
- Lean Philosophy
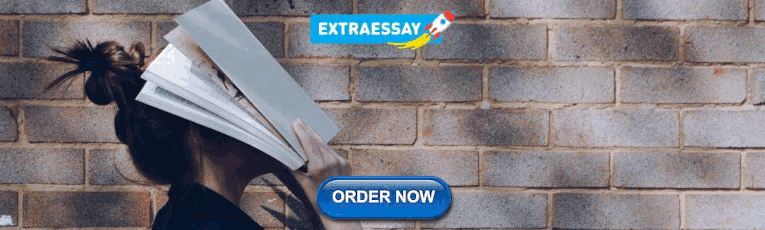
Eight Steps To Practical Problem Solving

The Toyota Way To Problem Solving
The art of problem solving is constantly trying to evolve and be re-branded by folks in various industries. While the new way might very well be an effective method in certain applications. A tried and true way of identifying and solving problems is the eight steps to practical problem solving developed by Toyota, years ago. The system is structured, but simple and practical enough to handle problems of the smallest nature, to the most complex issues.
Using a fundamental and strategic way to solve problems creates consistency within an organization. When you base your results off facts, experience and common sense, the results form in a rational and sustainable way.

The Eight Step Problem Solving Process
- Clarify the Problem
- Breakdown the Problem
- Set the Target
- Analyze the Root Cause
- Develop Countermeasures
- Implement Countermeasures
- Monitor Results and Process
- Standardize and Share Success
The eight steps to practical problem solving also include the Plan, Do, Check and Act (PDCA) cycle. Steps one through five are the planning process. The doing is found in step six. Step seven is the checking . Step eight involves acting out the results of the new standard.
This practical problem solving can be powerful tool to issues facing your organization. It allows organizations to have a common understanding of what defines a problem and what steps are going to be taken in order to overcome the problem efficiently.
The Eight Steps Broken Down:
Step 1: clarify the problem.
A problem can be defined in one of three ways. The first being, anything that is a deviation from the standard. The second could be the gap between the actual condition and the desired condition. With the third being an unfilled customer need.
In order to best clarify the problem, you have to see the problem with your own eyes. This gives you the details and hands-on experience that will allow you to move forward in the process.
Step 2: Breakdown the Problem
Once you’ve seen the problem first hand, you can begin to breakdown the problem into more detailed and specific problems. Remember, as you breakdown your problem you still need to see the smaller, individual problems with your own eyes. This is also a good time to study and analyze the different inputs and outputs of the process so that you can effectively prioritize your efforts. It is much more effective to manage and solve a bunch of micro-problems one at a time, rather than try and tackle a big problem with no direction.
Step 3: Set the Target

Step three is all about commitment and focus. Your attention should now turn towards focusing on what is needed to complete the project and how long it will take to finish. You should set targets that are challenging, but within limits and don’t put a strain on the organization that would hinder the improvement process.
Step 4: Analyze the Root Cause
This is a vital step when problem solving, because it will help you identify the actual factors that caused the issue in the first place. More often than not, there are multiple root causes to analyze. Make sure you are considering all potential root causes and addressing them properly. A proper root cause analysis, again involves you actually going to the cause itself instead of simply relying on reports.
Step 5: Develop Countermeasures
Once you’ve established your root causes, you can use that information to develop the countermeasures needed to remove the root causes. Your team should develop as many countermeasures needed to directly address any and all root causes. Once you’ve developed your countermeasures, you can begin to narrow them down to the most practical and effective based off your target.
Step 6: Implement Countermeasures
Now that you have developed your countermeasures and narrowed them down, it is time to see them through in a timely manner. Communication is extremely important in step six. You’ll want to seek ideas from the team and continue to work back through the PDCA cycle to ensure nothing is being missed along the way. Consider implementing one countermeasure at a time to monitor the effectiveness of each.
You will certainly make mistakes in throughout your problem solving processes, but your persistence is key, especially in step six.
Step 7: Monitor Results and Process
As mistakes happen and countermeasures fail, you need a system in place to review and modify them to get the intended result. You can also determine if the intended outcome was the result of the action of the countermeasure, or was it just a fluke? There is always room for improvement in the problem solving process, but you need to be able to recognize it when it comes to your attention.
Step 8: Standardize and Share Success
Now that you’ve encountered success along your problem solving path, it is time to set the new processes as the new standard within the organization and share them throughout the organization. It is also a good time to reflect on what you’ve learned and address any possible unresolved issues or troubles you have along the way. Ignoring unresolved issues will only lead to more problems down the road.
Finally, because you are a true Lean organization who believes continuous improvement never stops, it is time to tackle the next problem. Start the problem solving process over again and continue to work towards perfection.
Additional Resources
- 8D for Problem Solving – creativesafetysupply.com
- Training to Use 8D Problem-Solving Tactics – blog.creativesafetysupply.com
- The Great Root Cause Problem Solving Debate – realsafety.org
- Design Thinking: Empathy and Iteration for Innovation and Problem-Solving – creativesafetypublishing.com
- 10 Commandments to Continuous Improvement – lean-news.com
- Lean Manufacturing Implementation – The First 5 Steps – iecieeechallenge.org
- The Transitional Steps Involved In The 5s Principles During Implementation – 5snews.com
- “No Problem” is a Problem – jakegoeslean.com
- The Tools of Kaizen – blog.5stoday.com
Related posts:
- 3P and Lean
- The Vacation Paradox
- Why Single Minute Exchange of Die (SMED)?
- Total Quality Management And Kaizen Principles In Lean Management
- An Engaged Employee is a Productive Employee
- Jim Womack’s Top Misconceptions of the Lean Movement
- Muda, Mura, and Muri: The Three Wastes

Toyota Practical Problem Solving (PPS)—Introduction

The Framework: PDCA

- Plan is to identify and clarify the problem, including collecting data to understand the problem, setting a target, and doing a root-cause analysis.
- Do is the development and implementation of countermeasures.
- Check verifies whether these countermeasures were effective and the target has been reached.
- Act is to re-do and further improve if the targets have not been met (yet). If it was successful, the Act part looks for other locations and applications where this solution could be used (e.g., if it was a smaller trial to be rolled out on a larger scale). Toyota also shares these yokoten on an internal website with other plants.

Toyota practical problem solving consists of the steps as listed below. Note that sometimes you have a step more if you decide to split a step into two.
- Clarify the Problem
- Break Down the Problem
- Set a Target
- Root-Cause Analysis
- Develop Countermeasures and Implement
- Monitor Process and Results
- Standardize and Share
I will explain all these steps in much more detail, including the risks and difficulties, throughout this small series of posts. But before explaining these steps in detail, let me also show you the structure.
The Structure: A3
You probably know the structure already, or at least have heard of it: it is the famous A3 . This report, named after the standard A3 paper size, is commonly used at Toyota to tackle medium-sized problems. The A3 format was chosen because it was a good compromise between getting lots of data on a single page and also having a page small enough to be carried around on the shop floor. (And, as legend has it, A3 was supposedly the largest format to fit though a fax machine back in the day).
You will find all the steps from above again in this A3 format, an example of which is shown below, plus the obligatory header row with organizational data like title, date, and so on.

The A3 is intended to be filled out in pencil (not pen), which makes changing content easy by using an eraser. Nowadays digital tools are also often used, although Toyota still does this mostly by hand using pencil on paper. Digital A3s are easier to share and look prettier, but they are harder to make and much more effort is needed in creating the A3. If you have ever created an A3 in Microsoft Excel, you know what I am talking about (as Excel is wholly unsuited for such graphical work…Ugh!)
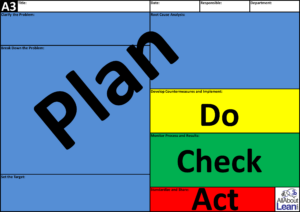
The “Do” part is actually quite small. If you understand the problem well, the solutions are rather easy. If you don’t understand the problem, you still may have a solution, but it will probably be an inferior one, if it works at all. Similarly, the Check and Act are also rather small.
In my experience, this is often done differently (and in my opinion worse) in many other Western companies. The focus is all on doing something, implementing some sort of solution. There is a bit of planning, but the vast majority of the effort goes into the “Do” part. The “Check” and “Act” parts are quite underdeveloped, if they exist at all.
A fancy presentation often substitutes for “Check,” resulting in many supposedly successful projects that did not improve much or even made it worse. Below I compared the normal representation of the PDCA circle having four equal quadrants with a PDCA circle based on the effort by Japanese or Toyota standards, and another PDCA circle based on the effort of (way too many) Western companies. I’ll let you be the judge on how this is in your company.
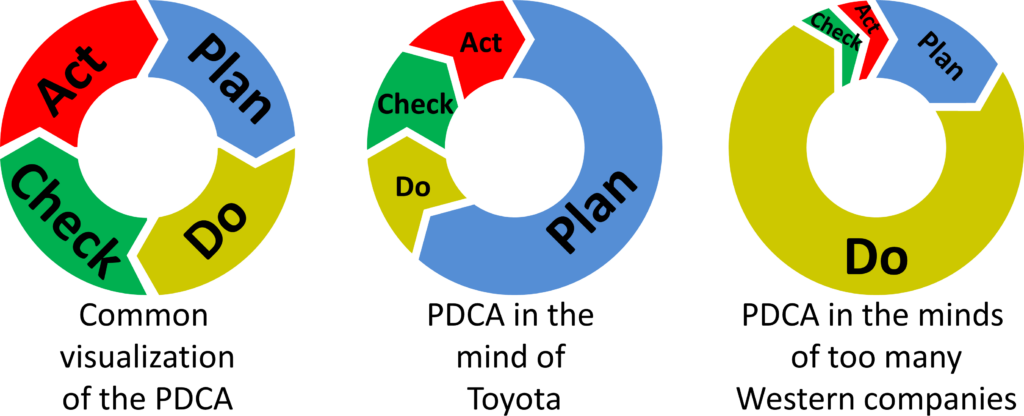
Over and over again I guide people through the practical problem-solving process, and at every single step they jump to a solution. Let’s take a (fictitious) example for the steps of the problem solving, where every step is going right for the solution, ignoring the initial purpose of the step:
- Clarify the Problem: Well, we need kanban!
- Break Down the Problem: Okay, how many kanban do we need?
- Set a Target: That’s how many kanban we need!
- Root-Cause Analysis: Um… we did this already. It’s the lack of kanban…
- Develop Countermeasures and Implement: Add kanban!
- Monitor Process and Results: Do we have kanban now? Yes, we do. Case closed.
- Standardize and Share: Hey, guys, use kanban!

PS: Many thanks to the team from the Toyota Lean Management Centre at the Toyota UK Deeside engine plant in Wales, where I participated in their 5-day course. This course gave us a lot of access to the Toyota shop floor, and we spent hours on the shop floor looking at processes. In my view, this the only generally accessible course by Toyota that gives such a level of shop floor involvement.
6 thoughts on “Toyota Practical Problem Solving (PPS)—Introduction”
Great ‘ Flow ‘ and easy to understand , specially for many who have limited exposure. Thanks
Thank you for sharing. PDCA is applicable on the shop floor, logistics, service industry – wherever Problems are accurately defined
Nice blog. I’m working in an NHS Production System (NHSps) design based on the Toyota and VMI Production Systems. Do you have any experience in this area?
Hi Tom, sorry, I am completely unfamiliar with the NHS production system. if you mean the National Health Service in the UK, I do have a bit of experience with lean Hospital.
Might I suggest a dry erase marker and a whiteboard? After a few times when the document structure is mostly stable, you can add lines with a permanent marker to fix the format in place. That way you’re not creating extra friction for the process.
As for actual A3, that works best when the A3 paper and printers that can print it are already readily available. I’m sure you ran across more than one business where just about every single printer on site can’t print anything larger than A4 or A3 paper simply isn’t available due to A4 used for everything.
Hi Andrey, I am a great fan of erasable notes, and use dry erase whiteboard markers a lot myself. An A3 printer is also really helpful, but just as you said, not every (small) business has one. For example, I only have an A4 printer in my office…
Leave a Comment
Notify me of new posts by email.

