze course completion rates for their customers. Or they might identify how many users access their products during a particular period. Perhaps they’ll use it to measure sales figures over the last five years. While the company might not draw firm conclusions from any of these insights, summarizing and describing the data will help them to determine how to proceed.
Learn more: What is descriptive analytics?
Diagnostic analysis
Diagnostic analytics focuses on understanding why something has happened . It is literally the diagnosis of a problem, just as a doctor uses a patient’s symptoms to diagnose a disease. Remember TopNotch Learning’s business problem? ‘Which factors are negatively impacting the customer experience?’ A diagnostic analysis would help answer this. For instance, it could help the company draw correlations between the issue (struggling to gain repeat business) and factors that might be causing it (e.g. project costs, speed of delivery, customer sector, etc.) Let’s imagine that, using diagnostic analytics, TopNotch realizes its clients in the retail sector are departing at a faster rate than other clients. This might suggest that they’re losing customers because they lack expertise in this sector. And that’s a useful insight!
Predictive analysis
Predictive analysis allows you to identify future trends based on historical data . In business, predictive analysis is commonly used to forecast future growth, for example. But it doesn’t stop there. Predictive analysis has grown increasingly sophisticated in recent years. The speedy evolution of machine learning allows organizations to make surprisingly accurate forecasts. Take the insurance industry. Insurance providers commonly use past data to predict which customer groups are more likely to get into accidents. As a result, they’ll hike up customer insurance premiums for those groups. Likewise, the retail industry often uses transaction data to predict where future trends lie, or to determine seasonal buying habits to inform their strategies. These are just a few simple examples, but the untapped potential of predictive analysis is pretty compelling.
Prescriptive analysis
Prescriptive analysis allows you to make recommendations for the future. This is the final step in the analytics part of the process. It’s also the most complex. This is because it incorporates aspects of all the other analyses we’ve described. A great example of prescriptive analytics is the algorithms that guide Google’s self-driving cars. Every second, these algorithms make countless decisions based on past and present data, ensuring a smooth, safe ride. Prescriptive analytics also helps companies decide on new products or areas of business to invest in.
Learn more: What are the different types of data analysis?
5. Step five: Sharing your results
You’ve finished carrying out your analyses. You have your insights. The final step of the data analytics process is to share these insights with the wider world (or at least with your organization’s stakeholders!) This is more complex than simply sharing the raw results of your work—it involves interpreting the outcomes, and presenting them in a manner that’s digestible for all types of audiences. Since you’ll often present information to decision-makers, it’s very important that the insights you present are 100% clear and unambiguous. For this reason, data analysts commonly use reports, dashboards, and interactive visualizations to support their findings.
How you interpret and present results will often influence the direction of a business. Depending on what you share, your organization might decide to restructure, to launch a high-risk product, or even to close an entire division. That’s why it’s very important to provide all the evidence that you’ve gathered, and not to cherry-pick data. Ensuring that you cover everything in a clear, concise way will prove that your conclusions are scientifically sound and based on the facts. On the flip side, it’s important to highlight any gaps in the data or to flag any insights that might be open to interpretation. Honest communication is the most important part of the process. It will help the business, while also helping you to excel at your job!
Tools for interpreting and sharing your findings
There are tons of data visualization tools available, suited to different experience levels. Popular tools requiring little or no coding skills include Google Charts , Tableau , Datawrapper , and Infogram . If you’re familiar with Python and R, there are also many data visualization libraries and packages available. For instance, check out the Python libraries Plotly , Seaborn , and Matplotlib . Whichever data visualization tools you use, make sure you polish up your presentation skills, too. Remember: Visualization is great, but communication is key!
You can learn more about storytelling with data in this free, hands-on tutorial . We show you how to craft a compelling narrative for a real dataset, resulting in a presentation to share with key stakeholders. This is an excellent insight into what it’s really like to work as a data analyst!
6. Step six: Embrace your failures
The last ‘step’ in the data analytics process is to embrace your failures. The path we’ve described above is more of an iterative process than a one-way street. Data analytics is inherently messy, and the process you follow will be different for every project. For instance, while cleaning data, you might spot patterns that spark a whole new set of questions. This could send you back to step one (to redefine your objective). Equally, an exploratory analysis might highlight a set of data points you’d never considered using before. Or maybe you find that the results of your core analyses are misleading or erroneous. This might be caused by mistakes in the data, or human error earlier in the process.
While these pitfalls can feel like failures, don’t be disheartened if they happen. Data analysis is inherently chaotic, and mistakes occur. What’s important is to hone your ability to spot and rectify errors. If data analytics was straightforward, it might be easier, but it certainly wouldn’t be as interesting. Use the steps we’ve outlined as a framework, stay open-minded, and be creative. If you lose your way, you can refer back to the process to keep yourself on track.
In this post, we’ve covered the main steps of the data analytics process. These core steps can be amended, re-ordered and re-used as you deem fit, but they underpin every data analyst’s work:
- Define the question —What business problem are you trying to solve? Frame it as a question to help you focus on finding a clear answer.
- Collect data —Create a strategy for collecting data. Which data sources are most likely to help you solve your business problem?
- Clean the data —Explore, scrub, tidy, de-dupe, and structure your data as needed. Do whatever you have to! But don’t rush…take your time!
- Analyze the data —Carry out various analyses to obtain insights. Focus on the four types of data analysis: descriptive, diagnostic, predictive, and prescriptive.
- Share your results —How best can you share your insights and recommendations? A combination of visualization tools and communication is key.
- Embrace your mistakes —Mistakes happen. Learn from them. This is what transforms a good data analyst into a great one.
What next? From here, we strongly encourage you to explore the topic on your own. Get creative with the steps in the data analysis process, and see what tools you can find. As long as you stick to the core principles we’ve described, you can create a tailored technique that works for you.
To learn more, check out our free, 5-day data analytics short course . You might also be interested in the following:
- These are the top 9 data analytics tools
- 10 great places to find free datasets for your next project
- How to build a data analytics portfolio
A Guide To The Methods, Benefits & Problems of The Interpretation of Data

Table of Contents
1) What Is Data Interpretation?
2) How To Interpret Data?
3) Why Data Interpretation Is Important?
4) Data Interpretation Skills
5) Data Analysis & Interpretation Problems
6) Data Interpretation Techniques & Methods
7) The Use of Dashboards For Data Interpretation
8) Business Data Interpretation Examples
Data analysis and interpretation have now taken center stage with the advent of the digital age… and the sheer amount of data can be frightening. In fact, a Digital Universe study found that the total data supply in 2012 was 2.8 trillion gigabytes! Based on that amount of data alone, it is clear the calling card of any successful enterprise in today’s global world will be the ability to analyze complex data, produce actionable insights, and adapt to new market needs… all at the speed of thought.
Business dashboards are the digital age tools for big data. Capable of displaying key performance indicators (KPIs) for both quantitative and qualitative data analyses, they are ideal for making the fast-paced and data-driven market decisions that push today’s industry leaders to sustainable success. Through the art of streamlined visual communication, data dashboards permit businesses to engage in real-time and informed decision-making and are key instruments in data interpretation. First of all, let’s find a definition to understand what lies behind this practice.
What Is Data Interpretation?
Data interpretation refers to the process of using diverse analytical methods to review data and arrive at relevant conclusions. The interpretation of data helps researchers to categorize, manipulate, and summarize the information in order to answer critical questions.
The importance of data interpretation is evident, and this is why it needs to be done properly. Data is very likely to arrive from multiple sources and has a tendency to enter the analysis process with haphazard ordering. Data analysis tends to be extremely subjective. That is to say, the nature and goal of interpretation will vary from business to business, likely correlating to the type of data being analyzed. While there are several types of processes that are implemented based on the nature of individual data, the two broadest and most common categories are “quantitative and qualitative analysis.”
Yet, before any serious data interpretation inquiry can begin, it should be understood that visual presentations of data findings are irrelevant unless a sound decision is made regarding measurement scales. Before any serious data analysis can begin, the measurement scale must be decided for the data as this will have a long-term impact on data interpretation ROI. The varying scales include:
- Nominal Scale: non-numeric categories that cannot be ranked or compared quantitatively. Variables are exclusive and exhaustive.
- Ordinal Scale: exclusive categories that are exclusive and exhaustive but with a logical order. Quality ratings and agreement ratings are examples of ordinal scales (i.e., good, very good, fair, etc., OR agree, strongly agree, disagree, etc.).
- Interval: a measurement scale where data is grouped into categories with orderly and equal distances between the categories. There is always an arbitrary zero point.
- Ratio: contains features of all three.
For a more in-depth review of scales of measurement, read our article on data analysis questions . Once measurement scales have been selected, it is time to select which of the two broad interpretation processes will best suit your data needs. Let’s take a closer look at those specific methods and possible data interpretation problems.
How To Interpret Data? Top Methods & Techniques
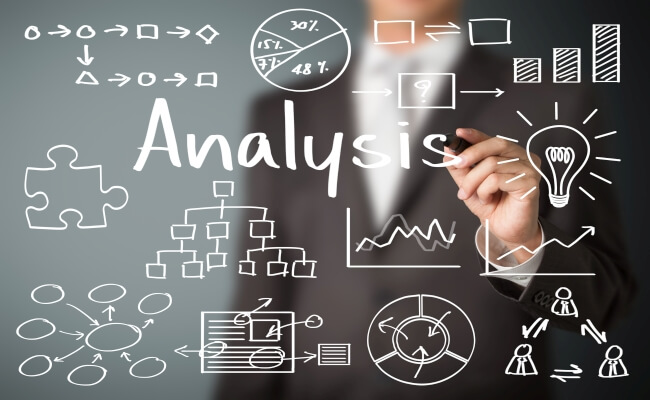
When interpreting data, an analyst must try to discern the differences between correlation, causation, and coincidences, as well as many other biases – but he also has to consider all the factors involved that may have led to a result. There are various data interpretation types and methods one can use to achieve this.
The interpretation of data is designed to help people make sense of numerical data that has been collected, analyzed, and presented. Having a baseline method for interpreting data will provide your analyst teams with a structure and consistent foundation. Indeed, if several departments have different approaches to interpreting the same data while sharing the same goals, some mismatched objectives can result. Disparate methods will lead to duplicated efforts, inconsistent solutions, wasted energy, and inevitably – time and money. In this part, we will look at the two main methods of interpretation of data: qualitative and quantitative analysis.
Qualitative Data Interpretation
Qualitative data analysis can be summed up in one word – categorical. With this type of analysis, data is not described through numerical values or patterns but through the use of descriptive context (i.e., text). Typically, narrative data is gathered by employing a wide variety of person-to-person techniques. These techniques include:
- Observations: detailing behavioral patterns that occur within an observation group. These patterns could be the amount of time spent in an activity, the type of activity, and the method of communication employed.
- Focus groups: Group people and ask them relevant questions to generate a collaborative discussion about a research topic.
- Secondary Research: much like how patterns of behavior can be observed, various types of documentation resources can be coded and divided based on the type of material they contain.
- Interviews: one of the best collection methods for narrative data. Inquiry responses can be grouped by theme, topic, or category. The interview approach allows for highly focused data segmentation.
A key difference between qualitative and quantitative analysis is clearly noticeable in the interpretation stage. The first one is widely open to interpretation and must be “coded” so as to facilitate the grouping and labeling of data into identifiable themes. As person-to-person data collection techniques can often result in disputes pertaining to proper analysis, qualitative data analysis is often summarized through three basic principles: notice things, collect things, and think about things.
After qualitative data has been collected through transcripts, questionnaires, audio and video recordings, or the researcher’s notes, it is time to interpret it. For that purpose, there are some common methods used by researchers and analysts.
- Content analysis : As its name suggests, this is a research method used to identify frequencies and recurring words, subjects, and concepts in image, video, or audio content. It transforms qualitative information into quantitative data to help discover trends and conclusions that will later support important research or business decisions. This method is often used by marketers to understand brand sentiment from the mouths of customers themselves. Through that, they can extract valuable information to improve their products and services. It is recommended to use content analytics tools for this method as manually performing it is very time-consuming and can lead to human error or subjectivity issues. Having a clear goal in mind before diving into it is another great practice for avoiding getting lost in the fog.
- Thematic analysis: This method focuses on analyzing qualitative data, such as interview transcripts, survey questions, and others, to identify common patterns and separate the data into different groups according to found similarities or themes. For example, imagine you want to analyze what customers think about your restaurant. For this purpose, you do a thematic analysis on 1000 reviews and find common themes such as “fresh food”, “cold food”, “small portions”, “friendly staff”, etc. With those recurring themes in hand, you can extract conclusions about what could be improved or enhanced based on your customer’s experiences. Since this technique is more exploratory, be open to changing your research questions or goals as you go.
- Narrative analysis: A bit more specific and complicated than the two previous methods, it is used to analyze stories and discover their meaning. These stories can be extracted from testimonials, case studies, and interviews, as these formats give people more space to tell their experiences. Given that collecting this kind of data is harder and more time-consuming, sample sizes for narrative analysis are usually smaller, which makes it harder to reproduce its findings. However, it is still a valuable technique for understanding customers' preferences and mindsets.
- Discourse analysis : This method is used to draw the meaning of any type of visual, written, or symbolic language in relation to a social, political, cultural, or historical context. It is used to understand how context can affect how language is carried out and understood. For example, if you are doing research on power dynamics, using discourse analysis to analyze a conversation between a janitor and a CEO and draw conclusions about their responses based on the context and your research questions is a great use case for this technique. That said, like all methods in this section, discourse analytics is time-consuming as the data needs to be analyzed until no new insights emerge.
- Grounded theory analysis : The grounded theory approach aims to create or discover a new theory by carefully testing and evaluating the data available. Unlike all other qualitative approaches on this list, grounded theory helps extract conclusions and hypotheses from the data instead of going into the analysis with a defined hypothesis. This method is very popular amongst researchers, analysts, and marketers as the results are completely data-backed, providing a factual explanation of any scenario. It is often used when researching a completely new topic or with little knowledge as this space to start from the ground up.
Quantitative Data Interpretation
If quantitative data interpretation could be summed up in one word (and it really can’t), that word would be “numerical.” There are few certainties when it comes to data analysis, but you can be sure that if the research you are engaging in has no numbers involved, it is not quantitative research, as this analysis refers to a set of processes by which numerical data is analyzed. More often than not, it involves the use of statistical modeling such as standard deviation, mean, and median. Let’s quickly review the most common statistical terms:
- Mean: A mean represents a numerical average for a set of responses. When dealing with a data set (or multiple data sets), a mean will represent the central value of a specific set of numbers. It is the sum of the values divided by the number of values within the data set. Other terms that can be used to describe the concept are arithmetic mean, average, and mathematical expectation.
- Standard deviation: This is another statistical term commonly used in quantitative analysis. Standard deviation reveals the distribution of the responses around the mean. It describes the degree of consistency within the responses; together with the mean, it provides insight into data sets.
- Frequency distribution: This is a measurement gauging the rate of a response appearance within a data set. When using a survey, for example, frequency distribution, it can determine the number of times a specific ordinal scale response appears (i.e., agree, strongly agree, disagree, etc.). Frequency distribution is extremely keen in determining the degree of consensus among data points.
Typically, quantitative data is measured by visually presenting correlation tests between two or more variables of significance. Different processes can be used together or separately, and comparisons can be made to ultimately arrive at a conclusion. Other signature interpretation processes of quantitative data include:
- Regression analysis: Essentially, it uses historical data to understand the relationship between a dependent variable and one or more independent variables. Knowing which variables are related and how they developed in the past allows you to anticipate possible outcomes and make better decisions going forward. For example, if you want to predict your sales for next month, you can use regression to understand what factors will affect them, such as products on sale and the launch of a new campaign, among many others.
- Cohort analysis: This method identifies groups of users who share common characteristics during a particular time period. In a business scenario, cohort analysis is commonly used to understand customer behaviors. For example, a cohort could be all users who have signed up for a free trial on a given day. An analysis would be carried out to see how these users behave, what actions they carry out, and how their behavior differs from other user groups.
- Predictive analysis: As its name suggests, the predictive method aims to predict future developments by analyzing historical and current data. Powered by technologies such as artificial intelligence and machine learning, predictive analytics practices enable businesses to identify patterns or potential issues and plan informed strategies in advance.
- Prescriptive analysis: Also powered by predictions, the prescriptive method uses techniques such as graph analysis, complex event processing, and neural networks, among others, to try to unravel the effect that future decisions will have in order to adjust them before they are actually made. This helps businesses to develop responsive, practical business strategies.
- Conjoint analysis: Typically applied to survey analysis, the conjoint approach is used to analyze how individuals value different attributes of a product or service. This helps researchers and businesses to define pricing, product features, packaging, and many other attributes. A common use is menu-based conjoint analysis, in which individuals are given a “menu” of options from which they can build their ideal concept or product. Through this, analysts can understand which attributes they would pick above others and drive conclusions.
- Cluster analysis: Last but not least, the cluster is a method used to group objects into categories. Since there is no target variable when using cluster analysis, it is a useful method to find hidden trends and patterns in the data. In a business context, clustering is used for audience segmentation to create targeted experiences. In market research, it is often used to identify age groups, geographical information, and earnings, among others.
Now that we have seen how to interpret data, let's move on and ask ourselves some questions: What are some of the benefits of data interpretation? Why do all industries engage in data research and analysis? These are basic questions, but they often don’t receive adequate attention.
Your Chance: Want to test a powerful data analysis software? Use our 14-days free trial & start extracting insights from your data!
Why Data Interpretation Is Important
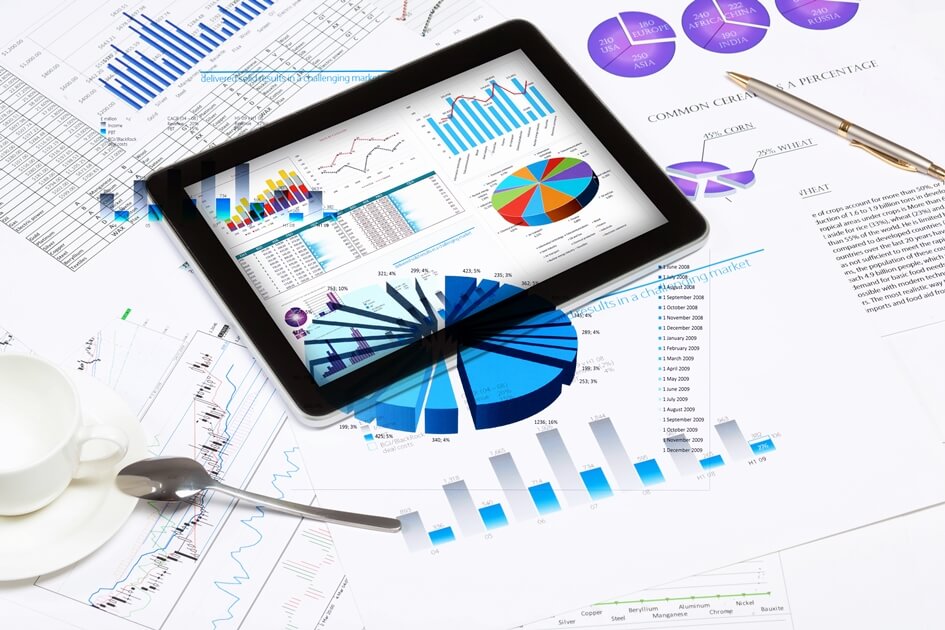
The purpose of collection and interpretation is to acquire useful and usable information and to make the most informed decisions possible. From businesses to newlyweds researching their first home, data collection and interpretation provide limitless benefits for a wide range of institutions and individuals.
Data analysis and interpretation, regardless of the method and qualitative/quantitative status, may include the following characteristics:
- Data identification and explanation
- Comparing and contrasting data
- Identification of data outliers
- Future predictions
Data analysis and interpretation, in the end, help improve processes and identify problems. It is difficult to grow and make dependable improvements without, at the very least, minimal data collection and interpretation. What is the keyword? Dependable. Vague ideas regarding performance enhancement exist within all institutions and industries. Yet, without proper research and analysis, an idea is likely to remain in a stagnant state forever (i.e., minimal growth). So… what are a few of the business benefits of digital age data analysis and interpretation? Let’s take a look!
1) Informed decision-making: A decision is only as good as the knowledge that formed it. Informed data decision-making can potentially set industry leaders apart from the rest of the market pack. Studies have shown that companies in the top third of their industries are, on average, 5% more productive and 6% more profitable when implementing informed data decision-making processes. Most decisive actions will arise only after a problem has been identified or a goal defined. Data analysis should include identification, thesis development, and data collection, followed by data communication.
If institutions only follow that simple order, one that we should all be familiar with from grade school science fairs, then they will be able to solve issues as they emerge in real-time. Informed decision-making has a tendency to be cyclical. This means there is really no end, and eventually, new questions and conditions arise within the process that need to be studied further. The monitoring of data results will inevitably return the process to the start with new data and sights.
2) Anticipating needs with trends identification: data insights provide knowledge, and knowledge is power. The insights obtained from market and consumer data analyses have the ability to set trends for peers within similar market segments. A perfect example of how data analytics can impact trend prediction is evidenced in the music identification application Shazam . The application allows users to upload an audio clip of a song they like but can’t seem to identify. Users make 15 million song identifications a day. With this data, Shazam has been instrumental in predicting future popular artists.
When industry trends are identified, they can then serve a greater industry purpose. For example, the insights from Shazam’s monitoring benefits not only Shazam in understanding how to meet consumer needs but also grant music executives and record label companies an insight into the pop-culture scene of the day. Data gathering and interpretation processes can allow for industry-wide climate prediction and result in greater revenue streams across the market. For this reason, all institutions should follow the basic data cycle of collection, interpretation, decision-making, and monitoring.
3) Cost efficiency: Proper implementation of analytics processes can provide businesses with profound cost advantages within their industries. A recent data study performed by Deloitte vividly demonstrates this in finding that data analysis ROI is driven by efficient cost reductions. Often, this benefit is overlooked because making money is typically viewed as “sexier” than saving money. Yet, sound data analyses have the ability to alert management to cost-reduction opportunities without any significant exertion of effort on the part of human capital.
A great example of the potential for cost efficiency through data analysis is Intel. Prior to 2012, Intel would conduct over 19,000 manufacturing function tests on their chips before they could be deemed acceptable for release. To cut costs and reduce test time, Intel implemented predictive data analyses. By using historical and current data, Intel now avoids testing each chip 19,000 times by focusing on specific and individual chip tests. After its implementation in 2012, Intel saved over $3 million in manufacturing costs. Cost reduction may not be as “sexy” as data profit, but as Intel proves, it is a benefit of data analysis that should not be neglected.
4) Clear foresight: companies that collect and analyze their data gain better knowledge about themselves, their processes, and their performance. They can identify performance challenges when they arise and take action to overcome them. Data interpretation through visual representations lets them process their findings faster and make better-informed decisions on the company's future.
Key Data Interpretation Skills You Should Have
Just like any other process, data interpretation and analysis require researchers or analysts to have some key skills to be able to perform successfully. It is not enough just to apply some methods and tools to the data; the person who is managing it needs to be objective and have a data-driven mind, among other skills.
It is a common misconception to think that the required skills are mostly number-related. While data interpretation is heavily analytically driven, it also requires communication and narrative skills, as the results of the analysis need to be presented in a way that is easy to understand for all types of audiences.
Luckily, with the rise of self-service tools and AI-driven technologies, data interpretation is no longer segregated for analysts only. However, the topic still remains a big challenge for businesses that make big investments in data and tools to support it, as the interpretation skills required are still lacking. It is worthless to put massive amounts of money into extracting information if you are not going to be able to interpret what that information is telling you. For that reason, below we list the top 5 data interpretation skills your employees or researchers should have to extract the maximum potential from the data.
- Data Literacy: The first and most important skill to have is data literacy. This means having the ability to understand, work, and communicate with data. It involves knowing the types of data sources, methods, and ethical implications of using them. In research, this skill is often a given. However, in a business context, there might be many employees who are not comfortable with data. The issue is the interpretation of data can not be solely responsible for the data team, as it is not sustainable in the long run. Experts advise business leaders to carefully assess the literacy level across their workforce and implement training instances to ensure everyone can interpret their data.
- Data Tools: The data interpretation and analysis process involves using various tools to collect, clean, store, and analyze the data. The complexity of the tools varies depending on the type of data and the analysis goals. Going from simple ones like Excel to more complex ones like databases, such as SQL, or programming languages, such as R or Python. It also involves visual analytics tools to bring the data to life through the use of graphs and charts. Managing these tools is a fundamental skill as they make the process faster and more efficient. As mentioned before, most modern solutions are now self-service, enabling less technical users to use them without problem.
- Critical Thinking: Another very important skill is to have critical thinking. Data hides a range of conclusions, trends, and patterns that must be discovered. It is not just about comparing numbers; it is about putting a story together based on multiple factors that will lead to a conclusion. Therefore, having the ability to look further from what is right in front of you is an invaluable skill for data interpretation.
- Data Ethics: In the information age, being aware of the legal and ethical responsibilities that come with the use of data is of utmost importance. In short, data ethics involves respecting the privacy and confidentiality of data subjects, as well as ensuring accuracy and transparency for data usage. It requires the analyzer or researcher to be completely objective with its interpretation to avoid any biases or discrimination. Many countries have already implemented regulations regarding the use of data, including the GDPR or the ACM Code Of Ethics. Awareness of these regulations and responsibilities is a fundamental skill that anyone working in data interpretation should have.
- Domain Knowledge: Another skill that is considered important when interpreting data is to have domain knowledge. As mentioned before, data hides valuable insights that need to be uncovered. To do so, the analyst needs to know about the industry or domain from which the information is coming and use that knowledge to explore it and put it into a broader context. This is especially valuable in a business context, where most departments are now analyzing data independently with the help of a live dashboard instead of relying on the IT department, which can often overlook some aspects due to a lack of expertise in the topic.
Common Data Analysis And Interpretation Problems
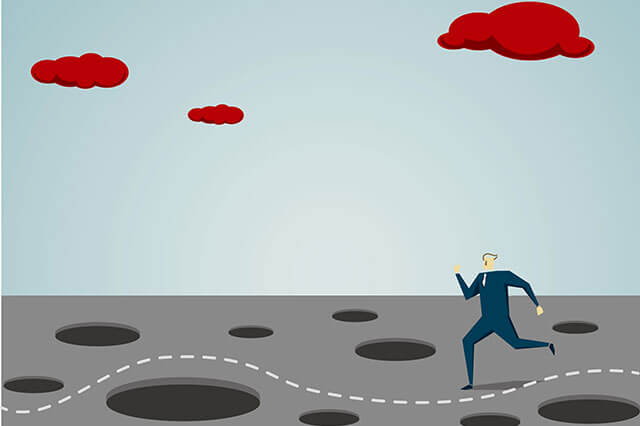
The oft-repeated mantra of those who fear data advancements in the digital age is “big data equals big trouble.” While that statement is not accurate, it is safe to say that certain data interpretation problems or “pitfalls” exist and can occur when analyzing data, especially at the speed of thought. Let’s identify some of the most common data misinterpretation risks and shed some light on how they can be avoided:
1) Correlation mistaken for causation: our first misinterpretation of data refers to the tendency of data analysts to mix the cause of a phenomenon with correlation. It is the assumption that because two actions occurred together, one caused the other. This is inaccurate, as actions can occur together, absent a cause-and-effect relationship.
- Digital age example: assuming that increased revenue results from increased social media followers… there might be a definitive correlation between the two, especially with today’s multi-channel purchasing experiences. But that does not mean an increase in followers is the direct cause of increased revenue. There could be both a common cause and an indirect causality.
- Remedy: attempt to eliminate the variable you believe to be causing the phenomenon.
2) Confirmation bias: our second problem is data interpretation bias. It occurs when you have a theory or hypothesis in mind but are intent on only discovering data patterns that support it while rejecting those that do not.
- Digital age example: your boss asks you to analyze the success of a recent multi-platform social media marketing campaign. While analyzing the potential data variables from the campaign (one that you ran and believe performed well), you see that the share rate for Facebook posts was great, while the share rate for Twitter Tweets was not. Using only Facebook posts to prove your hypothesis that the campaign was successful would be a perfect manifestation of confirmation bias.
- Remedy: as this pitfall is often based on subjective desires, one remedy would be to analyze data with a team of objective individuals. If this is not possible, another solution is to resist the urge to make a conclusion before data exploration has been completed. Remember to always try to disprove a hypothesis, not prove it.
3) Irrelevant data: the third data misinterpretation pitfall is especially important in the digital age. As large data is no longer centrally stored and as it continues to be analyzed at the speed of thought, it is inevitable that analysts will focus on data that is irrelevant to the problem they are trying to correct.
- Digital age example: in attempting to gauge the success of an email lead generation campaign, you notice that the number of homepage views directly resulting from the campaign increased, but the number of monthly newsletter subscribers did not. Based on the number of homepage views, you decide the campaign was a success when really it generated zero leads.
- Remedy: proactively and clearly frame any data analysis variables and KPIs prior to engaging in a data review. If the metric you use to measure the success of a lead generation campaign is newsletter subscribers, there is no need to review the number of homepage visits. Be sure to focus on the data variable that answers your question or solves your problem and not on irrelevant data.
4) Truncating an Axes: When creating a graph to start interpreting the results of your analysis, it is important to keep the axes truthful and avoid generating misleading visualizations. Starting the axes in a value that doesn’t portray the actual truth about the data can lead to false conclusions.
- Digital age example: In the image below, we can see a graph from Fox News in which the Y-axes start at 34%, making it seem that the difference between 35% and 39.6% is way higher than it actually is. This could lead to a misinterpretation of the tax rate changes.

