Buy new: $136.76

Download the free Kindle app and start reading Kindle books instantly on your smartphone, tablet, or computer - no Kindle device required .
Read instantly on your browser with Kindle for Web.
Using your mobile phone camera - scan the code below and download the Kindle app.

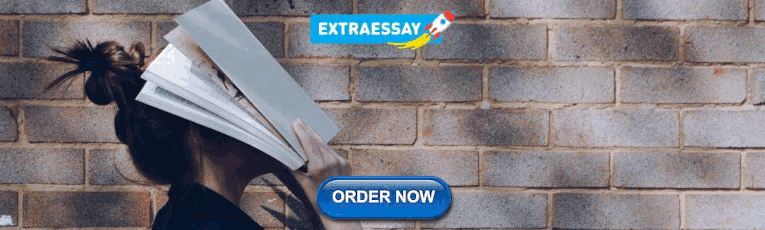
Image Unavailable

- To view this video download Flash Player
Artificial Intelligence in Education: Emerging Technologies, Models and Applications: Proceedings of 2021 2nd International Conference on Artificial ... and Communications Technologies, 104) 1st ed. 2022 Edition
Purchase options and add-ons.
This edited book is a collection of selected research papers presented at the 2021 2nd International Conference on Artificial Intelligence in Education Technology (AIET 2021), held in Wuhan, China on July 2-4, 2021. AIET establishes a platform for AI in education researchers to present research, exchange innovative ideas, propose new models, as well as demonstrate advanced methodologies and novel systems. Rapid developments in artificial intelligence (AI) and the disruptive potential of AI in educational use has drawn significant attention from the education community in recent years. For educators entering this uncharted territory, many theoretical and practical questions concerning AI in education are raised, and issues on AI’s technical, pedagogical, administrative and socio-cultural implications are being debated.
The book provides a comprehensive picture of the current status, emerging trends, innovations, theory, applications, challenges and opportunities of currentAI in education research. This timely publication is well-aligned with UNESCO’s Beijing Consensus on Artificial Intelligence (AI) and Education. It is committed to exploring how best to prepare our students and harness emerging technologies for achieving the Education 2030 Agenda as we move towards an era in which AI is transforming many aspects of our lives. Providing a broad coverage of recent technology-driven advances and addressing a number of learning-centric themes, the book is an informative and useful resource for researchers, practitioners, education leaders and policy-makers who are involved or interested in AI and education.
- ISBN-10 9811675260
- ISBN-13 978-9811675263
- Edition 1st ed. 2022
- Publisher Springer
- Publication date March 18, 2022
- Language English
- Dimensions 6.14 x 0.88 x 9.21 inches
- Print length 391 pages
- See all details

Editorial Reviews
From the back cover, about the author.
Dr. Eric Cheng is a specialist in educational management, knowledge management and Lesson Study. He is currently associate professor of the Department of Curriculum and Instruction of the Education University of Hong Kong. He is now serving as a school manager of Pentecostal Yu Leung Fat Primary School, C.C.C. Tam Lee Lai Fun Memorial Secondary School and E.L.C.H.K. Lutheran Secondary School. He is an Associate Editor of the International Journal of Educational Administration and Policy Studies (IJEAPS), a visiting scholar of Nagoya University, Aichi University of Education and Budapest Metropolitan University, an external examiner of The Open University of Hong Kong on the Master of Education programme and doctoral thesis examiner of Nottingham University on Doctor of Education program.
Dr. Rekha Koul is Dean International, Faculty of Humanities and Associate Professor at STEM Research Group, School of Education, Curtin University, Australia. She has nearly three decades of teaching and research experience. Her expertise lies in the development, refinement and validation of questionnaires; investigations of the effects of classroom environments on student outcomes; evaluation of educational programs; teacher-action research aimed at improving their environments and evaluation of curriculum. She has successfully secured many local, national and international research grants to the value of over one million dollars. Her publication record includes two books authored, seven books edited, eight book chapters and many journal articles published in peer-reviewed journals. She has delivered invited key note addresses and also conducts teacher professional learning workshops.
Rekha convenes International Conference on Science, Mathematics and Technology Education (icSTEM), a biannual conference in different parts of the world and has established a Learning Environment Research Centre in Indonesia.
She was the elected chair of Jumki Basu Scholarship (NARST 2016-18) and Programme Chair for Learning Environment SIG (AERA).
Dr. Tianchong Alex Wang is currently a Post-doctoral Fellow in the Department of Curriculum and Instruction at The Education University of Hong Kong. He received an EdD degree from the Chinese University of Hong Kong, an MSc degree from the University of Hong Kong, and a BA Hons degree from Hong Kong Baptist University. Dr Wang has a solid technical background in Information and Communication Technologies (ICTs); he is holding a number of professional qualifications such MCP, MCSA, MCSE, MCDBA, MCTS, MCITP, ACSP, ACTC, and THXCP. He is also a member of IEEE. As an advocate of using digital technologies to enhance education quality and inclusion., Dr Wang’s current research interests include AI in assessment, blended learning, mobile learning, flipped classrooms, ICT-enabled learning at scale, and digital learning for development. He has been participating research projects commissioned or supported by international organisations such as UNESCO, IDRC and The HEADFoundation as well as local research funding bodies such as Education Bureau (EDB) and The University Grants Committee (UGC) of Hong Kong. Dr Wang is a passionate teacher educator. He serves as a PT Lecturer at the University of Hong Kong who teaches in the MSc (IT in Education) programme as well as a resource person who conducts teacher capacity-building activities for international organisations and government agencies.
Product details
- Publisher : Springer; 1st ed. 2022 edition (March 18, 2022)
- Language : English
- Hardcover : 391 pages
- ISBN-10 : 9811675260
- ISBN-13 : 978-9811675263
- Item Weight : 1.66 pounds
- Dimensions : 6.14 x 0.88 x 9.21 inches
- #2,202 in Artificial Intelligence (Books)
- #2,628 in Database Storage & Design
- #6,288 in Statistics (Books)
Customer reviews
Customer Reviews, including Product Star Ratings help customers to learn more about the product and decide whether it is the right product for them.
To calculate the overall star rating and percentage breakdown by star, we don’t use a simple average. Instead, our system considers things like how recent a review is and if the reviewer bought the item on Amazon. It also analyzed reviews to verify trustworthiness.
No customer reviews
- Amazon Newsletter
- About Amazon
- Accessibility
- Sustainability
- Press Center
- Investor Relations
- Amazon Devices
- Amazon Science
- Sell on Amazon
- Sell apps on Amazon
- Supply to Amazon
- Protect & Build Your Brand
- Become an Affiliate
- Become a Delivery Driver
- Start a Package Delivery Business
- Advertise Your Products
- Self-Publish with Us
- Become an Amazon Hub Partner
- › See More Ways to Make Money
- Amazon Visa
- Amazon Store Card
- Amazon Secured Card
- Amazon Business Card
- Shop with Points
- Credit Card Marketplace
- Reload Your Balance
- Amazon Currency Converter
- Your Account
- Your Orders
- Shipping Rates & Policies
- Amazon Prime
- Returns & Replacements
- Manage Your Content and Devices
- Recalls and Product Safety Alerts
- Conditions of Use
- Privacy Notice
- Consumer Health Data Privacy Disclosure
- Your Ads Privacy Choices

Artificial intelligence in education
Artificial Intelligence (AI) has the potential to address some of the biggest challenges in education today, innovate teaching and learning practices, and accelerate progress towards SDG 4. However, rapid technological developments inevitably bring multiple risks and challenges, which have so far outpaced policy debates and regulatory frameworks. UNESCO is committed to supporting Member States to harness the potential of AI technologies for achieving the Education 2030 Agenda, while ensuring that its application in educational contexts is guided by the core principles of inclusion and equity. UNESCO’s mandate calls inherently for a human-centred approach to AI . It aims to shift the conversation to include AI’s role in addressing current inequalities regarding access to knowledge, research and the diversity of cultural expressions and to ensure AI does not widen the technological divides within and between countries. The promise of “AI for all” must be that everyone can take advantage of the technological revolution under way and access its fruits, notably in terms of innovation and knowledge.
Furthermore, UNESCO has developed within the framework of the Beijing Consensus a publication aimed at fostering the readiness of education policy-makers in artificial intelligence. This publication, Artificial Intelligence and Education: Guidance for Policy-makers , will be of interest to practitioners and professionals in the policy-making and education communities. It aims to generate a shared understanding of the opportunities and challenges that AI offers for education, as well as its implications for the core competencies needed in the AI era

The UNESCO Courier, October-December 2023

- Plurilingual
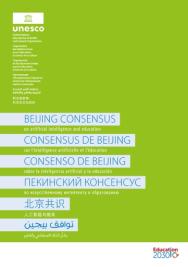
by Stefania Giannini, UNESCO Assistant Director-General for Education
International Forum on artificial intelligence and education
- More information
- Analytical report

Through its projects, UNESCO affirms that the deployment of AI technologies in education should be purposed to enhance human capacities and to protect human rights for effective human-machine collaboration in life, learning and work, and for sustainable development. Together with partners, international organizations, and the key values that UNESCO holds as pillars of their mandate, UNESCO hopes to strengthen their leading role in AI in education, as a global laboratory of ideas, standard setter, policy advisor and capacity builder. If you are interested in leveraging emerging technologies like AI to bolster the education sector, we look forward to partnering with you through financial, in-kind or technical advice contributions. 'We need to renew this commitment as we move towards an era in which artificial intelligence – a convergence of emerging technologies – is transforming every aspect of our lives (…),' said Ms Stefania Giannini, UNESCO Assistant Director-General for Education at the International Conference on Artificial Intelligence and Education held in Beijing in May 2019. 'We need to steer this revolution in the right direction, to improve livelihoods, to reduce inequalities and promote a fair and inclusive globalization.’'

Related items
- Artificial intelligence
International Conference on Artificial Intelligence in Education Technology
- 30 June - 2 July
- Berlin, Germany
Artificial Intelligence in Education Technologies: New Development and Innovative Practices
- Wuhan, China
Artificial Intelligence in Education: Emerging Technologies, Models and Applications
- Research article
- Open access
- Published: 24 April 2023
Artificial intelligence in higher education: the state of the field
- Helen Crompton ORCID: orcid.org/0000-0002-1775-8219 1 , 3 &
- Diane Burke 2
International Journal of Educational Technology in Higher Education volume 20 , Article number: 22 ( 2023 ) Cite this article
75k Accesses
66 Citations
59 Altmetric
Metrics details
This systematic review provides unique findings with an up-to-date examination of artificial intelligence (AI) in higher education (HE) from 2016 to 2022. Using PRISMA principles and protocol, 138 articles were identified for a full examination. Using a priori, and grounded coding, the data from the 138 articles were extracted, analyzed, and coded. The findings of this study show that in 2021 and 2022, publications rose nearly two to three times the number of previous years. With this rapid rise in the number of AIEd HE publications, new trends have emerged. The findings show that research was conducted in six of the seven continents of the world. The trend has shifted from the US to China leading in the number of publications. Another new trend is in the researcher affiliation as prior studies showed a lack of researchers from departments of education. This has now changed to be the most dominant department. Undergraduate students were the most studied students at 72%. Similar to the findings of other studies, language learning was the most common subject domain. This included writing, reading, and vocabulary acquisition. In examination of who the AIEd was intended for 72% of the studies focused on students, 17% instructors, and 11% managers. In answering the overarching question of how AIEd was used in HE, grounded coding was used. Five usage codes emerged from the data: (1) Assessment/Evaluation, (2) Predicting, (3) AI Assistant, (4) Intelligent Tutoring System (ITS), and (5) Managing Student Learning. This systematic review revealed gaps in the literature to be used as a springboard for future researchers, including new tools, such as Chat GPT.
A systematic review examining AIEd in higher education (HE) up to the end of 2022.
Unique findings in the switch from US to China in the most studies published.
A two to threefold increase in studies published in 2021 and 2022 to prior years.
AIEd was used for: Assessment/Evaluation, Predicting, AI Assistant, Intelligent Tutoring System, and Managing Student Learning.
Introduction
The use of artificial intelligence (AI) in higher education (HE) has risen quickly in the last 5 years (Chu et al., 2022 ), with a concomitant proliferation of new AI tools available. Scholars (viz., Chen et al., 2020 ; Crompton et al., 2020 , 2021 ) report on the affordances of AI to both instructors and students in HE. These benefits include the use of AI in HE to adapt instruction to the needs of different types of learners (Verdú et al., 2017 ), in providing customized prompt feedback (Dever et al., 2020 ), in developing assessments (Baykasoğlu et al., 2018 ), and predict academic success (Çağataylı & Çelebi, 2022 ). These studies help to inform educators about how artificial intelligence in education (AIEd) can be used in higher education.
Nonetheless, a gap has been highlighted by scholars (viz., Hrastinski et al., 2019 ; Zawacki-Richter et al., 2019 ) regarding an understanding of the collective affordances provided through the use of AI in HE. Therefore, the purpose of this study is to examine extant research from 2016 to 2022 to provide an up-to-date systematic review of how AI is being used in the HE context.
Artificial intelligence has become pervasive in the lives of twenty-first century citizens and is being proclaimed as a tool that can be used to enhance and advance all sectors of our lives (Górriz et al., 2020 ). The application of AI has attracted great interest in HE which is highly influenced by the development of information and communication technologies (Alajmi et al., 2020 ). AI is a tool used across subject disciplines, including language education (Liang et al., 2021 ), engineering education (Shukla et al., 2019 ), mathematics education (Hwang & Tu, 2021 ) and medical education (Winkler-Schwartz et al., 2019 ),
Artificial intelligence
The term artificial intelligence is not new. It was coined in 1956 by McCarthy (Cristianini, 2016 ) who followed up on the work of Turing (e.g., Turing, 1937 , 1950 ). Turing described the existence of intelligent reasoning and thinking that could go into intelligent machines. The definition of AI has grown and changed since 1956, as there has been significant advancements in AI capabilities. A current definition of AI is “computing systems that are able to engage in human-like processes such as learning, adapting, synthesizing, self-correction and the use of data for complex processing tasks” (Popenici et al., 2017 , p. 2). The interdisciplinary interest from scholars from linguistics, psychology, education, and neuroscience who connect AI to nomenclature, perceptions and knowledge in their own disciplines could create a challenge when defining AI. This has created the need to create categories of AI within specific disciplinary areas. This paper focuses on the category of AI in Education (AIEd) and how AI is specifically used in higher educational contexts.
As the field of AIEd is growing and changing rapidly, there is a need to increase the academic understanding of AIEd. Scholars (viz., Hrastinski et al., 2019 ; Zawacki-Richter et al., 2019 ) have drawn attention to the need to increase the understanding of the power of AIEd in educational contexts. The following section provides a summary of the previous research regarding AIEd.
Extant systematic reviews
This growing interest in AIEd has led scholars to investigate the research on the use of artificial intelligence in education. Some scholars have conducted systematic reviews to focus on a specific subject domain. For example, Liang et. al. ( 2021 ) conducted a systematic review and bibliographic analysis the roles and research foci of AI in language education. Shukla et. al. ( 2019 ) focused their longitudinal bibliometric analysis on 30 years of using AI in Engineering. Hwang and Tu ( 2021 ) conducted a bibliometric mapping analysis on the roles and trends in the use of AI in mathematics education, and Winkler-Schwartz et. al. ( 2019 ) specifically examined the use of AI in medical education in looking for best practices in the use of machine learning to assess surgical expertise. These studies provide a specific focus on the use of AIEd in HE but do not provide an understanding of AI across HE.
On a broader view of AIEd in HE, Ouyang et. al. ( 2022 ) conducted a systematic review of AIEd in online higher education and investigated the literature regarding the use of AI from 2011 to 2020. The findings show that performance prediction, resource recommendation, automatic assessment, and improvement of learning experiences are the four main functions of AI applications in online higher education. Salas-Pilco and Yang ( 2022 ) focused on AI applications in Latin American higher education. The results revealed that the main AI applications in higher education in Latin America are: (1) predictive modeling, (2) intelligent analytics, (3) assistive technology, (4) automatic content analysis, and (5) image analytics. These studies provide valuable information for the online and Latin American context but not an overarching examination of AIEd in HE.
Studies have been conducted to examine HE. Hinojo-Lucena et. al. ( 2019 ) conducted a bibliometric study on the impact of AIEd in HE. They analyzed the scientific production of AIEd HE publications indexed in Web of Science and Scopus databases from 2007 to 2017. This study revealed that most of the published document types were proceedings papers. The United States had the highest number of publications, and the most cited articles were about implementing virtual tutoring to improve learning. Chu et. al. ( 2022 ) reviewed the top 50 most cited articles on AI in HE from 1996 to 2020, revealing that predictions of students’ learning status were most frequently discussed. AI technology was most frequently applied in engineering courses, and AI technologies most often had a role in profiling and prediction. Finally, Zawacki-Richter et. al. ( 2019 ) analyzed AIEd in HE from 2007 to 2018 to reveal four primary uses of AIEd: (1) profiling and prediction, (2) assessment and evaluation, (3) adaptive systems and personalization, and (4) intelligent tutoring systems. There do not appear to be any studies examining the last 2 years of AIEd in HE, and these authors describe the rapid speed of both AI development and the use of AIEd in HE and call for further research in this area.
Purpose of the study
The purpose of this study is in response to the appeal from scholars (viz., Chu et al., 2022 ; Hinojo-Lucena et al., 2019 ; Zawacki-Richter et al., 2019 ) to research to investigate the benefits and challenges of AIEd within HE settings. As the academic knowledge of AIEd HE finished with studies examining up to 2020, this study provides the most up-to-date analysis examining research through to the end of 2022.
The overarching question for this study is: what are the trends in HE research regarding the use of AIEd? The first two questions provide contextual information, such as where the studies occurred and the disciplines AI was used in. These contextual details are important for presenting the main findings of the third question of how AI is being used in HE.
In what geographical location was the AIEd research conducted, and how has the trend in the number of publications evolved across the years?
What departments were the first authors affiliated with, and what were the academic levels and subject domains in which AIEd research was being conducted?
Who are the intended users of the AI technologies and what are the applications of AI in higher education?
A PRISMA systematic review methodology was used to answer three questions guiding this study. PRISMA principles (Page et al., 2021 ) were used throughout the study. The PRISMA extension Preferred Reporting Items for Systematic Reviews and Meta-Analysis for Protocols (PRISMA-P; Moher et al., 2015 ) were utilized in this study to provide an a priori roadmap to conduct a rigorous systematic review. Furthermore, the Preferred Reporting Items for Systematic Reviews and Meta-Analysis (PRISMA principles; Page et al., 2021 ) were used to search, identify, and select articles to be included in the research were used for searching, identifying, and selecting articles, then in how to read, extract, and manage the secondary data gathered from those studies (Moher et al., 2015 , PRISMA Statement, 2021 ). This systematic review approach supports an unbiased synthesis of the data in an impartial way (Hemingway & Brereton, 2009 ). Within the systematic review methodology, extracted data were aggregated and presented as whole numbers and percentages. A qualitative deductive and inductive coding methodology was also used to analyze extant data and generate new theories on the use of AI in HE (Gough et al., 2017 ).
The research begins with the search for the research articles to be included in the study. Based on the research question, the study parameters are defined including the search years, quality and types of publications to be included. Next, databases and journals are selected. A Boolean search is created and used for the search of those databases and journals. Once a set of publications are located from those searches, they are then examined against an inclusion and exclusion criteria to determine which studies will be included in the final study. The relevant data to match the research questions is then extracted from the final set of studies and coded. This method section is organized to describe each of these methods with full details to ensure transparency.
Search strategy
Only peer-reviewed journal articles were selected for examination in this systematic review. This ensured a level of confidence in the quality of the studies selected (Gough et al., 2017 ). The search parameters narrowed the search focus to include studies published in 2016 to 2022. This timeframe was selected to ensure the research was up to date, which is especially important with the rapid change in technology and AIEd.
The data retrieval protocol employed an electronic and a hand search. The electronic search included educational databases within EBSCOhost. Then an additional electronic search was conducted of Wiley Online Library, JSTOR, Science Direct, and Web of Science. Within each of these databases a full text search was conducted. Aligned to the research topic and questions, the Boolean search included terms related to AI, higher education, and learning. The Boolean search is listed in Table 1 . In the initial test search, the terms “machine learning” OR “intelligent support” OR “intelligent virtual reality” OR “chatbot” OR “automated tutor” OR “intelligent agent” OR “expert system” OR “neural network” OR “natural language processing” were used. These were removed as they were subcategories of terms found in Part 1 of the search. Furthermore, inclusion of these specific AI terms resulted in a large number of computer science courses that were focused on learning about AI and not the use of AI in learning.
Part 2 of the search ensured that articles involved formal university education. The terms higher education and tertiary were both used to recognize the different terms used in different countries. The final Boolean search was “Artificial intelligence” OR AI OR “smart technologies” OR “intelligent technologies” AND “higher education” OR tertiary OR graduate OR undergraduate. Scholars (viz., Ouyang et al., 2022 ) who conducted a systematic review on AIEd in HE up to 2020 noted that they missed relevant articles from their study, and other relevant journals should intentionally be examined. Therefore, a hand search was also conducted to include an examination of other journals relevant to AIEd that may not be included in the databases. This is important as the field of AIEd is still relatively new, and journals focused on this field may not yet be indexed in databases. The hand search included: The International Journal of Learning Analytics and Artificial Intelligence in Education, the International Journal of Artificial Intelligence in Education, and Computers & Education: Artificial Intelligence.
Electronic and hand searches resulted in 371 articles for possible inclusion. The search parameters within the electronic database search narrowed the search to articles published from 2016 to 2022, per-reviewed journal articles, and duplicates. Further screening was conducted manually, as each of the 138 articles were reviewed in full by two researchers to examine a match against the inclusion and exclusion criteria found in Table 2 .
The inter-rater reliability was calculated by percentage agreement (Belur et al., 2018 ). The researchers reached a 95% agreement for the coding. Further discussion of misaligned articles resulted in a 100% agreement. This screening process against inclusion and exclusion criteria resulted in the exclusion of 237 articles. This included the duplicates and those removed as part of the inclusion and exclusion criteria, see Fig. 1 . Leaving 138 articles for inclusion in this systematic review.