A Step-by-Step Guide to A3 Problem Solving Methodology
Problem-solving is an important component of any business or organization. It entails identifying, analyzing, and resolving problems in order to improve processes, drive results, and foster a culture of continuous improvement. A3 Problem solving is one of the most effective problem-solving methodologies.
A3 Problem Solving has numerous advantages, including improved communication, better decision-making, increased efficiency, and reduced waste. It is a powerful tool for businesses of all sizes and industries, and it is especially useful for solving complex and multi-faceted problems.
What is A3 Problem Solving?
A3 Problem Solving is a structured and systematic approach to problem-solving that makes use of a one-page document called an A3 report to visually represent the process. The A3 report provides an overview of the problem, data analysis, root causes, solutions, and results in a clear and concise manner. The method was created within the framework of the Lean manufacturing methodology and is based on the principles of continuous improvement and visual management.
Origin and History of A3 Problem Solving
Key principles of a3 problem solving.
The following are the key principles of A3 Problem Solving:
These principles serve as the foundation of the A3 Problem Solving methodology and are intended to assist organisations in continuously improving and achieving their objectives. Organizations can effectively solve problems, identify areas for improvement, and drive results by adhering to these principles.
Step 1: Define the Problem
Importance of clearly defining the problem.
However, if the problem is not clearly defined, the data gathered may be irrelevant or incorrect, resulting in incorrect conclusions and ineffective solutions. Furthermore, the process of collecting and analysing data can become time-consuming and inefficient, resulting in resource waste. Furthermore, if the problem is not accurately defined, the solutions developed may fail to address the root cause of the problem, resulting in ongoing issues and a lack of improvement.
Techniques for Defining the Problem
Best practice for defining the problem.
In addition to brainstorming, root cause analysis, process mapping, and Ishikawa diagram s, best practices should be followed when defining a problem in A3 Problem Solving. Among these best practices are:
Organizations can ensure that their problem is defined in a way that allows for effective data collection, analysis, and solution development by following these best practices. This will aid in the development of appropriate solutions and the effective resolution of the problem, resulting in improvements in the organization’s processes and outcomes.
Step 2: Gather Data
Gathering data in a3 problem solving.
Data collection is an important step in the A3 Problem Solving process because it allows organisations to gain a thorough understanding of the problem they are attempting to solve. This step entails gathering pertinent information about the problem, such as data on its origin, impact, and any related factors. This information is then used to help identify root causes and develop effective solutions.
Overall, data collection is an important step in the A3 Problem Solving process because it serves as the foundation for effective problem-solving. Organizations can gain a deep understanding of the problem they are attempting to solve and develop effective solutions that address its root cause by collecting and analysing relevant data.
Data Collection Methods
The best data collection method will be determined by the problem being solved and the type of data required. To gain a complete understanding of the problem, it is critical to use multiple data collection methods.
Tools for Data Analysis and Visualization
These tools can assist in organising data and making it easier to understand. They can also be used to generate visual representations of data, such as graphs and charts, to communicate the findings to others.
Step 3: Identify Root Causes
Overview of the root cause analysis process.
The process of determining the underlying causes of a problem is known as root cause analysis. This process can assist organisations in determining why a problem is occurring and what can be done to prevent it from recurring in the future. The goal of root cause analysis is to identify the underlying cause of a problem rather than just its symptoms, allowing it to be addressed more effectively.
Techniques for Identifying Root Causes
Best practices for conducting root cause analysis, step 4: develop solutions, solution development in a3 problem solving.
A3 solution development Problem solving is an iterative process in which options are generated and evaluated. The data gathered in the previous steps, as well as the insights and understanding gained from the root cause analysis, guide this process. The solution should be based on a thorough understanding of the problem and address the underlying cause.
Techniques for Developing Solutions
Best practice for developing solutions, step 5: implement solutions, the implementation process.
The implementation process entails putting the solutions developed in the previous step into action. This could include changes to processes, procedures, and systems, as well as employee training and education. To ensure that the solutions are effective, the implementation process should be well-planned and meticulously executed.
Techniques for Implementing Solutions
Best practice for implementing solutions.
It is critical to follow these best practices when implementing solutions in A3 Problem Solving:
Organizations can improve their problem-solving skills and achieve better results by following the key principles, techniques, and best practices outlined in this guide. As a result, both the organisation and its stakeholders will benefit from increased efficiency, effectiveness, and satisfaction. So, whether you’re an experienced problem solver or just getting started, consider incorporating the A3 Problem Solving methodology into your work and start reaping the benefits right away.
Was this helpful?
Daniel croft, lean manufacturing in the age of industry 4.0: how to incorporate technology for optimal results, the voice of the customer (voc), free lean six sigma templates.
Improve your Lean Six Sigma projects with our free templates. They're designed to make implementation and management easier, helping you achieve better results.
Understanding Process Performance: Pp and Ppk
Understand Process Performance (Pp) and Process Performance Index (Ppk) to assess and improve manufacturing processes.…
LIFO or FIFO for Stock Management?
Choosing between LIFO and FIFO for stock management depends on factors like product nature, market…
Are There Any Official Standards for Six Sigma?
Are there any official standards for Six Sigma? While Six Sigma is a well-defined methodology…
5S Floor Marking Best Practices
In lean manufacturing, the 5S System is a foundational tool, involving the steps: Sort, Set…
How to Measure the ROI of Continuous Improvement Initiatives
When it comes to business, knowing the value you’re getting for your money is crucial,…
8D Problem-Solving: Common Mistakes to Avoid
In today’s competitive business landscape, effective problem-solving is the cornerstone of organizational success. The 8D…
40 problem-solving techniques and processes
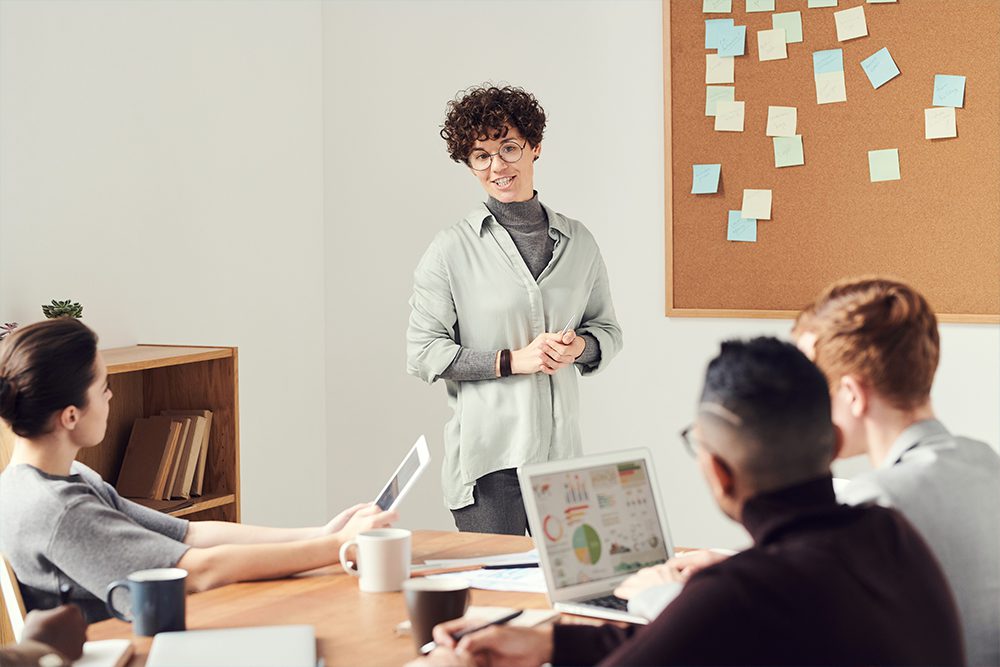
All teams and organizations encounter challenges. Approaching those challenges without a structured problem solving process can end up making things worse.
Proven problem solving techniques such as those outlined below can guide your group through a process of identifying problems and challenges , ideating on possible solutions , and then evaluating and implementing the most suitable .
In this post, you'll find problem-solving tools you can use to develop effective solutions. You'll also find some tips for facilitating the problem solving process and solving complex problems.
Design your next session with SessionLab
Join the 150,000+ facilitators using SessionLab.
Recommended Articles
A step-by-step guide to planning a workshop, 54 great online tools for workshops and meetings, how to create an unforgettable training session in 8 simple steps.
- 18 Free Facilitation Resources We Think You’ll Love
What is problem solving?
Problem solving is a process of finding and implementing a solution to a challenge or obstacle. In most contexts, this means going through a problem solving process that begins with identifying the issue, exploring its root causes, ideating and refining possible solutions before implementing and measuring the impact of that solution.
For simple or small problems, it can be tempting to skip straight to implementing what you believe is the right solution. The danger with this approach is that without exploring the true causes of the issue, it might just occur again or your chosen solution may cause other issues.
Particularly in the world of work, good problem solving means using data to back up each step of the process, bringing in new perspectives and effectively measuring the impact of your solution.
Effective problem solving can help ensure that your team or organization is well positioned to overcome challenges, be resilient to change and create innovation. In my experience, problem solving is a combination of skillset, mindset and process, and it’s especially vital for leaders to cultivate this skill.
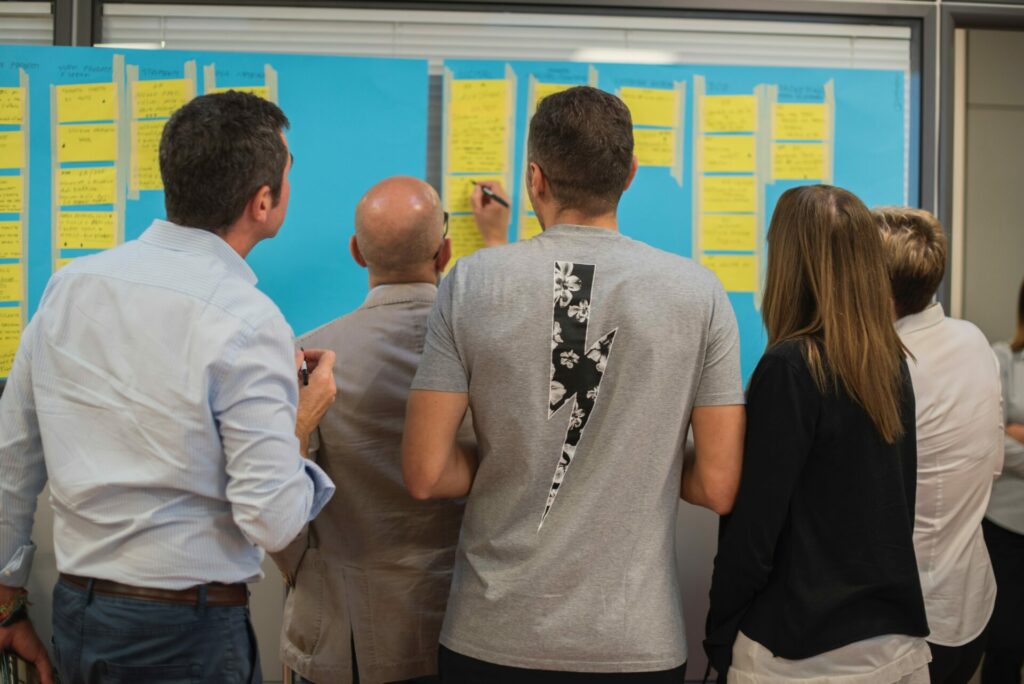
What is the seven step problem solving process?
A problem solving process is a step-by-step framework from going from discovering a problem all the way through to implementing a solution.
With practice, this framework can become intuitive, and innovative companies tend to have a consistent and ongoing ability to discover and tackle challenges when they come up.
You might see everything from a four step problem solving process through to seven steps. While all these processes cover roughly the same ground, I’ve found a seven step problem solving process is helpful for making all key steps legible.
We’ll outline that process here and then follow with techniques you can use to explore and work on that step of the problem solving process with a group.
The seven-step problem solving process is:
1. Problem identification
The first stage of any problem solving process is to identify the problem(s) you need to solve. This often looks like using group discussions and activities to help a group surface and effectively articulate the challenges they’re facing and wish to resolve.
Be sure to align with your team on the exact definition and nature of the problem you’re solving. An effective process is one where everyone is pulling in the same direction – ensure clarity and alignment now to help avoid misunderstandings later.
2. Problem analysis and refinement
The process of problem analysis means ensuring that the problem you are seeking to solve is the right problem . Choosing the right problem to solve means you are on the right path to creating the right solution.
At this stage, you may look deeper at the problem you identified to try and discover the root cause at the level of people or process. You may also spend some time sourcing data, consulting relevant parties and creating and refining a problem statement.
Problem refinement means adjusting scope or focus of the problem you will be aiming to solve based on what comes up during your analysis. As you analyze data sources, you might discover that the root cause means you need to adjust your problem statement. Alternatively, you might find that your original problem statement is too big to be meaningful approached within your current project.
Remember that the goal of any problem refinement is to help set the stage for effective solution development and deployment. Set the right focus and get buy-in from your team here and you’ll be well positioned to move forward with confidence.
3. Solution generation
Once your group has nailed down the particulars of the problem you wish to solve, you want to encourage a free flow of ideas connecting to solving that problem. This can take the form of problem solving games that encourage creative thinking or techniquess designed to produce working prototypes of possible solutions.
The key to ensuring the success of this stage of the problem solving process is to encourage quick, creative thinking and create an open space where all ideas are considered. The best solutions can often come from unlikely places and by using problem solving techniques that celebrate invention, you might come up with solution gold.
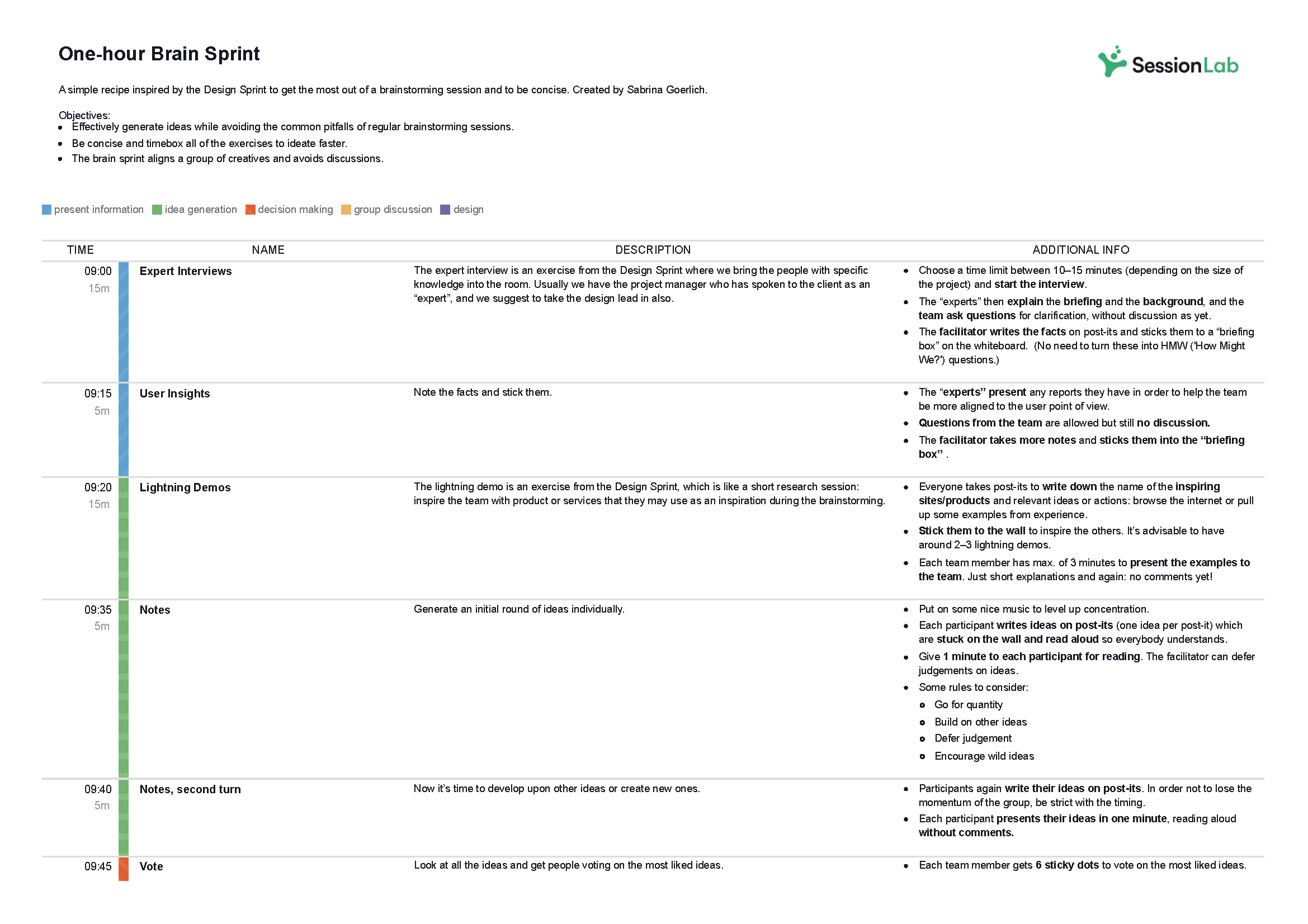
4. Solution development
No solution is perfect right out of the gate. It’s important to discuss and develop the solutions your group has come up with over the course of following the previous problem solving steps in order to arrive at the best possible solution. Problem solving games used in this stage involve lots of critical thinking, measuring potential effort and impact, and looking at possible solutions analytically.
During this stage, you will often ask your team to iterate and improve upon your front-running solutions and develop them further. Remember that problem solving strategies always benefit from a multitude of voices and opinions, and not to let ego get involved when it comes to choosing which solutions to develop and take further.
Finding the best solution is the goal of all problem solving workshops and here is the place to ensure that your solution is well thought out, sufficiently robust and fit for purpose.
5. Decision making and planning
Nearly there! Once you’ve got a set of possible, you’ll need to make a decision on which to implement. This can be a consensus-based group decision or it might be for a leader or major stakeholder to decide. You’ll find a set of effective decision making methods below.
Once your group has reached consensus and selected a solution, there are some additional actions that also need to be decided upon. You’ll want to work on allocating ownership of the project, figure out who will do what, how the success of the solution will be measured and decide the next course of action.
Set clear accountabilities, actions, timeframes, and follow-ups for your chosen solution. Make these decisions and set clear next-steps in the problem solving workshop so that everyone is aligned and you can move forward effectively as a group.
Ensuring that you plan for the roll-out of a solution is one of the most important problem solving steps. Without adequate planning or oversight, it can prove impossible to measure success or iterate further if the problem was not solved.
6. Solution implementation
This is what we were waiting for! All problem solving processes have the end goal of implementing an effective and impactful solution that your group has confidence in.
Project management and communication skills are key here – your solution may need to adjust when out in the wild or you might discover new challenges along the way. For some solutions, you might also implement a test with a small group and monitor results before rolling it out to an entire company.
You should have a clear owner for your solution who will oversee the plans you made together and help ensure they’re put into place. This person will often coordinate the implementation team and set-up processes to measure the efficacy of your solution too.
7. Solution evaluation
So you and your team developed a great solution to a problem and have a gut feeling it’s been solved. Work done, right? Wrong. All problem solving strategies benefit from evaluation, consideration, and feedback.
You might find that the solution does not work for everyone, might create new problems, or is potentially so successful that you will want to roll it out to larger teams or as part of other initiatives.
None of that is possible without taking the time to evaluate the success of the solution you developed in your problem solving model and adjust if necessary.
Remember that the problem solving process is often iterative and it can be common to not solve complex issues on the first try. Even when this is the case, you and your team will have generated learning that will be important for future problem solving workshops or in other parts of the organization.
It’s also worth underlining how important record keeping is throughout the problem solving process. If a solution didn’t work, you need to have the data and records to see why that was the case. If you go back to the drawing board, notes from the previous workshop can help save time.
What does an effective problem solving process look like?
Every effective problem solving process begins with an agenda . In our experience, a well-structured problem solving workshop is one of the best methods for successfully guiding a group from exploring a problem to implementing a solution.
The format of a workshop ensures that you can get buy-in from your group, encourage free-thinking and solution exploration before making a decision on what to implement following the session.
This Design Sprint 2.0 template is an effective problem solving process from top agency AJ&Smart. It’s a great format for the entire problem solving process, with four-days of workshops designed to surface issues, explore solutions and even test a solution.
Check it for an example of how you might structure and run a problem solving process and feel free to copy and adjust it your needs!
For a shorter process you can run in a single afternoon, this remote problem solving agenda will guide you effectively in just a couple of hours.
Whatever the length of your workshop, by using SessionLab, it’s easy to go from an idea to a complete agenda . Start by dragging and dropping your core problem solving activities into place . Add timings, breaks and necessary materials before sharing your agenda with your colleagues.
The resulting agenda will be your guide to an effective and productive problem solving session that will also help you stay organized on the day!
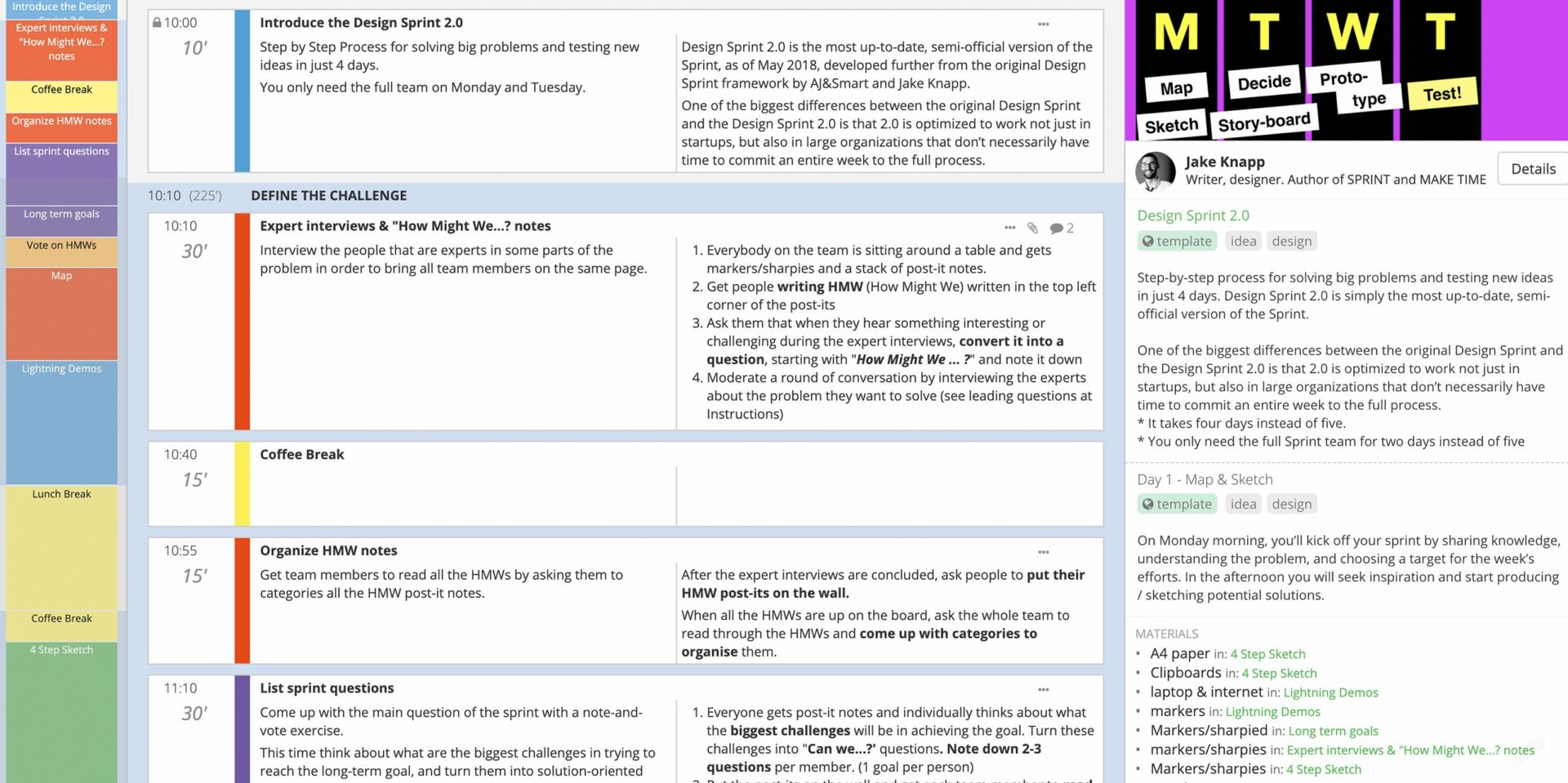
Complete problem-solving methods
In this section, we’ll look at in-depth problem-solving methods that provide a complete end-to-end process for developing effective solutions. These will help guide your team from the discovery and definition of a problem through to delivering the right solution.
If you’re looking for an all-encompassing method or problem-solving model, these processes are a great place to start. They’ll ask your team to challenge preconceived ideas and adopt a mindset for solving problems more effectively.
Six Thinking Hats
Individual approaches to solving a problem can be very different based on what team or role an individual holds. It can be easy for existing biases or perspectives to find their way into the mix, or for internal politics to direct a conversation.
Six Thinking Hats is a classic method for identifying the problems that need to be solved and enables your team to consider them from different angles, whether that is by focusing on facts and data, creative solutions, or by considering why a particular solution might not work.
Like all problem-solving frameworks, Six Thinking Hats is effective at helping teams remove roadblocks from a conversation or discussion and come to terms with all the aspects necessary to solve complex problems.
The Six Thinking Hats #creative thinking #meeting facilitation #problem solving #issue resolution #idea generation #conflict resolution The Six Thinking Hats are used by individuals and groups to separate out conflicting styles of thinking. They enable and encourage a group of people to think constructively together in exploring and implementing change, rather than using argument to fight over who is right and who is wrong.
Lightning Decision Jam
Featured courtesy of Jonathan Courtney of AJ&Smart Berlin, Lightning Decision Jam is one of those strategies that should be in every facilitation toolbox. Exploring problems and finding solutions is often creative in nature, though as with any creative process, there is the potential to lose focus and get lost.
Unstructured discussions might get you there in the end, but it’s much more effective to use a method that creates a clear process and team focus.
In Lightning Decision Jam, participants are invited to begin by writing challenges, concerns, or mistakes on post-its without discussing them before then being invited by the moderator to present them to the group.
From there, the team vote on which problems to solve and are guided through steps that will allow them to reframe those problems, create solutions and then decide what to execute on.
By deciding the problems that need to be solved as a team before moving on, this group process is great for ensuring the whole team is aligned and can take ownership over the next stages.
Lightning Decision Jam (LDJ) #action #decision making #problem solving #issue analysis #innovation #design #remote-friendly It doesn’t matter where you work and what your job role is, if you work with other people together as a team, you will always encounter the same challenges: Unclear goals and miscommunication that cause busy work and overtime Unstructured meetings that leave attendants tired, confused and without clear outcomes. Frustration builds up because internal challenges to productivity are not addressed Sudden changes in priorities lead to a loss of focus and momentum Muddled compromise takes the place of clear decision- making, leaving everybody to come up with their own interpretation. In short, a lack of structure leads to a waste of time and effort, projects that drag on for too long and frustrated, burnt out teams. AJ&Smart has worked with some of the most innovative, productive companies in the world. What sets their teams apart from others is not better tools, bigger talent or more beautiful offices. The secret sauce to becoming a more productive, more creative and happier team is simple: Replace all open discussion or brainstorming with a structured process that leads to more ideas, clearer decisions and better outcomes. When a good process provides guardrails and a clear path to follow, it becomes easier to come up with ideas, make decisions and solve problems. This is why AJ&Smart created Lightning Decision Jam (LDJ). It’s a simple and short, but powerful group exercise that can be run either in-person, in the same room, or remotely with distributed teams.
Problem Definition Process
While problems can be complex, the problem-solving methods you use to identify and solve those problems can often be simple in design.
By taking the time to truly identify and define a problem before asking the group to reframe the challenge as an opportunity, this method is a great way to enable change.
Begin by identifying a focus question and exploring the ways in which it manifests before splitting into five teams who will each consider the problem using a different method: escape, reversal, exaggeration, distortion or wishful. Teams develop a problem objective and create ideas in line with their method before then feeding them back to the group.
This method is great for enabling in-depth discussions while also creating space for finding creative solutions too!
Problem Definition #problem solving #idea generation #creativity #online #remote-friendly A problem solving technique to define a problem, challenge or opportunity and to generate ideas.
The 5 Whys
Sometimes, a group needs to go further with their strategies and analyze the root cause at the heart of organizational issues. An RCA or root cause analysis is the process of identifying what is at the heart of business problems or recurring challenges.
The 5 Whys is a simple and effective method of helping a group go find the root cause of any problem or challenge and conduct analysis that will deliver results.
By beginning with the creation of a problem statement and going through five stages to refine it, The 5 Whys provides everything you need to truly discover the cause of an issue.
The 5 Whys #hyperisland #innovation This simple and powerful method is useful for getting to the core of a problem or challenge. As the title suggests, the group defines a problems, then asks the question “why” five times, often using the resulting explanation as a starting point for creative problem solving.
World Cafe is a simple but powerful facilitation technique to help bigger groups to focus their energy and attention on solving complex problems.
World Cafe enables this approach by creating a relaxed atmosphere where participants are able to self-organize and explore topics relevant and important to them which are themed around a central problem-solving purpose. Create the right atmosphere by modeling your space after a cafe and after guiding the group through the method, let them take the lead!
Making problem-solving a part of your organization’s culture in the long term can be a difficult undertaking. More approachable formats like World Cafe can be especially effective in bringing people unfamiliar with workshops into the fold.
World Cafe #hyperisland #innovation #issue analysis World Café is a simple yet powerful method, originated by Juanita Brown, for enabling meaningful conversations driven completely by participants and the topics that are relevant and important to them. Facilitators create a cafe-style space and provide simple guidelines. Participants then self-organize and explore a set of relevant topics or questions for conversation.
Discovery & Action Dialogue (DAD)
One of the best approaches is to create a safe space for a group to share and discover practices and behaviors that can help them find their own solutions.
With DAD, you can help a group choose which problems they wish to solve and which approaches they will take to do so. It’s great at helping remove resistance to change and can help get buy-in at every level too!
This process of enabling frontline ownership is great in ensuring follow-through and is one of the methods you will want in your toolbox as a facilitator.
