
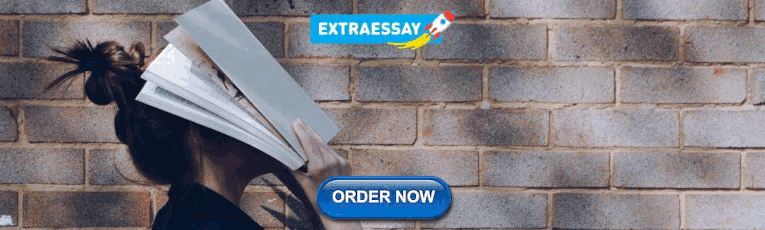
The “no first-person” myth
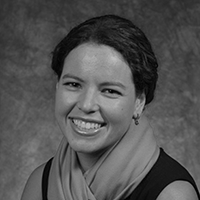
- First-Person Pronouns
- Research and Publication
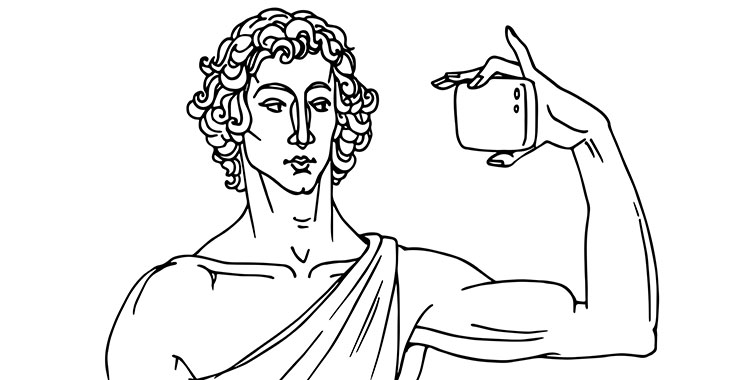
In this series, we look at common APA Style misconceptions and debunk these myths one by one.
Many writers believe the “no first-person” myth, which is that writers cannot use first-person pronouns such as “I” or “we” in an APA Style paper. This myth implies that writers must instead refer to themselves in the third person (e.g., as “the author” or “the authors”). However, APA Style has no such rule against using first-person pronouns and actually encourages their use to avoid ambiguity in attribution!
When expressing your own views or the views of yourself and fellow authors, use the pronouns “I” or “we” and the like . Similarly, when writing your paper, use first-person pronouns when describing work you did by yourself or work you and your fellow authors did together when conducting your research. For example, use “we interviewed participants” rather than “the authors interviewed participants.” When writing an APA Style paper by yourself, use the first-person pronoun “I” to refer to yourself. And use the pronoun “we” when writing an APA Style paper with others. Here are some phrases you might use in your paper:
“I think…” “I believe…” “I interviewed the participants…” “I analyzed the data…” “My analysis of the data revealed…” “We concluded…” “Our results showed…”
This guidance can be found in Section 4.16 of the Publication Manual of the American Psychological Association, Seventh Edition and in Section 2.16 of the Concise Guide to APA Style, Seventh Edition . It represents a continuation of a long-standing APA Style guideline that began with the second edition of the manual, in 1974.
Keep in mind that you should avoid using the editorial “we” to refer to people in general so that it is clear to readers to whom you are referring. Instead, use more specific nouns such as “people” or “researchers.”
As always, defer to your instructors’ guidelines when writing student papers. For example, your instructor may ask students to avoid using first-person language. If so, follow that guideline for work in your class.
Now that we’ve debunked another myth, go forth APA Style writers, using the first-person when appropriate!
What myth should we debunk next? Leave a comment below.
Related and recent
Comments are disabled due to your privacy settings. To re-enable, please adjust your cookie preferences.
APA Style Monthly
Subscribe to the APA Style Monthly newsletter to get tips, updates, and resources delivered directly to your inbox.
Welcome! Thank you for subscribing.
APA Style Guidelines
Browse APA Style writing guidelines by category
- Abbreviations
- Bias-Free Language
- Capitalization
- In-Text Citations
- Italics and Quotation Marks
- Paper Format
- Punctuation
- Spelling and Hyphenation
- Tables and Figures
Full index of topics
- PRO Courses Guides New Tech Help Pro Expert Videos About wikiHow Pro Upgrade Sign In
- EDIT Edit this Article
- EXPLORE Tech Help Pro About Us Random Article Quizzes Request a New Article Community Dashboard This Or That Game Popular Categories Arts and Entertainment Artwork Books Movies Computers and Electronics Computers Phone Skills Technology Hacks Health Men's Health Mental Health Women's Health Relationships Dating Love Relationship Issues Hobbies and Crafts Crafts Drawing Games Education & Communication Communication Skills Personal Development Studying Personal Care and Style Fashion Hair Care Personal Hygiene Youth Personal Care School Stuff Dating All Categories Arts and Entertainment Finance and Business Home and Garden Relationship Quizzes Cars & Other Vehicles Food and Entertaining Personal Care and Style Sports and Fitness Computers and Electronics Health Pets and Animals Travel Education & Communication Hobbies and Crafts Philosophy and Religion Work World Family Life Holidays and Traditions Relationships Youth
- Browse Articles
- Learn Something New
- Quizzes Hot
- This Or That Game
- Train Your Brain
- Explore More
- Support wikiHow
- About wikiHow
- Log in / Sign up
- Education and Communications
- Science Writing
How to Write a Hypothesis
Last Updated: May 2, 2023 Fact Checked
This article was co-authored by Bess Ruff, MA . Bess Ruff is a Geography PhD student at Florida State University. She received her MA in Environmental Science and Management from the University of California, Santa Barbara in 2016. She has conducted survey work for marine spatial planning projects in the Caribbean and provided research support as a graduate fellow for the Sustainable Fisheries Group. There are 9 references cited in this article, which can be found at the bottom of the page. This article has been fact-checked, ensuring the accuracy of any cited facts and confirming the authority of its sources. This article has been viewed 1,033,636 times.
A hypothesis is a description of a pattern in nature or an explanation about some real-world phenomenon that can be tested through observation and experimentation. The most common way a hypothesis is used in scientific research is as a tentative, testable, and falsifiable statement that explains some observed phenomenon in nature. [1] X Research source Many academic fields, from the physical sciences to the life sciences to the social sciences, use hypothesis testing as a means of testing ideas to learn about the world and advance scientific knowledge. Whether you are a beginning scholar or a beginning student taking a class in a science subject, understanding what hypotheses are and being able to generate hypotheses and predictions yourself is very important. These instructions will help get you started.
Preparing to Write a Hypothesis

- If you are writing a hypothesis for a school assignment, this step may be taken care of for you.

- Focus on academic and scholarly writing. You need to be certain that your information is unbiased, accurate, and comprehensive. Scholarly search databases such as Google Scholar and Web of Science can help you find relevant articles from reputable sources.
- You can find information in textbooks, at a library, and online. If you are in school, you can also ask for help from teachers, librarians, and your peers.

- For example, if you are interested in the effects of caffeine on the human body, but notice that nobody seems to have explored whether caffeine affects males differently than it does females, this could be something to formulate a hypothesis about. Or, if you are interested in organic farming, you might notice that no one has tested whether organic fertilizer results in different growth rates for plants than non-organic fertilizer.
- You can sometimes find holes in the existing literature by looking for statements like “it is unknown” in scientific papers or places where information is clearly missing. You might also find a claim in the literature that seems far-fetched, unlikely, or too good to be true, like that caffeine improves math skills. If the claim is testable, you could provide a great service to scientific knowledge by doing your own investigation. If you confirm the claim, the claim becomes even more credible. If you do not find support for the claim, you are helping with the necessary self-correcting aspect of science.
- Examining these types of questions provides an excellent way for you to set yourself apart by filling in important gaps in a field of study.

- Following the examples above, you might ask: "How does caffeine affect females as compared to males?" or "How does organic fertilizer affect plant growth compared to non-organic fertilizer?" The rest of your research will be aimed at answering these questions.

- Following the examples above, if you discover in the literature that there is a pattern that some other types of stimulants seem to affect females more than males, this could be a clue that the same pattern might be true for caffeine. Similarly, if you observe the pattern that organic fertilizer seems to be associated with smaller plants overall, you might explain this pattern with the hypothesis that plants exposed to organic fertilizer grow more slowly than plants exposed to non-organic fertilizer.
Formulating Your Hypothesis

- You can think of the independent variable as the one that is causing some kind of difference or effect to occur. In the examples, the independent variable would be biological sex, i.e. whether a person is male or female, and fertilizer type, i.e. whether the fertilizer is organic or non-organically-based.
- The dependent variable is what is affected by (i.e. "depends" on) the independent variable. In the examples above, the dependent variable would be the measured impact of caffeine or fertilizer.
- Your hypothesis should only suggest one relationship. Most importantly, it should only have one independent variable. If you have more than one, you won't be able to determine which one is actually the source of any effects you might observe.

- Don't worry too much at this point about being precise or detailed.
- In the examples above, one hypothesis would make a statement about whether a person's biological sex might impact the way the person is affected by caffeine; for example, at this point, your hypothesis might simply be: "a person's biological sex is related to how caffeine affects his or her heart rate." The other hypothesis would make a general statement about plant growth and fertilizer; for example your simple explanatory hypothesis might be "plants given different types of fertilizer are different sizes because they grow at different rates."

- Using our example, our non-directional hypotheses would be "there is a relationship between a person's biological sex and how much caffeine increases the person's heart rate," and "there is a relationship between fertilizer type and the speed at which plants grow."
- Directional predictions using the same example hypotheses above would be : "Females will experience a greater increase in heart rate after consuming caffeine than will males," and "plants fertilized with non-organic fertilizer will grow faster than those fertilized with organic fertilizer." Indeed, these predictions and the hypotheses that allow for them are very different kinds of statements. More on this distinction below.
- If the literature provides any basis for making a directional prediction, it is better to do so, because it provides more information. Especially in the physical sciences, non-directional predictions are often seen as inadequate.

- Where necessary, specify the population (i.e. the people or things) about which you hope to uncover new knowledge. For example, if you were only interested the effects of caffeine on elderly people, your prediction might read: "Females over the age of 65 will experience a greater increase in heart rate than will males of the same age." If you were interested only in how fertilizer affects tomato plants, your prediction might read: "Tomato plants treated with non-organic fertilizer will grow faster in the first three months than will tomato plants treated with organic fertilizer."

- For example, you would not want to make the hypothesis: "red is the prettiest color." This statement is an opinion and it cannot be tested with an experiment. However, proposing the generalizing hypothesis that red is the most popular color is testable with a simple random survey. If you do indeed confirm that red is the most popular color, your next step may be to ask: Why is red the most popular color? The answer you propose is your explanatory hypothesis .

- An easy way to get to the hypothesis for this method and prediction is to ask yourself why you think heart rates will increase if children are given caffeine. Your explanatory hypothesis in this case may be that caffeine is a stimulant. At this point, some scientists write a research hypothesis , a statement that includes the hypothesis, the experiment, and the prediction all in one statement.
- For example, If caffeine is a stimulant, and some children are given a drink with caffeine while others are given a drink without caffeine, then the heart rates of those children given a caffeinated drink will increase more than the heart rate of children given a non-caffeinated drink.

- Using the above example, if you were to test the effects of caffeine on the heart rates of children, evidence that your hypothesis is not true, sometimes called the null hypothesis , could occur if the heart rates of both the children given the caffeinated drink and the children given the non-caffeinated drink (called the placebo control) did not change, or lowered or raised with the same magnitude, if there was no difference between the two groups of children.
- It is important to note here that the null hypothesis actually becomes much more useful when researchers test the significance of their results with statistics. When statistics are used on the results of an experiment, a researcher is testing the idea of the null statistical hypothesis. For example, that there is no relationship between two variables or that there is no difference between two groups. [8] X Research source

Hypothesis Examples

Community Q&A

- Remember that science is not necessarily a linear process and can be approached in various ways. [10] X Research source Thanks Helpful 0 Not Helpful 0
- When examining the literature, look for research that is similar to what you want to do, and try to build on the findings of other researchers. But also look for claims that you think are suspicious, and test them yourself. Thanks Helpful 0 Not Helpful 0
- Be specific in your hypotheses, but not so specific that your hypothesis can't be applied to anything outside your specific experiment. You definitely want to be clear about the population about which you are interested in drawing conclusions, but nobody (except your roommates) will be interested in reading a paper with the prediction: "my three roommates will each be able to do a different amount of pushups." Thanks Helpful 0 Not Helpful 0

You Might Also Like

- ↑ https://undsci.berkeley.edu/for-educators/prepare-and-plan/correcting-misconceptions/#a4
- ↑ https://owl.purdue.edu/owl/general_writing/common_writing_assignments/research_papers/choosing_a_topic.html
- ↑ https://owl.purdue.edu/owl/subject_specific_writing/writing_in_the_social_sciences/writing_in_psychology_experimental_report_writing/experimental_reports_1.html
- ↑ https://www.grammarly.com/blog/how-to-write-a-hypothesis/
- ↑ https://grammar.yourdictionary.com/for-students-and-parents/how-create-hypothesis.html
- ↑ https://flexbooks.ck12.org/cbook/ck-12-middle-school-physical-science-flexbook-2.0/section/1.19/primary/lesson/hypothesis-ms-ps/
- ↑ https://iastate.pressbooks.pub/preparingtopublish/chapter/goal-1-contextualize-the-studys-methods/
- ↑ http://mathworld.wolfram.com/NullHypothesis.html
- ↑ http://undsci.berkeley.edu/article/scienceflowchart
About This Article

Before writing a hypothesis, think of what questions are still unanswered about a specific subject and make an educated guess about what the answer could be. Then, determine the variables in your question and write a simple statement about how they might be related. Try to focus on specific predictions and variables, such as age or segment of the population, to make your hypothesis easier to test. For tips on how to test your hypothesis, read on! Did this summary help you? Yes No
- Send fan mail to authors
Reader Success Stories

Onyia Maxwell
Sep 13, 2016
Did this article help you?

Nov 26, 2017

ABEL SHEWADEG
Jun 12, 2018

Connor Gilligan
Jan 2, 2017

Dec 30, 2017

Featured Articles

Trending Articles

Watch Articles

- Terms of Use
- Privacy Policy
- Do Not Sell or Share My Info
- Not Selling Info
Don’t miss out! Sign up for
wikiHow’s newsletter
Learn How To Write A Hypothesis For Your Next Research Project!

Undoubtedly, research plays a crucial role in substantiating or refuting our assumptions. These assumptions act as potential answers to our questions. Such assumptions, also known as hypotheses, are considered key aspects of research. In this blog, we delve into the significance of hypotheses. And provide insights on how to write them effectively. So, let’s dive in and explore the art of writing hypotheses together.
Table of Contents
What is a Hypothesis?
A hypothesis is a crucial starting point in scientific research. It is an educated guess about the relationship between two or more variables. In other words, a hypothesis acts as a foundation for a researcher to build their study.
Here are some examples of well-crafted hypotheses:
- Increased exposure to natural sunlight improves sleep quality in adults.
A positive relationship between natural sunlight exposure and sleep quality in adult individuals.
- Playing puzzle games on a regular basis enhances problem-solving abilities in children.
Engaging in frequent puzzle gameplay leads to improved problem-solving skills in children.
- Students and improved learning hecks.
S tudents using online paper writing service platforms (as a learning tool for receiving personalized feedback and guidance) will demonstrate improved writing skills. (compared to those who do not utilize such platforms).
- The use of APA format in research papers.
Using the APA format helps students stay organized when writing research papers. Organized students can focus better on their topics and, as a result, produce better quality work.
The Building Blocks of a Hypothesis
To better understand the concept of a hypothesis, let’s break it down into its basic components:
- Variables . A hypothesis involves at least two variables. An independent variable and a dependent variable. The independent variable is the one being changed or manipulated, while the dependent variable is the one being measured or observed.
- Relationship : A hypothesis proposes a relationship or connection between the variables. This could be a cause-and-effect relationship or a correlation between them.
- Testability : A hypothesis should be testable and falsifiable, meaning it can be proven right or wrong through experimentation or observation.
Types of Hypotheses
When learning how to write a hypothesis, it’s essential to understand its main types. These include; alternative hypotheses and null hypotheses. In the following section, we explore both types of hypotheses with examples.
Alternative Hypothesis (H1)
This kind of hypothesis suggests a relationship or effect between the variables. It is the main focus of the study. The researcher wants to either prove or disprove it. Many research divides this hypothesis into two subsections:
- Directional
This type of H1 predicts a specific outcome. Many researchers use this hypothesis to explore the relationship between variables rather than the groups.
- Non-directional
You can take a guess from the name. This type of H1 does not provide a specific prediction for the research outcome.
Here are some examples for your better understanding of how to write a hypothesis.
- Consuming caffeine improves cognitive performance. (This hypothesis predicts that there is a positive relationship between caffeine consumption and cognitive performance.)
- Aerobic exercise leads to reduced blood pressure. (This hypothesis suggests that engaging in aerobic exercise results in lower blood pressure readings.)
- Exposure to nature reduces stress levels among employees. (Here, the hypothesis proposes that employees exposed to natural environments will experience decreased stress levels.)
- Listening to classical music while studying increases memory retention. (This hypothesis speculates that studying with classical music playing in the background boosts students’ ability to retain information.)
- Early literacy intervention improves reading skills in children. (This hypothesis claims that providing early literacy assistance to children results in enhanced reading abilities.)
- Time management in nursing students. ( Students who use a nursing research paper writing service have more time to focus on their studies and can achieve better grades in other subjects. )
Null Hypothesis (H0)
A null hypothesis assumes no relationship or effect between the variables. If the alternative hypothesis is proven to be false, the null hypothesis is considered to be true. Usually a null hypothesis shows no direct correlation between the defined variables.
Here are some of the examples
- The consumption of herbal tea has no effect on sleep quality. (This hypothesis assumes that herbal tea consumption does not impact the quality of sleep.)
- The number of hours spent playing video games is unrelated to academic performance. (Here, the null hypothesis suggests that no relationship exists between video gameplay duration and academic achievement.)
- Implementing flexible work schedules has no influence on employee job satisfaction. (This hypothesis contends that providing flexible schedules does not affect how satisfied employees are with their jobs.)
- Writing ability of a 7th grader is not affected by reading editorial example. ( There is no relationship between reading an editorial example and improving a 7th grader’s writing abilities.)
- The type of lighting in a room does not affect people’s mood. (In this null hypothesis, there is no connection between the kind of lighting in a room and the mood of those present.)
- The use of social media during break time does not impact productivity at work. (This hypothesis proposes that social media usage during breaks has no effect on work productivity.)
As you learn how to write a hypothesis, remember that aiming for clarity, testability, and relevance to your research question is vital. By mastering this skill, you’re well on your way to conducting impactful scientific research. Good luck!
Importance of a Hypothesis in Research
A well-structured hypothesis is a vital part of any research project for several reasons:
- It provides clear direction for the study by setting its focus and purpose.
- It outlines expectations of the research, making it easier to measure results.
- It helps identify any potential limitations in the study, allowing researchers to refine their approach.
In conclusion, a hypothesis plays a fundamental role in the research process. By understanding its concept and constructing a well-thought-out hypothesis, researchers lay the groundwork for a successful, scientifically sound investigation.
How to Write a Hypothesis?
Here are five steps that you can follow to write an effective hypothesis.
Step 1: Identify Your Research Question
The first step in learning how to compose a hypothesis is to clearly define your research question. This question is the central focus of your study and will help you determine the direction of your hypothesis.
Step 2: Determine the Variables
When exploring how to write a hypothesis, it’s crucial to identify the variables involved in your study. You’ll need at least two variables:
- Independent variable : The factor you manipulate or change in your experiment.
- Dependent variable : The outcome or result you observe or measure, which is influenced by the independent variable.
Step 3: Build the Hypothetical Relationship
In understanding how to compose a hypothesis, constructing the relationship between the variables is key. Based on your research question and variables, predict the expected outcome or connection. This prediction should be specific, testable, and, if possible, expressed in the “If…then” format.
Step 4: Write the Null Hypothesis
When mastering how to write a hypothesis, it’s important to create a null hypothesis as well. The null hypothesis assumes no relationship or effect between the variables, acting as a counterpoint to your primary hypothesis.
Step 5: Review Your Hypothesis
Finally, when learning how to compose a hypothesis, it’s essential to review your hypothesis for clarity, testability, and relevance to your research question. Make any necessary adjustments to ensure it provides a solid basis for your study.
In conclusion, understanding how to write a hypothesis is crucial for conducting successful scientific research. By focusing on your research question and carefully building relationships between variables, you will lay a strong foundation for advancing research and knowledge in your field.
Hypothesis vs. Prediction: What’s the Difference?
Understanding the differences between a hypothesis and a prediction is crucial in scientific research. Often, these terms are used interchangeably, but they have distinct meanings and functions. This segment aims to clarify these differences and explain how to compose a hypothesis correctly, helping you improve the quality of your research projects.
Hypothesis: The Foundation of Your Research
A hypothesis is an educated guess about the relationship between two or more variables. It provides the basis for your research question and is a starting point for an experiment or observational study.
The critical elements for a hypothesis include:
- Specificity: A clear and concise statement that describes the relationship between variables.
- Testability: The ability to test the hypothesis through experimentation or observation.
To learn how to write a hypothesis, it’s essential to identify your research question first and then predict the relationship between the variables.
Prediction: The Expected Outcome
A prediction is a statement about a specific outcome you expect to see in your experiment or observational study. It’s derived from the hypothesis and provides a measurable way to test the relationship between variables.
Here’s an example of how to write a hypothesis and a related prediction:
- Hypothesis: Consuming a high-sugar diet leads to weight gain.
- Prediction: People who consume a high-sugar diet for six weeks will gain more weight than those who maintain a low-sugar diet during the same period.
Key Differences Between a Hypothesis and a Prediction
While a hypothesis and prediction are both essential components of scientific research, there are some key differences to keep in mind:
- A hypothesis is an educated guess that suggests a relationship between variables, while a prediction is a specific and measurable outcome based on that hypothesis.
- A hypothesis can give rise to multiple experiment or observational study predictions.
To conclude, understanding the differences between a hypothesis and a prediction, and learning how to write a hypothesis, are essential steps to form a robust foundation for your research. By creating clear, testable hypotheses along with specific, measurable predictions, you lay the groundwork for scientifically sound investigations.
Here’s a wrap-up for this guide on how to write a hypothesis. We’re confident this article was helpful for many of you. We understand that many students struggle with writing their school research . However, we hope to continue assisting you through our blog tutorial on writing different aspects of academic assignments.
For further information, you can check out our reverent blog or contact our professionals to avail amazing writing services. Paper perk experts tailor assignments to reflect your unique voice and perspectives. Our professionals make sure to stick around till your satisfaction. So what are you waiting for? Pick your required service and order away!
How to write a good hypothesis?
How to write a hypothesis in science, how to write a research hypothesis, how to write a null hypothesis, what is the format for a scientific hypothesis, how do you structure a proper hypothesis, can you provide an example of a hypothesis, what is the ideal hypothesis structure.
The ideal hypothesis structure includes the following;
- A clear statement of the relationship between variables.
- testable prediction.
- falsifiability.
If your hypothesis has all of these, it is both scientifically sound and effective.
How to write a hypothesis for product management?
Writing a hypothesis for product management involves a simple process:
- First, identify the problem or question you want to address.
- State your assumption or belief about the solution to that problem. .
- Make a hypothesis by predicting a specific outcome based on your assumption.
- Make sure your hypothesis is specific, measurable, and testable.
- Use experiments, data analysis, or user feedback to validate your hypothesis.
- Make informed decisions for product improvement.
Following these steps will help you in effectively formulating hypotheses for product management.
Order Original Papers & Essays
Your First Custom Paper Sample is on Us!

Timely Deliveries

No Plagiarism & AI

100% Refund
Try Our Free Paper Writing Service
Related blogs.

Connections with Writers and support
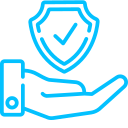
Privacy and Confidentiality Guarantee
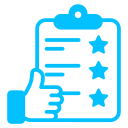
Average Quality Score
Have a language expert improve your writing
Run a free plagiarism check in 10 minutes, generate accurate citations for free.
- Knowledge Base
Hypothesis Testing | A Step-by-Step Guide with Easy Examples
Published on November 8, 2019 by Rebecca Bevans . Revised on June 22, 2023.
Hypothesis testing is a formal procedure for investigating our ideas about the world using statistics . It is most often used by scientists to test specific predictions, called hypotheses, that arise from theories.
There are 5 main steps in hypothesis testing:
- State your research hypothesis as a null hypothesis and alternate hypothesis (H o ) and (H a or H 1 ).
- Collect data in a way designed to test the hypothesis.
- Perform an appropriate statistical test .
- Decide whether to reject or fail to reject your null hypothesis.
- Present the findings in your results and discussion section.
Though the specific details might vary, the procedure you will use when testing a hypothesis will always follow some version of these steps.
Table of contents
Step 1: state your null and alternate hypothesis, step 2: collect data, step 3: perform a statistical test, step 4: decide whether to reject or fail to reject your null hypothesis, step 5: present your findings, other interesting articles, frequently asked questions about hypothesis testing.
After developing your initial research hypothesis (the prediction that you want to investigate), it is important to restate it as a null (H o ) and alternate (H a ) hypothesis so that you can test it mathematically.
The alternate hypothesis is usually your initial hypothesis that predicts a relationship between variables. The null hypothesis is a prediction of no relationship between the variables you are interested in.
- H 0 : Men are, on average, not taller than women. H a : Men are, on average, taller than women.
Here's why students love Scribbr's proofreading services
Discover proofreading & editing
For a statistical test to be valid , it is important to perform sampling and collect data in a way that is designed to test your hypothesis. If your data are not representative, then you cannot make statistical inferences about the population you are interested in.
There are a variety of statistical tests available, but they are all based on the comparison of within-group variance (how spread out the data is within a category) versus between-group variance (how different the categories are from one another).
If the between-group variance is large enough that there is little or no overlap between groups, then your statistical test will reflect that by showing a low p -value . This means it is unlikely that the differences between these groups came about by chance.
Alternatively, if there is high within-group variance and low between-group variance, then your statistical test will reflect that with a high p -value. This means it is likely that any difference you measure between groups is due to chance.
Your choice of statistical test will be based on the type of variables and the level of measurement of your collected data .
- an estimate of the difference in average height between the two groups.
- a p -value showing how likely you are to see this difference if the null hypothesis of no difference is true.
Based on the outcome of your statistical test, you will have to decide whether to reject or fail to reject your null hypothesis.
In most cases you will use the p -value generated by your statistical test to guide your decision. And in most cases, your predetermined level of significance for rejecting the null hypothesis will be 0.05 – that is, when there is a less than 5% chance that you would see these results if the null hypothesis were true.
In some cases, researchers choose a more conservative level of significance, such as 0.01 (1%). This minimizes the risk of incorrectly rejecting the null hypothesis ( Type I error ).
Prevent plagiarism. Run a free check.
The results of hypothesis testing will be presented in the results and discussion sections of your research paper , dissertation or thesis .
In the results section you should give a brief summary of the data and a summary of the results of your statistical test (for example, the estimated difference between group means and associated p -value). In the discussion , you can discuss whether your initial hypothesis was supported by your results or not.
In the formal language of hypothesis testing, we talk about rejecting or failing to reject the null hypothesis. You will probably be asked to do this in your statistics assignments.
However, when presenting research results in academic papers we rarely talk this way. Instead, we go back to our alternate hypothesis (in this case, the hypothesis that men are on average taller than women) and state whether the result of our test did or did not support the alternate hypothesis.
If your null hypothesis was rejected, this result is interpreted as “supported the alternate hypothesis.”
These are superficial differences; you can see that they mean the same thing.
You might notice that we don’t say that we reject or fail to reject the alternate hypothesis . This is because hypothesis testing is not designed to prove or disprove anything. It is only designed to test whether a pattern we measure could have arisen spuriously, or by chance.
If we reject the null hypothesis based on our research (i.e., we find that it is unlikely that the pattern arose by chance), then we can say our test lends support to our hypothesis . But if the pattern does not pass our decision rule, meaning that it could have arisen by chance, then we say the test is inconsistent with our hypothesis .
If you want to know more about statistics , methodology , or research bias , make sure to check out some of our other articles with explanations and examples.
- Normal distribution
- Descriptive statistics
- Measures of central tendency
- Correlation coefficient
Methodology
- Cluster sampling
- Stratified sampling
- Types of interviews
- Cohort study
- Thematic analysis
Research bias
- Implicit bias
- Cognitive bias
- Survivorship bias
- Availability heuristic
- Nonresponse bias
- Regression to the mean
Hypothesis testing is a formal procedure for investigating our ideas about the world using statistics. It is used by scientists to test specific predictions, called hypotheses , by calculating how likely it is that a pattern or relationship between variables could have arisen by chance.
A hypothesis states your predictions about what your research will find. It is a tentative answer to your research question that has not yet been tested. For some research projects, you might have to write several hypotheses that address different aspects of your research question.
A hypothesis is not just a guess — it should be based on existing theories and knowledge. It also has to be testable, which means you can support or refute it through scientific research methods (such as experiments, observations and statistical analysis of data).
Null and alternative hypotheses are used in statistical hypothesis testing . The null hypothesis of a test always predicts no effect or no relationship between variables, while the alternative hypothesis states your research prediction of an effect or relationship.
Cite this Scribbr article
If you want to cite this source, you can copy and paste the citation or click the “Cite this Scribbr article” button to automatically add the citation to our free Citation Generator.
Bevans, R. (2023, June 22). Hypothesis Testing | A Step-by-Step Guide with Easy Examples. Scribbr. Retrieved June 24, 2024, from https://www.scribbr.com/statistics/hypothesis-testing/
Is this article helpful?
Rebecca Bevans
Other students also liked, choosing the right statistical test | types & examples, understanding p values | definition and examples, what is your plagiarism score.
Have a language expert improve your writing
Run a free plagiarism check in 10 minutes, automatically generate references for free.
- Knowledge Base
- Methodology
- How to Write a Strong Hypothesis | Guide & Examples
How to Write a Strong Hypothesis | Guide & Examples
Published on 6 May 2022 by Shona McCombes .
A hypothesis is a statement that can be tested by scientific research. If you want to test a relationship between two or more variables, you need to write hypotheses before you start your experiment or data collection.
Table of contents
What is a hypothesis, developing a hypothesis (with example), hypothesis examples, frequently asked questions about writing hypotheses.
A hypothesis states your predictions about what your research will find. It is a tentative answer to your research question that has not yet been tested. For some research projects, you might have to write several hypotheses that address different aspects of your research question.
A hypothesis is not just a guess – it should be based on existing theories and knowledge. It also has to be testable, which means you can support or refute it through scientific research methods (such as experiments, observations, and statistical analysis of data).
Variables in hypotheses
Hypotheses propose a relationship between two or more variables . An independent variable is something the researcher changes or controls. A dependent variable is something the researcher observes and measures.
In this example, the independent variable is exposure to the sun – the assumed cause . The dependent variable is the level of happiness – the assumed effect .
Prevent plagiarism, run a free check.
Step 1: ask a question.
Writing a hypothesis begins with a research question that you want to answer. The question should be focused, specific, and researchable within the constraints of your project.
Step 2: Do some preliminary research
Your initial answer to the question should be based on what is already known about the topic. Look for theories and previous studies to help you form educated assumptions about what your research will find.
At this stage, you might construct a conceptual framework to identify which variables you will study and what you think the relationships are between them. Sometimes, you’ll have to operationalise more complex constructs.
Step 3: Formulate your hypothesis
Now you should have some idea of what you expect to find. Write your initial answer to the question in a clear, concise sentence.
Step 4: Refine your hypothesis
You need to make sure your hypothesis is specific and testable. There are various ways of phrasing a hypothesis, but all the terms you use should have clear definitions, and the hypothesis should contain:
- The relevant variables
- The specific group being studied
- The predicted outcome of the experiment or analysis
Step 5: Phrase your hypothesis in three ways
To identify the variables, you can write a simple prediction in if … then form. The first part of the sentence states the independent variable and the second part states the dependent variable.
In academic research, hypotheses are more commonly phrased in terms of correlations or effects, where you directly state the predicted relationship between variables.
If you are comparing two groups, the hypothesis can state what difference you expect to find between them.
Step 6. Write a null hypothesis
If your research involves statistical hypothesis testing , you will also have to write a null hypothesis. The null hypothesis is the default position that there is no association between the variables. The null hypothesis is written as H 0 , while the alternative hypothesis is H 1 or H a .
Research question | Hypothesis | Null hypothesis |
---|---|---|
What are the health benefits of eating an apple a day? | Increasing apple consumption in over-60s will result in decreasing frequency of doctor’s visits. | Increasing apple consumption in over-60s will have no effect on frequency of doctor’s visits. |
Which airlines have the most delays? | Low-cost airlines are more likely to have delays than premium airlines. | Low-cost and premium airlines are equally likely to have delays. |
Can flexible work arrangements improve job satisfaction? | Employees who have flexible working hours will report greater job satisfaction than employees who work fixed hours. | There is no relationship between working hour flexibility and job satisfaction. |
How effective is secondary school sex education at reducing teen pregnancies? | Teenagers who received sex education lessons throughout secondary school will have lower rates of unplanned pregnancy than teenagers who did not receive any sex education. | Secondary school sex education has no effect on teen pregnancy rates. |
What effect does daily use of social media have on the attention span of under-16s? | There is a negative correlation between time spent on social media and attention span in under-16s. | There is no relationship between social media use and attention span in under-16s. |
Hypothesis testing is a formal procedure for investigating our ideas about the world using statistics. It is used by scientists to test specific predictions, called hypotheses , by calculating how likely it is that a pattern or relationship between variables could have arisen by chance.
A hypothesis is not just a guess. It should be based on existing theories and knowledge. It also has to be testable, which means you can support or refute it through scientific research methods (such as experiments, observations, and statistical analysis of data).
A research hypothesis is your proposed answer to your research question. The research hypothesis usually includes an explanation (‘ x affects y because …’).
A statistical hypothesis, on the other hand, is a mathematical statement about a population parameter. Statistical hypotheses always come in pairs: the null and alternative hypotheses. In a well-designed study , the statistical hypotheses correspond logically to the research hypothesis.
Cite this Scribbr article
If you want to cite this source, you can copy and paste the citation or click the ‘Cite this Scribbr article’ button to automatically add the citation to our free Reference Generator.
McCombes, S. (2022, May 06). How to Write a Strong Hypothesis | Guide & Examples. Scribbr. Retrieved 24 June 2024, from https://www.scribbr.co.uk/research-methods/hypothesis-writing/
Is this article helpful?
Shona McCombes
Other students also liked, operationalisation | a guide with examples, pros & cons, what is a conceptual framework | tips & examples, a quick guide to experimental design | 5 steps & examples.