* Source : www.venngage.com *
- Remedy: Be careful with how your data is visualized. Be respectful and realistic with axes to avoid misinterpretation of your data. See below how the Fox News chart looks when using the correct axis values. This chart was created with datapine's modern online data visualization tool.
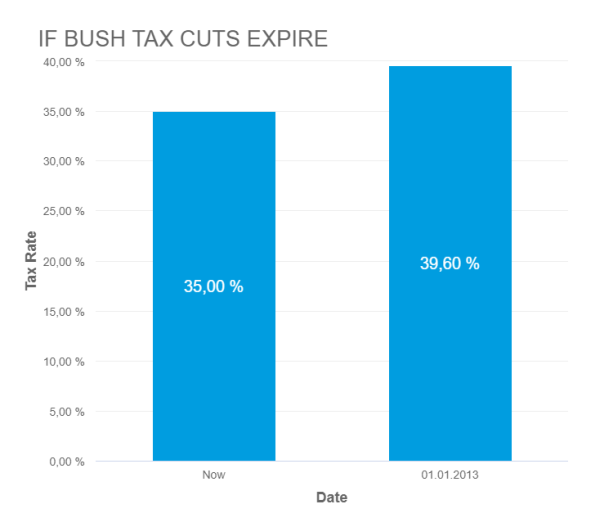
5) (Small) sample size: Another common problem is using a small sample size. Logically, the bigger the sample size, the more accurate and reliable the results. However, this also depends on the size of the effect of the study. For example, the sample size in a survey about the quality of education will not be the same as for one about people doing outdoor sports in a specific area.
- Digital age example: Imagine you ask 30 people a question, and 29 answer “yes,” resulting in 95% of the total. Now imagine you ask the same question to 1000, and 950 of them answer “yes,” which is again 95%. While these percentages might look the same, they certainly do not mean the same thing, as a 30-person sample size is not a significant number to establish a truthful conclusion.
- Remedy: Researchers say that in order to determine the correct sample size to get truthful and meaningful results, it is necessary to define a margin of error that will represent the maximum amount they want the results to deviate from the statistical mean. Paired with this, they need to define a confidence level that should be between 90 and 99%. With these two values in hand, researchers can calculate an accurate sample size for their studies.
6) Reliability, subjectivity, and generalizability : When performing qualitative analysis, researchers must consider practical and theoretical limitations when interpreting the data. In some cases, this type of research can be considered unreliable because of uncontrolled factors that might or might not affect the results. This is paired with the fact that the researcher has a primary role in the interpretation process, meaning he or she decides what is relevant and what is not, and as we know, interpretations can be very subjective.
Generalizability is also an issue that researchers face when dealing with qualitative analysis. As mentioned in the point about having a small sample size, it is difficult to draw conclusions that are 100% representative because the results might be biased or unrepresentative of a wider population.
While these factors are mostly present in qualitative research, they can also affect the quantitative analysis. For example, when choosing which KPIs to portray and how to portray them, analysts can also be biased and represent them in a way that benefits their analysis.
- Digital age example: Biased questions in a survey are a great example of reliability and subjectivity issues. Imagine you are sending a survey to your clients to see how satisfied they are with your customer service with this question: “How amazing was your experience with our customer service team?”. Here, we can see that this question clearly influences the response of the individual by putting the word “amazing” on it.
- Remedy: A solution to avoid these issues is to keep your research honest and neutral. Keep the wording of the questions as objective as possible. For example: “On a scale of 1-10, how satisfied were you with our customer service team?”. This does not lead the respondent to any specific answer, meaning the results of your survey will be reliable.
Data Interpretation Best Practices & Tips
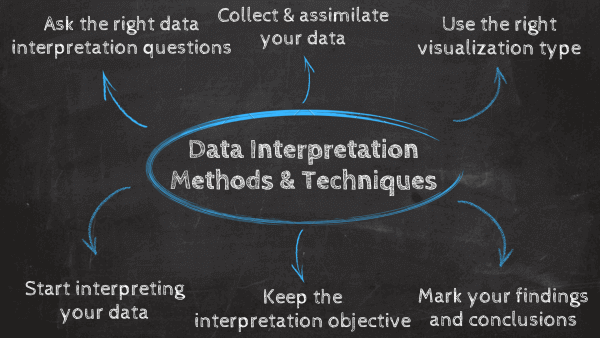
Data analysis and interpretation are critical to developing sound conclusions and making better-informed decisions. As we have seen with this article, there is an art and science to the interpretation of data. To help you with this purpose, we will list a few relevant techniques, methods, and tricks you can implement for a successful data management process.
As mentioned at the beginning of this post, the first step to interpreting data in a successful way is to identify the type of analysis you will perform and apply the methods respectively. Clearly differentiate between qualitative (observe, document, and interview notice, collect and think about things) and quantitative analysis (you lead research with a lot of numerical data to be analyzed through various statistical methods).
1) Ask the right data interpretation questions
The first data interpretation technique is to define a clear baseline for your work. This can be done by answering some critical questions that will serve as a useful guideline to start. Some of them include: what are the goals and objectives of my analysis? What type of data interpretation method will I use? Who will use this data in the future? And most importantly, what general question am I trying to answer?
Once all this information has been defined, you will be ready for the next step: collecting your data.
2) Collect and assimilate your data
Now that a clear baseline has been established, it is time to collect the information you will use. Always remember that your methods for data collection will vary depending on what type of analysis method you use, which can be qualitative or quantitative. Based on that, relying on professional online data analysis tools to facilitate the process is a great practice in this regard, as manually collecting and assessing raw data is not only very time-consuming and expensive but is also at risk of errors and subjectivity.
Once your data is collected, you need to carefully assess it to understand if the quality is appropriate to be used during a study. This means, is the sample size big enough? Were the procedures used to collect the data implemented correctly? Is the date range from the data correct? If coming from an external source, is it a trusted and objective one?
With all the needed information in hand, you are ready to start the interpretation process, but first, you need to visualize your data.
3) Use the right data visualization type
Data visualizations such as business graphs , charts, and tables are fundamental to successfully interpreting data. This is because data visualization via interactive charts and graphs makes the information more understandable and accessible. As you might be aware, there are different types of visualizations you can use, but not all of them are suitable for any analysis purpose. Using the wrong graph can lead to misinterpretation of your data, so it’s very important to carefully pick the right visual for it. Let’s look at some use cases of common data visualizations.
- Bar chart: One of the most used chart types, the bar chart uses rectangular bars to show the relationship between 2 or more variables. There are different types of bar charts for different interpretations, including the horizontal bar chart, column bar chart, and stacked bar chart.
- Line chart: Most commonly used to show trends, acceleration or decelerations, and volatility, the line chart aims to show how data changes over a period of time, for example, sales over a year. A few tips to keep this chart ready for interpretation are not using many variables that can overcrowd the graph and keeping your axis scale close to the highest data point to avoid making the information hard to read.
- Pie chart: Although it doesn’t do a lot in terms of analysis due to its uncomplex nature, pie charts are widely used to show the proportional composition of a variable. Visually speaking, showing a percentage in a bar chart is way more complicated than showing it in a pie chart. However, this also depends on the number of variables you are comparing. If your pie chart needs to be divided into 10 portions, then it is better to use a bar chart instead.
- Tables: While they are not a specific type of chart, tables are widely used when interpreting data. Tables are especially useful when you want to portray data in its raw format. They give you the freedom to easily look up or compare individual values while also displaying grand totals.
With the use of data visualizations becoming more and more critical for businesses’ analytical success, many tools have emerged to help users visualize their data in a cohesive and interactive way. One of the most popular ones is the use of BI dashboards . These visual tools provide a centralized view of various graphs and charts that paint a bigger picture of a topic. We will discuss the power of dashboards for an efficient data interpretation practice in the next portion of this post. If you want to learn more about different types of graphs and charts , take a look at our complete guide on the topic.
4) Start interpreting
After the tedious preparation part, you can start extracting conclusions from your data. As mentioned many times throughout the post, the way you decide to interpret the data will solely depend on the methods you initially decided to use. If you had initial research questions or hypotheses, then you should look for ways to prove their validity. If you are going into the data with no defined hypothesis, then start looking for relationships and patterns that will allow you to extract valuable conclusions from the information.
During the process of interpretation, stay curious and creative, dig into the data, and determine if there are any other critical questions that should be asked. If any new questions arise, you need to assess if you have the necessary information to answer them. Being able to identify if you need to dedicate more time and resources to the research is a very important step. No matter if you are studying customer behaviors or a new cancer treatment, the findings from your analysis may dictate important decisions in the future. Therefore, taking the time to really assess the information is key. For that purpose, data interpretation software proves to be very useful.
5) Keep your interpretation objective
As mentioned above, objectivity is one of the most important data interpretation skills but also one of the hardest. Being the person closest to the investigation, it is easy to become subjective when looking for answers in the data. A good way to stay objective is to show the information related to the study to other people, for example, research partners or even the people who will use your findings once they are done. This can help avoid confirmation bias and any reliability issues with your interpretation.
Remember, using a visualization tool such as a modern dashboard will make the interpretation process way easier and more efficient as the data can be navigated and manipulated in an easy and organized way. And not just that, using a dashboard tool to present your findings to a specific audience will make the information easier to understand and the presentation way more engaging thanks to the visual nature of these tools.
6) Mark your findings and draw conclusions
Findings are the observations you extracted from your data. They are the facts that will help you drive deeper conclusions about your research. For example, findings can be trends and patterns you found during your interpretation process. To put your findings into perspective, you can compare them with other resources that use similar methods and use them as benchmarks.
Reflect on your own thinking and reasoning and be aware of the many pitfalls data analysis and interpretation carry—correlation versus causation, subjective bias, false information, inaccurate data, etc. Once you are comfortable with interpreting the data, you will be ready to develop conclusions, see if your initial questions were answered, and suggest recommendations based on them.
Interpretation of Data: The Use of Dashboards Bridging The Gap
As we have seen, quantitative and qualitative methods are distinct types of data interpretation and analysis. Both offer a varying degree of return on investment (ROI) regarding data investigation, testing, and decision-making. But how do you mix the two and prevent a data disconnect? The answer is professional data dashboards.
For a few years now, dashboards have become invaluable tools to visualize and interpret data. These tools offer a centralized and interactive view of data and provide the perfect environment for exploration and extracting valuable conclusions. They bridge the quantitative and qualitative information gap by unifying all the data in one place with the help of stunning visuals.
Not only that, but these powerful tools offer a large list of benefits, and we will discuss some of them below.
1) Connecting and blending data. With today’s pace of innovation, it is no longer feasible (nor desirable) to have bulk data centrally located. As businesses continue to globalize and borders continue to dissolve, it will become increasingly important for businesses to possess the capability to run diverse data analyses absent the limitations of location. Data dashboards decentralize data without compromising on the necessary speed of thought while blending both quantitative and qualitative data. Whether you want to measure customer trends or organizational performance, you now have the capability to do both without the need for a singular selection.
2) Mobile Data. Related to the notion of “connected and blended data” is that of mobile data. In today’s digital world, employees are spending less time at their desks and simultaneously increasing production. This is made possible because mobile solutions for analytical tools are no longer standalone. Today, mobile analysis applications seamlessly integrate with everyday business tools. In turn, both quantitative and qualitative data are now available on-demand where they’re needed, when they’re needed, and how they’re needed via interactive online dashboards .
3) Visualization. Data dashboards merge the data gap between qualitative and quantitative data interpretation methods through the science of visualization. Dashboard solutions come “out of the box” and are well-equipped to create easy-to-understand data demonstrations. Modern online data visualization tools provide a variety of color and filter patterns, encourage user interaction, and are engineered to help enhance future trend predictability. All of these visual characteristics make for an easy transition among data methods – you only need to find the right types of data visualization to tell your data story the best way possible.
4) Collaboration. Whether in a business environment or a research project, collaboration is key in data interpretation and analysis. Dashboards are online tools that can be easily shared through a password-protected URL or automated email. Through them, users can collaborate and communicate through the data in an efficient way. Eliminating the need for infinite files with lost updates. Tools such as datapine offer real-time updates, meaning your dashboards will update on their own as soon as new information is available.
Examples Of Data Interpretation In Business
To give you an idea of how a dashboard can fulfill the need to bridge quantitative and qualitative analysis and help in understanding how to interpret data in research thanks to visualization, below, we will discuss three valuable examples to put their value into perspective.
1. Customer Satisfaction Dashboard
This market research dashboard brings together both qualitative and quantitative data that are knowledgeably analyzed and visualized in a meaningful way that everyone can understand, thus empowering any viewer to interpret it. Let’s explore it below.