Discovery & Action Dialogue (DAD) #idea generation #liberating structures #action #issue analysis #remote-friendly DADs make it easy for a group or community to discover practices and behaviors that enable some individuals (without access to special resources and facing the same constraints) to find better solutions than their peers to common problems. These are called positive deviant (PD) behaviors and practices. DADs make it possible for people in the group, unit, or community to discover by themselves these PD practices. DADs also create favorable conditions for stimulating participants’ creativity in spaces where they can feel safe to invent new and more effective practices. Resistance to change evaporates as participants are unleashed to choose freely which practices they will adopt or try and which problems they will tackle. DADs make it possible to achieve frontline ownership of solutions.
Design Sprint 2.0
Want to see how a team can solve big problems and move forward with prototyping and testing solutions in a few days? The Design Sprint 2.0 template from Jake Knapp, author of Sprint, is a complete agenda for a with proven results.
Developing the right agenda can involve difficult but necessary planning. Ensuring all the correct steps are followed can also be stressful or time-consuming depending on your level of experience.
Use this complete 4-day workshop template if you are finding there is no obvious solution to your challenge and want to focus your team around a specific problem that might require a shortcut to launching a minimum viable product or waiting for the organization-wide implementation of a solution.
Open space technology
Open space technology- developed by Harrison Owen – creates a space where large groups are invited to take ownership of their problem solving and lead individual sessions. Open space technology is a great format when you have a great deal of expertise and insight in the room and want to allow for different takes and approaches on a particular theme or problem you need to be solved.
Start by bringing your participants together to align around a central theme and focus their efforts. Explain the ground rules to help guide the problem-solving process and then invite members to identify any issue connecting to the central theme that they are interested in and are prepared to take responsibility for.
Once participants have decided on their approach to the core theme, they write their issue on a piece of paper, announce it to the group, pick a session time and place, and post the paper on the wall. As the wall fills up with sessions, the group is then invited to join the sessions that interest them the most and which they can contribute to, then you’re ready to begin!
Everyone joins the problem-solving group they’ve signed up to, record the discussion and if appropriate, findings can then be shared with the rest of the group afterward.
Open Space Technology #action plan #idea generation #problem solving #issue analysis #large group #online #remote-friendly Open Space is a methodology for large groups to create their agenda discerning important topics for discussion, suitable for conferences, community gatherings and whole system facilitation
Techniques to identify and analyze problems
Using a problem-solving method to help a team identify and analyze a problem can be a quick and effective addition to any workshop or meeting.
While further actions are always necessary, you can generate momentum and alignment easily, and these activities are a great place to get started.
We’ve put together this list of techniques to help you and your team with problem identification, analysis, and discussion that sets the foundation for developing effective solutions.
Let’s take a look!
Fishbone Analysis
Organizational or team challenges are rarely simple, and it’s important to remember that one problem can be an indication of something that goes deeper and may require further consideration to be solved.
Fishbone Analysis helps groups to dig deeper and understand the origins of a problem. It’s a great example of a root cause analysis method that is simple for everyone on a team to get their head around.
Participants in this activity are asked to annotate a diagram of a fish, first adding the problem or issue to be worked on at the head of a fish before then brainstorming the root causes of the problem and adding them as bones on the fish.
Using abstractions such as a diagram of a fish can really help a team break out of their regular thinking and develop a creative approach.
Fishbone Analysis #problem solving ##root cause analysis #decision making #online facilitation A process to help identify and understand the origins of problems, issues or observations.
Problem Tree
Encouraging visual thinking can be an essential part of many strategies. By simply reframing and clarifying problems, a group can move towards developing a problem solving model that works for them.
In Problem Tree, groups are asked to first brainstorm a list of problems – these can be design problems, team problems or larger business problems – and then organize them into a hierarchy. The hierarchy could be from most important to least important or abstract to practical, though the key thing with problem solving games that involve this aspect is that your group has some way of managing and sorting all the issues that are raised.
Once you have a list of problems that need to be solved and have organized them accordingly, you’re then well-positioned for the next problem solving steps.
Problem tree #define intentions #create #design #issue analysis A problem tree is a tool to clarify the hierarchy of problems addressed by the team within a design project; it represents high level problems or related sublevel problems.
SWOT Analysis
Chances are you’ve heard of the SWOT Analysis before. This problem-solving method focuses on identifying strengths, weaknesses, opportunities, and threats is a tried and tested method for both individuals and teams.
Start by creating a desired end state or outcome and bare this in mind – any process solving model is made more effective by knowing what you are moving towards. Create a quadrant made up of the four categories of a SWOT analysis and ask participants to generate ideas based on each of those quadrants.
Once you have those ideas assembled in their quadrants, cluster them together based on their affinity with other ideas. These clusters are then used to facilitate group conversations and move things forward.
SWOT analysis #gamestorming #problem solving #action #meeting facilitation The SWOT Analysis is a long-standing technique of looking at what we have, with respect to the desired end state, as well as what we could improve on. It gives us an opportunity to gauge approaching opportunities and dangers, and assess the seriousness of the conditions that affect our future. When we understand those conditions, we can influence what comes next.
Agreement-Certainty Matrix
Not every problem-solving approach is right for every challenge, and deciding on the right method for the challenge at hand is a key part of being an effective team.
The Agreement Certainty matrix helps teams align on the nature of the challenges facing them. By sorting problems from simple to chaotic, your team can understand what methods are suitable for each problem and what they can do to ensure effective results.
If you are already using Liberating Structures techniques as part of your problem-solving strategy, the Agreement-Certainty Matrix can be an invaluable addition to your process. We’ve found it particularly if you are having issues with recurring problems in your organization and want to go deeper in understanding the root cause.
Agreement-Certainty Matrix #issue analysis #liberating structures #problem solving You can help individuals or groups avoid the frequent mistake of trying to solve a problem with methods that are not adapted to the nature of their challenge. The combination of two questions makes it possible to easily sort challenges into four categories: simple, complicated, complex , and chaotic . A problem is simple when it can be solved reliably with practices that are easy to duplicate. It is complicated when experts are required to devise a sophisticated solution that will yield the desired results predictably. A problem is complex when there are several valid ways to proceed but outcomes are not predictable in detail. Chaotic is when the context is too turbulent to identify a path forward. A loose analogy may be used to describe these differences: simple is like following a recipe, complicated like sending a rocket to the moon, complex like raising a child, and chaotic is like the game “Pin the Tail on the Donkey.” The Liberating Structures Matching Matrix in Chapter 5 can be used as the first step to clarify the nature of a challenge and avoid the mismatches between problems and solutions that are frequently at the root of chronic, recurring problems.
Organizing and charting a team’s progress can be important in ensuring its success. SQUID (Sequential Question and Insight Diagram) is a great model that allows a team to effectively switch between giving questions and answers and develop the skills they need to stay on track throughout the process.
Begin with two different colored sticky notes – one for questions and one for answers – and with your central topic (the head of the squid) on the board. Ask the group to first come up with a series of questions connected to their best guess of how to approach the topic. Ask the group to come up with answers to those questions, fix them to the board and connect them with a line. After some discussion, go back to question mode by responding to the generated answers or other points on the board.
It’s rewarding to see a diagram grow throughout the exercise, and a completed SQUID can provide a visual resource for future effort and as an example for other teams.
SQUID #gamestorming #project planning #issue analysis #problem solving When exploring an information space, it’s important for a group to know where they are at any given time. By using SQUID, a group charts out the territory as they go and can navigate accordingly. SQUID stands for Sequential Question and Insight Diagram.
To continue with our nautical theme, Speed Boat is a short and sweet activity that can help a team quickly identify what employees, clients or service users might have a problem with and analyze what might be standing in the way of achieving a solution.
Methods that allow for a group to make observations, have insights and obtain those eureka moments quickly are invaluable when trying to solve complex problems.
In Speed Boat, the approach is to first consider what anchors and challenges might be holding an organization (or boat) back. Bonus points if you are able to identify any sharks in the water and develop ideas that can also deal with competitors!
Speed Boat #gamestorming #problem solving #action Speedboat is a short and sweet way to identify what your employees or clients don’t like about your product/service or what’s standing in the way of a desired goal.
The Journalistic Six
Some of the most effective ways of solving problems is by encouraging teams to be more inclusive and diverse in their thinking.
Based on the six key questions journalism students are taught to answer in articles and news stories, The Journalistic Six helps create teams to see the whole picture. By using who, what, when, where, why, and how to facilitate the conversation and encourage creative thinking, your team can make sure that the problem identification and problem analysis stages of the are covered exhaustively and thoughtfully. Reporter’s notebook and dictaphone optional.
The Journalistic Six – Who What When Where Why How #idea generation #issue analysis #problem solving #online #creative thinking #remote-friendly A questioning method for generating, explaining, investigating ideas.
Individual and group perspectives are incredibly important, but what happens if people are set in their minds and need a change of perspective in order to approach a problem more effectively?
Flip It is a method we love because it is both simple to understand and run, and allows groups to understand how their perspectives and biases are formed.
Participants in Flip It are first invited to consider concerns, issues, or problems from a perspective of fear and write them on a flip chart. Then, the group is asked to consider those same issues from a perspective of hope and flip their understanding.
No problem and solution is free from existing bias and by changing perspectives with Flip It, you can then develop a problem solving model quickly and effectively.
Flip It! #gamestorming #problem solving #action Often, a change in a problem or situation comes simply from a change in our perspectives. Flip It! is a quick game designed to show players that perspectives are made, not born.
LEGO Challenge
Now for an activity that is a little out of the (toy) box. LEGO Serious Play is a facilitation methodology that can be used to improve creative thinking and problem-solving skills.
The LEGO Challenge includes giving each member of the team an assignment that is hidden from the rest of the group while they create a structure without speaking.
What the LEGO challenge brings to the table is a fun working example of working with stakeholders who might not be on the same page to solve problems. Also, it’s LEGO! Who doesn’t love LEGO!
LEGO Challenge #hyperisland #team A team-building activity in which groups must work together to build a structure out of LEGO, but each individual has a secret “assignment” which makes the collaborative process more challenging. It emphasizes group communication, leadership dynamics, conflict, cooperation, patience and problem solving strategy.
What, So What, Now What?
If not carefully managed, the problem identification and problem analysis stages of the problem-solving process can actually create more problems and misunderstandings.
The What, So What, Now What? problem-solving activity is designed to help collect insights and move forward while also eliminating the possibility of disagreement when it comes to identifying, clarifying, and analyzing organizational or work problems.
Facilitation is all about bringing groups together so that might work on a shared goal and the best problem-solving strategies ensure that teams are aligned in purpose, if not initially in opinion or insight.
Throughout the three steps of this game, you give everyone on a team to reflect on a problem by asking what happened, why it is important, and what actions should then be taken.
This can be a great activity for bringing our individual perceptions about a problem or challenge and contextualizing it in a larger group setting. This is one of the most important problem-solving skills you can bring to your organization.
W³ – What, So What, Now What? #issue analysis #innovation #liberating structures You can help groups reflect on a shared experience in a way that builds understanding and spurs coordinated action while avoiding unproductive conflict. It is possible for every voice to be heard while simultaneously sifting for insights and shaping new direction. Progressing in stages makes this practical—from collecting facts about What Happened to making sense of these facts with So What and finally to what actions logically follow with Now What . The shared progression eliminates most of the misunderstandings that otherwise fuel disagreements about what to do. Voila!
Journalists
Problem analysis can be one of the most important and decisive stages of all problem-solving tools. Sometimes, a team can become bogged down in the details and are unable to move forward.
Journalists is an activity that can avoid a group from getting stuck in the problem identification or problem analysis stages of the process.
In Journalists, the group is invited to draft the front page of a fictional newspaper and figure out what stories deserve to be on the cover and what headlines those stories will have. By reframing how your problems and challenges are approached, you can help a team move productively through the process and be better prepared for the steps to follow.
Journalists #vision #big picture #issue analysis #remote-friendly This is an exercise to use when the group gets stuck in details and struggles to see the big picture. Also good for defining a vision.
Problem-solving techniques for brainstorming solutions
Now you have the context and background of the problem you are trying to solving, now comes the time to start ideating and thinking about how you’ll solve the issue.
Here, you’ll want to encourage creative, free thinking and speed. Get as many ideas out as possible and explore different perspectives so you have the raw material for the next step.
Looking at a problem from a new angle can be one of the most effective ways of creating an effective solution. TRIZ is a problem-solving tool that asks the group to consider what they must not do in order to solve a challenge.
By reversing the discussion, new topics and taboo subjects often emerge, allowing the group to think more deeply and create ideas that confront the status quo in a safe and meaningful way. If you’re working on a problem that you’ve tried to solve before, TRIZ is a great problem-solving method to help your team get unblocked.
Making Space with TRIZ #issue analysis #liberating structures #issue resolution You can clear space for innovation by helping a group let go of what it knows (but rarely admits) limits its success and by inviting creative destruction. TRIZ makes it possible to challenge sacred cows safely and encourages heretical thinking. The question “What must we stop doing to make progress on our deepest purpose?” induces seriously fun yet very courageous conversations. Since laughter often erupts, issues that are otherwise taboo get a chance to be aired and confronted. With creative destruction come opportunities for renewal as local action and innovation rush in to fill the vacuum. Whoosh!
Mindspin
Brainstorming is part of the bread and butter of the problem-solving process and all problem-solving strategies benefit from getting ideas out and challenging a team to generate solutions quickly.
With Mindspin, participants are encouraged not only to generate ideas but to do so under time constraints and by slamming down cards and passing them on. By doing multiple rounds, your team can begin with a free generation of possible solutions before moving on to developing those solutions and encouraging further ideation.
This is one of our favorite problem-solving activities and can be great for keeping the energy up throughout the workshop. Remember the importance of helping people become engaged in the process – energizing problem-solving techniques like Mindspin can help ensure your team stays engaged and happy, even when the problems they’re coming together to solve are complex.
MindSpin #teampedia #idea generation #problem solving #action A fast and loud method to enhance brainstorming within a team. Since this activity has more than round ideas that are repetitive can be ruled out leaving more creative and innovative answers to the challenge.
The Creativity Dice
One of the most useful problem solving skills you can teach your team is of approaching challenges with creativity, flexibility, and openness. Games like The Creativity Dice allow teams to overcome the potential hurdle of too much linear thinking and approach the process with a sense of fun and speed.
In The Creativity Dice, participants are organized around a topic and roll a dice to determine what they will work on for a period of 3 minutes at a time. They might roll a 3 and work on investigating factual information on the chosen topic. They might roll a 1 and work on identifying the specific goals, standards, or criteria for the session.
Encouraging rapid work and iteration while asking participants to be flexible are great skills to cultivate. Having a stage for idea incubation in this game is also important. Moments of pause can help ensure the ideas that are put forward are the most suitable.
The Creativity Dice #creativity #problem solving #thiagi #issue analysis Too much linear thinking is hazardous to creative problem solving. To be creative, you should approach the problem (or the opportunity) from different points of view. You should leave a thought hanging in mid-air and move to another. This skipping around prevents premature closure and lets your brain incubate one line of thought while you consciously pursue another.
Idea and Concept Development
Brainstorming without structure can quickly become chaotic or frustrating. In a problem-solving context, having an ideation framework to follow can help ensure your team is both creative and disciplined.
In this method, you’ll find an idea generation process that encourages your group to brainstorm effectively before developing their ideas and begin clustering them together. By using concepts such as Yes and…, more is more and postponing judgement, you can create the ideal conditions for brainstorming with ease.
Idea & Concept Development #hyperisland #innovation #idea generation Ideation and Concept Development is a process for groups to work creatively and collaboratively to generate creative ideas. It’s a general approach that can be adapted and customized to suit many different scenarios. It includes basic principles for idea generation and several steps for groups to work with. It also includes steps for idea selection and development.
Problem-solving techniques for developing and refining solutions
The success of any problem-solving process can be measured by the solutions it produces. After you’ve defined the issue, explored existing ideas, and ideated, it’s time to develop and refine your ideas in order to bring them closer to a solution that actually solves the problem.
Use these problem-solving techniques when you want to help your team think through their ideas and refine them as part of your problem solving process.
Improved Solutions
After a team has successfully identified a problem and come up with a few solutions, it can be tempting to call the work of the problem-solving process complete. That said, the first solution is not necessarily the best, and by including a further review and reflection activity into your problem-solving model, you can ensure your group reaches the best possible result.
One of a number of problem-solving games from Thiagi Group, Improved Solutions helps you go the extra mile and develop suggested solutions with close consideration and peer review. By supporting the discussion of several problems at once and by shifting team roles throughout, this problem-solving technique is a dynamic way of finding the best solution.
Improved Solutions #creativity #thiagi #problem solving #action #team You can improve any solution by objectively reviewing its strengths and weaknesses and making suitable adjustments. In this creativity framegame, you improve the solutions to several problems. To maintain objective detachment, you deal with a different problem during each of six rounds and assume different roles (problem owner, consultant, basher, booster, enhancer, and evaluator) during each round. At the conclusion of the activity, each player ends up with two solutions to her problem.
Four Step Sketch
Creative thinking and visual ideation does not need to be confined to the opening stages of your problem-solving strategies. Exercises that include sketching and prototyping on paper can be effective at the solution finding and development stage of the process, and can be great for keeping a team engaged.
By going from simple notes to a crazy 8s round that involves rapidly sketching 8 variations on their ideas before then producing a final solution sketch, the group is able to iterate quickly and visually. Problem-solving techniques like Four-Step Sketch are great if you have a group of different thinkers and want to change things up from a more textual or discussion-based approach.
Four-Step Sketch #design sprint #innovation #idea generation #remote-friendly The four-step sketch is an exercise that helps people to create well-formed concepts through a structured process that includes: Review key information Start design work on paper, Consider multiple variations , Create a detailed solution . This exercise is preceded by a set of other activities allowing the group to clarify the challenge they want to solve. See how the Four Step Sketch exercise fits into a Design Sprint
Ensuring that everyone in a group is able to contribute to a discussion is vital during any problem solving process. Not only does this ensure all bases are covered, but its then easier to get buy-in and accountability when people have been able to contribute to the process.
1-2-4-All is a tried and tested facilitation technique where participants are asked to first brainstorm on a topic on their own. Next, they discuss and share ideas in a pair before moving into a small group. Those groups are then asked to present the best idea from their discussion to the rest of the team.
This method can be used in many different contexts effectively, though I find it particularly shines in the idea development stage of the process. Giving each participant time to concretize their ideas and develop them in progressively larger groups can create a great space for both innovation and psychological safety.
1-2-4-All #idea generation #liberating structures #issue analysis With this facilitation technique you can immediately include everyone regardless of how large the group is. You can generate better ideas and more of them faster than ever before. You can tap the know-how and imagination that is distributed widely in places not known in advance. Open, generative conversation unfolds. Ideas and solutions are sifted in rapid fashion. Most importantly, participants own the ideas, so follow-up and implementation is simplified. No buy-in strategies needed! Simple and elegant!
15% Solutions
Some problems are simpler than others and with the right problem-solving activities, you can empower people to take immediate actions that can help create organizational change.
Part of the liberating structures toolkit, 15% solutions is a problem-solving technique that focuses on finding and implementing solutions quickly. A process of iterating and making small changes quickly can help generate momentum and an appetite for solving complex problems.
Problem-solving strategies can live and die on whether people are onboard. Getting some quick wins is a great way of getting people behind the process.