How to Develop a Good Research Hypothesis
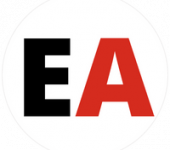
The story of a research study begins by asking a question. Researchers all around the globe are asking curious questions and formulating research hypothesis. However, whether the research study provides an effective conclusion depends on how well one develops a good research hypothesis. Research hypothesis examples could help researchers get an idea as to how to write a good research hypothesis.
This blog will help you understand what is a research hypothesis, its characteristics and, how to formulate a research hypothesis
Table of Contents
What is Hypothesis?
Hypothesis is an assumption or an idea proposed for the sake of argument so that it can be tested. It is a precise, testable statement of what the researchers predict will be outcome of the study. Hypothesis usually involves proposing a relationship between two variables: the independent variable (what the researchers change) and the dependent variable (what the research measures).
What is a Research Hypothesis?
Research hypothesis is a statement that introduces a research question and proposes an expected result. It is an integral part of the scientific method that forms the basis of scientific experiments. Therefore, you need to be careful and thorough when building your research hypothesis. A minor flaw in the construction of your hypothesis could have an adverse effect on your experiment. In research, there is a convention that the hypothesis is written in two forms, the null hypothesis, and the alternative hypothesis (called the experimental hypothesis when the method of investigation is an experiment).
Characteristics of a Good Research Hypothesis
As the hypothesis is specific, there is a testable prediction about what you expect to happen in a study. You may consider drawing hypothesis from previously published research based on the theory.
A good research hypothesis involves more effort than just a guess. In particular, your hypothesis may begin with a question that could be further explored through background research.
To help you formulate a promising research hypothesis, you should ask yourself the following questions:
- Is the language clear and focused?
- What is the relationship between your hypothesis and your research topic?
- Is your hypothesis testable? If yes, then how?
- What are the possible explanations that you might want to explore?
- Does your hypothesis include both an independent and dependent variable?
- Can you manipulate your variables without hampering the ethical standards?
- Does your research predict the relationship and outcome?
- Is your research simple and concise (avoids wordiness)?
- Is it clear with no ambiguity or assumptions about the readers’ knowledge
- Is your research observable and testable results?
- Is it relevant and specific to the research question or problem?
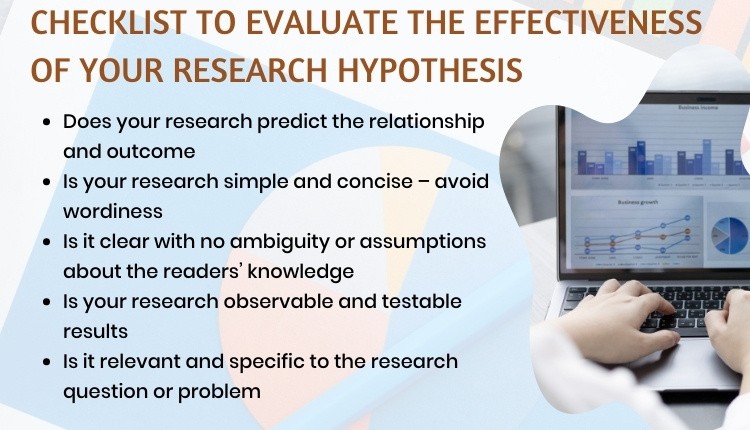
The questions listed above can be used as a checklist to make sure your hypothesis is based on a solid foundation. Furthermore, it can help you identify weaknesses in your hypothesis and revise it if necessary.
Source: Educational Hub
How to formulate a research hypothesis.
A testable hypothesis is not a simple statement. It is rather an intricate statement that needs to offer a clear introduction to a scientific experiment, its intentions, and the possible outcomes. However, there are some important things to consider when building a compelling hypothesis.
1. State the problem that you are trying to solve.
Make sure that the hypothesis clearly defines the topic and the focus of the experiment.
2. Try to write the hypothesis as an if-then statement.
Follow this template: If a specific action is taken, then a certain outcome is expected.
3. Define the variables
Independent variables are the ones that are manipulated, controlled, or changed. Independent variables are isolated from other factors of the study.
Dependent variables , as the name suggests are dependent on other factors of the study. They are influenced by the change in independent variable.
4. Scrutinize the hypothesis
Evaluate assumptions, predictions, and evidence rigorously to refine your understanding.
Types of Research Hypothesis
The types of research hypothesis are stated below:
1. Simple Hypothesis
It predicts the relationship between a single dependent variable and a single independent variable.
2. Complex Hypothesis
It predicts the relationship between two or more independent and dependent variables.
3. Directional Hypothesis
It specifies the expected direction to be followed to determine the relationship between variables and is derived from theory. Furthermore, it implies the researcher’s intellectual commitment to a particular outcome.
4. Non-directional Hypothesis
It does not predict the exact direction or nature of the relationship between the two variables. The non-directional hypothesis is used when there is no theory involved or when findings contradict previous research.
5. Associative and Causal Hypothesis
The associative hypothesis defines interdependency between variables. A change in one variable results in the change of the other variable. On the other hand, the causal hypothesis proposes an effect on the dependent due to manipulation of the independent variable.
6. Null Hypothesis
Null hypothesis states a negative statement to support the researcher’s findings that there is no relationship between two variables. There will be no changes in the dependent variable due the manipulation of the independent variable. Furthermore, it states results are due to chance and are not significant in terms of supporting the idea being investigated.
7. Alternative Hypothesis
It states that there is a relationship between the two variables of the study and that the results are significant to the research topic. An experimental hypothesis predicts what changes will take place in the dependent variable when the independent variable is manipulated. Also, it states that the results are not due to chance and that they are significant in terms of supporting the theory being investigated.
Research Hypothesis Examples of Independent and Dependent Variables
Research Hypothesis Example 1 The greater number of coal plants in a region (independent variable) increases water pollution (dependent variable). If you change the independent variable (building more coal factories), it will change the dependent variable (amount of water pollution).
Research Hypothesis Example 2 What is the effect of diet or regular soda (independent variable) on blood sugar levels (dependent variable)? If you change the independent variable (the type of soda you consume), it will change the dependent variable (blood sugar levels)
You should not ignore the importance of the above steps. The validity of your experiment and its results rely on a robust testable hypothesis. Developing a strong testable hypothesis has few advantages, it compels us to think intensely and specifically about the outcomes of a study. Consequently, it enables us to understand the implication of the question and the different variables involved in the study. Furthermore, it helps us to make precise predictions based on prior research. Hence, forming a hypothesis would be of great value to the research. Here are some good examples of testable hypotheses.
More importantly, you need to build a robust testable research hypothesis for your scientific experiments. A testable hypothesis is a hypothesis that can be proved or disproved as a result of experimentation.
Importance of a Testable Hypothesis
To devise and perform an experiment using scientific method, you need to make sure that your hypothesis is testable. To be considered testable, some essential criteria must be met:
- There must be a possibility to prove that the hypothesis is true.
- There must be a possibility to prove that the hypothesis is false.
- The results of the hypothesis must be reproducible.
Without these criteria, the hypothesis and the results will be vague. As a result, the experiment will not prove or disprove anything significant.
What are your experiences with building hypotheses for scientific experiments? What challenges did you face? How did you overcome these challenges? Please share your thoughts with us in the comments section.
Frequently Asked Questions
The steps to write a research hypothesis are: 1. Stating the problem: Ensure that the hypothesis defines the research problem 2. Writing a hypothesis as an 'if-then' statement: Include the action and the expected outcome of your study by following a ‘if-then’ structure. 3. Defining the variables: Define the variables as Dependent or Independent based on their dependency to other factors. 4. Scrutinizing the hypothesis: Identify the type of your hypothesis
Hypothesis testing is a statistical tool which is used to make inferences about a population data to draw conclusions for a particular hypothesis.
Hypothesis in statistics is a formal statement about the nature of a population within a structured framework of a statistical model. It is used to test an existing hypothesis by studying a population.
Research hypothesis is a statement that introduces a research question and proposes an expected result. It forms the basis of scientific experiments.
The different types of hypothesis in research are: • Null hypothesis: Null hypothesis is a negative statement to support the researcher’s findings that there is no relationship between two variables. • Alternate hypothesis: Alternate hypothesis predicts the relationship between the two variables of the study. • Directional hypothesis: Directional hypothesis specifies the expected direction to be followed to determine the relationship between variables. • Non-directional hypothesis: Non-directional hypothesis does not predict the exact direction or nature of the relationship between the two variables. • Simple hypothesis: Simple hypothesis predicts the relationship between a single dependent variable and a single independent variable. • Complex hypothesis: Complex hypothesis predicts the relationship between two or more independent and dependent variables. • Associative and casual hypothesis: Associative and casual hypothesis predicts the relationship between two or more independent and dependent variables. • Empirical hypothesis: Empirical hypothesis can be tested via experiments and observation. • Statistical hypothesis: A statistical hypothesis utilizes statistical models to draw conclusions about broader populations.

Wow! You really simplified your explanation that even dummies would find it easy to comprehend. Thank you so much.
Thanks a lot for your valuable guidance.
I enjoy reading the post. Hypotheses are actually an intrinsic part in a study. It bridges the research question and the methodology of the study.
Useful piece!
This is awesome.Wow.
It very interesting to read the topic, can you guide me any specific example of hypothesis process establish throw the Demand and supply of the specific product in market
Nicely explained
It is really a useful for me Kindly give some examples of hypothesis
It was a well explained content ,can you please give me an example with the null and alternative hypothesis illustrated
clear and concise. thanks.
So Good so Amazing
Good to learn
Thanks a lot for explaining to my level of understanding
Explained well and in simple terms. Quick read! Thank you
It awesome. It has really positioned me in my research project
Rate this article Cancel Reply
Your email address will not be published.
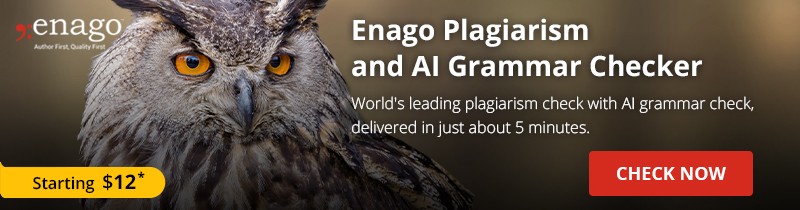
Enago Academy's Most Popular Articles
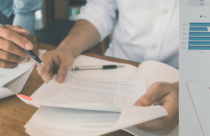
- Reporting Research
Choosing the Right Analytical Approach: Thematic analysis vs. content analysis for data interpretation
In research, choosing the right approach to understand data is crucial for deriving meaningful insights.…
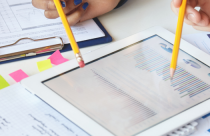
Comparing Cross Sectional and Longitudinal Studies: 5 steps for choosing the right approach
The process of choosing the right research design can put ourselves at the crossroads of…
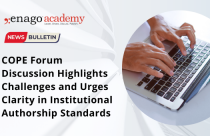
- Industry News
COPE Forum Discussion Highlights Challenges and Urges Clarity in Institutional Authorship Standards
The COPE forum discussion held in December 2023 initiated with a fundamental question — is…
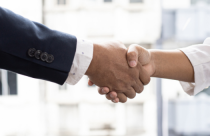
- Career Corner
Unlocking the Power of Networking in Academic Conferences
Embarking on your first academic conference experience? Fear not, we got you covered! Academic conferences…
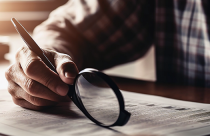
Research Recommendations – Guiding policy-makers for evidence-based decision making
Research recommendations play a crucial role in guiding scholars and researchers toward fruitful avenues of…
Choosing the Right Analytical Approach: Thematic analysis vs. content analysis for…
Comparing Cross Sectional and Longitudinal Studies: 5 steps for choosing the right…
How to Design Effective Research Questionnaires for Robust Findings