**click to enlarge**
The value of this template lies in its highly visual nature. As mentioned earlier, visuals make the interpretation process way easier and more efficient. Having critical pieces of data represented with colorful and interactive icons and graphs makes it possible to uncover insights at a glance. For example, the colors green, yellow, and red on the charts for the NPS and the customer effort score allow us to conclude that most respondents are satisfied with this brand with a short glance. A further dive into the line chart below can help us dive deeper into this conclusion, as we can see both metrics developed positively in the past 6 months.
The bottom part of the template provides visually stunning representations of different satisfaction scores for quality, pricing, design, and service. By looking at these, we can conclude that, overall, customers are satisfied with this company in most areas.
2. Brand Analysis Dashboard
Next, in our list of data interpretation examples, we have a template that shows the answers to a survey on awareness for Brand D. The sample size is listed on top to get a perspective of the data, which is represented using interactive charts and graphs.
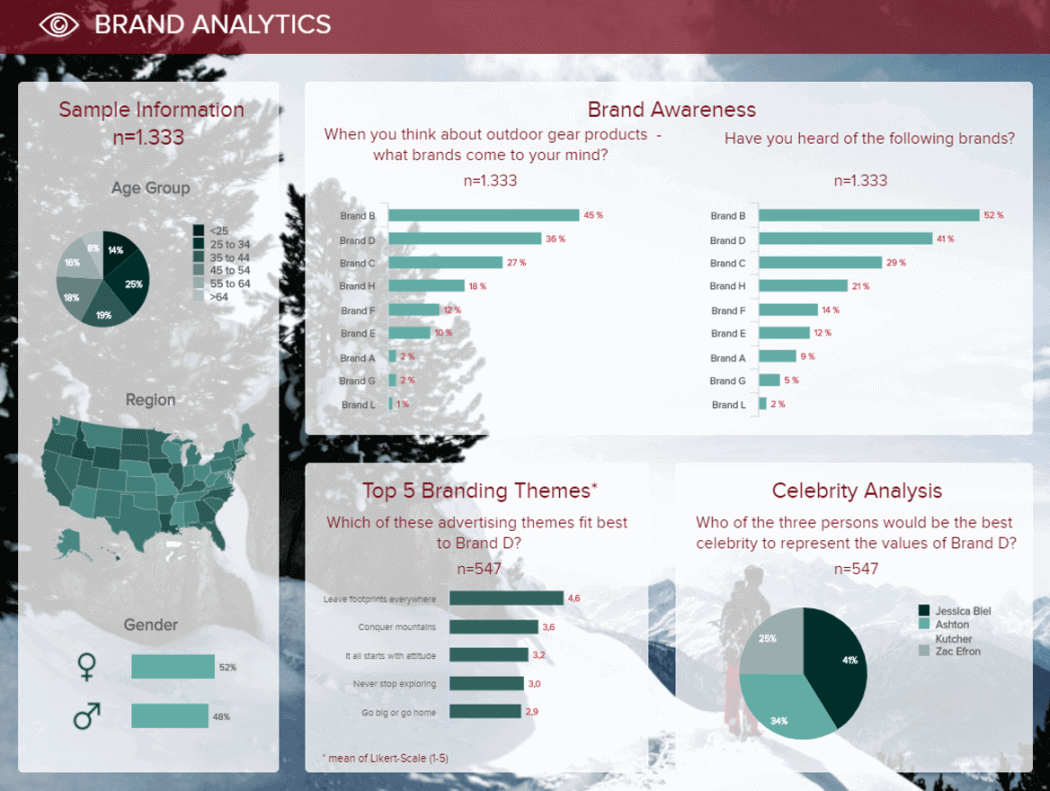
When interpreting information, context is key to understanding it correctly. For that reason, the dashboard starts by offering insights into the demographics of the surveyed audience. In general, we can see ages and gender are diverse. Therefore, we can conclude these brands are not targeting customers from a specified demographic, an important aspect to put the surveyed answers into perspective.
Looking at the awareness portion, we can see that brand B is the most popular one, with brand D coming second on both questions. This means brand D is not doing wrong, but there is still room for improvement compared to brand B. To see where brand D could improve, the researcher could go into the bottom part of the dashboard and consult the answers for branding themes and celebrity analysis. These are important as they give clear insight into what people and messages the audience associates with brand D. This is an opportunity to exploit these topics in different ways and achieve growth and success.
3. Product Innovation Dashboard
Our third and last dashboard example shows the answers to a survey on product innovation for a technology company. Just like the previous templates, the interactive and visual nature of the dashboard makes it the perfect tool to interpret data efficiently and effectively.
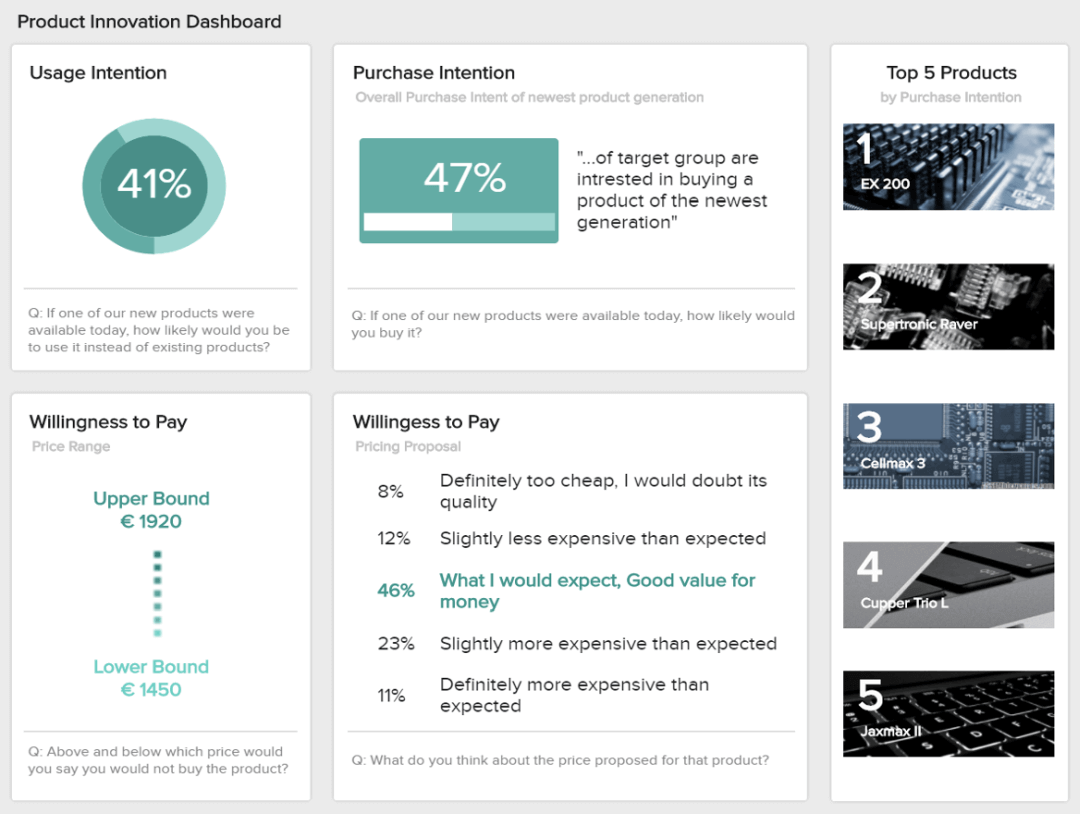
Starting from right to left, we first get a list of the top 5 products by purchase intention. This information lets us understand if the product being evaluated resembles what the audience already intends to purchase. It is a great starting point to see how customers would respond to the new product. This information can be complemented with other key metrics displayed in the dashboard. For example, the usage and purchase intention track how the market would receive the product and if they would purchase it, respectively. Interpreting these values as positive or negative will depend on the company and its expectations regarding the survey.
Complementing these metrics, we have the willingness to pay. Arguably, one of the most important metrics to define pricing strategies. Here, we can see that most respondents think the suggested price is a good value for money. Therefore, we can interpret that the product would sell for that price.
To see more data analysis and interpretation examples for different industries and functions, visit our library of business dashboards .
To Conclude…
As we reach the end of this insightful post about data interpretation and analysis, we hope you have a clear understanding of the topic. We've covered the definition and given some examples and methods to perform a successful interpretation process.
The importance of data interpretation is undeniable. Dashboards not only bridge the information gap between traditional data interpretation methods and technology, but they can help remedy and prevent the major pitfalls of the process. As a digital age solution, they combine the best of the past and the present to allow for informed decision-making with maximum data interpretation ROI.
To start visualizing your insights in a meaningful and actionable way, test our online reporting software for free with our 14-day trial !
- Skip to main content
- Skip to primary sidebar
- Skip to footer
- QuestionPro

- Solutions Industries Gaming Automotive Sports and events Education Government Travel & Hospitality Financial Services Healthcare Cannabis Technology Use Case NPS+ Communities Audience Contactless surveys Mobile LivePolls Member Experience GDPR Positive People Science 360 Feedback Surveys
- Resources Blog eBooks Survey Templates Case Studies Training Help center