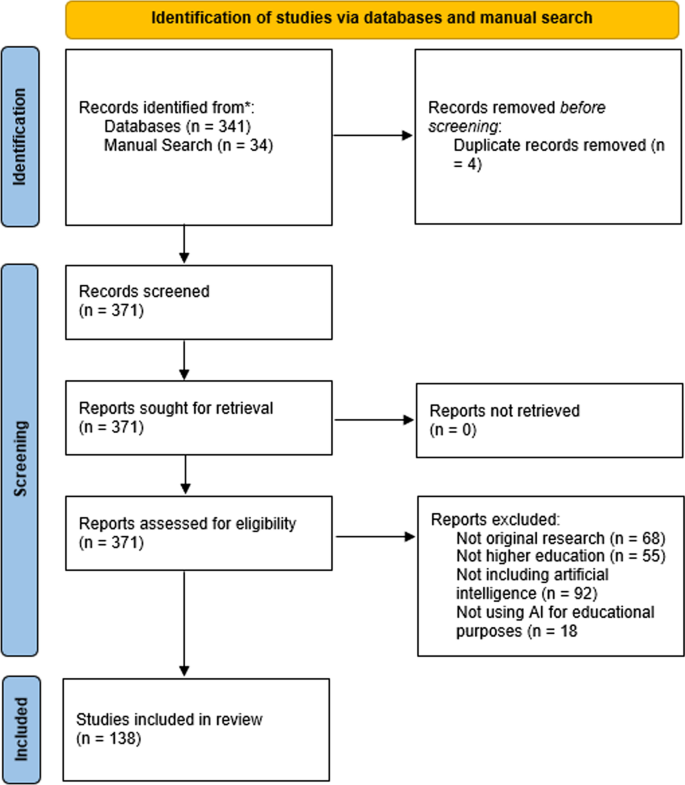
(From: Page et al., 2021 )
PRISMA flow chart of article identification and screening
The 138 articles were then coded to answer each of the research questions using deductive and inductive coding methods. Deductive coding involves examining data using a priori codes. A priori are pre-determined criteria and this process was used to code the countries, years, author affiliations, academic levels, and domains in the respective groups. Author affiliations were coded using the academic department of the first author of the study. First authors were chosen as that person is the primary researcher of the study and this follows past research practice (e.g., Zawacki-Richter et al., 2019 ). Who the AI was intended for was also coded using the a priori codes of Student, Instructor, Manager or Others. The Manager code was used for those who are involved in organizational tasks, e.g., tracking enrollment. Others was used for those not fitting the other three categories.
Inductive coding was used for the overarching question of this study in examining how the AI was being used in HE. Researchers of extant systematic reviews on AIEd in HE (viz., Chu et al., 2022 ; Zawacki-Richter et al., 2019 ) often used an a priori framework as researchers matched the use of AI to pre-existing frameworks. A grounded coding methodology (Strauss & Corbin, 1995 ) was selected for this study to allow findings of the trends on AIEd in HE to emerge from the data. This is important as it allows a direct understanding of how AI is being used rather than how researchers may think it is being used and fitting the data to pre-existing ideas.
Grounded coding process involved extracting how the AI was being used in HE from the articles. “In vivo” (Saldana, 2015 ) coding was also used alongside grounded coding. In vivo codes are when codes use language directly from the article to capture the primary authors’ language and ensure consistency with their findings. The grounded coding design used a constant comparative method. Researchers identified important text from articles related to the use of AI, and through an iterative process, initial codes led to axial codes with a constant comparison of uses of AI with uses of AI, then of uses of AI with codes, and codes with codes. Codes were deemed theoretically saturated when the majority of the data fit with one of the codes. For both the a priori and the grounded coding, two researchers coded and reached an inter-rater percentage agreement of 96%. After discussing misaligned articles, a 100% agreement was achieved.
Findings and discussion
The findings and discussion section are organized by the three questions guiding this study. The first two questions provide contextual information on the AIEd research, and the final question provides a rigorous investigation into how AI is being used in HE.
RQ1. In what geographical location was the AIEd research conducted, and how has the trend in the number of publications evolved across the years?
The 138 studies took place across 31 countries in six of seven continents of the world. Nonetheless, that distribution was not equal across continents. Asia had the largest number of AIEd studies in HE at 41%. Of the seven countries represented in Asia, 42 of the 58 studies were conducted in Taiwan and China. Europe, at 30%, was the second largest continent and had 15 countries ranging from one to eight studies a piece. North America, at 21% of the studies was the continent with the third largest number of studies, with the USA producing 21 of the 29 studies in that continent. The 21 studies from the USA places it second behind China. Only 1% of studies were conducted in South America and 2% in Africa. See Fig. 2 for a visual representation of study distribution across countries. Those continents with high numbers of studies are from high income countries and those with low numbers have a paucity of publications in low-income countries.
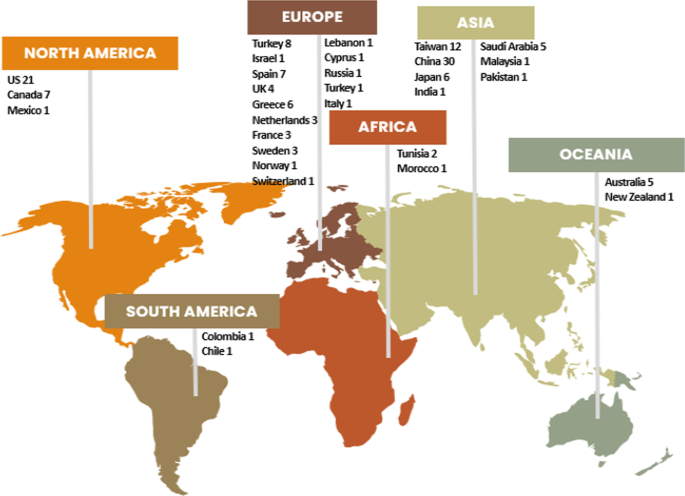
Geographical distribution of the AIEd HE studies
Data from Zawacki-Richter et. al.’s ( 2019 ) 2007–2018 systematic review examining countries found that the USA conducted the most studies across the globe at 43 out of 146, and China had the second largest at eleven of the 146 papers. Researchers have noted a rapid trend in Chinese researchers publishing more papers on AI and securing more patents than their US counterparts in a field that was originally led by the US (viz., Li et al., 2021 ). The data from this study corroborate this trend in China leading in the number of AIEd publications.
With the accelerated use of AI in society, gathering data to examine the use of AIEd in HE is useful in providing the scholarly community with specific information on that growth and if it is as prolific as anticipated by scholars (e.g., Chu et al., 2022 ). The analysis of data of the 138 studies shows that the trend towards the use of AIEd in HE has greatly increased. There is a drop in 2019, but then a great rise in 2021 and 2022; see Fig. 3 .
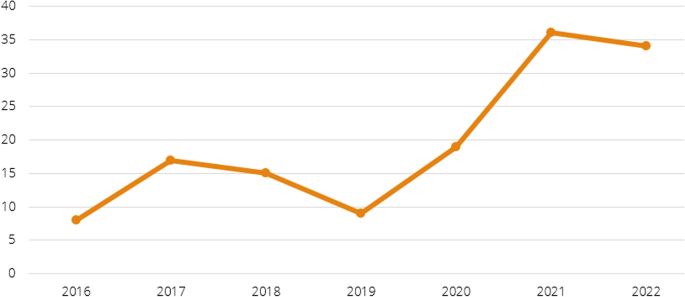
Chronological trend in AIEd in HE
Data on the rise in AIEd in HE is similar to the findings of Chu et. al. ( 2022 ) who noted an increase from 1996 to 2010 and 2011–2020. Nonetheless Chu’s parameters are across decades, and the rise is to be anticipated with a relatively new technology across a longitudinal review. Data from this study show a dramatic rise since 2020 with a 150% increase from the prior 2 years 2020–2019. The rise in 2021 and 2022 in HE could have been caused by the vast increase in HE faculty having to teach with technology during the pandemic lockdown. Faculty worldwide were using technologies, including AI, to explore how they could continue teaching and learning that was often face-to-face prior to lockdown. The disadvantage of this rapid adoption of technology is that there was little time to explore the possibilities of AI to transform learning, and AI may have been used to replicate past teaching practices, without considering new strategies previously inconceivable with the affordances of AI.
However, in a further examination of the research from 2021 to 2022, it appears that there are new strategies being considered. For example, Liu et. al.’s, 2022 study used AIEd to provide information on students’ interactions in an online environment and examine their cognitive effort. In Yao’s study in 2022, he examined the use of AI to determine student emotions while learning.
RQ2. What departments were the first authors affiliated with, and what were the academic levels and subject domains in which AIEd research was being conducted?
Department affiliations
Data from the AIEd HE studies show that of the first authors were most frequently from colleges of education (28%), followed by computer science (20%). Figure 4 presents the 15 academic affiliations of the authors found in the studies. The wide variety of affiliations demonstrate the variety of ways AI can be used in various educational disciplines, and how faculty in diverse areas, including tourism, music, and public affairs were interested in how AI can be used for educational purposes.
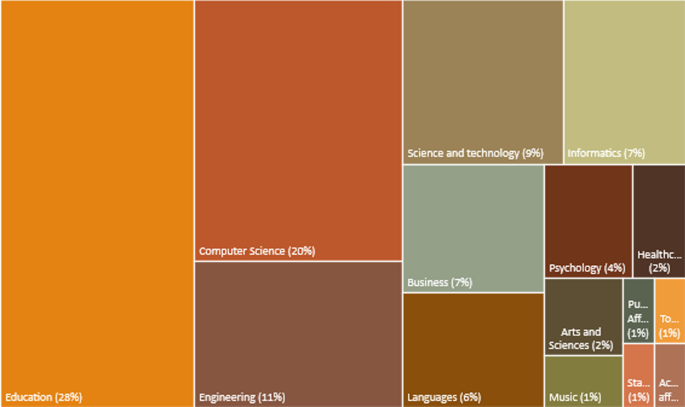
Research affiliations
In an extant AIED HE systematic review, Zawacki-Richter et. al.’s ( 2019 ) named their study Systematic review of research on artificial intelligence applications in higher education—where are the educators? In this study, the authors were keen to highlight that of the AIEd studies in HE, only six percent were written by researchers directly connected to the field of education, (i.e., from a college of education). The researchers found a great lack in pedagogical and ethical implications of implementing AI in HE and that there was a need for more educational perspectives on AI developments from educators conducting this work. It appears from our data that educators are now showing greater interest in leading these research endeavors, with the highest affiliated group belonging to education. This may again be due to the pandemic and those in the field of education needing to support faculty in other disciplines, and/or that they themselves needed to explore technologies for their own teaching during the lockdown. This may also be due to uptake in professors in education becoming familiar with AI tools also driven by a societal increased attention. As the focus of much research by education faculty is on teaching and learning, they are in an important position to be able to share their research with faculty in other disciplines regarding the potential affordances of AIEd.
Academic levels
The a priori coding of academic levels show that the majority of studies involved undergraduate students with 99 of the 138 (72%) focused on these students. This was in comparison to the 12 of 138 (9%) for graduate students. Some of the studies used AI for both academic levels: see Fig. 5
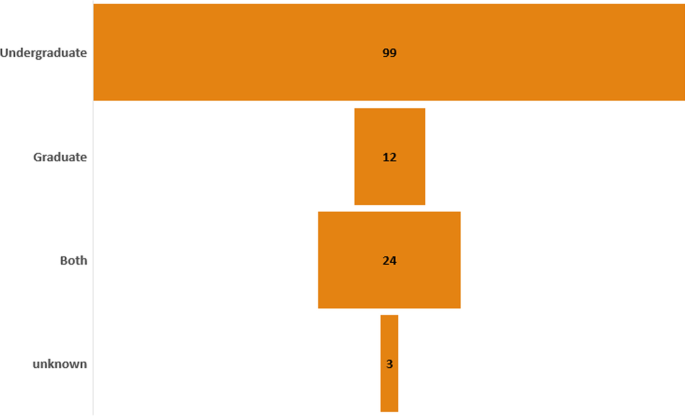
Academic level distribution by number of articles
This high percentage of studies focused on the undergraduate population was congruent with an earlier AIED HE systematic review (viz., Zawacki-Richter et al., 2019 ) who also reported student academic levels. This focus on undergraduate students may be due to the variety of affordances offered by AIEd, such as predictive analytics on dropouts and academic performance. These uses of AI may be less required for graduate students who already have a record of performance from their undergraduate years. Another reason for this demographic focus can also be convenience sampling, as researchers in HE typically has a much larger and accessible undergraduate population than graduates. This disparity between undergraduates and graduate populations is a concern, as AIEd has the potential to be valuable in both settings.
Subject domains
The studies were coded into 14 areas in HE; with 13 in a subject domain and one category of AIEd used in HE management of students; See Fig. 6 . There is not a wide difference in the percentages of top subject domains, with language learning at 17%, computer science at 16%, and engineering at 12%. The management of students category appeared third on the list at 14%. Prior studies have also found AIEd often used for language learning (viz., Crompton et al., 2021 ; Zawacki-Richter et al., 2019 ). These results are different, however, from Chu et. al.’s ( 2022 ) findings that show engineering dramatically leading with 20 of the 50 studies, with other subjects, such as language learning, appearing once or twice. This study appears to be an outlier that while the searches were conducted in similar databases, the studies only included 50 studies from 1996 to 2020.
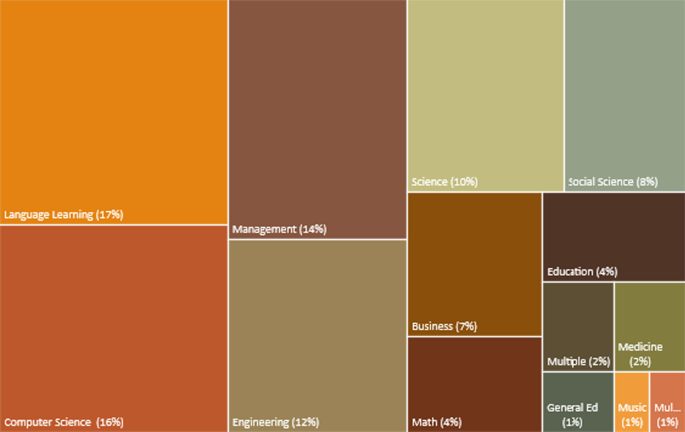
Subject domains of AIEd in HE
Previous scholars primarily focusing on language learning using AI for writing, reading, and vocabulary acquisition used the affordances of natural language processing and intelligent tutoring systems (e.g., Liang et al., 2021 ). This is similar to the findings in studies with AI used for automated feedback of writing in a foreign language (Ayse et al., 2022 ), and AI translation support (Al-Tuwayrish, 2016 ). The large use of AI for managerial activities in this systematic review focused on making predictions (12 studies) and then admissions (three studies). This is positive to see this use of AI to look across multiple databases to see trends emerging from data that may not have been anticipated and cross referenced before (Crompton et al., 2022 ). For example, to examine dropouts, researchers may consider examining class attendance, and may not examine other factors that appear unrelated. AI analysis can examine all factors and may find that dropping out is due to factors beyond class attendance.
RQ3. Who are the intended users of the AI technologies and what are the applications of AI in higher education?
Intended user of AI
Of the 138 articles, the a priori coding shows that 72% of the studies focused on Students, followed by a focus on Instructors at 17%, and Managers at 11%, see Fig. 7 . The studies provided examples of AI being used to provide support to students, such as access to learning materials for inclusive learning (Gupta & Chen, 2022 ), provide immediate answers to student questions, self-testing opportunities (Yao, 2022 ), and instant personalized feedback (Mousavi et al., 2020 ).
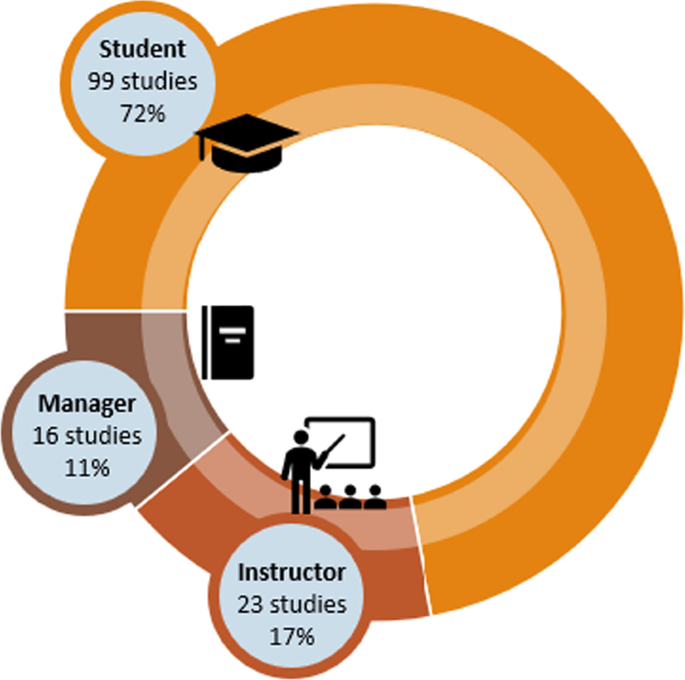
Intended user
The data revealed a large emphasis on students in the use of AIEd in HE. This user focus is different from a recent systematic review on AIEd in K-12 that found that AIEd studies in K-12 settings prioritized teachers (Crompton et al., 2022 ). This may appear that HE uses AI to focus more on students than in K-12. However, this large number of student studies in HE may be due to the student population being more easily accessibility to HE researchers who may study their own students. The ethical review process is also typically much shorter in HE than in K-12. Therefore, the data on the intended focus should be reviewed while keeping in mind these other explanations. It was interesting that Managers were the lowest focus in K-12 and also in this study in HE. AI has great potential to collect, cross reference and examine data across large datasets that can allow data to be used for actionable insight. More focus on the use of AI by managers would tap into this potential.
How is AI used in HE
Using grounded coding, the use of AIEd from each of the 138 articles was examined and six major codes emerged from the data. These codes provide insight into how AI was used in HE. The five codes are: (1) Assessment/Evaluation, (2) Predicting, (3) AI Assistant, (4) Intelligent Tutoring System (ITS), and (5) Managing Student Learning. For each of these codes there are also axial codes, which are secondary codes as subcategories from the main category. Each code is delineated below with a figure of the codes with further descriptive information and examples.
Assessment/evaluation
Assessment and Evaluation was the most common use of AIEd in HE. Within this code there were six axial codes broken down into further codes; see Fig. 8 . Automatic assessment was most common, seen in 26 of the studies. It was interesting to see that this involved assessment of academic achievement, but also other factors, such as affect.
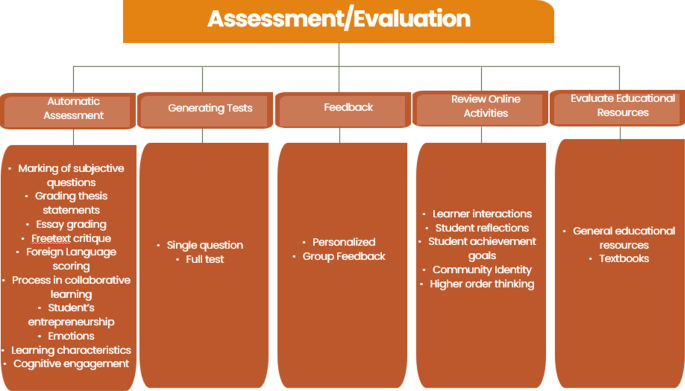
Codes and axial codes for assessment and evaluation
Automatic assessment was used to support a variety of learners in HE. As well as reducing the time it takes for instructors to grade (Rutner & Scott, 2022 ), automatic grading showed positive use for a variety of students with diverse needs. For example, Zhang and Xu ( 2022 ) used automatic assessment to improve academic writing skills of Uyghur ethnic minority students living in China. Writing has a variety of cultural nuances and in this study the students were shown to engage with the automatic assessment system behaviorally, cognitively, and affectively. This allowed the students to engage in self-regulated learning while improving their writing.
Feedback was a description often used in the studies, as students were given text and/or images as feedback as a formative evaluation. Mousavi et. al. ( 2020 ) developed a system to provide first year biology students with an automated personalized feedback system tailored to the students’ specific demographics, attributes, and academic status. With the unique feature of AIEd being able to analyze multiple data sets involving a variety of different students, AI was used to assess and provide feedback on students’ group work (viz., Ouatik et al., 2021 ).
AI also supports instructors in generating questions and creating multiple question tests (Yang et al., 2021 ). For example, (Lu et al., 2021 ) used natural language processing to create a system that automatically created tests. Following a Turing type test, researchers found that AI technologies can generate highly realistic short-answer questions. The ability for AI to develop multiple questions is a highly valuable affordance as tests can take a great deal of time to make. However, it would be important for instructors to always confirm questions provided by the AI to ensure they are correct and that they match the learning objectives for the class, especially in high value summative assessments.
The axial code within assessment and evaluation revealed that AI was used to review activities in the online space. This included evaluating student’s reflections, achievement goals, community identity, and higher order thinking (viz., Huang et al., 2021 ). Three studies used AIEd to evaluate educational materials. This included general resources and textbooks (viz., Koć‑Januchta et al., 2022 ). It is interesting to see the use of AI for the assessment of educational products, rather than educational artifacts developed by students. While this process may be very similar in nature, this shows researchers thinking beyond the traditional use of AI for assessment to provide other affordances.
Predicting was a common use of AIEd in HE with 21 studies focused specifically on the use of AI for forecasting trends in data. Ten axial codes emerged on the way AI was used to predict different topics, with nine focused on predictions regarding students and the other on predicting the future of higher education. See Fig. 9 .