It can be extremely empowering for a team to realize that problem-solving techniques can be deployed quickly and easily and delineate between things they can positively impact and those things they cannot change.
15% Solutions #action #liberating structures #remote-friendly You can reveal the actions, however small, that everyone can do immediately. At a minimum, these will create momentum, and that may make a BIG difference. 15% Solutions show that there is no reason to wait around, feel powerless, or fearful. They help people pick it up a level. They get individuals and the group to focus on what is within their discretion instead of what they cannot change. With a very simple question, you can flip the conversation to what can be done and find solutions to big problems that are often distributed widely in places not known in advance. Shifting a few grains of sand may trigger a landslide and change the whole landscape.
Problem-solving techniques for making decisions and planning
After your group is happy with the possible solutions you’ve developed, now comes the time to choose which to implement. There’s more than one way to make a decision and the best option is often dependant on the needs and set-up of your group.
Sometimes, it’s the case that you’ll want to vote as a group on what is likely to be the most impactful solution. Other times, it might be down to a decision maker or major stakeholder to make the final decision. Whatever your process, here’s some techniques you can use to help you make a decision during your problem solving process.
How-Now-Wow Matrix
The problem-solving process is often creative, as complex problems usually require a change of thinking and creative response in order to find the best solutions. While it’s common for the first stages to encourage creative thinking, groups can often gravitate to familiar solutions when it comes to the end of the process.
When selecting solutions, you don’t want to lose your creative energy! The How-Now-Wow Matrix from Gamestorming is a great problem-solving activity that enables a group to stay creative and think out of the box when it comes to selecting the right solution for a given problem.
Problem-solving techniques that encourage creative thinking and the ideation and selection of new solutions can be the most effective in organisational change. Give the How-Now-Wow Matrix a go, and not just for how pleasant it is to say out loud.
How-Now-Wow Matrix #gamestorming #idea generation #remote-friendly When people want to develop new ideas, they most often think out of the box in the brainstorming or divergent phase. However, when it comes to convergence, people often end up picking ideas that are most familiar to them. This is called a ‘creative paradox’ or a ‘creadox’. The How-Now-Wow matrix is an idea selection tool that breaks the creadox by forcing people to weigh each idea on 2 parameters.
Impact and Effort Matrix
All problem-solving techniques hope to not only find solutions to a given problem or challenge but to find the best solution. When it comes to finding a solution, groups are invited to put on their decision-making hats and really think about how a proposed idea would work in practice.
The Impact and Effort Matrix is one of the problem-solving techniques that fall into this camp, empowering participants to first generate ideas and then categorize them into a 2×2 matrix based on impact and effort.
Activities that invite critical thinking while remaining simple are invaluable. Use the Impact and Effort Matrix to move from ideation and towards evaluating potential solutions before then committing to them.
Impact and Effort Matrix #gamestorming #decision making #action #remote-friendly In this decision-making exercise, possible actions are mapped based on two factors: effort required to implement and potential impact. Categorizing ideas along these lines is a useful technique in decision making, as it obliges contributors to balance and evaluate suggested actions before committing to them.
If you’ve followed each of the problem-solving steps with your group successfully, you should move towards the end of your process with heaps of possible solutions developed with a specific problem in mind. But how do you help a group go from ideation to putting a solution into action?
Dotmocracy – or Dot Voting -is a tried and tested method of helping a team in the problem-solving process make decisions and put actions in place with a degree of oversight and consensus.
One of the problem-solving techniques that should be in every facilitator’s toolbox, Dot Voting is fast and effective and can help identify the most popular and best solutions and help bring a group to a decision effectively.
Dotmocracy #action #decision making #group prioritization #hyperisland #remote-friendly Dotmocracy is a simple method for group prioritization or decision-making. It is not an activity on its own, but a method to use in processes where prioritization or decision-making is the aim. The method supports a group to quickly see which options are most popular or relevant. The options or ideas are written on post-its and stuck up on a wall for the whole group to see. Each person votes for the options they think are the strongest, and that information is used to inform a decision.
Straddling the gap between decision making and planning, MoSCoW is a simple and effective method that allows a group team to easily prioritize a set of possible options.
Use this method in a problem solving process by collecting and summarizing all your possible solutions and then categorize them into 4 sections: “Must have”, “Should have”, “Could have”, or “Would like but won‘t get”.
This method is particularly useful when its less about choosing one possible solution and more about prioritorizing which to do first and which may not fit in the scope of your project. In my experience, complex challenges often require multiple small fixes, and this method can be a great way to move from a pile of things you’d all like to do to a structured plan.
MoSCoW #define intentions #create #design #action #remote-friendly MoSCoW is a method that allows the team to prioritize the different features that they will work on. Features are then categorized into “Must have”, “Should have”, “Could have”, or “Would like but won‘t get”. To be used at the beginning of a timeslot (for example during Sprint planning) and when planning is needed.
When it comes to managing the rollout of a solution, clarity and accountability are key factors in ensuring the success of the project. The RAACI chart is a simple but effective model for setting roles and responsibilities as part of a planning session.
Start by listing each person involved in the project and put them into the following groups in order to make it clear who is responsible for what during the rollout of your solution.
- Responsibility (Which person and/or team will be taking action?)
- Authority (At what “point” must the responsible person check in before going further?)
- Accountability (Who must the responsible person check in with?)
- Consultation (Who must be consulted by the responsible person before decisions are made?)
- Information (Who must be informed of decisions, once made?)
Ensure this information is easily accessible and use it to inform who does what and who is looped into discussions and kept up to date.
RAACI #roles and responsibility #teamwork #project management Clarifying roles and responsibilities, levels of autonomy/latitude in decision making, and levels of engagement among diverse stakeholders.
Problem-solving warm-up activities
All facilitators know that warm-ups and icebreakers are useful for any workshop or group process. Problem-solving workshops are no different.
Use these problem-solving techniques to warm up a group and prepare them for the rest of the process. Activating your group by tapping into some of the top problem-solving skills can be one of the best ways to see great outcomes from your session.
Check-in / Check-out
Solid processes are planned from beginning to end, and the best facilitators know that setting the tone and establishing a safe, open environment can be integral to a successful problem-solving process. Check-in / Check-out is a great way to begin and/or bookend a problem-solving workshop. Checking in to a session emphasizes that everyone will be seen, heard, and expected to contribute.
If you are running a series of meetings, setting a consistent pattern of checking in and checking out can really help your team get into a groove. We recommend this opening-closing activity for small to medium-sized groups though it can work with large groups if they’re disciplined!
Check-in / Check-out #team #opening #closing #hyperisland #remote-friendly Either checking-in or checking-out is a simple way for a team to open or close a process, symbolically and in a collaborative way. Checking-in/out invites each member in a group to be present, seen and heard, and to express a reflection or a feeling. Checking-in emphasizes presence, focus and group commitment; checking-out emphasizes reflection and symbolic closure.
Doodling Together
Thinking creatively and not being afraid to make suggestions are important problem-solving skills for any group or team, and warming up by encouraging these behaviors is a great way to start.
Doodling Together is one of our favorite creative ice breaker games – it’s quick, effective, and fun and can make all following problem-solving steps easier by encouraging a group to collaborate visually. By passing cards and adding additional items as they go, the workshop group gets into a groove of co-creation and idea development that is crucial to finding solutions to problems.
Doodling Together #collaboration #creativity #teamwork #fun #team #visual methods #energiser #icebreaker #remote-friendly Create wild, weird and often funny postcards together & establish a group’s creative confidence.
Show and Tell
You might remember some version of Show and Tell from being a kid in school and it’s a great problem-solving activity to kick off a session.
Asking participants to prepare a little something before a workshop by bringing an object for show and tell can help them warm up before the session has even begun! Games that include a physical object can also help encourage early engagement before moving onto more big-picture thinking.
By asking your participants to tell stories about why they chose to bring a particular item to the group, you can help teams see things from new perspectives and see both differences and similarities in the way they approach a topic. Great groundwork for approaching a problem-solving process as a team!
Show and Tell #gamestorming #action #opening #meeting facilitation Show and Tell taps into the power of metaphors to reveal players’ underlying assumptions and associations around a topic The aim of the game is to get a deeper understanding of stakeholders’ perspectives on anything—a new project, an organizational restructuring, a shift in the company’s vision or team dynamic.
Constellations
Who doesn’t love stars? Constellations is a great warm-up activity for any workshop as it gets people up off their feet, energized, and ready to engage in new ways with established topics. It’s also great for showing existing beliefs, biases, and patterns that can come into play as part of your session.
Using warm-up games that help build trust and connection while also allowing for non-verbal responses can be great for easing people into the problem-solving process and encouraging engagement from everyone in the group. Constellations is great in large spaces that allow for movement and is definitely a practical exercise to allow the group to see patterns that are otherwise invisible.
Constellations #trust #connection #opening #coaching #patterns #system Individuals express their response to a statement or idea by standing closer or further from a central object. Used with teams to reveal system, hidden patterns, perspectives.
Draw a Tree
Problem-solving games that help raise group awareness through a central, unifying metaphor can be effective ways to warm-up a group in any problem-solving model.
Draw a Tree is a simple warm-up activity you can use in any group and which can provide a quick jolt of energy. Start by asking your participants to draw a tree in just 45 seconds – they can choose whether it will be abstract or realistic.
Once the timer is up, ask the group how many people included the roots of the tree and use this as a means to discuss how we can ignore important parts of any system simply because they are not visible.
All problem-solving strategies are made more effective by thinking of problems critically and by exposing things that may not normally come to light. Warm-up games like Draw a Tree are great in that they quickly demonstrate some key problem-solving skills in an accessible and effective way.
Draw a Tree #thiagi #opening #perspectives #remote-friendly With this game you can raise awarness about being more mindful, and aware of the environment we live in.
Closing activities for a problem-solving process
Each step of the problem-solving workshop benefits from an intelligent deployment of activities, games, and techniques. Bringing your session to an effective close helps ensure that solutions are followed through on and that you also celebrate what has been achieved.
Here are some problem-solving activities you can use to effectively close a workshop or meeting and ensure the great work you’ve done can continue afterward.
One Breath Feedback
Maintaining attention and focus during the closing stages of a problem-solving workshop can be tricky and so being concise when giving feedback can be important. It’s easy to incur “death by feedback” should some team members go on for too long sharing their perspectives in a quick feedback round.
One Breath Feedback is a great closing activity for workshops. You give everyone an opportunity to provide feedback on what they’ve done but only in the space of a single breath. This keeps feedback short and to the point and means that everyone is encouraged to provide the most important piece of feedback to them.
One breath feedback #closing #feedback #action This is a feedback round in just one breath that excels in maintaining attention: each participants is able to speak during just one breath … for most people that’s around 20 to 25 seconds … unless of course you’ve been a deep sea diver in which case you’ll be able to do it for longer.
Who What When Matrix
Matrices feature as part of many effective problem-solving strategies and with good reason. They are easily recognizable, simple to use, and generate results.
The Who What When Matrix is a great tool to use when closing your problem-solving session by attributing a who, what and when to the actions and solutions you have decided upon. The resulting matrix is a simple, easy-to-follow way of ensuring your team can move forward.
Great solutions can’t be enacted without action and ownership. Your problem-solving process should include a stage for allocating tasks to individuals or teams and creating a realistic timeframe for those solutions to be implemented or checked out. Use this method to keep the solution implementation process clear and simple for all involved.
Who/What/When Matrix #gamestorming #action #project planning With Who/What/When matrix, you can connect people with clear actions they have defined and have committed to.
Response cards
Group discussion can comprise the bulk of most problem-solving activities and by the end of the process, you might find that your team is talked out!
Providing a means for your team to give feedback with short written notes can ensure everyone is head and can contribute without the need to stand up and talk. Depending on the needs of the group, giving an alternative can help ensure everyone can contribute to your problem-solving model in the way that makes the most sense for them.
Response Cards is a great way to close a workshop if you are looking for a gentle warm-down and want to get some swift discussion around some of the feedback that is raised.
Response Cards #debriefing #closing #structured sharing #questions and answers #thiagi #action It can be hard to involve everyone during a closing of a session. Some might stay in the background or get unheard because of louder participants. However, with the use of Response Cards, everyone will be involved in providing feedback or clarify questions at the end of a session.
Tips for effective problem solving
Problem-solving activities are only one part of the puzzle. While a great method can help unlock your team’s ability to solve problems, without a thoughtful approach and strong facilitation the solutions may not be fit for purpose.
Let’s take a look at some problem-solving tips you can apply to any process to help it be a success!
Clearly define the problem
Jumping straight to solutions can be tempting, though without first clearly articulating a problem, the solution might not be the right one. Many of the problem-solving activities below include sections where the problem is explored and clearly defined before moving on.
This is a vital part of the problem-solving process and taking the time to fully define an issue can save time and effort later. A clear definition helps identify irrelevant information and it also ensures that your team sets off on the right track.
Don’t jump to conclusions
It’s easy for groups to exhibit cognitive bias or have preconceived ideas about both problems and potential solutions. Be sure to back up any problem statements or potential solutions with facts, research, and adequate forethought.
The best techniques ask participants to be methodical and challenge preconceived notions. Make sure you give the group enough time and space to collect relevant information and consider the problem in a new way. By approaching the process with a clear, rational mindset, you’ll often find that better solutions are more forthcoming.
Try different approaches
Problems come in all shapes and sizes and so too should the methods you use to solve them. If you find that one approach isn’t yielding results and your team isn’t finding different solutions, try mixing it up. You’ll be surprised at how using a new creative activity can unblock your team and generate great solutions.
Don’t take it personally
Depending on the nature of your team or organizational problems, it’s easy for conversations to get heated. While it’s good for participants to be engaged in the discussions, ensure that emotions don’t run too high and that blame isn’t thrown around while finding solutions.
You’re all in it together, and even if your team or area is seeing problems, that isn’t necessarily a disparagement of you personally. Using facilitation skills to manage group dynamics is one effective method of helping conversations be more constructive.
Get the right people in the room
Your problem-solving method is often only as effective as the group using it. Getting the right people on the job and managing the number of people present is important too!
If the group is too small, you may not get enough different perspectives to effectively solve a problem. If the group is too large, you can go round and round during the ideation stages.
Creating the right group makeup is also important in ensuring you have the necessary expertise and skillset to both identify and follow up on potential solutions. Carefully consider who to include at each stage to help ensure your problem-solving method is followed and positioned for success.
Create psychologically safe spaces for discussion
Identifying a problem accurately also requires that all members of a group are able to contribute their views in an open and safe manner.
It can be tough for people to stand up and contribute if the problems or challenges are emotive or personal in nature. Try and create a psychologically safe space for these kinds of discussions and where possible, create regular opportunities for challenges to be brought up organically.
Document everything
The best solutions can take refinement, iteration, and reflection to come out. Get into a habit of documenting your process in order to keep all the learnings from the session and to allow ideas to mature and develop. Many of the methods below involve the creation of documents or shared resources. Be sure to keep and share these so everyone can benefit from the work done!
Bring a facilitator
Facilitation is all about making group processes easier. With a subject as potentially emotive and important as problem-solving, having an impartial third party in the form of a facilitator can make all the difference in finding great solutions and keeping the process moving. Consider bringing a facilitator to your problem-solving session to get better results and generate meaningful solutions!
Develop your problem-solving skills
It takes time and practice to be an effective problem solver. While some roles or participants might more naturally gravitate towards problem-solving, it can take development and planning to help everyone create better solutions.
You might develop a training program, run a problem-solving workshop or simply ask your team to practice using the techniques below. Check out our post on problem-solving skills to see how you and your group can develop the right mental process and be more resilient to issues too!
Design a great agenda
Workshops are a great format for solving problems. With the right approach, you can focus a group and help them find the solutions to their own problems. But designing a process can be time-consuming and finding the right activities can be difficult.
Check out our workshop planning guide to level-up your agenda design and start running more effective workshops. Need inspiration? Check out templates designed by expert facilitators to help you kickstart your process!
Save time and effort creating an effective problem solving process
A structured problem solving process is a surefire way of solving tough problems, discovering creative solutions and driving organizational change. But how can you design for successful outcomes?
With SessionLab, it’s easy to design engaging workshops that deliver results. Drag, drop and reorder blocks to build your agenda. When you make changes or update your agenda, your session timing adjusts automatically , saving you time on manual adjustments.
Collaborating with stakeholders or clients? Share your agenda with a single click and collaborate in real-time. No more sending documents back and forth over email.
Explore how to use SessionLab to design effective problem solving workshops or watch this five minute video to see the planner in action!
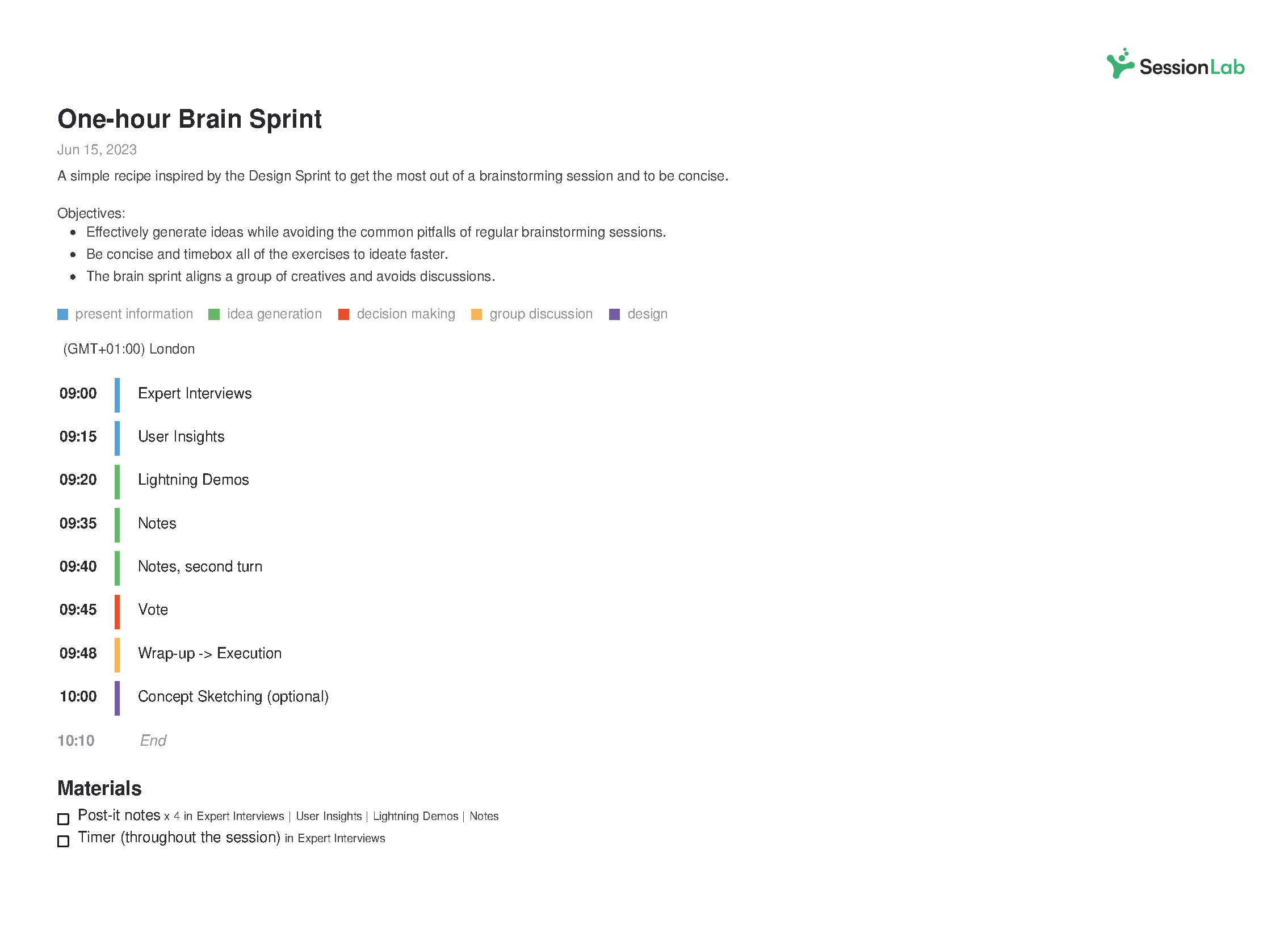
Over to you
The problem-solving process can often be as complicated and multifaceted as the problems they are set-up to solve. With the right problem-solving techniques and a mix of exercises designed to guide discussion and generate purposeful ideas, we hope we’ve given you the tools to find the best solutions as simply and easily as possible.
Is there a problem-solving technique that you are missing here? Do you have a favorite activity or method you use when facilitating? Let us know in the comments below, we’d love to hear from you!
James Smart is Head of Content at SessionLab. He’s also a creative facilitator who has run workshops and designed courses for establishments like the National Centre for Writing, UK. He especially enjoys working with young people and empowering others in their creative practice.
thank you very much for these excellent techniques
Certainly wonderful article, very detailed. Shared!
Your list of techniques for problem solving can be helpfully extended by adding TRIZ to the list of techniques. TRIZ has 40 problem solving techniques derived from methods inventros and patent holders used to get new patents. About 10-12 are general approaches. many organization sponsor classes in TRIZ that are used to solve business problems or general organiztational problems. You can take a look at TRIZ and dwonload a free internet booklet to see if you feel it shound be included per your selection process.
Leave a Comment Cancel reply
Your email address will not be published. Required fields are marked *
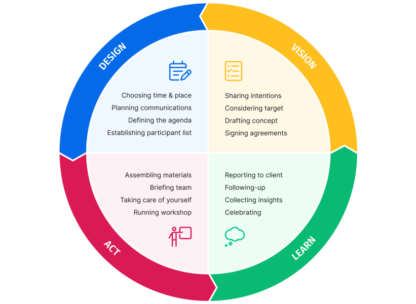
Going from a mere idea to a workshop that delivers results for your clients can feel like a daunting task. In this piece, we will shine a light on all the work behind the scenes and help you learn how to plan a workshop from start to finish. On a good day, facilitation can feel like effortless magic, but that is mostly the result of backstage work, foresight, and a lot of careful planning. Read on to learn a step-by-step approach to breaking the process of planning a workshop into small, manageable chunks. The flow starts with the first meeting with a client to define the purposes of a workshop.…
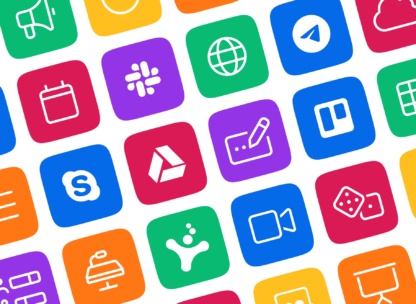
Effective online tools are a necessity for smooth and engaging virtual workshops and meetings. But how do you choose the right ones? Do you sometimes feel that the good old pen and paper or MS Office toolkit and email leaves you struggling to stay on top of managing and delivering your workshop? Fortunately, there are plenty of great workshop tools to make your life easier when you need to facilitate a meeting and lead workshops. In this post, we’ll share our favorite online tools you can use to make your life easier and run better workshops and meetings. In fact, there are plenty of free online workshop tools and meeting…
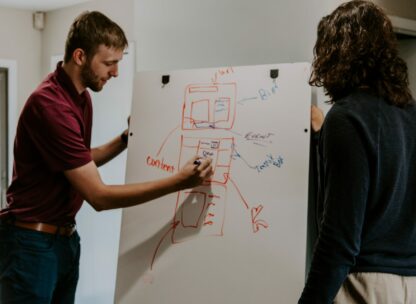
How does learning work? A clever 9-year-old once told me: “I know I am learning something new when I am surprised.” The science of adult learning tells us that, in order to learn new skills (which, unsurprisingly, is harder for adults to do than kids) grown-ups need to first get into a specific headspace. In a business, this approach is often employed in a training session where employees learn new skills or work on professional development. But how do you ensure your training is effective? In this guide, we'll explore how to create an effective training session plan and run engaging training sessions. As team leader, project manager, or consultant,…
Design your next workshop with SessionLab
Join the 150,000 facilitators using SessionLab
Sign up for free