Home Market Research Research Tools and Apps
Data Interpretation: Definition and Steps with Examples
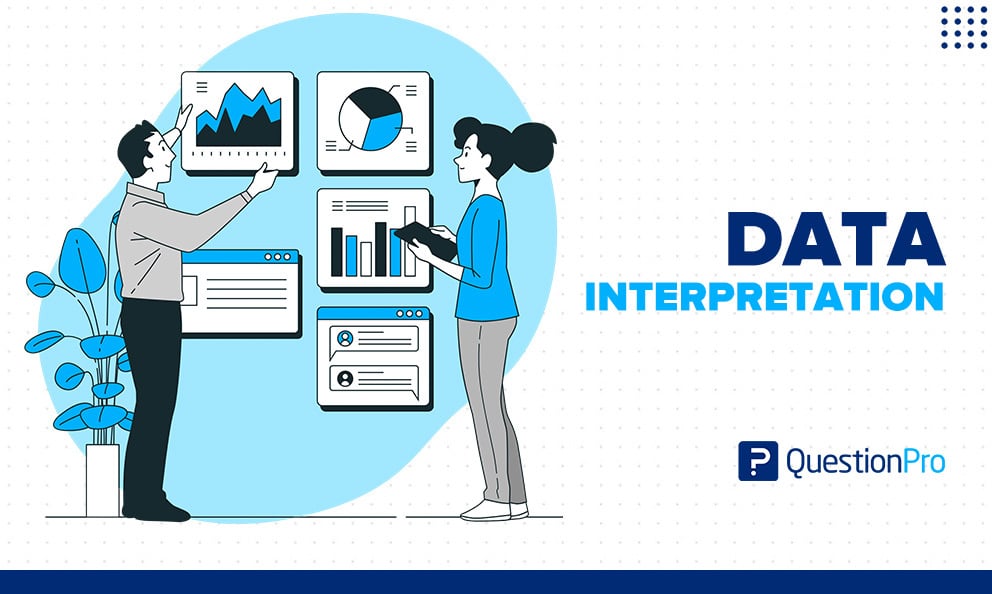
A good data interpretation process is key to making your data usable. It will help you make sure you’re drawing the correct conclusions and acting on your information.
No matter what, data is everywhere in the modern world. There are two groups and organizations: those drowning in data or not using it appropriately and those benefiting.
In this blog, you will learn the definition of data interpretation and its primary steps and examples.
What is Data Interpretation
Data interpretation is the process of reviewing data and arriving at relevant conclusions using various analytical research methods. Data analysis assists researchers in categorizing, manipulating data , and summarizing data to answer critical questions.
LEARN ABOUT: Level of Analysis
In business terms, the interpretation of data is the execution of various processes. This process analyzes and revises data to gain insights and recognize emerging patterns and behaviors. These conclusions will assist you as a manager in making an informed decision based on numbers while having all of the facts at your disposal.
Importance of Data Interpretation
Raw data is useless unless it’s interpreted. Data interpretation is important to businesses and people. The collected data helps make informed decisions.
Make better decisions
Any decision is based on the information that is available at the time. People used to think that many diseases were caused by bad blood, which was one of the four humors. So, the solution was to get rid of the bad blood. We now know that things like viruses, bacteria, and immune responses can cause illness and can act accordingly.
In the same way, when you know how to collect and understand data well, you can make better decisions. You can confidently choose a path for your organization or even your life instead of working with assumptions.
The most important thing is to follow a transparent process to reduce mistakes and tiredness when making decisions.
Find trends and take action
Another practical use of data interpretation is to get ahead of trends before they reach their peak. Some people have made a living by researching industries, spotting trends, and then making big bets on them.
LEARN ABOUT: Action Research
With the proper data interpretations and a little bit of work, you can catch the start of trends and use them to help your business or yourself grow.
Better resource allocation
The last importance of data interpretation we will discuss is the ability to use people, tools, money, etc., more efficiently. For example, If you know via strong data interpretation that a market is underserved, you’ll go after it with more energy and win.
In the same way, you may find out that a market you thought was a good fit is actually bad. This could be because the market is too big for your products to serve, there is too much competition, or something else.
No matter what, you can move the resources you need faster and better to get better results.
What are the steps in interpreting data?
Here are some steps to interpreting data correctly.
Gather the data
The very first step in data interpretation is gathering all relevant data. You can do this by first visualizing it in a bar, graph, or pie chart. This step aims to analyze the data accurately and without bias. Now is the time to recall how you conducted your research.
Here are two question patterns that will help you to understand better.
- Were there any flaws or changes that occurred during the data collection process?
- Have you saved any observatory notes or indicators?
You can proceed to the next stage when you have all of your data.
- Develop your discoveries
This is a summary of your findings. Here, you thoroughly examine the data to identify trends, patterns, or behavior. If you are researching a group of people using a sample population, this is the section where you examine behavioral patterns. You can compare these deductions to previous data sets, similar data sets, or general hypotheses in your industry. This step’s goal is to compare these deductions before drawing any conclusions.
- Draw Conclusions
After you’ve developed your findings from your data sets, you can draw conclusions based on your discovered trends. Your findings should address the questions that prompted your research. If they do not respond, inquire about why; it may produce additional research or questions.
LEARN ABOUT: Research Process Steps
- Give recommendations
The interpretation procedure of data comes to a close with this stage. Every research conclusion must include a recommendation. As recommendations are a summary of your findings and conclusions, they should be brief. There are only two options for recommendations; you can either recommend a course of action or suggest additional research.
Data interpretation examples
Here are two examples of data interpretations to help you understand it better:
Let’s say your users fall into four age groups. So a company can see which age group likes their content or product. Based on bar charts or pie charts, they can develop a marketing strategy to reach uninvolved groups or an outreach strategy to grow their core user base.
Another example of data analysis is the use of recruitment CRM by businesses. They utilize it to find candidates, track their progress, and manage their entire hiring process to determine how they can better automate their workflow.
Overall, data interpretation is an essential factor in data-driven decision-making. It should be performed on a regular basis as part of an iterative interpretation process. Investors, developers, and sales and acquisition professionals can benefit from routine data interpretation. It is what you do with those insights that determine the success of your business.
Contact QuestionPro experts if you need assistance conducting research or creating a data analysis. We can walk you through the process and help you make the most of your data.
MORE LIKE THIS
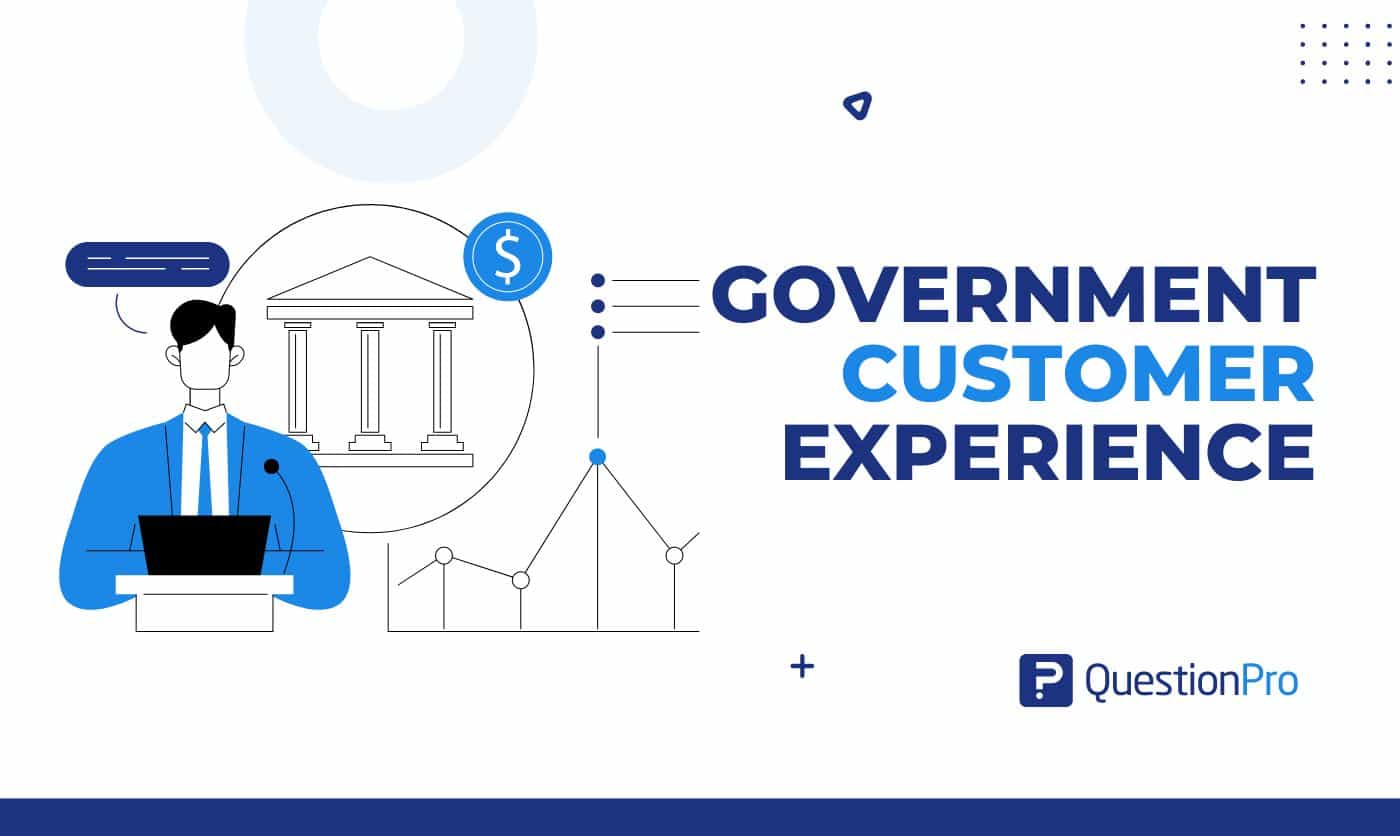
Government Customer Experience: Impact on Government Service
Apr 11, 2024
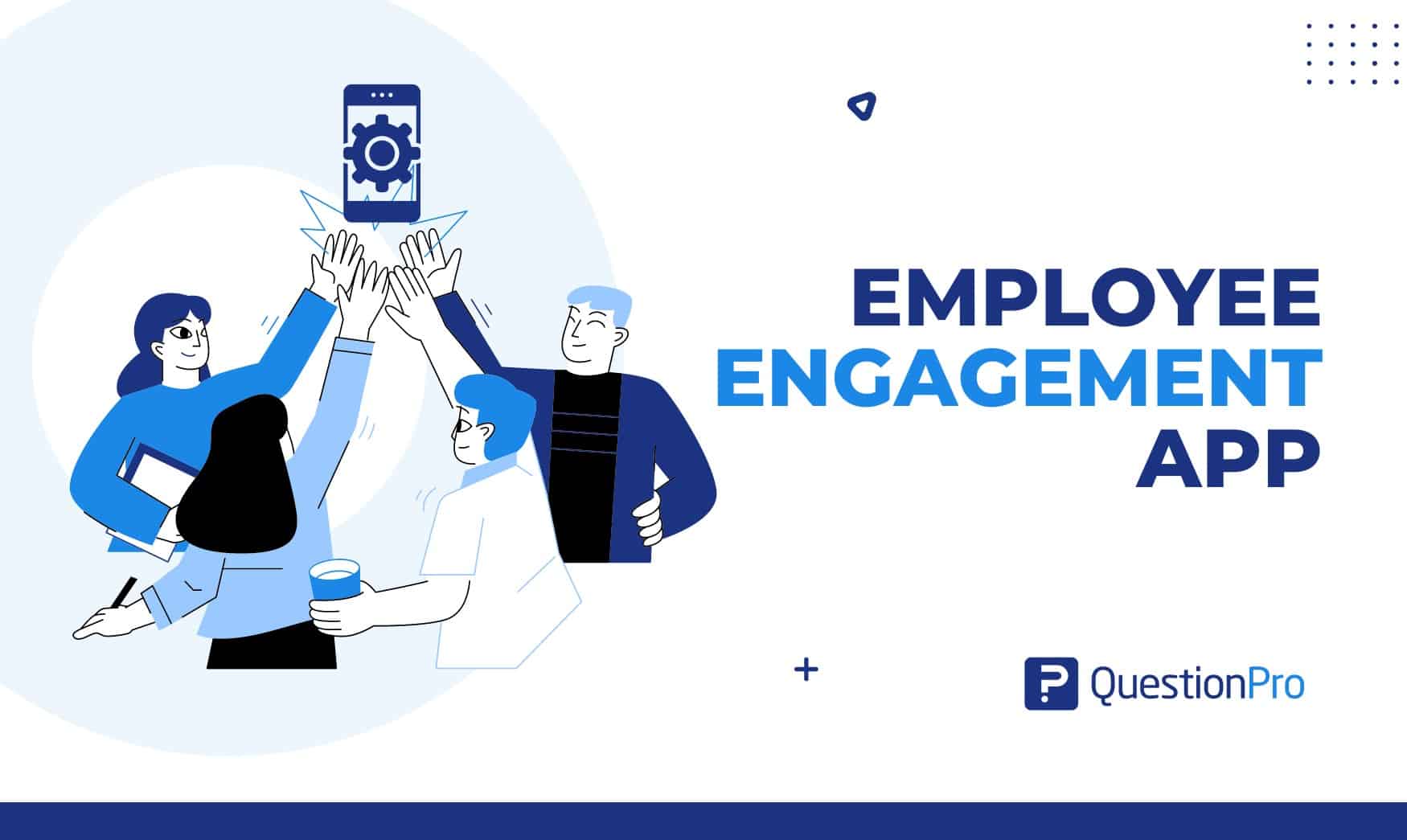
Employee Engagement App: Top 11 For Workforce Improvement
Apr 10, 2024
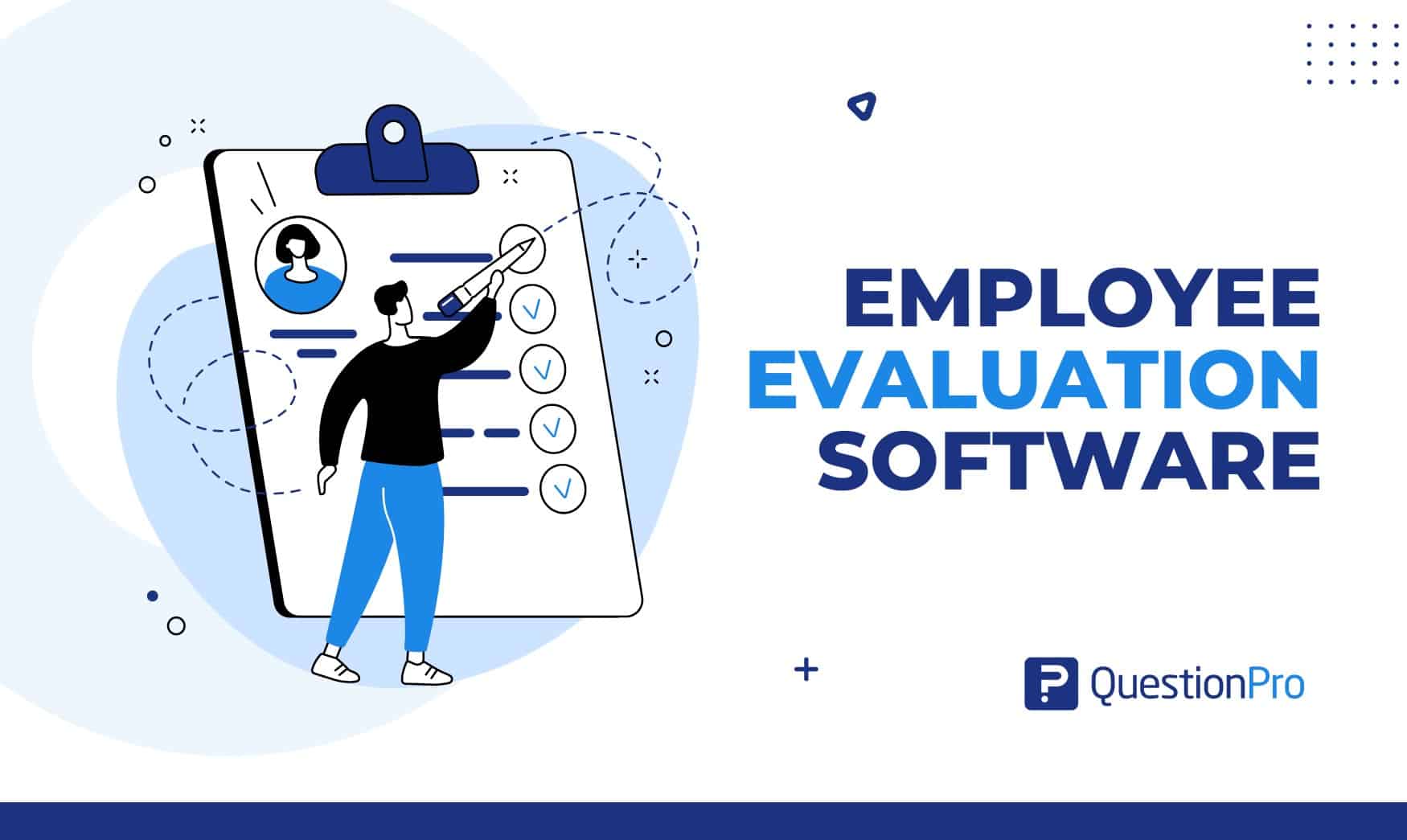
Top 15 Employee Evaluation Software to Enhance Performance
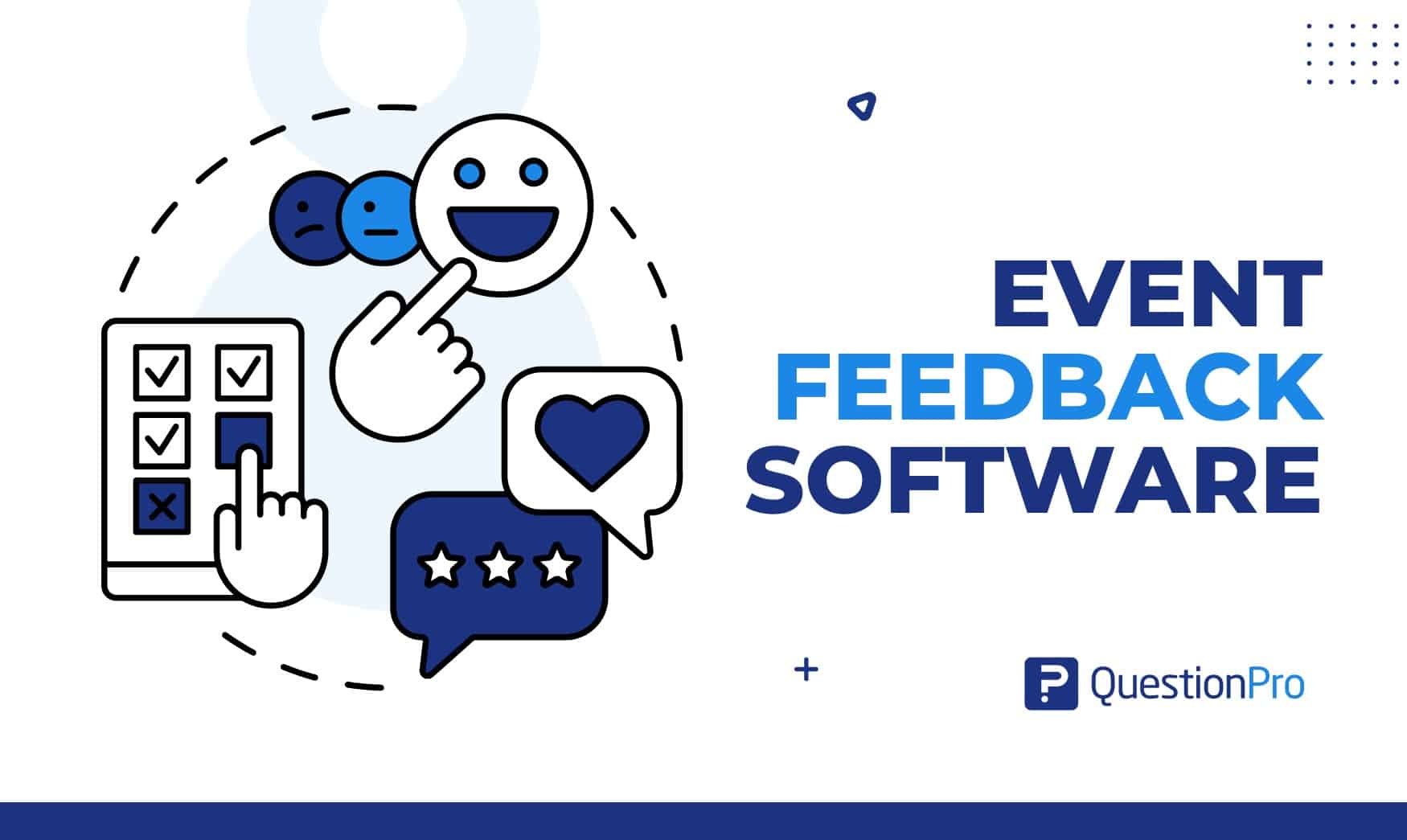
Event Feedback Software: Top 11 Best in 2024
Apr 9, 2024
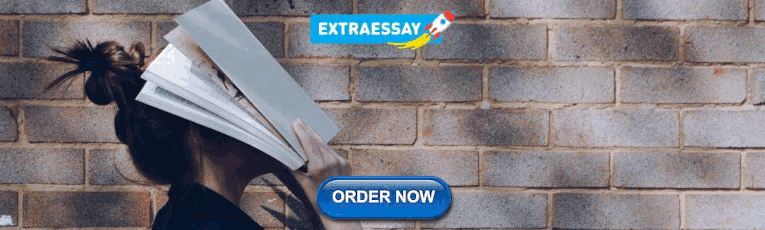
Other categories
- Academic Research
- Artificial Intelligence
- Assessments
- Brand Awareness
- Case Studies
- Communities
- Consumer Insights
- Customer effort score
- Customer Engagement
- Customer Experience
- Customer Loyalty
- Customer Research
- Customer Satisfaction
- Employee Benefits
- Employee Engagement
- Employee Retention
- Friday Five
- General Data Protection Regulation
- Insights Hub
- Life@QuestionPro
- Market Research
- Mobile diaries
- Mobile Surveys
- New Features
- Online Communities
- Question Types
- Questionnaire
- QuestionPro Products
- Release Notes
- Research Tools and Apps
- Revenue at Risk
- Survey Templates
- Training Tips
- Uncategorized
- Video Learning Series
- What’s Coming Up
- Workforce Intelligence