Sign-up to read more
Subscribe for free to get unrestricted access to all our resources on research writing and academic publishing including:
- 2000+ blog articles
- 50+ Webinars
- 10+ Expert podcasts
- 50+ Infographics
- 10+ Checklists
- Research Guides
We hate spam too. We promise to protect your privacy and never spam you.
I am looking for Editing/ Proofreading services for my manuscript Tentative date of next journal submission:
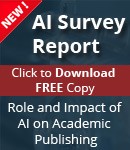
What would be most effective in reducing research misconduct?
Monday, July 1, 2024
- Are first-person pronouns acceptable in scientific writing?
February 23, 2011 Filed under Blog , Featured , Popular , Writing
Interestingly, this rule seems to have originated with Francis Bacon to give scientific writing more objectivity.
In Eloquent Science (pp. 76-77), I advocate that first-person pronouns are acceptable in limited contexts. Avoid their use in rote descriptions of your methodology (“We performed the assay…”). Instead, use them to communicate that an action or a decision that you performed affects the outcome of the research.
NO FIRST-PERSON PRONOUN: Given option A and option B, the authors chose option B to more accurately depict the location of the front. FIRST-PERSON PRONOUN: Given option A and option B, we chose option B to more accurately depict the location of the front.
So, what do other authors think? I have over 30 books on scientific writing and have read numerous articles on this point. Here are some quotes from those who expressed their opinion on this matter and I was able to find from the index of the book or through a quick scan of the book.
“Because of this [avoiding first-person pronouns], the scientist commonly uses verbose (and imprecise) statements such as “It was found that” in preference to the short, unambiguous “I found.” Young scientists should renounce the false modesty of their predecessors. Do not be afraid to name the agent of the action in a sentence, even when it is “I” or “we.”” — How to Write and Publish a Scientific Paper by Day and Gastel, pp. 193-194 “Who is the universal ‘it’, the one who hides so bashfully, but does much thinking and assuming? “ It is thought that … is a meaningless phrase and unnecessary exercise in modesty. The reader wants to know who did the thinking or assuming, the author, or some other expert.” — The Science Editor’s Soapbox by Lipton, p. 43 “I pulled 40 journals at random from one of my university’s technical library’s shelves…. To my surprise, in 32 out of the 40 journals, the authors indeed made liberal use of “I” and “we.” — Style for Students by Joe Schall, p. 63 “Einstein occasionally used the first person. He was not only a great scientist, but a great scientific writer. Feynman also used the first person on occasion, as did Curie, Darwin, Lyell, and Freud. As long as the emphasis remains on your work and not you, there is nothing wrong with judicious use of the first person.” — The Craft of Scientific Writing by Michael Alley, p. 107 “One of the most epochal papers in all of 20th-century science, Watson and Crick’s article defies nearly every major rule you are likely to find in manuals on scientific writing…. There is the frequent use of “we”…. This provides an immediate human presence, allowing for constant use of active voice. It also gives the impression that the authors are telling us their actual thought processes.” — The Chicago Guide to Communicating Science by Scott L. Montgomery, p. 18 “We believe in the value of a long tradition (which some deplore) arguing that it is inappropriate for the author of a scientific document to refer to himself or herself directly, in the first person…. There is no place for the subjectivity implicit in personal intrusion on the part of the one who conducted the research—especially since the section is explicitly labeled “Results”…. If first-person pronouns are appropriate anywhere in a dissertation, it would be in the Discussion section…because different people might indeed draw different inferences from a given set of facts.” — The Art of Scientific Writing by Ebel et al., p. 79. [After arguing for two pages on clearly explaining why the first person should not be used…] “The first person singular is appropriate when the personal element is strong, for example, when taking a position in a controversy. But this tends to weaken the writer’s credibility. The writer usually wants to make clear that anyone considering the same evidence would take the same position. Using the third person helps to express the logical impersonal character and generality of an author’s position, whereas the first person makes it seem more like personal opinion.” — The Scientist’s Handbook for Writing Papers and Dissertations by Antoinette Wilkinson, p. 76.
So, I can find only one source on my bookshelf advocating against use of the first-person pronouns in all situations (Wilkinson). Even the Ebel et al. quote I largely agree with.
Thus, first-person pronouns in scientific writing are acceptable if used in a limited fashion and to enhance clarity.
Isn’t it telling that Ebel et al begin their argument against usage of the first person with the phrase ““We believe …”?
That is a reall good point, Kirk. Thanks for pointing that out!
This argument is approximately correct, but in my opinion off point. The use of first person should always be minimized in scientific writing, but not because it is unacceptable or even uncommon. It should be minimized because it is ineffective, and it is usually badly so. Specifically, the purpose of scientific writing is to create a convincing argument based on data collected during the evaluation of a hypothesis. This is basic scientific method. The strength of this argument depends on the data, not on the person who collected it. Using first person deemphasises the data, which weakens the argument and opens the door for subjective criticism to be used to rebut what should be objective data. For example, suppose I hypothesized that the sun always rises in the east, and I make daily observations over the course of a year to support that hypothesis. I could say, “I have shown that the sun always rises in the east”. A critic might respond by simply saying that I am crazy, and that I got it wrong. In other words, it can easily become an argument about “me”. However, if I said “Daily observations over the course of a year showed that the sun always rises in the east”, then any subsequent argument must rebut the data and not rebut “me”. Actually, I would never say this using either of those formulations. I would say, “Daily observations over the course of a year were consistent with the hypothesis that the sun always rises in the east.” This is basic scientific expository writing.
Finally, if one of my students EVER wrote “it was found that …”, I would hit him or her over the head with a very large stick. That is just as bad as “I found that …”, and importantly, those are NOT the only two options. The correct way to say this in scientific writing is, “the data showed that …”.
In general, I agree with you. We should omit ourselves from our science to emphasize what the data demonstrate.
My only qualification is that, as scientists, the collection, observation, and interpretation of data is difficult to disconnect from its human aspects. Being a human endeavor, science is necessarily affected by the humans themselves who do the work.
Thanks for your comment!
i could not understand why 1st person I is used with plural verb
Not sure that I completely understand your question, but grammatically “I” should only be used with a singular verb. If you use “I” in scientific writing, only do so with single-authored papers.
Does that answer your question?
why do we use ‘have’ with ‘i’ pronoun?
I wouldn’t view it as “I” goes with the plural verb “have”, but that “have” can be used with a number of different persons, regardless of whether it is singular or plural.
First person singular: “I have” Second person singular: “You have” Third person singular: “He/She/It has”
First person plural: “We have” Second person plural: “All of you have” Third person plural: “They have”
I know it perhaps doesn’t make sense, but that is the way English works.
I hope that helps.
I think there are a few cases where personal pronouns would be acceptable. If you are introducing a new section in a thesis or even an article, you might want to say “we begin with a description of the data in section 2” etc, rather than the cumbersome “this paper will begin with …”. Also in discussions of future work, it would make sense to say “we intend to explore X, Y and Z”.
I loved reading this, my Prof. and I were debating about this. He wants me to say “I analyzed” and I want to say “problem notification database analysed revealed that…”
I’m writing a paper for a conference. I wonder if I can defy a Professor in Korea:)
I disagree that writing in the 3rd person makes writing more objective. I also disagree that it “opens the door for subjective criticism to be used to rebut what should be objective data”. In fact, using the 3rd person obstructs reality. There are people behind the research who both make mistakes and do great things. It is no less true for science than it is for other subjects that 3rd person obstructs the author of an action and makes the idea being conveyed less clear. I find it odd that scientific writing guides instruct authors to BOTH use active voice AND use only the 3rd person. It is impossible to do both. Active voice means that there is a subject, a strong verb (not a version of the verb “to be”) and an object. When I say “The solution was mixed”, it is BOTH 3rd person and passive voice. The only way to construct that sentence without passive voice is to say “We mixed the solution”. Honestly, after spending most of the first part of my life in English classes and then transitioning to science, I find most scientific writing an abomonination.
Hi Kathleen,
I think it is great that you have had your feet in both English and science. For many of us who have struggled as writers, those people are great role models to aspire to.
An anecdote: my wife’s research student turned in a brief report on his work to date. She was showing me how well written his work was, really pretty advanced for an undergrad physics student. Later, she found out that he was trying to decide between majoring in physics and majoring in English.
Hi David. Thanks very much for your tips. Very interesting article. Did you just tweet that you should keep “I” and “We” out of the abstract? I am translating a psychology article from Spanish into English, and I’ve come up against an unwieldly sentence (the very last one in the abstract) that basically wants to say “We propose a number of strategies for improving the impact of the psychological treatments[…]” Would you say it’s a no-no? I tend to avoid personal pronouns in academic articles as much as poss, but it just sounds like the most natural option in this case. Perhaps I could put, “This article proposes a number of treatments…”? Strictly speaking it’s not the article that’s doing the proposing, obviously. I’d be very grateful to have your opinion. Thanks a lot. Best regards. Louisa
Yes, it’s difficult. How about going passive? “A number of treatments are proposed….”?
The comments against using first person, which are rampant in science education, are silly. Go read Nature or Science. I believe Kathleen makes a fantastic point.
Just happened across this blog while searching for something else, and procrastination rules, ok?
My pet hate is lecturers who uncritically criticise students for using the third person. Close behind is institutional guidance/insistence on third person ‘scientific writing’. Both are hugely ironic, the first because it is typically uncritical and purely traditional (we are employed to teach others to be critical and challenge tradition), the second because there is so little empirical evidence to suggest that the scientific method is third person.
I very much appreciated Bill Lott’s response because a) it was critical and b) it discussed the issue of good and bad writing as opposed to first and third person. However I would still suggest that the way he would report his exemplar data is all but first person:
“Daily observations over the course of a year were consistent with the hypothesis that the sun always rises in the east.”
Who did the observations if not the first person? All that is missing is My or Our at the beginning of the sentence and hey presto
Another facet of writing is that it disappears if not frequently watered and tended to.
Even though this is an old article, I’d like to add my 2c to the thread.
I think the use of the 3rd person is pompous, verbose and obtuse – it uses many words to say the same thing in a flowery way.
“It is the opinion of the author that” as opposed to “I think that”
Anybody reading the article knows that it’s written by a person / persons who did the research on the topic, who are either presenting their findings or opinion. The whole 3rd person thing seems to be a game, and I for one, HATE writing about myself in the 3rd person.
That being said, it seems to be the convention that the 3rd person is used, and I probably will write my paper in the 3rd person anyway, just to not rock the boat.
But I wish that the pomposity would stop and we would get more advocates for writing in plain English.
Hi, i was wondering… can “We” be said in a scientific school report?
Depends on the context, I guess. I would follow the same advice as above.
Thanks for all the tips. Don’t forget that in the future historians are going to want to know who did what and when. Scientists may not think it important, but historians will (especially if it is a significant contribution). Furthermore, by not revealing particulars regarding individual contributions opens the door for many scientists to falsify the historical record in their favor (I have experienced this first hand in a recent publication).
i think it is soo weird to use first person in reports…….third persons will be more effective when used and that will give a clear explanations to the audience
Even Nature journals are encouraging “we” in the manuscript.
“Nature journals prefer authors to write in the active voice (“we performed the experiment…”) as experience has shown that readers find concepts and results to be conveyed more clearly if written directly.”
https://www.nature.com/authors/author_resources/how_write.html
[…] Is trouwens iets dat blijkbaar al lang voor discussies zorgt, als je deze links bekijkt: Are first-person pronouns acceptable in scientific writing? : eloquentscience.com Use of the word "I" in scientific papers Zelfs wikipedia heeft er een artikel over: […]
[…] There was some discussion on Twitter about whether or not to write in the 1st person. The Lab & Field pointed out that Francis Bacon may have been responsible for the movement to avoid it in scientific writing… […]
[…] ¿Son aceptables los pronombres en primera persona en publicaciones científicas? [ENG] […]
[…] do discuss this among themselves. For example, see Yateendra Joshi and Professor David M. Schultz. Professor Schultz notes that the use of the first person in science appears to be as common among […]
[…] http://eloquentscience.com/2011/02/are-first-person-pronouns-acceptable-in-scientific-writing/ […]
[…] There’s no rule about the passive voice in science. People seem to think that it’s “scientific” writing, but it isn’t. It’s just bad writing. There’s actually no rule against first person pronouns either! Read this for more on the use of the first-person in scientific writing. […]
To order, visit:
The American Meteorological Society (preferred)
The University of Chicago Press
eNews & Updates
Sign up to receive breaking news as well as receive other site updates!
David M. Schultz is a Professor of Synoptic Meteorology at the Centre for Atmospheric Science, Department of Earth and Environmental Sciences, and the Centre for Crisis Studies and Mitigation, The University of Manchester. He served as Chief Editor for Monthly Weather Review from 2008 to 2022. In 2014 and 2017, he received the University of Manchester Teaching Excellence Award, the only academic to have twice done so. He has published over 190 peer-reviewed journal articles. [Read more]
- Search for:
Latest Tweets
currently unavailable
Recent Posts
- Top 40 potential questions to be asked in a PhD viva or defense
- Free Writing and Publishing Online Workshop: 19–20 June 2024
- Editorial: How to Be a More Effective Author
- How to be a more effective reviewer
- The Five Most Common Problems with Introductions
- Eliminate excessive and unnecessary acronyms from your scientific writing
- Chinese translation of Eloquent Science now available
- How Bill Paxton Helped Us Understand Tornadoes in Europe
- Publishing Academic Papers Workshop
- Past or Present Tense?
- Rejected for publication: What now?
- Thermodynamic diagrams for free
- Do you end with a ‘thank you’ or ‘questions?’ slide?
- Presentations
Copyright © 2024 EloquentScience.com · All Rights Reserved · StudioPress theme customized by Insojourn Design · Log in
- Bipolar Disorder
- Therapy Center
- When To See a Therapist
- Types of Therapy
- Best Online Therapy
- Best Couples Therapy
- Best Family Therapy
- Managing Stress
- Sleep and Dreaming
- Understanding Emotions
- Self-Improvement
- Healthy Relationships
- Student Resources
- Personality Types
- Guided Meditations
- Verywell Mind Insights
- 2024 Verywell Mind 25
- Mental Health in the Classroom
- Editorial Process
- Meet Our Review Board
- Crisis Support
How to Write a Great Hypothesis
Hypothesis Definition, Format, Examples, and Tips
Kendra Cherry, MS, is a psychosocial rehabilitation specialist, psychology educator, and author of the "Everything Psychology Book."
:max_bytes(150000):strip_icc():format(webp)/IMG_9791-89504ab694d54b66bbd72cb84ffb860e.jpg)
Amy Morin, LCSW, is a psychotherapist and international bestselling author. Her books, including "13 Things Mentally Strong People Don't Do," have been translated into more than 40 languages. Her TEDx talk, "The Secret of Becoming Mentally Strong," is one of the most viewed talks of all time.
:max_bytes(150000):strip_icc():format(webp)/VW-MIND-Amy-2b338105f1ee493f94d7e333e410fa76.jpg)
Verywell / Alex Dos Diaz
- The Scientific Method
Hypothesis Format
Falsifiability of a hypothesis.
- Operationalization
Hypothesis Types
Hypotheses examples.
- Collecting Data
A hypothesis is a tentative statement about the relationship between two or more variables. It is a specific, testable prediction about what you expect to happen in a study. It is a preliminary answer to your question that helps guide the research process.
Consider a study designed to examine the relationship between sleep deprivation and test performance. The hypothesis might be: "This study is designed to assess the hypothesis that sleep-deprived people will perform worse on a test than individuals who are not sleep-deprived."
At a Glance
A hypothesis is crucial to scientific research because it offers a clear direction for what the researchers are looking to find. This allows them to design experiments to test their predictions and add to our scientific knowledge about the world. This article explores how a hypothesis is used in psychology research, how to write a good hypothesis, and the different types of hypotheses you might use.
The Hypothesis in the Scientific Method
In the scientific method , whether it involves research in psychology, biology, or some other area, a hypothesis represents what the researchers think will happen in an experiment. The scientific method involves the following steps:
- Forming a question
- Performing background research
- Creating a hypothesis
- Designing an experiment
- Collecting data
- Analyzing the results
- Drawing conclusions
- Communicating the results
The hypothesis is a prediction, but it involves more than a guess. Most of the time, the hypothesis begins with a question which is then explored through background research. At this point, researchers then begin to develop a testable hypothesis.
Unless you are creating an exploratory study, your hypothesis should always explain what you expect to happen.
In a study exploring the effects of a particular drug, the hypothesis might be that researchers expect the drug to have some type of effect on the symptoms of a specific illness. In psychology, the hypothesis might focus on how a certain aspect of the environment might influence a particular behavior.
Remember, a hypothesis does not have to be correct. While the hypothesis predicts what the researchers expect to see, the goal of the research is to determine whether this guess is right or wrong. When conducting an experiment, researchers might explore numerous factors to determine which ones might contribute to the ultimate outcome.
In many cases, researchers may find that the results of an experiment do not support the original hypothesis. When writing up these results, the researchers might suggest other options that should be explored in future studies.
In many cases, researchers might draw a hypothesis from a specific theory or build on previous research. For example, prior research has shown that stress can impact the immune system. So a researcher might hypothesize: "People with high-stress levels will be more likely to contract a common cold after being exposed to the virus than people who have low-stress levels."
In other instances, researchers might look at commonly held beliefs or folk wisdom. "Birds of a feather flock together" is one example of folk adage that a psychologist might try to investigate. The researcher might pose a specific hypothesis that "People tend to select romantic partners who are similar to them in interests and educational level."
Elements of a Good Hypothesis
So how do you write a good hypothesis? When trying to come up with a hypothesis for your research or experiments, ask yourself the following questions:
- Is your hypothesis based on your research on a topic?
- Can your hypothesis be tested?
- Does your hypothesis include independent and dependent variables?
Before you come up with a specific hypothesis, spend some time doing background research. Once you have completed a literature review, start thinking about potential questions you still have. Pay attention to the discussion section in the journal articles you read . Many authors will suggest questions that still need to be explored.
How to Formulate a Good Hypothesis
To form a hypothesis, you should take these steps:
- Collect as many observations about a topic or problem as you can.
- Evaluate these observations and look for possible causes of the problem.
- Create a list of possible explanations that you might want to explore.
- After you have developed some possible hypotheses, think of ways that you could confirm or disprove each hypothesis through experimentation. This is known as falsifiability.
In the scientific method , falsifiability is an important part of any valid hypothesis. In order to test a claim scientifically, it must be possible that the claim could be proven false.
Students sometimes confuse the idea of falsifiability with the idea that it means that something is false, which is not the case. What falsifiability means is that if something was false, then it is possible to demonstrate that it is false.
One of the hallmarks of pseudoscience is that it makes claims that cannot be refuted or proven false.
The Importance of Operational Definitions
A variable is a factor or element that can be changed and manipulated in ways that are observable and measurable. However, the researcher must also define how the variable will be manipulated and measured in the study.
Operational definitions are specific definitions for all relevant factors in a study. This process helps make vague or ambiguous concepts detailed and measurable.
For example, a researcher might operationally define the variable " test anxiety " as the results of a self-report measure of anxiety experienced during an exam. A "study habits" variable might be defined by the amount of studying that actually occurs as measured by time.
These precise descriptions are important because many things can be measured in various ways. Clearly defining these variables and how they are measured helps ensure that other researchers can replicate your results.
Replicability
One of the basic principles of any type of scientific research is that the results must be replicable.
Replication means repeating an experiment in the same way to produce the same results. By clearly detailing the specifics of how the variables were measured and manipulated, other researchers can better understand the results and repeat the study if needed.
Some variables are more difficult than others to define. For example, how would you operationally define a variable such as aggression ? For obvious ethical reasons, researchers cannot create a situation in which a person behaves aggressively toward others.
To measure this variable, the researcher must devise a measurement that assesses aggressive behavior without harming others. The researcher might utilize a simulated task to measure aggressiveness in this situation.
Hypothesis Checklist
- Does your hypothesis focus on something that you can actually test?
- Does your hypothesis include both an independent and dependent variable?
- Can you manipulate the variables?
- Can your hypothesis be tested without violating ethical standards?
The hypothesis you use will depend on what you are investigating and hoping to find. Some of the main types of hypotheses that you might use include:
- Simple hypothesis : This type of hypothesis suggests there is a relationship between one independent variable and one dependent variable.
- Complex hypothesis : This type suggests a relationship between three or more variables, such as two independent and dependent variables.
- Null hypothesis : This hypothesis suggests no relationship exists between two or more variables.
- Alternative hypothesis : This hypothesis states the opposite of the null hypothesis.
- Statistical hypothesis : This hypothesis uses statistical analysis to evaluate a representative population sample and then generalizes the findings to the larger group.
- Logical hypothesis : This hypothesis assumes a relationship between variables without collecting data or evidence.
A hypothesis often follows a basic format of "If {this happens} then {this will happen}." One way to structure your hypothesis is to describe what will happen to the dependent variable if you change the independent variable .
The basic format might be: "If {these changes are made to a certain independent variable}, then we will observe {a change in a specific dependent variable}."
A few examples of simple hypotheses:
- "Students who eat breakfast will perform better on a math exam than students who do not eat breakfast."
- "Students who experience test anxiety before an English exam will get lower scores than students who do not experience test anxiety."
- "Motorists who talk on the phone while driving will be more likely to make errors on a driving course than those who do not talk on the phone."
- "Children who receive a new reading intervention will have higher reading scores than students who do not receive the intervention."
Examples of a complex hypothesis include:
- "People with high-sugar diets and sedentary activity levels are more likely to develop depression."
- "Younger people who are regularly exposed to green, outdoor areas have better subjective well-being than older adults who have limited exposure to green spaces."
Examples of a null hypothesis include:
- "There is no difference in anxiety levels between people who take St. John's wort supplements and those who do not."
- "There is no difference in scores on a memory recall task between children and adults."
- "There is no difference in aggression levels between children who play first-person shooter games and those who do not."
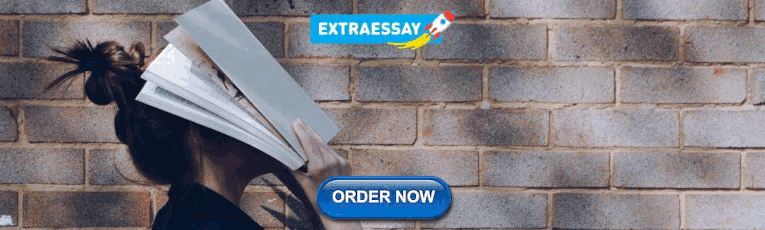
Examples of an alternative hypothesis:
- "People who take St. John's wort supplements will have less anxiety than those who do not."
- "Adults will perform better on a memory task than children."
- "Children who play first-person shooter games will show higher levels of aggression than children who do not."
Collecting Data on Your Hypothesis
Once a researcher has formed a testable hypothesis, the next step is to select a research design and start collecting data. The research method depends largely on exactly what they are studying. There are two basic types of research methods: descriptive research and experimental research.
Descriptive Research Methods
Descriptive research such as case studies , naturalistic observations , and surveys are often used when conducting an experiment is difficult or impossible. These methods are best used to describe different aspects of a behavior or psychological phenomenon.
Once a researcher has collected data using descriptive methods, a correlational study can examine how the variables are related. This research method might be used to investigate a hypothesis that is difficult to test experimentally.
Experimental Research Methods
Experimental methods are used to demonstrate causal relationships between variables. In an experiment, the researcher systematically manipulates a variable of interest (known as the independent variable) and measures the effect on another variable (known as the dependent variable).
Unlike correlational studies, which can only be used to determine if there is a relationship between two variables, experimental methods can be used to determine the actual nature of the relationship—whether changes in one variable actually cause another to change.
The hypothesis is a critical part of any scientific exploration. It represents what researchers expect to find in a study or experiment. In situations where the hypothesis is unsupported by the research, the research still has value. Such research helps us better understand how different aspects of the natural world relate to one another. It also helps us develop new hypotheses that can then be tested in the future.
Thompson WH, Skau S. On the scope of scientific hypotheses . R Soc Open Sci . 2023;10(8):230607. doi:10.1098/rsos.230607
Taran S, Adhikari NKJ, Fan E. Falsifiability in medicine: what clinicians can learn from Karl Popper [published correction appears in Intensive Care Med. 2021 Jun 17;:]. Intensive Care Med . 2021;47(9):1054-1056. doi:10.1007/s00134-021-06432-z
Eyler AA. Research Methods for Public Health . 1st ed. Springer Publishing Company; 2020. doi:10.1891/9780826182067.0004
Nosek BA, Errington TM. What is replication ? PLoS Biol . 2020;18(3):e3000691. doi:10.1371/journal.pbio.3000691
Aggarwal R, Ranganathan P. Study designs: Part 2 - Descriptive studies . Perspect Clin Res . 2019;10(1):34-36. doi:10.4103/picr.PICR_154_18
Nevid J. Psychology: Concepts and Applications. Wadworth, 2013.
By Kendra Cherry, MSEd Kendra Cherry, MS, is a psychosocial rehabilitation specialist, psychology educator, and author of the "Everything Psychology Book."
How to Generate a Hypothesis in the 3rd Person
Anne mullenniex.
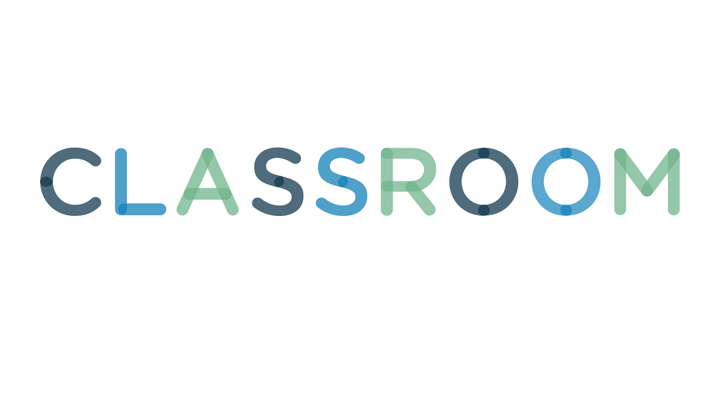
The Scientific Method is a way to study and explain the natural world, and can be used in every day applications, as well. This method consists of a logical sequence of steps that include observing a phenomenon; generating a scientific question about the phenomenon; postulating a tentative, falsifiable answer to that question; testing the possible answer by experimentation; and collecting and analyzing the results of your experiment. The tentative answer portion of this method is called a hypothesis and can be written as a prediction. Generating a hypothesis in the third person means taking yourself or another person out of the equation.
Ask your scientific question. For example: “Why doesn’t my lawnmower work?”
Change the first person point of view by removing the “I” and “my” to the third person objective point of view. This is the point of view of an objective narrator. For example: “Why doesn’t the lawnmower work?”
Stay in the third person objective point of view and state your hypothesis by answering the question. For example: “The lawnmower doesn’t work because it is out of gas.”
State your hypothesis, alternatively, as an answer to the question in a predictive “if…then” format. For example: “If the lawnmower isn’t working because it is out of gas, then it will work once gas is added to the tank.”
About the Author
Anne Mullenniex has been writing for eHow since 2009. She has owned and operated computer, construction, and insurance businesses. She has a bachelor's and a master's degree in biology and has taught at a community college. She is now working as an artist/artisan in multiple media.
Related Articles
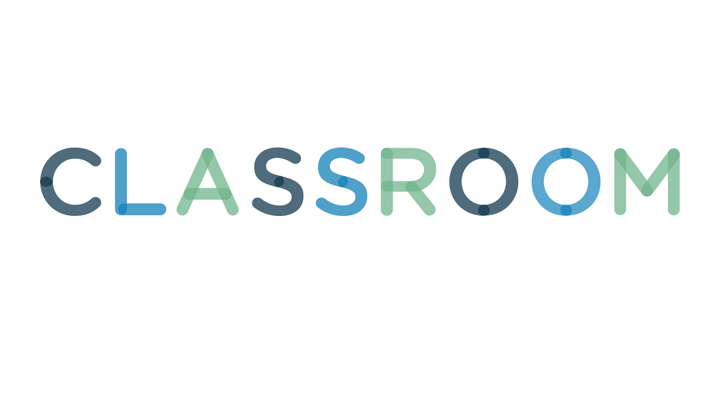
How to Do a Proposal for a Fifth Grade Science Project
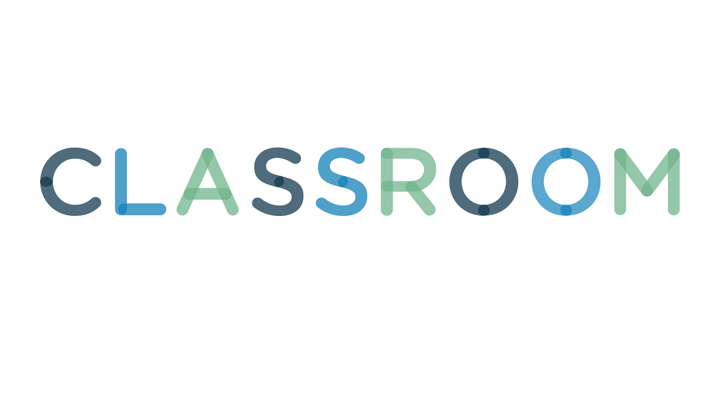
How to Address the State Attorney General in a Letter
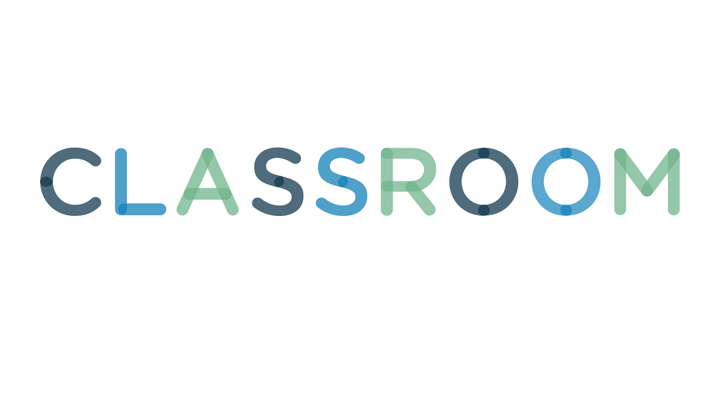
How to Write Up a Science Experiment in 3rd Grade
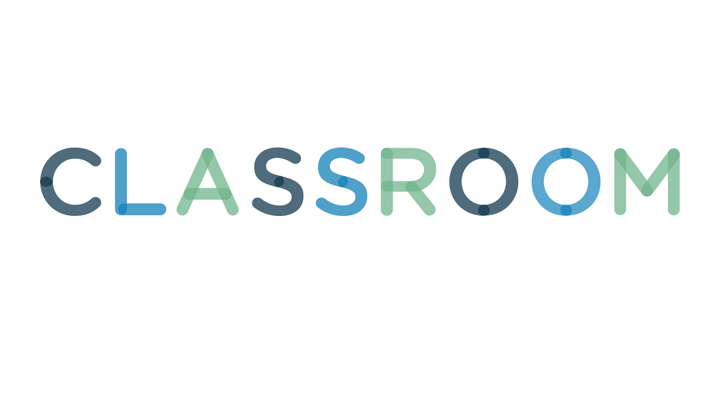
How to Write a Research Report for a Science Fair
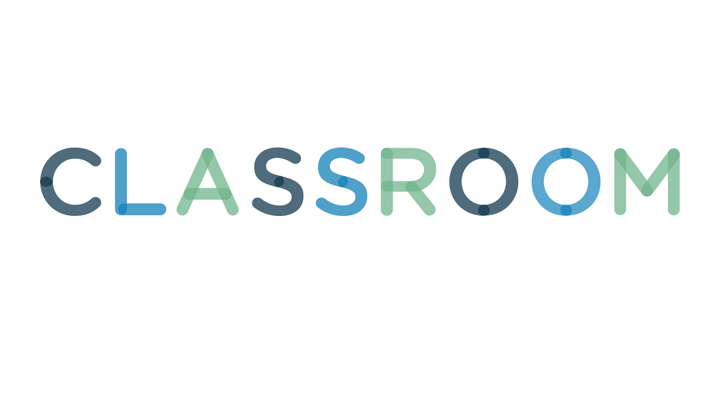
The Process Android.Process.Acore Stopped Unexpectedly
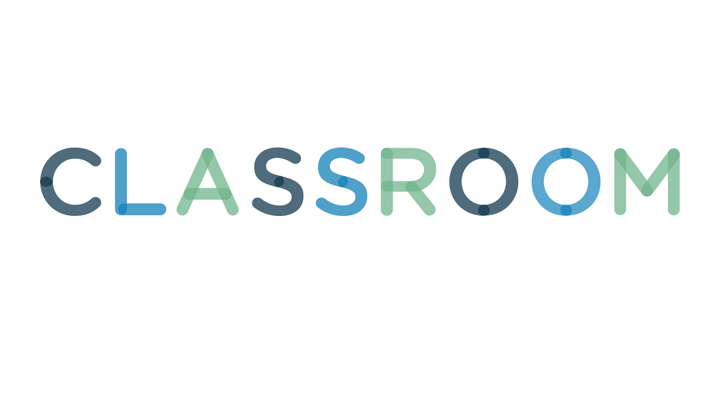
How to Write a Discussion for an APA Style Paper
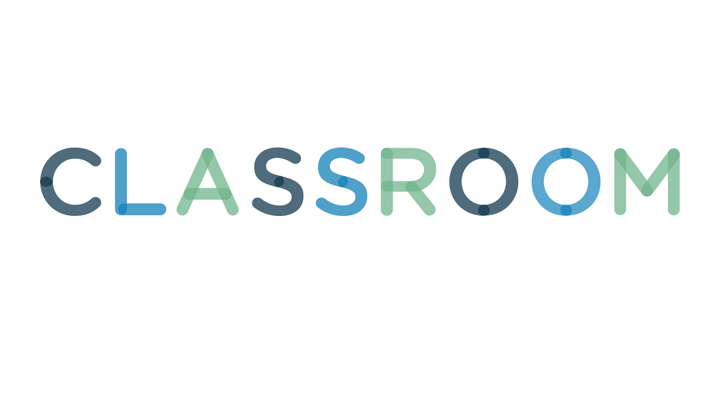
How to Write a 4th Grade Science Fair Report
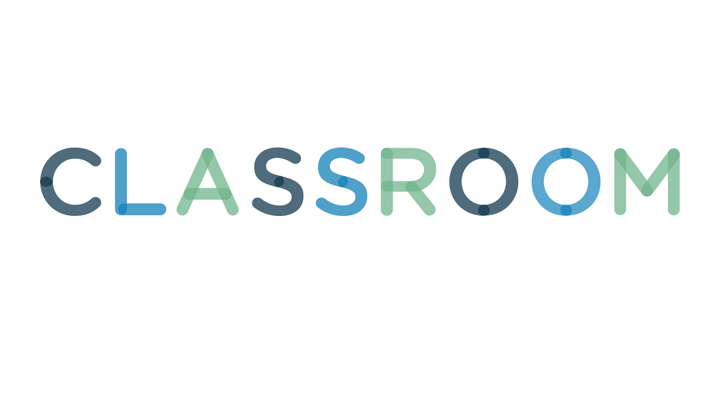
How to Write a Grade 10 Lab Report
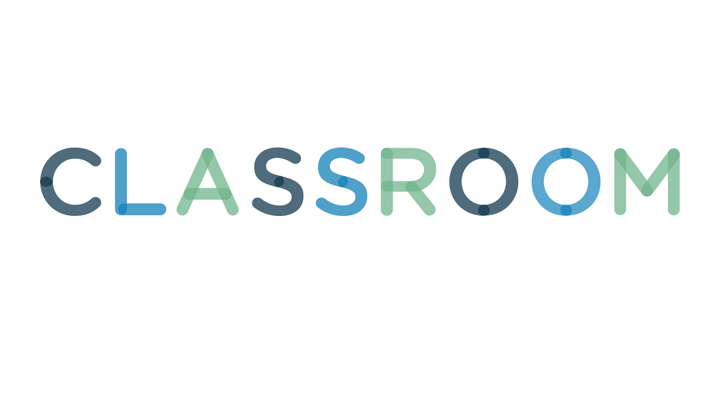
What Is a Problem Statement in a Lab Report?
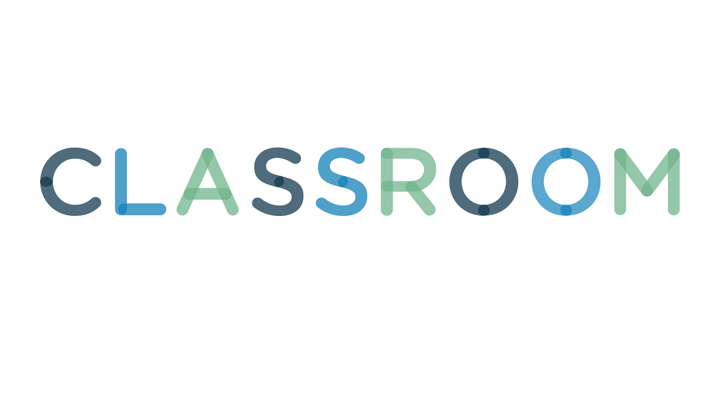
List of Pharmacy Schools Without PCAT Requirements
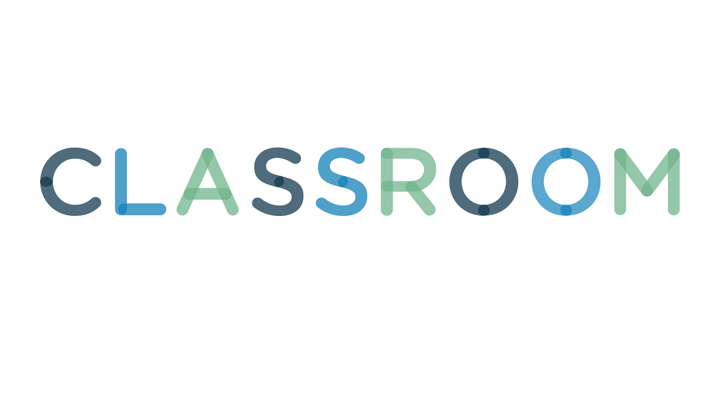
What Is a Conditional Statement in Math?
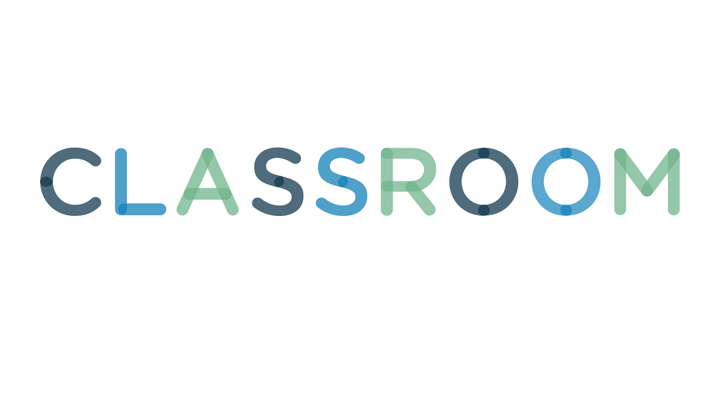
Steps to Writing Research Paper Abstracts
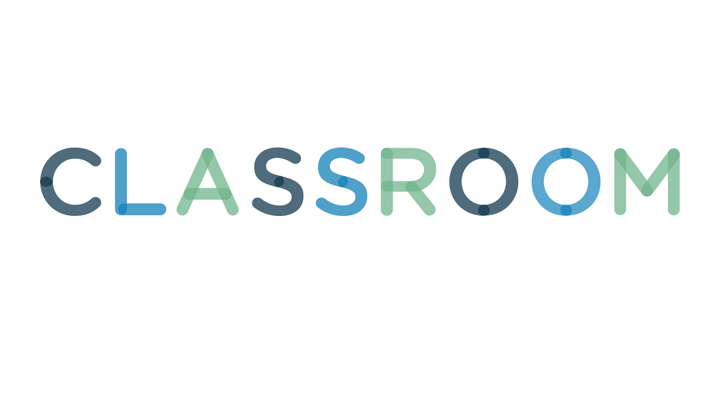
What Kind of Human Errors Can Occur During Experiments?
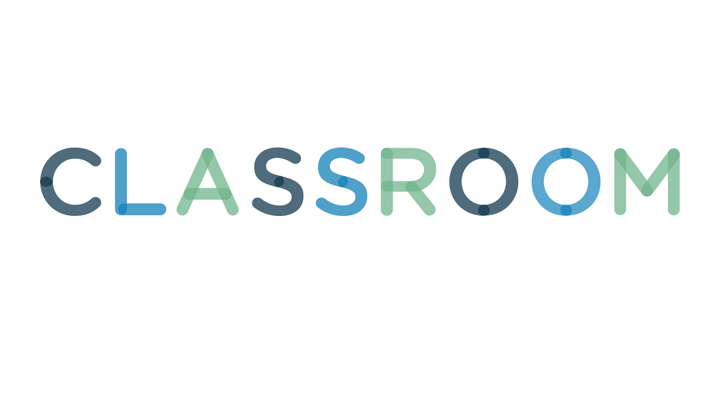
How Can I Find What Precinct I Live in?
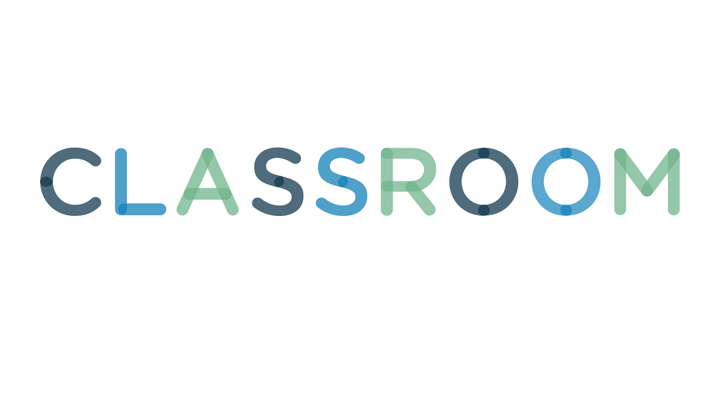
How to Export Zotero to Evernote
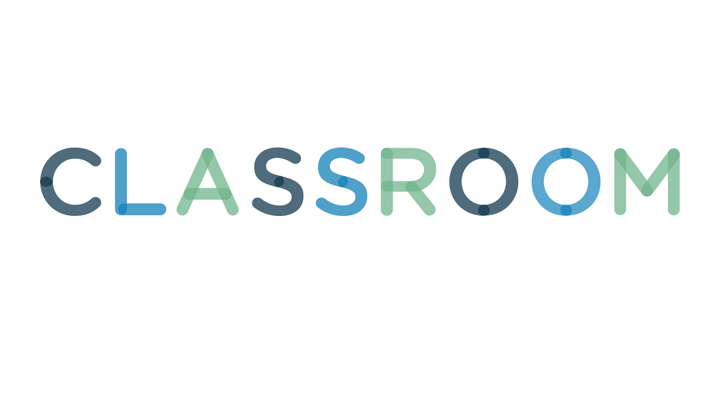
How to Write a Proposal for a Computer Science Topic
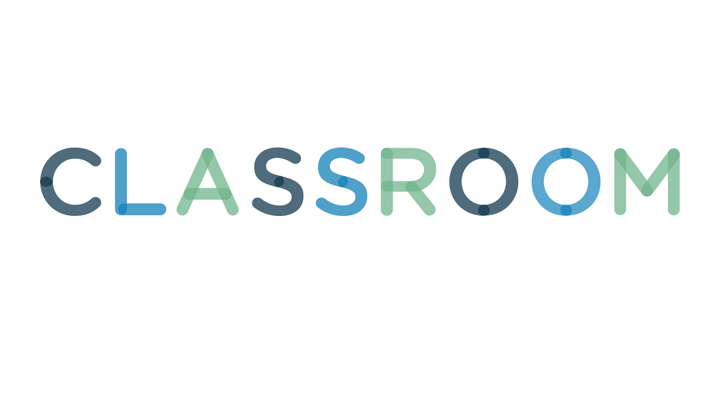
Where Is the Chrome Cache Saved?
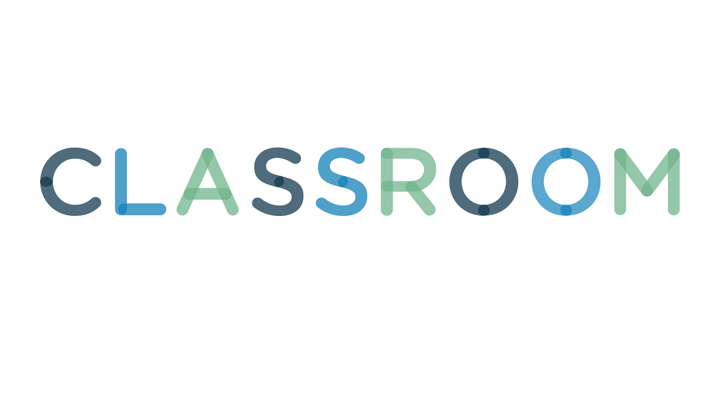
How Good of a Friend is Your BFF?
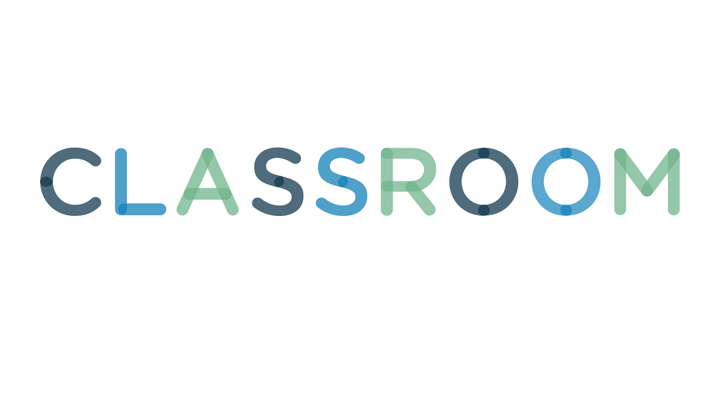
How to Solve Simultaneous Equations With a Ti 89
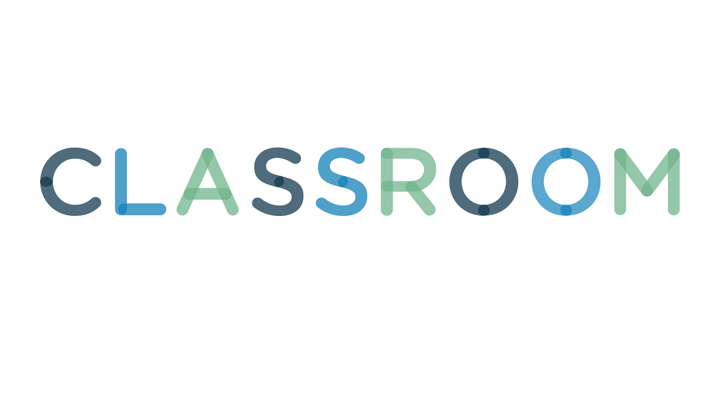
How to Write a Parenthetical Notation
Regardless of how old we are, we never stop learning. Classroom is the educational resource for people of all ages. Whether you’re studying times tables or applying to college, Classroom has the answers.
- Accessibility
- Terms of Use
- Privacy Policy
- Copyright Policy
- Manage Preferences
© 2020 Leaf Group Ltd. / Leaf Group Media, All Rights Reserved. Based on the Word Net lexical database for the English Language. See disclaimer .