What is Problem Solving? (Steps, Techniques, Examples)
What is problem solving, definition and importance.
Problem solving is the process of finding solutions to obstacles or challenges you encounter in your life or work. It is a crucial skill that allows you to tackle complex situations, adapt to changes, and overcome difficulties with ease. Mastering this ability will contribute to both your personal and professional growth, leading to more successful outcomes and better decision-making.
Problem-Solving Steps
The problem-solving process typically includes the following steps:
- Identify the issue : Recognize the problem that needs to be solved.
- Analyze the situation : Examine the issue in depth, gather all relevant information, and consider any limitations or constraints that may be present.
- Generate potential solutions : Brainstorm a list of possible solutions to the issue, without immediately judging or evaluating them.
- Evaluate options : Weigh the pros and cons of each potential solution, considering factors such as feasibility, effectiveness, and potential risks.
- Select the best solution : Choose the option that best addresses the problem and aligns with your objectives.
- Implement the solution : Put the selected solution into action and monitor the results to ensure it resolves the issue.
- Review and learn : Reflect on the problem-solving process, identify any improvements or adjustments that can be made, and apply these learnings to future situations.
Defining the Problem
To start tackling a problem, first, identify and understand it. Analyzing the issue thoroughly helps to clarify its scope and nature. Ask questions to gather information and consider the problem from various angles. Some strategies to define the problem include:
- Brainstorming with others
- Asking the 5 Ws and 1 H (Who, What, When, Where, Why, and How)
- Analyzing cause and effect
- Creating a problem statement
Generating Solutions
Once the problem is clearly understood, brainstorm possible solutions. Think creatively and keep an open mind, as well as considering lessons from past experiences. Consider:
- Creating a list of potential ideas to solve the problem
- Grouping and categorizing similar solutions
- Prioritizing potential solutions based on feasibility, cost, and resources required
- Involving others to share diverse opinions and inputs
Evaluating and Selecting Solutions
Evaluate each potential solution, weighing its pros and cons. To facilitate decision-making, use techniques such as:
- SWOT analysis (Strengths, Weaknesses, Opportunities, Threats)
- Decision-making matrices
- Pros and cons lists
- Risk assessments
After evaluating, choose the most suitable solution based on effectiveness, cost, and time constraints.
Implementing and Monitoring the Solution
Implement the chosen solution and monitor its progress. Key actions include:
- Communicating the solution to relevant parties
- Setting timelines and milestones
- Assigning tasks and responsibilities
- Monitoring the solution and making adjustments as necessary
- Evaluating the effectiveness of the solution after implementation
Utilize feedback from stakeholders and consider potential improvements. Remember that problem-solving is an ongoing process that can always be refined and enhanced.
Problem-Solving Techniques
During each step, you may find it helpful to utilize various problem-solving techniques, such as:
- Brainstorming : A free-flowing, open-minded session where ideas are generated and listed without judgment, to encourage creativity and innovative thinking.
- Root cause analysis : A method that explores the underlying causes of a problem to find the most effective solution rather than addressing superficial symptoms.
- SWOT analysis : A tool used to evaluate the strengths, weaknesses, opportunities, and threats related to a problem or decision, providing a comprehensive view of the situation.
- Mind mapping : A visual technique that uses diagrams to organize and connect ideas, helping to identify patterns, relationships, and possible solutions.
Brainstorming
When facing a problem, start by conducting a brainstorming session. Gather your team and encourage an open discussion where everyone contributes ideas, no matter how outlandish they may seem. This helps you:
- Generate a diverse range of solutions
- Encourage all team members to participate
- Foster creative thinking
When brainstorming, remember to:
- Reserve judgment until the session is over
- Encourage wild ideas
- Combine and improve upon ideas
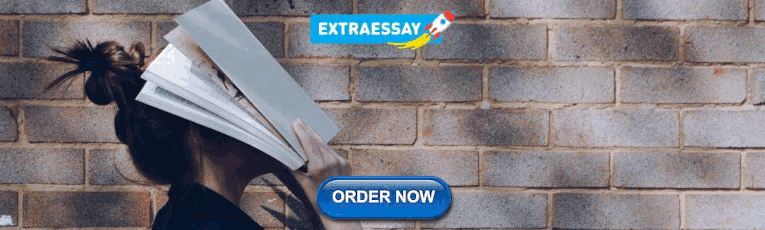
Root Cause Analysis
For effective problem-solving, identifying the root cause of the issue at hand is crucial. Try these methods:
- 5 Whys : Ask “why” five times to get to the underlying cause.
- Fishbone Diagram : Create a diagram representing the problem and break it down into categories of potential causes.
- Pareto Analysis : Determine the few most significant causes underlying the majority of problems.
SWOT Analysis
SWOT analysis helps you examine the Strengths, Weaknesses, Opportunities, and Threats related to your problem. To perform a SWOT analysis:
- List your problem’s strengths, such as relevant resources or strong partnerships.
- Identify its weaknesses, such as knowledge gaps or limited resources.
- Explore opportunities, like trends or new technologies, that could help solve the problem.
- Recognize potential threats, like competition or regulatory barriers.
SWOT analysis aids in understanding the internal and external factors affecting the problem, which can help guide your solution.
Mind Mapping
A mind map is a visual representation of your problem and potential solutions. It enables you to organize information in a structured and intuitive manner. To create a mind map:
- Write the problem in the center of a blank page.
- Draw branches from the central problem to related sub-problems or contributing factors.
- Add more branches to represent potential solutions or further ideas.
Mind mapping allows you to visually see connections between ideas and promotes creativity in problem-solving.
Examples of Problem Solving in Various Contexts
In the business world, you might encounter problems related to finances, operations, or communication. Applying problem-solving skills in these situations could look like:
- Identifying areas of improvement in your company’s financial performance and implementing cost-saving measures
- Resolving internal conflicts among team members by listening and understanding different perspectives, then proposing and negotiating solutions
- Streamlining a process for better productivity by removing redundancies, automating tasks, or re-allocating resources
In educational contexts, problem-solving can be seen in various aspects, such as:
- Addressing a gap in students’ understanding by employing diverse teaching methods to cater to different learning styles
- Developing a strategy for successful time management to balance academic responsibilities and extracurricular activities
- Seeking resources and support to provide equal opportunities for learners with special needs or disabilities
Everyday life is full of challenges that require problem-solving skills. Some examples include:
- Overcoming a personal obstacle, such as improving your fitness level, by establishing achievable goals, measuring progress, and adjusting your approach accordingly
- Navigating a new environment or city by researching your surroundings, asking for directions, or using technology like GPS to guide you
- Dealing with a sudden change, like a change in your work schedule, by assessing the situation, identifying potential impacts, and adapting your plans to accommodate the change.
- How to Resolve Employee Conflict at Work [Steps, Tips, Examples]
- How to Write Inspiring Core Values? 5 Steps with Examples
- 30 Employee Feedback Examples (Positive & Negative)
A3 8 Step Practical Problem Solving – Skill Level 1: Knowledge
Process – what are key aspects to a3 practical problem solving.
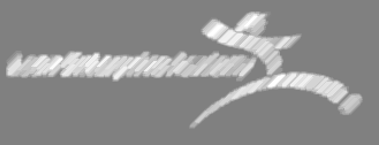
Process Teach Point Video
Watch Video in full screen mode when possible
Key Learning Points
Key Learning Points from Video
1 – “Go and See” at the workplace – grasp the real situation.
2 – Follow Structured Scientific Method – not guessing. In Practical Problem Solving it is 8 Steps.
3 – Follow Plan – Do – Check – Act an approach of rapid trials and tests to prove out theories and learn.
4 – Take a step towards where we ultimately want to be (One problem at a time.)
The fundamental thinking basis for Problem Solving is Plan-Do-Check-Act (PDCA)
Problem Solving Framework
Types of Problems
Not one size or problem solving approach fits all as there are different Types of Problems.
Art Smalley’s Book describes Four Main Types of Problems as:
Type 1 = Troubleshooting
Type 2 = Gap from Sta ndard
Type 3 = Target Condition
Type 4 = Open Ended
If we understand the types of problems, we stand a better chance of applying an appropriate process to “solving” them. Thus we avoid the issues in the famous quote from Abraham Maslow, “I suppose it is tempting, if the only tool you have is a hammer, to treat everything as if it were a nail.”
Most business problems fall into four main categories (see the diagram below), each requiring different thought processes, improvement methods, and management cadences:
Before using a method to solve a problem you need to understand what type of problem you are trying to solve.
Need to consider the Type of Problems, their Properties, the Analysis required and Level in the organisation.
Otherwise you waste time and resource.
Problem Solving Methods
Reacting to an unexpected event – For Type 1 Troubleshooting
Typically lots of them in the day, easy to fix quickly and you don’t need to analyse them so much.
Like changing a flat tyre or putting out a fire, you just fix it now! Everyone can do this. Like reacting to Andon cord pulls on a moving assembly line.
A 4 Step approach based upon the fundamentals of: Concern, Contain, Cause, Countermeasure & Confirm to tackle one off or step change type gaps in the workplace (Type 2 & 3).
Generally taught by functional and team leaders to natural work teams
Problem solving thinking captured on a quadrant chart. Uses the 7 Problem Solving tools to support fast but structured approach to problem solving, designed for first line leaders – not experts or after extensive training. Simple visual tools are used to capture the thinking.
An 8 step problem solving approach taking the Rapid method further. For current and longer term business issues requiring more analysis to get to the root causes.
For Type 2 & 3 mainly although thinking way helps considerably with Type 4 problems.
Some problems need a little more time and deeper thinking in order to solve them. The root causes and countermeasures are not that straight forward or immediately obvious as in Rapid for example. Uses the 7 Problem Solving tools to support logical thinking.
Performed by Leaders and functional Specialists typically. A3’s are used to summarise the thinking, capture the story and share the learning.
React, Rapid and Practical can be used to tackle most problems encountered within the organisation ~ >90%.
Used for innovative problem solving to seek out new solutions. Achieves radical improvement, often a new product, process, system or value for the customer well beyond current levels.
Many different methods can be used but usually technical or specialist knowledge is required. These are infrequent, hard problems to solve typically.
For use on problems often with multiple drivers in play. Experimentation may be needed to learn and understand what is happening and what is needed.
Can take some time and deep analysis but the results can be a step change in performance.
“Organisations and individuals at all levels fall into this trap of having one primary or standard way of solving every problem,” said Smalley, who learned problem solving at Toyota’s historic Kamigo engine plant from Harada-san.
Why Start with Practical Problem Solving ?
- Practical Problem Solving is a proven method based on Toyota’s 8 Step approach for developing people to solve problems.
- Practical Problem Solving enables Managers, Team leaders and Functional Specialists etc. to tackle most medium and mid complexity problems for their current and near term business challenges in their organisations.
- Once you have achieved level 3 capable skill in Practical Problem Solving, you should then be capable of the using the Rapid Problem Solving methods to work on the many small problems.
In the “Skill Level 2” course you will gain a deep Understanding of A3 8 Step Practical Problem Solving and learn how to visualise your problem solving story onto an A3 sized document.
Now move onto the next Topic .
Best Practices
Top 5 ways to practice problem-solving, practice problem-solving in your daily life. use our handy guide to improve this crucial workplace skill and boost your professional performance..
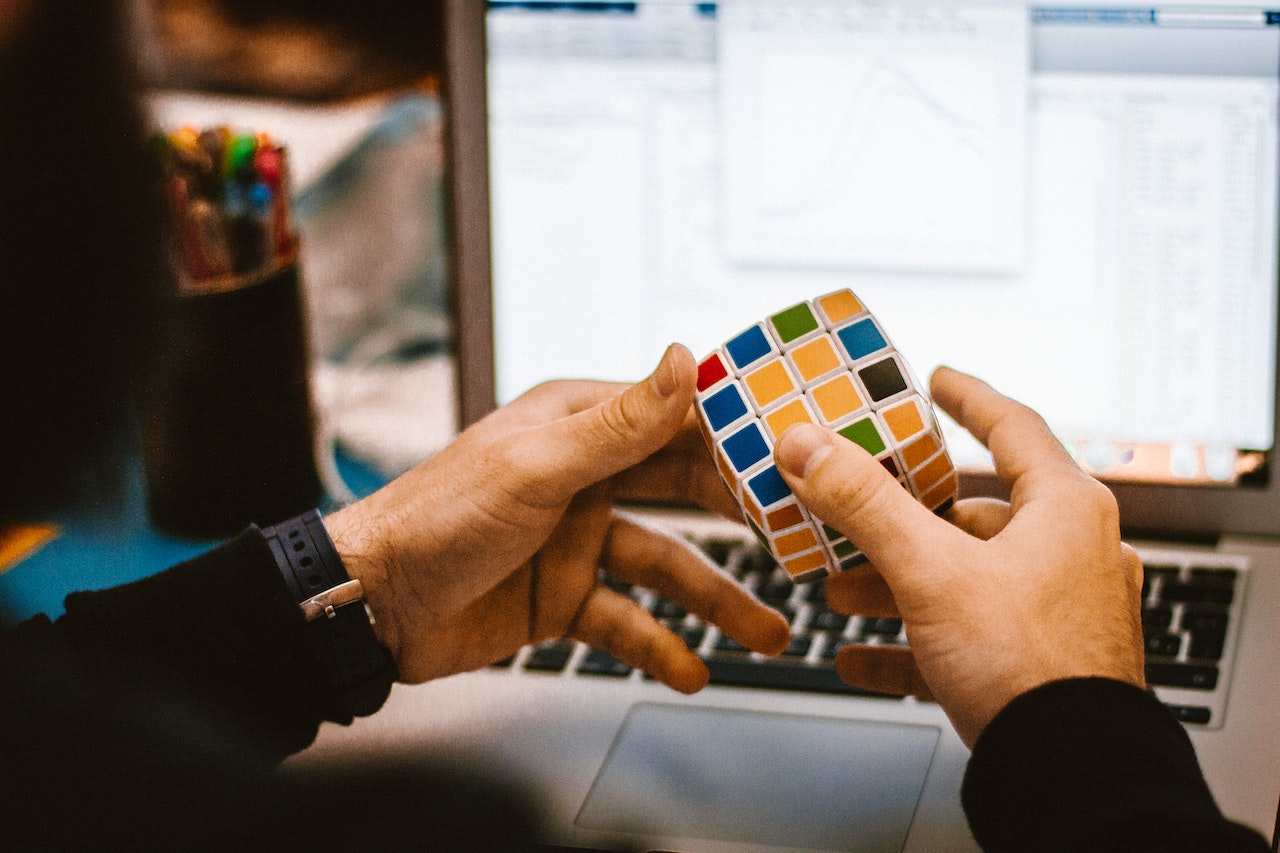
Problem-solving is something we do every day. From deciding what to make for dinner when we haven’t grocery shopped to figuring out how to increase our sales by 30% by the end of the month, we constantly run into obstacles in our personal and professional lives.
No one is a master problem-solver right away — it takes patience and time to develop this skill. Practicing problem-solving in low-stakes situations prepares us for when it really counts: a high-pressure setting. Luckily, we know the best ways to practice problem-solving, how to develop your problem-solving skills, and strategies for solving problems quickly and practically.
What is problem-solving?
We can break down the problem-solving process into three main parts: identifying a problem, brainstorming solutions, and taking action. Like any other skill, this process becomes easier with practice. Learning actionable problem-solving techniques can also help us to respond to difficult situations quickly and effectively.
Problem-solving skills are a great help in the workplace — especially for business owners responsible for running all aspects of a business. These skills empower us in both our professional and personal lives and give us the independence to tackle roadblocks on our own — a great asset for solopreneurs.
How to develop problem-solving skills
Practicing problem-solving doesn’t have to be a chore. Here are five exciting ways to flex your creativity and problem-solving muscles:
1. Puzzles and brainteasers
These are fantastic ways to exercise our brains in a controlled and relaxed environment. When we have ample time and no deadlines to brainstorm potential solutions, we’re more likely to try new methods, experiment, and find the problem-solving strategy that works best for us.
Practicing this skill can be as simple as picking up a book of sudoku or sitting down for a crossword every week. Choose activities that you enjoy and make you excited to learn.
Board games and video games provide both entertainment and complex problems to tackle. While immersed in the world or storyline of the game, we’re often required to overcome challenges by discovering a step-by-step journey to the solutions.
Strategy games in particular can strengthen our ability to plan and adapt to sudden changes. Because these games are just for fun, we can break down the problem with patience and take as many attempts as we need.
Starting a new hobby, like a craft or sport, encourages us to embrace a beginner’s mind and find creative solutions while we learn. Whether it’s knitting or basketball, each new journey is going to have hiccups we must persevere through and overcome.
4. Case studies
If games and puzzles seem a little too abstract to help with professional problem-solving, reading case studies is a powerful alternative. Many case studies published by companies and authors break down the minutiae of problem-solving in real-world settings, including the stress and deadlines of an office environment .
By working through these cases from the comfort of our homes, we learn to consider problems from new perspectives and build the know-how to replicate these strategies — even under stress.
{{mid-cta-product}}
Mastering problem-solving
Once we feel comfortable practicing our problem-solving, it’s time to put our skills into action to tackle real-world problems. Here are four ways you can work more quickly and efficiently in high-pressure situations:
- Make sure you understand the problem and can explain it to others. The first step to solving a problem is knowing it inside and out, and an excellent way to test this is by explaining it to peers or team members.
- Lose the fear of making mistakes. The best solution is the one that works most quickly and effectively for you. There’s no “wrong” answer, and there are likely many viable strategies you could choose.
- Use visual techniques. Good problem solvers understand the methods that work best for them. If staring at words on a page isn’t working, try visualizing the problem through diagrams, mind maps, and other visual tools.
- Reframe the problem as a question you can answer. Thinking of problems as questions allows you to focus on their answers. For example, turn “My clients are upset we don’t communicate enough” into “How can I improve communication with my clients?”
Problem-solving strategies
If you’re looking to really level up your problem-solving, we have three strategies you can implement.
1. IDEAL problem solving
This 5-step process is easy to remember because it’s based on the acronym IDEAL:
- Identify the problem at hand. Ensure you understand the scope of the problem, the facts you already have, and what you need to find out.
- Define an outcome. What does a successful solution look like? Outline an objective that’s agreed upon by you and any other parties involved.
- Explore strategies. This step is where diagrams, mind maps, and brainstorming can come into play. It doesn’t matter how far-fetched an idea seems — write it down anyway.
- Anticipate outcomes. Examine your best strategies and make your best guess at what the outcome of each will look like. Pick a solution from your list based on which you think will be most successful. You can never know for certain what will happen, but you must take a step forward to move the process along. If you fail, turn to your next best strategy.
- Look back and learn. Reflection is crucial to becoming better at problem-solving. After you make a decision and act on it, reflect on the journey and the outcome. Did you achieve what you wanted to? Would another strategy have been more efficient?
2. Practical problem-solving
This 8-step method centers around solving problems in a team setting, though it can be adapted to individual use:
- Clarify the problem . Ensure all your team members are on the same page by discussing the problem and landing on a definition.
- Break down the problem. If a problem is complex, break it down into more manageable sections. This way, you can employ strategies for each facet of the problem rather than getting stuck on the big picture. You and your team are less likely to become discouraged if you can celebrate successes along the way.
- Set a target. Agree on an end goal and deadline for solving the problem. This will keep everyone accountable to the same solution.
- Analyze the source of the problem. Determine the crux of the problem by tracing back to its root cause. Try using a mind map and beginning at the root of issue and tracing backward.
- Develop countermeasures to correct the problem. Brainstorm solutions with your peers and weigh what the most effective methods might be. Like the IDEAL strategy, this is the time to anticipate the outcomes of your solutions and choose the best step forward.
- Act on the countermeasures. Once you’ve chosen your measures, it’s time to implement them. Delegate tasks amongst your team and offer each other support.
- Monitor your progress. Track your team’s progress with milestones or metrics so everyone’s on the same page about the problem’s status.
- Set a standard and share. How often should team members check in? Set clear expectations and ensure the team conducts regular follow-ups to keep everyone updated on the project's progress — and, of course, when the problem is solved.
3. Lightning Decision Jam
This 9-step process focuses on speed and aims to efficiently generate a variety of solutions to a group of problems. The steps are quick and snappy:
- Write down your problems. What issues are you looking to solve?
- Present each problem to the team (if you’re not working alone). Outline a concise overview of each problem.
- Vote on what problems are priorities (or rank them yourself). Number your problems based on urgency and importance.
- Rewrite priority problems as challenges. Translate your top problems into concrete challenges you or your team must overcome. What are the causes and who do they affect?
- Brainstorm solutions. Have a rapid-fire session and write everything down — there are no wrong answers.
- Vote for the best solutions (or rank them yourself). What solutions from your brainstorming are the most practical, efficient, and promising?
- Prioritize the most relevant solutions. Among the popular solutions, which are most relevant? Weigh effort versus impact and consider your individual or team strengths.
- Decide what to act on first. Tackle the easiest tasks with the highest impact first, then work your way to the most difficult actions that will be the least impactful.
- Delegate actions and tasks (if you’re not working alone). You know what you have to do and how to get there, so assign work based on your team’s skillset. Who is the best fit for each task?
The best time to start is now
Unfortunately, there’s no recipe for solving every problem you come across. But practicing your problem-solving will unearth your strengths and weaknesses and teach you the necessary techniques to tackle whatever difficulties come your way head-on. So grab a puzzle, dust off a game, or turn your attention to that issue at work — it’s time to get problem-solving.
Excellent communication is a crucial component of effective problem-solving. Try Practice’s all-in-one client management system today to streamline client conversations and give you back some time to focus on tackling client and business complications.
Give your clients a simple and professional experience
Simple client management designed for teams, free form templates from practice.
Start your business coaching journey with this comprehensive New Client Intake Form. Get the insight and clarity you need to make the best decisions for your clients!
This Post Session Reflection Form Template will help professionals accurately reflect on their sessions and help their clients develop further. Try it today!
Take your coaching to the next level with our professional Coaching Discovery Call Form Template. Get your free copy today!
More articles from Practice
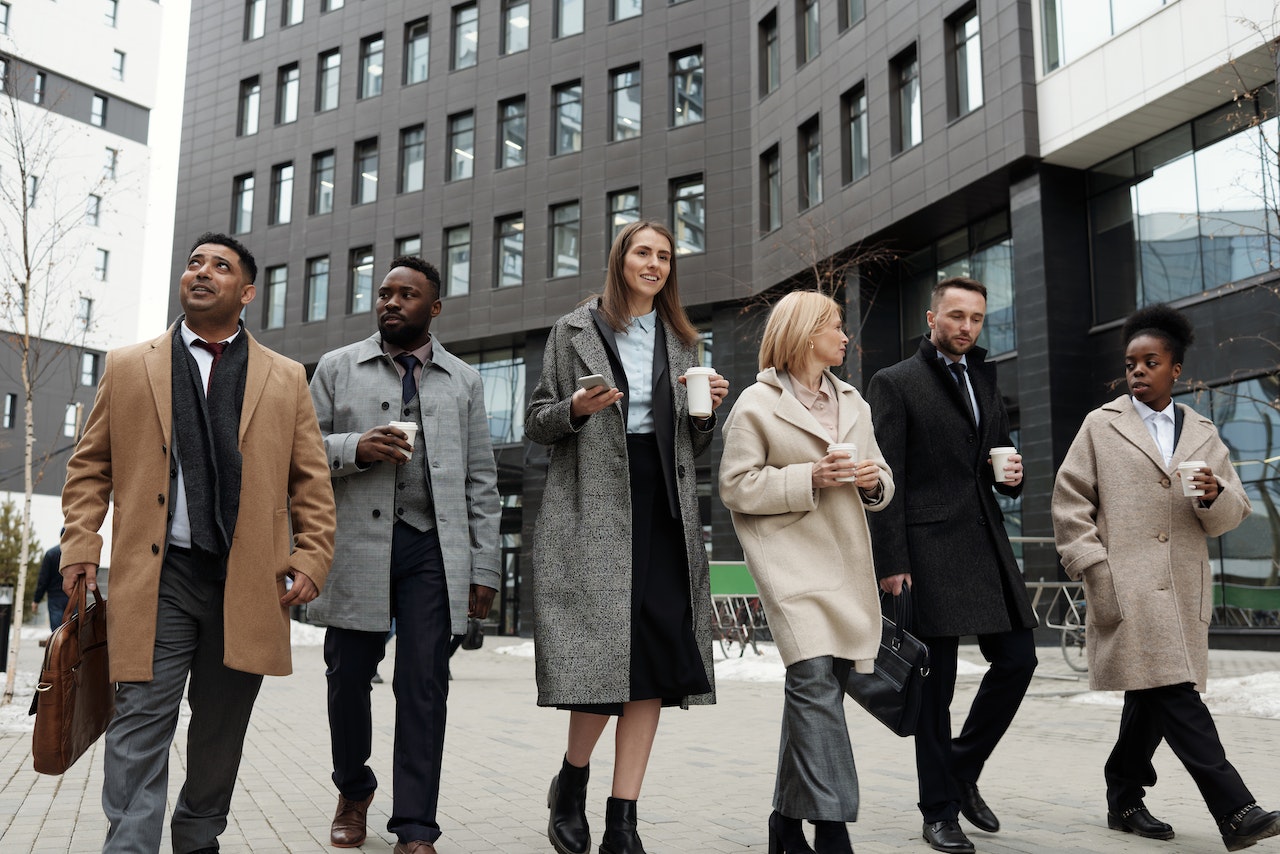
How Leaders Can Use Coaching to Improve Performance