- Certifications

Data Analysis 101: How to Make Your Presentations Practical and Effective
- December 27, 2022
- 53 Comments
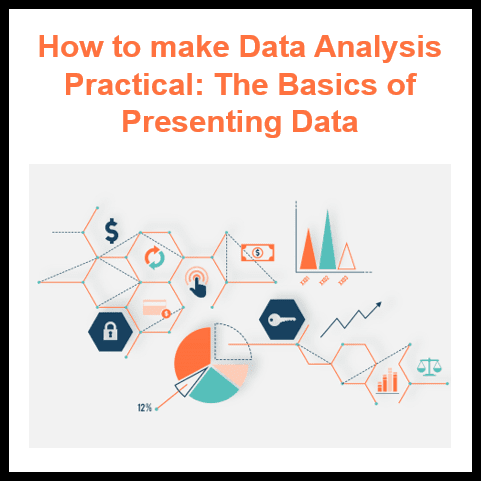
Understanding Importance of Data Analysis
The results of data analysis can give business the vital insights they need to turn in to successful and profitable ventures. It could be the difference between a successful business operation and a business operation that is in trouble.
Data analysis, though one of the most in-demand job roles globally, doesn’t require a degree in statistics or mathematics to do well, and employers from a wide variety of industries are very keen to recruit data analysts.
Businesses hire data analysts in the field of finance, marketing, administration, HR, IT and procurement, to name just a few. Understand the big picture and provide answers. By engaging in data analysis, you can actually delve deep and discover hidden truths that most business people would never be able to do.
What skills you should master to be a data analyst?
While Data Analyst roles are on the rise, there are certain skills that are vital for anyone who wants to become a data analyst . Before the job, a candidate needs to have either a degree in statistics, business or computer science or a related subject, or work experience in these areas.
If you’re interested in becoming a data analyst, you’ll need to know:
- Programming and algorithms
- Data Visualization
- Open-source and cloud technologies
- No coding experience is required.
How much is a data analyst worth? Data analysts earn an average salary of £32,403 per annum, according to jobs site Glassdoor. This pays for a salary, with benefits such as medical insurance and paid leave included in the starting salary. If you think you have the right skills, there are plenty of roles on offer.
What data analysis entails
Data analysis is an analytical process which involves recording and tabulating (recording and entering, entering and tabulating) the quantities of a product, such as numbers of units produced, costs of materials and expenses.
While data analyst can take different forms, for example in databases, in other structures such as spreadsheets, numbers are the main means of data entry. This involves entering and entering the required data in a data analysis system such as Excel.
For example, although a database doesn’t require a data analyst, it can still benefit from data analysis techniques such as binomial testing, ANOVA and Fisher’s exact tests. Where is the data analysis courses in IT? Given the ever-increasing reliance on technology in business, data analysis courses are vital skills.
What are the types of data analysis methods?
- Cluster analysis
The act of grouping a specific set of data in a manner that those elements are more similar to one another than to those in other groups – hence the term ‘cluster.’ Since there is no special target variable while doing clustering, the method is often used to find hidden patterns in the data. The approach is purposely used to offer additional context to a particular trend or dataset.
- Cohort analysis
This type of data analysis method uses historical data to examine and compare a determined segment of users’ behavior, which can then be grouped with others with similar characteristics. By using this data analysis methodology, it’s possible to gain a wealth of insight into consumer needs or a firm understanding of a broader target group.
A dependent variable is an element of a complex system that is assumed to have a single cause, but it’s affected by multiple factors, thus giving researchers an indication as to how a complex system function.
- Regression analysis
The regression analysis is used to predict how the value of a dependent variable changes when one or more independent variables change, stay the same or the dependent variable is not moved. Regression is a sophisticated statistical method that includes mathematical functions that are typically called “segmentation,” “distribution,” and “intercept” functions.
Regression is a type of regression analysis that only contains linear and quadratic functions. You can change the types of factors (or the independent variables) that are selected in regression analysis (it’s typically called “nonlinear regression analysis”) by changing the order in which the models are constructed.To begin, let’s explain how regression analysis works.
Examples in business world
The Oracle Corporation is one of the first multinational companies to adopt this type of analysis method, based on which the company was able to develop predictive modelling systems for marketing purposes.
In a more specific sense, a Regression analysis is a popular type of data analysis used for analyzing the likelihood that a random variable will move up or down a range of parameters in response to a change in a specific control variable.
Companies who use this type of analysis are looking for trends and patterned performance over time. For example, how a company may respond to a rising cost of labor and its effect on its business bottom line, a weather-related issue like an earthquake, a new advertising campaign, or even a surge in customer demand in some areas.
What are basic pointers to consider while presenting data
Recognize that presentation matters.
Too often, analysts make the mistake of presenting information in order to show an abstracted version of it. For instance, say a B2B company has 4 ways to improve their sales funnel:
- More Visually Engaging
- More Easily Transacted
- More Cost Effective
Then, “informative” would mean that a B2B company needs to optimize their sales funnel to each of these to be more “convenient, faster, easier, more visually engaging, or most cost effective.” Sure, it would be nice if they all improved – they would all provide a competitive advantage in some way. But that’s not what the data tells us.
Don’t scare people with numbers
When you’re presenting data, show as many as possible, in as many charts as possible. Then, try to talk through the implications of the data, rather than overwhelming people with an overwhelming amount of data.
Why? Research suggests that when a number is presented in a visual, people become more likely to process it and learn from it. I recommend using video, text, graphs, and pictures to represent your numbers. This creates a more visually appealing data set. The number of followers on Twitter is visually appealing. The number of followers on Facebook is visually appealing. But nobody looks at their Twitter followers. If you don’t know what your numbers mean, how will your audience? That doesn’t mean numbers aren’t important.
Maximize the data pixel ratio
The more data you show to a critical stakeholder, the more likely they are to get lost and distracted from what you’re actually trying to communicate. This is especially important in the case of people in the sales and marketing function.
Do you have a sales person out in the field who is trying to close a deal? It would be a shame if that person got lost in your Excel analytics and lost out on the sale. This problem also occurs on the web.
Consider how web visitors respond to large, colorful charts and graphs. If we’re talking about visualizations that depict web performance, a visual might be helpful. But how often do we see this done? Research shows that people respond better to web-based data in a simplified, less complex format.
Save 3-D for the movies
There are great stories in the universe. This is an oversimplification, but if you look at history, humans only understand stories. We are great storytellers. We develop, through trial and error, our own intuition about the “right” way to tell stories.
One of the most powerful and effective ways to present data is to go beyond the visual to the audible, that is, to tell stories in a way that people can relate to. Everything you hear about computers being a series of numbers is wrong. We visualize numbers in a precise, quantitative way. But the numbers are not a collection of isolated events. To understand them, we need to understand the broader context.
Friends don’t let friends use pie charts
Businesses and analysts have done this since pie charts first appeared on Microsoft Excel sheets. When presenting data, break down your pie chart into its component segments.
As opposed to an equal-sized circle for the average earnings for all the employees, share a pie chart where the percentages for each individual segment are different, with a link to the corresponding chart.
Pair with explanatory text, show their correlation, and make your choice based on your audience, not on whether you want to scare or “educate” them. The majority of audiences will see the same image, regardless of whether it’s presented in a bar chart, bar chart, line chart, or something else.
Choose the appropriate chart
Does the data make logical sense? Check your assumptions against the data. Are the graphs charting only part of the story? Include other variables in the graphs. Avoid using axis labels to mislead. Never rely on axes to infer, “logical” conclusions. Trust your eyes: you know what information your brain can process.
Think of numbers like music — they are pleasing, but not overwhelming. Save 3D for the movies. When everyone is enjoying 4K, 8K, and beyond, it’s hard to envision your audience without the new stuff. I remember the first time I got to see HDTV. At home, I sat behind a chair and kept turning around to watch the TV. But at the theatre, I didn’t need a chair. All I had to do was look up, and see the giant screen, the contrast, and the detail.
Don’t mix chart types for no reason
Excel chart s with colored areas help people focus. Arrows give us scale. Assume your audience doesn’t understand what you’re saying, even if they do. Nobody wants to open a recipe book to learn how to cook soup. Instead, we start with a recipe.
Use a formula to communicate your analysis with as few words as possible. Keep it simple. Resist the urge to over-complicate your presentation. A word cloud is not a word cloud. A bar chart is not a bar chart. If you use a word cloud to illustrate a chart, consider replacing a few words with a gif. A bar chart doesn’t need clouds. And a bar chart doesn’t need clouds. If there’s one thing that’s sure to confuse your audience, it’s bar charts.
Use color with intention
Use color with intention. It’s not about pretty. When it comes to presenting data clearly, “informative” is more important than “beautiful.”
However, visualizations like maps, axes, or snapshots can help visual communication to avoid this pitfall. If you are going to show a few locations on a map, make sure each location has a voice and uses a distinct color. Avoid repeating colors from the map or bottom bar in all the visuals. Be consistent with how you present the data . A pie chart is not very interesting if all it shows is a bunch of varying sizes of the pie.
Data analysis in the workplace, and how it will impact the future of business
Business leaders are taking note of the importance of data analysis skills in their organisation, as it can make an enormous impact on business.
Larger organisations such as Google, Amazon and Facebook employ huge teams of analysts to create their data and statistics. We are already seeing the rise of the next generation of big data analysts – those who can write code that analyses and visualizes the data and report back information to a company to help it improve efficiency and increase revenue.
The increasing need for high-level understanding of data analysis has already led to the role of data analyst becoming available at university level. It is no longer a mandatory business qualification but one that can enhance your CV.
By understanding the importance of each variable, you can improve your business by managing your time and creating more effective systems and processes for running your business. The focus shifts from just providing services to providing value to your customers, creating a better, more intuitive experience for them so they can work with your company for the long-term.
Adopting these small steps will allow you to be more effective in your business and go from being an employee to an entrepreneur.
Share This Post:
53 thoughts on “data analysis 101: how to make your presentations practical and effective”.
Buy Zyvox Online – Special offer: Save up to $498 – buy antibiotics online and get discount for all purchased!
Thanks again for the post.Thanks Again. Cool.
Thanks for great information. What trips can you recommend in 2024? Astro tourism, eco diving, home swapping, train stations are the new food destinations,sports tourism, coolcationing, gig tripping, private group travel?
Enjoyed every bit of your article.Really looking forward to read more. Great.
Really appreciate you sharing this post. Want more.
I am so grateful for your blog. Really Great.
Hey, thanks for the blog post.
Great, thanks for sharing this blog.Thanks Again. Will read on…
Thanks for sharing, this is a fantastic blog.Thanks Again. Great.
Thanks for sharing, this is a fantastic article.Really thank you! Fantastic.
Major thankies for the article post.Thanks Again. Will read on…
Hey, thanks for the blog post. Cool.
A round of applause for your blog post.Really looking forward to read more. Cool.
Appreciate you sharing, great article post. Great.
wow, awesome blog.Much thanks again. Cool.
Say, you got a nice blog.Really thank you! Cool.
Enjoyed every bit of your blog article.Much thanks again. Want more.
A round of applause for your blog post.Much thanks again. Cool.
I’m not sure where you’re getting your info, but good topic. I needs to spend some time learning more or understanding more. Thanks for wonderful info I was looking for this info for my mission.
Im thankful for the blog post.Much thanks again.
A big thank you for your article.Really thank you!
I truly appreciate this article post.Really looking forward to read more. Really Cool.
I really enjoy the article post.Much thanks again.
wow, awesome blog.Thanks Again. Will read on…
Awesome blog post.Much thanks again. Much obliged.
Im thankful for the blog.Much thanks again. Want more.
Thanks a lot for the post.Much thanks again. Want more.
I really liked your article.Really thank you! Really Cool.
A round of applause for your post.Thanks Again. Much obliged.
Say, you got a nice article.Really thank you! Fantastic.
I value the blog article. Really Cool.
A round of applause for your blog article.Really looking forward to read more. Great.
Really appreciate you sharing this blog article. Really Cool.
Really informative article. Really Great.
Fantastic article post.Really looking forward to read more. Really Great.
I really liked your post.Much thanks again. Much obliged.
Beneficial document helps make frequent advance, appreciate it write about, this pile-up connected with expertise is usually to hold finding out, focus is usually the beginning of money.
I really liked your blog article.Much thanks again. Really Great.
I really liked your article post.Really thank you! Fantastic.
Great, thanks for sharing this blog.Thanks Again. Awesome.
Enjoyed every bit of your blog article. Cool.
I really enjoy the blog. Want more.
Im grateful for the blog.Thanks Again. Awesome.
Very neat blog.Thanks Again. Cool.
Muchos Gracias for your blog article.
Im grateful for the post.Really looking forward to read more. Keep writing.
Great, thanks for sharing this post.Really looking forward to read more. Cool.
Thank you ever so for you article post.Really looking forward to read more. Will read on…
Great article post.Much thanks again. Great.
I really like and appreciate your blog post.Thanks Again. Awesome.
Really appreciate you sharing this blog. Really Cool.
Greetings! Incredibly helpful suggestions within this short article! It’s the very little adjustments that make the greatest modifications. Quite a few many thanks for sharing!
Awesome blog.Really looking forward to read more. Much obliged.
Add a Comment Cancel reply
Save my name, email, and website in this browser for the next time I comment.
Get A 5X Raise In Salary
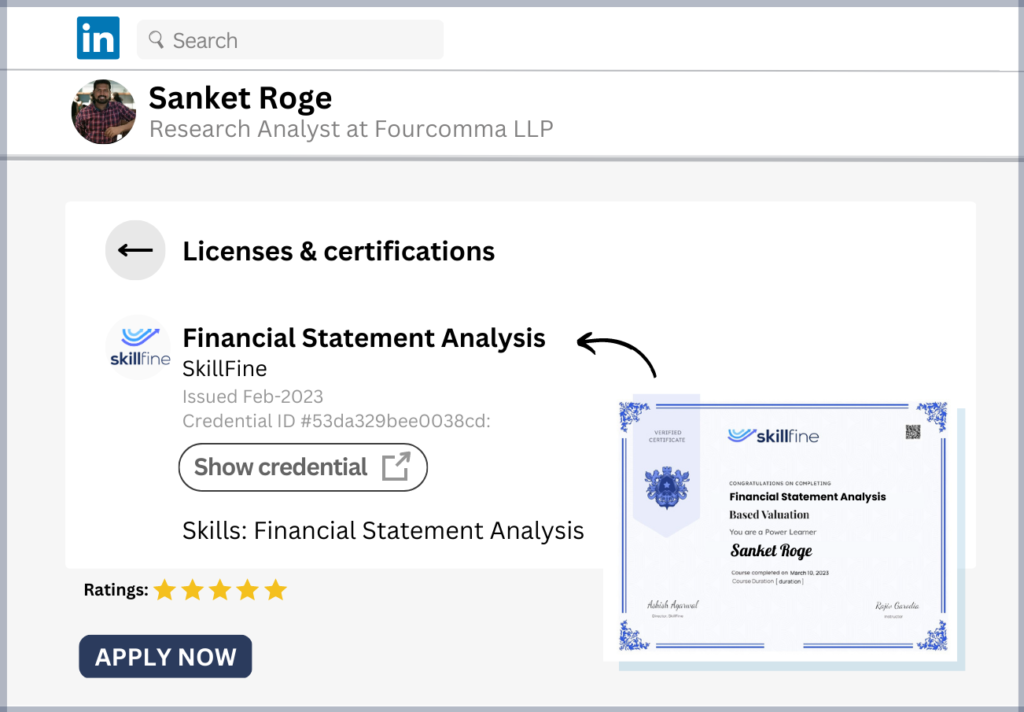
Reset Password
Insert/edit link.
Enter the destination URL
Or link to existing content
Data Collection, Presentation and Analysis
- First Online: 25 May 2023
Cite this chapter
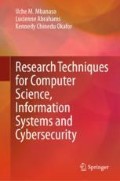
- Uche M. Mbanaso 4 ,
- Lucienne Abrahams 5 &
- Kennedy Chinedu Okafor 6
482 Accesses
This chapter covers the topics of data collection, data presentation and data analysis. It gives attention to data collection for studies based on experiments, on data derived from existing published or unpublished data sets, on observation, on simulation and digital twins, on surveys, on interviews and on focus group discussions. One of the interesting features of this chapter is the section dealing with using measurement scales in quantitative research, including nominal scales, ordinal scales, interval scales and ratio scales. It explains key facets of qualitative research including ethical clearance requirements. The chapter discusses the importance of data visualization as key to effective presentation of data, including tabular forms, graphical forms and visual charts such as those generated by Atlas.ti analytical software.
This is a preview of subscription content, log in via an institution to check access.
Access this chapter
- Available as PDF
- Read on any device
- Instant download
- Own it forever
- Available as EPUB and PDF
- Durable hardcover edition
- Dispatched in 3 to 5 business days
- Free shipping worldwide - see info
Tax calculation will be finalised at checkout
Purchases are for personal use only
Institutional subscriptions
Bibliography
Abdullah, M. F., & Ahmad, K. (2013). The mapping process of unstructured data to structured data. Proceedings of the 2013 International Conference on Research and Innovation in Information Systems (ICRIIS) , Malaysia , 151–155. https://doi.org/10.1109/ICRIIS.2013.6716700
Adnan, K., & Akbar, R. (2019). An analytical study of information extraction from unstructured and multidimensional big data. Journal of Big Data, 6 , 91. https://doi.org/10.1186/s40537-019-0254-8
Article Google Scholar
Alsheref, F. K., & Fattoh, I. E. (2020). Medical text annotation tool based on IBM Watson Platform. Proceedings of the 2020 6th international conference on advanced computing and communication systems (ICACCS) , India , 1312–1316. https://doi.org/10.1109/ICACCS48705.2020.9074309
Cinque, M., Cotroneo, D., Della Corte, R., & Pecchia, A. (2014). What logs should you look at when an application fails? Insights from an industrial case study. Proceedings of the 2014 44th Annual IEEE/IFIP International Conference on Dependable Systems and Networks , USA , 690–695. https://doi.org/10.1109/DSN.2014.69
Gideon, L. (Ed.). (2012). Handbook of survey methodology for the social sciences . Springer.
Google Scholar
Leedy, P., & Ormrod, J. (2015). Practical research planning and design (12th ed.). Pearson Education.
Madaan, A., Wang, X., Hall, W., & Tiropanis, T. (2018). Observing data in IoT worlds: What and how to observe? In Living in the Internet of Things: Cybersecurity of the IoT – 2018 (pp. 1–7). https://doi.org/10.1049/cp.2018.0032
Chapter Google Scholar
Mahajan, P., & Naik, C. (2019). Development of integrated IoT and machine learning based data collection and analysis system for the effective prediction of agricultural residue/biomass availability to regenerate clean energy. Proceedings of the 2019 9th International Conference on Emerging Trends in Engineering and Technology – Signal and Information Processing (ICETET-SIP-19) , India , 1–5. https://doi.org/10.1109/ICETET-SIP-1946815.2019.9092156 .
Mahmud, M. S., Huang, J. Z., Salloum, S., Emara, T. Z., & Sadatdiynov, K. (2020). A survey of data partitioning and sampling methods to support big data analysis. Big Data Mining and Analytics, 3 (2), 85–101. https://doi.org/10.26599/BDMA.2019.9020015
Miswar, S., & Kurniawan, N. B. (2018). A systematic literature review on survey data collection system. Proceedings of the 2018 International Conference on Information Technology Systems and Innovation (ICITSI) , Indonesia , 177–181. https://doi.org/10.1109/ICITSI.2018.8696036
Mosina, C. (2020). Understanding the diffusion of the internet: Redesigning the global diffusion of the internet framework (Research report, Master of Arts in ICT Policy and Regulation). LINK Centre, University of the Witwatersrand. https://hdl.handle.net/10539/30723
Nkamisa, S. (2021). Investigating the integration of drone management systems to create an enabling remote piloted aircraft regulatory environment in South Africa (Research report, Master of Arts in ICT Policy and Regulation). LINK Centre, University of the Witwatersrand. https://hdl.handle.net/10539/33883
QuestionPro. (2020). Survey research: Definition, examples and methods . https://www.questionpro.com/article/survey-research.html
Rajanikanth, J. & Kanth, T. V. R. (2017). An explorative data analysis on Bangalore City Weather with hybrid data mining techniques using R. Proceedings of the 2017 International Conference on Current Trends in Computer, Electrical, Electronics and Communication (CTCEEC) , India , 1121-1125. https://doi/10.1109/CTCEEC.2017.8455008
Rao, R. (2003). From unstructured data to actionable intelligence. IT Professional, 5 , 29–35. https://www.researchgate.net/publication/3426648_From_Unstructured_Data_to_Actionable_Intelligence
Schulze, P. (2009). Design of the research instrument. In P. Schulze (Ed.), Balancing exploitation and exploration: Organizational antecedents and performance effects of innovation strategies (pp. 116–141). Gabler. https://doi.org/10.1007/978-3-8349-8397-8_6
Usanov, A. (2015). Assessing cybersecurity: A meta-analysis of threats, trends and responses to cyber attacks . The Hague Centre for Strategic Studies. https://www.researchgate.net/publication/319677972_Assessing_Cyber_Security_A_Meta-analysis_of_Threats_Trends_and_Responses_to_Cyber_Attacks
Van de Kaa, G., De Vries, H. J., van Heck, E., & van den Ende, J. (2007). The emergence of standards: A meta-analysis. Proceedings of the 2007 40th Annual Hawaii International Conference on Systems Science (HICSS’07) , USA , 173a–173a. https://doi.org/10.1109/HICSS.2007.529
Download references
Author information
Authors and affiliations.
Centre for Cybersecurity Studies, Nasarawa State University, Keffi, Nigeria
Uche M. Mbanaso
LINK Centre, University of the Witwatersrand, Johannesburg, South Africa
Lucienne Abrahams
Department of Mechatronics Engineering, Federal University of Technology, Owerri, Nigeria
Kennedy Chinedu Okafor
You can also search for this author in PubMed Google Scholar
Rights and permissions
Reprints and permissions
Copyright information
© 2023 The Author(s), under exclusive license to Springer Nature Switzerland AG
About this chapter
Mbanaso, U.M., Abrahams, L., Okafor, K.C. (2023). Data Collection, Presentation and Analysis. In: Research Techniques for Computer Science, Information Systems and Cybersecurity. Springer, Cham. https://doi.org/10.1007/978-3-031-30031-8_7
Download citation
DOI : https://doi.org/10.1007/978-3-031-30031-8_7
Published : 25 May 2023
Publisher Name : Springer, Cham
Print ISBN : 978-3-031-30030-1
Online ISBN : 978-3-031-30031-8
eBook Packages : Engineering Engineering (R0)
Share this chapter
Anyone you share the following link with will be able to read this content:
Sorry, a shareable link is not currently available for this article.
Provided by the Springer Nature SharedIt content-sharing initiative
- Publish with us
Policies and ethics
- Find a journal
- Track your research
data analysis, interpretation, and presentation
Depends on data.
quantitative
qualitative
mixed methods
translating raw data
questionnaires