Scientific Method: Step 3: HYPOTHESIS
- Step 1: QUESTION
- Step 2: RESEARCH
- Step 3: HYPOTHESIS
- Step 4: EXPERIMENT
- Step 5: DATA
- Step 6: CONCLUSION
Step 3: State your hypothesis
Now it's time to state your hypothesis . The hypothesis is an educated guess as to what will happen during your experiment.
The hypothesis is often written using the words "IF" and "THEN." For example, " If I do not study, then I will fail the test." The "if' and "then" statements reflect your independent and dependent variables .
The hypothesis should relate back to your original question and must be testable .
A word about variables...
Your experiment will include variables to measure and to explain any cause and effect. Below you will find some useful links describing the different types of variables.
- "What are independent and dependent variables" NCES
- [VIDEO] Biology: Independent vs. Dependent Variables (Nucleus Medical Media) Video explaining independent and dependent variables, with examples.
Resource Links
- What is and How to Write a Good Hypothesis in Research? (Elsevier)
- Hypothesis brochure from Penn State/Berks
- << Previous: Step 2: RESEARCH
- Next: Step 4: EXPERIMENT >>
- Last Updated: Jun 21, 2024 4:43 PM
- URL: https://harford.libguides.com/scientific_method
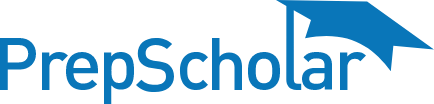
Choose Your Test
Sat / act prep online guides and tips, what is a hypothesis and how do i write one.
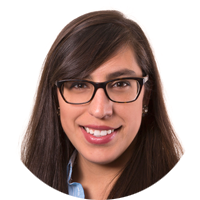
General Education
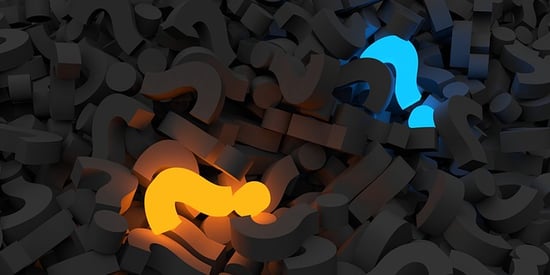
Think about something strange and unexplainable in your life. Maybe you get a headache right before it rains, or maybe you think your favorite sports team wins when you wear a certain color. If you wanted to see whether these are just coincidences or scientific fact, you would form a hypothesis, then create an experiment to see whether that hypothesis is true or not.
But what is a hypothesis, anyway? If you’re not sure about what a hypothesis is--or how to test for one!--you’re in the right place. This article will teach you everything you need to know about hypotheses, including:
- Defining the term “hypothesis”
- Providing hypothesis examples
- Giving you tips for how to write your own hypothesis
So let’s get started!
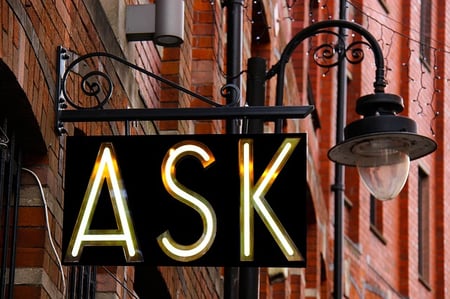
What Is a Hypothesis?
Merriam Webster defines a hypothesis as “an assumption or concession made for the sake of argument.” In other words, a hypothesis is an educated guess . Scientists make a reasonable assumption--or a hypothesis--then design an experiment to test whether it’s true or not. Keep in mind that in science, a hypothesis should be testable. You have to be able to design an experiment that tests your hypothesis in order for it to be valid.
As you could assume from that statement, it’s easy to make a bad hypothesis. But when you’re holding an experiment, it’s even more important that your guesses be good...after all, you’re spending time (and maybe money!) to figure out more about your observation. That’s why we refer to a hypothesis as an educated guess--good hypotheses are based on existing data and research to make them as sound as possible.
Hypotheses are one part of what’s called the scientific method . Every (good) experiment or study is based in the scientific method. The scientific method gives order and structure to experiments and ensures that interference from scientists or outside influences does not skew the results. It’s important that you understand the concepts of the scientific method before holding your own experiment. Though it may vary among scientists, the scientific method is generally made up of six steps (in order):
- Observation
- Asking questions
- Forming a hypothesis
- Analyze the data
- Communicate your results
You’ll notice that the hypothesis comes pretty early on when conducting an experiment. That’s because experiments work best when they’re trying to answer one specific question. And you can’t conduct an experiment until you know what you’re trying to prove!
Independent and Dependent Variables
After doing your research, you’re ready for another important step in forming your hypothesis: identifying variables. Variables are basically any factor that could influence the outcome of your experiment . Variables have to be measurable and related to the topic being studied.
There are two types of variables: independent variables and dependent variables. I ndependent variables remain constant . For example, age is an independent variable; it will stay the same, and researchers can look at different ages to see if it has an effect on the dependent variable.
Speaking of dependent variables... dependent variables are subject to the influence of the independent variable , meaning that they are not constant. Let’s say you want to test whether a person’s age affects how much sleep they need. In that case, the independent variable is age (like we mentioned above), and the dependent variable is how much sleep a person gets.
Variables will be crucial in writing your hypothesis. You need to be able to identify which variable is which, as both the independent and dependent variables will be written into your hypothesis. For instance, in a study about exercise, the independent variable might be the speed at which the respondents walk for thirty minutes, and the dependent variable would be their heart rate. In your study and in your hypothesis, you’re trying to understand the relationship between the two variables.
Elements of a Good Hypothesis
The best hypotheses start by asking the right questions . For instance, if you’ve observed that the grass is greener when it rains twice a week, you could ask what kind of grass it is, what elevation it’s at, and if the grass across the street responds to rain in the same way. Any of these questions could become the backbone of experiments to test why the grass gets greener when it rains fairly frequently.
As you’re asking more questions about your first observation, make sure you’re also making more observations . If it doesn’t rain for two weeks and the grass still looks green, that’s an important observation that could influence your hypothesis. You'll continue observing all throughout your experiment, but until the hypothesis is finalized, every observation should be noted.
Finally, you should consult secondary research before writing your hypothesis . Secondary research is comprised of results found and published by other people. You can usually find this information online or at your library. Additionally, m ake sure the research you find is credible and related to your topic. If you’re studying the correlation between rain and grass growth, it would help you to research rain patterns over the past twenty years for your county, published by a local agricultural association. You should also research the types of grass common in your area, the type of grass in your lawn, and whether anyone else has conducted experiments about your hypothesis. Also be sure you’re checking the quality of your research . Research done by a middle school student about what minerals can be found in rainwater would be less useful than an article published by a local university.
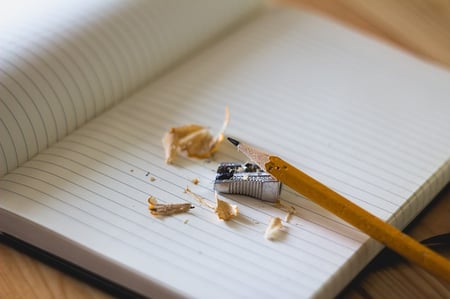
Writing Your Hypothesis
Once you’ve considered all of the factors above, you’re ready to start writing your hypothesis. Hypotheses usually take a certain form when they’re written out in a research report.
When you boil down your hypothesis statement, you are writing down your best guess and not the question at hand . This means that your statement should be written as if it is fact already, even though you are simply testing it.
The reason for this is that, after you have completed your study, you'll either accept or reject your if-then or your null hypothesis. All hypothesis testing examples should be measurable and able to be confirmed or denied. You cannot confirm a question, only a statement!
In fact, you come up with hypothesis examples all the time! For instance, when you guess on the outcome of a basketball game, you don’t say, “Will the Miami Heat beat the Boston Celtics?” but instead, “I think the Miami Heat will beat the Boston Celtics.” You state it as if it is already true, even if it turns out you’re wrong. You do the same thing when writing your hypothesis.
Additionally, keep in mind that hypotheses can range from very specific to very broad. These hypotheses can be specific, but if your hypothesis testing examples involve a broad range of causes and effects, your hypothesis can also be broad.
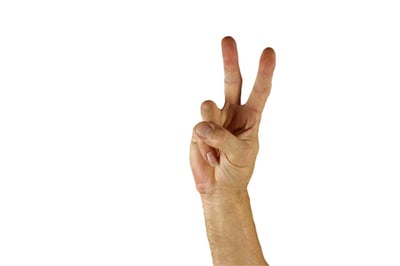
The Two Types of Hypotheses
Now that you understand what goes into a hypothesis, it’s time to look more closely at the two most common types of hypothesis: the if-then hypothesis and the null hypothesis.
#1: If-Then Hypotheses
First of all, if-then hypotheses typically follow this formula:
If ____ happens, then ____ will happen.
The goal of this type of hypothesis is to test the causal relationship between the independent and dependent variable. It’s fairly simple, and each hypothesis can vary in how detailed it can be. We create if-then hypotheses all the time with our daily predictions. Here are some examples of hypotheses that use an if-then structure from daily life:
- If I get enough sleep, I’ll be able to get more work done tomorrow.
- If the bus is on time, I can make it to my friend’s birthday party.
- If I study every night this week, I’ll get a better grade on my exam.
In each of these situations, you’re making a guess on how an independent variable (sleep, time, or studying) will affect a dependent variable (the amount of work you can do, making it to a party on time, or getting better grades).
You may still be asking, “What is an example of a hypothesis used in scientific research?” Take one of the hypothesis examples from a real-world study on whether using technology before bed affects children’s sleep patterns. The hypothesis read s:
“We hypothesized that increased hours of tablet- and phone-based screen time at bedtime would be inversely correlated with sleep quality and child attention.”
It might not look like it, but this is an if-then statement. The researchers basically said, “If children have more screen usage at bedtime, then their quality of sleep and attention will be worse.” The sleep quality and attention are the dependent variables and the screen usage is the independent variable. (Usually, the independent variable comes after the “if” and the dependent variable comes after the “then,” as it is the independent variable that affects the dependent variable.) This is an excellent example of how flexible hypothesis statements can be, as long as the general idea of “if-then” and the independent and dependent variables are present.
#2: Null Hypotheses
Your if-then hypothesis is not the only one needed to complete a successful experiment, however. You also need a null hypothesis to test it against. In its most basic form, the null hypothesis is the opposite of your if-then hypothesis . When you write your null hypothesis, you are writing a hypothesis that suggests that your guess is not true, and that the independent and dependent variables have no relationship .
One null hypothesis for the cell phone and sleep study from the last section might say:
“If children have more screen usage at bedtime, their quality of sleep and attention will not be worse.”
In this case, this is a null hypothesis because it’s asking the opposite of the original thesis!
Conversely, if your if-then hypothesis suggests that your two variables have no relationship, then your null hypothesis would suggest that there is one. So, pretend that there is a study that is asking the question, “Does the amount of followers on Instagram influence how long people spend on the app?” The independent variable is the amount of followers, and the dependent variable is the time spent. But if you, as the researcher, don’t think there is a relationship between the number of followers and time spent, you might write an if-then hypothesis that reads:
“If people have many followers on Instagram, they will not spend more time on the app than people who have less.”
In this case, the if-then suggests there isn’t a relationship between the variables. In that case, one of the null hypothesis examples might say:
“If people have many followers on Instagram, they will spend more time on the app than people who have less.”
You then test both the if-then and the null hypothesis to gauge if there is a relationship between the variables, and if so, how much of a relationship.
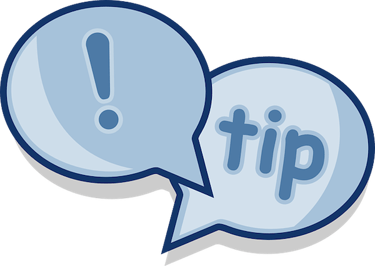
4 Tips to Write the Best Hypothesis
If you’re going to take the time to hold an experiment, whether in school or by yourself, you’re also going to want to take the time to make sure your hypothesis is a good one. The best hypotheses have four major elements in common: plausibility, defined concepts, observability, and general explanation.
#1: Plausibility
At first glance, this quality of a hypothesis might seem obvious. When your hypothesis is plausible, that means it’s possible given what we know about science and general common sense. However, improbable hypotheses are more common than you might think.
Imagine you’re studying weight gain and television watching habits. If you hypothesize that people who watch more than twenty hours of television a week will gain two hundred pounds or more over the course of a year, this might be improbable (though it’s potentially possible). Consequently, c ommon sense can tell us the results of the study before the study even begins.
Improbable hypotheses generally go against science, as well. Take this hypothesis example:
“If a person smokes one cigarette a day, then they will have lungs just as healthy as the average person’s.”
This hypothesis is obviously untrue, as studies have shown again and again that cigarettes negatively affect lung health. You must be careful that your hypotheses do not reflect your own personal opinion more than they do scientifically-supported findings. This plausibility points to the necessity of research before the hypothesis is written to make sure that your hypothesis has not already been disproven.
#2: Defined Concepts
The more advanced you are in your studies, the more likely that the terms you’re using in your hypothesis are specific to a limited set of knowledge. One of the hypothesis testing examples might include the readability of printed text in newspapers, where you might use words like “kerning” and “x-height.” Unless your readers have a background in graphic design, it’s likely that they won’t know what you mean by these terms. Thus, it’s important to either write what they mean in the hypothesis itself or in the report before the hypothesis.
Here’s what we mean. Which of the following sentences makes more sense to the common person?
If the kerning is greater than average, more words will be read per minute.
If the space between letters is greater than average, more words will be read per minute.
For people reading your report that are not experts in typography, simply adding a few more words will be helpful in clarifying exactly what the experiment is all about. It’s always a good idea to make your research and findings as accessible as possible.
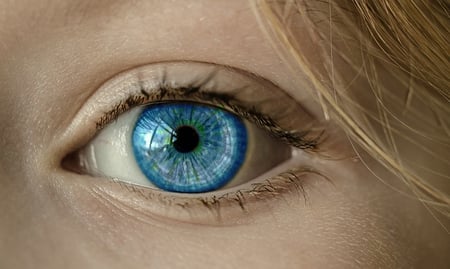
Good hypotheses ensure that you can observe the results.
#3: Observability
In order to measure the truth or falsity of your hypothesis, you must be able to see your variables and the way they interact. For instance, if your hypothesis is that the flight patterns of satellites affect the strength of certain television signals, yet you don’t have a telescope to view the satellites or a television to monitor the signal strength, you cannot properly observe your hypothesis and thus cannot continue your study.
Some variables may seem easy to observe, but if you do not have a system of measurement in place, you cannot observe your hypothesis properly. Here’s an example: if you’re experimenting on the effect of healthy food on overall happiness, but you don’t have a way to monitor and measure what “overall happiness” means, your results will not reflect the truth. Monitoring how often someone smiles for a whole day is not reasonably observable, but having the participants state how happy they feel on a scale of one to ten is more observable.
In writing your hypothesis, always keep in mind how you'll execute the experiment.
#4: Generalizability
Perhaps you’d like to study what color your best friend wears the most often by observing and documenting the colors she wears each day of the week. This might be fun information for her and you to know, but beyond you two, there aren’t many people who could benefit from this experiment. When you start an experiment, you should note how generalizable your findings may be if they are confirmed. Generalizability is basically how common a particular phenomenon is to other people’s everyday life.
Let’s say you’re asking a question about the health benefits of eating an apple for one day only, you need to realize that the experiment may be too specific to be helpful. It does not help to explain a phenomenon that many people experience. If you find yourself with too specific of a hypothesis, go back to asking the big question: what is it that you want to know, and what do you think will happen between your two variables?
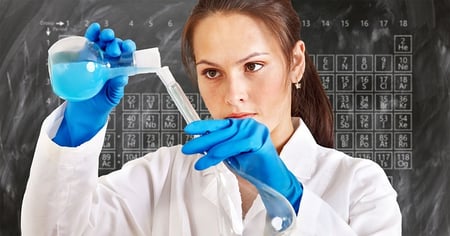
Hypothesis Testing Examples
We know it can be hard to write a good hypothesis unless you’ve seen some good hypothesis examples. We’ve included four hypothesis examples based on some made-up experiments. Use these as templates or launch pads for coming up with your own hypotheses.
Experiment #1: Students Studying Outside (Writing a Hypothesis)
You are a student at PrepScholar University. When you walk around campus, you notice that, when the temperature is above 60 degrees, more students study in the quad. You want to know when your fellow students are more likely to study outside. With this information, how do you make the best hypothesis possible?
You must remember to make additional observations and do secondary research before writing your hypothesis. In doing so, you notice that no one studies outside when it’s 75 degrees and raining, so this should be included in your experiment. Also, studies done on the topic beforehand suggested that students are more likely to study in temperatures less than 85 degrees. With this in mind, you feel confident that you can identify your variables and write your hypotheses:
If-then: “If the temperature in Fahrenheit is less than 60 degrees, significantly fewer students will study outside.”
Null: “If the temperature in Fahrenheit is less than 60 degrees, the same number of students will study outside as when it is more than 60 degrees.”
These hypotheses are plausible, as the temperatures are reasonably within the bounds of what is possible. The number of people in the quad is also easily observable. It is also not a phenomenon specific to only one person or at one time, but instead can explain a phenomenon for a broader group of people.
To complete this experiment, you pick the month of October to observe the quad. Every day (except on the days where it’s raining)from 3 to 4 PM, when most classes have released for the day, you observe how many people are on the quad. You measure how many people come and how many leave. You also write down the temperature on the hour.
After writing down all of your observations and putting them on a graph, you find that the most students study on the quad when it is 70 degrees outside, and that the number of students drops a lot once the temperature reaches 60 degrees or below. In this case, your research report would state that you accept or “failed to reject” your first hypothesis with your findings.
Experiment #2: The Cupcake Store (Forming a Simple Experiment)
Let’s say that you work at a bakery. You specialize in cupcakes, and you make only two colors of frosting: yellow and purple. You want to know what kind of customers are more likely to buy what kind of cupcake, so you set up an experiment. Your independent variable is the customer’s gender, and the dependent variable is the color of the frosting. What is an example of a hypothesis that might answer the question of this study?
Here’s what your hypotheses might look like:
If-then: “If customers’ gender is female, then they will buy more yellow cupcakes than purple cupcakes.”
Null: “If customers’ gender is female, then they will be just as likely to buy purple cupcakes as yellow cupcakes.”
This is a pretty simple experiment! It passes the test of plausibility (there could easily be a difference), defined concepts (there’s nothing complicated about cupcakes!), observability (both color and gender can be easily observed), and general explanation ( this would potentially help you make better business decisions ).
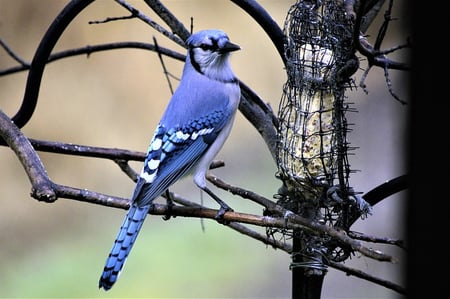
Experiment #3: Backyard Bird Feeders (Integrating Multiple Variables and Rejecting the If-Then Hypothesis)
While watching your backyard bird feeder, you realized that different birds come on the days when you change the types of seeds. You decide that you want to see more cardinals in your backyard, so you decide to see what type of food they like the best and set up an experiment.
However, one morning, you notice that, while some cardinals are present, blue jays are eating out of your backyard feeder filled with millet. You decide that, of all of the other birds, you would like to see the blue jays the least. This means you'll have more than one variable in your hypothesis. Your new hypotheses might look like this:
If-then: “If sunflower seeds are placed in the bird feeders, then more cardinals will come than blue jays. If millet is placed in the bird feeders, then more blue jays will come than cardinals.”
Null: “If either sunflower seeds or millet are placed in the bird, equal numbers of cardinals and blue jays will come.”
Through simple observation, you actually find that cardinals come as often as blue jays when sunflower seeds or millet is in the bird feeder. In this case, you would reject your “if-then” hypothesis and “fail to reject” your null hypothesis . You cannot accept your first hypothesis, because it’s clearly not true. Instead you found that there was actually no relation between your different variables. Consequently, you would need to run more experiments with different variables to see if the new variables impact the results.
Experiment #4: In-Class Survey (Including an Alternative Hypothesis)
You’re about to give a speech in one of your classes about the importance of paying attention. You want to take this opportunity to test a hypothesis you’ve had for a while:
If-then: If students sit in the first two rows of the classroom, then they will listen better than students who do not.
Null: If students sit in the first two rows of the classroom, then they will not listen better or worse than students who do not.
You give your speech and then ask your teacher if you can hand out a short survey to the class. On the survey, you’ve included questions about some of the topics you talked about. When you get back the results, you’re surprised to see that not only do the students in the first two rows not pay better attention, but they also scored worse than students in other parts of the classroom! Here, both your if-then and your null hypotheses are not representative of your findings. What do you do?
This is when you reject both your if-then and null hypotheses and instead create an alternative hypothesis . This type of hypothesis is used in the rare circumstance that neither of your hypotheses is able to capture your findings . Now you can use what you’ve learned to draft new hypotheses and test again!
Key Takeaways: Hypothesis Writing
The more comfortable you become with writing hypotheses, the better they will become. The structure of hypotheses is flexible and may need to be changed depending on what topic you are studying. The most important thing to remember is the purpose of your hypothesis and the difference between the if-then and the null . From there, in forming your hypothesis, you should constantly be asking questions, making observations, doing secondary research, and considering your variables. After you have written your hypothesis, be sure to edit it so that it is plausible, clearly defined, observable, and helpful in explaining a general phenomenon.
Writing a hypothesis is something that everyone, from elementary school children competing in a science fair to professional scientists in a lab, needs to know how to do. Hypotheses are vital in experiments and in properly executing the scientific method . When done correctly, hypotheses will set up your studies for success and help you to understand the world a little better, one experiment at a time.
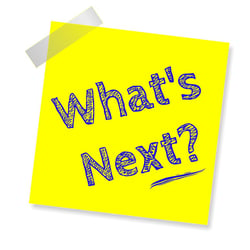
What’s Next?
If you’re studying for the science portion of the ACT, there’s definitely a lot you need to know. We’ve got the tools to help, though! Start by checking out our ultimate study guide for the ACT Science subject test. Once you read through that, be sure to download our recommended ACT Science practice tests , since they’re one of the most foolproof ways to improve your score. (And don’t forget to check out our expert guide book , too.)
If you love science and want to major in a scientific field, you should start preparing in high school . Here are the science classes you should take to set yourself up for success.
If you’re trying to think of science experiments you can do for class (or for a science fair!), here’s a list of 37 awesome science experiments you can do at home
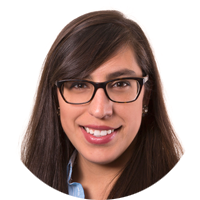
Ashley Sufflé Robinson has a Ph.D. in 19th Century English Literature. As a content writer for PrepScholar, Ashley is passionate about giving college-bound students the in-depth information they need to get into the school of their dreams.
Ask a Question Below
Have any questions about this article or other topics? Ask below and we'll reply!
Improve With Our Famous Guides
- For All Students
The 5 Strategies You Must Be Using to Improve 160+ SAT Points
How to Get a Perfect 1600, by a Perfect Scorer
Series: How to Get 800 on Each SAT Section:
Score 800 on SAT Math
Score 800 on SAT Reading
Score 800 on SAT Writing
Series: How to Get to 600 on Each SAT Section:
Score 600 on SAT Math
Score 600 on SAT Reading
Score 600 on SAT Writing
Free Complete Official SAT Practice Tests
What SAT Target Score Should You Be Aiming For?
15 Strategies to Improve Your SAT Essay
The 5 Strategies You Must Be Using to Improve 4+ ACT Points
How to Get a Perfect 36 ACT, by a Perfect Scorer
Series: How to Get 36 on Each ACT Section:
36 on ACT English
36 on ACT Math
36 on ACT Reading
36 on ACT Science
Series: How to Get to 24 on Each ACT Section:
24 on ACT English
24 on ACT Math
24 on ACT Reading
24 on ACT Science
What ACT target score should you be aiming for?
ACT Vocabulary You Must Know
ACT Writing: 15 Tips to Raise Your Essay Score
How to Get Into Harvard and the Ivy League
How to Get a Perfect 4.0 GPA
How to Write an Amazing College Essay
What Exactly Are Colleges Looking For?
Is the ACT easier than the SAT? A Comprehensive Guide
Should you retake your SAT or ACT?
When should you take the SAT or ACT?
Stay Informed
Get the latest articles and test prep tips!
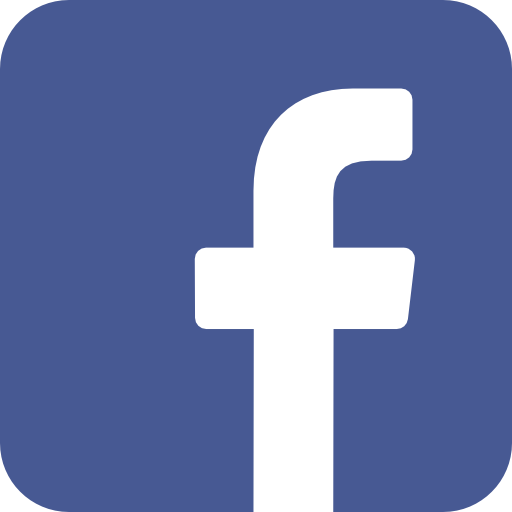
Stats and R
Hypothesis test by hand.
- Confidence interval
Hypothesis test
- Inferential statistics
Descriptive versus inferential statistics
Motivations and limitations, step #1: stating the null and alternative hypothesis, step #2: computing the test statistic, step #3: finding the critical value, why don’t we accept \(h_0\) , step #3: computing the p -value, step #4: concluding and interpreting the results, step #2: computing the confidence interval, step #3: concluding and interpreting the results, which method to choose.
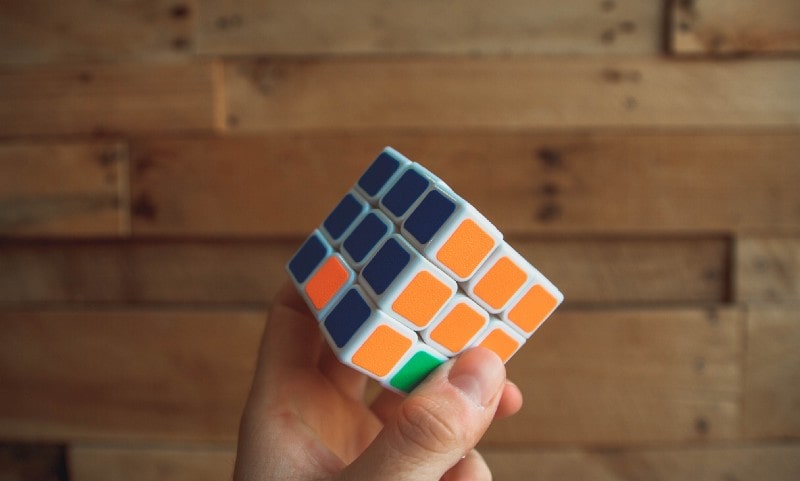
Remember that descriptive statistics is the branch of statistics aiming at describing and summarizing a set of data in the best possible manner, that is, by reducing it down to a few meaningful key measures and visualizations—with as little loss of information as possible. In other words, the branch of descriptive statistics helps to have a better understanding and a clear image about a set of observations thanks to summary statistics and graphics. With descriptive statistics, there is no uncertainty because we describe only the group of observations that we decided to work on and no attempt is made to generalize the observed characteristics to another or to a larger group of observations.
Inferential statistics , one the other hand, is the branch of statistics that uses a random sample of data taken from a population to make inferences, i.e., to draw conclusions about the population of interest (see the difference between population and sample if you need a refresh of the two concepts). In other words, information from the sample is used to make generalizations about the parameter of interest in the population.
The two most important tools used in the domain of inferential statistics are:
- hypothesis test (which is the main subject of the present article), and
- confidence interval (which is briefly discussed in this section )
Via my teaching tasks, I realized that many students (especially in introductory statistic classes) struggle to perform hypothesis tests and interpret the results. It seems to me that these students often encounter difficulties mainly because hypothesis testing is rather unclear and abstract to them.
One of the reason it looks abstract to them is because they do not understand the final goal of hypothesis testing—the “why” behind this tool. They often do inferential statistics without understanding the reasoning behind it, as if they were following a cooking recipe which does not require any thinking. However, as soon as they understand the principle underlying hypothesis testing, it is much easier for them to apply the concepts and solve the exercises.
For this reason, I though it would be useful to write an article on the goal of hypothesis tests (the “why?”), in which context they should be used (the “when?”), how they work (the “how?”) and how to interpret the results (the “so what?”). Like anything else in statistics, it becomes much easier to apply a concept in practice when we understand what we are testing or what we are trying to demonstrate beforehand.
In this article, I present—as comprehensibly as possible—the different steps required to perform and conclude a hypothesis test by hand .
These steps are illustrated with a basic example. This will build the theoretical foundations of hypothesis testing, which will in turn be of great help for the understanding of most statistical tests .
Hypothesis tests come in many forms and can be used for many parameters or research questions. The steps I present in this article are not applicable to all hypothesis test, unfortunately.
They are however, appropriate for at least the most common hypothesis tests—the tests on:
- One mean: \(\mu\)
- independent samples: \(\mu_1\) and \(\mu_2\)
- paired samples: \(\mu_D\)
- One proportion: \(p\)
- Two proportions: \(p_1\) and \(p_2\)
- One variance: \(\sigma^2\)
- Two variances: \(\sigma^2_1\) and \(\sigma^2_2\)
The good news is that the principles behind these 6 statistical tests (and many more) are exactly the same. So if you understand the intuition and the process for one of them, all others pretty much follow.
Unlike descriptive statistics where we only describe the data at hand, hypothesis tests use a subset of observations , referred as a sample , to draw conclusions about a population .
One may wonder why we would try to “guess” or make inference about a parameter of a population based on a sample, instead of simply collecting data for the entire population, compute statistics we are interested in and take decisions based upon that.
The main reason we actually use a sample instead of the entire population is because, most of the time, collecting data on the entire population is practically impossible, too complex, too expensive, it would take too long, or a combination of any of these. 1
So the overall objective of a hypothesis test is to draw conclusions in order to confirm or refute a belief about a population , based on a smaller group of observations.
In practice, we take some measurements of the variable of interest—representing the sample(s)—and we check whether our measurements are likely or not given our assumption (our belief). Based on the probability of observing the sample(s) we have, we decide whether we can trust our belief or not.
Hypothesis tests have many practical applications.
Here are different situations illustrating when the 6 tests mentioned above would be appropriate:
- One mean: suppose that a health professional would like to test whether the mean weight of Belgian adults is different than 80 kg (176.4 lbs).
- Independent samples: suppose that a physiotherapist would like to test the effectiveness of a new treatment by measuring the mean response time (in seconds) for patients in a control group and patients in a treatment group, where patients in the two groups are different.
- Paired samples: suppose that a physiotherapist would like to test the effectiveness of a new treatment by measuring the mean response time (in seconds) before and after a treatment, where patients are measured twice—before and after treatment, so patients are the same in the 2 samples.
- One proportion: suppose that a political pundit would like to test whether the proportion of citizens who are going to vote for a specific candidate is smaller than 30%.
- Two proportions: suppose that a doctor would like to test whether the proportion of smokers is different between professional and amateur athletes.
- One variance: suppose that an engineer would like to test whether a voltmeter has a lower variability than what is imposed by the safety standards.
- Two variances: suppose that, in a factory, two production lines work independently from each other. The financial manager would like to test whether the costs of the weekly maintenance of these two machines have the same variance. Note that a test on two variances is also often performed to verify the assumption of equal variances, which is required for several other statistical tests, such as the Student’s t-test for instance.
Of course, this is a non-exhaustive list of potential applications and many research questions can be answered thanks to a hypothesis test.
One important point to remember is that in hypothesis testing we are always interested in the population and not in the sample. The sample is used for the aim of drawing conclusions about the population, so we always test in terms of the population.
Usually, hypothesis tests are used to answer research questions in confirmatory analyses . Confirmatory analyses refer to statistical analyses where hypotheses—deducted from theory—are defined beforehand (preferably before data collection). In this approach, the researcher has a specific idea about the variables under consideration and she is trying to see if her idea, specified as hypotheses, is supported by data.
On the other hand, hypothesis tests are rarely used in exploratory analyses. 2 Exploratory analyses aims to uncover possible relationships between the variables under investigation. In this approach, the researcher does not have any clear theory-driven assumptions or ideas in mind before data collection. This is the reason exploratory analyses are sometimes referred as hypothesis-generating analyses—they are used to create some hypotheses, which in turn may be tested via confirmatory analyses at a later stage.
There are, to my knowledge, 3 different methods to perform a hypothesis tests:
Method A: Comparing the test statistic with the critical value
Method b: comparing the p -value with the significance level \(\alpha\), method c: comparing the target parameter with the confidence interval.
Although the process for these 3 approaches may slightly differ, they all lead to the exact same conclusions. Using one method or another is, therefore, more often than not a matter of personal choice or a matter of context. See this section to know which method I use depending on the context.
I present the 3 methods in the following sections, starting with, in my opinion, the most comprehensive one when it comes to doing it by hand: comparing the test statistic with the critical value.
For the three methods, I will explain the required steps to perform a hypothesis test from a general point of view and illustrate them with the following situation: 3
Suppose a health professional who would like to test whether the mean weight of Belgian adults is different than 80 kg.
Note that, as for most hypothesis tests, the test we are going to use as example below requires some assumptions. Since the aim of the present article is to explain a hypothesis test, we assume that all assumptions are met. For the interested reader, see the assumptions (and how to verify them) for this type of hypothesis test in the article presenting the one-sample t-test .
Method A, which consists in comparing the test statistic with the critical value, boils down to the following 4 steps:
- Stating the null and alternative hypothesis
- Computing the test statistic
- Finding the critical value
- Concluding and interpreting the results
Each step is detailed below.
As discussed before, a hypothesis test first requires an idea, that is, an assumption about a phenomenon. This assumption, referred as hypothesis, is derived from the theory and/or the research question.
Since a hypothesis test is used to confirm or refute a prior belief, we need to formulate our belief so that there is a null and an alternative hypothesis . Those hypotheses must be mutually exclusive , which means that they cannot be true at the same time. This is step #1.
In the context of our scenario, the null and alternative hypothesis are thus:
- Null hypothesis \(H_0: \mu = 80\)
- Alternative hypothesis \(H_1: \mu \ne 80\)
When stating the null and alternative hypothesis, bear in mind the following three points:
- We are always interested in the population and not in the sample. This is the reason \(H_0\) and \(H_1\) will always be written in terms of the population and not in terms of the sample (in this case, \(\mu\) and not \(\bar{x}\) ).
- The assumption we would like to test is often the alternative hypothesis. If the researcher wanted to test whether the mean weight of Belgian adults was less than 80 kg, she would have stated \(H_0: \mu = 80\) (or equivalently, \(H_0: \mu \ge 80\) ) and \(H_1: \mu < 80\) . 4 Do not mix the null with the alternative hypothesis, or the conclusions will be diametrically opposed!
- The null hypothesis is often the status quo. For instance, suppose that a doctor wants to test whether the new treatment A is more efficient than the old treatment B. The status quo is that the new and old treatments are equally efficient. Assuming a larger value is better, she will then write \(H_0: \mu_A = \mu_B\) (or equivalently, \(H_0: \mu_A - \mu_B = 0\) ) and \(H_1: \mu_A > \mu_B\) (or equivalently, \(H_0: \mu_A - \mu_B > 0\) ). On the opposite, if the lower the better, she would have written \(H_0: \mu_A = \mu_B\) (or equivalently, \(H_0: \mu_A - \mu_B = 0\) ) and \(H_1: \mu_A < \mu_B\) (or equivalently, \(H_0: \mu_A - \mu_B < 0\) ).
The test statistic (often called t-stat ) is, in some sense, a metric indicating how extreme the observations are compared to the null hypothesis . The higher the t-stat (in absolute value), the more extreme the observations are.
There are several formulas to compute the t-stat, with one formula for each type of hypothesis test—one or two means, one or two proportions, one or two variances. This means that there is a formula to compute the t-stat for a hypothesis test on one mean, another formula for a test on two means, another for a test on one proportion, etc. 5
The only difficulty in this second step is to choose the appropriate formula. As soon as you know which formula to use based on the type of test, you simply have to apply it to the data. For the interested reader, see the different formulas to compute the t-stat for the most common tests in this Shiny app .
Luckily, formulas for hypothesis tests on one and two means, and one and two proportions follow the same structure.
Computing the test statistic for these tests is similar than scaling a random variable (a process also knows as “standardization” or “normalization”) which consists in subtracting the mean from that random variable, and dividing the result by the standard deviation:
\[Z = \frac{X - \mu}{\sigma}\]
For these 4 hypothesis tests (one/two means and one/two proportions), computing the test statistic is like scaling the estimator (computed from the sample) corresponding to the parameter of interest (in the population). So we basically subtract the target parameter from the point estimator and then divide the result by the standard error (which is equivalent to the standard deviation but for an estimator).
If this is unclear, here is how the test statistic (denoted \(t_{obs}\) ) is computed in our scenario (assuming that the variance of the population is unknown):
\[t_{obs} = \frac{\bar{x} - \mu}{\frac{s}{\sqrt{n}}}\]
- \(\bar{x}\) is the sample mean (i.e., the estimator)
- \(\mu\) is the mean under the null hypothesis (i.e., the target parameter)
- \(s\) is the sample standard deviation
- \(n\) is the sample size
- ( \(\frac{s}{\sqrt{n}}\) is the standard error)
Notice the similarity between the formula of this test statistic and the formula used to standardize a random variable. This structure is the same for a test on two means, one proportion and two proportions, except that the estimator, the parameter and the standard error are, of course, slightly different for each type of test.
Suppose that in our case we have a sample mean of 71 kg ( \(\bar{x}\) = 71), a sample standard deviation of 13 kg ( \(s\) = 13) and a sample size of 10 adults ( \(n\) = 10). Remember that the population mean (the mean under the null hypothesis) is 80 kg ( \(\mu\) = 80).
The t-stat is thus:
\[t_{obs} = \frac{\bar{x} - \mu}{\frac{s}{\sqrt{n}}} = \frac{71 - 80}{\frac{13}{\sqrt{10}}} = -2.189\]
Although formulas are different depending on which parameter you are testing, the value found for the test statistic gives us an indication on how extreme our observations are.
We keep this value of -2.189 in mind because it will be used again in step #4.
Although the t-stat gives us an indication of how extreme our observations are, we cannot tell whether this “score of extremity” is too extreme or not based on its value only.
So, at this point, we cannot yet tell whether our data are too extreme or not. For this, we need to compare our t-stat with a threshold—referred as critical value —given by the probability distribution tables (and which can, of course, also be found with R).
In the same way that the formula to compute the t-stat is different for each parameter of interest, the underlying probability distribution—and thus the statistical table—on which the critical value is based is also different for each target parameter. This means that, in addition to choosing the appropriate formula to compute the t-stat, we also need to select the appropriate probability distribution depending on the parameter we are testing.
Luckily, there are only 4 different probability distributions for the 6 hypothesis tests covered in this article (one/two means, one/two proportions and one/two variances):
- test on one and two means with known population variance(s)
- test on two paired samples where the variance of the difference between the 2 samples \(\sigma^2_D\) is known
- test on one and two proportions (given that some assumptions are met)
- test on one and two means with un known population variance(s)
- test on two paired samples where the variance of the difference between the 2 samples \(\sigma^2_D\) is un known
- test on one variance
- test on two variances
Each probability distribution also has its own parameters (up to two parameters for the 4 distribution considered here), defining its shape and/or location. Parameter(s) of a probability distribution can be seen as its DNA; meaning that the distribution is entirely defined by its parameter(s).
Taking our initial scenario—a health professional who would like to test whether the mean weight of Belgian adults is different than 80 kg—as example.
The underlying probability distribution of a test on one mean is either the standard Normal or the Student distribution, depending on whether the variance of the population (not sample variance!) is known or unknown: 6
- If the population variance is known \(\rightarrow\) the standard Normal distribution is used
- If the population variance is un known \(\rightarrow\) the Student distribution is used
If no population variance is explicitly given, you can assume that it is unknown since you cannot compute it based on a sample. If you could compute it, that would mean you have access to the entire population and there is, in this case, no point in performing a hypothesis test (you could simply use some descriptive statistics to confirm or refute your belief).
In our example, no population variance is specified so it is assumed to be unknown. We therefore use the Student distribution.
The Student distribution has one parameter which defines it; the number of degrees of freedom. The number of degrees of freedom depends on the type of hypothesis test. For instance, the number of degrees of freedom for a test on one mean is equal to the number of observations minus one ( \(n\) - 1). Without going too far into the details, the - 1 comes from the fact that there is one quantity which is estimated (i.e., the mean). 7 The sample size being equal to 10 in our example, the degrees of freedom is equal to \(n\) - 1 = 10 - 1 = 9.
There is only one last element missing to find the critical value: the significance level . The significance level , denoted \(\alpha\) , is the probability of wrongly rejecting the null hypothesis, so the probability of rejecting the null hypothesis although it is in reality true . In this sense, it is an error (type I error, as opposed to the type II error 8 ) that we accept to deal with, in order to be able to draw conclusions about a population based on a subset of it.
As you may have read in many statistical textbooks, the significance level is very often set to 5%. 9 In some fields (such as medicine or engineering, among others), the significance level is also sometimes set to 1% to decrease the error rate.
It is best to specify the significance level before performing a hypothesis test to avoid the temptation to set the significance level in accordance to the results (the temptation is even bigger when the results are on the edge of being significant). As I always tell my students, you cannot “guess” nor compute the significance level. Therefore, if it is not explicitly specified, you can safely assume it is 5%. In our case, we did not indicate it, so we take \(\alpha\) = 5% = 0.05.
Furthermore, in our example, we want to test whether the mean weight of Belgian adults is different than 80 kg. Since we do not specify the direction of the test, it is a two-sided test . If we wanted to test that the mean weight was less than 80 kg ( \(H_1: \mu <\) 80) or greater than 80 kg ( \(H_1: \mu >\) 80), we would have done a one-sided test.
Make sure that you perform the correct test (two-sided or one-sided) because it has an impact on how to find the critical value (see more in the following paragraphs).
So now that we know the appropriate distribution (Student distribution), its parameter (degrees of freedom (df) = 9), the significance level ( \(\alpha\) = 0.05) and the direction (two-sided), we have all we need to find the critical value in the statistical tables :
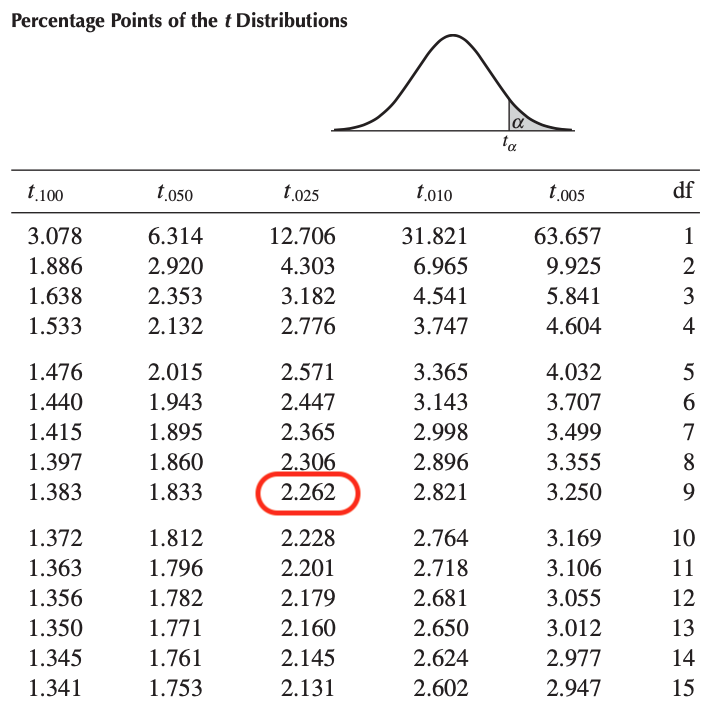
By looking at the row df = 9 and the column \(t_.025\) in the Student’s distribution table, we find a critical value of:
\[t_{n-1; \alpha / 2} = t_{9; 0.025} = 2.262\]
One may wonder why we take \(t_{\alpha/2} = t_.025\) and not \(t_\alpha = t_.05\) since the significance level is 0.05. The reason is that we are doing a two-sided test ( \(H_1: \mu \ne\) 80), so the error rate of 0.05 must be divided in 2 to find the critical value to the right of the distribution. Since the Student’s distribution is symmetric, the critical value to the left of the distribution is simply: -2.262.
Visually, the error rate of 0.05 is partitioned into two parts:
- 0.025 to the left of -2.262 and
- 0.025 to the right of 2.262
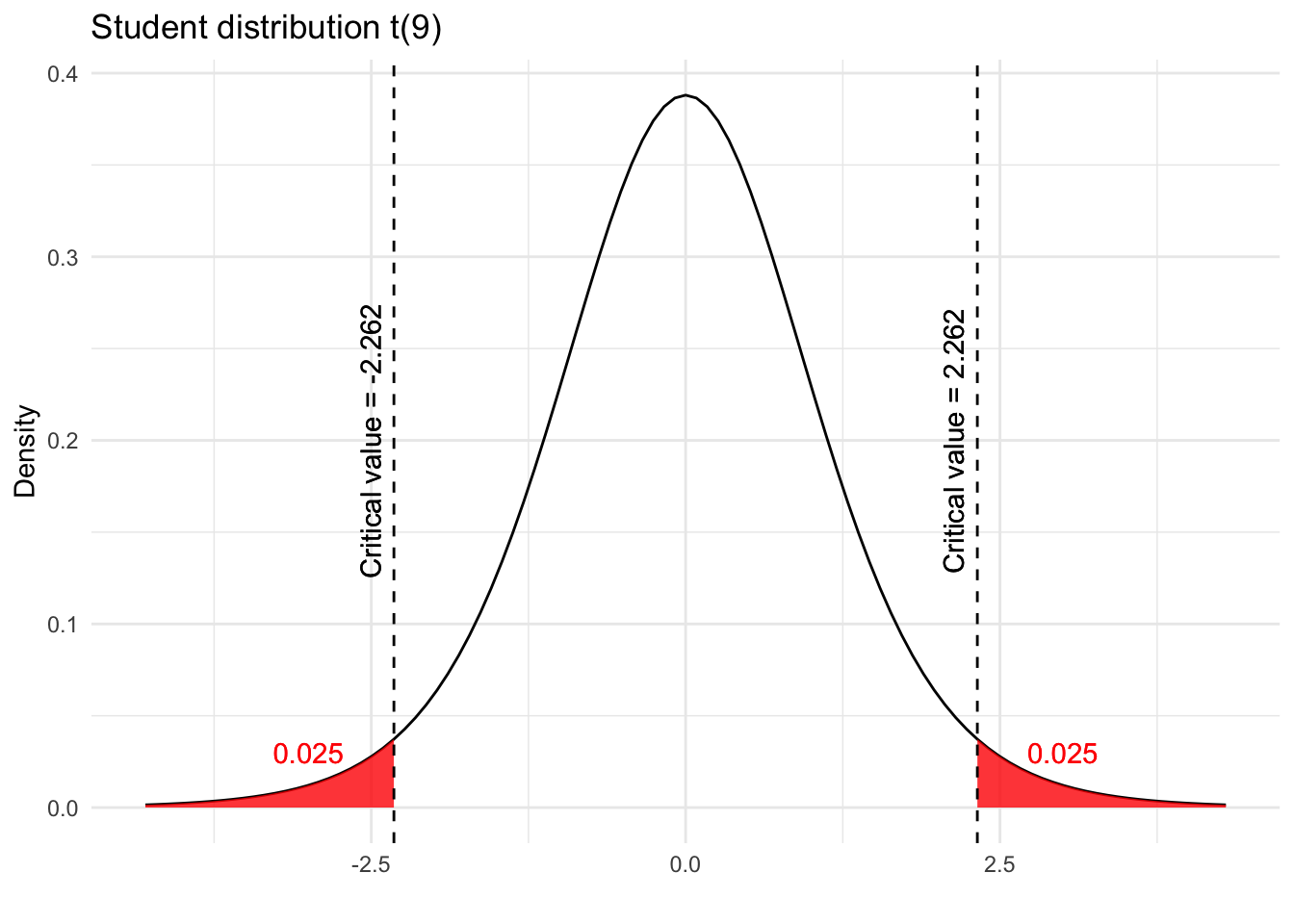
We keep in mind these critical values of -2.262 and 2.262 for the fourth and last step.
Note that the red shaded areas in the previous plot are also known as the rejection regions. More on that in the following section.
These critical values can also be found in R, thanks to the qt() function:
The qt() function is used for the Student’s distribution ( q stands for quantile and t for Student). There are other functions accompanying the different distributions:
- qnorm() for the Normal distribution
- qchisq() for the Chi-square distribution
- qf() for the Fisher distribution
In this fourth and last step, all we have to do is to compare the test statistic (computed in step #2) with the critical values (found in step #3) in order to conclude the hypothesis test .
The only two possibilities when concluding a hypothesis test are:
- Rejection of the null hypothesis
- Non-rejection of the null hypothesis
In our example of adult weight, remember that:
- the t-stat is -2.189
- the critical values are -2.262 and 2.262
Also remember that:
- the t-stat gives an indication on how extreme our sample is compared to the null hypothesis
- the critical values are the threshold from which the t-stat is considered as too extreme
To compare the t-stat with the critical values, I always recommend to plot them:
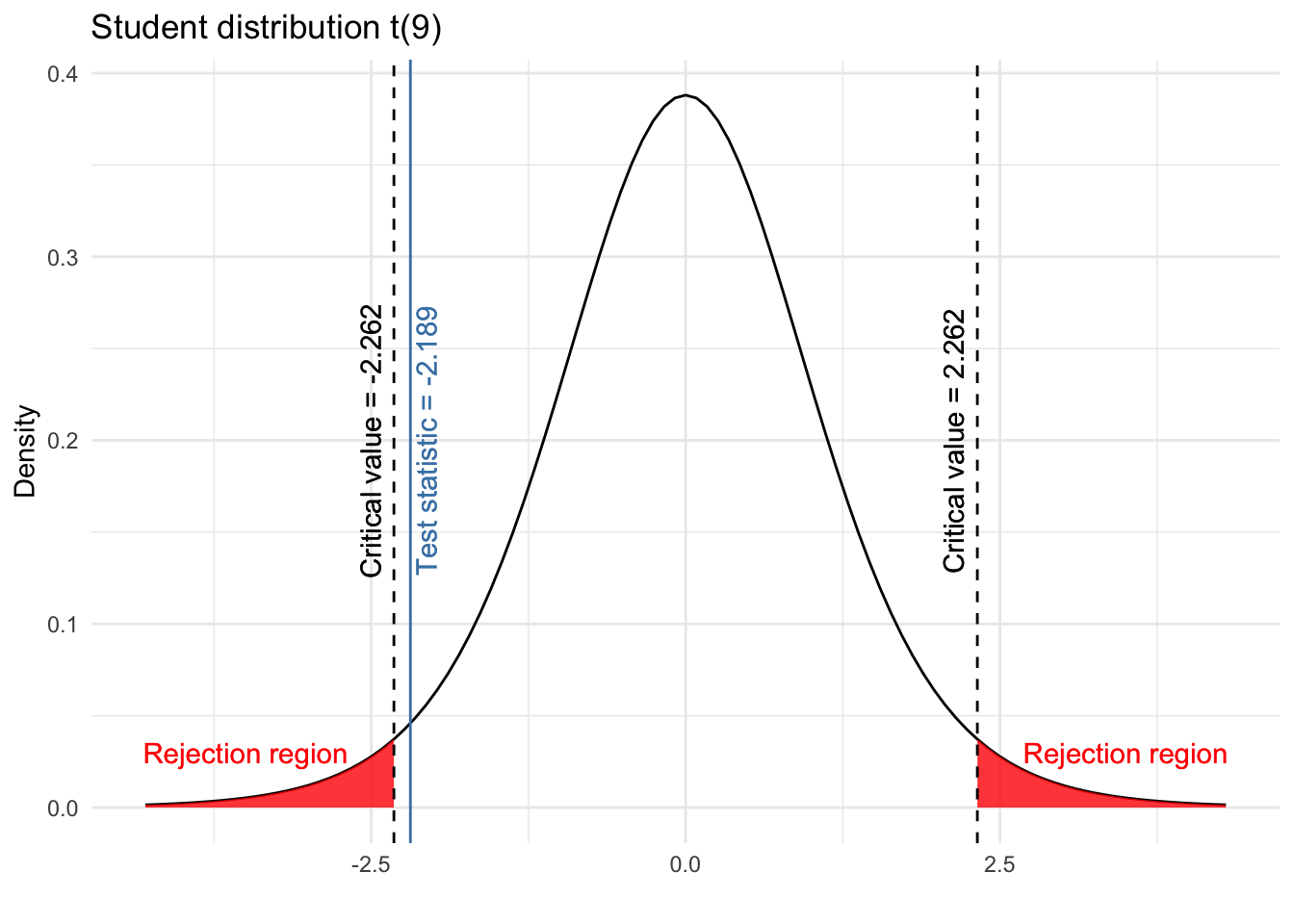
These two critical values form the rejection regions (the red shaded areas):
- from \(- \infty\) to -2.262, and
- from 2.262 to \(\infty\)
If the t-stat lies within one of the rejection region, we reject the null hypothesis . On the contrary, if the t-stat does not lie within any of the rejection region, we do not reject the null hypothesis .
As we can see from the above plot, the t-stat is less extreme than the critical value and therefore does not lie within any of the rejection region. In conclusion, we do not reject the null hypothesis that \(\mu = 80\) .
This is the conclusion in statistical terms but they are meaningless without proper interpretation. So it is a good practice to also interpret the result in the context of the problem:
At the 5% significance level, we do not reject the hypothesis that the mean weight of Belgian adults is 80 kg.
From a more philosophical (but still very important) perspective, note that we wrote “we do not reject the null hypothesis” and “we do not reject the hypothesis that the mean weight of Belgian adults is equal to 80 kg”. We did not write “we accept the null hypothesis” nor “the mean weight of Belgian adults is 80 kg”.
The reason is due to the fact that, in hypothesis testing, we conclude something about the population based on a sample. There is, therefore, always some uncertainty and we cannot be 100% sure that our conclusion is correct.
Perhaps it is the case that the mean weight of Belgian adults is in reality different than 80 kg, but we failed to prove it based on the data at hand. It may be the case that if we had more observations, we would have rejected the null hypothesis (since all else being equal, a larger sample size implies a more extreme t-stat). Or, it may be the case that even with more observations, we would not have rejected the null hypothesis because the mean weight of Belgian adults is in reality close to 80 kg. We cannot distinguish between the two.
So we can just say that we did not find enough evidence against the hypothesis that the mean weight of Belgian adults is 80 kg, but we do not conclude that the mean is equal to 80 kg.
If the difference is still not clear to you, the following example may help. Suppose a person is suspected of having committed a crime. This person is either innocent—the null hypothesis—or guilty—the alternative hypothesis. In the attempt to know if the suspect committed the crime, the police collects as much information and proof as possible. This is similar to the researcher collecting data to form a sample. And then the judge, based on the collected evidence, decides whether the suspect is considered as innocent or guilty. If there is enough evidence that the suspect committed the crime, the judge will conclude that the suspect is guilty. In other words, she will reject the null hypothesis of the suspect being innocent because there are enough evidence that the suspect committed the crime.
This is similar to the t-stat being more extreme than the critical value: we have enough information (based on the sample) to say that the null hypothesis is unlikely because our data would be too extreme if the null hypothesis were true. Since the sample cannot be “wrong” (it corresponds to the collected data), the only remaining possibility is that the null hypothesis is in fact wrong. This is the reason we write “we reject the null hypothesis”.
On the other hand, if there is not enough evidence that the suspect committed the crime (or no evidence at all), the judge will conclude that the suspect is considered as not guilty. In other words, she will not reject the null hypothesis of the suspect being innocent. But even if she concludes that the suspect is considered as not guilty, she will never be 100% sure that he is really innocent.
It may be the case that:
- the suspect did not commit the crime, or
- the suspect committed the crime but the police was not able to collect enough information against the suspect.
In the former case the suspect is really innocent, whereas in the latter case the suspect is guilty but the police and the judge failed to prove it because they failed to find enough evidence against him. Similar to hypothesis testing, the judge has to conclude the case by considering the suspect not guilty, without being able to distinguish between the two.
This is the main reason we write “we do not reject the null hypothesis” or “we fail to reject the null hypothesis” (you may even read in some textbooks conclusion such as “there is no sufficient evidence in the data to reject the null hypothesis”), and we do not write “we accept the null hypothesis”.
I hope this metaphor helped you to understand the reason why we reject the null hypothesis instead of accepting it.
In the following sections, we present two other methods used in hypothesis testing.
These methods will result in the exact same conclusion: non-rejection of the null hypothesis, that is, we do not reject the hypothesis that the mean weight of Belgian adults is 80 kg. It is thus presented only if you prefer to use these methods over the first one.
Method B, which consists in computing the p -value and comparing this p -value with the significance level \(\alpha\) , boils down to the following 4 steps:
- Computing the p -value
In this second method which uses the p -value, the first and second steps are similar than in the first method.
The null and alternative hypotheses remain the same:
- \(H_0: \mu = 80\)
- \(H_1: \mu \ne 80\)
Remember that the formula for the t-stat is different depending on the type of hypothesis test (one or two means, one or two proportions, one or two variances). In our case of one mean with unknown variance, we have:
The p -value is the probability (so it goes from 0 to 1) of observing a sample at least as extreme as the one we observed if the null hypothesis were true. In some sense, it gives you an indication on how likely your null hypothesis is . It is also defined as the smallest level of significance for which the data indicate rejection of the null hypothesis.
For more information about the p -value, I recommend reading this note about the p -value and the significance level \(\alpha\) .
Formally, the p -value is the area beyond the test statistic. Since we are doing a two-sided test, the p -value is thus the sum of the area above 2.189 and below -2.189.
Visually, the p -value is the sum of the two blue shaded areas in the following plot:
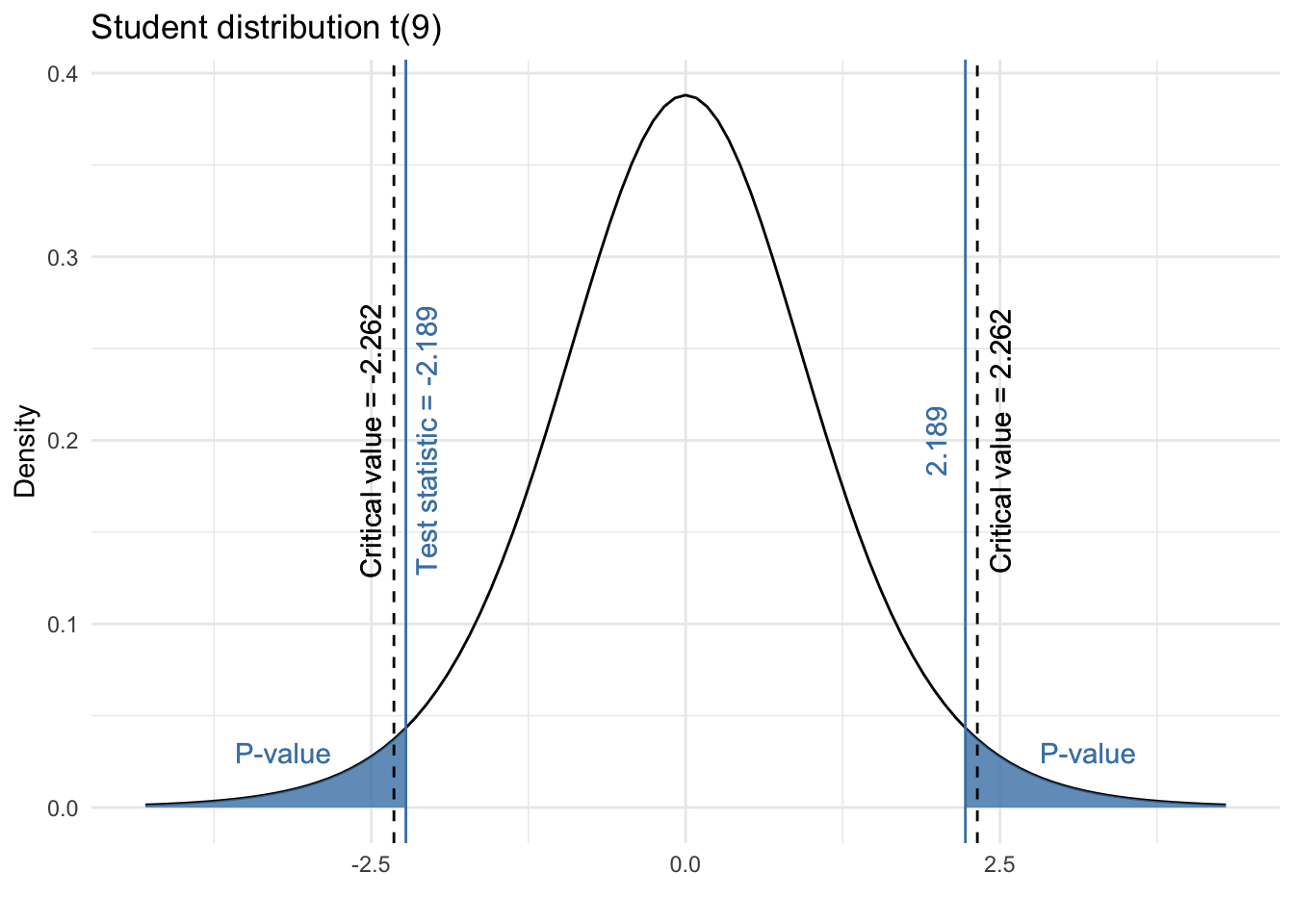
The p -value can computed with precision in R with the pt() function:
The p -value is 0.0563, which indicates that there is a 5.63% chance to observe a sample at least as extreme as the one observed if the null hypothesis were true. This already gives us a hint on whether our t-stat is too extreme or not (and thus whether our null hypothesis is likely or not), but we formally conclude in step #4.
Like the qt() function to find the critical value, we use pt() to find the p -value because the underlying distribution is the Student’s distribution.
Use pnorm() , pchisq() and pf() for the Normal, Chi-square and Fisher distribution, respectively. See also this Shiny app to compute the p -value given a certain t-stat for most probability distributions.
If you do not have access to a computer (during exams for example) you will not be able to compute the p -value precisely, but you can bound it using the statistical table referring to your test.
In our case, we use the Student distribution and we look at the row df = 9 (since df = n - 1):
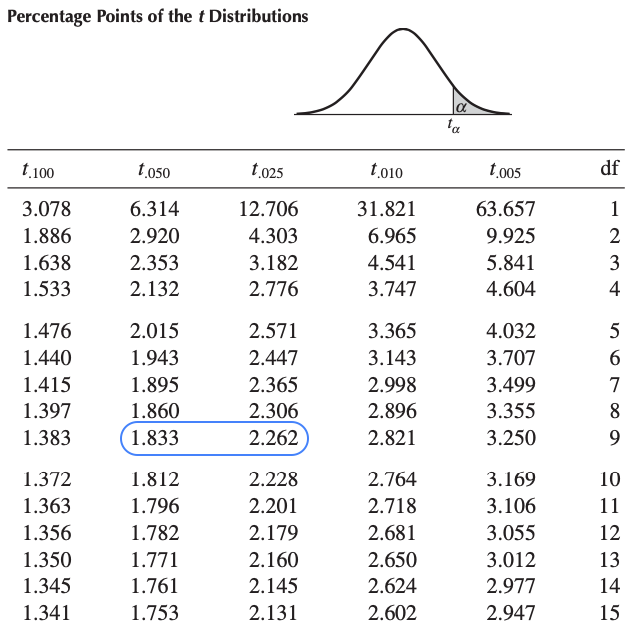
- The test statistic is -2.189
- We take the absolute value, which gives 2.189
- The value 2.189 is between 1.833 and 2.262 (highlighted in blue in the above table)
- the area to the right of 1.833 is 0.05
- the area to the right of 2.262 is 0.025
- So we know that the area to the right of 2.189 must be between 0.025 and 0.05
- Since the Student distribution is symmetric, we know that the area to the left of -2.189 must also be between 0.025 and 0.05
- Therefore, the sum of the two areas must be between 0.05 and 0.10
- In other words, the p -value is between 0.05 and 0.10 (i.e., 0.05 < p -value < 0.10)
Although we could not compute it precisely, it is enough to conclude our hypothesis test in the last step.
The final step is now to simply compare the p -value (computed in step #3) with the significance level \(\alpha\) . As for all statistical tests :
- If the p -value is smaller than \(\alpha\) ( p -value < 0.05) \(\rightarrow H_0\) is unlikely \(\rightarrow\) we reject the null hypothesis
- If the p -value is greater than or equal to \(\alpha\) ( p -value \(\ge\) 0.05) \(\rightarrow H_0\) is likely \(\rightarrow\) we do not reject the null hypothesis
No matter if we take into consideration the exact p -value (i.e., 0.0563) or the bounded one (0.05 < p -value < 0.10), it is larger than 0.05, so we do not reject the null hypothesis. 10 In the context of the problem, we do not reject the null hypothesis that the mean weight of Belgian adults is 80 kg.
Remember that rejecting (or not rejecting) a null hypothesis at the significance level \(\alpha\) using the critical value method (method A) is equivalent to rejecting (or not rejecting) the null hypothesis when the p -value is lower (equal or greater) than \(\alpha\) (method B).
This is the reason we find the exact same conclusion than with method A, and why you should too if you use both methods on the same data and with the same significance level.
Method C, which consists in computing the confidence interval and comparing this confidence interval with the target parameter (the parameter under the null hypothesis), boils down to the following 3 steps:
- Computing the confidence interval
In this last method which uses the confidence interval, the first step is similar than in the first two methods.
Like hypothesis testing, confidence intervals are a well-known tool in inferential statistics.
Confidence interval is an estimation procedure which produces an interval (i.e., a range of values) containing the true parameter with a certain —usually high— probability .
In the same way that there is a formula for each type of hypothesis test when computing the test statistics, there exists a formula for each type of confidence interval. Formulas for the different types of confidence intervals can be found in this Shiny app .
Here is the formula for a confidence interval on one mean \(\mu\) (with unknown population variance):
\[ (1-\alpha)\text{% CI for } \mu = \bar{x} \pm t_{\alpha/2, n - 1} \frac{s}{\sqrt{n}} \]
where \(t_{\alpha/2, n - 1}\) is found in the Student distribution table (and is similar to the critical value found in step #3 of method A).
Given our data and with \(\alpha\) = 0.05, we have:
\[ \begin{aligned} 95\text{% CI for } \mu &= \bar{x} \pm t_{\alpha/2, n - 1} \frac{s}{\sqrt{n}} \\ &= 71 \pm 2.262 \frac{13}{\sqrt{10}} \\ &= [61.70; 80.30] \end{aligned} \]
The 95% confidence interval for \(\mu\) is [61.70; 80.30] kg. But what does a 95% confidence interval mean?
We know that this estimation procedure has a 95% probability of producing an interval containing the true mean \(\mu\) . In other words, if we construct many confidence intervals (with different samples of the same size), 95% of them will , on average, include the mean of the population (the true parameter). So on average, 5% of these confidence intervals will not cover the true mean.
If you wish to decrease this last percentage, you can decrease the significance level (set \(\alpha\) = 0.01 or 0.02 for instance). All else being equal, this will increase the range of the confidence interval and thus increase the probability that it includes the true parameter.
The final step is simply to compare the confidence interval (constructed in step #2) with the value of the target parameter (the value under the null hypothesis, mentioned in step #1):
- If the confidence interval does not include the hypothesized value \(\rightarrow H_0\) is unlikely \(\rightarrow\) we reject the null hypothesis
- If the confidence interval includes the hypothesized value \(\rightarrow H_0\) is likely \(\rightarrow\) we do not reject the null hypothesis
In our example:
- the hypothesized value is 80 (since \(H_0: \mu\) = 80)
- 80 is included in the 95% confidence interval since it goes from 61.70 to 80.30 kg
- So we do not reject the null hypothesis
In the terms of the problem, we do not reject the hypothesis that the mean weight of Belgian adults is 80 kg.
As you can see, the conclusion is equivalent than with the critical value method (method A) and the p -value method (method B). Again, this must be the case since we use the same data and the same significance level \(\alpha\) for all three methods.
All three methods give the same conclusion. However, each method has its own advantage so I usually select the most convenient one depending on the situation:
- It is, in my opinion, the easiest and most straightforward method of the three when I do not have access to R.
- In addition to being able to know whether the null hypothesis is rejected or not, computing the exact p -value can be very convenient so I tend to use this method if I have access to R.
- If I need to test several hypothesized values , I tend to choose this method because I can construct one single confidence interval and compare it to as many values as I want. For example, with our 95% confidence interval [61.70; 80.30], I know that any value below 61.70 kg and above 80.30 kg will be rejected, without testing it for each value.
In this article, we reviewed the goals and when hypothesis testing is used. We then showed how to do a hypothesis test by hand through three different methods (A. critical value , B. p -value and C. confidence interval ). We also showed how to interpret the results in the context of the initial problem.
Although all three methods give the exact same conclusion when using the same data and the same significance level (otherwise there is a mistake somewhere), I also presented my personal preferences when it comes to choosing one method over the other two.
Thanks for reading.
I hope this article helped you to understand the structure of a hypothesis by hand. I remind you that, at least for the 6 hypothesis tests covered in this article, the formulas are different, but the structure and the reasoning behind it remain the same. So you basically have to know which formulas to use, and simply follow the steps mentioned in this article.
For the interested reader, I created two accompanying Shiny apps:
- Hypothesis testing and confidence intervals : after entering your data, the app illustrates all the steps in order to conclude the test and compute a confidence interval. See more information in this article .
- How to read statistical tables : the app helps you to compute the p -value given a t-stat for most probability distributions. See more information in this article .
As always, if you have a question or a suggestion related to the topic covered in this article, please add it as a comment so other readers can benefit from the discussion.
Suppose a researcher wants to test whether Belgian women are taller than French women. Suppose a health professional would like to know whether the proportion of smokers is different among athletes and non-athletes. It would take way too long to measure the height of all Belgian and French women and to ask all athletes and non-athletes their smoking habits. So most of the time, decisions are based on a representative sample of the population and not on the whole population. If we could measure the entire population in a reasonable time frame, we would not do any inferential statistics. ↩︎
Don’t get me wrong, this does not mean that hypothesis tests are never used in exploratory analyses. It is just much less frequent in exploratory research than in confirmatory research. ↩︎
You may see more or less steps in other articles or textbooks, depending on whether these steps are detailed or concise. Hypothesis testing should, however, follows the same process regardless of the number of steps. ↩︎
For one-sided tests, writing \(H_0: \mu = 80\) or \(H_0: \mu \ge 80\) are both correct. The point is that the null and alternative hypothesis must be mutually exclusive since you are testing one hypothesis against the other, so both cannot be true at the same time. ↩︎
To be complete, there are even different formulas within each type of test, depending on whether some assumptions are met or not. For the interested reader, see all the different scenarios and thus the different formulas for a test on one mean and on two means . ↩︎
There are more uncertainty if the population variance is unknown than if it is known, and this greater uncertainty is taken into account by using the Student distribution instead of the standard Normal distribution. Also note that as the sample size increases, the degrees of freedom of the Student distribution increases and the two distributions become more and more similar. For large sample size (usually from \(n >\) 30), the Student distribution becomes so close to the standard Normal distribution that, even if the population variance is unknown, the standard Normal distribution can be used. ↩︎
For a test on two independent samples, the degrees of freedom is \(n_1 + n_2 - 2\) , where \(n_1\) and \(n_2\) are the size of the first and second sample, respectively. Note the - 2 due to the fact that in this case, two quantities are estimated. ↩︎
The type II error is the probability of not rejecting the null hypothesis although it is in reality false. ↩︎
Whether this is a good or a bad standard is a question that comes up often and is debatable. This is, however, beyond the scope of the article. ↩︎
Again, p -values found via a statistical table or via R must be coherent. ↩︎
Related articles
- One-sample Wilcoxon test in R
- Correlation coefficient and correlation test in R
- One-proportion and chi-square goodness of fit test
- How to perform a one-sample t-test by hand and in R: test on one mean
Liked this post?
- Get updates every time a new article is published (no spam and unsubscribe anytime):
Yes, receive new posts by email
- Support the blog
FAQ Contribute Sitemap
Purdue Online Writing Lab Purdue OWL® College of Liberal Arts
APA Stylistics: Basics
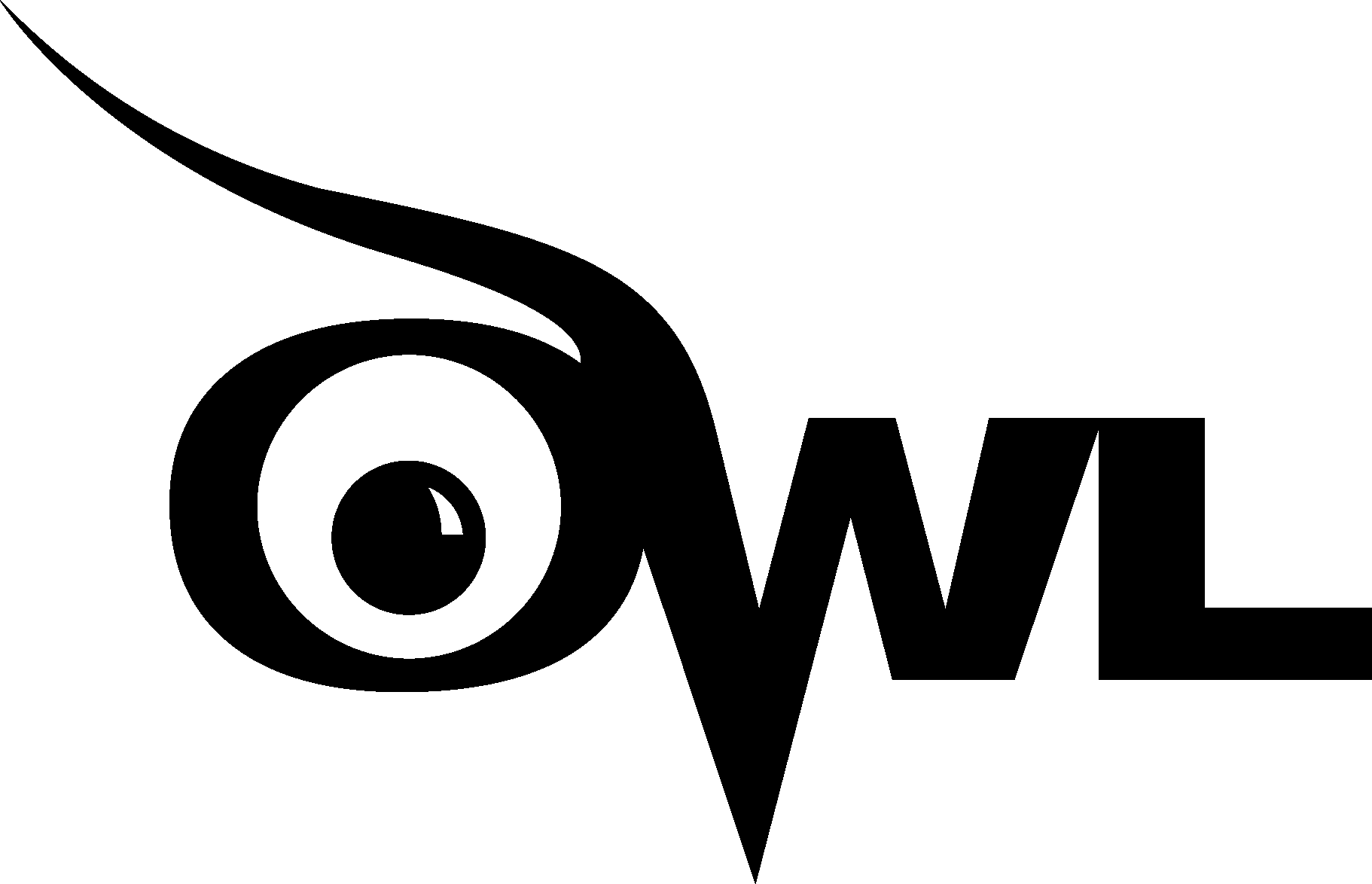
Welcome to the Purdue OWL
This page is brought to you by the OWL at Purdue University. When printing this page, you must include the entire legal notice.
Copyright ©1995-2018 by The Writing Lab & The OWL at Purdue and Purdue University. All rights reserved. This material may not be published, reproduced, broadcast, rewritten, or redistributed without permission. Use of this site constitutes acceptance of our terms and conditions of fair use.
Note: This page reflects APA 6, which is now out of date. It will remain online until 2021, but will not be updated. There is currently no equivalent 7th edition page, but we're working on one. Thank you for your patience. Here is a link to our APA 7 "General Format" page .
Writing in APA is more than simply learning the formula for citations or following a certain page layout. APA also includes the stylistics of your writing, from point of view to word choice.
Point of View and Voice
When writing in APA Style, you can use the first person point of view when discussing your research steps ("I studied ...") and when referring to yourself and your co-authors ("We examined the literature ..."). Use first person to discuss research steps rather than anthropomorphising the work. For example, a study cannot "control" or "interpret"; you and your co-authors, however, can.
In general, you should foreground the research and not the researchers ("The results indicate ... "). Avoid using the editorial "we"; if you use "we" in your writing, be sure that "we" refers to you and your fellow researchers.
It is a common misconception that foregrounding the research requires using the passive voice ("Experiments have been conducted ..."). This is inaccurate. Rather, you would use pronouns in place of "experiments" ("We conducted experiments ...").
APA Style encourages using the active voice ("We interpreted the results ..."). The active voice is particularly important in experimental reports, where the subject performing the action should be clearly identified (e.g. "We interviewed ..." vs. "The participants responded ...").
Consult the OWL handout for more on the distinction between passive and active voice .
Switching verb tenses can cause confusion for your readers, so you should be consistent in the tense you use. When discussing literature reviews and experimental procedures that have already happened, use past tense ("Our study showed" ) or present perfect tense ("studies have proven" ). Also use past tense when discussing results ("students’ concentration increased" ), but use present tense when discussing what your results mean and what conclusions you can draw from them ("Our study illustrates" ).
Clarity and Conciseness
Clarity and conciseness in writing are important when conveying research in APA Style. You don't want to misrepresent the details of a study or confuse your readers with wordiness or unnecessarily complex sentences.
For clarity, be specific rather than vague in descriptions and explanations. Unpack details accurately to provide adequate information to your readers so they can follow the development of your study.
Example: "It was predicted that marital conflict would predict behavior problems in school-aged children."
To clarify this vague hypothesis, use parallel structure to outline specific ideas:
"The first hypothesis stated that marital conflict would predict behavior problems in school-aged children. The second hypothesis stated that the effect would be stronger for girls than for boys. The third hypothesis stated that older girls would be more affected by marital conflict than younger girls."
To be more concise, particularly in introductory material or abstracts, you should eliminate unnecessary words and condense information when you can (see the OWL handout on Conciseness in academic writing for suggestions).
Example: The above list of hypotheses might be rephrased concisely as: "The authors wanted to investigate whether marital conflict would predict behavior problems in children and they wanted to know if the effect was greater for girls than for boys, particularly when they examined two different age groups of girls."
Balancing the need for clarity, which can require unpacking information, and the need for conciseness, which requires condensing information, is a challenge. Study published articles and reports in your field for examples of how to achieve this balance.
Word Choice
You should even be careful in selecting certain words or terms. Within the social sciences, commonly used words take on different meanings and can have a significant effect on how your readers interpret your reported findings or claims. To increase clarity, avoid bias, and control how your readers will receive your information, you should make certain substitutions:
- Use terms like "participants" or "respondents" (rather than "subjects") to indicate how individuals were involved in your research
- Use terms like "children" or "community members" to provide more detail about who was participating in the study
- Use phrases like "The evidence suggests ..." or "Our study indicates ..." rather than referring to "proof" or "proves" because no single study can prove a theory or hypothesis
As with the other stylistic suggestions here, you should study the discourse of your field to see what terminology is most often used.
Avoiding Poetic Language
Writing papers in APA Style is unlike writing in more creative or literary styles that draw on poetic expressions and figurative language. Such linguistic devices can detract from conveying your information clearly and may come across to readers as forced when it is inappropriately used to explain an issue or your findings.
Therefore, you should:
- Minimize the amount of figurative language used in an APA paper, such as metaphors and analogies unless they are helpful in conveying a complex idea,
- Avoid rhyming schemes, alliteration, or other poetic devices typically found in verse
- Use simple, descriptive adjectives and plain language that does not risk confusing your meaning.
- Comprehensive Learning Paths
- 150+ Hours of Videos
- Complete Access to Jupyter notebooks, Datasets, References.