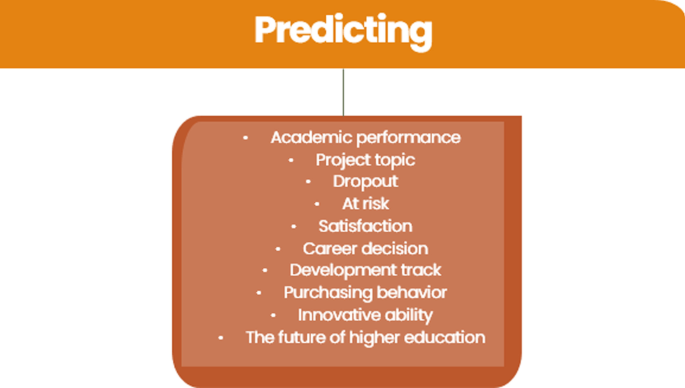
Predicting axial codes
Extant systematic reviews on HE highlighted the use of AIEd for prediction (viz., Chu et al., 2022 ; Hinojo-Lucena et al., 2019 ; Ouyang et al., 2022 ; Zawacki-Richter et al., 2019 ). Ten of the articles in this study used AI for predicting academic performance. Many of the axial codes were often overlapping, such as predicting at risk students, and predicting dropouts; however, each provided distinct affordances. An example of this is the study by Qian et. al. ( 2021 ). These researchers examined students taking a MOOC course. MOOCs can be challenging environments to determine information on individual students with the vast number of students taking the course (Krause & Lowe, 2014 ). However, Qian et al., used AIEd to predict students’ future grades by inputting 17 different learning features, including past grades, into an artificial neural network. The findings were able to predict students’ grades and highlight students at risk of dropping out of the course.
In a systematic review on AIEd within the K-12 context (viz., Crompton et al., 2022 ), prediction was less pronounced in the findings. In the K-12 setting, there was a brief mention of the use of AI in predicting student academic performance. One of the studies mentioned students at risk of dropping out, but this was immediately followed by questions about privacy concerns and describing this as “sensitive”. The use of prediction from the data in this HE systematic review cover a wide range of AI predictive affordances. students Sensitivity is still important in a HE setting, but it is positive to see the valuable insight it provides that can be used to avoid students failing in their goals.
AI assistant
The studies evaluated in this review indicated that the AI Assistant used to support learners had a variety of different names. This code included nomenclature such as, virtual assistant, virtual agent, intelligent agent, intelligent tutor, and intelligent helper. Crompton et. al. ( 2022 ), described the difference in the terms to delineate the way that the AI appeared to the user. For example, if there was an anthropomorphic presence to the AI, such as an avatar, or if the AI appeared to support via other means, such as text prompt. The findings of this systematic review align to Crompton et. al.’s ( 2022 ) descriptive differences of the AI Assistant. Furthermore, this code included studies that provide assistance to students, but may not have specifically used the word assistance. These include the use of chatbots for student outreach, answering questions, and providing other assistance. See Fig. 10 for the axial codes for AI Assistant.
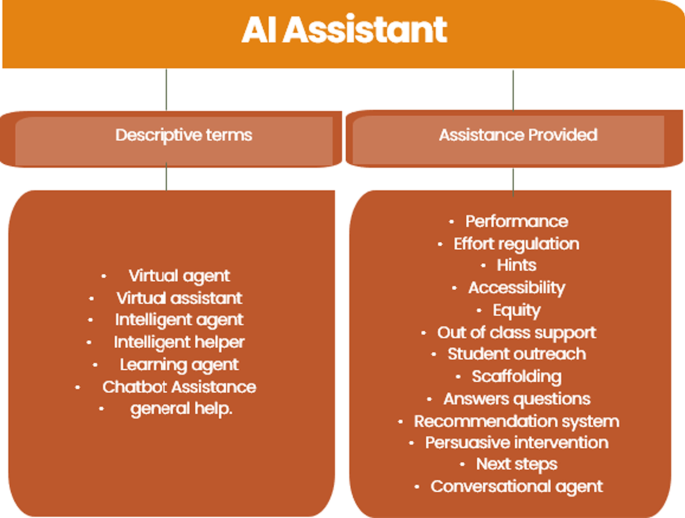
AI assistant axial codes
Many of these assistants offered multiple supports to students, such as Alex , the AI described as a virtual change agent in Kim and Bennekin’s ( 2016 ) study. Alex interacted with students in a college mathematics course by asking diagnostic questions and gave support depending on student needs. Alex’s support was organized into four stages: (1) goal initiation (“Want it”), (2) goal formation (“Plan for it”), (3) action control (“Do it”), and (4) emotion control (“Finish it”). Alex provided responses depending on which of these four areas students needed help. These messages supported students with the aim of encouraging persistence in pursuing their studies and degree programs and improving performance.
The role of AI in providing assistance connects back to the seminal work of Vygotsky ( 1978 ) and the Zone of Proximal Development (ZPD). ZPD highlights the degree to which students can rapidly develop when assisted. Vygotsky described this assistance often in the form of a person. However, with technological advancements, the use of AI assistants in these studies are providing that support for students. The affordances of AI can also ensure that the support is timely without waiting for a person to be available. Also, assistance can consider aspects on students’ academic ability, preferences, and best strategies for supporting. These features were evident in Kim and Bennekin’s ( 2016 ) study using Alex.
Intelligent tutoring system
The use of Intelligent Tutoring Systems (ITS) was revealed in the grounded coding. ITS systems are adaptive instructional systems that involve the use of AI techniques and educational methods. An ITS system customizes educational activities and strategies based on student’s characteristics and needs (Mousavinasab et al., 2021 ). While ITS may be an anticipated finding in AIED HE systematic reviews, it was interesting that extant reviews similar to this study did not always describe their use in HE. For example, Ouyang et. al. ( 2022 ), included “intelligent tutoring system” in search terms describing it as a common technique, yet ITS was not mentioned again in the paper. Zawacki-Richter et. al. ( 2019 ) on the other hand noted that ITS was in the four overarching findings of the use of AIEd in HE. Chu et. al. ( 2022 ) then used Zawacki-Richter’s four uses of AIEd for their recent systematic review.
In this systematic review, 18 studies specifically mentioned that they were using an ITS. The ITS code did not necessitate axial codes as they were performing the same type of function in HE, namely, in providing adaptive instruction to the students. For example, de Chiusole et. al. ( 2020 ) developed Stat-Knowlab, an ITS that provides the level of competence and best learning path for each student. Thus Stat-Knowlab personalizes students’ learning and provides only educational activities that the student is ready to learn. This ITS is able to monitor the evolution of the learning process as the student interacts with the system. In another study, Khalfallah and Slama ( 2018 ) built an ITS called LabTutor for engineering students. LabTutor served as an experienced instructor in enabling students to access and perform experiments on laboratory equipment while adapting to the profile of each student.
The student population in university classes can go into the hundreds and with the advent of MOOCS, class sizes can even go into the thousands. Even in small classes of 20 students, the instructor cannot physically provide immediate unique personalize questions to each student. Instructors need time to read and check answers and then take further time to provide feedback before determining what the next question should be. Working with the instructor, AIEd can provide that immediate instruction, guidance, feedback, and following questioning without delay or becoming tired. This appears to be an effective use of AIEd, especially within the HE context.
Managing student learning
Another code that emerged in the grounded coding was focused on the use of AI for managing student learning. AI is accessed to manage student learning by the administrator or instructor to provide information, organization, and data analysis. The axial codes reveal the trends in the use of AI in managing student learning; see Fig. 11 .
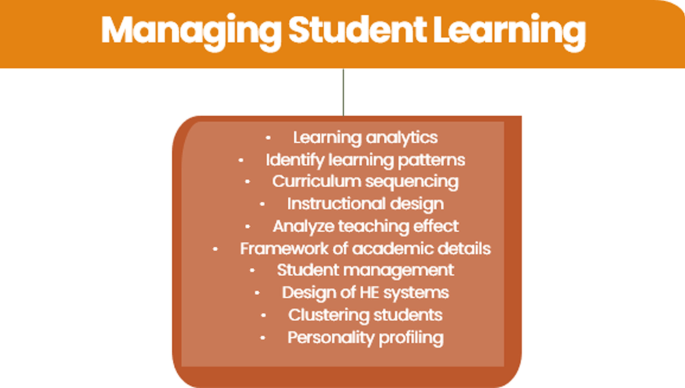
Learning analytics was an a priori term often found in studies which describes “the measurement, collection, analysis and reporting of data about learners and their contexts, for purposes of understanding and optimizing learning and the environments in which it occurs” (Long & Siemens, 2011 , p. 34). The studies investigated in this systematic review were across grades and subject areas and provided administrators and instructors different types of information to guide their work. One of those studies was conducted by Mavrikis et. al. ( 2019 ) who described learning analytics as teacher assistance tools. In their study, learning analytics were used in an exploratory learning environment with targeted visualizations supporting classroom orchestration. These visualizations, displayed as screenshots in the study, provided information such as the interactions between the students, goals achievements etc. These appear similar to infographics that are brightly colored and draw the eye quickly to pertinent information. AI is also used for other tasks, such as organizing the sequence of curriculum in pacing guides for future groups of students and also designing instruction. Zhang ( 2022 ) described how designing an AI teaching system of talent cultivation and using the digital affordances to establish a quality assurance system for practical teaching, provides new mechanisms for the design of university education systems. In developing such a system, Zhang found that the stability of the instructional design, overcame the drawbacks of traditional manual subjectivity in the instructional design.
Another trend that emerged from the studies was the use of AI to manage student big data to support learning. Ullah and Hafiz ( 2022 ) lament that using traditional methods, including non-AI digital techniques, asking the instructor to pay attention to every student’s learning progress is very difficult and that big data analysis techniques are needed. The ability to look across and within large data sets to inform instruction is a valuable affordance of AIEd in HE. While the use of AIEd to manage student learning emerged from the data, this study uncovered only 19 studies in 7 years (2016–2022) that focused on the use of AIEd to manage student data. This lack of the use was also noted in a recent study in the K-12 space (Crompton et al., 2022 ). In Chu et. al.’s ( 2022 ) study examining the top 50 most cited AIEd articles, they did not report the use of AIEd for managing student data in the top uses of AIEd HE. It would appear that more research should be conducted in this area to fully explore the possibilities of AI.
Gaps and future research
From this systematic review, six gaps emerged in the data providing opportunities for future studies to investigate and provide a fuller understanding of how AIEd can used in HE. (1) The majority of the research was conducted in high income countries revealing a paucity of research in developing countries. More research should be conducted in these developing countries to expand the level of understanding about how AI can enhance learning in under-resourced communities. (2) Almost 50% of the studies were conducted in the areas of language learning, computer science and engineering. Research conducted by members from multiple, different academic departments would help to advance the knowledge of the use of AI in more disciplines. (3) This study revealed that faculty affiliated with schools of education are taking an increasing role in researching the use of AIEd in HE. As this body of knowledge grows, faculty in Schools of Education should share their research regarding the pedagogical affordances of AI so that this knowledge can be applied by faculty across disciplines. (4) The vast majority of the research was conducted at the undergraduate level. More research needs to be done at the graduate student level, as AI provides many opportunities in this environment. (5) Little study was done regarding how AIEd can assist both instructors and managers in their roles in HE. The power of AI to assist both groups further research. (6) Finally, much of the research investigated in this systematic review revealed the use of AIEd in traditional ways that enhance or make more efficient current practices. More research needs to focus on the unexplored affordances of AIEd. As AI becomes more advanced and sophisticated, new opportunities will arise for AIEd. Researchers need to be on the forefront of these possible innovations.
In addition, empirical exploration is needed for new tools, such as ChatGPT that was available for public use at the end of 2022. With the time it takes for a peer review journal article to be published, ChatGPT did not appear in the articles for this study. What is interesting is that it could fit with a variety of the use codes found in this study, with students getting support in writing papers and instructors using Chat GPT to assess students work and with help writing emails or descriptions for students. It would be pertinent for researchers to explore Chat GPT.
Limitations
The findings of this study show a rapid increase in the number of AIEd studies published in HE. However, to ensure a level of credibility, this study only included peer review journal articles. These articles take months to publish. Therefore, conference proceedings and gray literature such as blogs and summaries may reveal further findings not explored in this study. In addition, the articles in this study were all published in English which excluded findings from research published in other languages.
In response to the call by Hinojo-Lucena et. al. ( 2019 ), Chu et. al. ( 2022 ), and Zawacki-Richter et. al. ( 2019 ), this study provides unique findings with an up-to-date examination of the use of AIEd in HE from 2016 to 2022. Past systematic reviews examined the research up to 2020. The findings of this study show that in 2021 and 2022, publications rose nearly two to three times the number of previous years. With this rapid rise in the number of AIEd HE publications, new trends have emerged.
The findings show that of the 138 studies examined, research was conducted in six of the seven continents of the world. In extant systematic reviews showed that the US led by a large margin in the number of studies published. This trend has now shifted to China. Another shift in AIEd HE is that while extant studies lamented the lack of focus on professors of education leading these studies, this systematic review found education to be the most common department affiliation with 28% and computer science coming in second at 20%. Undergraduate students were the most studied students at 72%. Similar to the findings of other studies, language learning was the most common subject domain. This included writing, reading, and vocabulary acquisition. In examination of who the AIEd was intended for, 72% of the studies focused on students, 17% instructors, and 11% managers.
Grounded coding was used to answer the overarching question of how AIEd was used in HE. Five usage codes emerged from the data: (1) Assessment/Evaluation, (2) Predicting, (3) AI Assistant, (4) Intelligent Tutoring System (ITS), and (5) Managing Student Learning. Assessment and evaluation had a wide variety of purposes, including assessing academic progress and student emotions towards learning, individual and group evaluations, and class based online community assessments. Predicting emerged as a code with ten axial codes, as AIEd predicted dropouts and at-risk students, innovative ability, and career decisions. AI Assistants were specific to supporting students in HE. These assistants included those with an anthropomorphic presence, such as virtual agents and persuasive intervention through digital programs. ITS systems were not always noted in extant systematic reviews but were specifically mentioned in 18 of the studies in this review. ITS systems in this study provided customized strategies and approaches to student’s characteristics and needs. The final code in this study highlighted the use of AI in managing student learning, including learning analytics, curriculum sequencing, instructional design, and clustering of students.
The findings of this study provide a springboard for future academics, practitioners, computer scientists, policymakers, and funders in understanding the state of the field in AIEd HE, how AI is used. It also provides actionable items to ameliorate gaps in the current understanding. As the use AIEd will only continue to grow this study can serve as a baseline for further research studies in the use of AIEd in HE.
Availability of data and materials
The datasets used and/or analysed during the current study are available from the corresponding author on reasonable request.
Alajmi, Q., Al-Sharafi, M. A., & Abuali, A. (2020). Smart learning gateways for Omani HEIs towards educational technology: Benefits, challenges and solutions. International Journal of Information Technology and Language Studies, 4 (1), 12–17.
Google Scholar
Al-Tuwayrish, R. K. (2016). An evaluative study of machine translation in the EFL scenario of Saudi Arabia. Advances in Language and Literary Studies, 7 (1), 5–10.
Ayse, T., & Nil, G. (2022). Automated feedback and teacher feedback: Writing achievement in learning English as a foreign language at a distance. The Turkish Online Journal of Distance Education, 23 (2), 120–139. https://doi.org/10.7575/aiac.alls.v.7n.1p.5
Article Google Scholar
Baykasoğlu, A., Özbel, B. K., Dudaklı, N., Subulan, K., & Şenol, M. E. (2018). Process mining based approach to performance evaluation in computer-aided examinations. Computer Applications in Engineering Education, 26 (5), 1841–1861. https://doi.org/10.1002/cae.21971
Belur, J., Tompson, L., Thornton, A., & Simon, M. (2018). Interrater reliability in systematic review methodology: Exploring variation in coder decision-making. Sociological Methods & Research, 13 (3), 004912411887999. https://doi.org/10.1177/0049124118799372
Çağataylı, M., & Çelebi, E. (2022). Estimating academic success in higher education using big five personality traits, a machine learning approach. Arab Journal Scientific Engineering, 47 , 1289–1298. https://doi.org/10.1007/s13369-021-05873-4
Chen, L., Chen, P., & Lin, Z. (2020). Artificial intelligence in education: A review. IEEE Access, 8 , 75264–75278. https://doi.org/10.1109/ACCESS.2020.2988510
Chu, H., Tu, Y., & Yang, K. (2022). Roles and research trends of artificial intelligence in higher education: A systematic review of the top 50 most-cited articles. Australasian Journal of Educational Technology, 38 (3), 22–42. https://doi.org/10.14742/ajet.7526
Cristianini, N. (2016). Intelligence reinvented. New Scientist, 232 (3097), 37–41. https://doi.org/10.1016/S0262-4079(16)31992-3
Crompton, H., Bernacki, M. L., & Greene, J. (2020). Psychological foundations of emerging technologies for teaching and learning in higher education. Current Opinion in Psychology, 36 , 101–105. https://doi.org/10.1016/j.copsyc.2020.04.011
Crompton, H., & Burke, D. (2022). Artificial intelligence in K-12 education. SN Social Sciences, 2 , 113. https://doi.org/10.1007/s43545-022-00425-5
Crompton, H., Jones, M., & Burke, D. (2022). Affordances and challenges of artificial intelligence in K-12 education: A systematic review. Journal of Research on Technology in Education . https://doi.org/10.1080/15391523.2022.2121344
Crompton, H., & Song, D. (2021). The potential of artificial intelligence in higher education. Revista Virtual Universidad Católica Del Norte, 62 , 1–4. https://doi.org/10.35575/rvuen.n62a1
de Chiusole, D., Stefanutti, L., Anselmi, P., & Robusto, E. (2020). Stat-Knowlab. Assessment and learning of statistics with competence-based knowledge space theory. International Journal of Artificial Intelligence in Education, 30 , 668–700. https://doi.org/10.1007/s40593-020-00223-1
Dever, D. A., Azevedo, R., Cloude, E. B., & Wiedbusch, M. (2020). The impact of autonomy and types of informational text presentations in game-based environments on learning: Converging multi-channel processes data and learning outcomes. International Journal of Artificial Intelligence in Education, 30 (4), 581–615. https://doi.org/10.1007/s40593-020-00215-1
Górriz, J. M., Ramírez, J., Ortíz, A., Martínez-Murcia, F. J., Segovia, F., Suckling, J., Leming, M., Zhang, Y. D., Álvarez-Sánchez, J. R., Bologna, G., Bonomini, P., Casado, F. E., Charte, D., Charte, F., Contreras, R., Cuesta-Infante, A., Duro, R. J., Fernández-Caballero, A., Fernández-Jover, E., … Ferrández, J. M. (2020). Artificial intelligence within the interplay between natural and artificial computation: Advances in data science, trends and applications. Neurocomputing, 410 , 237–270. https://doi.org/10.1016/j.neucom.2020.05.078
Gough, D., Oliver, S., & Thomas, J. (2017). An introduction to systematic reviews (2nd ed.). Sage.
Gupta, S., & Chen, Y. (2022). Supporting inclusive learning using chatbots? A chatbot-led interview study. Journal of Information Systems Education, 33 (1), 98–108.
Hemingway, P. & Brereton, N. (2009). In Hayward Medical Group (Ed.). What is a systematic review? Retrieved from http://www.medicine.ox.ac.uk/bandolier/painres/download/whatis/syst-review.pdf
Hinojo-Lucena, F., Arnaz-Diaz, I., Caceres-Reche, M., & Romero-Rodriguez, J. (2019). A bibliometric study on its impact the scientific literature. Education Science . https://doi.org/10.3390/educsci9010051
Hrastinski, S., Olofsson, A. D., Arkenback, C., Ekström, S., Ericsson, E., Fransson, G., Jaldemark, J., Ryberg, T., Öberg, L.-M., Fuentes, A., Gustafsson, U., Humble, N., Mozelius, P., Sundgren, M., & Utterberg, M. (2019). Critical imaginaries and reflections on artificial intelligence and robots in postdigital K-12 education. Postdigital Science and Education, 1 (2), 427–445. https://doi.org/10.1007/s42438-019-00046-x
Huang, C., Wu, X., Wang, X., He, T., Jiang, F., & Yu, J. (2021). Exploring the relationships between achievement goals, community identification and online collaborative reflection. Educational Technology & Society, 24 (3), 210–223.
Hwang, G. J., & Tu, Y. F. (2021). Roles and research trends of artificial intelligence in mathematics education: A bibliometric mapping analysis and systematic review. Mathematics, 9 (6), 584. https://doi.org/10.3390/math9060584
Khalfallah, J., & Slama, J. B. H. (2018). The effect of emotional analysis on the improvement of experimental e-learning systems. Computer Applications in Engineering Education, 27 (2), 303–318. https://doi.org/10.1002/cae.22075
Kim, C., & Bennekin, K. N. (2016). The effectiveness of volition support (VoS) in promoting students’ effort regulation and performance in an online mathematics course. Instructional Science, 44 , 359–377. https://doi.org/10.1007/s11251-015-9366-5
Koć-Januchta, M. M., Schönborn, K. J., Roehrig, C., Chaudhri, V. K., Tibell, L. A. E., & Heller, C. (2022). “Connecting concepts helps put main ideas together”: Cognitive load and usability in learning biology with an AI-enriched textbook. International Journal of Educational Technology in Higher Education, 19 (11), 11. https://doi.org/10.1186/s41239-021-00317-3
Krause, S. D., & Lowe, C. (2014). Invasion of the MOOCs: The promise and perils of massive open online courses . Parlor Press.
Li, D., Tong, T. W., & Xiao, Y. (2021). Is China emerging as the global leader in AI? Harvard Business Review. https://hbr.org/2021/02/is-china-emerging-as-the-global-leader-in-ai
Liang, J. C., Hwang, G. J., Chen, M. R. A., & Darmawansah, D. (2021). Roles and research foci of artificial intelligence in language education: An integrated bibliographic analysis and systematic review approach. Interactive Learning Environments . https://doi.org/10.1080/10494820.2021.1958348
Liu, S., Hu, T., Chai, H., Su, Z., & Peng, X. (2022). Learners’ interaction patterns in asynchronous online discussions: An integration of the social and cognitive interactions. British Journal of Educational Technology, 53 (1), 23–40. https://doi.org/10.1111/bjet.13147
Long, P., & Siemens, G. (2011). Penetrating the fog: Analytics in learning and education. Educause Review, 46 (5), 31–40.