observations
begins with initial reactions or observations
identify patterns
calculating values
data cleansing: check for errors
use structured frameworks and theories
interpretation
parallel with analysis
results interpreted different ways
make sure data supports conclusion
avoid biases
avoid over claiming
presentation
different methods, depends on goals
affects interpretation

quantitative analysis
Statistical analysis.
percentages
individual differences
task times: 45, 50, 55, 55, 60, 65
task times: 45, 50, 55, 55, 60, 300
task times: 10, 10, 50, 55, 60, 300
median: 52.5
data format
table: rows and columns
how to represent responses and measures
participants as rows
responses: single or multiple
spreadsheet applications
analysis tools: R, SPSS, SAS

scatter plots
presenting percentages
almost always better ways
comparison difficult when percentages similar
include percentages as text
provide overview with other representations
qualitative analysis
Initial approach.
gain overall understanding
look for interesting features
highlight common, record surprises
inductive vs deductive
inductive: extract concepts from the data
deductive: use existing theory to categorize
depends on data and goals
inductive, then deductive
reliable analysis
replication
training towards consensus
inter-rater reliability or agreement
Cohen's kappa
transcription: oTranscribe , Otter.ai , Trint
coding and analysis: Dedoose, Atlas.ai, Nvivo
identifying themes
Categorizing data.
analyzing critical incidents
thematic analysis
identify, analyze, and report patterns
themes represent important, relevant, or unexpected patterns
open coding: initial pass
axial coding: themes across participants, connections, categories
selective coding: validate relationships, consistency, themes
find further themes
step back, look at big picture
affinity diagrams
organize ideas and insights into hierarchy
groupings emerge through data
analysis frame chosen beforehand
study goals
categorization schemes
evolve with analysis
- Verbalizations show evidence of dissatisfaction about an aspect of the interface.
- Verbalizations show evidence of confusion/uncertainty about an aspect of the interface.
- Verbalizations show evidence of dissatisfaction about aspects of the content of the electronic text.
critical incident analysis
help deal with lots of data
identify significant subsets
critical events
focus on important or unique events
apply in both data gathering and analysis
useful when cause of problem unknown
disadvantages
may miss routine incidents
not as good for general task analysis
interpreting qualitative data
Analytic frameworks, conversation analysis, discourse analysis, content analysis, interaction analysis, grounded theory.
semantics of conversation in detail
focus on how conversation conducted
compare conversations across different media
voice-assisted technologies
voice assistants
conversational interactions
how do these devices change social behaviors? in home? at work?
do these devices change the way we talk?
interaction interwoven with other activities Martin Porcheron et al. [2018]
interleaving conversations with people and devices
instructing rather than conversing
communication tools
zoom, discord
mediate much of our communication
do these tools change the way we talk to each other?
the ways we collaborate?
meaning of what is said and how words are used
context important
strongly interpretive
no objective scientific truth
how people use language to construct perspectives

identify subtle and implicit meaning
communication: e-mails, social media, interviews
scraping data
time consuming
analysis via statistics and visualizations
tag / word clouds

classifying data into themes
studying frequency of theme occurrence
used for a range of media
used in conjunction with other approaches
interactions of humans with each other and objects
verbal and non-verbal
data: video recordings
knowledge and action are social
goal: derive generalizations of activities based on actions
interaction analysis: first step
teams suggest general patterns from multiple observations
collaborative
first: create content logs summarizing happenings
categories emerge through repeated play and discussion
intentions, motivations, and understandings
cannibalizing videos
extracting interesting material
reclassifying in term of material
instances assembled in playlist
derive theory grounded in data
from sociology
theory: set of concepts that constitute a framework for explaining or predicting phenomena
grounded theory: method
alternating data collection and analysis
identify themes, then refine with more data
continues until saturation, no new emergent themes
balance objectivity and sensitivity
goal: define properties and dimensions of relevant themes (categories)
grounded theory: data gathering
focus on analysis
interviews and observations
written records and diagrammatic representations
physical code books
grounded theory: coding
open coding: categories, properties, and dimensions discovered
axial coding: flesh out categories and relate to subcategories
selecting coding: refining and integrating categories
grounded theory: analytic tools
question the data: different perspectives, escape ruts
analysis of a word, phrase, or sentence: different perspectives
comparison: between objects or categories → alternative interpretations
interpreting and presenting findings
Presenting findings.
tables of numbers and text
graphical devices, such as charts and diagrams
set of themes or categories
choice depends on the data and analytic method
details of data collection and analysis
set of complementary representations
structured notations
analyze, capture, and present information
clear syntax and semantics
structured notation: advantages
meaning of symbols well-defined
highlights what to look for
enforces precision in expression
structured notation: disadvantages
by highlighting, ignores other aspects
precise expression lost on an unfamiliar audience
combine with other more accessible formats
storytelling
easy and intuitive approach
stories told by participants during data gathering
stories based on observation
stories constructed from snippets or repeated episodes in data
scenarios derived from stories collected during data gathering

summarizing findings
combination of presentation formats
careful interpretation and presentation important
not specific to interaction design
common pitfall: over-generalizing results
reading for next class
Chapter 12: Design, Prototyping, and Construction Interaction Design: Beyond Human-Computer Interaction

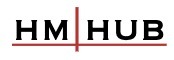
Data Analysis, Interpretation, and Presentation Techniques: A Guide to Making Sense of Your Research Data
by Prince Kumar
Last updated: 27 February 2023
Table of Contents
Data analysis, interpretation, and presentation are crucial aspects of conducting high-quality research. Data analysis involves processing and analyzing the data to derive meaningful insights, while data interpretation involves making sense of the insights and drawing conclusions. Data presentation involves presenting the data in a clear and concise way to communicate the research findings. In this article, we will discuss the techniques for data analysis, interpretation, and presentation.
1. Data Analysis Techniques
Data analysis techniques involve processing and analyzing the data to derive meaningful insights. The choice of data analysis technique depends on the research question and objectives. Some common data analysis techniques are:
a. Descriptive Statistics
Descriptive statistics involves summarizing and describing the data using measures such as mean, median, and standard deviation.
b. Inferential Statistics
Inferential statistics involves making inferences about the population based on the sample data. This technique involves hypothesis testing, confidence intervals, and regression analysis.
c. Content Analysis
Content analysis involves analyzing the text, images, or videos to identify patterns and themes.
d. Data Mining
Data mining involves using statistical and machine learning techniques to analyze large datasets and identify patterns.
2. Data Interpretation Techniques
Data interpretation involves making sense of the insights derived from the data analysis. The choice of data interpretation technique depends on the research question and objectives. Some common data interpretation techniques are:
a. Data Visualization
Data visualization involves presenting the data in a visual format, such as charts, graphs, or tables, to communicate the insights effectively.
b. Storytelling
Storytelling involves presenting the data in a narrative format, such as a story, to make the insights more relatable and memorable.
c. Comparative Analysis
Comparative analysis involves comparing the research findings with the existing literature or benchmarks to draw conclusions.
3. Data Presentation Techniques
Data presentation involves presenting the data in a clear and concise way to communicate the research findings. The choice of data presentation technique depends on the research question and objectives. Some common data presentation techniques are:
a. Tables and Graphs
Tables and graphs are effective data presentation techniques for presenting numerical data.
b. Infographics
Infographics are effective data presentation techniques for presenting complex data in a visual and easy-to-understand format.
c. Data Storytelling
Data storytelling involves presenting the data in a narrative format to communicate the research findings effectively.
In conclusion, data analysis, interpretation, and presentation are crucial aspects of conducting high-quality research. By using the appropriate data analysis, interpretation, and presentation techniques, researchers can derive meaningful insights, make sense of the insights, and communicate the research findings effectively. By conducting high-quality data analysis, interpretation, and presentation in research, researchers can provide valuable insights into the research question and objectives.
How useful was this post?
5 star mean very useful & 1 star means not useful at all.
Average rating / 5. Vote count:
No votes so far! Be the first to rate this post.
We are sorry that this post was not useful for you! 😔
Let us improve this post!
Tell us how we can improve this post?
Syllabus – Research Methodology
01 Introduction To Research Methodology
- Meaning and objectives of Research
- Types of Research
- Research Approaches
- Significance of Research
- Research methods vs Methodology
- Research Process
- Criteria of Good Research
- Problems faced by Researchers
- Techniques Involved in defining a problem
02 Research Design
- Meaning and Need for Research Design
- Features and important concepts relating to research design
- Different Research design
- Important Experimental Designs
03 Sample Design
- Introduction to Sample design
- Censure and sample survey
- Implications of Sample design
- Steps in sampling design
- Criteria for selecting a sampling procedure
- Characteristics of a good sample design
- Different types of Sample design
- Measurement Scales
- Important scaling Techniques
04 Methods of Data Collection
- Introduction
- Collection of Primary Data
- Collection through Questionnaire and schedule collection of secondary data
- Differences in Questionnaire and schedule
- Different methods to collect secondary data
05 Data Analysis Interpretation and Presentation Techniques
- Hypothesis Testing
- Basic concepts concerning Hypothesis Testing
- Procedure and flow diagram for Hypothesis Testing
- Test of Significance
- Chi-Square Analysis
- Report Presentation Techniques
404 Not found