4 Tips to Practice Effective, Professional Communication

5 Tips to Start Coaching Your Team for Performance Improvement
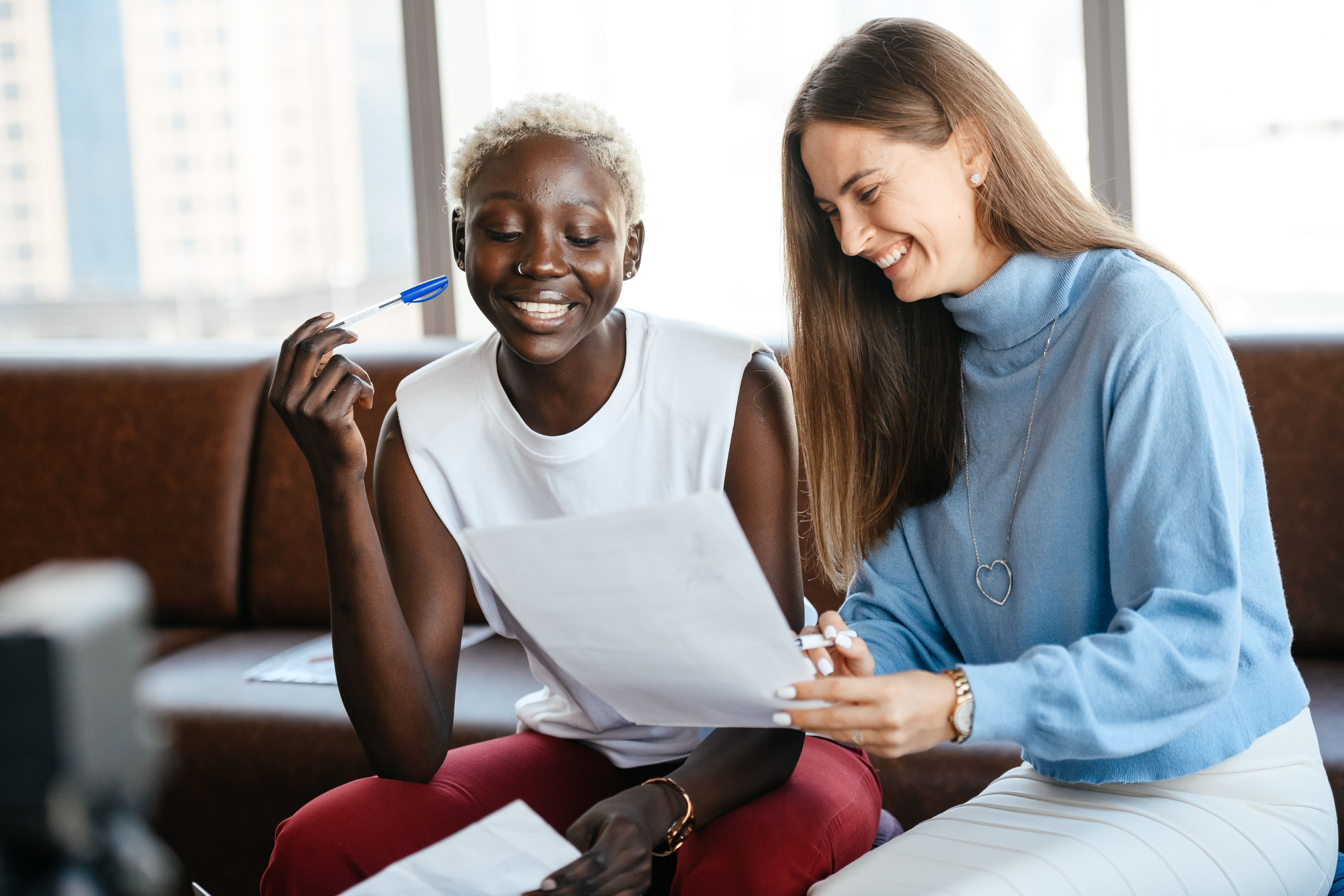
5 Ways to Practice Positive Thinking for Coaches

How to Improve Your Emotional Intelligence in the Workplace
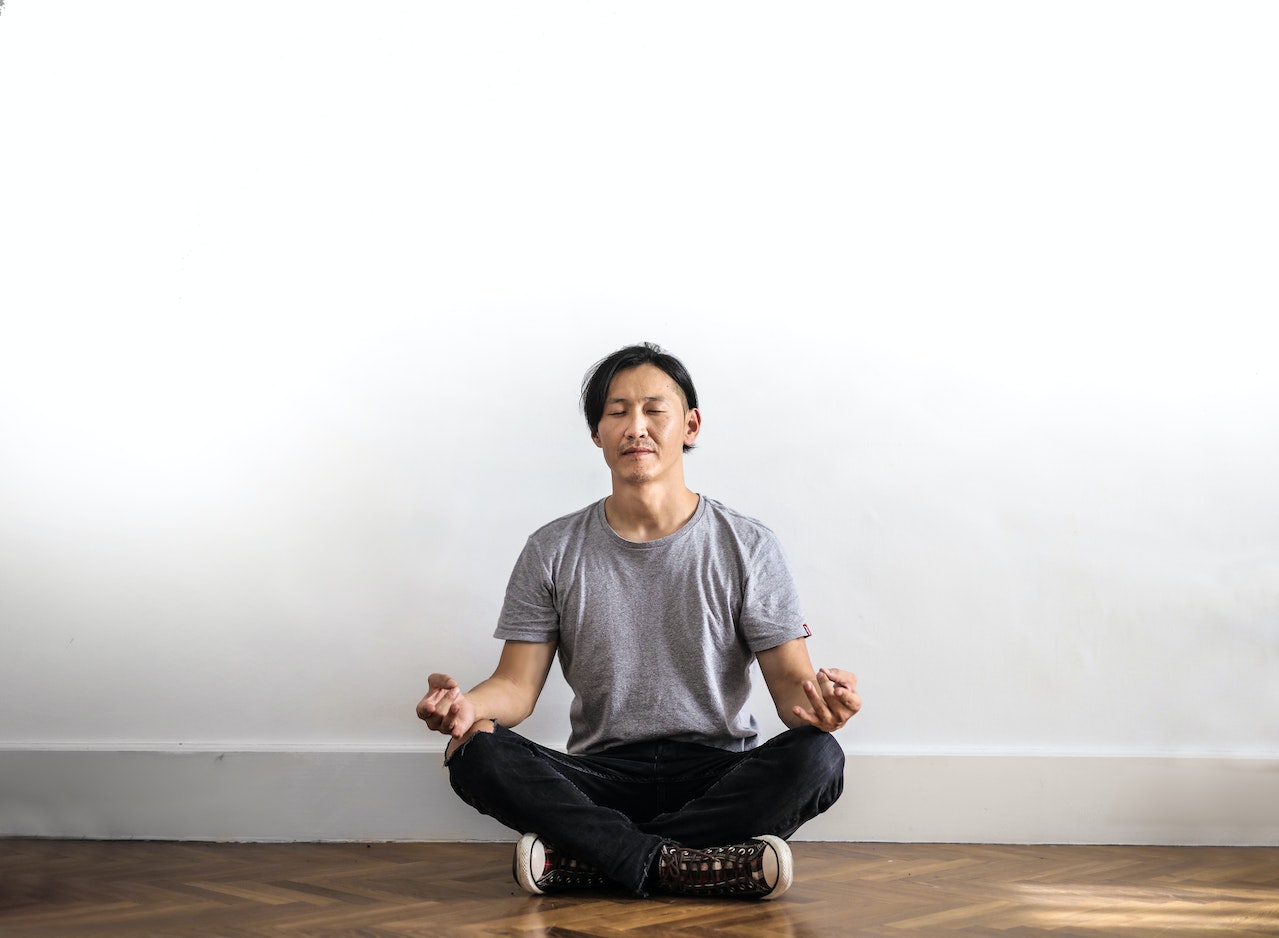
How to Practice Mindfulness: 6 Easy Ways
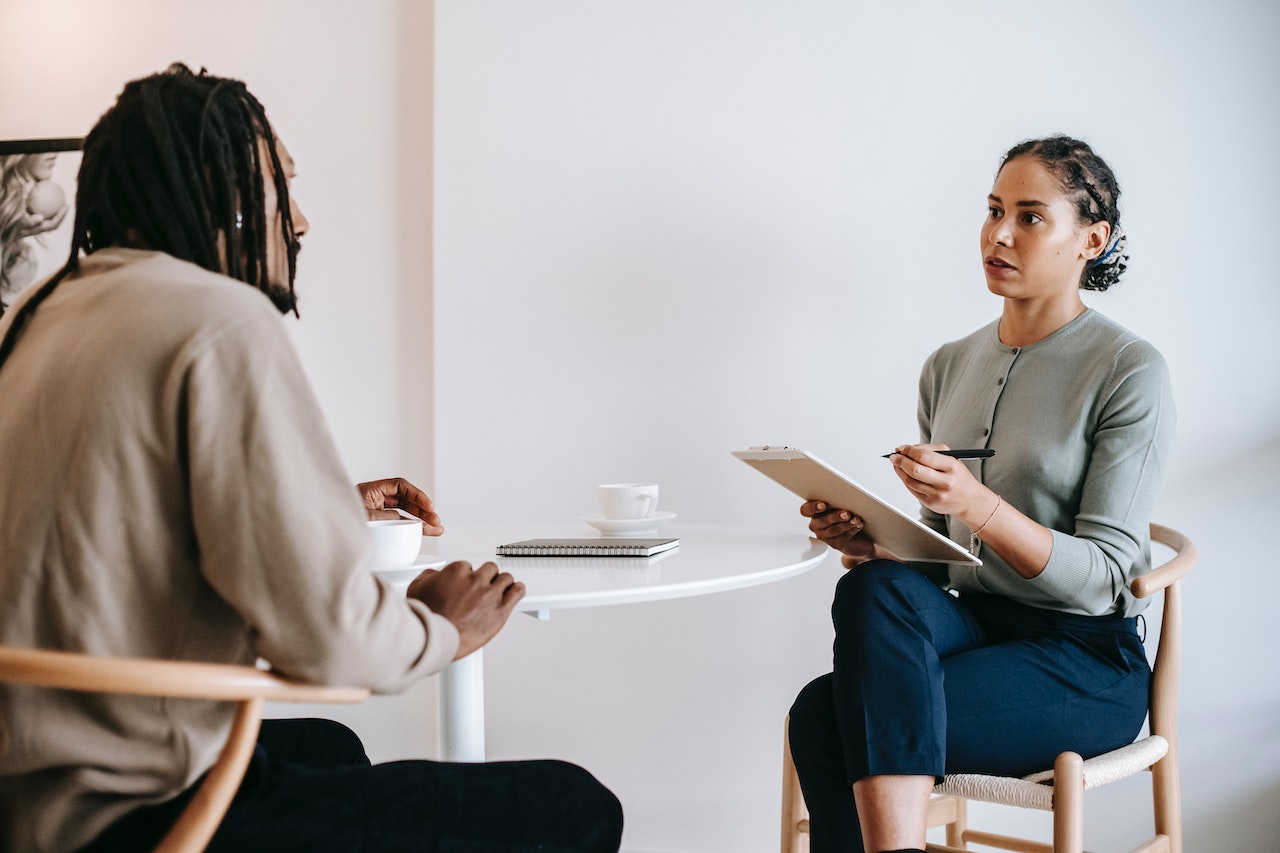
Feedback Best Practices: 6 Ways to Be Effective