Lu, O. H. T., Huang, A. Y. Q., Tsai, D. C. L., & Yang, S. J. H. (2021). Expert-authored and machine-generated short-answer questions for assessing students learning performance. Educational Technology & Society, 24 (3), 159–173.
Mavrikis, M., Geraniou, E., Santos, S. G., & Poulovassilis, A. (2019). Intelligent analysis and data visualization for teacher assistance tools: The case of exploratory learning. British Journal of Educational Technology, 50 (6), 2920–2942. https://doi.org/10.1111/bjet.12876
Moher, D., Shamseer, L., Clarke, M., Ghersi, D., Liberati, A., Petticrew, M., Shekelle, P., & Stewart, L. (2015). Preferred reporting items for systematic review and meta-analysis protocols (PRISMA-P) 2015 statement. Systematic Reviews, 4 (1), 1–9. https://doi.org/10.1186/2046-4053-4-1
Mousavi, A., Schmidt, M., Squires, V., & Wilson, K. (2020). Assessing the effectiveness of student advice recommender agent (SARA): The case of automated personalized feedback. International Journal of Artificial Intelligence in Education, 31 (2), 603–621. https://doi.org/10.1007/s40593-020-00210-6
Mousavinasab, E., Zarifsanaiey, N., Kalhori, S. R. N., Rakhshan, M., Keikha, L., & Saeedi, M. G. (2021). Intelligent tutoring systems: A systematic review of characteristics, applications, and evaluation methods. Interactive Learning Environments, 29 (1), 142–163. https://doi.org/10.1080/10494820.2018.1558257
Ouatik, F., Ouatikb, F., Fadlic, H., Elgoraria, A., Mohadabb, M. E. L., Raoufia, M., et al. (2021). E-Learning & decision making system for automate students assessment using remote laboratory and machine learning. Journal of E-Learning and Knowledge Society, 17 (1), 90–100. https://doi.org/10.20368/1971-8829/1135285
Ouyang, F., Zheng, L., & Jiao, P. (2022). Artificial intelligence in online higher education: A systematic review of empirical research from 2011–2020. Education and Information Technologies, 27 , 7893–7925. https://doi.org/10.1007/s10639-022-10925-9
Page, M. J., McKenzie, J. E., Bossuyt, P. M., Boutron, I., Hoffmann, T., Mulrow, C., Shamseer, L., Tetzlaff, J. M., Akl, E. A., Brennan, S. E., Chou, R., Glanville, J., Grimshaw, J. M., Hróbjartsson, A., Lalu, M. M., Li, T., Loder, E. W., Mayo-Wilson, E., McDonald, S., … Moher, D. (2021). The PRISMA 2020 statement: An updated guideline for reporting systematic reviews. British Medical Journal . https://doi.org/10.1136/bmj.n71
Popenici, S. A. D., & Kerr, S. (2017). Exploring the impact of artificial intelligence on teaching and learning in higher education. Research and Practice in Technology Enhanced Learning, 12 (22), 1–13. https://doi.org/10.1186/s41039-017-0062-8
PRISMA Statement. (2021). PRISMA endorsers. PRISMA statement website. http://www.prisma-statement.org/Endorsement/PRISMAEndorsers
Qian, Y., Li, C.-X., Zou, X.-G., Feng, X.-B., Xiao, M.-H., & Ding, Y.-Q. (2022). Research on predicting learning achievement in a flipped classroom based on MOOCs by big data analysis. Computer Applied Applications in Engineering Education, 30 , 222–234. https://doi.org/10.1002/cae.22452
Rutner, S. M., & Scott, R. A. (2022). Use of artificial intelligence to grade student discussion boards: An exploratory study. Information Systems Education Journal, 20 (4), 4–18.
Salas-Pilco, S., & Yang, Y. (2022). Artificial Intelligence application in Latin America higher education: A systematic review. International Journal of Educational Technology in Higher Education, 19 (21), 1–20. https://doi.org/10.1186/S41239-022-00326-w
Saldana, J. (2015). The coding manual for qualitative researchers (3rd ed.). Sage.
Shukla, A. K., Janmaijaya, M., Abraham, A., & Muhuri, P. K. (2019). Engineering applications of artificial intelligence: A bibliometric analysis of 30 years (1988–2018). Engineering Applications of Artificial Intelligence, 85 , 517–532. https://doi.org/10.1016/j.engappai.2019.06.010
Strauss, A., & Corbin, J. (1995). Grounded theory methodology: An overview. In N. K. Denzin & Y. S. Lincoln (Eds.), Handbook of qualitative research (pp. 273–285). Sage.
Turing, A. M. (1937). On computable numbers, with an application to the Entscheidungs problem. Proceedings of the London Mathematical Society, 2 (1), 230–265.
Turing, A. M. (1950). Computing machinery and intelligence. Mind, 59 , 443–460.
MathSciNet Google Scholar
Ullah, H., & Hafiz, M. A. (2022). Exploring effective classroom management strategies in secondary schools of Punjab. Journal of the Research Society of Pakistan, 59 (1), 76.
Verdú, E., Regueras, L. M., Gal, E., et al. (2017). Integration of an intelligent tutoring system in a course of computer network design. Educational Technology Research and Development, 65 , 653–677. https://doi.org/10.1007/s11423-016-9503-0
Vygotsky, L. S. (1978). Mind and society: The development of higher psychological processes . Harvard University Press.
Winkler-Schwartz, A., Bissonnette, V., Mirchi, N., Ponnudurai, N., Yilmaz, R., Ledwos, N., Siyar, S., Azarnoush, H., Karlik, B., & Del Maestro, R. F. (2019). Artificial intelligence in medical education: Best practices using machine learning to assess surgical expertise in virtual reality simulation. Journal of Surgical Education, 76 (6), 1681–1690. https://doi.org/10.1016/j.jsurg.2019.05.015
Yang, A. C. M., Chen, I. Y. L., Flanagan, B., & Ogata, H. (2021). Automatic generation of cloze items for repeated testing to improve reading comprehension. Educational Technology & Society, 24 (3), 147–158.
Yao, X. (2022). Design and research of artificial intelligence in multimedia intelligent question answering system and self-test system. Advances in Multimedia . https://doi.org/10.1155/2022/2156111
Zawacki-Richter, O., Marín, V. I., Bond, M., & Gouverneur, F. (2019). Systematic review of research on artificial intelligence applications in higher education—Where are the educators? International Journal of Educational Technology in Higher Education, 16 (1), 1–27. https://doi.org/10.1186/s41239-019-0171-0
Zhang, F. (2022). Design and application of artificial intelligence technology-driven education and teaching system in universities. Computational and Mathematical Methods in Medicine . https://doi.org/10.1155/2022/8503239
Zhang, Z., & Xu, L. (2022). Student engagement with automated feedback on academic writing: A study on Uyghur ethnic minority students in China. Journal of Multilingual and Multicultural Development . https://doi.org/10.1080/01434632.2022.2102175
Download references
Acknowledgements
The authors would like to thank Mildred Jones, Katherina Nako, Yaser Sendi, and Ricardo Randall for data gathering and organization.
Author information
Authors and affiliations.
Department of Teaching and Learning, Old Dominion University, Norfolk, USA
Helen Crompton
ODUGlobal, Norfolk, USA
Diane Burke
RIDIL, ODUGlobal, Norfolk, USA
You can also search for this author in PubMed Google Scholar
Contributions
HC: Conceptualization; Data curation; Project administration; Formal analysis; Methodology; Project administration; original draft; and review & editing. DB: Conceptualization; Data curation; Project administration; Formal analysis; Methodology; Project administration; original draft; and review & editing. Both authors read and approved this manuscript.
Corresponding author
Correspondence to Helen Crompton .
Ethics declarations
Competing interests.
The authors declare that they have no competing interests.
Additional information
Publisher's note.
Springer Nature remains neutral with regard to jurisdictional claims in published maps and institutional affiliations.
Rights and permissions
Open Access This article is licensed under a Creative Commons Attribution 4.0 International License, which permits use, sharing, adaptation, distribution and reproduction in any medium or format, as long as you give appropriate credit to the original author(s) and the source, provide a link to the Creative Commons licence, and indicate if changes were made. The images or other third party material in this article are included in the article's Creative Commons licence, unless indicated otherwise in a credit line to the material. If material is not included in the article's Creative Commons licence and your intended use is not permitted by statutory regulation or exceeds the permitted use, you will need to obtain permission directly from the copyright holder. To view a copy of this licence, visit http://creativecommons.org/licenses/by/4.0/ .
Reprints and permissions
About this article
Cite this article.
Crompton, H., Burke, D. Artificial intelligence in higher education: the state of the field. Int J Educ Technol High Educ 20 , 22 (2023). https://doi.org/10.1186/s41239-023-00392-8
Download citation
Received : 30 January 2023
Accepted : 23 March 2023
Published : 24 April 2023
DOI : https://doi.org/10.1186/s41239-023-00392-8
Share this article
Anyone you share the following link with will be able to read this content:
Sorry, a shareable link is not currently available for this article.
Provided by the Springer Nature SharedIt content-sharing initiative
- Artificial Intelligence
- Systematic review
- Higher education
How AI can accelerate students’ holistic development and make teaching more fulfilling
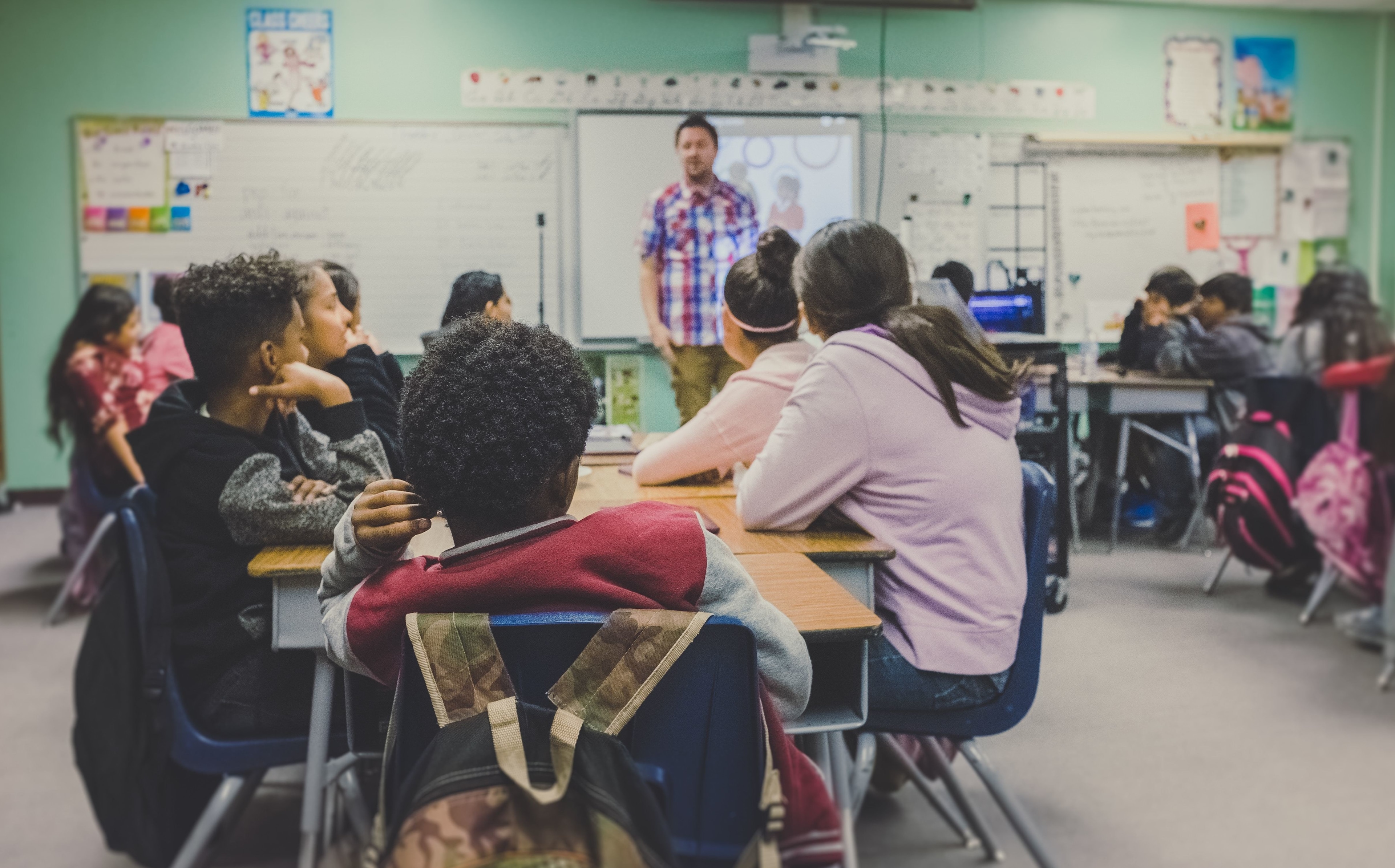
AI can free up time in the classroom. Image: Kenny Eliason on Unsplash
.chakra .wef-1c7l3mo{-webkit-transition:all 0.15s ease-out;transition:all 0.15s ease-out;cursor:pointer;-webkit-text-decoration:none;text-decoration:none;outline:none;color:inherit;}.chakra .wef-1c7l3mo:hover,.chakra .wef-1c7l3mo[data-hover]{-webkit-text-decoration:underline;text-decoration:underline;}.chakra .wef-1c7l3mo:focus,.chakra .wef-1c7l3mo[data-focus]{box-shadow:0 0 0 3px rgba(168,203,251,0.5);} Wendy Kopp
Bo stjerne thomsen.
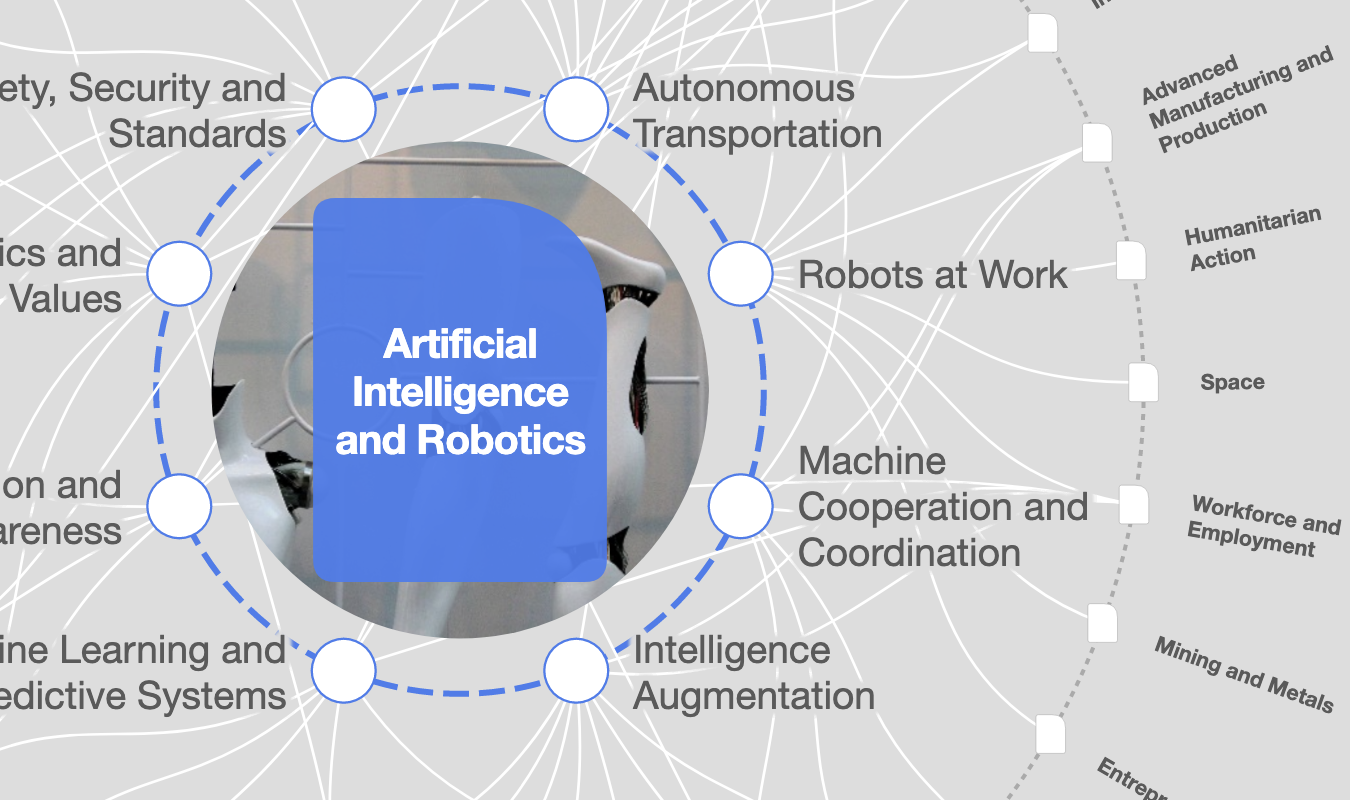
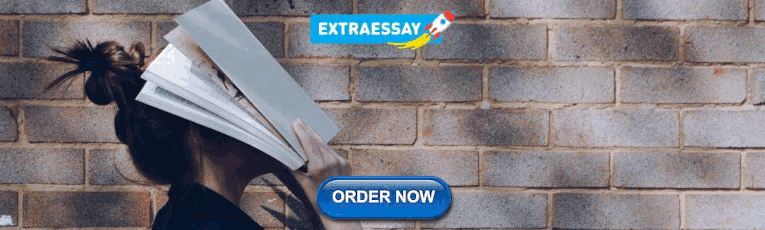
.chakra .wef-9dduvl{margin-top:16px;margin-bottom:16px;line-height:1.388;font-size:1.25rem;}@media screen and (min-width:56.5rem){.chakra .wef-9dduvl{font-size:1.125rem;}} Explore and monitor how .chakra .wef-15eoq1r{margin-top:16px;margin-bottom:16px;line-height:1.388;font-size:1.25rem;color:#F7DB5E;}@media screen and (min-width:56.5rem){.chakra .wef-15eoq1r{font-size:1.125rem;}} Artificial Intelligence is affecting economies, industries and global issues
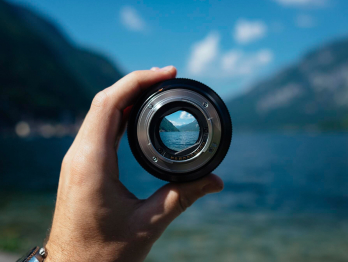
.chakra .wef-1nk5u5d{margin-top:16px;margin-bottom:16px;line-height:1.388;color:#2846F8;font-size:1.25rem;}@media screen and (min-width:56.5rem){.chakra .wef-1nk5u5d{font-size:1.125rem;}} Get involved with our crowdsourced digital platform to deliver impact at scale
Stay up to date:, society and equity.
Listen to the article
- Advances in artificial intelligence (AI) could transform education systems and make them more equitable.
- It can accelerate the long overdue transformation of education systems towards inclusive learning that will prepare young people to thrive and shape a better future.
- At the same time, teachers can use these technologies to enhance their teaching practice and professional experience.
With the rapidly accelerating integration of artificial intelligence (AI) in our work, life, and classrooms, educators all over the world are re-evaluating the purpose of education in light of these outsized implications. At Teach For All and the LEGO Foundation, we see the potential of AI to accelerate the long overdue transformation of education systems towards inclusive learning that will prepare young people to thrive and shape a better future.
At the same time, we see huge opportunities for teachers to use these technologies to enhance their own teaching practice and professional experience.
Have you read?
Our children are growing up with ai. here's what you need to know, chatgpt and cheating: 5 ways to change how students are graded, here's what americans think about generative ai like chatgpt and dall-e, the future of jobs report 2023.
Dialogue on the future of work and education has long emphasized the importance of developing skills and values that are uniquely human and less likely to be replaced by technology. The rise of ChatGPT is yet another proof point. Most students and teachers agree that ChatGPT is “just another example of why we can’t keep doing things the old way for schools in the modern world”. (Although please note that this blog was written in the "old way" as an interactive collaboration between humans, and not generated by AI.)
How AI tools can help educators and students
Our hope is that the advent of AI will spur educators, students, parents, and policy-makers to come together to consider what skills our students really need to navigate uncertainty, solve complex challenges and shape meaningful futures in a changing economy. This means embracing the challenge to provide learning that fosters agency, awareness, critical thinking and problem-solving skills, connectedness, and well-being. We already see that AI tools deployed by well-trained and well-supported teachers can be invaluable for accelerating progress towards this vision.
AI can help foster the skills students will need to navigate and shape the future. Tools like ChatGPT, as Dr. Kathy Hirsh-Pasek and Elias Blinkoff argue , can help promote students’ critical thinking when used in sophisticated ways for deeper, more engaged learning.
Vriti Saraf, CEO and founder of K20 Educators, in New York, agrees: “The less students need educators to be the main source of knowledge, the more educators can focus on developing the ability to curate, guide, critically assess learning, and help students gain skills that are so much more important than memorizing information.”