Hypothesis Testing – A Deep Dive into Hypothesis Testing, The Backbone of Statistical Inference
- September 21, 2023
Explore the intricacies of hypothesis testing, a cornerstone of statistical analysis. Dive into methods, interpretations, and applications for making data-driven decisions.

In this Blog post we will learn:
- What is Hypothesis Testing?
- Steps in Hypothesis Testing 2.1. Set up Hypotheses: Null and Alternative 2.2. Choose a Significance Level (α) 2.3. Calculate a test statistic and P-Value 2.4. Make a Decision
- Example : Testing a new drug.
- Example in python
1. What is Hypothesis Testing?
In simple terms, hypothesis testing is a method used to make decisions or inferences about population parameters based on sample data. Imagine being handed a dice and asked if it’s biased. By rolling it a few times and analyzing the outcomes, you’d be engaging in the essence of hypothesis testing.
Think of hypothesis testing as the scientific method of the statistics world. Suppose you hear claims like “This new drug works wonders!” or “Our new website design boosts sales.” How do you know if these statements hold water? Enter hypothesis testing.
2. Steps in Hypothesis Testing
- Set up Hypotheses : Begin with a null hypothesis (H0) and an alternative hypothesis (Ha).
- Choose a Significance Level (α) : Typically 0.05, this is the probability of rejecting the null hypothesis when it’s actually true. Think of it as the chance of accusing an innocent person.
- Calculate Test statistic and P-Value : Gather evidence (data) and calculate a test statistic.
- p-value : This is the probability of observing the data, given that the null hypothesis is true. A small p-value (typically ≤ 0.05) suggests the data is inconsistent with the null hypothesis.
- Decision Rule : If the p-value is less than or equal to α, you reject the null hypothesis in favor of the alternative.
2.1. Set up Hypotheses: Null and Alternative
Before diving into testing, we must formulate hypotheses. The null hypothesis (H0) represents the default assumption, while the alternative hypothesis (H1) challenges it.
For instance, in drug testing, H0 : “The new drug is no better than the existing one,” H1 : “The new drug is superior .”
2.2. Choose a Significance Level (α)
When You collect and analyze data to test H0 and H1 hypotheses. Based on your analysis, you decide whether to reject the null hypothesis in favor of the alternative, or fail to reject / Accept the null hypothesis.
The significance level, often denoted by $α$, represents the probability of rejecting the null hypothesis when it is actually true.
In other words, it’s the risk you’re willing to take of making a Type I error (false positive).
Type I Error (False Positive) :
- Symbolized by the Greek letter alpha (α).
- Occurs when you incorrectly reject a true null hypothesis . In other words, you conclude that there is an effect or difference when, in reality, there isn’t.
- The probability of making a Type I error is denoted by the significance level of a test. Commonly, tests are conducted at the 0.05 significance level , which means there’s a 5% chance of making a Type I error .
- Commonly used significance levels are 0.01, 0.05, and 0.10, but the choice depends on the context of the study and the level of risk one is willing to accept.
Example : If a drug is not effective (truth), but a clinical trial incorrectly concludes that it is effective (based on the sample data), then a Type I error has occurred.
Type II Error (False Negative) :
- Symbolized by the Greek letter beta (β).
- Occurs when you accept a false null hypothesis . This means you conclude there is no effect or difference when, in reality, there is.
- The probability of making a Type II error is denoted by β. The power of a test (1 – β) represents the probability of correctly rejecting a false null hypothesis.
Example : If a drug is effective (truth), but a clinical trial incorrectly concludes that it is not effective (based on the sample data), then a Type II error has occurred.
Balancing the Errors :
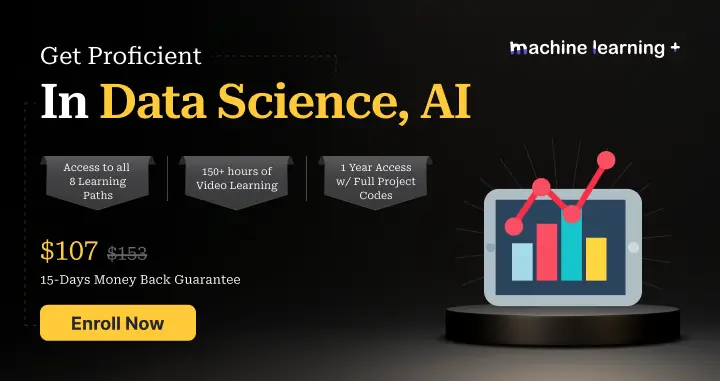
In practice, there’s a trade-off between Type I and Type II errors. Reducing the risk of one typically increases the risk of the other. For example, if you want to decrease the probability of a Type I error (by setting a lower significance level), you might increase the probability of a Type II error unless you compensate by collecting more data or making other adjustments.
It’s essential to understand the consequences of both types of errors in any given context. In some situations, a Type I error might be more severe, while in others, a Type II error might be of greater concern. This understanding guides researchers in designing their experiments and choosing appropriate significance levels.
2.3. Calculate a test statistic and P-Value
Test statistic : A test statistic is a single number that helps us understand how far our sample data is from what we’d expect under a null hypothesis (a basic assumption we’re trying to test against). Generally, the larger the test statistic, the more evidence we have against our null hypothesis. It helps us decide whether the differences we observe in our data are due to random chance or if there’s an actual effect.
P-value : The P-value tells us how likely we would get our observed results (or something more extreme) if the null hypothesis were true. It’s a value between 0 and 1. – A smaller P-value (typically below 0.05) means that the observation is rare under the null hypothesis, so we might reject the null hypothesis. – A larger P-value suggests that what we observed could easily happen by random chance, so we might not reject the null hypothesis.
2.4. Make a Decision
Relationship between $α$ and P-Value
When conducting a hypothesis test:
We then calculate the p-value from our sample data and the test statistic.
Finally, we compare the p-value to our chosen $α$:
- If $p−value≤α$: We reject the null hypothesis in favor of the alternative hypothesis. The result is said to be statistically significant.
- If $p−value>α$: We fail to reject the null hypothesis. There isn’t enough statistical evidence to support the alternative hypothesis.
3. Example : Testing a new drug.
Imagine we are investigating whether a new drug is effective at treating headaches faster than drug B.
Setting Up the Experiment : You gather 100 people who suffer from headaches. Half of them (50 people) are given the new drug (let’s call this the ‘Drug Group’), and the other half are given a sugar pill, which doesn’t contain any medication.
- Set up Hypotheses : Before starting, you make a prediction:
- Null Hypothesis (H0): The new drug has no effect. Any difference in healing time between the two groups is just due to random chance.
- Alternative Hypothesis (H1): The new drug does have an effect. The difference in healing time between the two groups is significant and not just by chance.
Calculate Test statistic and P-Value : After the experiment, you analyze the data. The “test statistic” is a number that helps you understand the difference between the two groups in terms of standard units.
For instance, let’s say:
- The average healing time in the Drug Group is 2 hours.
- The average healing time in the Placebo Group is 3 hours.
The test statistic helps you understand how significant this 1-hour difference is. If the groups are large and the spread of healing times in each group is small, then this difference might be significant. But if there’s a huge variation in healing times, the 1-hour difference might not be so special.
Imagine the P-value as answering this question: “If the new drug had NO real effect, what’s the probability that I’d see a difference as extreme (or more extreme) as the one I found, just by random chance?”
For instance:
- P-value of 0.01 means there’s a 1% chance that the observed difference (or a more extreme difference) would occur if the drug had no effect. That’s pretty rare, so we might consider the drug effective.
- P-value of 0.5 means there’s a 50% chance you’d see this difference just by chance. That’s pretty high, so we might not be convinced the drug is doing much.
- If the P-value is less than ($α$) 0.05: the results are “statistically significant,” and they might reject the null hypothesis , believing the new drug has an effect.
- If the P-value is greater than ($α$) 0.05: the results are not statistically significant, and they don’t reject the null hypothesis , remaining unsure if the drug has a genuine effect.
4. Example in python
For simplicity, let’s say we’re using a t-test (common for comparing means). Let’s dive into Python:
Making a Decision : “The results are statistically significant! p-value < 0.05 , The drug seems to have an effect!” If not, we’d say, “Looks like the drug isn’t as miraculous as we thought.”
5. Conclusion
Hypothesis testing is an indispensable tool in data science, allowing us to make data-driven decisions with confidence. By understanding its principles, conducting tests properly, and considering real-world applications, you can harness the power of hypothesis testing to unlock valuable insights from your data.
More Articles
Correlation – connecting the dots, the role of correlation in data analysis, sampling and sampling distributions – a comprehensive guide on sampling and sampling distributions, law of large numbers – a deep dive into the world of statistics, central limit theorem – a deep dive into central limit theorem and its significance in statistics, skewness and kurtosis – peaks and tails, understanding data through skewness and kurtosis”, similar articles, complete introduction to linear regression in r, how to implement common statistical significance tests and find the p value, logistic regression – a complete tutorial with examples in r.
Subscribe to Machine Learning Plus for high value data science content
© Machinelearningplus. All rights reserved.

Machine Learning A-Z™: Hands-On Python & R In Data Science
Free sample videos:.

- Skip to secondary menu
- Skip to main content
- Skip to primary sidebar
Statistics By Jim
Making statistics intuitive
Statistical Hypothesis Testing Overview
By Jim Frost 59 Comments
In this blog post, I explain why you need to use statistical hypothesis testing and help you navigate the essential terminology. Hypothesis testing is a crucial procedure to perform when you want to make inferences about a population using a random sample. These inferences include estimating population properties such as the mean, differences between means, proportions, and the relationships between variables.
This post provides an overview of statistical hypothesis testing. If you need to perform hypothesis tests, consider getting my book, Hypothesis Testing: An Intuitive Guide .
Why You Should Perform Statistical Hypothesis Testing

Hypothesis testing is a form of inferential statistics that allows us to draw conclusions about an entire population based on a representative sample. You gain tremendous benefits by working with a sample. In most cases, it is simply impossible to observe the entire population to understand its properties. The only alternative is to collect a random sample and then use statistics to analyze it.
While samples are much more practical and less expensive to work with, there are trade-offs. When you estimate the properties of a population from a sample, the sample statistics are unlikely to equal the actual population value exactly. For instance, your sample mean is unlikely to equal the population mean. The difference between the sample statistic and the population value is the sample error.
Differences that researchers observe in samples might be due to sampling error rather than representing a true effect at the population level. If sampling error causes the observed difference, the next time someone performs the same experiment the results might be different. Hypothesis testing incorporates estimates of the sampling error to help you make the correct decision. Learn more about Sampling Error .
For example, if you are studying the proportion of defects produced by two manufacturing methods, any difference you observe between the two sample proportions might be sample error rather than a true difference. If the difference does not exist at the population level, you won’t obtain the benefits that you expect based on the sample statistics. That can be a costly mistake!
Let’s cover some basic hypothesis testing terms that you need to know.
Background information : Difference between Descriptive and Inferential Statistics and Populations, Parameters, and Samples in Inferential Statistics
Hypothesis Testing
Hypothesis testing is a statistical analysis that uses sample data to assess two mutually exclusive theories about the properties of a population. Statisticians call these theories the null hypothesis and the alternative hypothesis. A hypothesis test assesses your sample statistic and factors in an estimate of the sample error to determine which hypothesis the data support.
When you can reject the null hypothesis, the results are statistically significant, and your data support the theory that an effect exists at the population level.
The effect is the difference between the population value and the null hypothesis value. The effect is also known as population effect or the difference. For example, the mean difference between the health outcome for a treatment group and a control group is the effect.
Typically, you do not know the size of the actual effect. However, you can use a hypothesis test to help you determine whether an effect exists and to estimate its size. Hypothesis tests convert your sample effect into a test statistic, which it evaluates for statistical significance. Learn more about Test Statistics .
An effect can be statistically significant, but that doesn’t necessarily indicate that it is important in a real-world, practical sense. For more information, read my post about Statistical vs. Practical Significance .
Null Hypothesis
The null hypothesis is one of two mutually exclusive theories about the properties of the population in hypothesis testing. Typically, the null hypothesis states that there is no effect (i.e., the effect size equals zero). The null is often signified by H 0 .
In all hypothesis testing, the researchers are testing an effect of some sort. The effect can be the effectiveness of a new vaccination, the durability of a new product, the proportion of defect in a manufacturing process, and so on. There is some benefit or difference that the researchers hope to identify.
However, it’s possible that there is no effect or no difference between the experimental groups. In statistics, we call this lack of an effect the null hypothesis. Therefore, if you can reject the null, you can favor the alternative hypothesis, which states that the effect exists (doesn’t equal zero) at the population level.
You can think of the null as the default theory that requires sufficiently strong evidence against in order to reject it.
For example, in a 2-sample t-test, the null often states that the difference between the two means equals zero.
When you can reject the null hypothesis, your results are statistically significant. Learn more about Statistical Significance: Definition & Meaning .
Related post : Understanding the Null Hypothesis in More Detail
Alternative Hypothesis
The alternative hypothesis is the other theory about the properties of the population in hypothesis testing. Typically, the alternative hypothesis states that a population parameter does not equal the null hypothesis value. In other words, there is a non-zero effect. If your sample contains sufficient evidence, you can reject the null and favor the alternative hypothesis. The alternative is often identified with H 1 or H A .
For example, in a 2-sample t-test, the alternative often states that the difference between the two means does not equal zero.
You can specify either a one- or two-tailed alternative hypothesis:
If you perform a two-tailed hypothesis test, the alternative states that the population parameter does not equal the null value. For example, when the alternative hypothesis is H A : μ ≠ 0, the test can detect differences both greater than and less than the null value.
A one-tailed alternative has more power to detect an effect but it can test for a difference in only one direction. For example, H A : μ > 0 can only test for differences that are greater than zero.
Related posts : Understanding T-tests and One-Tailed and Two-Tailed Hypothesis Tests Explained

P-values are the probability that you would obtain the effect observed in your sample, or larger, if the null hypothesis is correct. In simpler terms, p-values tell you how strongly your sample data contradict the null. Lower p-values represent stronger evidence against the null. You use P-values in conjunction with the significance level to determine whether your data favor the null or alternative hypothesis.
Related post : Interpreting P-values Correctly
Significance Level (Alpha)

For instance, a significance level of 0.05 signifies a 5% risk of deciding that an effect exists when it does not exist.
Use p-values and significance levels together to help you determine which hypothesis the data support. If the p-value is less than your significance level, you can reject the null and conclude that the effect is statistically significant. In other words, the evidence in your sample is strong enough to be able to reject the null hypothesis at the population level.
Related posts : Graphical Approach to Significance Levels and P-values and Conceptual Approach to Understanding Significance Levels
Types of Errors in Hypothesis Testing
Statistical hypothesis tests are not 100% accurate because they use a random sample to draw conclusions about entire populations. There are two types of errors related to drawing an incorrect conclusion.
- False positives: You reject a null that is true. Statisticians call this a Type I error . The Type I error rate equals your significance level or alpha (α).
- False negatives: You fail to reject a null that is false. Statisticians call this a Type II error. Generally, you do not know the Type II error rate. However, it is a larger risk when you have a small sample size , noisy data, or a small effect size. The type II error rate is also known as beta (β).
Statistical power is the probability that a hypothesis test correctly infers that a sample effect exists in the population. In other words, the test correctly rejects a false null hypothesis. Consequently, power is inversely related to a Type II error. Power = 1 – β. Learn more about Power in Statistics .
Related posts : Types of Errors in Hypothesis Testing and Estimating a Good Sample Size for Your Study Using Power Analysis
Which Type of Hypothesis Test is Right for You?
There are many different types of procedures you can use. The correct choice depends on your research goals and the data you collect. Do you need to understand the mean or the differences between means? Or, perhaps you need to assess proportions. You can even use hypothesis testing to determine whether the relationships between variables are statistically significant.
To choose the proper statistical procedure, you’ll need to assess your study objectives and collect the correct type of data . This background research is necessary before you begin a study.
Related Post : Hypothesis Tests for Continuous, Binary, and Count Data
Statistical tests are crucial when you want to use sample data to make conclusions about a population because these tests account for sample error. Using significance levels and p-values to determine when to reject the null hypothesis improves the probability that you will draw the correct conclusion.
To see an alternative approach to these traditional hypothesis testing methods, learn about bootstrapping in statistics !
If you want to see examples of hypothesis testing in action, I recommend the following posts that I have written:
- How Effective Are Flu Shots? This example shows how you can use statistics to test proportions.
- Fatality Rates in Star Trek . This example shows how to use hypothesis testing with categorical data.
- Busting Myths About the Battle of the Sexes . A fun example based on a Mythbusters episode that assess continuous data using several different tests.
- Are Yawns Contagious? Another fun example inspired by a Mythbusters episode.
Share this:

Reader Interactions
January 14, 2024 at 8:43 am
Hello professor Jim, how are you doing! Pls. What are the properties of a population and their examples? Thanks for your time and understanding.
January 14, 2024 at 12:57 pm
Please read my post about Populations vs. Samples for more information and examples.
Also, please note there is a search bar in the upper-right margin of my website. Use that to search for topics.
July 5, 2023 at 7:05 am
Hello, I have a question as I read your post. You say in p-values section
“P-values are the probability that you would obtain the effect observed in your sample, or larger, if the null hypothesis is correct. In simpler terms, p-values tell you how strongly your sample data contradict the null. Lower p-values represent stronger evidence against the null.”
But according to your definition of effect, the null states that an effect does not exist, correct? So what I assume you want to say is that “P-values are the probability that you would obtain the effect observed in your sample, or larger, if the null hypothesis is **incorrect**.”
July 6, 2023 at 5:18 am
Hi Shrinivas,
The correct definition of p-value is that it is a probability that exists in the context of a true null hypothesis. So, the quotation is correct in stating “if the null hypothesis is correct.”
Essentially, the p-value tells you the likelihood of your observed results (or more extreme) if the null hypothesis is true. It gives you an idea of whether your results are surprising or unusual if there is no effect.
Hence, with sufficiently low p-values, you reject the null hypothesis because it’s telling you that your sample results were unlikely to have occurred if there was no effect in the population.
I hope that helps make it more clear. If not, let me know I’ll attempt to clarify!
May 8, 2023 at 12:47 am
Thanks a lot Ny best regards
May 7, 2023 at 11:15 pm
Hi Jim Can you tell me something about size effect? Thanks
May 8, 2023 at 12:29 am
Here’s a post that I’ve written about Effect Sizes that will hopefully tell you what you need to know. Please read that. Then, if you have any more specific questions about effect sizes, please post them there. Thanks!
January 7, 2023 at 4:19 pm
Hi Jim, I have only read two pages so far but I am really amazed because in few paragraphs you made me clearly understand the concepts of months of courses I received in biostatistics! Thanks so much for this work you have done it helps a lot!
January 10, 2023 at 3:25 pm
Thanks so much!
June 17, 2021 at 1:45 pm
Can you help in the following question: Rocinante36 is priced at ₹7 lakh and has been designed to deliver a mileage of 22 km/litre and a top speed of 140 km/hr. Formulate the null and alternative hypotheses for mileage and top speed to check whether the new models are performing as per the desired design specifications.
April 19, 2021 at 1:51 pm
Its indeed great to read your work statistics.
I have a doubt regarding the one sample t-test. So as per your book on hypothesis testing with reference to page no 45, you have mentioned the difference between “the sample mean and the hypothesised mean is statistically significant”. So as per my understanding it should be quoted like “the difference between the population mean and the hypothesised mean is statistically significant”. The catch here is the hypothesised mean represents the sample mean.
Please help me understand this.
Regards Rajat
April 19, 2021 at 3:46 pm
Thanks for buying my book. I’m so glad it’s been helpful!
The test is performed on the sample but the results apply to the population. Hence, if the difference between the sample mean (observed in your study) and the hypothesized mean is statistically significant, that suggests that population does not equal the hypothesized mean.
For one sample tests, the hypothesized mean is not the sample mean. It is a mean that you want to use for the test value. It usually represents a value that is important to your research. In other words, it’s a value that you pick for some theoretical/practical reasons. You pick it because you want to determine whether the population mean is different from that particular value.
I hope that helps!
November 5, 2020 at 6:24 am
Jim, you are such a magnificent statistician/economist/econometrician/data scientist etc whatever profession. Your work inspires and simplifies the lives of so many researchers around the world. I truly admire you and your work. I will buy a copy of each book you have on statistics or econometrics. Keep doing the good work. Remain ever blessed
November 6, 2020 at 9:47 pm
Hi Renatus,
Thanks so much for you very kind comments. You made my day!! I’m so glad that my website has been helpful. And, thanks so much for supporting my books! 🙂
November 2, 2020 at 9:32 pm
Hi Jim, I hope you are aware of 2019 American Statistical Association’s official statement on Statistical Significance: https://www.tandfonline.com/doi/full/10.1080/00031305.2019.1583913 In case you do not bother reading the full article, may I quote you the core message here: “We conclude, based on our review of the articles in this special issue and the broader literature, that it is time to stop using the term “statistically significant” entirely. Nor should variants such as “significantly different,” “p < 0.05,” and “nonsignificant” survive, whether expressed in words, by asterisks in a table, or in some other way."
With best wishes,
November 3, 2020 at 2:09 am
I’m definitely aware of the debate surrounding how to use p-values most effectively. However, I need to correct you on one point. The link you provide is NOT a statement by the American Statistical Association. It is an editorial by several authors.
There is considerable debate over this issue. There are problems with p-values. However, as the authors state themselves, much of the problem is over people’s mindsets about how to use p-values and their incorrect interpretations about what statistical significance does and does not mean.
If you were to read my website more thoroughly, you’d be aware that I share many of their concerns and I address them in multiple posts. One of the authors’ key points is the need to be thoughtful and conduct thoughtful research and analysis. I emphasize this aspect in multiple posts on this topic. I’ll ask you to read the following three because they all address some of the authors’ concerns and suggestions. But you might run across others to read as well.
Five Tips for Using P-values to Avoid Being Misled How to Interpret P-values Correctly P-values and the Reproducibility of Experimental Results
September 24, 2020 at 11:52 pm
HI Jim, i just want you to know that you made explanation for Statistics so simple! I should say lesser and fewer words that reduce the complexity. All the best! 🙂
September 25, 2020 at 1:03 am
Thanks, Rene! Your kind words mean a lot to me! I’m so glad it has been helpful!
September 23, 2020 at 2:21 am
Honestly, I never understood stats during my entire M.Ed course and was another nightmare for me. But how easily you have explained each concept, I have understood stats way beyond my imagination. Thank you so much for helping ignorant research scholars like us. Looking forward to get hardcopy of your book. Kindly tell is it available through flipkart?
September 24, 2020 at 11:14 pm
I’m so happy to hear that my website has been helpful!
I checked on flipkart and it appears like my books are not available there. I’m never exactly sure where they’re available due to the vagaries of different distribution channels. They are available on Amazon in India.
Introduction to Statistics: An Intuitive Guide (Amazon IN) Hypothesis Testing: An Intuitive Guide (Amazon IN)
July 26, 2020 at 11:57 am
Dear Jim I am a teacher from India . I don’t have any background in statistics, and still I should tell that in a single read I can follow your explanations . I take my entire biostatistics class for botany graduates with your explanations. Thanks a lot. May I know how I can avail your books in India
July 28, 2020 at 12:31 am
Right now my books are only available as ebooks from my website. However, soon I’ll have some exciting news about other ways to obtain it. Stay tuned! I’ll announce it on my email list. If you’re not already on it, you can sign up using the form that is in the right margin of my website.
June 22, 2020 at 2:02 pm
Also can you please let me if this book covers topics like EDA and principal component analysis?
June 22, 2020 at 2:07 pm
This book doesn’t cover principal components analysis. Although, I wouldn’t really classify that as a hypothesis test. In the future, I might write a multivariate analysis book that would cover this and others. But, that’s well down the road.
My Introduction to Statistics covers EDA. That’s the largely graphical look at your data that you often do prior to hypothesis testing. The Introduction book perfectly leads right into the Hypothesis Testing book.
June 22, 2020 at 1:45 pm
Thanks for the detailed explanation. It does clear my doubts. I saw that your book related to hypothesis testing has the topics that I am studying currently. I am looking forward to purchasing it.
Regards, Take Care
June 19, 2020 at 1:03 pm
For this particular article I did not understand a couple of statements and it would great if you could help: 1)”If sample error causes the observed difference, the next time someone performs the same experiment the results might be different.” 2)”If the difference does not exist at the population level, you won’t obtain the benefits that you expect based on the sample statistics.”
I discovered your articles by chance and now I keep coming back to read & understand statistical concepts. These articles are very informative & easy to digest. Thanks for the simplifying things.
June 20, 2020 at 9:53 pm
I’m so happy to hear that you’ve found my website to be helpful!
To answer your questions, keep in mind that a central tenant of inferential statistics is that the random sample that a study drew was only one of an infinite number of possible it could’ve drawn. Each random sample produces different results. Most results will cluster around the population value assuming they used good methodology. However, random sampling error always exists and makes it so that population estimates from a sample almost never exactly equal the correct population value.
So, imagine that we’re studying a medication and comparing the treatment and control groups. Suppose that the medicine is truly not effect and that the population difference between the treatment and control group is zero (i.e., no difference.) Despite the true difference being zero, most sample estimates will show some degree of either a positive or negative effect thanks to random sampling error. So, just because a study has an observed difference does not mean that a difference exists at the population level. So, on to your questions:
1. If the observed difference is just random error, then it makes sense that if you collected another random sample, the difference could change. It could change from negative to positive, positive to negative, more extreme, less extreme, etc. However, if the difference exists at the population level, most random samples drawn from the population will reflect that difference. If the medicine has an effect, most random samples will reflect that fact and not bounce around on both sides of zero as much.
2. This is closely related to the previous answer. If there is no difference at the population level, but say you approve the medicine because of the observed effects in a sample. Even though your random sample showed an effect (which was really random error), that effect doesn’t exist. So, when you start using it on a larger scale, people won’t benefit from the medicine. That’s why it’s important to separate out what is easily explained by random error versus what is not easily explained by it.
I think reading my post about how hypothesis tests work will help clarify this process. Also, in about 24 hours (as I write this), I’ll be releasing my new ebook about Hypothesis Testing!
May 29, 2020 at 5:23 am
Hi Jim, I really enjoy your blog. Can you please link me on your blog where you discuss about Subgroup analysis and how it is done? I need to use non parametric and parametric statistical methods for my work and also do subgroup analysis in order to identify potential groups of patients that may benefit more from using a treatment than other groups.
May 29, 2020 at 2:12 pm
Hi, I don’t have a specific article about subgroup analysis. However, subgroup analysis is just the dividing up of a larger sample into subgroups and then analyzing those subgroups separately. You can use the various analyses I write about on the subgroups.
Alternatively, you can include the subgroups in regression analysis as an indicator variable and include that variable as a main effect and an interaction effect to see how the relationships vary by subgroup without needing to subdivide your data. I write about that approach in my article about comparing regression lines . This approach is my preferred approach when possible.
April 19, 2020 at 7:58 am
sir is confidence interval is a part of estimation?
April 17, 2020 at 3:36 pm
Sir can u plz briefly explain alternatives of hypothesis testing? I m unable to find the answer
April 18, 2020 at 1:22 am
Assuming you want to draw conclusions about populations by using samples (i.e., inferential statistics ), you can use confidence intervals and bootstrap methods as alternatives to the traditional hypothesis testing methods.
March 9, 2020 at 10:01 pm
Hi JIm, could you please help with activities that can best teach concepts of hypothesis testing through simulation, Also, do you have any question set that would enhance students intuition why learning hypothesis testing as a topic in introductory statistics. Thanks.
March 5, 2020 at 3:48 pm
Hi Jim, I’m studying multiple hypothesis testing & was wondering if you had any material that would be relevant. I’m more trying to understand how testing multiple samples simultaneously affects your results & more on the Bonferroni Correction
March 5, 2020 at 4:05 pm
I write about multiple comparisons (aka post hoc tests) in the ANOVA context . I don’t talk about Bonferroni Corrections specifically but I cover related types of corrections. I’m not sure if that exactly addresses what you want to know but is probably the closest I have already written. I hope it helps!
January 14, 2020 at 9:03 pm
Thank you! Have a great day/evening.
January 13, 2020 at 7:10 pm
Any help would be greatly appreciated. What is the difference between The Hypothesis Test and The Statistical Test of Hypothesis?
January 14, 2020 at 11:02 am
They sound like the same thing to me. Unless this is specialized terminology for a particular field or the author was intending something specific, I’d guess they’re one and the same.
April 1, 2019 at 10:00 am
so these are the only two forms of Hypothesis used in statistical testing?
April 1, 2019 at 10:02 am
Are you referring to the null and alternative hypothesis? If so, yes, that’s those are the standard hypotheses in a statistical hypothesis test.
April 1, 2019 at 9:57 am
year very insightful post, thanks for the write up
October 27, 2018 at 11:09 pm
hi there, am upcoming statistician, out of all blogs that i have read, i have found this one more useful as long as my problem is concerned. thanks so much
October 27, 2018 at 11:14 pm
Hi Stano, you’re very welcome! Thanks for your kind words. They mean a lot! I’m happy to hear that my posts were able to help you. I’m sure you will be a fantastic statistician. Best of luck with your studies!
October 26, 2018 at 11:39 am
Dear Jim, thank you very much for your explanations! I have a question. Can I use t-test to compare two samples in case each of them have right bias?
October 26, 2018 at 12:00 pm
Hi Tetyana,
You’re very welcome!
The term “right bias” is not a standard term. Do you by chance mean right skewed distributions? In other words, if you plot the distribution for each group on a histogram they have longer right tails? These are not the symmetrical bell-shape curves of the normal distribution.
If that’s the case, yes you can as long as you exceed a specific sample size within each group. I include a table that contains these sample size requirements in my post about nonparametric vs parametric analyses .
Bias in statistics refers to cases where an estimate of a value is systematically higher or lower than the true value. If this is the case, you might be able to use t-tests, but you’d need to be sure to understand the nature of the bias so you would understand what the results are really indicating.
I hope this helps!
April 2, 2018 at 7:28 am
Simple and upto the point 👍 Thank you so much.
April 2, 2018 at 11:11 am
Hi Kalpana, thanks! And I’m glad it was helpful!
March 26, 2018 at 8:41 am
Am I correct if I say: Alpha – Probability of wrongly rejection of null hypothesis P-value – Probability of wrongly acceptance of null hypothesis
March 28, 2018 at 3:14 pm
You’re correct about alpha. Alpha is the probability of rejecting the null hypothesis when the null is true.
Unfortunately, your definition of the p-value is a bit off. The p-value has a fairly convoluted definition. It is the probability of obtaining the effect observed in a sample, or more extreme, if the null hypothesis is true. The p-value does NOT indicate the probability that either the null or alternative is true or false. Although, those are very common misinterpretations. To learn more, read my post about how to interpret p-values correctly .
March 2, 2018 at 6:10 pm
I recently started reading your blog and it is very helpful to understand each concept of statistical tests in easy way with some good examples. Also, I recommend to other people go through all these blogs which you posted. Specially for those people who have not statistical background and they are facing to many problems while studying statistical analysis.
Thank you for your such good blogs.
March 3, 2018 at 10:12 pm
Hi Amit, I’m so glad that my blog posts have been helpful for you! It means a lot to me that you took the time to write such a nice comment! Also, thanks for recommending by blog to others! I try really hard to write posts about statistics that are easy to understand.
January 17, 2018 at 7:03 am
I recently started reading your blog and I find it very interesting. I am learning statistics by my own, and I generally do many google search to understand the concepts. So this blog is quite helpful for me, as it have most of the content which I am looking for.
January 17, 2018 at 3:56 pm
Hi Shashank, thank you! And, I’m very glad to hear that my blog is helpful!
January 2, 2018 at 2:28 pm
thank u very much sir.
January 2, 2018 at 2:36 pm
You’re very welcome, Hiral!
November 21, 2017 at 12:43 pm
Thank u so much sir….your posts always helps me to be a #statistician
November 21, 2017 at 2:40 pm
Hi Sachin, you’re very welcome! I’m happy that you find my posts to be helpful!
November 19, 2017 at 8:22 pm
great post as usual, but it would be nice to see an example.
November 19, 2017 at 8:27 pm
Thank you! At the end of this post, I have links to four other posts that show examples of hypothesis tests in action. You’ll find what you’re looking for in those posts!
Comments and Questions Cancel reply