Professional Development
What is a life coach and how to become one.
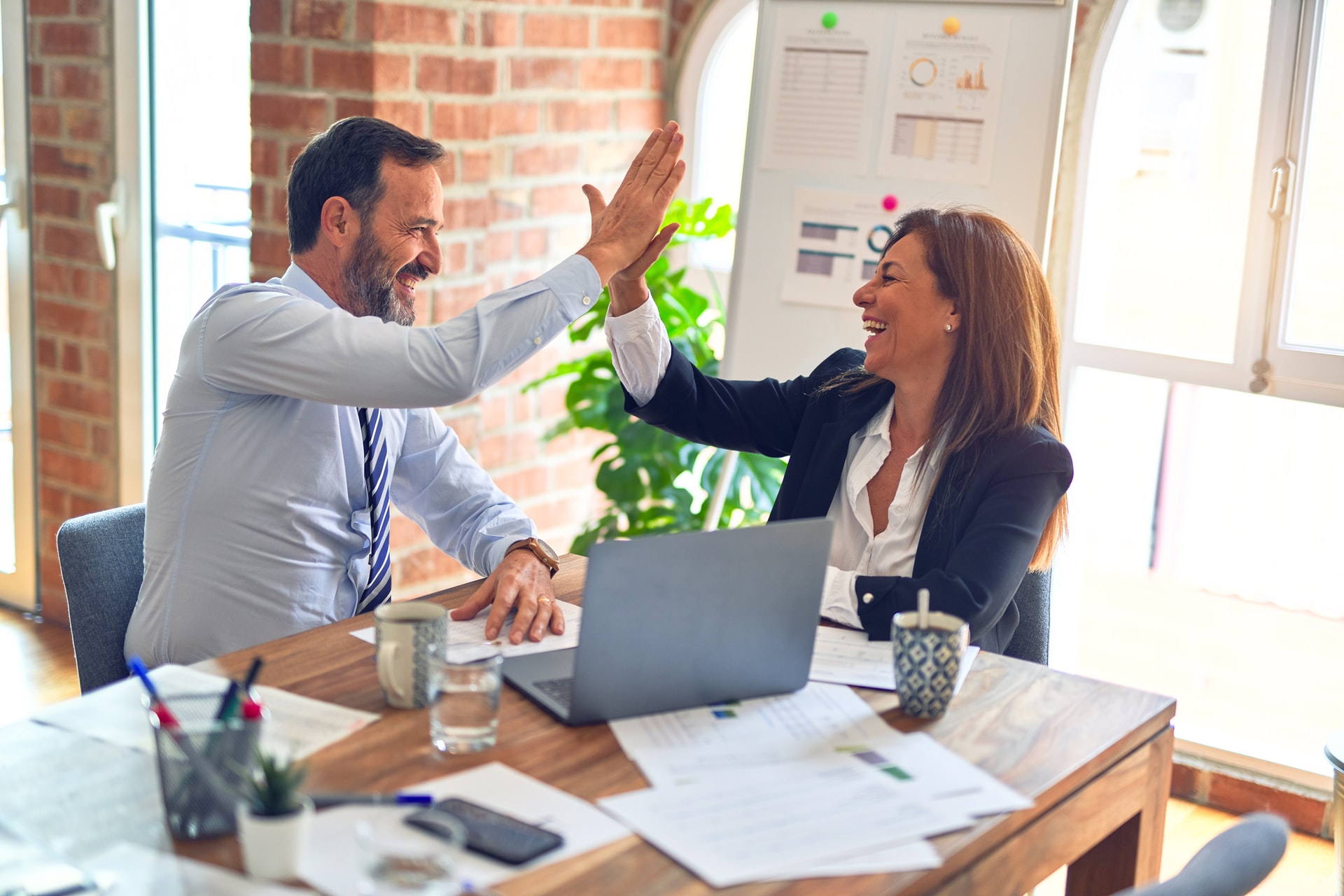
Client Success
The importance of customer service for successful coaching.

Reimagining Trust and Excellence in Life Coaching
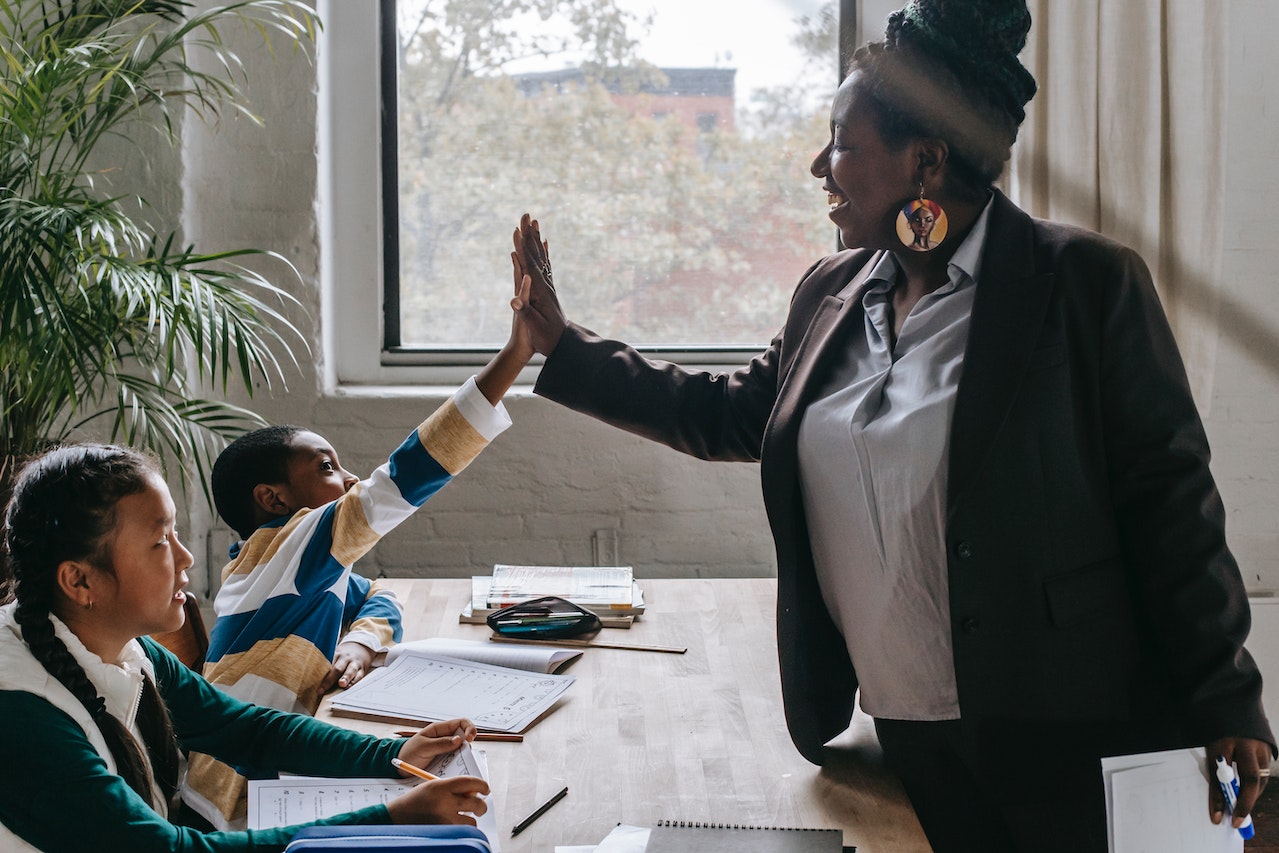
Guides & Templates
Tutor vs. teacher: what sets them apart.
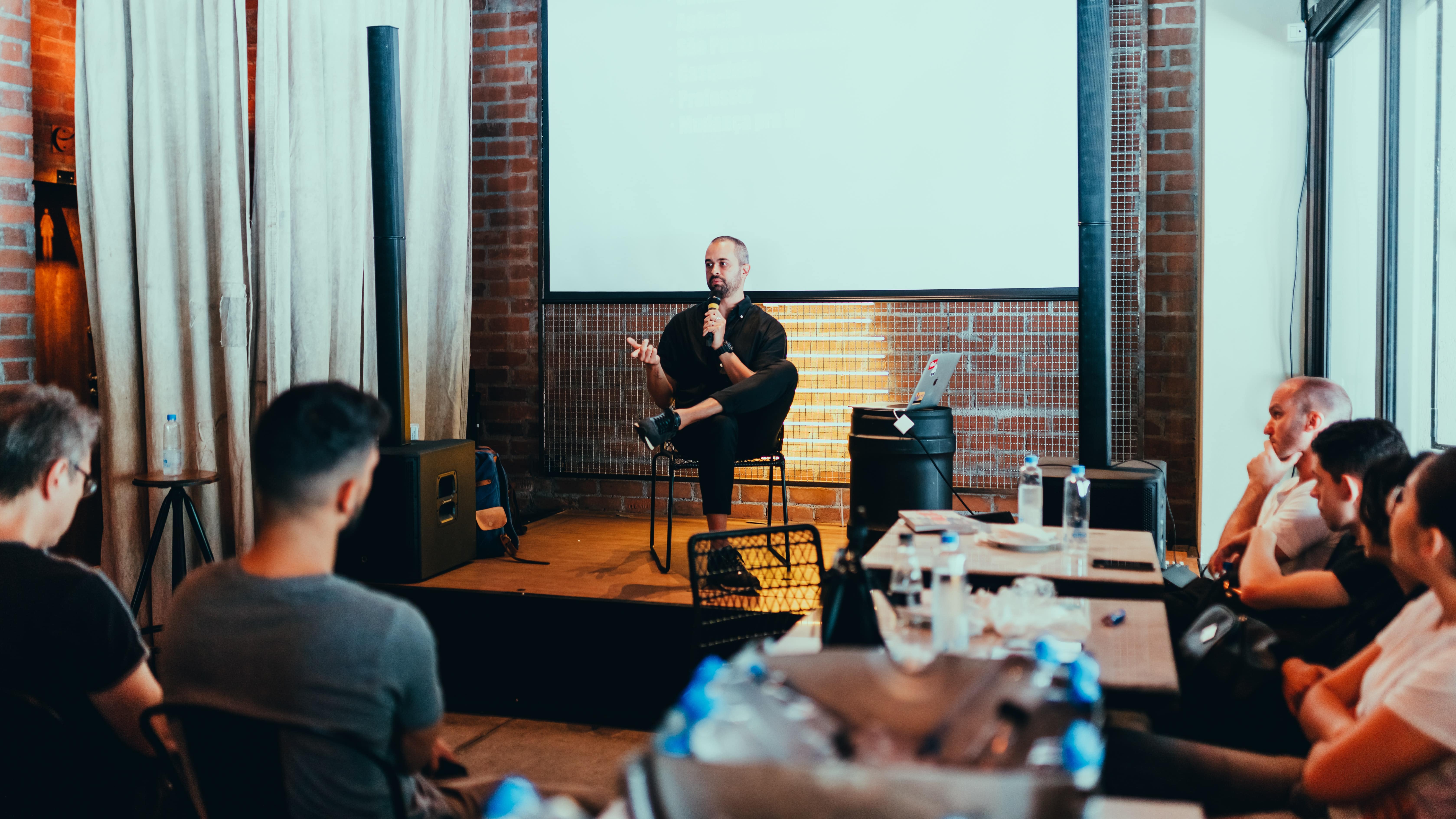
7 Communication Best Practices for Coaching
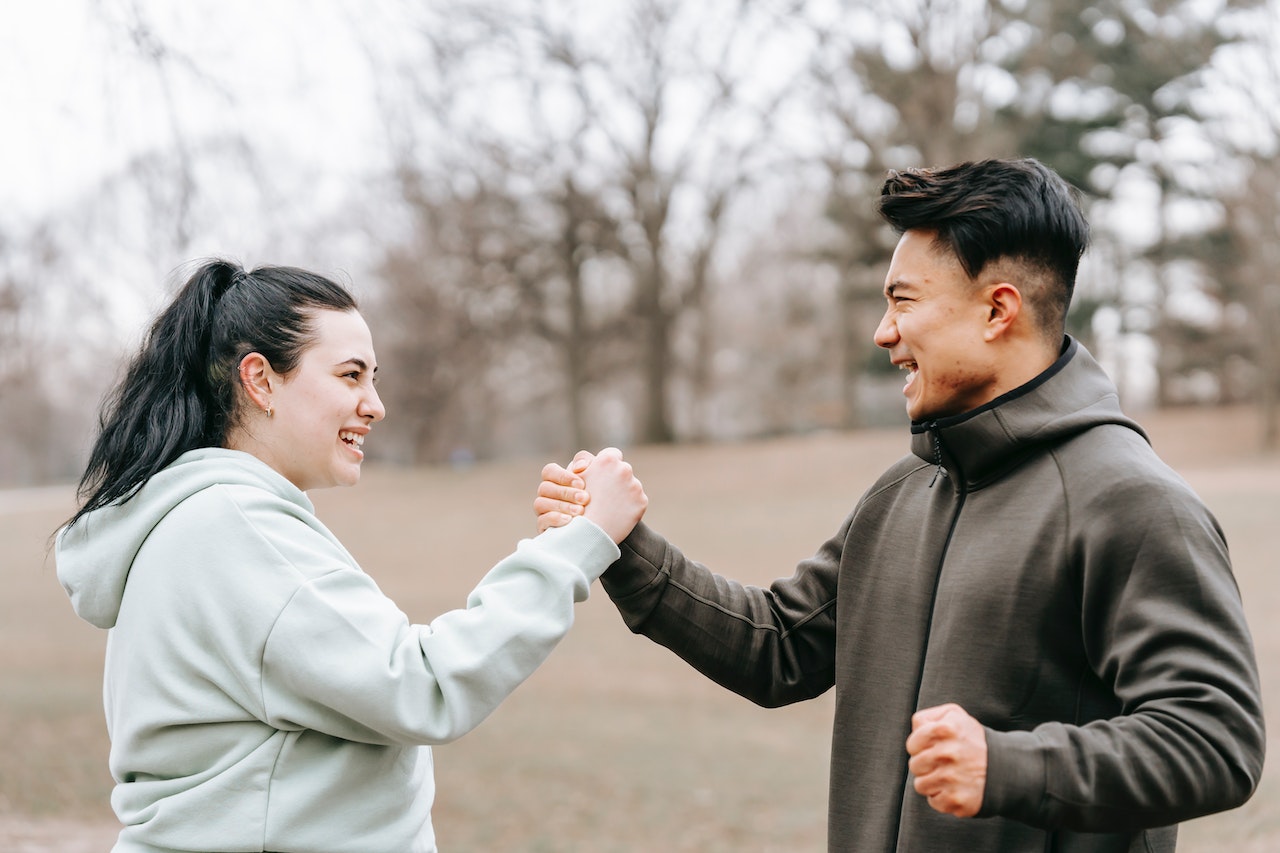
Fitness Coach Versus Personal Trainer: What’s the Difference?
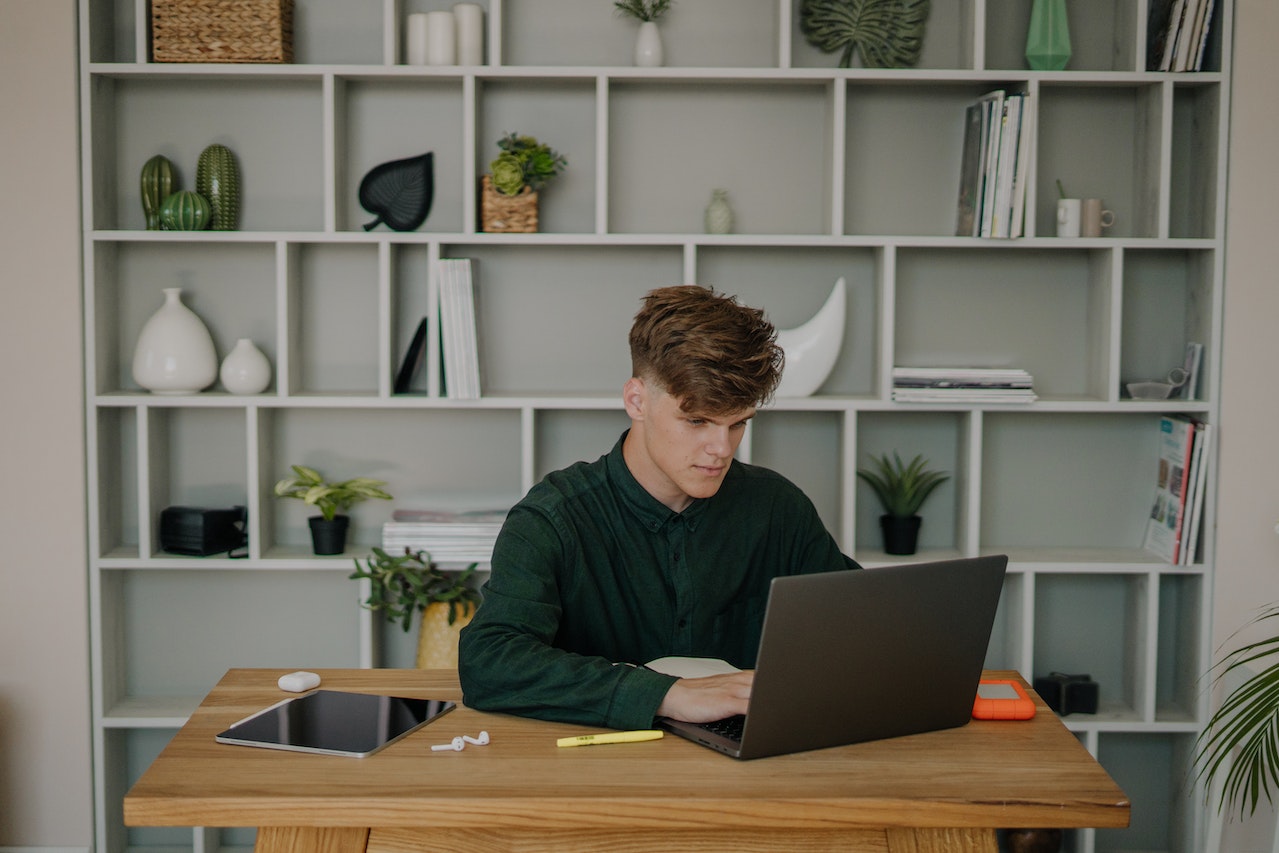
How Much Do Virtual Assistants Cost? A Complete Guide to Pricing
.png)
About Practice
4 ways practice helps coaches get organized.
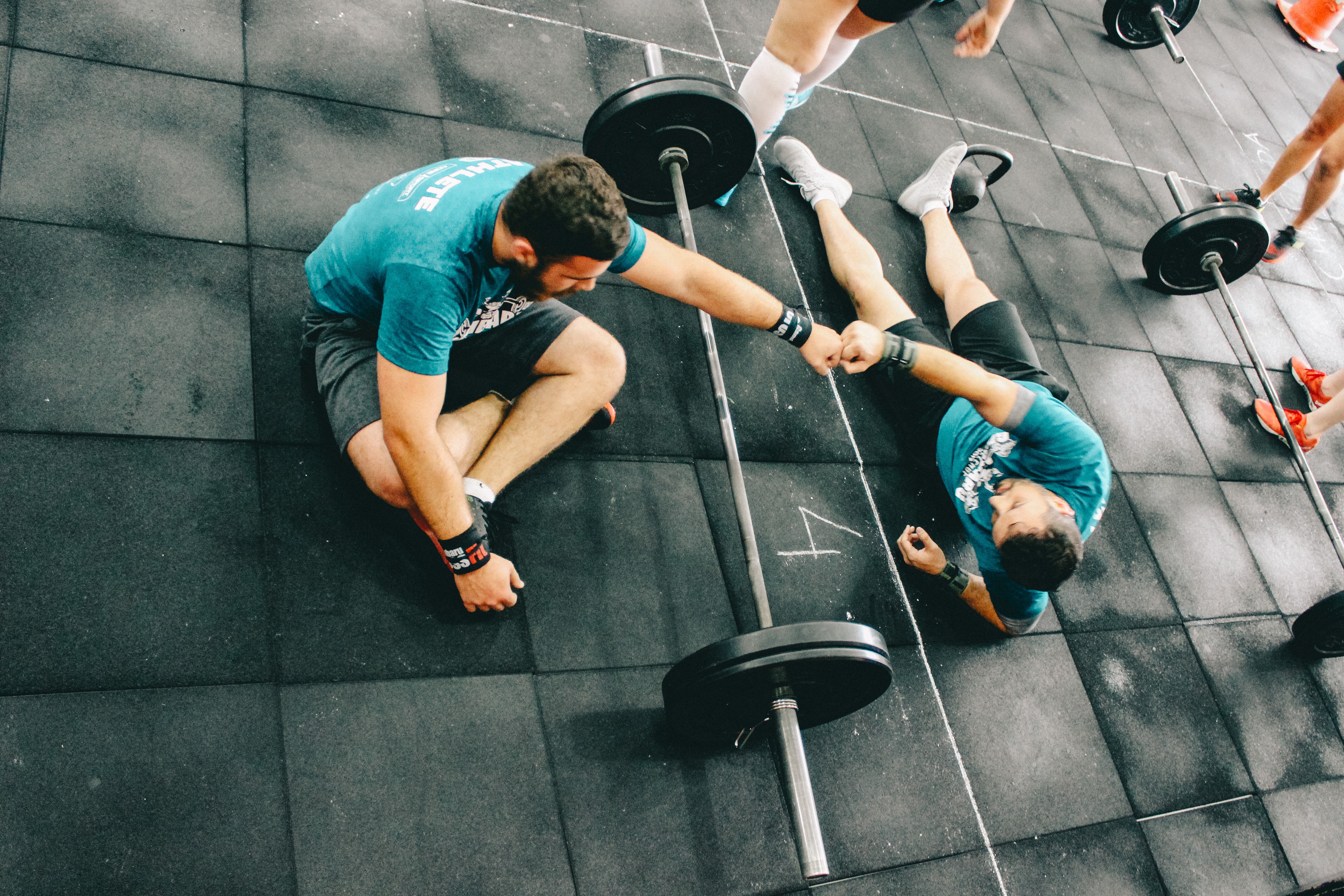
From Passion to Profit: How to Become an Online Personal Trainer (A Comprehensive Guide)
- Bipolar Disorder
- Therapy Center
- When To See a Therapist
- Types of Therapy
- Best Online Therapy
- Best Couples Therapy
- Managing Stress
- Sleep and Dreaming
- Understanding Emotions
- Self-Improvement
- Healthy Relationships
- Student Resources
- Personality Types
- Sweepstakes
- Guided Meditations
- Verywell Mind Insights
- 2024 Verywell Mind 25
- Mental Health in the Classroom
- Editorial Process
- Meet Our Review Board
- Crisis Support
Problem-Solving Strategies and Obstacles
JGI / Jamie Grill / Getty Images
- Application
- Improvement
From deciding what to eat for dinner to considering whether it's the right time to buy a house, problem-solving is a large part of our daily lives. Learn some of the problem-solving strategies that exist and how to use them in real life, along with ways to overcome obstacles that are making it harder to resolve the issues you face.
What Is Problem-Solving?
In cognitive psychology , the term 'problem-solving' refers to the mental process that people go through to discover, analyze, and solve problems.
A problem exists when there is a goal that we want to achieve but the process by which we will achieve it is not obvious to us. Put another way, there is something that we want to occur in our life, yet we are not immediately certain how to make it happen.
Maybe you want a better relationship with your spouse or another family member but you're not sure how to improve it. Or you want to start a business but are unsure what steps to take. Problem-solving helps you figure out how to achieve these desires.
The problem-solving process involves:
- Discovery of the problem
- Deciding to tackle the issue
- Seeking to understand the problem more fully
- Researching available options or solutions
- Taking action to resolve the issue
Before problem-solving can occur, it is important to first understand the exact nature of the problem itself. If your understanding of the issue is faulty, your attempts to resolve it will also be incorrect or flawed.
Problem-Solving Mental Processes
Several mental processes are at work during problem-solving. Among them are:
- Perceptually recognizing the problem
- Representing the problem in memory
- Considering relevant information that applies to the problem
- Identifying different aspects of the problem
- Labeling and describing the problem
Problem-Solving Strategies
There are many ways to go about solving a problem. Some of these strategies might be used on their own, or you may decide to employ multiple approaches when working to figure out and fix a problem.
An algorithm is a step-by-step procedure that, by following certain "rules" produces a solution. Algorithms are commonly used in mathematics to solve division or multiplication problems. But they can be used in other fields as well.
In psychology, algorithms can be used to help identify individuals with a greater risk of mental health issues. For instance, research suggests that certain algorithms might help us recognize children with an elevated risk of suicide or self-harm.
One benefit of algorithms is that they guarantee an accurate answer. However, they aren't always the best approach to problem-solving, in part because detecting patterns can be incredibly time-consuming.
There are also concerns when machine learning is involved—also known as artificial intelligence (AI)—such as whether they can accurately predict human behaviors.
Heuristics are shortcut strategies that people can use to solve a problem at hand. These "rule of thumb" approaches allow you to simplify complex problems, reducing the total number of possible solutions to a more manageable set.
If you find yourself sitting in a traffic jam, for example, you may quickly consider other routes, taking one to get moving once again. When shopping for a new car, you might think back to a prior experience when negotiating got you a lower price, then employ the same tactics.
While heuristics may be helpful when facing smaller issues, major decisions shouldn't necessarily be made using a shortcut approach. Heuristics also don't guarantee an effective solution, such as when trying to drive around a traffic jam only to find yourself on an equally crowded route.
Trial and Error
A trial-and-error approach to problem-solving involves trying a number of potential solutions to a particular issue, then ruling out those that do not work. If you're not sure whether to buy a shirt in blue or green, for instance, you may try on each before deciding which one to purchase.
This can be a good strategy to use if you have a limited number of solutions available. But if there are many different choices available, narrowing down the possible options using another problem-solving technique can be helpful before attempting trial and error.
In some cases, the solution to a problem can appear as a sudden insight. You are facing an issue in a relationship or your career when, out of nowhere, the solution appears in your mind and you know exactly what to do.
Insight can occur when the problem in front of you is similar to an issue that you've dealt with in the past. Although, you may not recognize what is occurring since the underlying mental processes that lead to insight often happen outside of conscious awareness .
Research indicates that insight is most likely to occur during times when you are alone—such as when going on a walk by yourself, when you're in the shower, or when lying in bed after waking up.
How to Apply Problem-Solving Strategies in Real Life
If you're facing a problem, you can implement one or more of these strategies to find a potential solution. Here's how to use them in real life:
- Create a flow chart . If you have time, you can take advantage of the algorithm approach to problem-solving by sitting down and making a flow chart of each potential solution, its consequences, and what happens next.
- Recall your past experiences . When a problem needs to be solved fairly quickly, heuristics may be a better approach. Think back to when you faced a similar issue, then use your knowledge and experience to choose the best option possible.
- Start trying potential solutions . If your options are limited, start trying them one by one to see which solution is best for achieving your desired goal. If a particular solution doesn't work, move on to the next.
- Take some time alone . Since insight is often achieved when you're alone, carve out time to be by yourself for a while. The answer to your problem may come to you, seemingly out of the blue, if you spend some time away from others.
Obstacles to Problem-Solving
Problem-solving is not a flawless process as there are a number of obstacles that can interfere with our ability to solve a problem quickly and efficiently. These obstacles include:
- Assumptions: When dealing with a problem, people can make assumptions about the constraints and obstacles that prevent certain solutions. Thus, they may not even try some potential options.
- Functional fixedness : This term refers to the tendency to view problems only in their customary manner. Functional fixedness prevents people from fully seeing all of the different options that might be available to find a solution.
- Irrelevant or misleading information: When trying to solve a problem, it's important to distinguish between information that is relevant to the issue and irrelevant data that can lead to faulty solutions. The more complex the problem, the easier it is to focus on misleading or irrelevant information.
- Mental set: A mental set is a tendency to only use solutions that have worked in the past rather than looking for alternative ideas. A mental set can work as a heuristic, making it a useful problem-solving tool. However, mental sets can also lead to inflexibility, making it more difficult to find effective solutions.
How to Improve Your Problem-Solving Skills
In the end, if your goal is to become a better problem-solver, it's helpful to remember that this is a process. Thus, if you want to improve your problem-solving skills, following these steps can help lead you to your solution:
- Recognize that a problem exists . If you are facing a problem, there are generally signs. For instance, if you have a mental illness , you may experience excessive fear or sadness, mood changes, and changes in sleeping or eating habits. Recognizing these signs can help you realize that an issue exists.
- Decide to solve the problem . Make a conscious decision to solve the issue at hand. Commit to yourself that you will go through the steps necessary to find a solution.
- Seek to fully understand the issue . Analyze the problem you face, looking at it from all sides. If your problem is relationship-related, for instance, ask yourself how the other person may be interpreting the issue. You might also consider how your actions might be contributing to the situation.
- Research potential options . Using the problem-solving strategies mentioned, research potential solutions. Make a list of options, then consider each one individually. What are some pros and cons of taking the available routes? What would you need to do to make them happen?
- Take action . Select the best solution possible and take action. Action is one of the steps required for change . So, go through the motions needed to resolve the issue.
- Try another option, if needed . If the solution you chose didn't work, don't give up. Either go through the problem-solving process again or simply try another option.
You can find a way to solve your problems as long as you keep working toward this goal—even if the best solution is simply to let go because no other good solution exists.
Sarathy V. Real world problem-solving . Front Hum Neurosci . 2018;12:261. doi:10.3389/fnhum.2018.00261
Dunbar K. Problem solving . A Companion to Cognitive Science . 2017. doi:10.1002/9781405164535.ch20
Stewart SL, Celebre A, Hirdes JP, Poss JW. Risk of suicide and self-harm in kids: The development of an algorithm to identify high-risk individuals within the children's mental health system . Child Psychiat Human Develop . 2020;51:913-924. doi:10.1007/s10578-020-00968-9
Rosenbusch H, Soldner F, Evans AM, Zeelenberg M. Supervised machine learning methods in psychology: A practical introduction with annotated R code . Soc Personal Psychol Compass . 2021;15(2):e12579. doi:10.1111/spc3.12579
Mishra S. Decision-making under risk: Integrating perspectives from biology, economics, and psychology . Personal Soc Psychol Rev . 2014;18(3):280-307. doi:10.1177/1088868314530517
Csikszentmihalyi M, Sawyer K. Creative insight: The social dimension of a solitary moment . In: The Systems Model of Creativity . 2015:73-98. doi:10.1007/978-94-017-9085-7_7
Chrysikou EG, Motyka K, Nigro C, Yang SI, Thompson-Schill SL. Functional fixedness in creative thinking tasks depends on stimulus modality . Psychol Aesthet Creat Arts . 2016;10(4):425‐435. doi:10.1037/aca0000050
Huang F, Tang S, Hu Z. Unconditional perseveration of the short-term mental set in chunk decomposition . Front Psychol . 2018;9:2568. doi:10.3389/fpsyg.2018.02568
National Alliance on Mental Illness. Warning signs and symptoms .
Mayer RE. Thinking, problem solving, cognition, 2nd ed .
Schooler JW, Ohlsson S, Brooks K. Thoughts beyond words: When language overshadows insight. J Experiment Psychol: General . 1993;122:166-183. doi:10.1037/0096-3445.2.166
By Kendra Cherry, MSEd Kendra Cherry, MS, is a psychosocial rehabilitation specialist, psychology educator, and author of the "Everything Psychology Book."
More From Forbes
8 consistent behaviors of practically perfect problem solvers.
- Share to Facebook
- Share to Twitter
- Share to Linkedin
The concept of a business strategy, analytics, search for solutions, the search output. Labyrinth of ... [+] colorful wooden blocks. People in the maze, finding a way out. The man in the maze.
This past month I celebrated my 42nd anniversary with my dear wife. One of her finest qualities is her ability to solve problems. I admit, while I like to analyze and contemplate the various approaches to solving a problem, she just gets it done. It turns out she is not the only female with this fantastic quality. According to our database, there is a trend among male and female leaders in solving problems.
In a dataset of over 47,000 men and 24,000 women leaders, men were rated higher on their technical/professional acumen. Still, women were rated higher on their ability to solve problems. The data came from 360-degree evaluations on each leader with an average of 13 assessments from their manager, peers, direct reports, and others. The graph below displays those results that were statistically significant.
ZFCO Study 2021- Problem Solving/Technical Expertise Scores
How These Skills Were Evaluated
I evaluated Technical/Professional Acumen by evaluating if people sought after the leader's opinions, had knowledge and skills that help achieve team results and if their ideas were trusted because of their in-depth knowledge.
Problem Solving was evaluated by assessing the leader's ability to anticipate and respond quickly to problems, was trusted to use good judgment in making decisions, and their ability to spot new trends and opportunities early.
Understanding the Results
The results for the analysis were confusing because it was clear that being rated better at technical expertise should give men an advantage at solving problems.
To verify the results, I re-ran the data with more of an experimental design selecting only 50% of the cases for the analysis. In the experimental design, the results yielded the same conclusions. Technical/Professional Expertise rated significantly higher for men (T-Value 2.276, Sig. 0.023). Problem Solving rated significantly higher for women (T-Value 2.432, Sig. 0.015).
So... What Does This Mean?
To better understand what created the differences in the results on Problem Solving, I analyzed the top behaviors highly correlated with problem-solving. Why? Because these were behaviors that would impact the perceptions about HOW problems were solved. I then compared the results for men versus women on 16 behaviors and found that 12 behaviors showed significant differences between men and women. Four of the behaviors were rated more positively for men, and eight were rated more positively for women. The graph below shows the 12 items that were significantly different for women and men. The items that were significantly different for men clustered around two issues. Technical/Professional expertise was rated significantly higher on two items and can influence problem-solving ability. The two other items focused on strategic perspective. Having the ability to understand how a problem connects to the vision and direction of the organization was a skill that also helped men solve problems. While these four behaviors were helpful for men, the eight behaviors that women performed more effectively made a bigger difference in the results.
In the end, regardless of men or women, these behaviors teach us some essential requirements for practical problem-solving that we ALL need to improve!
8 Behaviors of Practically Perfect Problem Solvers
What is evident in this analysis is that having an excellent solution to a problem, and a clear context for how that solution aligns with the strategy, are not the only two issues that impact a leader's expertise in problem-solving. Once again, the behaviors listed below influenced the PERCEPTIONS of others on problem-solving. So, what do others perceive these practically perfect problem-solvers to do?
ZFCO Problem Solving Study (2021)
1. They get it done right away . In other studies comparing men and women leaders, we have found that this competency consistently shows the most significant difference between genders. It is easy for problems to get put on the "I will do that later list." It seems we can learn a lot from the women in our lives that take on problems sooner and invest more energy to get them corrected.
2. They model this behavior to those around them . If you want to change the way others perceive you in that behavior, you need to be considered the role model for it. Be consistent. Those who are good role models are more trusted.
3. They don't work on problems alone . Many problems in an organization flow across multiple groups and cannot be resolved without collaboration. Women were rated as better at collaboration. Men tended to act more independently. If you're going to solve a problem quickly, involve those around you and share the glory.
4. They know how to explain the problem and solution effectively. A significant part of solving any problem is having good communication between everyone impacted by the problem. The first benefit of good communication is that it helps everyone understand the problem, when it occurs, and why it occurs. Second, the solution needs to be communicated to all involved parties.
5. They can influence others to stretch their abilities to solve the problem. Many problems are hard to solve and require that people do something differently than they have done in the past. This requires you to be more skillful at getting others to step up and stretch to accomplish difficult goals.
6. They quickly recognize where change is needed. It is easy to ignore many problems. It's harder to identify where change is needed and do something about it quickly.
7. They know how to focus on top priorities. We cannot fix everything, so concentrating on top priorities makes it possible for an organization to change the most pressing issues.
8. They understand the full context. Solving problems requires that all people affected know how their role impacts the problem to be solved. Solving problems is more than just coming up with an excellent solution.
Aren't you grateful for the problem solvers in your life? Problems can be stressful, overwhelming, and many of us want to pretend they don't exist. We want to hide them in a corner and tiptoe around them, pretending they are not there, and if we don't give them attention, they will walk away. They won't.
Problems get solved when someone takes the initiative to fix the problem, understands their role in the solution, and cooperates. Problem-solving frequently requires the efforts and involvement of others. If you have a significant problem that needs to be solved in your organization, be the person that doesn’t just understand the problem but solves it.
- Editorial Standards
- Reprints & Permissions
Developing Statistics Learning Materials on YouTube Media and Blogs for Improving Mathematics Problem-Solving skills and Learning Achievement
New citation alert added.
This alert has been successfully added and will be sent to:
You will be notified whenever a record that you have chosen has been cited.
To manage your alert preferences, click on the button below.
New Citation Alert!
Please log in to your account
Information & Contributors
Bibliometrics & citations, index terms.
Applied computing
Computer-managed instruction
Recommendations
Exploring university students' achievement, motivation, and receptivity of flipped learning in an engineering mathematics course.
This article is aimed at exploring students' learning achievement, motivation, and receptivity towards the flipped classroom in a university engineering mathematics course with a quasi-experimental design. Moreover, the study compared a half-semester ...
Improving Learning Motivation and Academic Achievement by Flipped Learning
Since the introduction history of flipped learning is short, there have been few previous studies on the impact of this on individual learners. The purpose of this study is to investigate the effectiveness of flipped learning, which is emerging as an ...
The effects of a computer mini-course in test-taking skills on student achievement in general mathematics
Information, published in.