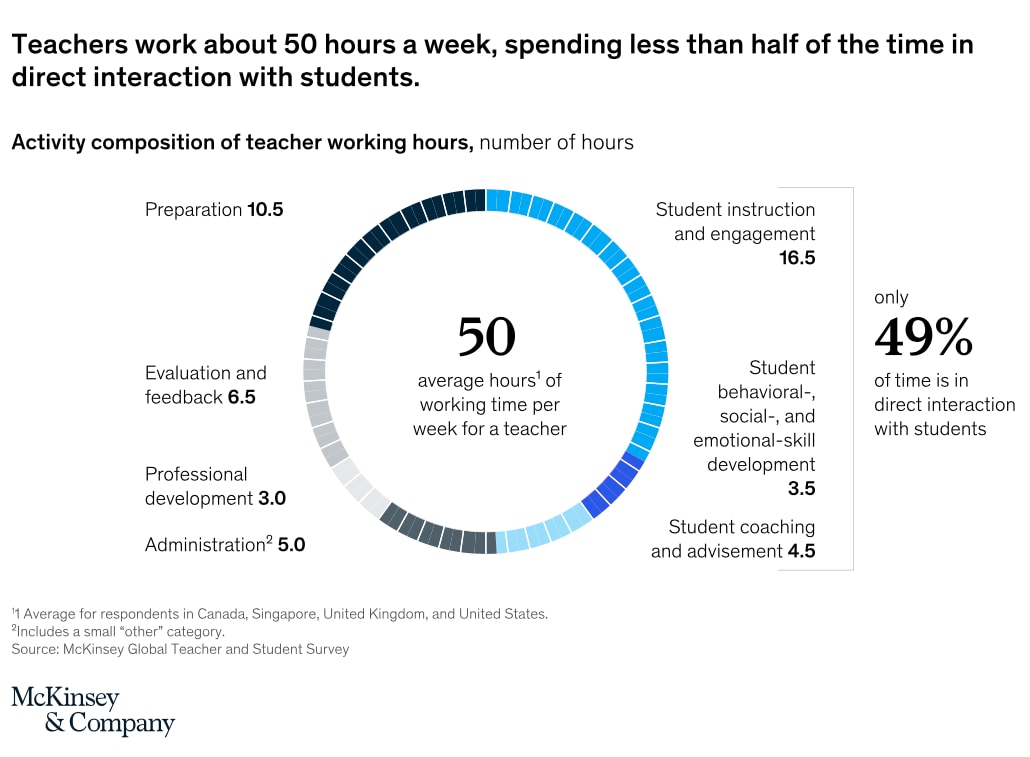
Noting the increased importance of social-emotional learning, Henry May, CEO of Co-School in Bogota, Colombia, notes that teachers have an essential role in shaping childhood experiences away from screens. So, "teachers must be trained on how to educate students on ethical principles; how to use AI tools appropriately; and how to mitigate the potential risk of AI to reduce human connection and belonging and increase loneliness."
Another potential benefit is that AI can free up time in the classroom. Teachers often cite unmanageable administrative tasks as their greatest source of exhaustion and burnout. By automating routine administrative tasks, AI could help streamline teacher workflows, giving them more time to build relationships with students and foster their learning and development.
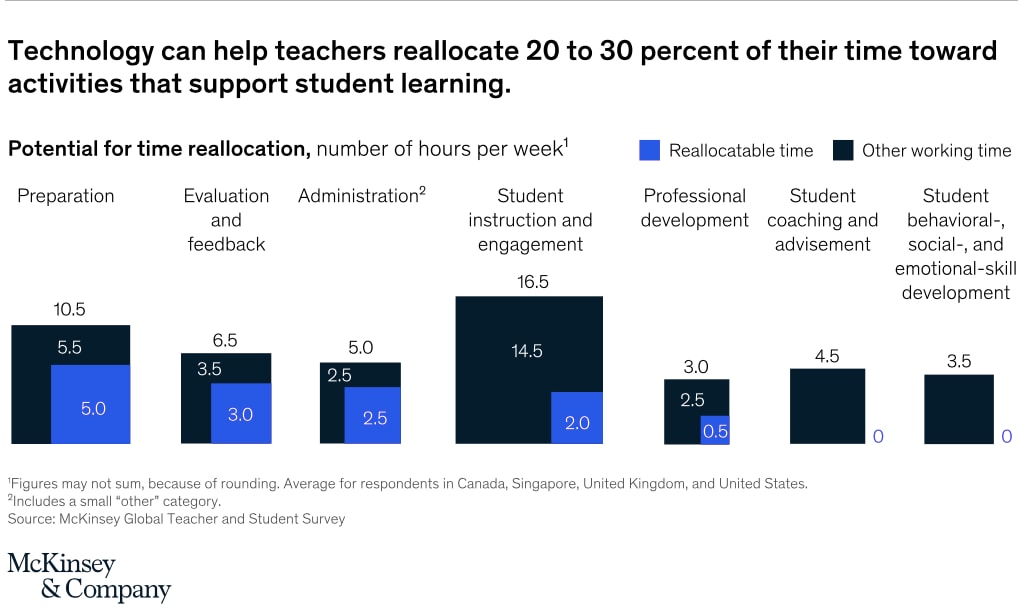
A classroom view of AI tools
Quim Sabría, a former teacher in Barcelona, Spain and co-founder of Edpuzzle, says AI could improve teacher productivity across areas like lesson planning and differentiation, grading and providing quality feedback, teacher-parent communication, and professional development.
In Lagos, Nigeria, teachers are beginning to see the efficiency and ease that AI brings to their work. Oluwaseun Kayode, who taught in Lagos and founded Schoolinka, is currently seeing an increasing number of teachers from across West Africa using AI to identify children’s literacy levels, uncover where students are struggling, and deepen personalized learning experiences.
In the US state of Illinois, a similar pattern is seen where Diego Marin , an 8th-grade math teacher, likens ChatGPT to “a personalized 1:1 tutor that is super valuable for students.”
The Top 10 Emerging Technologies of 2023 report outlined the technologies poised to positively impact society in the next few years, from health technology to AI to sustainable computing.
The World Economic Forum’s Centre for the Fourth Industrial Revolution is driving responsible technology governance, enabling industry transformation, addressing planetary health, and promoting equity and inclusion.
Learn more about our impact:
- Digital inclusion: Our EDISON Alliance is mobilizing leaders from across sectors to accelerate digital inclusion, having positively impacted the lives of 454 million people through the activation of 250 initiatives across 90 countries.
- AI in developing economies: Our Centre for the Fourth Industrial Revolution Rwanda is promoting the adoption of new technologies in the country, enabling over 4,000 daily health consultations using AI.
- Innovative healthcare: Our Medicine from the Sky initiative is using drones to deliver medicine to remote areas in India, completing over 950 successful drone flights.
- AI for agriculture: We are working with the Government of India to scale up agricultural technology in the country, helping more than 7,000 farmers monitor the health of their crops and soil using AI.
Want to know more about our centre’s impact or get involved? Contact us .
Equitable use of AI in education
We see tremendous promise for AI to spur educators around the world to reorient their energy away from routine administrative tasks towards accelerating students’ growth and learning, thus making teaching more fulfilling.
But we need to stay vigilant to ensure that AI is a force for equity and quality. We’ve all heard stories of technology being used to create shortcuts for lesson planning, and we must keep fine-tuning AI so that it does not replicate existing biases.
AI tools can be a catalyst for the transformation of our education systems but only with a commitment to a shared vision for equitable holistic education that gives all children the opportunity to thrive. To ensure AI benefits all students, including the most marginalized, we recommend being mindful of the following principles:
- Co-creation: Bring together ed-tech executives and equity-conscious educators from diverse communities to collaborate on applications of AI that reflect strong pedagogy, address local needs and cultural contexts, and overcome existing biases and inequities.
- Easy entry points: Support teachers to access and apply technologies to reduce administrative burdens and provide more personalized learning by providing open access resources and collaborative spaces to help them integrate AI into their work.
- Digital literacy: Invest in IT fundamentals and AI literacy to mitigate the growing digital divide, ensuring access to devices, bandwidth, and digital literacy development for teachers and students to overcome barriers.
- Best practice: Collect and shareinspiring examples of teachers using technologies to support student voice, curiosity, and agency for more active forms of learning to help inspire other teachers to leverage AI in these ways.
- Innovation and adaptation: Work with school leaders to support teacher professional development and foster a culture of innovation and adaptability. Recognize and reward teachers for new applications of AI and encourage peer-to-peer learning and specialized training.
Don't miss any update on this topic
Create a free account and access your personalized content collection with our latest publications and analyses.
License and Republishing
World Economic Forum articles may be republished in accordance with the Creative Commons Attribution-NonCommercial-NoDerivatives 4.0 International Public License, and in accordance with our Terms of Use.
The views expressed in this article are those of the author alone and not the World Economic Forum.
Related topics:
The agenda .chakra .wef-n7bacu{margin-top:16px;margin-bottom:16px;line-height:1.388;font-weight:400;} weekly.
A weekly update of the most important issues driving the global agenda
.chakra .wef-1dtnjt5{display:-webkit-box;display:-webkit-flex;display:-ms-flexbox;display:flex;-webkit-align-items:center;-webkit-box-align:center;-ms-flex-align:center;align-items:center;-webkit-flex-wrap:wrap;-ms-flex-wrap:wrap;flex-wrap:wrap;} More on Forum Institutional .chakra .wef-nr1rr4{display:-webkit-inline-box;display:-webkit-inline-flex;display:-ms-inline-flexbox;display:inline-flex;white-space:normal;vertical-align:middle;text-transform:uppercase;font-size:0.75rem;border-radius:0.25rem;font-weight:700;-webkit-align-items:center;-webkit-box-align:center;-ms-flex-align:center;align-items:center;line-height:1.2;-webkit-letter-spacing:1.25px;-moz-letter-spacing:1.25px;-ms-letter-spacing:1.25px;letter-spacing:1.25px;background:none;padding:0px;color:#B3B3B3;-webkit-box-decoration-break:clone;box-decoration-break:clone;-webkit-box-decoration-break:clone;}@media screen and (min-width:37.5rem){.chakra .wef-nr1rr4{font-size:0.875rem;}}@media screen and (min-width:56.5rem){.chakra .wef-nr1rr4{font-size:1rem;}} See all

What to expect at the Special Meeting on Global Cooperation, Growth and Energy for Development
Spencer Feingold and Gayle Markovitz
April 19, 2024
From 'Quit-Tok' to proximity bias, here are 11 buzzwords from the world of hybrid work
Kate Whiting
April 17, 2024
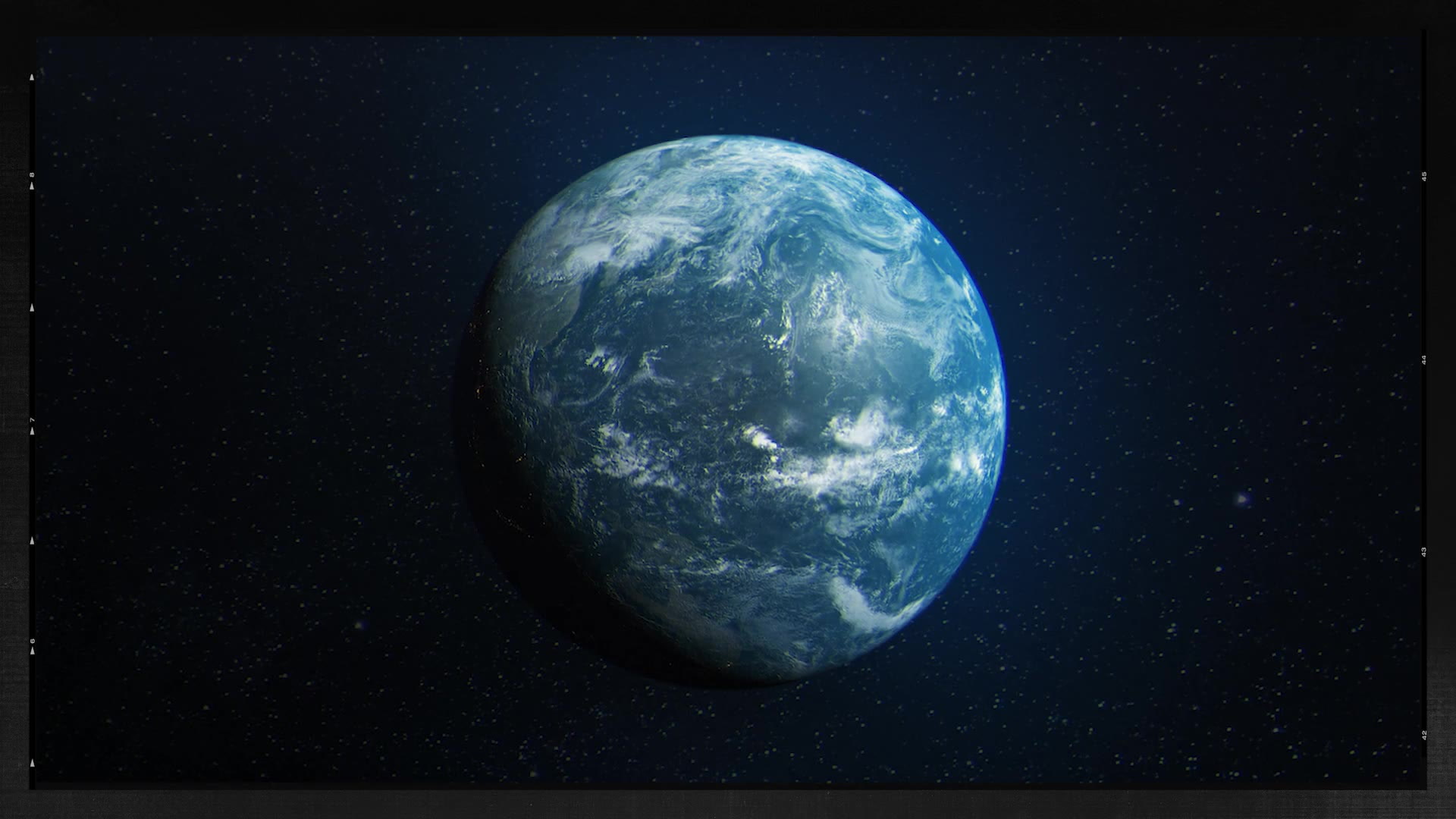
Davos 2024 Opening Film
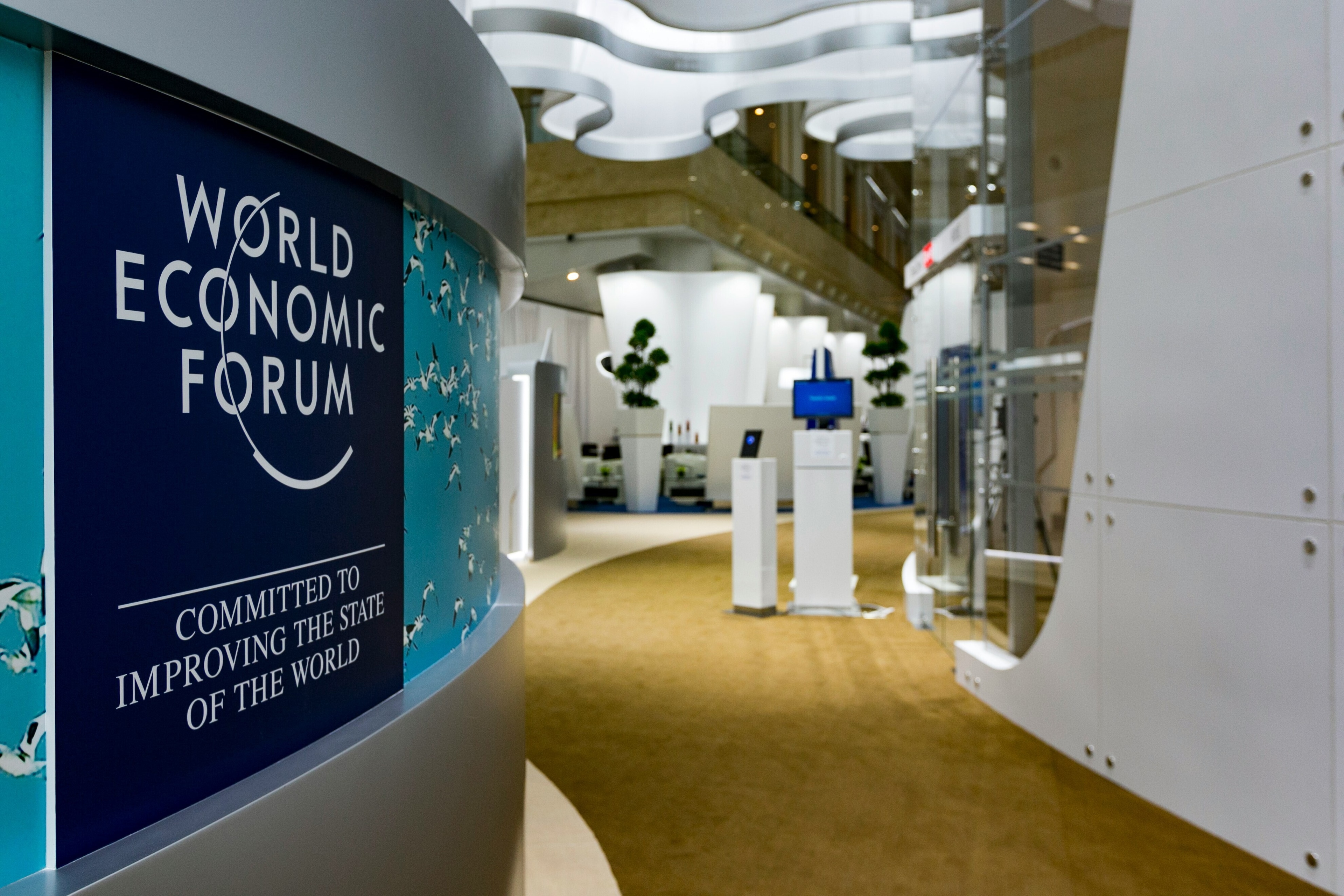
Building trust amid uncertainty – 3 risk experts on the state of the world in 2024
Andrea Willige
March 27, 2024
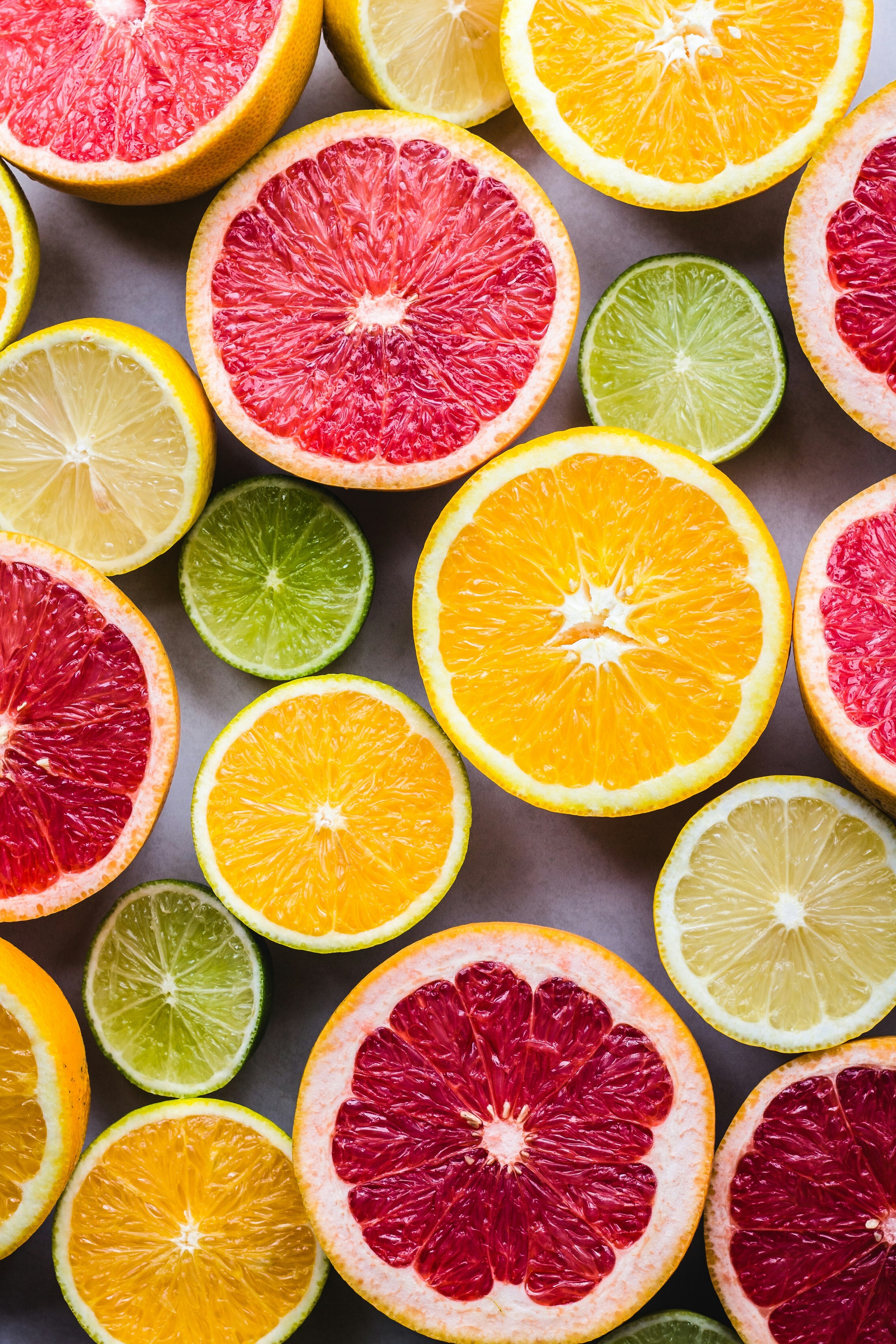
Why obesity is rising and how we can live healthy lives
Shyam Bishen
March 20, 2024
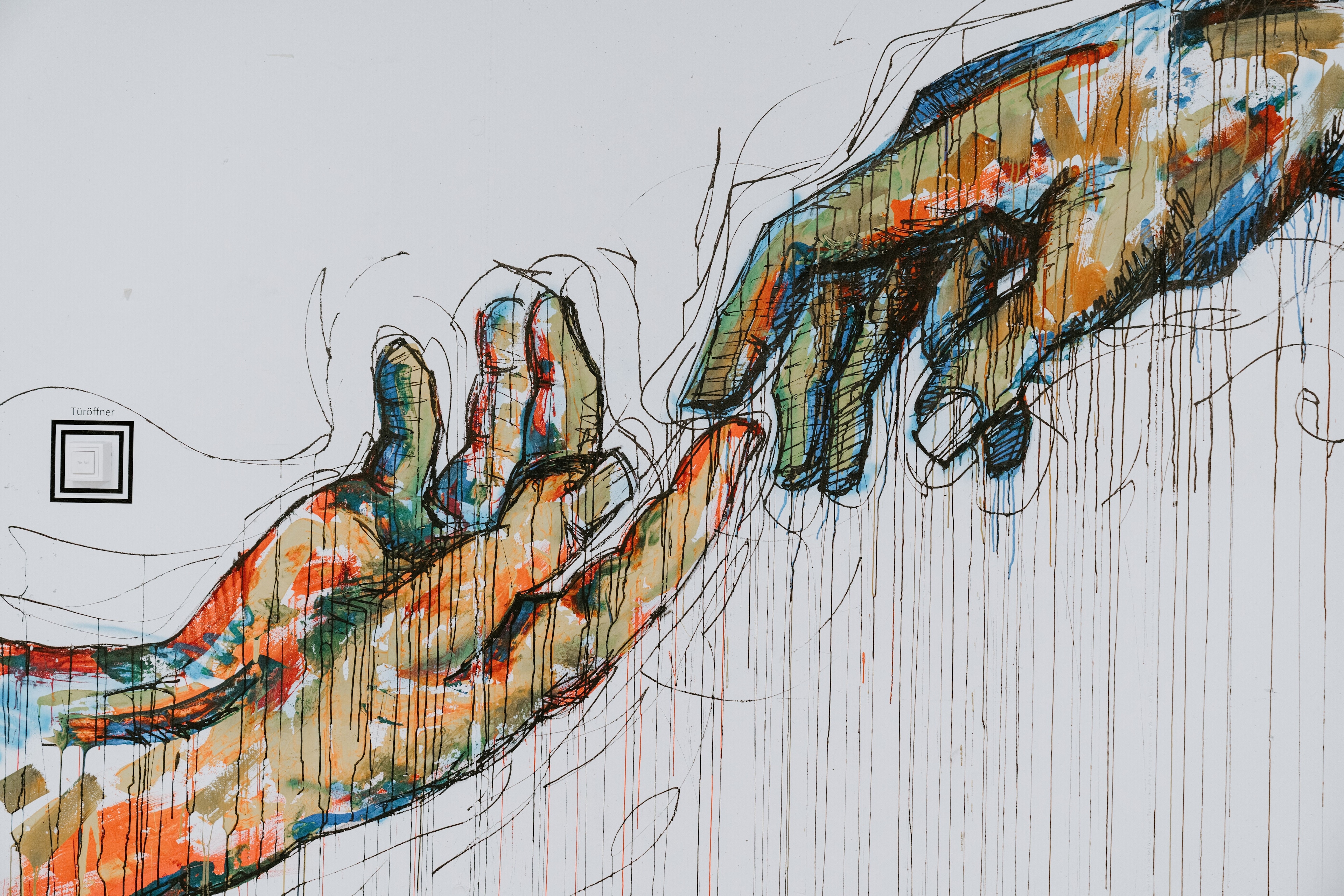
Global cooperation is stalling – but new trade pacts show collaboration is still possible. Here are 6 to know about
Simon Torkington
March 15, 2024
- About the Repository
Towards a tripartite research agenda: A scoping review of artificial intelligence in education research
- Department of Curriculum and Instruction (C&I)
Research output : Chapter in Book/Report/Conference proceeding › Chapters
- Artificial intelligence in education
- Scoping review
This output contributes to the following UN Sustainable Development Goals (SDGs)
Access to Document
- 10.1007/978-981-16-7527-0_1
Other files and links
- Find@EdUHK Library
Fingerprint
- Artificial intelligence Engineering & Materials Science 100%
- Education Engineering & Materials Science 96%
- Blueprints Engineering & Materials Science 21%
- Social sciences Engineering & Materials Science 20%
The present and future of AI
Finale doshi-velez on how ai is shaping our lives and how we can shape ai.