An official website of the United States government
The .gov means it’s official. Federal government websites often end in .gov or .mil. Before sharing sensitive information, make sure you’re on a federal government site.
The site is secure. The https:// ensures that you are connecting to the official website and that any information you provide is encrypted and transmitted securely.
- Publications
- Account settings
Preview improvements coming to the PMC website in October 2024. Learn More or Try it out now .
- Advanced Search
- Journal List
- Am J Pharm Educ
- v.74(8); 2010 Oct 11
Presenting and Evaluating Qualitative Research
The purpose of this paper is to help authors to think about ways to present qualitative research papers in the American Journal of Pharmaceutical Education . It also discusses methods for reviewers to assess the rigour, quality, and usefulness of qualitative research. Examples of different ways to present data from interviews, observations, and focus groups are included. The paper concludes with guidance for publishing qualitative research and a checklist for authors and reviewers.
INTRODUCTION
Policy and practice decisions, including those in education, increasingly are informed by findings from qualitative as well as quantitative research. Qualitative research is useful to policymakers because it often describes the settings in which policies will be implemented. Qualitative research is also useful to both pharmacy practitioners and pharmacy academics who are involved in researching educational issues in both universities and practice and in developing teaching and learning.
Qualitative research involves the collection, analysis, and interpretation of data that are not easily reduced to numbers. These data relate to the social world and the concepts and behaviors of people within it. Qualitative research can be found in all social sciences and in the applied fields that derive from them, for example, research in health services, nursing, and pharmacy. 1 It looks at X in terms of how X varies in different circumstances rather than how big is X or how many Xs are there? 2 Textbooks often subdivide research into qualitative and quantitative approaches, furthering the common assumption that there are fundamental differences between the 2 approaches. With pharmacy educators who have been trained in the natural and clinical sciences, there is often a tendency to embrace quantitative research, perhaps due to familiarity. A growing consensus is emerging that sees both qualitative and quantitative approaches as useful to answering research questions and understanding the world. Increasingly mixed methods research is being carried out where the researcher explicitly combines the quantitative and qualitative aspects of the study. 3 , 4
Like healthcare, education involves complex human interactions that can rarely be studied or explained in simple terms. Complex educational situations demand complex understanding; thus, the scope of educational research can be extended by the use of qualitative methods. Qualitative research can sometimes provide a better understanding of the nature of educational problems and thus add to insights into teaching and learning in a number of contexts. For example, at the University of Nottingham, we conducted in-depth interviews with pharmacists to determine their perceptions of continuing professional development and who had influenced their learning. We also have used a case study approach using observation of practice and in-depth interviews to explore physiotherapists' views of influences on their leaning in practice. We have conducted in-depth interviews with a variety of stakeholders in Malawi, Africa, to explore the issues surrounding pharmacy academic capacity building. A colleague has interviewed and conducted focus groups with students to explore cultural issues as part of a joint Nottingham-Malaysia pharmacy degree program. Another colleague has interviewed pharmacists and patients regarding their expectations before and after clinic appointments and then observed pharmacist-patient communication in clinics and assessed it using the Calgary Cambridge model in order to develop recommendations for communication skills training. 5 We have also performed documentary analysis on curriculum data to compare pharmacist and nurse supplementary prescribing courses in the United Kingdom.
It is important to choose the most appropriate methods for what is being investigated. Qualitative research is not appropriate to answer every research question and researchers need to think carefully about their objectives. Do they wish to study a particular phenomenon in depth (eg, students' perceptions of studying in a different culture)? Or are they more interested in making standardized comparisons and accounting for variance (eg, examining differences in examination grades after changing the way the content of a module is taught). Clearly a quantitative approach would be more appropriate in the last example. As with any research project, a clear research objective has to be identified to know which methods should be applied.
Types of qualitative data include:
- Audio recordings and transcripts from in-depth or semi-structured interviews
- Structured interview questionnaires containing substantial open comments including a substantial number of responses to open comment items.
- Audio recordings and transcripts from focus group sessions.
- Field notes (notes taken by the researcher while in the field [setting] being studied)
- Video recordings (eg, lecture delivery, class assignments, laboratory performance)
- Case study notes
- Documents (reports, meeting minutes, e-mails)
- Diaries, video diaries
- Observation notes
- Press clippings
- Photographs
RIGOUR IN QUALITATIVE RESEARCH
Qualitative research is often criticized as biased, small scale, anecdotal, and/or lacking rigor; however, when it is carried out properly it is unbiased, in depth, valid, reliable, credible and rigorous. In qualitative research, there needs to be a way of assessing the “extent to which claims are supported by convincing evidence.” 1 Although the terms reliability and validity traditionally have been associated with quantitative research, increasingly they are being seen as important concepts in qualitative research as well. Examining the data for reliability and validity assesses both the objectivity and credibility of the research. Validity relates to the honesty and genuineness of the research data, while reliability relates to the reproducibility and stability of the data.
The validity of research findings refers to the extent to which the findings are an accurate representation of the phenomena they are intended to represent. The reliability of a study refers to the reproducibility of the findings. Validity can be substantiated by a number of techniques including triangulation use of contradictory evidence, respondent validation, and constant comparison. Triangulation is using 2 or more methods to study the same phenomenon. Contradictory evidence, often known as deviant cases, must be sought out, examined, and accounted for in the analysis to ensure that researcher bias does not interfere with or alter their perception of the data and any insights offered. Respondent validation, which is allowing participants to read through the data and analyses and provide feedback on the researchers' interpretations of their responses, provides researchers with a method of checking for inconsistencies, challenges the researchers' assumptions, and provides them with an opportunity to re-analyze their data. The use of constant comparison means that one piece of data (for example, an interview) is compared with previous data and not considered on its own, enabling researchers to treat the data as a whole rather than fragmenting it. Constant comparison also enables the researcher to identify emerging/unanticipated themes within the research project.
STRENGTHS AND LIMITATIONS OF QUALITATIVE RESEARCH
Qualitative researchers have been criticized for overusing interviews and focus groups at the expense of other methods such as ethnography, observation, documentary analysis, case studies, and conversational analysis. Qualitative research has numerous strengths when properly conducted.
Strengths of Qualitative Research
- Issues can be examined in detail and in depth.
- Interviews are not restricted to specific questions and can be guided/redirected by the researcher in real time.
- The research framework and direction can be quickly revised as new information emerges.
- The data based on human experience that is obtained is powerful and sometimes more compelling than quantitative data.
- Subtleties and complexities about the research subjects and/or topic are discovered that are often missed by more positivistic enquiries.
- Data usually are collected from a few cases or individuals so findings cannot be generalized to a larger population. Findings can however be transferable to another setting.
Limitations of Qualitative Research
- Research quality is heavily dependent on the individual skills of the researcher and more easily influenced by the researcher's personal biases and idiosyncrasies.
- Rigor is more difficult to maintain, assess, and demonstrate.
- The volume of data makes analysis and interpretation time consuming.
- It is sometimes not as well understood and accepted as quantitative research within the scientific community
- The researcher's presence during data gathering, which is often unavoidable in qualitative research, can affect the subjects' responses.
- Issues of anonymity and confidentiality can present problems when presenting findings
- Findings can be more difficult and time consuming to characterize in a visual way.
PRESENTATION OF QUALITATIVE RESEARCH FINDINGS
The following extracts are examples of how qualitative data might be presented:
Data From an Interview.
The following is an example of how to present and discuss a quote from an interview.
The researcher should select quotes that are poignant and/or most representative of the research findings. Including large portions of an interview in a research paper is not necessary and often tedious for the reader. The setting and speakers should be established in the text at the end of the quote.
The student describes how he had used deep learning in a dispensing module. He was able to draw on learning from a previous module, “I found that while using the e learning programme I was able to apply the knowledge and skills that I had gained in last year's diseases and goals of treatment module.” (interviewee 22, male)
This is an excerpt from an article on curriculum reform that used interviews 5 :
The first question was, “Without the accreditation mandate, how much of this curriculum reform would have been attempted?” According to respondents, accreditation played a significant role in prompting the broad-based curricular change, and their comments revealed a nuanced view. Most indicated that the change would likely have occurred even without the mandate from the accreditation process: “It reflects where the profession wants to be … training a professional who wants to take on more responsibility.” However, they also commented that “if it were not mandated, it could have been a very difficult road.” Or it “would have happened, but much later.” The change would more likely have been incremental, “evolutionary,” or far more limited in its scope. “Accreditation tipped the balance” was the way one person phrased it. “Nobody got serious until the accrediting body said it would no longer accredit programs that did not change.”
Data From Observations
The following example is some data taken from observation of pharmacist patient consultations using the Calgary Cambridge guide. 6 , 7 The data are first presented and a discussion follows:
Pharmacist: We will soon be starting a stop smoking clinic. Patient: Is the interview over now? Pharmacist: No this is part of it. (Laughs) You can't tell me to bog off (sic) yet. (pause) We will be starting a stop smoking service here, Patient: Yes. Pharmacist: with one-to-one and we will be able to help you or try to help you. If you want it. In this example, the pharmacist has picked up from the patient's reaction to the stop smoking clinic that she is not receptive to advice about giving up smoking at this time; in fact she would rather end the consultation. The pharmacist draws on his prior relationship with the patient and makes use of a joke to lighten the tone. He feels his message is important enough to persevere but he presents the information in a succinct and non-pressurised way. His final comment of “If you want it” is important as this makes it clear that he is not putting any pressure on the patient to take up this offer. This extract shows that some patient cues were picked up, and appropriately dealt with, but this was not the case in all examples.
Data From Focus Groups
This excerpt from a study involving 11 focus groups illustrates how findings are presented using representative quotes from focus group participants. 8
Those pharmacists who were initially familiar with CPD endorsed the model for their peers, and suggested it had made a meaningful difference in the way they viewed their own practice. In virtually all focus groups sessions, pharmacists familiar with and supportive of the CPD paradigm had worked in collaborative practice environments such as hospital pharmacy practice. For these pharmacists, the major advantage of CPD was the linking of workplace learning with continuous education. One pharmacist stated, “It's amazing how much I have to learn every day, when I work as a pharmacist. With [the learning portfolio] it helps to show how much learning we all do, every day. It's kind of satisfying to look it over and see how much you accomplish.” Within many of the learning portfolio-sharing sessions, debates emerged regarding the true value of traditional continuing education and its outcome in changing an individual's practice. While participants appreciated the opportunity for social and professional networking inherent in some forms of traditional CE, most eventually conceded that the academic value of most CE programming was limited by the lack of a systematic process for following-up and implementing new learning in the workplace. “Well it's nice to go to these [continuing education] events, but really, I don't know how useful they are. You go, you sit, you listen, but then, well I at least forget.”
The following is an extract from a focus group (conducted by the author) with first-year pharmacy students about community placements. It illustrates how focus groups provide a chance for participants to discuss issues on which they might disagree.
Interviewer: So you are saying that you would prefer health related placements? Student 1: Not exactly so long as I could be developing my communication skill. Student 2: Yes but I still think the more health related the placement is the more I'll gain from it. Student 3: I disagree because other people related skills are useful and you may learn those from taking part in a community project like building a garden. Interviewer: So would you prefer a mixture of health and non health related community placements?
GUIDANCE FOR PUBLISHING QUALITATIVE RESEARCH
Qualitative research is becoming increasingly accepted and published in pharmacy and medical journals. Some journals and publishers have guidelines for presenting qualitative research, for example, the British Medical Journal 9 and Biomedcentral . 10 Medical Education published a useful series of articles on qualitative research. 11 Some of the important issues that should be considered by authors, reviewers and editors when publishing qualitative research are discussed below.
Introduction.
A good introduction provides a brief overview of the manuscript, including the research question and a statement justifying the research question and the reasons for using qualitative research methods. This section also should provide background information, including relevant literature from pharmacy, medicine, and other health professions, as well as literature from the field of education that addresses similar issues. Any specific educational or research terminology used in the manuscript should be defined in the introduction.
The methods section should clearly state and justify why the particular method, for example, face to face semistructured interviews, was chosen. The method should be outlined and illustrated with examples such as the interview questions, focusing exercises, observation criteria, etc. The criteria for selecting the study participants should then be explained and justified. The way in which the participants were recruited and by whom also must be stated. A brief explanation/description should be included of those who were invited to participate but chose not to. It is important to consider “fair dealing,” ie, whether the research design explicitly incorporates a wide range of different perspectives so that the viewpoint of 1 group is never presented as if it represents the sole truth about any situation. The process by which ethical and or research/institutional governance approval was obtained should be described and cited.
The study sample and the research setting should be described. Sampling differs between qualitative and quantitative studies. In quantitative survey studies, it is important to select probability samples so that statistics can be used to provide generalizations to the population from which the sample was drawn. Qualitative research necessitates having a small sample because of the detailed and intensive work required for the study. So sample sizes are not calculated using mathematical rules and probability statistics are not applied. Instead qualitative researchers should describe their sample in terms of characteristics and relevance to the wider population. Purposive sampling is common in qualitative research. Particular individuals are chosen with characteristics relevant to the study who are thought will be most informative. Purposive sampling also may be used to produce maximum variation within a sample. Participants being chosen based for example, on year of study, gender, place of work, etc. Representative samples also may be used, for example, 20 students from each of 6 schools of pharmacy. Convenience samples involve the researcher choosing those who are either most accessible or most willing to take part. This may be fine for exploratory studies; however, this form of sampling may be biased and unrepresentative of the population in question. Theoretical sampling uses insights gained from previous research to inform sample selection for a new study. The method for gaining informed consent from the participants should be described, as well as how anonymity and confidentiality of subjects were guaranteed. The method of recording, eg, audio or video recording, should be noted, along with procedures used for transcribing the data.
Data Analysis.
A description of how the data were analyzed also should be included. Was computer-aided qualitative data analysis software such as NVivo (QSR International, Cambridge, MA) used? Arrival at “data saturation” or the end of data collection should then be described and justified. A good rule when considering how much information to include is that readers should have been given enough information to be able to carry out similar research themselves.
One of the strengths of qualitative research is the recognition that data must always be understood in relation to the context of their production. 1 The analytical approach taken should be described in detail and theoretically justified in light of the research question. If the analysis was repeated by more than 1 researcher to ensure reliability or trustworthiness, this should be stated and methods of resolving any disagreements clearly described. Some researchers ask participants to check the data. If this was done, it should be fully discussed in the paper.
An adequate account of how the findings were produced should be included A description of how the themes and concepts were derived from the data also should be included. Was an inductive or deductive process used? The analysis should not be limited to just those issues that the researcher thinks are important, anticipated themes, but also consider issues that participants raised, ie, emergent themes. Qualitative researchers must be open regarding the data analysis and provide evidence of their thinking, for example, were alternative explanations for the data considered and dismissed, and if so, why were they dismissed? It also is important to present outlying or negative/deviant cases that did not fit with the central interpretation.
The interpretation should usually be grounded in interviewees or respondents' contributions and may be semi-quantified, if this is possible or appropriate, for example, “Half of the respondents said …” “The majority said …” “Three said…” Readers should be presented with data that enable them to “see what the researcher is talking about.” 1 Sufficient data should be presented to allow the reader to clearly see the relationship between the data and the interpretation of the data. Qualitative data conventionally are presented by using illustrative quotes. Quotes are “raw data” and should be compiled and analyzed, not just listed. There should be an explanation of how the quotes were chosen and how they are labeled. For example, have pseudonyms been given to each respondent or are the respondents identified using codes, and if so, how? It is important for the reader to be able to see that a range of participants have contributed to the data and that not all the quotes are drawn from 1 or 2 individuals. There is a tendency for authors to overuse quotes and for papers to be dominated by a series of long quotes with little analysis or discussion. This should be avoided.
Participants do not always state the truth and may say what they think the interviewer wishes to hear. A good qualitative researcher should not only examine what people say but also consider how they structured their responses and how they talked about the subject being discussed, for example, the person's emotions, tone, nonverbal communication, etc. If the research was triangulated with other qualitative or quantitative data, this should be discussed.
Discussion.
The findings should be presented in the context of any similar previous research and or theories. A discussion of the existing literature and how this present research contributes to the area should be included. A consideration must also be made about how transferrable the research would be to other settings. Any particular strengths and limitations of the research also should be discussed. It is common practice to include some discussion within the results section of qualitative research and follow with a concluding discussion.
The author also should reflect on their own influence on the data, including a consideration of how the researcher(s) may have introduced bias to the results. The researcher should critically examine their own influence on the design and development of the research, as well as on data collection and interpretation of the data, eg, were they an experienced teacher who researched teaching methods? If so, they should discuss how this might have influenced their interpretation of the results.
Conclusion.
The conclusion should summarize the main findings from the study and emphasize what the study adds to knowledge in the area being studied. Mays and Pope suggest the researcher ask the following 3 questions to determine whether the conclusions of a qualitative study are valid 12 : How well does this analysis explain why people behave in the way they do? How comprehensible would this explanation be to a thoughtful participant in the setting? How well does the explanation cohere with what we already know?
CHECKLIST FOR QUALITATIVE PAPERS
This paper establishes criteria for judging the quality of qualitative research. It provides guidance for authors and reviewers to prepare and review qualitative research papers for the American Journal of Pharmaceutical Education . A checklist is provided in Appendix 1 to assist both authors and reviewers of qualitative data.
ACKNOWLEDGEMENTS
Thank you to the 3 reviewers whose ideas helped me to shape this paper.
Appendix 1. Checklist for authors and reviewers of qualitative research.
Introduction
- □ Research question is clearly stated.
- □ Research question is justified and related to the existing knowledge base (empirical research, theory, policy).
- □ Any specific research or educational terminology used later in manuscript is defined.
- □ The process by which ethical and or research/institutional governance approval was obtained is described and cited.
- □ Reason for choosing particular research method is stated.
- □ Criteria for selecting study participants are explained and justified.
- □ Recruitment methods are explicitly stated.
- □ Details of who chose not to participate and why are given.
- □ Study sample and research setting used are described.
- □ Method for gaining informed consent from the participants is described.
- □ Maintenance/Preservation of subject anonymity and confidentiality is described.
- □ Method of recording data (eg, audio or video recording) and procedures for transcribing data are described.
- □ Methods are outlined and examples given (eg, interview guide).
- □ Decision to stop data collection is described and justified.
- □ Data analysis and verification are described, including by whom they were performed.
- □ Methods for identifying/extrapolating themes and concepts from the data are discussed.
- □ Sufficient data are presented to allow a reader to assess whether or not the interpretation is supported by the data.
- □ Outlying or negative/deviant cases that do not fit with the central interpretation are presented.
- □ Transferability of research findings to other settings is discussed.
- □ Findings are presented in the context of any similar previous research and social theories.
- □ Discussion often is incorporated into the results in qualitative papers.
- □ A discussion of the existing literature and how this present research contributes to the area is included.
- □ Any particular strengths and limitations of the research are discussed.
- □ Reflection of the influence of the researcher(s) on the data, including a consideration of how the researcher(s) may have introduced bias to the results is included.
Conclusions
- □ The conclusion states the main finings of the study and emphasizes what the study adds to knowledge in the subject area.
Analyzing and Presenting Results from Descriptive Studies
Introduction
Disease surveillance systems and health data sources provide the raw information necessary to monitor trends in health and disease. Descriptive epidemiology provides a way of organizing and analyzing these data in order to understand variations in disease frequency geographically and over time, and how disease (or health) varies among people based on a host of personal characteristics (person, place, and time). This makes it possible to identify trends in health and disease and also provides a means of planning resources for populations. In addition, descriptive epidemiology is important for generating hypotheses (possible explanations) about the determinants of health and disease. By generating hypotheses, descriptive epidemiology also provides the starting point for analytic epidemiology, which formally tests associations between potential determinants and health or disease outcomes. Specific tasks of descriptive epidemiology are the following:
- Monitoring and reporting on the health status and health related behaviors in populations
- Identifying emerging health problems
- Alerting us to potential threats from bioterrorism
- Establishing public health priorities for a population
- Evaluating the effectiveness of intervention programs and
- Exploring potential associations between "risk factors" and health outcomes in order to generate hypotheses about the determinants of disease.
Key Questions:
How can I summarize data?
How do I produce basic figures and tables?
How can I analyze the correlation between two continuous variables?
How can I apply this to the analysis and description of an ecologic study?
How can I use R to do descriptive analyses?
Learning Objectives
After successfully completing this unit, the student will be able to:
- Identify the different classes of variables (discrete [dichotomous, categorical, ordinal], continuous, time to event)
- For continuous variables distinguish when to use mean and standard deviation versus median and interquartile range (IQR) to characterize the center and variability in data.
- Use R to compute mean, variance, standard deviation, median, and interquartile range (IQR).
- Use R to compute the correlation coefficient for an ecological study
Basic Concepts
Types of variables.
Procedures to summarize data and to perform subsequent analysis differ depending on the type of data (or variables) that are available. As a result, it is important to have a clear understanding of how variables are classified.
There are three general classifications of variables:
1) Discrete Variables: variables that assume only a finite number of values, for example, race categorized as non-Hispanic white, Hispanic, black, Asian, other. Discrete variables focus on the frequency of observations and can be presented as the number, the percentage, or the proportion of observations within a given category.
Discrete variables may be further subdivided into:
2) Continuous Variables: These are sometimes called quantitative or measurement variables; they can take on any value within a range of plausible values. For example, total serum cholesterol level, height, weight and systolic blood pressure are examples of continuous variables. Continuous variables (i.e., measurement variables) are summarized by finding a central measure, such as a mean or a median, as appropriate, and characterizing the variability of spread around the central measure .
3) Time to Event Variables: these reflect the time to a particular event such as a heart attack, cancer remission or death. This module will focus primarily on summarizing and presenting discrete variables and continuous variables; time to event variables will be addressed in a later module.
This module will introduce basic concepts for analyzing and presenting data from exploratory (descriptive) studies that are essential for disease surveillance, for assessing the health and health-related behaviors in a population, or for generating hypotheses about the determinants of health or disease. However, students may want to refer to other learning modules that address these concepts in greater detail. These can be found using the following links:
Link to module - Basic Concepts for Biostatistics
Link to module - Summarizing Data
Link to module - Data Presentation
Population Parameters versus Sample Statistics
A descriptive measure for an entire population is a ''parameter.'' There are many population parameters, for example, the population size (N) is one parameter, and the mean diastolic blood pressure or the mean body weight of a population would be other parameters that relate to continuous variables. Other population parameters focus on discrete variables, such as the percentage of current smokers in the population or the percentage of people with type 2 diabetes mellitus. Health-related behaviors can also be thought of this way, such as the percentage of the population that gets vaccinated against the flu each year or the percentage who routinely wear a seatbelt when driving.
However, it is generally not feasible to directly measure parameters, since it requires collecting information from all members of the population. We, therefore, take samples from the population, and the descriptive measures for a sample are referred to as ''sample statistics'' or simply ''statistics.'' For example, the mean diastolic blood pressure, the mean body weight, and the percentage of smokers in a sample from the population would be sample statistics. In the image below the true mean diastolic blood pressure for the population of adults in Massachusetts is 78 millimeters of mercury (mm Hg); this is a population parameter. The image also shows the mean diastolic blood pressure in three separate samples. These means are sample statistics which we might use in order to estimate the parameter for the entire population. However, note that the sample statistics are all a little bit different, and none of them are exactly the sample as the population parameter.