- Walden University
- Faculty Portal
Webinar Transcripts: Appropriate Use of First Person and Avoiding Bias
Appropriate use of first person and avoiding bias.
Presented July 17, 2019
View the recording
Last updated 8/25/2019
Visual: Slide changes to the following: Housekeeping
- Will be available online within 24 hours.
- Polls, files, and links are interactive.
- Now: Use the Q&A box.
- Later: Send to [email protected] or visit our Live Chat Hours .
- Ask in the Q&A box.
- Choose “Help” in the upper right-hand corner of the webinar room
Audio: Hello everyone and welcome to today's webinar entitled appropriate use of first-person and avoiding bias. I’m Michael Dusek and I’m a writing instructor in the Walden writing center I’ll be working behind the scenes of today's webinar. Before we begin and I hand the session over to today's presenter, Kacy, let me go over a few housekeeping items.
First, we are recording this webinar so you are welcome to access it at a later date via our webinar archive and in fact note that we record all of our webinars at the writing center so you are welcome to look through that archive for other recordings that might interest you as well.
Furthermore, we might mention a few webinars that will be a helpful follow-up to this webinar during the session so feel free to explore the webinar archive at your leisure.
Also, whether you are attending this webinar live or watch a recording, note that you’ll be able to participate in any polls that we use, files we share or links we provide. You can also access the PowerPoint slides Kacy will be sharing which are located in the files pod.
Lastly, we also welcome questions and comments throughout the session via the Q & A box. I will be watching the Q & A box and will be happy to answer questions throughout the session as Kacy is presenting. You're also welcome to present any technical issues you have to me there although note there is a help option at the right corner of your screen. This is Adobes Technical Support so that is probably the best place to go if tech issues persist. Okay, with that I will hand over the session to our presenter, Kacy Walz.
Visual: Slide changes to the title of the webinar, “Appropriate Use of First Person and Avoiding Bias” and the speaker’s name and information: Kacy Walz, Writing Instructor, Walden University Writing Center
Audio: Kacy: Hello, and thank you all for joining us and as Michael said my name is Kacy Walz and I'm also a writing instructor at the Walden writing center and I am calling in today from St. Louis, Missouri, where it is very hot and I hope you are all enjoying nice weather wherever you are.
Visual: Slide changes to the following: Today’s Learning Objectives:
- Identify appropriate uses
- Identify inappropriate uses
- What constitutes objectivity?
- Ways to avoid bias
- Understanding implicit bias
Audio: In our webinar we have a few learning objectives. First, we are going to go over the use of first-person or personal pronouns, which are those pronouns like I, me, my, and we are going to go over the appropriate uses for these pronouns and also where it might be inappropriate to use those first-person pronouns.
We are also going to be talking about avoiding bias. So, we’ll go over what constitutes bias and what constitutes objectivity too. We'll talk about some ways to avoid bias and then we are going to have a little bit of information about understanding implicit bias and how it impacts your writing and your other scholarly courses.
Visual: Slide changes to the following: Use of First person
- Allowed by both Walden and APA…
…when used appropriately.
Section 3.09 in APA Manual
Writing Center website
Audio: First off, we are going to talk about first person. First person is allowed by both Walden and APA when it’s used appropriately. Now if you have any questions about this, you can check these different websites or the section 3.09 in the APA manual but contrary to some confusion, you should be using first person in your scholarly writing.
Visual: Slide changes to the following: Considerations
- Program/degree level
- Assignment requirements
- Professor preferences
Audio: There are some different considerations of course for how often or when you're going to use that first person.
First off will be the program for the degree level so certain programs are going to have different rules about when you are using first person and also depending on what level you are at there might be some different expectations. You might also have some different assignment requirements so if the assignment is specifically telling you not to use first person, we don't want to give you any misconceptions about that. You want to follow your assignment directions. And then also going along with that professor preferences -- different professors will have different ways that they like you to present your information and at the writing center we are always going to defer to your professor, so whatever he or she prefers is how you want to go about with your writing.
Visual: Slide changes to the following: When to Use the First Person:
To avoid “the author” and “this researcher”
- I sent the surveys to the participants.
- I replicated McCaskey ’ s (2012) study with a fourth-grade population.
Audio: So, here are some places that you want to avoid, I’m sorry that you want to use the first person. You want to avoid saying the author or this researcher when you’re talking specifically about yourself. Some examples are this researcher sent the surveys to the participants. Or in this second example McCaskey conducted a study of standardized test performance in third grade English language learners. The author replicated this study with a fourth-grade population. Here you can see how both of these instances by using the author or the researcher it’s a little bit trickier for our reader to understand who exactly is doing all of this. In the first one we can clarify basically saying I sent the surveys to the participants. If you are the one conducting this research process take ownership of that and claim that as something you've actually done if you are the one who sent out the survey.
Similarly, with the second one, I replicated McCaskey's study with a fourth-grade population clarifies that when this writer was saying the author, they actually meant themselves – they were the ones who replicated the study. If I am a reader coming across these sentences, I might think that writer is still talking about McCaskey so therefore McCaskey is getting the credit for this second study with the fourth-grade population when actually it’s the writer that did all that work, so you want to claim that credit by using first person.
Audio: Here is another example. So here we have afterschool programs have a documented connection to students’ physical fitness and their education. Gortmaker found that students activity levels increased by 10 minutes when physical fitness was integrated with afterschool programs. I will use this model to implement a similar program in my own school district. By continuing Gortmakers focus on physical fitness and education or afterschool programs, I will show the effectiveness of integrating the two focuses. And so here again you can see how using that first person clarifies for your reader what part of this is coming from Gortmaker and what part is actually being completed by me, the writer.
To avoid anthropomorphism
- In this paper, I will examine…
- In this section, I will explore…
Additional Resource!
What is anthropomorphism?
Audio: You also want to use first person to avoid what we call anthropomorphism. Anthropomorphism is basically just a fancy word for when you give agency to an inanimate object. Some examples here are, this paper will examine or this section will explore. So a paper on its own can’t examine anything and a section cannot explore anything. Instead we can say in this paper, I will examine because you, as the writer and scholar are perfectly able to examine something. Or you could say in this section, I will explore and so you are clarifying for your reader that this specific section is working to achieve something but you're not giving this section or the paper that kind of agency or animation as it in self is going to be examining or exploring.
To explain what you will do or show in a paper
- I will do this…
- I will show that…
- I will summarize this…
- I will conclude with…
Audio: So, you want to use first person to explain what you will do or show in a paper like in those previous examples, so you might say I will do this or I’ll show that and provide some kind of argument. I am going to summarize this or I will conclude with so using that first person to let your reader know that this is what they can expect is a really good use of first-person.
To avoid passive voice
- I will suggest a healthcare initiative.
- I will analyze three education theories.
- I will explain ways that I will manage my time.
- Active and Passive Voice
Audio: You also want to use first person to avoid passive voice and I think this is something that can be a little bit confusing because this is a comment that I make on a lot of papers but basically when you use passive voice it’s just unclear to your reader what the specific subject is or who is doing the action. Here we have a healthcare initiative will be suggested. Who is suggesting this healthcare initiative? Right? As a reader I am not sure. Three education theories will be analyzed. Again, I am not sure who was doing the analysis here, I don’t know what the actual subject of that sentence is or ways that time will be managed will be explained by me. And I think this third example is a good illustration of where passive voice can become a little bit clunky and make it where you are using more words than you need to use and it gets in the way of the overall readability of your paper.
So instead I could write I will suggest a healthcare initiative. So, there's no question in who is making this recommendation, it is the writer. I’m not referencing any other resources at least in this paper. I will analyze three education theories. Again, you’re taking credit for the work you’re the one providing this analysis you're going to give and then I will explain ways that I will manage my time. So again, it is much more direct. It might not be that many fewer words but it is just a little bit more clear and does not seem quite so circular when you have all of that passive voice method involved.
Visual: Slide changes to the following: Putting it All together
Choose one sentence; revise it and submit it to the chat box.
This section explores the theories of positivism, functionalism, and social construction, after which their applicability to national health care will be analyzed. The author also compares these theories to her hospital, illustrating that functionalism is most appropriate for this organization.
Audio: So, I have a chance for you guys to put this into practice so in the chat box take one of these sentences and just revise it and submit it into the chat box. I’m going to put this on silence for a bit and give you guys a chance
[Silence as participants respond]
I am seeing a lot of great responses come in and I think you guys are picking up on the really important pieces that we wanted you to note. First off several of you have noticed that the section can’t explore anything so we need to provide some subject and a lot of you put in this section I will explore or simply I will explore two theories so you are clarifying who exactly it is who is doing this exploring, who was providing these theories. Similarly, some of you are pointing out this idea of what is, whose hospital is her hospital? The author also compares these theories to her hospital and with your revisions I see that you are working through what that specifically means and are making great points that as readers that is very unclear whereas if I revise this to: I will also compare these theories to my hospital or the hospital where I work, that is going to clarify and give a focus point for your reader so now it is pinpointed what specific hospital you’re talking about. We don't have this confusion of did I miss something or did I misread another part of this paper where they are talking about this so you're doing a great job. Thank you so much for participating.
In this section, I explore the theories of positivism, functionalism, and social construction, after which I analyze their applicability to national health care. I also compare these theories to my hospital, illustrating that functionalism is most appropriate for my organization.
Here is the way that I revised that chat box. Similarly, to what a lot of you have written in the chat box, in this section, I explore the theories of positivism, functionalism and social construction, after which I analyze their applicability to national healthcare. I also compare these theories to my hospital illustrating that functionalism is most appropriate for my organization. So again we’ve got all that information cleared up I know that I should not be looking for any citations because these are going to be my own ideas and my own work and it’s clear that I am talking about a specific organization and my reader has a sense of what specific hospital, what specific organization I am talking about.
I don't know if you heard that loud clap of thunder all of a sudden. It just all of a sudden started raining here. It kind of scared me. But anyway.
Visual: Slide changes to the following: In appropriate Use of First Person
- Sometimes weakens your argument
- May be perceived as bias
Audio: Now we're going to go over some inappropriate uses of first-person. So, this is where I think a lot of the confusion stems and people are afraid to use first person in their scholarly writing because sometimes using first person can weaken your argument or lots of times teachers or people who are commenting on papers might recommend that you avoid first-person so that your argument seems stronger or you seem more assured of the points that you are making. Additionally, can also sometimes be perceived as bias so whether or not you actually have that bias or you are trying to present something as an argument, using that first-person can lead your reader to believe that there is bias there.
Visual: Slide changes to the following: Inappropriate Use of the First Person
To express opinions or beliefs
- Teachers need to pay attention to students ’ individual needs in order to...
- Nurses should be more caring towards their patients in order to...
Audio: So, here are some more examples of that. I think that teachers need to pay attention to students’ individual needs. I believe that nurses should be more caring towards their patients. I think these are kind of the classic examples of when reviewers are going to recommend that you avoid first-person. Because you don't really need that I think or I believe because the act of writing that sentence is going to make it clear to your reader that this is what you think or believe. Instead you can simply say teachers need to pay attention to students’ individual needs and then you can build that into your argument, in order to and so it’s very clear that this is your point that you are making and this is the argument that you want to lead into with that statement, teachers need to pay attention.
Similarly, we could just say nurses should be more caring towards their patients in order to, and so again we are introducing the specific arguments but because I don't have any citation and because I’m just presenting this within my writing it’s going to be clear to my reader that this is my point, what I believe.
To make assumptions about your audience.
- Educators have a responsibility to address all students’ learning styles.
- The United States entered a new period of educational reform in the 1970s.
Audio: Another place where it can be tricky and I see this a lot in papers too, is using second person, basically when you are bringing your reader into a group or you are implicating them in some way along with yourself. So, we have a responsibility as educators to ensure that all students’ learning styles are addressed. Right? That is going to assume something about whoever it is that is reading it that they are also going to be part of that group that I am claiming for myself.
Our nation entered a new period of educational reform in the 1970s. You’re making the assumption that whoever is reading this paper is also going to claim your nation as their own, is going to know specifically what nation you are referring to when you are talking about those educational reforms. So instead you can simply say educators have a responsibility to address all students’ learning styles. That way it’s very clear to your reader who you are talking about because maybe your reader does identify as part of that group. Similarly, but they are not sure what specific group you are talking about. Are you talking about parents, are you talking about school administrators? Here specifically we want to talk about educators so we are going to make that very clear by simply saying educators have that responsibility.
In this next sentence we can say the United States entered a new period of educational reform in the 1970s which makes it very clear what nation you are talking about, particularly as Walden students you might have readers from all over the world. So saying our nation is going to be very confusing to someone who does not live in the United States or who lives in a different country that you do, if they are reading your paper and feel they are expected to know what nation experienced educational reform in the 1970s because probably there’s going to be more than one, there’s going to be more than one nation for whatever you are talking about so you want to make sure that is really clear.
Visual: Slide changes to the following: Putting It All Together
In my opinion, business managers should pay more attention to employees’ strengths. In the organization I volunteer for, I think many employees have strengths that managers overlook. Members of our profession must remain aware of the importance of recognizing employees’ individual abilities to effectively build a team.
Audio: Here is another chat opportunity so again you're going to take one of these sentences and revise it so that you are using first person when you want to use it and you are avoiding it when maybe it is not necessary, when it’s going to make your paper stronger to avoid that use of first-person. I am going to mute for about two minutes and give you time to answer.
[silence as participants respond]
Great I see a lot of you have picked up on the fact that we don't need that, in my opinion. We can just start with business managers should pay more attention to some of you have added that in order to at the end so I am not just stating this opinion but I want to state this opinion so that it can lead into some larger argument. Let me just to go over my own corrections here which I think again is very similar to what you all have typed in the chat box. Business managers should pay more attention to employee strengths in order to be effective leaders. In the organization I volunteer for, a lot of you noted we still want to keep that first-person, many employees have strengths that managers overlook. Managers must remain aware of the importance of recognizing employees’ individual abilities to effectively build a team.
Business managers should pay more attention to employees’ strengths in order to be effective leaders. In the organization I volunteer for, many employees have strengths that managers overlook. Managers must remain aware of the importance of recognizing employees’ individual abilities to effectively build a team.
So first-person should be used in a few of those areas, right? Because it makes it clear for the reader specifically which organization you are talking about -- it kind of gives you a little bit of claim too. I have noticed this in my own organization it gives you that extra credibility, but also we have removed the second person of members of our profession because we don't know specifically which profession the reader might be in or how they might identify themselves in this specific situation. Instead we can just say managers in general should remain aware. Right?
Great job. It looks like a lot if you even went above and beyond and did not just take one sentence but decided to revise the whole thing, so great job. You guys are rocking along.
Visual: Slide changes to the following: Questions?
Submit in the Q&A Box! Up Next: Objectivity and Avoiding Bias.
Audio: We're going to take a quick minute here before we move on to talking about bias. Michael are there any questions that might be helpful to discuss as a larger group?
Michael: Hey Kacy at this point the Q&A box has been pretty quiet. If you have any questions that you would like to get answered as the pr0esentation continues feel free to drop them in the Q&A box and I will respond to them as soon as I can, but at this point I think you're good to move on.
Kacy: Please do if you have any questions be sure to let us know in that chat box and we can talk about them as a larger group or Michael can just answer them directly.
Visual: Slide changes to the following: Objectivity & Avoiding Bias
What does objectivity and avoiding bias in writing mean to you?
According to APA (2010), “scientific writing must be free of implied or irrelevant evaluation of the group or groups being studied” (p.233).
Audio: Before we get into talking about bias and objectivity, I just want to give you a minute to think about what objectivity and avoiding bias in writing means to you. So, why do you think that is important to be objective and to avoid bias when you are crafting your papers? I’ll just maybe go on mute for another minute or so while you enter that in the chat box.
We have this little citation from APA. According to APA scientific writing must be free of implied or irrelevant evaluation of the group or groups being studied and that’s really important particularly a lot of the projects that Walden students create are dealing with social sciences or dealing with groups of individuals. We focus a lot on social change and so maintaining that objectivity and avoiding bias is very important in all scholarly writing but especially for Walden students and it looks like you all are also picking up on the really important parts about why you want to maintain objectivity in writing. Some people have pointed out that you don't want to be misunderstood, you don’t want people to think that you are making a certain assumption or argument that you are not intending with some unintentionally bias sounding language. You want to make sure that your writing is credible and if it sounds like the writing is overly biased or is not objective that definitely takes away from your credibility as a writer.
You want to always, always be supporting your arguments with evidence so it shouldn’t be based on opinion. You want to clearly present that you have researched your argument, that you have a strong foundation for it and you are not just making things up and that is why citations are so important in making sure that you are providing that good research. Awesome.
- Objectivity is
The standard for social science publication
Different from nonacademic sources
- Objectivity is not
Passionless or robotic
Missing your voice
Audio: Some things about objectivity is, it is a standard for social science publication. Make sure you are being objective because that is a major goal for a lot of scholars is to be published and it’s different in terms of what objectivity means for academic sources versus non-academic sources.
What objectivity is not however, it does not mean that your writing is passionless or robotic and it does not mean that you are not including your own voice, you definitely you want to include argumentation. You want to include your own points of scholar and you want to make your writing interesting and to be engaging for your reader. Being passionless or robotic does not mean that you are being objective so I think that’s something that we want to get out of the way right up front. You can be very passionate about a topic but still not be writing in a biased or nonobjective way.
Visual: Slide changes to the following: Why avoid bias?
- Don’t want to offend your reader
- Want your reader to see you as an authority
- Wat to be, and appear to be, open-minded
Audio: These are some things that you all pointed out in the chat box. But just to kind of go over, you want to avoid bias because you don't want to offend your reader. I saw some mention that being biased in your writing could affect relationships that you have, professional relationships that you are developing over the course of your scholarly career and you definitely want to avoid offending anybody by potentially having bias in your writing
You want your reader to see you as an authority, so lots of you talked about credibility and the importance of backing up all of the arguments you make with strong evidence. And you want to be and appear to be open-minded. We often talk about scholarship as joining a conversation and you don't want to be that one person in the corner that nobody wants to talk to because they are completely only going to look at everything in their own way. Or if they are not going to consider anybody else's ideas or thoughts you're not going to change their mind. You want to be the participant in the conversation, who is taking different ideas and building off of them and debating them in a way that is respectful and clear but also well supported and not emotion-based. These are some really important things about why you want to avoid bias in your writing.
Visual: Slide changes to the following: Avoiding Bias: Avoiding Generalizations
People from Tennessee are obsessed with football.
- Many Tennesseans are avid fans of football (Manning, 2009).
Generalizations: Statements that oversimplify a situation or ignore outliers, sometimes called “blanket statements.”
Scholarly Voice
Audio: Here are some examples of bias that I think sometimes it can be confusing by what we mean by bias. And bias sounds like a really bad word but it can be a simple, kind of generalization that you may make without realizing you are making it. This example, people from Tennessee are obsessed with football. Right? Statements like this are suggesting that an entire group of people from an entire state are all going to have the same obsessions or are all going to have the same likes or dislikes. So, instead you can just say that many Tennesseans are avid fans of football. Right? And here we even have this citation to support it. Maybe Manning has taken some kind of survey of everybody who lives in Tennessee and the majority of them have said yes, I’m an avid football fan.
I’m not saying generally just making this blanket statement if you’re from Tennessee you’re obsessed with football, I actually have a citation to support this and I'm being much more careful about how I am presenting that information. I like to think about it as you don't want to be so easily discredited. If I find one person from Tennessee in a situation who is not obsessed with football than that entire sentence becomes moot, it is no longer an arguable sentence because I found this one exemption. Whereas with the second, many are avid fans of football, that is going to be a lot more difficult to disprove particularly when I have that citation that is supporting that claim suggesting somebody has already done the research to back this up.
Visual: Slide changes to the following: Avoiding Bias: Using Evidence
Third-grade boys are chronically disruptive, while the girls are always eager to please.
- In Clooney ’ s (2008) study of Kansas City third-graders, 35% of the boys and 68% of the girls were able to complete instructions for a tedious assignment without showing signs of agitation.
- Answer the question “Says who?” or “According to whom?” for your reader.
Using Evidence
Audio: Similarly, third grade boys are chronically disruptive while the girls are always eager to please. And again, you can kind of see how if I can find one third grade boy who is not chronically disruptive then I have completely blown this sentence apart. Instead I can use research and clear citations and then also these very specific statistics to clarify what I actually mean.
So, in Clooney's study of Kansas City third-graders, now I’m being even more specific about the population I'm talking about, 35% of the boys and 68% of the girls were able to complete instructions for a tedious assignment without showing signs of agitation. Here I have really clear information about the way that the gender breaks up in this particular study and I also have a clear idea of what I mean by disruptive or eager to please. Right, you can think about maybe yourself as a third grader or a third grader you know who has been forced to sit down and complete some really tedious task and maybe for a long period of time and how long it will take to start getting bored or start getting antsy. We have a much clearer idea of specifically what we mean when we are saying that maybe girls are able to stay still for a longer period of time or are able to focus on a task for a longer period of time, generally in comparison to boys.
High school administrators should include teachers in their decision making.
- High school administrators should include teachers in their decision making. Doing so, according to Jones (2013), promotes teachers’ acceptance of administrative decisions and policies, as well as ensures administration understands teachers’ perspectives when making decisions.
- Pair or support your own ideas with evidence.
Audio: We also want to avoid bias by providing evidence and this is kind of feeding back into our earlier slides talking about adding that, in order to, at the end of a statement. So here we have High School administrators should include teachers in their decision making. This is going to be my argument. Right? I don’t need to say I think or I believe, but I can just say high school administrators should include teachers. And then I'm going to add some research to support my claim, to support my reasoning for why I believe it is important. Doing so, according to Jones, promotes teacher's acceptance of administrative decisions and policies, as well as ensures administration understands teachers’ perspectives when making decisions. So here it’s clear why I’m making this suggestion. It is not that I am making this blanket assumption that administrators are not including teachers in their decision-making or I am suggesting that administrators have not thought about this themselves, instead I am using some research to back up my point about why this is so important.
Policemen must show sensitivity when communicating with demented people to be more effective public servants.
- Police officers must show sensitivity when communicating with people with dementia in order to be more effective public servants.
Avoid words or phrases that imply judgments related to race/ethnicity, gender, sexual orientation, disability, or age
Audio: And another similar example to that, policemen must show sensitivity when communicating with demented people to be more effective public servants. We’ve got a lot of things going on with this sentence that might show some unintentional bias. And so first off instead of saying policemen we could say police officers because there are people of both genders who serve as police, must show sensitivity when communicating with people with dementia, so basically that follows a person first descriptor.
We have several pages maybe Michael can try to find links to our pages about avoiding bias in scholarly writing but there is a practice where unless you know otherwise, unless you have specifically spoken to this group of people, you want to put their personhood before a descriptor or an identity. So, it is people with dementia rather than demented people. There are some exceptions to that and we talk about that in those pages, but in general you want to try to put the person first. So, you want to avoid any words or phrases that imply judgment so policemen might suggest that you are implying that only male individuals serve as police officers same thing with disability. We don't want to think of a disability as coming first. They are people first so we use that people first language instead. That can be tricky so if there are questions hopefully, we can talk about that more.
Visual: Slide changes to the following: Avoiding Bias: Understanding Implicit Bias
- Everyone has implicit biases
- Based on evolution
- Knowing what your own biases are will help you avoid them
- Do I personally know anyone who might fall into this category?
- What have my experiences been with individuals who I would categorize this way?
- Would I make this same kind of statement about a single race, ethnicity, gender, etc.?
Audio: And so finally understanding implicit bias. Implicit bias is something that is becoming more and more apparent in scholarly language and in studies but basically it is a principle that everyone has implicit biases they are based on evolution. Basically, our brains are just not able to make individualized judgments on people as quickly as we would like or as quickly as is sometimes necessary and so instead, we are really good at creating categories and being able to recognize patterns. So, we all have these implicit biases, and sometimes they serve us well and sometimes they are going to impact us in terms of avoiding bias in things like scholarly writing.
The key is, is just knowing what your biases might be because this will help you pay attention to where they might pop up in your writing or in your speaking or in your day to day interactions.
In order to complicate things when you think about maybe assumptions you might make about a larger group you can ask yourself these questions. Do I personally know anyone who might fall into this category? If I am talking about maybe say I am talking about football players in general, do I know anyone who plays football and do I know them well enough that I could maybe make some more individualized comments about that person versus talking about football players in general?
You want to think about what have my experiences been with individuals who I would categorize this way? Have they been predominantly positive, have they been predominantly negative? And think about if these experiences are having an effect on how you are talking or writing about that group of people. And then would you make the same kind of statement about a single race, ethnicity, gender, sexual orientation, etc. because often times it’s similar to that earlier comment about all Tennessee residents being obsessed with football. Right? It’s not a super necessarily a hurtful comment or damaging comment but I think we tend to be much more aware of things like race and gender, religion and so if it is not the kind of thing that you would say about everybody of a certain race or everybody of a certain gender, it is probably a good idea to rethink if you want to make it about this group.
One example I have is, for myself, is looking around at a restaurant and seeing a group of people younger than myself who are all on their cell phones, who are texting on their phones instead of having conversations with each other. I noticed that I could make a quick judgment about them about what their priorities are, about the ways they are interacting with their friends, the way they are interacting with their loved ones but the reality is I have no idea. Maybe they are actually playing a game together and that is interacting with all the people at their table or I don't know what the specific situation is that has them all on their phones rather than talking to each other. Also just because if I see one table was acting that way it does not mean that everybody of that age group is going to behave that way and I would never want to say something like that about a specific race or a specific gender so that is a good thing to take a step back and say I would never say that about all people of one religion are always on their phone, you know? It's a good thing to take that step back and say I am probably being a little bit biased here and I can ask myself these questions to make sure that I do not include that bias in a larger conversation or if I am trying to write something scholarly about millennials or I technically fall into the group of millennials.
Visual: Slide changes to the following: Avoiding Bias: Awareness of Background
- Assumptions about professions
- Beliefs about specific populations
- Preference for familiar people/situations
- Over sympathizing
Know what biases you bring to the discussion. Ask what influences your perception of ideas and situations.
Audio: And then you also, so going into that implicit bias you want to be aware of your own background we have certain assumptions about professions or populations. We have different preferences for people for certain situations for certain people that we find familiar and there’s also the idea of over sympathizing. We could also have biases that are, and it sort of beneficial in a way -- but we want to make sure we are being objective and talk about all groups in a similar manner, not giving favoritism to any one group or population.
You want to know what biases you are bringing to the discussion and then ask yourself what influences your perception of these different situations or of these different ideas and I think a lot of times what I love about working with students from Walden is they are often working on projects that are really important to them that they are very passionate about and then sometimes that’s when these biases can come out whether it is biased towards or bias against any specific group so it's really important to just be aware that we all have those biases and we want to make sure they are not negatively impacting our scholarly writing.
Visual: Slide changes to the following: Putting it All Together
What areas of this paragraph could we revise to avoid bias? What changes would you make?
Patients never seem to pay attention to the discharge instructions nurses give them. I think one way we could help alleviate this issue is by providing instructions that are easier to read and more clearly organized. Patients who are in the hospital are often vulnerable (McClean, 2014), and it is so sad to see them fail to understand or follow discharge instructions.
Audio: This is our final chat for the webinar. Putting together all of these different ideas that we’ve talked about, what areas of this paragraph could maybe be revised to avoid bias and what changes would you make? You can either revise the sentences the way we’ve been doing previous chat boxes – you could just point out problematic things or things you might want to change. I will go on mute for about two minutes.
So, I can see a lot of you are picking up on the kind of significance that certain words have and I think that’s really great. We did not even specifically talk about that in this webinar but pointing out that words like never or sad, are kind of weighted, they kind of have this extra meaning that goes along with them and so even though we have that theme -- that might sort of lessen this claim a little bit, the idea that patients never pay attention is probably overly biased, we could find that one patient will pay attention to the discharge instructions and that sentence no longer holds water.
Patients often fail to pay attention to the discharge instructions nurses give them. Dashner (2012) noted this failure, finding that patients failed to follow any or all of their discharge instructions 45% of the time. I think One way we hospitals could help alleviate this issue is by providing instructions that are easier to read and more clearly organized. Patients who are in the hospital are often vulnerable (McClean, 2014), and hospitals should do what they can to help ensure patients are able to understand and follow discharge instructions.
And then some of you are pointing out. We don't need to have this I think, and it is so sad is also one of those I think statements. So, we can get rid of that wording. So, here's another way that we can revise these with all of these pieces that you have brought up the chat box. Patients often fail to pay attention to the discharge instructions nurses give them. I saw quite a few of you had revised that sentence to be similar to that. Dashner noted this failure finding that patients fail to follow any or all of their discharge instructions 45% of the time. That is obviously added information that you did not have but I noticed that some were commenting on can we add some support for this claim? Can we back it up at all? That is how we're going to do that.
One way hospitals could alleviate this issue, so it is much more clear who this writer is addressing by saying we they are talk about hospitals, is by providing instructions that are easier to read and more clearly organized. Patients who are in the hospital are often vulnerable and hospitals should do what they can to help ensure patients are able to understand and follow discharge instructions. I think that also addresses that the language was almost patronizing. It seemed like the writer was talking down to patients or was talking down to the people that are providing these instructions. So instead we could reword that so it is clear what the recommendation is and why it is important without finding without sounding as if the writer is discounting the hard work that people are doing, that the nurses are doing that patients themselves, there's probably a number of reasons why discharge instructions could get ignored or misunderstood. By re wording this so that we can avoid those charged or weighted words we can avoid sounding overly biased.
Visual: Slide changes to the following: Questions: Ask Now or Later
[email protected] • Live Chat Hours
Learn More:
Check out the modules Avoiding Bias and Clarifying the Actor
Make a Paper Review Appointment!
Assist students in becoming better academic writers by providing online, asynchronous feedback by appointment.
Audio: We do have more time Michael were there any questions that came in during the second half of the webinar that might be helpful to discuss?
Michael: It has been pretty quiet in the Q&A box so we are good to continue on in the webinar.
Kacy: I just want to thank you again for participating and I will hand it off to you Michael to close out.
Michael: Thanks Kacy sounds good. If you have questions after this webinar, feel free to reach out to the writing center with those. We have a general email address here [email protected] that’s an email address that we monitor daily so you can send us a question there and we will get back to you with a response within 24 hours. Also, there are certain times when we offer a live chat service where there will also be a writing instructor like myself or Kacy sitting monitoring a chat box. The hours this is available is on the writing center homepage but if you would like a quick clarification or deal one on one with a person that would be the place to go for that.
Another resource we have that could help you with avoiding bias would be our modules. You could find a link in the middle of this slide, avoiding bias and clarifying the actor. Modules are a situation where you do a bit of reading and learning and you are quizzed on it and if that quiz method is helpful for you and learning that might be helpful to check out.
Lastly we offer paper review appointments, this would be a time where you could schedule to have a writing instructor take a look at a specific piece of writing that you have so we are able to give you one to one feedback tailored to your writing and offer you some opportunities for revisions and ways to improve as an academic writing.
These are all great services and resources for you so I would encourage you all to take advantage of them if you feel that they are necessary.
With that then, again on behalf of Kacy thank you. We are going to wrap up this webinar, I hope everyone has a great night or day. Goodbye.
[End of webinar]
- Previous Page: Practical Tips to Successfully Write in Academic American English
- Next Page: Writing for Social Change: Exploring Perspectives
- Office of Student Disability Services
Walden Resources
Departments.
- Academic Residencies
- Academic Skills
- Career Planning and Development
- Customer Care Team
- Field Experience
- Military Services
- Student Success Advising
- Writing Skills
Centers and Offices
- Center for Social Change
- Office of Academic Support and Instructional Services
- Office of Degree Acceleration
- Office of Research and Doctoral Services
- Office of Student Affairs
Student Resources
- Doctoral Writing Assessment
- Form & Style Review
- Quick Answers
- ScholarWorks
- SKIL Courses and Workshops
- Walden Bookstore
- Walden Catalog & Student Handbook
- Student Safety/Title IX
- Legal & Consumer Information
- Website Terms and Conditions
- Cookie Policy
- Accessibility
- Accreditation
- State Authorization
- Net Price Calculator
- Contact Walden
Walden University is a member of Adtalem Global Education, Inc. www.adtalem.com Walden University is certified to operate by SCHEV © 2024 Walden University LLC. All rights reserved.
Quick-Start Guide for Students
1. sign up for a hypothesis account:.
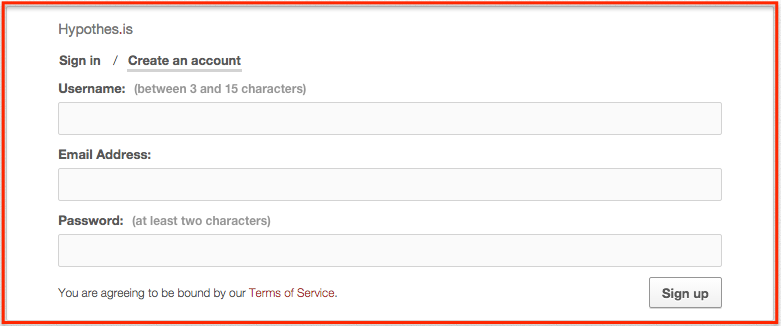
Go to the registration page. All you’ll need to sign up for a Hypothesis account is an email address and a username. You should receive a confirmation email shortly–check your spam box if not.
While you will register at the Hypothesis site, you’ll more typically be signing in through the annotation sidebar after activating Hypothesis using the Chrome extension or some other means:
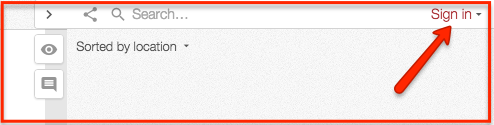
2. Get the Chrome extension:
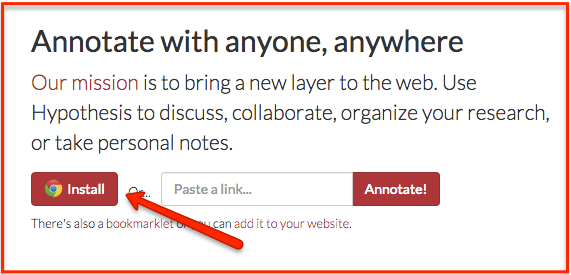
Because Chrome is the optimal browser for using Hypothesis, we recommend downloading Chrome on your personal computer if you haven’t already and adding our Chrome extension. You can do so at our website or through the Chrome store . Here’s a tutorial slideshow on how to install the Chrome extension . And here’s a video that walks you through the process:
https://www.youtube.com/watch?v=MjSpol-oPm4
Note: There are other ways to use Hypothesis outside of the Chrome extension (like a bookmarklet for other browsers , but unless you are annotating on a website where Hypothesis is automatically embedded, we recommend using the Chrome extension.)
3. “Go Forth and Annotate”:
Navigate to a webpage that you are assigned to annotate and activate the Chrome extension by pressing the greyed out button in the upper righthand corner of the browser:
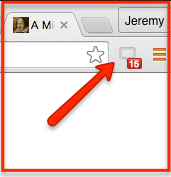
When activated, the extension button will darken and the Hypothesis side bar will appear collapsed:
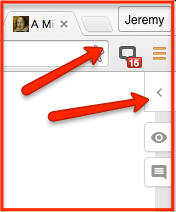
Click highlighted text to see existing annotation–you can practice on this very page, indeed this very highlighted phrase:
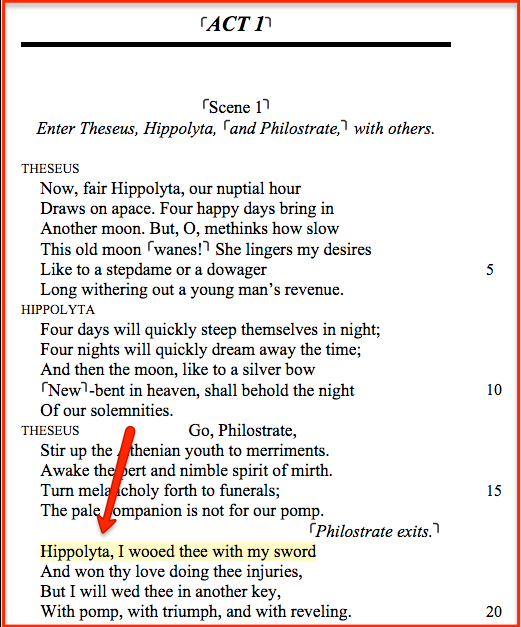
Expand the sidebar to scroll through the existing annotations.
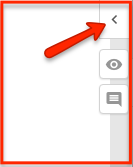
To create your own annotation, select text, click the “New Note” button–the other button is for private highlights.
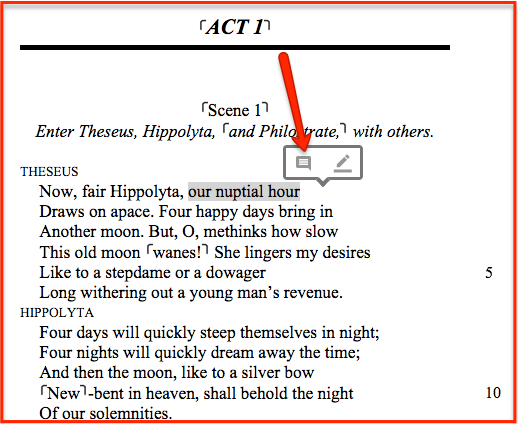
Enter your annotation content in the composition window and click “Post to Public”–you can also click the adjacent arrow to make the annotation private or “Only Me”:
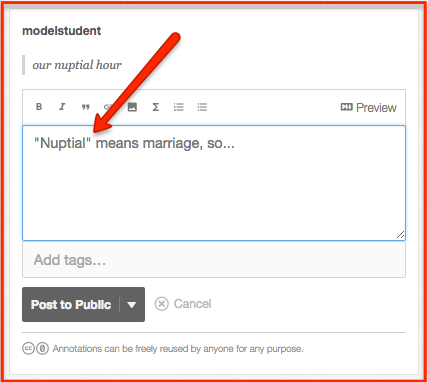
Note: You can always view all your annotations in a stream at your “My Annotations” page on Hypothesis:
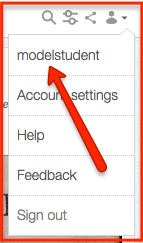
Your annotations are editable and deletable there as well.
Add images, links, and video, or format your text using the editing buttons at the top of the composition window:
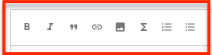
You can also make page-level annotations by clicking the same note button in the sidebar.
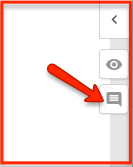
See this blog for a full explanation of the different types of annotation that can be made in Hypothesis, including replies. For tips on best annotation practices (how much text to select, when and how to add images and links, etc.), check out this page .
4. If you have been instructed to annotate as part of a group:
Follow the group link your teacher or professor has shared with the class in order to join the group:

Click the button to join the group–you may be asked to sign in first if you have not already done so:
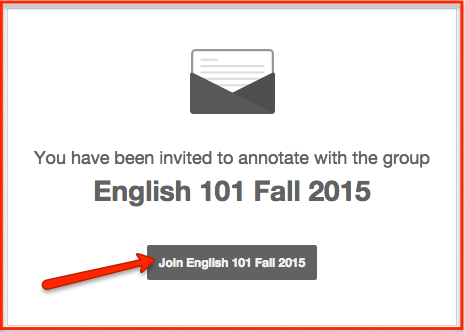
Next, navigate to the text you are assigned to annotate. Toggle the view finder in the upper left of the hypothes,is sidebar to the group name–the default is the “Public” annotation layer:
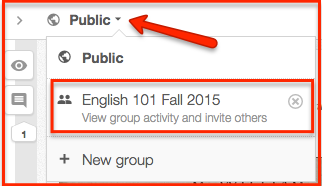
Once you and your classmates have annotated texts as part of the group, links to those pages will appear on the group’s home page as seen here:
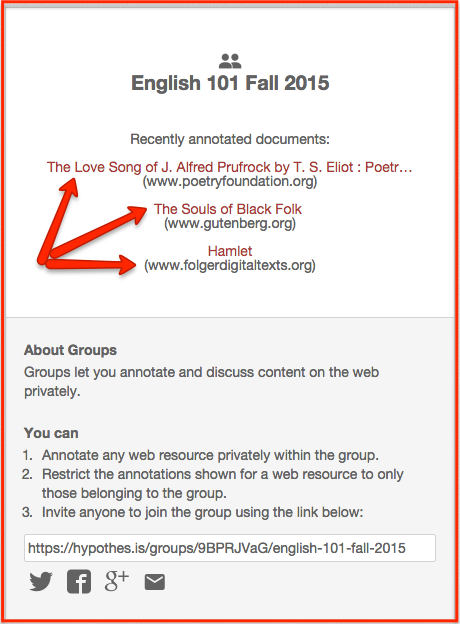
For a more in-depth tutorial on joining and annotating as part of a hypothes.is group, follow this link .
Visit our Student Resource Guide for more guides and tutorials about using Hypothesis in the classroom.
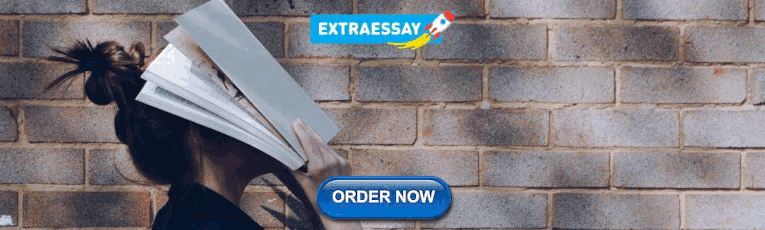
IMAGES
VIDEO
COMMENTS
For example, use "we interviewed participants" rather than "the authors interviewed participants." When writing an APA Style paper by yourself, use the first-person pronoun "I" to refer to yourself. And use the pronoun "we" when writing an APA Style paper with others. Here are some phrases you might use in your paper:
Developing a hypothesis (with example) Step 1. Ask a question. Writing a hypothesis begins with a research question that you want to answer. The question should be focused, specific, and researchable within the constraints of your project. Example: Research question.
Learning how to write a hypothesis comes down to knowledge and strategy. So where do you start? Learn how to make your hypothesis strong step-by-step here.
Using First-Person Pronouns. In most academic writing, first-person pronouns should be avoided. For instance, when writing a research project, words such as "I," "we," "my," or "our" should probably not be used. The same principle applies to lab reports, research papers, literature reviews, and rhetorical analyses, among many ...
Simple Hypothesis Examples. Increasing the amount of natural light in a classroom will improve students' test scores. Drinking at least eight glasses of water a day reduces the frequency of headaches in adults. Plant growth is faster when the plant is exposed to music for at least one hour per day.
1. Select a topic. Pick a topic that interests you, and that you think it would be good to know more about. [2] If you are writing a hypothesis for a school assignment, this step may be taken care of for you. 2. Read existing research. Gather all the information you can about the topic you've selected.
Many researchers use this hypothesis to explore the relationship between variables rather than the groups. Non-directional; You can take a guess from the name. This type of H1 does not provide a specific prediction for the research outcome. ... First, identify the problem or question you want to address. State your assumption or belief about ...
Present the findings in your results and discussion section. Though the specific details might vary, the procedure you will use when testing a hypothesis will always follow some version of these steps. Table of contents. Step 1: State your null and alternate hypothesis. Step 2: Collect data. Step 3: Perform a statistical test.
Step 5: Phrase your hypothesis in three ways. To identify the variables, you can write a simple prediction in if … then form. The first part of the sentence states the independent variable and the second part states the dependent variable. If a first-year student starts attending more lectures, then their exam scores will improve.
The steps to write a research hypothesis are: 1. Stating the problem: Ensure that the hypothesis defines the research problem. 2. Writing a hypothesis as an 'if-then' statement: Include the action and the expected outcome of your study by following a 'if-then' structure. 3.
Using first person deemphasises the data, which weakens the argument and opens the door for subjective criticism to be used to rebut what should be objective data. For example, suppose I hypothesized that the sun always rises in the east, and I make daily observations over the course of a year to support that hypothesis.
A hypothesis is a tentative statement about the relationship between two or more variables. It is a specific, testable prediction about what you expect to happen in a study. It is a preliminary answer to your question that helps guide the research process. Consider a study designed to examine the relationship between sleep deprivation and test ...
The Scientific Method is a way to study and explain the natural world, and can be used in every day applications, as well. This method consists of a logical sequence of steps that include observing a phenomenon; generating a scientific question about the phenomenon; postulating a tentative, falsifiable answer to that ...
Now it's time to state your hypothesis. The hypothesis is an educated guess as to what will happen during your experiment. The hypothesis is often written using the words "IF" and "THEN." For example, "If I do not study, then I will fail the test." The "if' and "then" statements reflect your independent and dependent variables.
Merriam Webster defines a hypothesis as "an assumption or concession made for the sake of argument.". In other words, a hypothesis is an educated guess. Scientists make a reasonable assumption--or a hypothesis--then design an experiment to test whether it's true or not.
A hypothesis is a tentative, testable answer to a scientific question. Once a scientist has a scientific question she is interested in, the scientist reads up to find out what is already known on the topic. Then she uses that information to form a tentative answer to her scientific question. Sometimes people refer to the tentative answer as "an ...
They often do inferential statistics without understanding the reasoning behind it, as if they were following a cooking recipe which does not require any thinking. ... a hypothesis test first requires an idea, that is, an assumption about a phenomenon. This assumption, referred as hypothesis, is derived from the theory and/or the research ...
When writing in APA Style, you can use the first person point of view when discussing your research steps ("I studied ...") and when referring to yourself and your co-authors ("We examined the literature ..."). Use first person to discuss research steps rather than anthropomorphising the work. For example, a study cannot "control" or "interpret ...
If the P-value is greater than ($α$) 0.05: the results are not statistically significant, and they don't reject the null hypothesis, remaining unsure if the drug has a genuine effect. 4. Example in python. For simplicity, let's say we're using a t-test (common for comparing means). Let's dive into Python:
Hypothesis testing is a crucial procedure to perform when you want to make inferences about a population using a random sample. These inferences include estimating population properties such as the mean, differences between means, proportions, and the relationships between variables. This post provides an overview of statistical hypothesis testing.
I am going to summarize this or I will conclude with so using that first person to let your reader know that this is what they can expect is a really good use of first-person. Visual: Slide changes to the following: When to Use the First Person: To avoid passive voice. A healthcare initiative will be suggested. I will suggest a healthcare ...
Quick-Start Guide for Students. 1. Sign up for a Hypothesis account: Go to the registration page. All you'll need to sign up for a Hypothesis account is an email address and a username. You should receive a confirmation email shortly-check your spam box if not. While you will register at the Hypothesis site, you'll more typically be ...