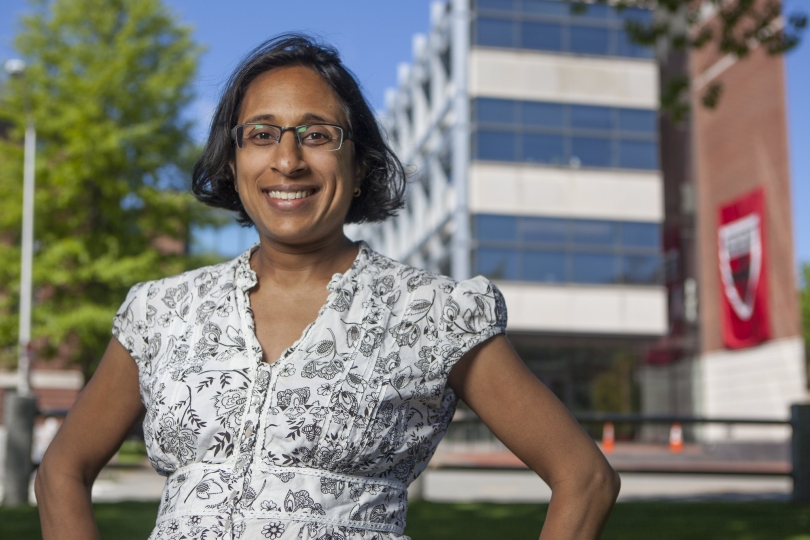
Finale Doshi-Velez, the John L. Loeb Professor of Engineering and Applied Sciences. (Photo courtesy of Eliza Grinnell/Harvard SEAS)
How has artificial intelligence changed and shaped our world over the last five years? How will AI continue to impact our lives in the coming years? Those were the questions addressed in the most recent report from the One Hundred Year Study on Artificial Intelligence (AI100), an ongoing project hosted at Stanford University, that will study the status of AI technology and its impacts on the world over the next 100 years.
The 2021 report is the second in a series that will be released every five years until 2116. Titled “Gathering Strength, Gathering Storms,” the report explores the various ways AI is increasingly touching people’s lives in settings that range from movie recommendations and voice assistants to autonomous driving and automated medical diagnoses .
Barbara Grosz , the Higgins Research Professor of Natural Sciences at the Harvard John A. Paulson School of Engineering and Applied Sciences (SEAS) is a member of the standing committee overseeing the AI100 project and Finale Doshi-Velez , Gordon McKay Professor of Computer Science, is part of the panel of interdisciplinary researchers who wrote this year’s report.
We spoke with Doshi-Velez about the report, what it says about the role AI is currently playing in our lives, and how it will change in the future.
Q: Let's start with a snapshot: What is the current state of AI and its potential?
Doshi-Velez: Some of the biggest changes in the last five years have been how well AIs now perform in large data regimes on specific types of tasks. We've seen [DeepMind’s] AlphaZero become the best Go player entirely through self-play, and everyday uses of AI such as grammar checks and autocomplete, automatic personal photo organization and search, and speech recognition become commonplace for large numbers of people.
In terms of potential, I'm most excited about AIs that might augment and assist people. They can be used to drive insights in drug discovery, help with decision making such as identifying a menu of likely treatment options for patients, and provide basic assistance, such as lane keeping while driving or text-to-speech based on images from a phone for the visually impaired. In many situations, people and AIs have complementary strengths. I think we're getting closer to unlocking the potential of people and AI teams.
There's a much greater recognition that we should not be waiting for AI tools to become mainstream before making sure they are ethical.
Q: Over the course of 100 years, these reports will tell the story of AI and its evolving role in society. Even though there have only been two reports, what's the story so far?
There's actually a lot of change even in five years. The first report is fairly rosy. For example, it mentions how algorithmic risk assessments may mitigate the human biases of judges. The second has a much more mixed view. I think this comes from the fact that as AI tools have come into the mainstream — both in higher stakes and everyday settings — we are appropriately much less willing to tolerate flaws, especially discriminatory ones. There's also been questions of information and disinformation control as people get their news, social media, and entertainment via searches and rankings personalized to them. So, there's a much greater recognition that we should not be waiting for AI tools to become mainstream before making sure they are ethical.
Q: What is the responsibility of institutes of higher education in preparing students and the next generation of computer scientists for the future of AI and its impact on society?
First, I'll say that the need to understand the basics of AI and data science starts much earlier than higher education! Children are being exposed to AIs as soon as they click on videos on YouTube or browse photo albums. They need to understand aspects of AI such as how their actions affect future recommendations.
But for computer science students in college, I think a key thing that future engineers need to realize is when to demand input and how to talk across disciplinary boundaries to get at often difficult-to-quantify notions of safety, equity, fairness, etc. I'm really excited that Harvard has the Embedded EthiCS program to provide some of this education. Of course, this is an addition to standard good engineering practices like building robust models, validating them, and so forth, which is all a bit harder with AI.
I think a key thing that future engineers need to realize is when to demand input and how to talk across disciplinary boundaries to get at often difficult-to-quantify notions of safety, equity, fairness, etc.
Q: Your work focuses on machine learning with applications to healthcare, which is also an area of focus of this report. What is the state of AI in healthcare?
A lot of AI in healthcare has been on the business end, used for optimizing billing, scheduling surgeries, that sort of thing. When it comes to AI for better patient care, which is what we usually think about, there are few legal, regulatory, and financial incentives to do so, and many disincentives. Still, there's been slow but steady integration of AI-based tools, often in the form of risk scoring and alert systems.
In the near future, two applications that I'm really excited about are triage in low-resource settings — having AIs do initial reads of pathology slides, for example, if there are not enough pathologists, or get an initial check of whether a mole looks suspicious — and ways in which AIs can help identify promising treatment options for discussion with a clinician team and patient.
Q: Any predictions for the next report?
I'll be keen to see where currently nascent AI regulation initiatives have gotten to. Accountability is such a difficult question in AI, it's tricky to nurture both innovation and basic protections. Perhaps the most important innovation will be in approaches for AI accountability.
Topics: AI / Machine Learning , Computer Science
Cutting-edge science delivered direct to your inbox.
Join the Harvard SEAS mailing list.
Scientist Profiles
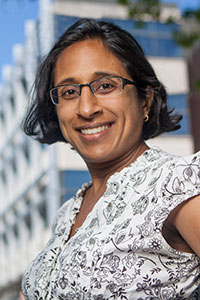
Finale Doshi-Velez
Herchel Smith Professor of Computer Science
Press Contact
Leah Burrows | 617-496-1351 | [email protected]
Related News
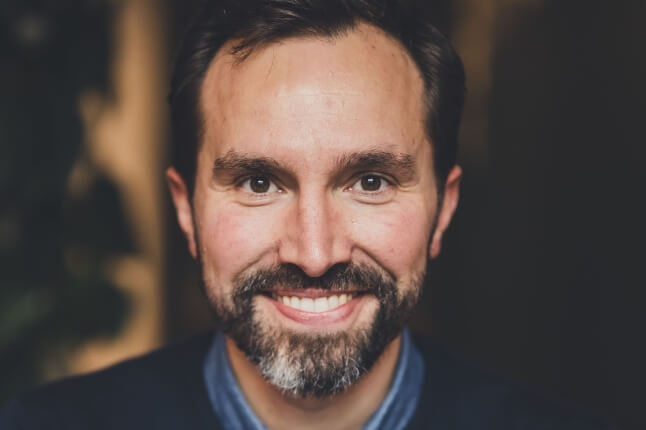
Alumni profile: Jacomo Corbo, Ph.D. '08
Racing into the future of machine learning
AI / Machine Learning , Computer Science
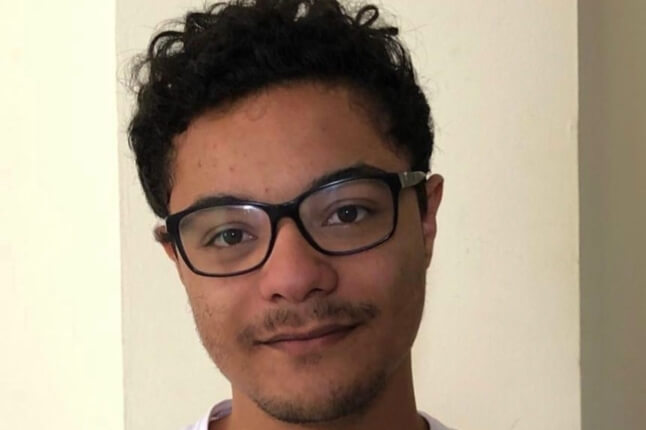
Ph.D. student Monteiro Paes named Apple Scholar in AI/ML
Monteiro Paes studies fairness and arbitrariness in machine learning models
AI / Machine Learning , Applied Mathematics , Awards , Graduate Student Profile
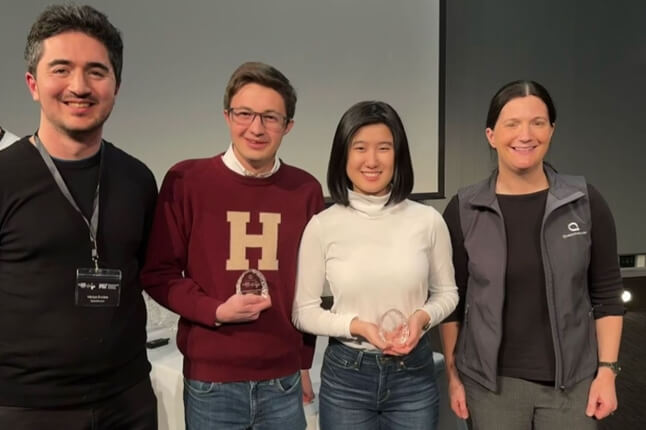
A new phase for Harvard Quantum Computing Club
SEAS students place second at MIT quantum hackathon
Computer Science , Quantum Engineering , Undergraduate Student Profile
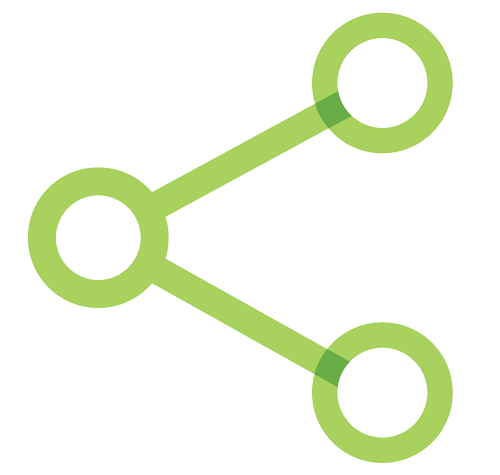
- New! Member Benefit New! Member Benefit
- Featured Analytics Hub
- Resources Resources
- Member Directory
- Networking Communities
- Advertise, Exhibit, Sponsor
- Find or Post Jobs
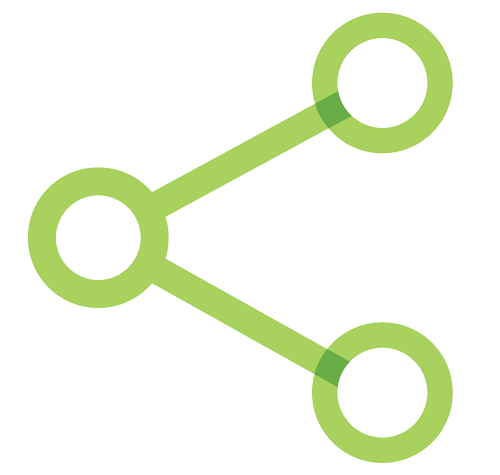
- Learn and Engage Learn and Engage
- Bridge Program
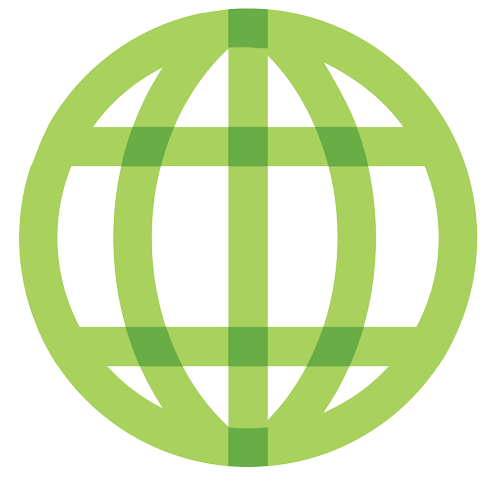
- Compare AACSB-Accredited Schools
- Explore Programs
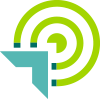
- Advocacy Advocacy
- Featured AACSB Announces 2024 Class of Influential Leaders
- Diversity, Equity, Inclusion, and Belonging
- Influential Leaders
- Innovations That Inspire
- Connect With Us Connect With Us
- Accredited School Search
- Accreditation
- Learning and Events
- Advertise, Sponsor, Exhibit
- Tips and Advice
- Is Business School Right for Me?
Innovative AI Applications for Education

- Avatars fill in for professors when students anywhere in the world have questions at any time of day.
- Simulations and VR experiences help students learn teamwork and leadership skills under challenging conditions.
- An AI-powered career coach analyzes a student’s career ambitions and suggests the courses and training opportunities that will provide the proper preparation.
As companies increasingly integrate artificial intelligence (AI) into their operations, it becomes more essential that business students gain a mastery of emerging technologies. Business schools are responding to the need with a range of initiatives that explore the possibilities and perils of virtual reality (VR), extended reality (XR), ChatGPT, and other digital tools.
Here, we look at six European schools that have reached beyond the constraints of traditional classrooms and created quirky ways to make AI a part of learning. They’re not only delivering content in radical new formats, but helping students perfect the skills they’ll need in tomorrow’s workplace.
The eProf Will Take Your Questions Now
At ESSEC Business School in Cergy, France, associate professor Fabrice Cavarretta wondered if AI could play a role in allowing him to share his knowledge and research. To find out, he came up with an avatar of himself that he named eProf Cavarretta.
He created it by feeding the body of his work—including various documents that ranged from practitioner articles to research papers—to a customized version of ChatGPT. He then instructed ChatGPT to answer questions in ways that are similar to how he explains concepts in his classes.
Now, students anywhere in the world can consult the avatar at any time. Learners currently taking one of his courses don’t need to raise a question in class; they can ask the avatar for clarification. Former students facing new problems or looking to refresh their knowledge can turn to the eProf whenever it’s convenient for them. Instead of querying an algorithm and receiving general answers, they can get Cavarretta’s expert perspective on any of his specific teachings or research methods.
Not only does the avatar have infinite patience, but it also allows for one-on-one interactions. Just as important, its broad scalability means Cavarretta can reach more students via avatar than he ever could on his own.
Learning Journeys on Earth, in Space
The scalability of AI is a major advantage for INSEAD Business School in Fontainebleau, France, which maintains an XR immersive learning library dedicated to management education and research. The portal allows users to access 20 VR experiences covering a range of management topics.
In one of these experiences, learners are virtually transported to a peaceful beach in Zanzibar. Various exotic juice bars dot the white sand that borders the clear turquoise waters. The bars are all close to each other, look the same, and sell similar products—so how do customers choose which bar to buy from?
INSEAD Business School’s extended reality learning library gives learners access to 20 virtual reality experiences covering a range of topics.
Participants meet two juice bar owners to gather details on their different business outlooks and competitive advantages. After completing their consultations, learners offer strategic advice on how to expand the avocado shake business. Through the simulation, students gain insights into sustainable growth, have a chance to devise innovative solutions, and get practice acting as consultants.
In another XR experience, students undertake a mission to Mars, where a new base needs to be built quickly. However, on the way, an accident occurs on the space shuttle, and learners must come up with ideas for how to save both the payload and the lives of the crew members. Working in teams, participants interview members of the crew, assess team dynamics, and recommend the best solutions to Mission Control. The objective of the simulation is for students to learn how to work in teams to generate innovative ideas in challenging environments.
More than 40 faculty members at INSEAD are using XR in their teaching, employing it in their research, or developing VR experiences. These experiences are available to other institutions around the world to use their own classrooms.
Critical Mission, Essential Learning
Similarly, ESMT Berlin is relying on an immersive VR simulation to drop participants into an imaginative yet perilous metaverse landscape.
“The simulation is centered around a critical mission where teams are plunged into an unstable setting threatened by environmental degradation,” says Mandy Hübener, director of executive education. “The simulation divides participants into two roles: One group uses VR to construct a bridge across hazardous terrains, embodying a hands-on, collaborative effort. Simultaneously, people in a second group located in a separate room act as strategic planners. This group, connected via audio, observes the VR team’s efforts and provides guidance, utilizing additional information to navigate challenges and allocate resources effectively.”
Through the exercise, participants work within a custom metaverse space to enhance their skills in hybrid teamwork, strategic thinking, and adaptability. As they seek solutions in a simulated high-stakes situation, they prepare to handle the complexities of real-world challenges.
AI is also a component in ESMT Berlin’s executive programs. A new challenge developed by Carolin Puppel, program director of executive education, teaches students how to use generative AI to complement human decision-making.
In ESMT Berlin’s virtual reality simulations, students build skills in teamwork, strategic thinking, and adaptability, which prepares them to handle real-world challenges.
Participants play the role of the chief quality officer at AquaPure. The company, which specializes in flavored bottled water, is facing a crisis because some products potentially have been contaminated. Using lab reports, customer feedback, and sales data, participants must determine how severe the contamination is and what actions they should take. These range from instituting a full product recall to staging customer service interventions. At the same time, participants must shape the appropriate communications.
Taking on tasks such as writing prompts and checking facts, team members learn to use AI tools such as ChatGPT as they analyze complex data, validate their findings, generate solutions, and make decisions. Because they are engaging with the tools on practical tasks, participants learn how to create effective prompts, address the limitations of AI, confirm accuracy, and understand the real dynamics at play when humans collaborate with technology.
Virtual, Immersive, and Personalized
Technology is a growing component of educational delivery at NEOMA Business School, which has locations in Reims, Rouen, and Paris, France. In 2020, the school opened a virtual campus , an online space that allows students to join seminars and meetings or even play football on the digital pitch.
The school also has brought VR cases into its classrooms. For instance, NEOMA partnered with ENEDIS, a major electricity grid operator in France, to create an immersive case study experience for its Global Executive MBA.
In addition, the school uses AI-powered adaptive learning processes to sort students into slower or faster learners. This allows faculty to adapt the type and quantity of exercises they provide in the classroom while putting more focus on areas that are more challenging. Taking these insights one step further, professors can turn fast learners into mentors who help other students and thus contribute to the success of the entire class.
Alain Goudey, professor of marketing and associate dean for digital at NEOMA, has written about how tools such as ChatGPT can improve education by providing students with personalized assessments and high-quality feedback, thus freeing up some of the instructor’s time and attention. He says, “The time saved means there’s more human interaction, which is beneficial for teachers and students alike.”
The Career Coach With All the Answers
AI can be useful outside of the classroom, too, as POLIMI Graduate School of Management in Milan demonstrates with its career coach, FLEXA . The tool was created through a partnership with Microsoft.