In order to illustrate some fundamentals, let's consider a very small sample with data shown in the table below.
Table - Data Values for a Small Sample
Note that the data table has continuous variables (age, length of stay in the hospital, body mass index) and discrete variables that are dichotomous (type 2 diabetes and current smoking). Let's focus first on the continuous variables which we will summarize by computing a central measure and an indication of how much spread there is around that central estimate.
Measures of Central Tendency and Variability
There are three sample statistics that describe the center of the data for a continuous variable. There are:
- The Mean : the average of all the values
- The Median : The "middle" value, such that half of the observations are below this value, and half are above.
- The Mode : The most frequently observed value.
The mean and the median will be most useful to us for analyzing and presenting the results of exploratory studies.
One way to summarize age for the small data set above would be to determine the frequency of subjects by age group as show in the table below.
This makes it easier to understand the age structure of the group. One could also summarize the age structure by creating a frequency histogram as shown in the figure below.

If there are no extreme or outlying values of the variable (as in this case), the mean is the most appropriate summary of a typical value.
The sample mean is computed by summing all of the values for a particular variable in the sample and dividing by the number of values in the sample.
So, the general formula is
The X with the bar over it represents the sample mean, and it is read as "X bar". The Σ indicates summation (i.e., sum of the X's or sum of the ages in this example).
Sample Variance and Standard Deviation
When the mean is appropriate to characterize the central values, the variability or spread of values around the mean can be characterized with the variance or the standard deviation. If all of the observed values in a sample are close to the sample mean, the standard deviation will be small (i.e., close to zero), and if the observed values vary widely around the sample mean, the standard deviation will be large. If all of the values in the sample are identical, the sample standard deviation will be zero.
To compute the sample standard deviation we begin by computing the variance (s 2 ) as follows:
The variance is essentially the mean of the squared deviations, although we divide by n-1 in order to avoid underestimating the population variance. We can compute this manually by first computing the deviations from the mean and then squaring them and adding the squared deviations from the mean as shown in the table below.
Table - Computation of Variance for Age
However, the more common measure of variability in a sample is the sample standard deviation (s) , defined as the square root of the sample variance:
Computing Mean, Variance, and Standard Deviation in R
These computations are easy using the R statistical package. First, I will create a data set with the ten observed ages in the example above using the concatenation function in R.
> agedata <- c(63, 74, 75, 74, 70, 72, 81, 68, 67, 77)
To calculate the mean:
> mean(agedata)
To calculate the variance:
> var(agedata)
[1] 27.65556
To calculate the standard deviation for age:
> sd(agedata)
[1] 5.258855
Next, we will examine length of stay in the hospital (days) which is also a continuous variable. As we did with age, we could summarize hospital length of stay by looking at the frequency, e.g., how many patients stayed 1, 2, 3, 4, etc. days.
And one again, we could also present the same information with a frequency histogram as shown below.

Here, most patients stayed in the hospital for only 2 or 3 days, but there were outliers who stayed 5, 7, and 9 days. This is a skewed distribution, and in this case the mean would be a misleading characterization of the central value. Rather than compute a mean, it would be more informative to compute the median value, i.e., the "middle" value, such that half of the observations are below this value, and half are above.
To compute the median one would first order the data.
- If the sample size is an odd number, the mean is the middle value.
- If the sample size is an even number, the median is the mean of the two middle values.
However, R is a more convenient way to do this, because it will also enable you to see the interquartile range (IQR) which is a useful way of characterizing the variability or spread of the data.
Computing Median and Interquartile Range with R
We can again create a small data set for hospital length of stay using the concatenation function in R:
> hospLOS <- c(2,2,2,2,3,3,3,5,7,9)
and we can then compute the median.
> median(hospLOS)
However, it is more useful to use the "summary()" command.
> summary(hospLOS)
Min. 1st Qu. Median Mean 3rd Qu. Max.
2.0 2.0 3.0 3.8 4.5 9.0
The quartiles divide the data into 4 roughly equal groups as illustrated below.

When a data set has outliers or extreme values, we summarize a typical value using the median as opposed to the mean. When a data set has outliers, variability is often summarized by a statistic called the interquartile range , which is the difference between the first and third quartiles. The first quartile, denoted Q 1 , is the value in the data set that holds 25% of the values below it. The third quartile, denoted Q 3 , is the value in the data set that holds 25% of the values above it.
To summarize:
• No outliers: sample mean and standard deviation summarize location and variability.
• When there are outliers or skewed data , median and interquartile range (IQR) best summarize location and variability, where IQR = Q3-Q1
Box-Whisker Plots
Box-whisker plots are very useful for comparing distributions. A box-whisker plot divides the observations into 4 roughly equal quartiles. The whiskers represent the minimum and maximum observed values. The right side of the box indicates Q1, below which are the lowest 25% of observations, and the left side of the box is Q3, above which are the highest 25% of observations. The lowest 25% of observations are below Q1 and the highest 25% are above Q3. The median value is shown within the box.

Data Presentation
There are two fundamental methods for presenting summary information: tables and graphs.
- Tables are generally best if you want to be able to look up specific information or if the values must be reported precisely.
- Graphics are best for illustrating trends and making comparisons
For examples of how to create effective tables and graphs and how to avoid pitfalls in data presentation, please refer to the following two online learning modules:
Case Series - Summary of Findings and Presentation
Nguyen Duc Hien, Nguyen Hong Ha, et al: Human infection with highly pathogenic avian influenza virus (H5N1) in Northern Vietnam, 2004–2005 . Emerg Infect Dis. 2009 Jan; 15(1): 19–23.
Link to the complete article
This is a small, but important case series reported in 2009. Shown below are the abstract and slightly modified versions of the two tables presented in the report.
Note that both continuous and discrete variables are reported, and note that the authors used the mean and standard deviation for variables like age, but they used median and IQR for many other variables because their distributions were skewed. Note also that discrete variables and continuous variables can be presented in the same table, but it is essential to specify how each characteristic is being presented.
Table 1. Characteristics of 29 patients infected with highly pathogenic avian influenza virus (H5N1), northern Vietnam, 2004–2005*
Table Legend: *IQR, interquartile range;
†Poultry, a history of exposure to sick or healthy poultry; sick poultry or person, a history of exposure to sick poultry or a family infected with avian influenza (H5N1).
Table 2 below shows selected laboratory findings among survivors versus patients who died. Leukocytes are white blood cells, and neutrophils are a specific type of white blood cell; the lower numbers of these two counts in those who died suggests that the immune system was overwhelmed. Hemoglobin is a measure of red blood cells and oxygen carrying capacity. Platelets are essential elements for blood clotting. Albumin is the most abundant protein in blood. AST is an abbreviation for aspartate aminotransferase, an enzyme that is abundant in the liver; high levels of AST in the blood frequently indicate liver damage. Urea nitrogen is a measure of kidney function; high levels of urea nitrogen suggest compromised kidney function but could also be indicative of dehydration.
Table 2. Initial laboratory results for 29 patients infected with highly pathogenic avian influenza virus
†p<0.05, by Wilcoxon test or Fisher exact test.
We will address p-values and statistical tests like the Wilcoxon test and the Fisher exact test in subsequent modules.
A Cross-Sectional Survey
In 2002 John Snow, Inc. (JSI) worked with the town of Weymouth, Massachusetts to identify unmet health needs in the town and devise a plan to prioritize unmet health needs and key risk factors that may be modified through lifestyle changes. The project conducted a mail survey of a random sample of 5,000 households as well as a survey of all 3,400 Weymouth public school students in grades nine through twelve. The information assisted the Town's decision-making about priorities for improving services and designing interventions that may prevent or reduce the incidence of ill health. Below you will find links to PDF versions of the full surveys and a link to a subset of the data and a key for identifying the variables and the coded responses.
Link to the Adult Survey Questionnaire
Link to a subset of the data from the Adult Survey
Link to description of the variable names and codes for the adult survey data
link to the Student Survey Questionnaire
Open the link to the Adult Survey Questionnaire and scan through it to get an idea of how a carefully constructed survey tool looks. Note the efforts to make the question explicit and clear.

Ecologic Studies
In ecologic studies the unit of observation for the exposure of interest is the average level of exposure in different populations or groups, and the outcome of interest is the overall frequency of disease for those populations or groups. In this regard, ecologic studies are different from all other epidemiologic studies, for which the unit of observation is exposure status and outcome status for individual people. As a result, ecologic studies need to be interpreted with caution. Nevertheless, they can be informative, and this module will focus on their analysis, interpretation, and presentation using correlation and simple linear regression.
Computing the Correlation Coefficient
The module on Descriptive Studies showed an ecologic study correlating per capita meat consumption and incidence of colon cancer in women from 22 countries. Investigators used commerce data to compute the overall consumption of meat by various nations. They then calculated the average (per capita) meat consumption per person by dividing total national meat consumption by the number of people in a given country. There is a clear linear trend; countries with the lowest meat consumption have the lowest rates of colon cancer, and the colon cancer rate among these countries progressively increases as meat consumption increases.

Note that in reality, people's meat consumption probably varied widely within nations, and the exposure that was calculated was an average that assumes that everyone ate the average amount of meat. This average exposure was then correlated with the overall disease frequency in each country. The example here suggests that the frequency of colon cancer increases as meat consumption increases.
How can we analyze and present this type of information?
As noted in the module on Descriptive Studies, ecologic studies invite us to assess the association between the the independent variable (in this case, per capita meat consumption) and the dependent variable (in this case, the outcome, incidence of colon cancer in women) by computing the correlation coefficient ("r"). This section will provide a brief outline of correlation analysis and demonstrate how to use the R statistical package to compute correlation coefficients. Correlation analysis and simple linear regression are described in a later module for this course.
Link to module on Correlation and Linear Regression.
The most commonly used type of correlation is Pearson correlation, named after Karl Pearson, introduced this statistic around the turn of the 20 th century. Pearson's r measures the linear relationship between two variables, say X and Y . A correlation of 1 indicates the data points perfectly lie on a line for which Y increases as X increases. A value of -1 also implies the data points lie on a line; however, Y decreases as X increases. The formula for r is:
where Cov(x,y) is the covariance of x and y defined as
The variances of x and y measure the variability of the x scores and y scores around their respective sample means of X and Y considered separately. The covariance measures the variability of the (x,y) pairs around the mean of x and mean of y, considered simultaneously.
We can combine all of this into the following equation:
In the "Other Resources" listed to the left of this page there is a link to a data file called "Meat-CancerEcologic.csv" which has three columns: Country, Grams (per capita meat consumption), and Incidence (Incidence of colon cancer per 100,000 women).
If I import this data set into the R Studio, I can compute the correlations coefficient and then plot the points using the following commands. First, I created a data frame called "meat,":and then I computed the correlation coefficient.
> meat <- Meat-CancerEcologic
> attach(meat)
> cor (grams, incidence)
[1] 0.9005721
The correlation coefficient of 0.9005721 indicates a strong positive correlation between national per capita meat consumption and national incidence of colon cancer in women.
Next, I created a scatter plot of the data.
> plot(grams, incidence, col="red", pch =24)

Visual inspection of the plot suggests a linear relationship with a strong positive correlation, and the correlation coefficient r=0.90 confirms this.
Download the data set and try it yourself.
Brief Comments About Data Presentation
In order to be useful, the data must be organized and analyzed in a thoughtful, structured way, and the results must be be communicated in a clear, effective way to both the public health workforce and the community at large. Some simple standards are useful to promote clear presentation. Compiled data are commonly summarized in tables, graphs, or some combination.
Simple guidelines for tables.
- Provide a concise descriptive title.
- Label the rows and columns.
- Provide the units in the column headers.
- Provide the column total, if appropriate.
- If necessary, additional explanatory information may be provided in a footnoted legend immediately beneath the title.
Table - Treatment with Anti-hypertensive Medication in Men and Women
Simple guidelines for figures:
- Include a concise descriptive title.
- Label the axes clearly showing units where appropriate.
- Use appropriate scales for the vertical and horizontal axes that display the results without exaggerating them with ranges that are either too expansive or too restrictive.
- For line graphs with multiple groups include a simple legend if necessary.
Figure - Relative Frequency of Anti-hypertensive Medication Use in Men and Women

Additional resources for summarizing and presenting data:
- Online learning module on "Data Presentation." (Link to Data Presentation module)
- Online learning module on "Summarizing Data". (Link the Summarizing Data module)
- The CDC also provides another good resource for advice about organizing data. (Link to CDC page on organizing data.)
Answer to Question on Page 3 Regarding Confidence Interval for the Body Mass Index
The Framingham Heart Study reported that in a sample of 3,326 subjects the mean body mass index was 28.15, and the standard deviation was 5.32. What was the 95% confidence interval for the population's mean body mass index?
So the 95% confidence interval is
Interpretation:
Our estimate of the mean BMI in the population is 28.15. With 95% confidence the true mean is likely to be between 27.97 and 28.33.
Answer to 95% Confidence Interval for the Case-Fatality Rate from Bird Flu - page 3
The point estimate is
There are 7 persons who died and 22 who did not, so we can use the following formula:
Substituting:
So, the 95% confidence interval is 0.085, 0.391.
Our best estimate of the case-fatality rate from bird flu is 24%. With 95% confidence the true case-fatality rate is likely to be between 8.5% to 39.1%.
Note that this 95% confidence interval is quite broad because of the small sample size (n=29).
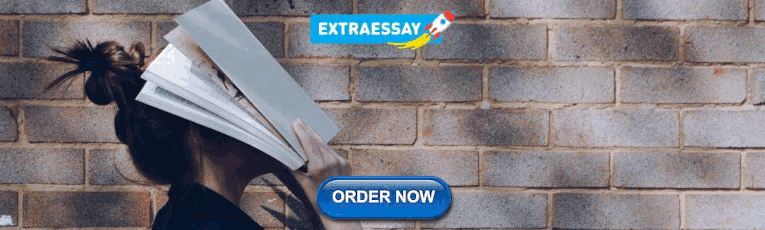
IMAGES
VIDEO
COMMENTS
A proper data presentation includes the interpretation of that data, the reason why it's included, and why it matters to your research. ... In the histogram data analysis presentation example, imagine an instructor analyzing a class's grades to identify the most common score range. A histogram could effectively display the distribution.
Here's my five-step routine to make and deliver your data presentation right where it is intended —. 1. Understand Your Data & Make It Seen. Data slides aren't really about data; they're about the meaning of that data. As data professionals, everyone approaches data differently.
Data interpretation is a crucial aspect of data analysis and enables organizations to turn large amounts of data into actionable insights. The guide covered the definition, importance, types, methods, benefits, process, analysis, tools, use cases, and best practices of data interpretation. As technology continues to advance, the methods and ...
TheJoelTruth. While a good presentation has data, data alone doesn't guarantee a good presentation. It's all about how that data is presented. The quickest way to confuse your audience is by ...
1. Step one: Defining the question. The first step in any data analysis process is to define your objective. In data analytics jargon, this is sometimes called the 'problem statement'. Defining your objective means coming up with a hypothesis and figuring how to test it.
2. Brand Analysis Dashboard. Next, in our list of data interpretation examples, we have a template that shows the answers to a survey on awareness for Brand D. The sample size is listed on top to get a perspective of the data, which is represented using interactive charts and graphs. **click to enlarge**.
Another example of data analysis is the use of recruitment CRM by businesses. They utilize it to find candidates, track their progress, and manage their entire hiring process to determine how they can better automate their workflow. Conclusion. Overall, data interpretation is an essential factor in data-driven decision-making.
What data analysis entails. Data analysis is an analytical process which involves recording and tabulating (recording and entering, entering and tabulating) the quantities of a product, such as numbers of units produced, costs of materials and expenses. While data analyst can take different forms, for example in databases, in other structures ...
Key Objectives of Data Presentation. Here are some key objectives to think about when presenting financial analysis: Visual communication. Audience and context. Charts, graphs, and images. Focus on important points. Design principles. Storytelling. Persuasiveness.
Presentation length. This is my formula to determine how many slides to include in my main presentation assuming I spend about five minutes per slide. (Presentation length in minutes-10 minutes for questions ) / 5 minutes per slide. For an hour presentation that comes out to ( 60-10 ) / 5 = 10 slides.
There are 4 modules in this course. This course focuses on the analysis and interpretation of data. The focus will be placed on data preparation and description and quantitative and qualitative data analysis. The course commences with a discussion of data preparation, scale internal consistency, appropriate data analysis and the Pearson ...
DATA PRESENTATION, ANALYSIS AND INTERPRETATION. 4.0 Introduction. This chapter is concerned with data pres entation, of the findings obtained through the study. The. findings are presented in ...
Abstract. This chapter covers the topics of data collection, data presentation and data analysis. It gives attention to data collection for studies based on experiments, on data derived from existing published or unpublished data sets, on observation, on simulation and digital twins, on surveys, on interviews and on focus group discussions.
This lesson discusses the meaning of data analysis, presentation, interpretation and discussion of findings. Using example of a research title, the lesson wi...
analysis. begins with initial reactions or observations. identify patterns. calculating values. data cleansing: check for errors. interpretation. parallel with analysis. results interpreted different ways. make sure data supports conclusion. avoid biases. avoid over claiming. presentation. different methods, depends on goals. affects ...
In conclusion, data analysis, interpretation, and presentation are crucial aspects of conducting high-quality research. By using the appropriate data analysis, interpretation, and presentation techniques, researchers can derive meaningful insights, make sense of the insights, and communicate the research findings effectively.
To complete this study properly, it is necessary to analyse the data collected in order to test the hypothesis and answer the research questions. As already indicated in the preceding chapter, data is interpreted in a descriptive form. This chapter comprises the analysis, presentation and interpretation of the findings resulting from this study.
"Data analysis is the process of bringing order, structure and meaning to the mass of collected data. It is a messy, ambiguous, time-consuming, creative, and fascinating process. It does not proceed in a linear fashion; it is not neat. Qualitative data analysis is a search for general statements about relationships among categories of data."
Data examination involves processing plus analyzing the information for derive meaningful insights, while data interpretation involves making sense of the insights and drawing conclusions. Data presentation include presenting the data in a clear and concise way to compose the investigation findings.
The purpose of this paper is to help authors to think about ways to present qualitative research papers in the American Journal of Pharmaceutical Education. It also discusses methods for reviewers to assess the rigour, quality, and usefulness of qualitative research. Examples of different ways to present data from interviews, observations, and ...
Descriptive epidemiology provides a way of organizing and analyzing these data in order to understand variations in disease frequency geographically and over time, and how disease (or health) varies among people based on a host of personal characteristics (person, place, and time). This makes it possible to identify trends in health and disease ...
This video consists of very useful tips in writing chapter 4, the presentation, analysis and interpretation of data. Happy research writing guys! ♥️♥️♥️May...
Creation of Chapter 4 or Presentation, Interpretation and Analysis of Data