POLIMI’s AI career coach analyzes a student’s career ambitions, proposes training opportunities, and suggests which skills might need improvement.
Acting as a digital mentor, the AI application analyzes a student’s career ambitions and proposes the best training opportunities. It even allows participants to go through an assessment phase to evaluate the skills they need to improve before they embark on their personalized learning paths.
To create programs tailored to unique individuals, FLEXA sorts through about 800,000 pieces of learning material, from digital courses and webinars to podcasts, articles, and case studies. Because it maintains profiles of interested professionals, FLEXA also can serve as a digital recruiting platform for corporations looking to hire new talent.
An IDEA for the Future Classroom
As these examples show, today’s business schools are highly focused on answering a critical question: How will technology shape the future of learning?
It’s a question that’s getting particular attention at Imperial College Business School’s Innovation, Digital Education, and Analytics Lab ( IDEA Lab ) in London. In one initiative, which considers how AI can be integrated into learning, faculty are using course materials and personas to create AI avatars and simulations. As students converse with the avatars to deepen their understanding of specific topics, AI turns passive content consumption into interactive dialogues.
Through rapid advancements in AI and XR, society is witnessing a unique convergence of digital and physical worlds and unlocking an entirely new set of tools to build solutions, says the IDEA Lab’s executive director Monica Arés. This rare moment in the trajectory of education, she adds, will increase student engagement and retention by enabling personalized learning at scale.
As other schools come up with their own ways of integrating AI into the classroom, they will create more fun, intriguing, and innovative learning experiences. Sandy beaches and space shuttles are just the beginning—who knows where business education might go next?
- artificial intelligence
- experiential learning
- future of business education
- learner engagement
- online learning
- virtual reality
Smart learning: AI resources every educator should know
April 18, 2024.
By Microsoft Education Team
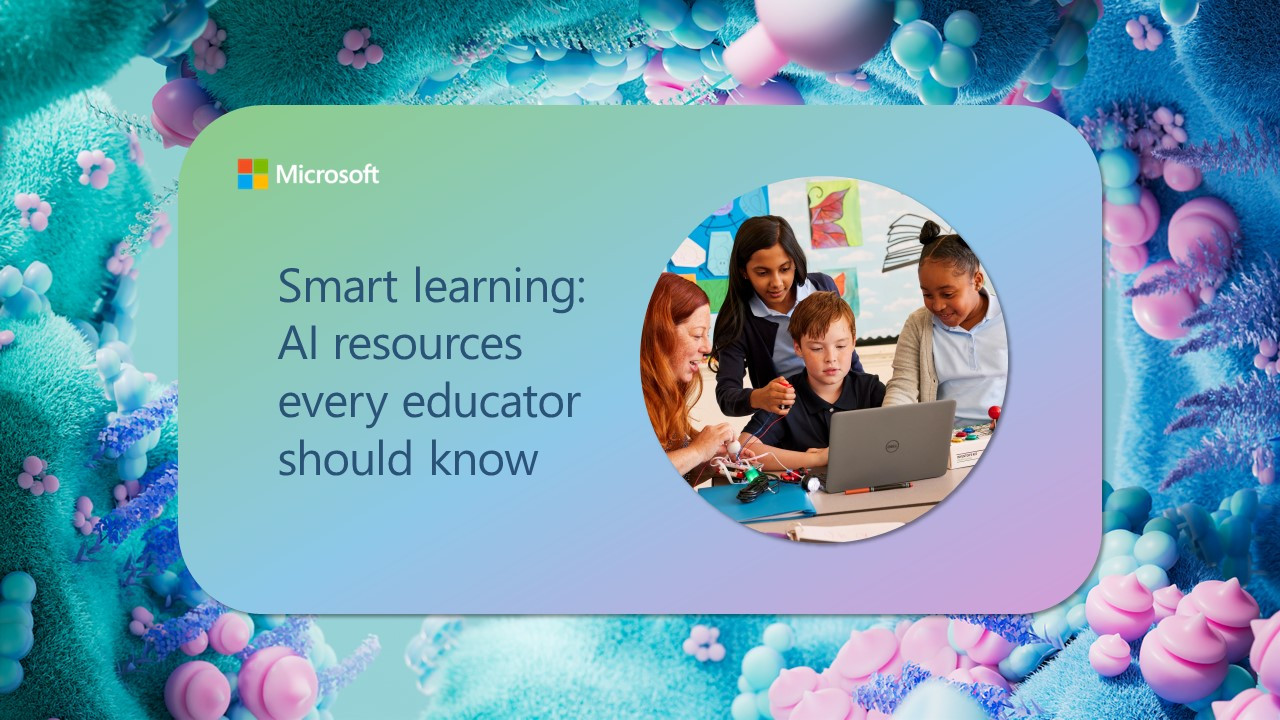
Share this article
On April 19 in the United States, we celebrate National AI Literacy Day , a nationwide initiative aimed at fostering understanding and engagement with AI. With developments in AI happening so quickly and new products and features launching all the time, it can be difficult to keep up. We want to equip you with the knowledge needed to navigate the world of AI. We’ve gathered some resources, activities, and training to help you get up to speed on all things AI, and how it can be useful in education. In addition to the items below, you’ll find a collection of AI for education resources and learning opportunities on the Microsoft Learn Educator Center .
AI literacy is crucial in today's digital age, where AI technologies are increasingly integrated into our daily lives. Our latest insights from the AI in Education: Microsoft Special Report demonstrate a disconnect—use of AI in education is outpacing the understanding of the technology. There’s an urgent need to increase AI literacy and AI integration in strategic priorities, standards, policies, and professional development.
For educators, it's about preparing your students for a future where AI will be a fundamental part of many professions. It’s also about guiding learners to use AI tools safely and responsibly, ensuring they understand the implications of AI on privacy and data security. By fostering AI literacy, we can shape a future where everyone can navigate and benefit from AI advancements confidently and responsibly. Whether you’re a teacher, parent/guardian, or curious learner, here are some valuable resources to enhance your AI literacy from Microsoft Education .
11 resources for educators to amp up your AI literacy
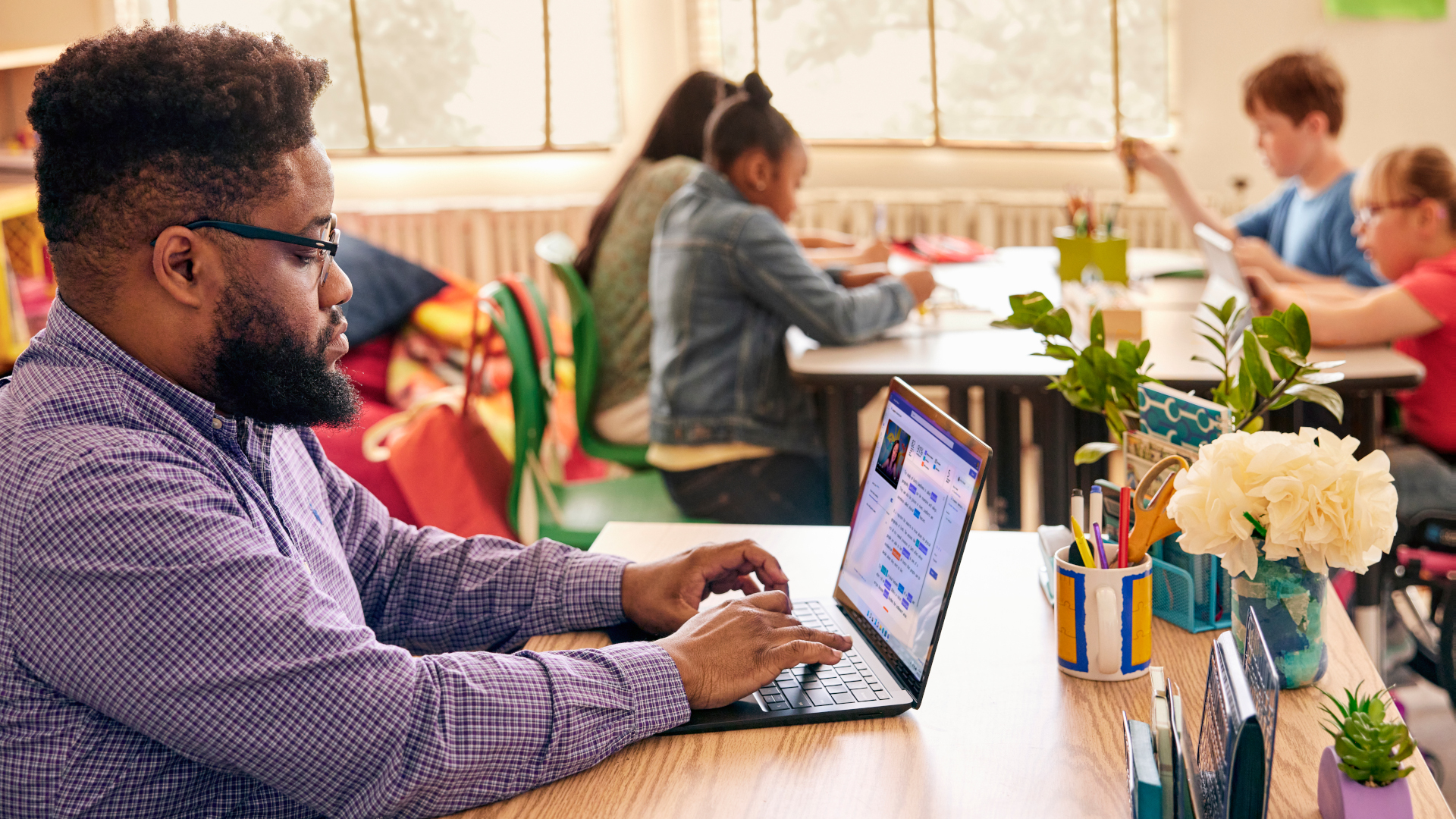
For National AI Literacy Day 2024, explore the AI in education professional development opportunities available from Microsoft.
AI in education professional development
- AI for Educators training : Spend a few hours on your professional development and learn about the history of AI, large language models (LLMs), generative AI, how to create a prompt, and uses of AI in education. The AI for Educators Learning Path on Microsoft Learn is made up of three modules: “Empower educators to explore the potential of artificial intelligence,” “Enhance teaching and learning with Microsoft Copilot,” and “Equip and support learners with AI tools from Microsoft.” From now until April 30, 2024, participate in the AI skills challenge for educators and benchmark your progress against fellow educators and friends.
- Flip AI for educators series : Flip offers free professional development training sessions that provide easy-to-follow instructions, best practices, and inspiration on various topics, including AI! You can catch up on the great AI for educators series events that have already happened, and be sure to register for upcoming professional development events as well.
- Microsoft Education AI Toolkit : The Microsoft Education AI Toolkit provides education leaders with relevant background knowledge, strategies, and recommendations for launching AI initiatives in K-20 settings. It also includes customer stories and technical profiles that showcase how institutions around the globe are already using AI for teaching, learning, and administration. What’s inside of the toolkit provides those in charge with the necessary information that they need to jumpstart their own AI journey. Learn more about the Microsoft Education AI Toolkit and how to use this resource in this article: Kickstart your school’s AI journey with the Microsoft Education AI Toolkit .
Get started using Microsoft Copilot in education
- Introduction to Microsoft Copilot : Learn all about Microsoft Copilot, your AI-powered assistant for education. Get an overview of how to use Copilot, as well as ideas and inspiration for how you can use Copilot to save time, differentiate instruction, and enhance student learning. You can save or print this quick guide to Microsoft Copilot to refer back to as needed.
- Copilot resources for education : Dive deeper into what Copilot can do with resources for education. Whether you’re an educator, IT professional, or parent/guardian, you’ll find helpful resources to get started using Copilot.
- Copilot lab : While it’s not specific to education, the Copilot lab is a great resource to help you learn more about Copilot, how to write a prompt, and ideas of how to get started using Copilot.
Improve your students’ AI literacy, too!
By fostering AI literacy, together we can shape a future where everyone can navigate and benefit from AI advancements.
- Classroom toolkit: Unlocking generative AI safely and responsibly is a creative resource that blends engaging narrative stories with instructional information to create an immersive and effective learning experience for educators and students aged 13-15 years. The toolkit is designed to assist educators in initiating important conversations about responsible AI practices in the classroom, such as the critical topics of content fabrications, privacy considerations, bias awareness, and mental wellbeing.
- Minecraft AI Prompt Lab : Embracing the ever-changing world of education calls for innovation and tech-savvy teaching methods. The Minecraft AI Prompt Lab is a new series of resources that demonstrates how to use Microsoft Copilot with Minecraft Education to design amazing learning experiences. Crafted for educators like you, this game-changing guide is here to revolutionize the way you deliver educational content with Minecraft. In Prompt Lab: Module 1 , learn how to write prompts, develop learning content and assessments, and generate creative ideas for lesson plans will help you unlock the power of game-based learning with Minecraft Education. In Prompt Lab: Module 2 , learn the basics of Code Builder, the in-game coding feature of Minecraft Education.
- Minecraft Hour of Code: Generation AI : All students deserve opportunities to explore AI technology to understand its implications, access career pathways, and be empowered to safely, confidently navigate an AI-powered world. Designed for anyone ages 7 and up, Minecraft Hour of Code: Generation AI is a fun, accessible way to explore the fundamentals of coding and responsible AI. Students will venture through time to create helpful AI-powered inventions to solve problems and make daily life easier. Learn coding basics and essential principles of computer science, all while encouraging thoughtful discussions around responsible coding and AI development. With free downloadable educator resources exploring the amazing potential of AI has never been more exiting or immersive!
Online safety and information literacy are the foundation of AI literacy
- Microsoft Family Safety Toolkit : To help young people, educators, and families navigate the digital world, Microsoft has also released an online safety resource, the Microsoft Family Safety Toolkit . This toolkit provides guidance on how to leverage Microsoft’s safety features and family safety settings to support and enhance digital parenting, plus guidance for families looking to navigate the world of generative AI together. Bonus resource for young children: PBS Kids launched an educational series on AI supported by Microsoft.
- Search Progress and Coach : Empowering learners to seek, evaluate, and use online sources responsibly is a critical step in helping them to navigate AI-generated content and the wider information ecosystem with confidence. This short course on our newest Learning Accelerators, Search Progress and Search Coach , showcases how educators can help foster information literacy skills through any research-based assignment in Microsoft Teams for Education.
Let’s celebrate knowledge, curiosity, and the transformative power of AI. Join us this National AI Literacy Day to explore these resources and take a step towards a more informed and inclusive future with AI. Whether you're an educator looking to bring AI into the classroom or a parent guiding your child in the digital world, these resources will equip you with the knowledge to embrace AI's potential responsibly. Let's celebrate the day by committing to lifelong learning and curiosity in the ever-evolving field of AI.
Related stories
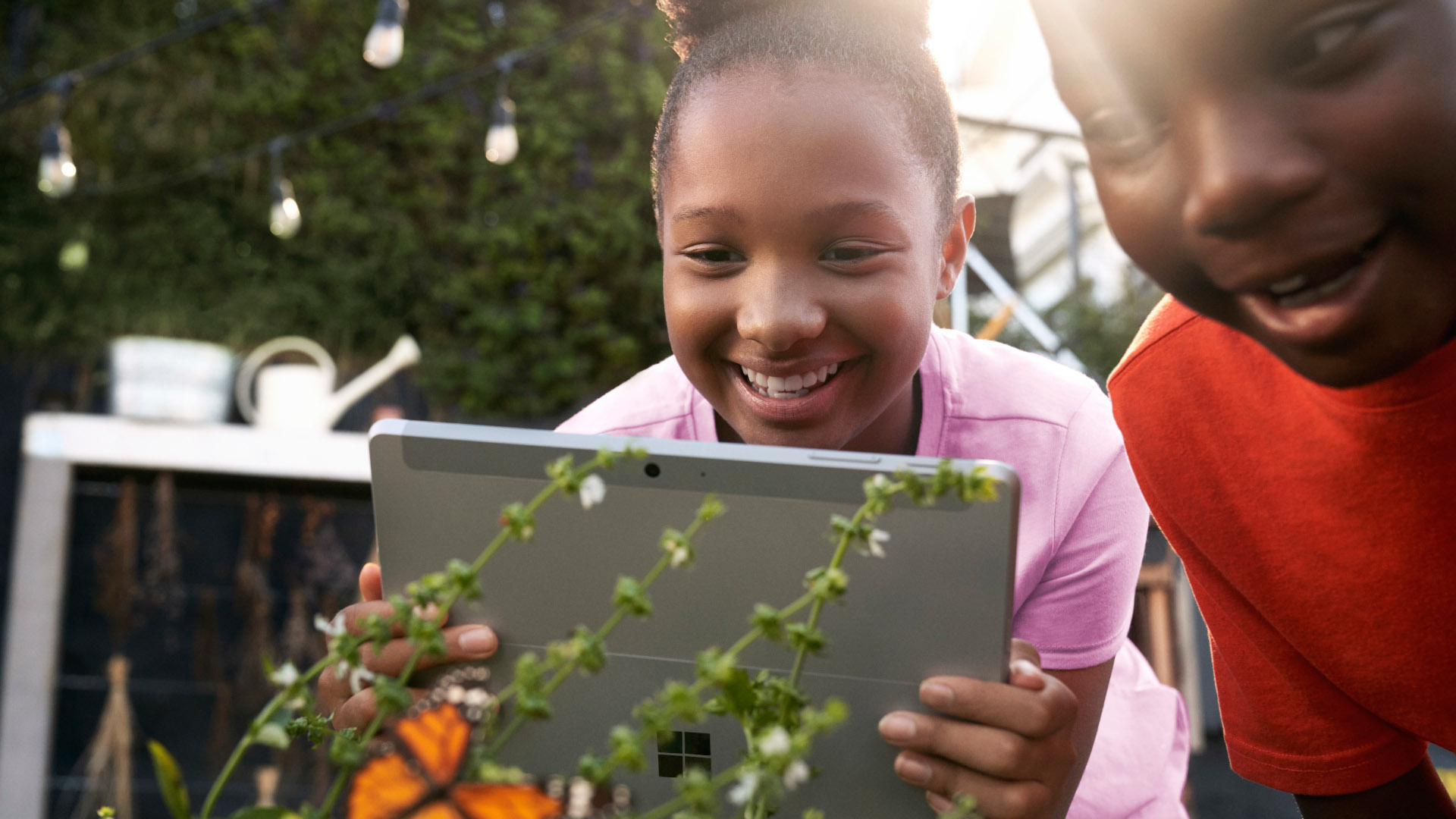
How to celebrate Earth Day 2024 with your students
Spark your students' curiosity with Earth Day activities and more from Microsoft. From video games to projects, try these fun Earth Day activities for your class.
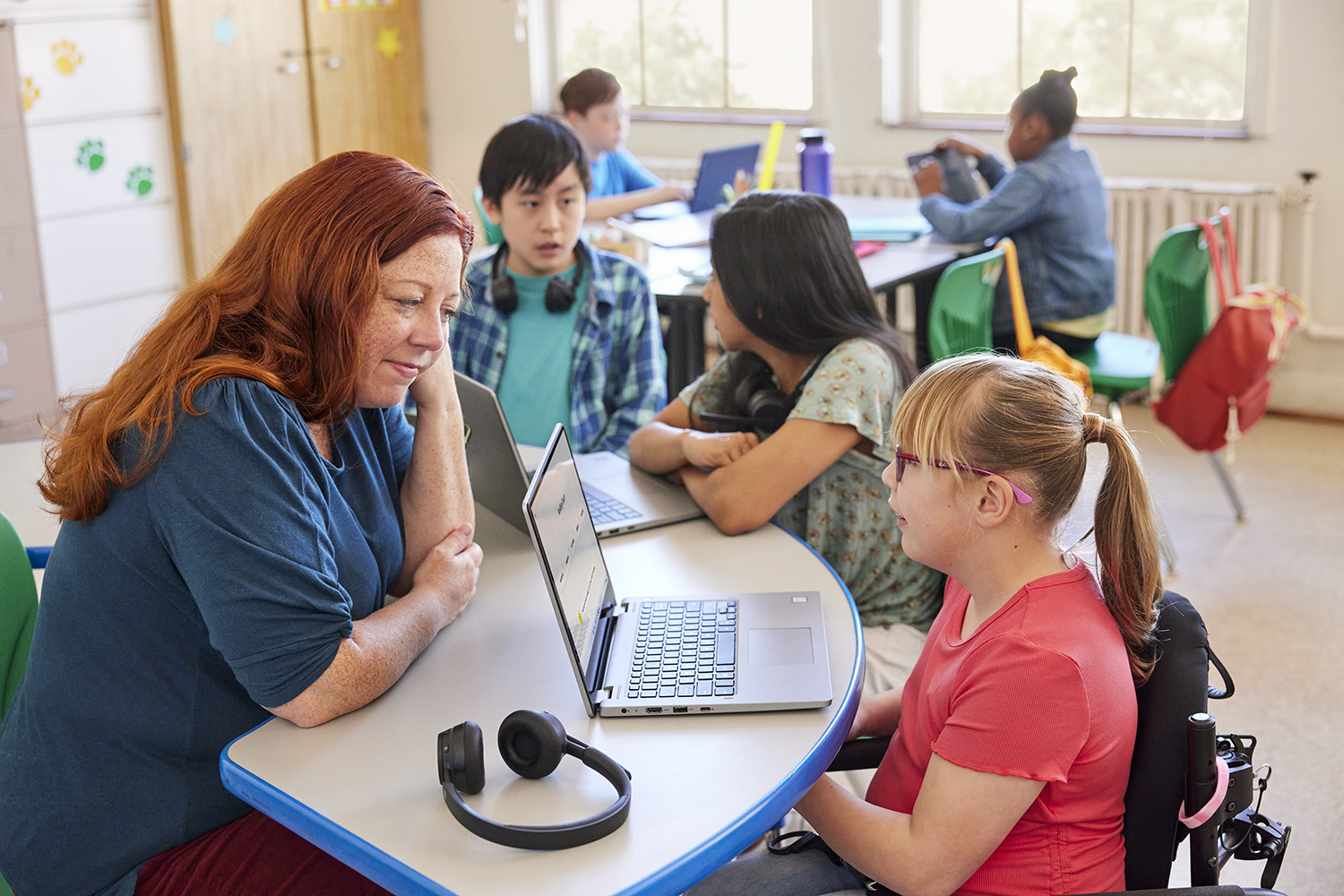
Stay ahead with 8 new updates from Microsoft Education
We recognize that teachers often look for new and effective ways to engage their students and support their learning goals. That's why we're constantly working to improve Microsoft Education solutions, features, and training, so you can have access to powerful classroom tools for teaching.
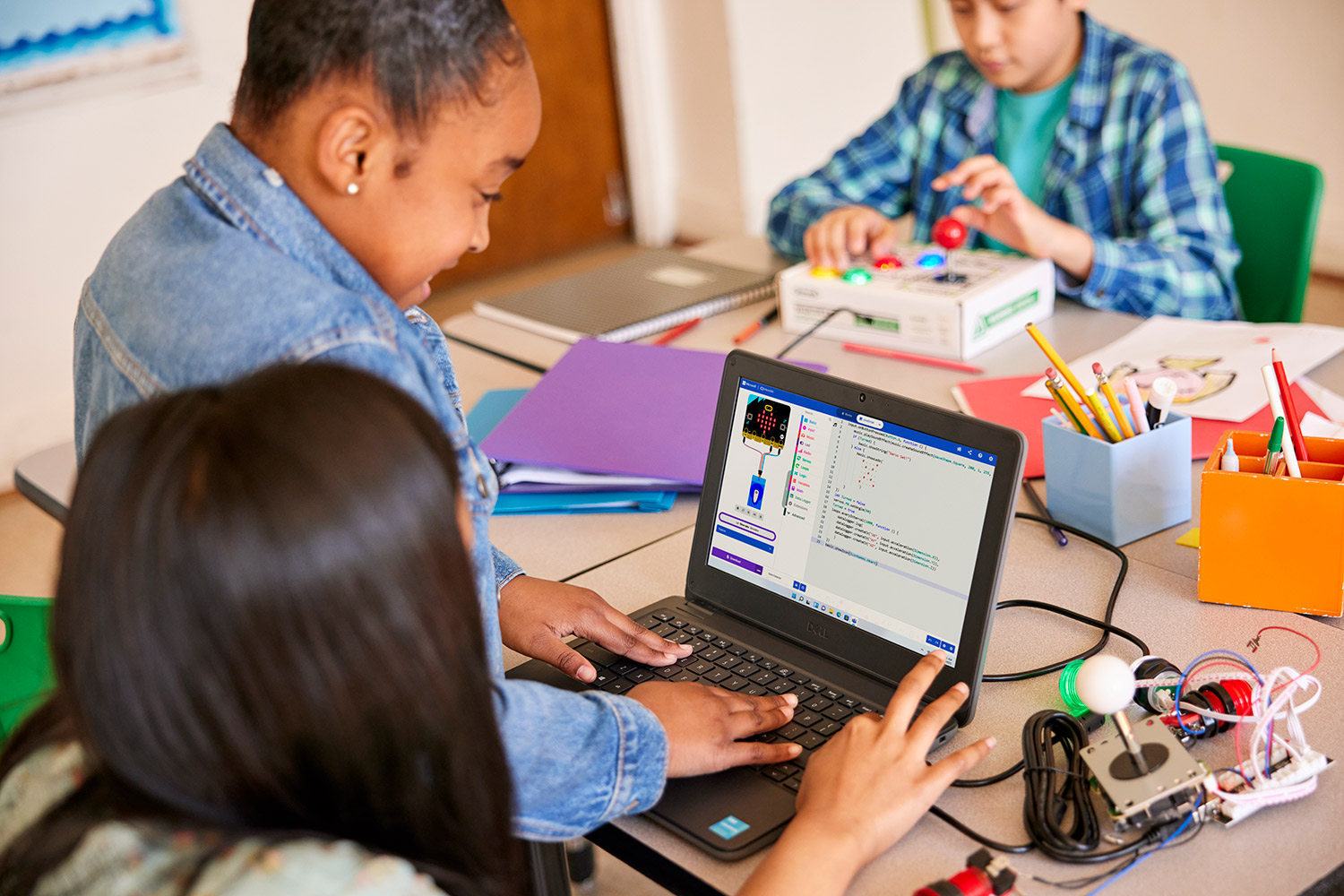
Gearing up for Computer Science Education Week
It’s the most wonderful time of the year: Computer Science Education Week! All year round, but especially December 4–10, 2023, we aim to inspire students to engage in computer science with opportunities to learn about AI, advocate for equity in digital careers, and become responsible coders.
- SCHOOL STORIES
- MICROSOFT EDUCATOR CENTER
- CONTACT SALES

Reading List: Machine Learning and Artificial Intelligence in Education – A Critical Perspective
Edited by: Pati Ruiz, Aditi Mallavarapu, and Arun Balajiee
The following reading list was compiled to provide an overview of the current state of Machine Learning (ML) and Artificial Intelligence (AI) in education, also referred to as AI in Education Research (AIED). Some articles are focused broadly on research themes in AI/ML as they are applied for teaching and learning scenarios, while others more specifically on the intersection of equity, bias, ethics, of using ML/AI methods and the mechanics of the methods themselves. An additional reading section identifies relevant news articles, reports, and a podcast on these topics.
Included under each article is a brief bulleted overview of what each article covers and how much technical background each article requires.
Last Updated: 9/19/2022
Algorithmic Bias in Education Citation: Baker, R. S., & Hawn, A. (2021). Algorithmic Bias in Education. International Journal of Artificial Intelligence in Education. https://doi.org/10.1007/s40593-021-00285-9 Background Needed: Low/Medium Covers:
- This review focuses on solidifying the current understanding of the concrete impacts of algorithmic bias in education.
- The authors discuss theoretical and formal perspectives on algorithmic bias and review the evidence around algorithmic bias in education.
- Finally, the authors propose a framework for moving from unknown bias to known bias and from fairness to equity and discuss obstacles to addressing these challenges and propose four areas for mitigating and resolving the problems of algorithmic bias in AIED systems and other educational technology.
Academic Articles
Culture in Computer-Based Learning Systems: Challenges and Opportunities Citation: Baker, R. S., Walker, E., Ogan, A., & Madaio, M. (2020). Culture in Computer-Based Learning Systems: Challenges and Opportunities. https://doi.org/10.5281/zenodo.4057223 Background Needed: Medium Covers:
- The paper reviews various learning theories related to culture that can be used to predict learning behavior.
- Identifies that it is not necessarily true that learners from collectivistic cultures would participate in group collaborative behavior since some results also indicate that learners from individualistic cultures also participate well in group discussions.
- Compares and contrasts Hofstede’s model of culture with a simpler Inglehart-Welzel model as a trade-off between simplicity and degree of validation of individual values/individualism. Also, discusses Culture Based Learning (while there is no set definition, the paper cites literature that discusses Culture Based Learning).
- The paper authors also acknowledge their own cultural background in the context of this discussion and that their perspectives may or may not be biased because of this background.
Algorithmic Fairness in Education Citation: Kizilcec, R. F. & Lee, H. (2022). Algorithmic Fairness in Education. In W. Holmes & K. Porayska-Pomsta (Eds.), Ethics in Artificial Intelligence in Education, Taylor & Francis. https://arxiv.org/abs/2007.05443 Background Needed: Medium Covers:
- Draws parallels to prior literature on educational access, bias, and discrimination.
- Examines core components of algorithmic systems (measurement, model learning, and action) to identify sources of bias and discrimination in the process of developing and deploying these systems.
- Provides recommendations for policy makers and developers of educational technology offer guidance for how to promote algorithmic fairness in education.
Unwritten Magic: Participatory Design of AI Dialogue to Empower Marginalized Voices Citation: Buddemeyer A., Nwogu J., Solyst J., Walker E., Nkrumah T., Ogan A., Hatley L., and Stewart A (2022). Unwritten Magic: Participatory Design of AI Dialogue to Empower Marginalized Voices. https://doi.org/10.1145/3524458.3547119 Background Needed: Medium Covers:
- Participatory Design of dialogue systems with students of color in middle school.
- One-week Youth Advisory Group (YAG) at a middle school in Washington D.C., to learn programming in Scratch.
- Understand the linguistic features needed to develop a cultural-sensitive dialogue system.
Towards a Tripartite Research Agenda: A Scoping Review of Artificial Intelligence in Education Research Citation: Wan, T., & Cheng, E. C. K. (2022). Towards a Tripartite Research Agenda: A Scoping Review of Artificial Intelligence in Education Research . In E. C. K. Cheng, R. B. Koul, T. Wang, & X. Yu (Eds.), Artificial Intelligence in Education: Emerging Technologies, Models and Applications (pp. 3–24). Springer Singapore. Background Needed: Low/Medium Covers:
- This paper reviews research studies on artificial intelligence in education (AIED) published from 2001 to 2021
- Learning from AI
- Learning about AI, and
- Learning with AI
On the genealogy of machine learning datasets: A critical history of ImageNet Citation: Denton, E., Hanna, A., Amironesei, R., Smart, A., & Nicole, H. (2021). On the genealogy of machine learning datasets: A critical history of ImageNet. Big Data & Society. https://doi.org/10.1177/20539517211035955 Background Needed: Medium Covers:
- This paper conceptualizes machine learning datasets as a type of informational infrastructure in response to growing concerns of bias, discrimination, and unfairness perpetuated by algorithmic systems.
- The paper examines the norms, values, and assumptions embedded in machine learning datasets and examines the histories and modes of constitution at play in their creation.
- The researchers trace the discourses around large computer vision datasets and contribute to the development of the standards and norms around data development in machine learning and artificial intelligence research.
Evolution and Revolution in Artificial Intelligence in Education Citation: Roll, I., & Wylie, R. (2016). Evolution and Revolution in Artificial Intelligence in Education. International Journal of Artificial Intelligence in Education, 26(2), 582–599. Evolution and Revolution in Artificial Intelligence in Education | SpringerLink Background Needed: None/Low Covers:
- The authors take a historical perspective to identify the past foci that occupy the field of AIED. They consider 47 papers from three crucial years (early, middle, and recent years of AIED) in the history of the Journal of AIED (1994, 2004, and 2014).
- The historical perspective considers focus of research (e.g., modeling, system review, evaluation), domain (e.g., STEM, language learning), types of problems (e.g. sequential problems, complex problems), collaboration structures (number of learners and machines), technology (e.g., computers, hand-helds), settings (e.g. school, workplace) and learning goals of each of the works.
- They map this historical trajectory to current developments along the three dimensions of (1) Goals, (2) Practices and (3) Environments in AI research for education.
A Review of Artificial Intelligence (AI) in Education from 2010 to 2020 Citation: Zhai, X., Chu, X., Chai, C. S., Jong, M. S. Y., Istenic, A., Spector, M., Liu, J. B., Yuan, J., & Li, Y. (2021). A Review of Artificial Intelligence (AI) in Education from 2010 to 2020. Complexity, 2021, 539–547. https://doi.org/10.1155/2021/8812542 Background Needed: Medium (technical jargon) Covers:
- This paper presents a content analysis of 100 papers where artificial intelligence (AI) has been applied to the education sector.
- Explores research trends as well as challenges for education that may be caused by AI including inappropriate use of AI techniques, changing roles of teachers and students, as well as social and ethical issues.
- The authors define three layers of research interests: development layer – which includes mostly the methodology (classification, matching, recommendation, and deep learning), application layer which includes the end goal of applying the methods (feedback, reasoning, and adaptive learning), and integration layer that connects the computational elements in the methods back to the learning processes and targets (affection computing, role-playing, immersive learning, and gamification).
- The authors also discuss challenges caused by AI due to inappropriate use of AI techniques, changing roles of teachers and students, and consideration of social and ethical issues along these perspectives.
Additional Readings and Resources
News and magazine articles.
Datasheets for Datasets Citation: Gebru, T., Morgenstern, J., Vecchione, B., Vaughan, J. W., Wallach, H., III, H. D., & Crawford, K. (2021). Datasheets for Datasets. Communications of the ACM, 64(12), 86–92. https://doi.org/10.1145/3458723 Background Needed: None Covers:
- Identifies issues with machine learning models that can reduce or amplify unwanted societal biases embedded in their training datasets.
- Describes datasheets as a way to address the gap in documenting machine learning datasets by documenting the contexts and contents of datasets including: motivation; composition; collection processes; and recommended users.
The problems AI has today go back centuries Citation: Hao, K. (2020, July 21). The problems AI has today go back centuries. MIT Technology Review. https://www.technologyreview.com/2020/07/31/1005824/decolonial-ai-for-everyone/ Background Needed: None
How to solve AI’s inequality problem Citation: Rotman, D. (2022, April 19). How to solve AI’s inequality problem. MIT Technology Review. https://www.technologyreview.com/2022/04/19/1049378/ai-inequality-problem/ Background Needed: None
AI and education: Guidance for policy-makers Citation: Miao, F., Holmes, W., Ronghuai Huang, & Hui Zhang. (n.d.). AI and education: Guidance for policy-makers. UNESCO. https://unesdoc.unesco.org/ark:/48223/pf0000376709 Background Needed: Low
Trustworthy artificial intelligence (AI) in education (No. 218) Citation: Vincent-Lancrin, S., & Vlies, R. van der. (2020). Trustworthy artificial intelligence (AI) in education (No. 218). OECD Publishing. https://doi.org/10.1787/a6c90fa9-en Background Needed: Low
AI in Education Podcast Citation: Bowen, D., Worrall, B., & Hickin, L. (n.d.). AI in Education Podcast. https://aipodcast.education/ Background Needed: None
You Look Like a Thing and I Love You: How Artificial Intelligence Works and Why It’s Making the World a Weirder Place Citation: Shane, J. (2019). You Look Like a Thing and I Love You: How Artificial Intelligence Works and Why It’s Making the World a Weirder Place . Little, Brown. Background Needed: None

- CIRCLS’23 Convening
- 2023 Community Report
- Outcomes Report
- Convening Recordings
- CIRCLS Perspectives
- RETTL Guidelines
- Project Spotlights
- Project Tag Map
- Brokering Tool
- Educator CIRCLS
- Emerging Scholar CIRCLS
- Five Ways CIRCLS Can Help You
- Rapid Community Report Database
- Publications and Videos
- Professional Development Tools
- Newsletters
- Share a Resource
- Solicitations
- Calls for Submissions
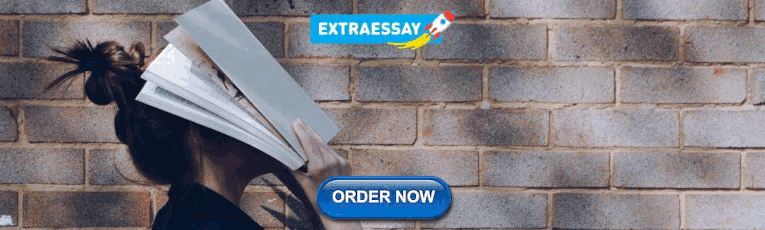
COMMENTS
Book Title: Artificial Intelligence in Education: Emerging Technologies, Models and Applications. Book Subtitle: Proceedings of 2021 2nd International Conference on Artificial Intelligence in Education Technology. Editors: Eric C. K. Cheng, Rekha B. Koul, Tianchong Wang, Xinguo Yu
Artificial Intelligence in Education: Emerging Technologies, Models and Applications. : This edited book is a collection of selected research papers presented at the 2021 2nd International Conference on Artificial Intelligence in Education Technology (AIET 2021), held in Wuhan, China on July 2-4, 2021. AIET establishes a platform for AI in ...
Request PDF | Artificial Intelligence in Education: Emerging Technologies, Models and Applications | This edited book is a collection of selected research papers presented at the 2021 2nd ...
Artificial intelligence in education: Emerging technologies, models and applications Chi Keung Eric CHENG (Editor), Rekha B. KOUL (Editor), Tianchong WANG (Editor), Xinguo YU (Editor) Department of Curriculum and Instruction (C&I)
This timely publication is well-aligned with UNESCO's Beijing Consensus on Artificial Intelligence (AI) and Education. It is committed to exploring how best to prepare our students and harness emerging technologies for achieving the Education 2030 Agenda as we move towards an era in which AI is transforming many aspects of our lives.
2.1 Prolific countries. Artificial intelligence in education (AIEd) research has been conducted in many countries around the world. The 40 articles reported AIEd research studies in 16 countries (See Table 1).USA was so far the most prolific, with nine articles meeting all criteria applied in this study, and noticeably seven of them were conducted in K-12.
UNESCO is committed to supporting Member States to harness the potential of AI technologies for achieving the Education 2030 Agenda, while ensuring that its application in educational contexts is guided by the core principles of inclusion and equity. UNESCO's mandate calls inherently for a human-centred approach to AI.
In the past few decades, technology has completely transformed the world around us. Indeed, experts believe that the next big digital transformation in how we live, communicate, work, trade and learn will be driven by Artificial Intelligence (AI) [83]. This paper presents a high-level industrial and academic overview of AI in Education (AIEd). It presents the focus of latest research in AIEd ...
The Digital and eTextbook ISBNs for Artificial Intelligence in Education: Emerging Technologies, Models and Applications are 9789811675270, 9811675279 and the print ISBNs are 9789811675263, 9811675260. Save up to 80% versus print by going digital with VitalSource.
International Conference on Artificial Intelligence in Education Technology. Search within this conference. Search. 2023 AIET 2023. ... Artificial Intelligence in Education: Emerging Technologies, Models and Applications. 26 Papers. 1 Volume.
Artificial intelligence (AI) applications in education are on the rise and have received a lot of attention in the last couple of years. AI and adaptive learning technologies are prominently featured as important developments in educational technology in the 2018 Horizon report (Educause, 2018), with a time to adoption of 2 or 3 years.According to the report, experts anticipate AI in education ...
Artificial Intelligence Applications and Technologies. AI-directed learning applies techniques such as fuzzy logic, decision trees, neural networks, Bayesian networks, and hidden Markova models to support electronic learning (e-learning) and various forms of education and training (Colchester et al. Citation 2017).The constructivist learning paradigm supports learner-centered and learner ...
Three paradigms are proposed for Artificial Intelligence in Education (AIED). • Three paradigms are AI-directed, AI-supported, and AI-empowered. • AI is used to represent knowledge models and direct cognitive learning in Paradigm One. • AI is used to support learning while learners work as collaborators with AI in Paradigm Two. •
Artificial intelligence and generative models such as ChatGPT accounts for the new requirements and convenience of learning and at least in theory, can embody a learner's socio-cultural background. However, this AI transition has magnified many pedagogical issues related to quality including the digital divide between those who have access to ...
The use of artificial intelligence (AI) in higher education (HE) has risen quickly in the last 5 years (Chu et al., 2022), with a concomitant proliferation of new AI tools available.Scholars (viz., Chen et al., 2020; Crompton et al., 2020, 2021) report on the affordances of AI to both instructors and students in HE.These benefits include the use of AI in HE to adapt instruction to the needs of ...
Advances in artificial intelligence (AI) could transform education systems and make them more equitable. It can accelerate the long overdue transformation of education systems towards inclusive learning that will prepare young people to thrive and shape a better future.; At the same time, teachers can use these technologies to enhance their teaching practice and professional experience.
A wide range of manuscripts were yielded from the education and educational research category of the Social Sciences Citation Index (SSCI) database, and papers from an AIED-specialised journal were also included. 135 of those meeting the selection criteria were analysed with content analysis and categorical meta-trends analysis.
Analysis of the impact of generative artificial intelligence model education applications The application mode is shifting from specialized to general purpose. In this mode, intelligent teaching assistants, intelligent learning companions, intelligent mentors, and other segmented systems targeting different users and scenarios will share a ...
The technological advancement and rapid development of artificial intelligence have led to a growing number of studies investigating pedagogical innovations incorporated with emerging technologies in this digital era. An increasing amount of empirical evidence has suggested the potential benefits of incorporating digital technologies and artificial intelligence in various educational contexts ...
These emerging technologies focus on informal science education and represent an intersection of AI with modern video game and computer graphics techniques. ... models, and pedagogy. Artificial intelligence and machine learning courses were at the forefront of this trend and are also being used to serve personalized, managed content in the back ...
Artificial Intelligence and the Future of Teaching and Learning. The U.S. Department of Education Office of Educational Technology's new policy report, Artificial Intelligence and the Future of Teaching and Learning: Insights and Recommendations, addresses the clear need for sharing knowledge, engaging educators, and refining technology plans and policies for artificial intelligence (AI) use ...
As an emerging novel technology, the integration of AI into education (Artificial Intelligence in Education -AIEd) arose as an interdisciplinary subfield in the early 1980s (Baker, 2021).
The 2021 report is the second in a series that will be released every five years until 2116. Titled "Gathering Strength, Gathering Storms," the report explores the various ways AI is increasingly touching people's lives in settings that range from movie recommendations and voice assistants to autonomous driving and automated medical ...
As companies increasingly integrate artificial intelligence (AI) into their operations, it becomes more essential that business students gain a mastery of emerging technologies. Business schools are responding to the need with a range of initiatives that explore the possibilities and perils of virtual reality (VR), extended reality (XR ...
Artificial intelligence (AI) has a lot of potential for innovation in educational technology and to impact traditional teaching methods. Last year, the World Economy Forum (WEF) found that ...
The use of Emerging Technologies, such as Artificial Intelligence (AI), Learning Analytics (LA) and Extended Reality (XR) applications, in higher education has proliferated in recent times, as these technologies are considered to have a significant impact on the future of postsecondary teaching and learning.
The AI for Educators Learning Path on Microsoft Learn is made up of three modules: "Empower educators to explore the potential of artificial intelligence," "Enhance teaching and learning with Microsoft Copilot," and "Equip and support learners with AI tools from Microsoft.". From now until April 30, 2024, participate in the AI ...
In E. C. K. Cheng, R. B. Koul, T. Wang, & X. Yu (Eds.), Artificial Intelligence in Education: Emerging Technologies, Models and Applications (pp. 3-24). Springer Singapore. Background Needed: Low/Medium Covers: This paper reviews research studies on artificial intelligence in education (AIED) published from 2001 to 2021