Association for Computing Machinery
New York, NY, United States
Publication History
Permissions, check for updates, author tags.
- Learning achievement
- Normal gain
- Problem solving skill
- Research-article
- Refereed limited
Contributors
Other metrics, bibliometrics, article metrics.
- 0 Total Citations
- 0 Total Downloads
- Downloads (Last 12 months) 0
- Downloads (Last 6 weeks) 0
View Options
Login options.
Check if you have access through your login credentials or your institution to get full access on this article.
Full Access
View options.
View or Download as a PDF file.
View online with eReader .
HTML Format
View this article in HTML Format.
Share this Publication link
Copying failed.
Share on social media
Affiliations, export citations.
- Please download or close your previous search result export first before starting a new bulk export. Preview is not available. By clicking download, a status dialog will open to start the export process. The process may take a few minutes but once it finishes a file will be downloadable from your browser. You may continue to browse the DL while the export process is in progress. Download
- Download citation
- Copy citation
We are preparing your search results for download ...
We will inform you here when the file is ready.
Your file of search results citations is now ready.
Your search export query has expired. Please try again.
7 Spiritual Habits to Do Every Monday, to Focus Your Week on Christ
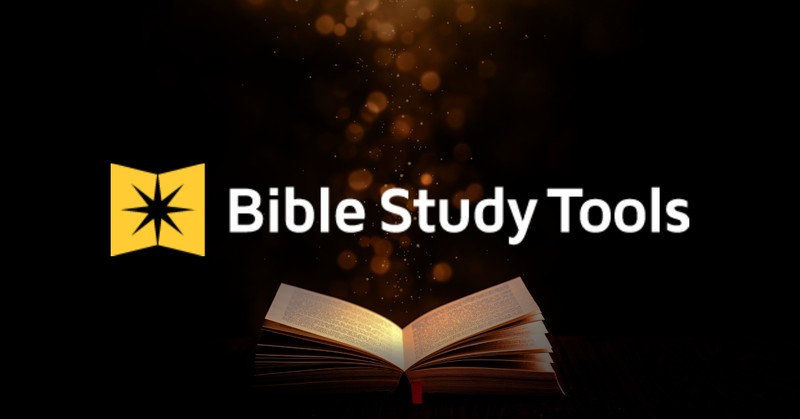
While Sunday is the start of the week, especially for Christians, Monday is often the day where people really have to lock into their tasks, start problem-solving, and deal with the realities of the week. It is easy to focus on Christ on the Sabbath, as it is a day set aside to rest and develop one’s relationship with the Savior. However, our relationship with Christ is the most important one anyone will have, and it requires daily nurturing. Building some habits on Monday to start off the week focused on the Lord is one way to grow that relationship deeper and stronger. Every day can be a day to draw closer to the Lord, even the one that is commonly voted the worst day of the week.
These seven habits can be incorporated into the believer’s Monday routine, to make sure they start the week focused on the Lord. You can try one or two of them, or all of them. See what best fits your schedule.
Photo credit: ©Getty Images/Nora Carol Photography
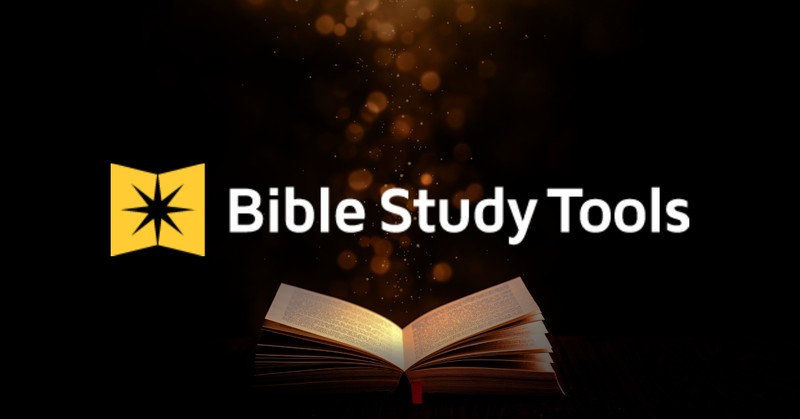
1. Have Certain Topics to Pray Over Every Monday
There are some prayer requests that take time to be answered, and so they need to be lifted up to the Lord regularly. Sometimes there are also pressing needs that weigh heavy on our hearts at the start of the week. Maybe these come from work, school, personal issues, or relationship dynamics.
Having certain topics, people, or issues to be praying over on select days of the week can help ensure we don’t forget to pray for important topics and they don’t get lost in the business of life. Monday topics can be work, someone’s illness, school, friends, and world affairs – whatever is personal and important to you.
“Do not be anxious about anything, but in everything by prayer and supplication with thanksgiving let your requests be made known to God” ( Philippians 4:6 ).
2. Meditate on the Lessons from Sunday’s Sermon
It can be easy to listen to a sermon and not absorb it. The Bible encourages us to meditate on God’s Word, and the book of Acts records how the early church would gather regularly to talk about the things of God. While on a Monday morning a believer today may have to go to work, focus on tasks at home, or go to school, we can still carve out time throughout the day to reflect on the sermon from the day before. Talk about it with friends or family, or review any notes you may have taken.
“Let the word of Christ dwell in you richly, teaching and admonishing one another in all wisdom, singing psalms and hymns and spiritual songs, with thankfulness in your hearts to God” ( Colossians 3:16 ).
Photo credit: ©Getty Images/Amber N Ford
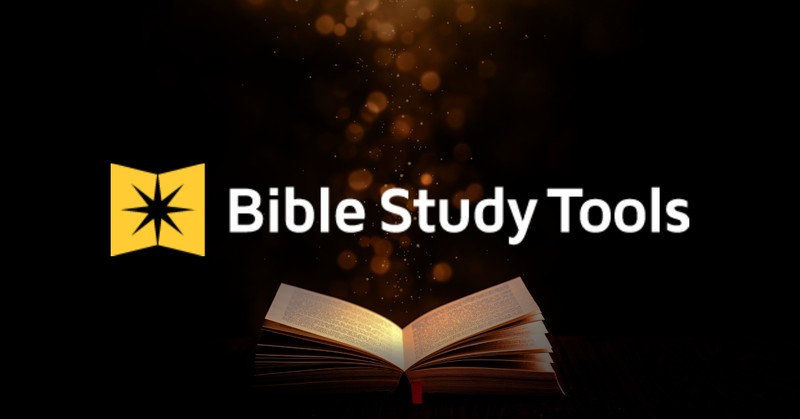
3. Make a List of That Week’s Tasks, and Pray for God’s Help with Them
This habit is both practical and spiritual, as it invites the Lord to be a help, not just in big spiritual problems, but in the daily ins and outs of life. Whatever tasks need to get accomplished that week, ask the Lord to support the efforts, to bless them, and to guide you. The Lord promises He is with those who love Him at all times, and that includes in the mundane tasks of life, in secular work, and in the little things. Relying on God is a sure way to be in His will, and to find burdens less difficult to carry.
“Behold, God is my helper; the Lord is the upholder of my life” ( Psalm 54:4 ).
4. Pick a Verse or Passage to Memorize during the Week
It can be difficult to memorize Scripture , especially when modern schedules keep us going all the time and social media serves as a distraction during periods of relaxation. Having one verse or one small passage that is the object of study for the week can be a good way to learn more of the Bible, to grow closer to God, and to have a deeper understanding of the Scriptures.
Monday is a great day to sit down and pick a verse or section of the Bible to learn that week, and then start the process of memorizing. Some people need to repeat it out loud, while others need to copy it. Find whatever works best for you, but starting on Monday and having seven days to practice the verse can help you truly meditate on the meaning and the message.
“But as for you, continue in what you have learned and have firmly believed, knowing from whom you learned it and how from childhood you have been acquainted with the sacred writings, which are able to make you wise for salvation through faith in Christ Jesus. All Scripture is breathed out by God and profitable for teaching, for reproof, for correction, and for training in righteousness, that the man of God may be complete, equipped for every good work” ( 2 Timothy 3:14-17 ).
Photo credit: ©Getty Images/BitsAndSplits
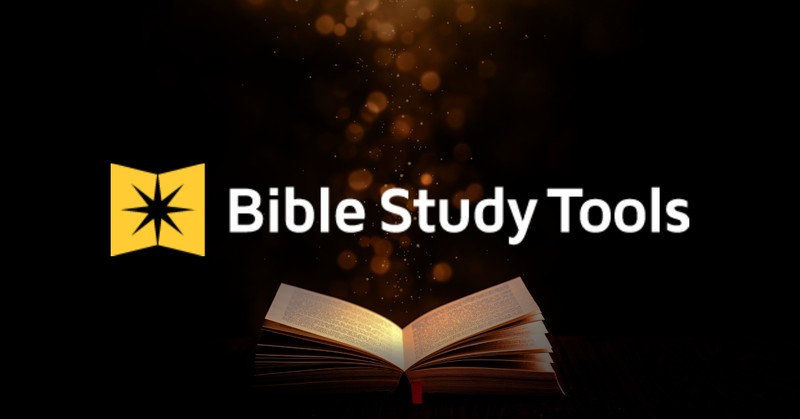
5. Reach Out to Friends or Family for Prayer Requests
Christians should pray for others. For the nonbeliever, we should pray for their salvation, for their health, and for the working of the Holy Spirit . Sometimes a nonbeliever is open to a Christian friend praying for them, and so reaching out and asking if there’s any way we can be praying for them can be an opportunity to minister to them, and even share the gospel.
Christians should also lift up each other, and should weep with those who weep and rejoice with those who rejoice. Touching base with family and friends on Sunday night or on Monday to see if there is anything where they would appreciate prayer support is a way to strengthen those relationships, and to follow Christ’s example.
“And so, from the day we heard, we have not ceased to pray for you, asking that you may be filled with the knowledge of his will in all spiritual wisdom and understanding, so as to walk in a manner worthy of the Lord, fully pleasing to him: bearing fruit in every good work and increasing in the knowledge of God; being strengthened with all power, according to his glorious might, for all endurance and patience with joy; giving thanks to the Father, who has qualified you to share in the inheritance of the saints in light” ( Colossians 1:9-12 ).
6. Choose a Worship Song of the Week
Worship is an important part of the Christian life; it is not always manifested in music, but it is a common, even universal, way believers praise God. If there is a particular song God is laying on your heart, one that is speaking to your life circumstances, or even if you are just interested in expanding your catalogue of worship songs, picking a song to focus on that week can help you learn more about God and draw closer to Him.
Pick a song on Monday, listen to it every day (though maybe not all day every day), and look up the words. Maybe research the history of the song, as it may be inspirational or give you insight to deepen its meeting.
“Oh come, let us worship and bow down; let us kneel before the Lord, our Maker!” ( Psalm 95:6 ).
Photo credit: ©Getty Images/Viktoriia Hnatiuk
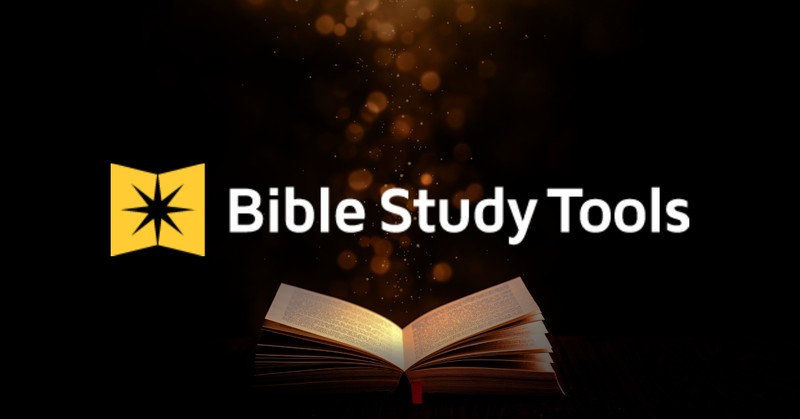
7. Pray for God to Lead You to Someone Who Needs to Hear the Gospel
Jesus wants everyone to repent of their sins, put their faith in Him, and receive the gift of eternal life. Once someone does so and becomes a Christian, part of their journey is to share the good news of salvation with others who are lost and in darkness. While not every Christian is going to find someone every day (or even every week) with whom they can share the Gospel, being on the lookout and praying for God to lead puts the believer in the will of God. It also conforms them more greatly to the image of Christ, who is always knocking on the door of the hearts of people, waiting for them to receive Him.
“’For everyone who calls on the name of the Lord will be saved.’ How then will they call on him in whom they have not believed? And how are they to believe in him of whom they have never heard? And how are they to hear without someone preaching?” ( Romans 10:13-14 ).
Having a relationship with Jesus Christ is the most important decision that someone can make, and nurturing it is an important part of their journey of sanctification. Just because it is Monday doesn’t mean Jesus isn’t reaching out, wanting to spend time with the people who love Him. He is also reaching out to the people who are still His enemies and have not put their faith in Him as Lord and Savior. While Mondays may be busy, there is always a way to connect with God, and to make Christ the center of life.
Photo credit: ©Getty Images/master1305
Bethany Verrett is a freelance writer who uses her passion for God, reading, and writing to glorify God. She and her husband have lived all over the country serving their Lord and Savior in ministry. She has a blog on graceandgrowing.com .
Improved multi-strategy adaptive Grey Wolf Optimization for practical engineering applications and high-dimensional problem solving
- Open access
- Published: 05 September 2024
- Volume 57 , article number 277 , ( 2024 )
Cite this article
You have full access to this open access article
- Mingyang Yu 1 ,
- Jing Xu 1 ,
- Weiyun Liang 1 ,
- Sixu Bao 1 &
- Lin Tang 2
The Grey Wolf Optimization (GWO) is a highly effective meta-heuristic algorithm leveraging swarm intelligence to tackle real-world optimization problems. However, when confronted with large-scale problems, GWO encounters hurdles in convergence speed and problem-solving capabilities. To address this, we propose an Improved Adaptive Grey Wolf Optimization (IAGWO), which significantly enhances exploration of the search space through refined search mechanisms and adaptive strategy. Primarily, we introduce the incorporation of velocity and the Inverse Multiquadratic Function (IMF) into the search mechanism. This integration not only accelerates convergence speed but also maintains accuracy. Secondly, we implement an adaptive strategy for population updates, enhancing the algorithm's search and optimization capabilities dynamically. The efficacy of our proposed IAGWO is demonstrated through comparative experiments conducted on benchmark test sets, including CEC 2017, CEC 2020, CEC 2022, and CEC 2013 large-scale global optimization suites. At CEC2017, CEC 2020 (10/20 dimensions), CEC 2022 (10/20 dimensions), and CEC 2013, respectively, it outperformed other comparative algorithms by 88.2%, 91.5%, 85.4%, 96.2%, 97.4%, and 97.2%. Results affirm that our algorithm surpasses state-of-the-art approaches in addressing large-scale problems. Moreover, we showcase the broad application potential of the algorithm by successfully solving 19 real-world engineering challenges.
Explore related subjects
- Artificial Intelligence
Avoid common mistakes on your manuscript.
1 Introduction
The rapid advancement of science, technology, and industry has given rise to a multitude of intricate optimization problems. These problems frequently entail numerous variables, constraints, and objectives. Their solution spaces are huge and complex, and it is difficult for traditional deterministic optimization methods to obtain satisfactory solutions in acceptable time (Deng et al. 2022 ; Guo et al. 2023 ; Zhou et al. 2022 ). To cope with these challenges, researchers in the field of computational intelligence have started to search for new approaches. Among them, metaheuristic algorithms have attracted much attention due to their high efficiency, universal applicability and powerful global search capability (Aldosari et al. 2022 ; Chauhan et al., 2024; Chen et al. 2023 ).
When dealing with engineering problems, constraints are a crucial consideration. Constraints may be physical limitations or requirements and restrictions of the project. In the field of engineering, there are various techniques available for handling these constraints to ensure that projects proceed as expected and achieve their intended goals. One common technique for constraint handling is optimization algorithms. Optimization algorithms assist engineers in finding the best solution given certain constraints. These algorithms can be mathematical optimization methods such as linear programming, integer programming, or nonlinear programming, or they can be heuristic algorithms such as genetic algorithms, simulated annealing, or particle swarm optimization. By leveraging these algorithms, engineers can find the optimal design or decision solution while taking into account various constraints (Fu et al. 2024b ; Li et al. 2023 ).
MH algorithms are inspired by certain phenomena in nature, such as PSO (Kennedy and Eberhart 1995b ), Firefly Algorithm (FA) (Yang 2009 ), Sine Cosine Algorithm (SCA) (Mirjalili 2016 ), Wind Driven Optimization (WDO) (Bayraktar et al. 2010), Fruit Fly Optimization Algorithm (FOA) (Pan 2012 ), Competitive Swarm Optimizer (Chauhan et al. 2024 ), Fox optimizer(FOX) (Mohammed and Rashid 2023 ), Fitness Dependent Optimizer(FDO) (Abdullah and Ahmed 2019 ) and so on. These algorithms often do not rely on the specific nature of the problem, but instead draw on nature's strategies for stochastic search, which can effectively avoid falling into local optima (Abdel-Basset et al. 2023 ). With the development of deep learning, neural networks and other machine learning techniques, researchers have begun to try to combine these techniques with metaheuristic algorithms to further improve the efficiency of solving complex optimization problems (Garg et al. 2023 ). In recent years, with the wide application of heuristic intelligent optimization algorithms in numerical optimization solving, various swarm intelligence algorithms have been proposed (Fu et al. 2022 ).
The popularity of MH algorithms has four distinct advantages: practicality, generalizability, non-leading properties and avoidance of local optima (Fu et al. 2023a ). First, based on their natural theoretical framework, these strategies are relatively intuitive to construct and deploy, thus allowing engineers and researchers to rapidly integrate them into concrete applications (Havaei & Sandidzadeh 2023 ). Next, since these algorithms treat the problem as an unknown mechanism, they can be applied to a wide range of different tasks such as selection (Said, Elarbi, Bechikh, Coello Coello, & Said, 2023), shop visit balancing (Xia et al. 2023 ) and engineering problems (Nadimi-Shahraki et al. 2022 ). Further, these methods do not rely on derivative information and are particularly good for dealing with nonlinear problems (Aldosari et al. 2022 ). Ultimately, with the help of a global search strategy and a stochastic strategy for updating the location, they can efficiently jump out of the local optimum, which is particularly effective for those scenarios where there are multiple locally optimal solutions.
The existing MH algorithm mainly include: Physics-based algorithms (PhA), Swarm Intelligence Algorithms (SI), Natural Evolutionary Algorithms (EA), and Human-based algorithms (Abualigah et al. 2021 ). In the course of evolution, cooperative behavior between individuals has been gradually formed through natural selection over a long period of time. For example, Trojovský and Dehghani, 2022 proposed a pelican optimization algorithm (POA) inspired by pelican predation. The Genetic Algorithm (GA) is a method of optimization based on the principles of natural evolution. It was proposed by John Holland and his colleagues. is a typical example inspired by Darwinian evolution (Bäck & Schwefel 1993 ). Differential Evolution (DE), based on the concepts of natural selection and reproduction in Darwinian evolution(Storn and Price 1997 ); Genetic Programming (GP), inspired by biological evolution processes; and Evolution Strategies (ES) (Wei 2012 ). Among these, Genetic Algorithms (GA) and Differential Evolution (DE) are widely considered to be the most popular evolutionary algorithms, having garnered significant attention and being applied in numerous applications. Physical method is the result of the interaction of physical law and chemical variation. For example, the Chernobyl Disaster Optimizer (CDO) is an optimization algorithm inspired by the core explosion at the Chernobyl nuclear power plant. (Shehadeh 2023 ). The Galaxy Swarm Optimization (GSO) (Muthiah-Nakarajan and Noel 2016 ) algorithm, inspired by the motion of galaxies; the Firefly Algorithm (FFA), drawing inspiration from soil fertility in agriculture (Shayanfar and Gharehchopogh 2018 ); the Firefly Algorithm (FFA), drawing inspiration from soil fertility in agriculture (Eskandar et al. 2012 ); and the Gravitational Search Algorithm (GSA), derived from Newton's law of universal gravitation and kinematic laws (Rashedi et al. 2009 ).In sharp contrast, a variety of human behaviors are simulated based on human behavior patterns, such as the "Alpine skiing Optimization (ASO)" proposed by Professor Yuan, a new idea influenced by the competitive behavior of athletes. Each of these different Metaheuristic algorithms has its own characteristics. According to different problems and requirements, appropriate algorithms can be selected to solve the optimal problems (Yuan et al. 2022 ). Particle Swarm Optimization (PSO) (Kennedy and Eberhart 1995a ) is inspired by the foraging behavior of bird flocks and fish schools. The Ant Colony Optimization (ACO) algorithm (Dorigo et al. 2006 ) is inspired by the social behavior of ant colonies during foraging. The Pathfinder Algorithm (PFA) (Yapici & Cetinkaya 2019 ) is inspired by the collective action of animal populations in finding optimal food areas or prey. The Harris Hawk Optimization algorithm (HHO)(Heidari et al. 2019 ) is based on the predatory process of Harris hawks hunting rabbits. The Sparrow Search Algorithm (SSA) (Xue and Shen 2020 ) is inspired by the foraging and anti-predatory behavior of sparrows. The Dung Beetle Optimization algorithm (DBO) (Xue and Shen 2022 ) is inspired by the rolling, dancing, foraging, stealing, and reproductive behaviors of dung beetles. The Remora Optimization Algorithm (ROA) (Jia et al. 2021 ) is inspired by the behavior of remoras adhering to different-sized hosts to facilitate foraging. The Black Widow Optimization algorithm (BWO) (Hayyolalam and Kazem 2020 ) is inspired by the unique reproductive behavior of black widow spiders. Dikshit Chauhan et al. proposed the Artificial Electric Field Algorithm (AFFEA) based on a series of learning strategies (Chauhan and Yadav 2024a , b ). Additionally, the Secretary Bird Optimization Algorithm (SBOA) was introduced based on the survival behavior of secretary birds in their natural environment (Fu et al. 2024b ), while the Red-Billed Blue Magpie Optimizer (RBMO) was proposed by simulating the search, chase, prey attack, and food storage behaviors of red-billed blue magpies (Fu et al. 2024a ).
Generally, the optimization process of the MH algorithm can be divided into two main steps (Saka et al. 2016 ): exploration and exploitation. In the exploration phase, the algorithm mainly focuses on searching all corners of the solution space to ensure that no possible optimal solution area is missed; while in the exploitation phase, the algorithm will focus on known high-quality solutions and further deepen the search in order to find the real the optimal solution. These two phases complement each other and ensure that the algorithm has both breadth and depth. GWO is inspired by the hunting behavior of grey wolves (Mirjalili et al. 2014 ). GWO effectively balances the two stages of exploration and exploitation by combining the social behavior of grey wolves with a dynamically adjusted location update strategy, thereby ensuring good global and local search capabilities.
Since its introduction in 2014, GWO has received widespread attention from scholars at home and abroad for its simplicity and efficiency, and has become an important tool for solving complex optimization problems (Fan and Yu 2022 ). However, similar to other optimization algorithms, the GWO algorithm does have some limitations although it has shown quite good performance in many optimization problems. In particularly, it is prone to suffer from prematurity and local optimality when dealing with multimodal function problems. As the iterative process of the GWO progresses, the inherent social hierarchy mechanism within the wolf population leads to a decrease in diversity. This mechanism prioritizes the positions and decisions of the leading wolves (Alpha, Beta, and Delta), influencing the entire pack's movement. As a result, the population tends to converge towards the leaders’ positions. However, this strong convergence driven by the social hierarchy can also lead to a drawback. The population may start to aggregate too closely or blindly around the leaders’ current positions. This phenomenon, often referred to as premature convergence, limits the algorithm’s ability to thoroughly explore the solution space. Consequently, the algorithm might struggle to escape local optima, as the current best solutions (guided by the leading wolves) might not always represent the global optimum. The pack, following the leaders too closely, can get trapped in these local optima, lacking the diversity or exploratory behavior needed to venture out and discover better solutions elsewhere in the search space. (Wang et al. 2018 ). In addition, when global exploration transitions to local mining, the algorithm may lose the ability to explore a wider solution space and overly concentrate on a specific region for detailed search. Such a centralized strategy, although helpful in finding the optimal solution in the local region accurately, may also lead the algorithm to ignore other promising regions (Wolpert and Macready 1997 ). Despite there has been various GWO variants, such as Advanced Grey Wolf Optimizer (AGWO) (Meng et al. 2021 ), Exponential Neighborhood Grey Wolf Optimization (EN-GWO) (Mohakud and Dash 2022 ), Hybrid Grey Wolf Optimizer with Mutation Operator (DE-GWO) (Gupta and Deep 2017 ), and others (Ambika et al. 2022; Biabani et al. 2022 ). These improved versions do not break through in solving the large-scale global optimization problems of CEC 2022 and CEC 2013. Moreover, their performance in dealing with complex problems remains unsatisfactory.
To improve the performance of the GWO, this study incorporates several key enhancements. Firstly, the search mechanism from PSO is employed to increase population diversity. This addition helps in broadening the search scope of the algorithm. Secondly, the IMF is used to adjust inertia weights, a strategy that aids in fine-tuning the balance between exploration and exploitation. Lastly, an adaptive mechanism based on the Sigmoid function is introduced for updating the positions of individuals within the population. This adaptive update strategy strengthens the group's ability to escape local optima, enhancing the overall effectiveness of the GWO algorithm in finding optimal solutions.
An improved adaptive grey wolf optimization (IAGWO) is proposed to address the shortcomings of the GWO algorithm. The main contributions are as follows.
The PSO search mechanism is introduced to enhances the algorithm's search efficiency and robustness by updating grey wolf positions early in each iteration. Additionally, the dynamic adjustment of inertia weights through the IMF boosts global search capability initially and local search effectiveness later.
Adaptive position updating strategy based on Sigmoid function to balance the exploration and exploitation of IAGWO.
To evaluate the exploration and exploitation capabilities of IAGWO, extensive experimentation is conducted using a suite of 67 test functions, which includes benchmarks from the CEC 2014, CEC 2017, CEC 2020, CEC 2022, and CEC 2013 for large-scale global optimization problems.
The effectiveness and accuracy of IAGWO in solving practical engineering design challenges are thoroughly assessed through its application to 19 diverse engineering design challenges.
The paper is organized as follows: Sect. 2 provides a brief review of the previous enhancements and potential application directions of the GWO. Section 3 details the original GWO algorithm and the proposed improvement strategy. Section 4 evaluates IAGWO performance through relevant experiments and in-depth analysis. Finally, Sect. 5 concludes this paper with a summary of the results and an outlook on future research directions.
2 Related work
In recent years, there has been a significant focus among researchers on enhancing the GWO. These improvements are aimed at boosting the algorithm's search performance and effectiveness. Scholars have explored various approaches to achieve this, including aspects such as adjusting the algorithm parameters, improving the speed and position equations, and combining it with other algorithms.
Yu et al. ( 2023 ) adopted a new update search mechanism, improved control parameters, mutation driven strategy and greedy selection strategy to improve GWO in the search process. (Singh and Bansal 2022a ) proposed a hybrid GWO and Differential Evolution (HGWODE) algorithm and applied it to UAV path planning. (Cuong-Le et al. 2022 ) introduced an equation to control the moving strategy of the algorithm in each iteration and proposed New Balance Grey Wolf Optimizer (NB-GWO), which was used to optimize the hyperparameters of the deep neural network for damage detection of two-dimensional concrete frames. Liu et al. ( 2023 ) proposed a hybrid differential evolution GWO (DE-GWO) algorithm and applied it to gas emission identification and localization. Luo et al. ( 2023 ) introduced butterfly optimization algorithm and opposition-based learning method based on elite strategy, adaptive nonlinear inertia weight strategy and random walk law to improve the shortcomings of slow convergence speed and low accuracy of GWO algorithm when dealing with high-dimensional complex problem. To address the issue of premature convergence encountered by the classic GWO in some situations due to the stagnation of sub-optimal solutions, Gupta et al. introduced an enhanced leadership-inspired grey wolf optimizer for global optimization problems (GLF-GWO)(Gupta and Deep 2020 ), Addressing the issues of slow convergence speed and insufficient global exploration in GWO, which can lead to settling in local optimal states and failure to achieve global optimal solutions, Singh et al. proposed a novel mutation-driven modified grey wolf optimizer (MDM-GWO) (Singh and Bansal 2022b ). MDM-GWO integrates new update search mechanisms, modified control parameters, mutation-driven schemes, and greedy selection methods into the search process of GWO. Addressing the issues of slow convergence speed and susceptibility to local optima in the Grey Wolf Optimizer (GWO) algorithm, Zhang et al. proposed a nonlinear control parameter strategy based on a sinusoidal function (GWO-SIN) and a nonlinear control parameter combination strategy (GWO-COM) (Zhang et al. 2019 ).
Soliman et al. ( 2022 ) proposed a novel hybrid African vultures–grey wolf optimizer (AV–GWO) approach to precisely estimate the electrical parameters of such TDM. Nadimi-Shahraki et al. ( 2021 ) introduced an enhanced variant of the Grey Wolf Optimization algorithm, termed I-GWO. The algorithm, based on a dimensionally learned hunting and searching (DLH) strategy, uniquely constructs hunting domains for each Wolf and enables them to share information about neighboring domains with each other. This enhances the algorithm's local and global search capabilities for more balanced performance, while also helping to maintain population diversity. A. Abushawish and A. Jarndal (Abushawish and Jarndal 2021 ) jointly proposed a new hybrid algorithm named GWO-CS that combines the advantages of Cuckoo Search (CS) algorithm and GWO algorithm. This algorithm primarily incorporates the position update equation from the CS to further refine the global search process of the GWO. Aimed at solving the problems of poor stability and easily falling into the local optimal solution in the grey wolf optimizer (GWO) algorithm, Liu et al. proposed an improved GWO algorithm based on the differential evolution (DE) algorithm and the OTSU algorithm is proposed (DE-OTSU-GWO) (Liu, Sun, Yu, Wang, & Zhou, 2020). The multithreshold OTSU, Tsallis entropy, and DE algorithm are combined with the GWO algorithm. The multithreshold OTSU algorithm is used to calculate the fitness of the initial population. The population is updated using the GWO algorithm and the DE algorithm through the Tsallis entropy algorithm for crossover steps. Multithreshold OTSU calculates the fitness in the initial population and makes the initial stage basically stable. Tsallis entropy calculates the fitness quickly. The DE algorithm can solve the local optimal solution of GWO. To address the issues of GWO's susceptibility to local optima and its low exploration capabilities, Hardi Mohammed et al. proposed the Enhanced GWO (EGWO) (Mohammed et al. 2024 ). EGWO employs diverse methods to improve the performance of GWO, utilizing gamma, z-position, and the golden ratio.
Liu et al. ( 2022 ) introduced a novel improvement strategy for the GWO algorithm, known as the exponential convergence factor improvement strategy. This strategy is designed to more accurately simulate the actual search process of grey wolves. It incorporates dynamic weighting factors and enhances control parameters to reduce the likelihood of the GWO algorithm getting stuck in local optima. However, despite these improvements, experimental findings indicate that GWO still faces challenges in accurately handling high-dimensional functions. Şenel et al. ( 2019 ) integrated a differential disturbance operator into the GWO algorithm. This addition brought an element of exploration into the exploitation phase, thereby enhancing the GWO algorithm's overall optimization capabilities. Jangir and Jangir ( 2018 ) proposed a multi-objective version of the GWO algorithm, named NSGWO. This algorithm utilizes a crowding distance mechanism to select the optimal solution from a set of Pareto optimal solutions. This approach helps guide the search towards the dominant region in multi-objective search spaces. NSGWO was tested on a variety of standard unconstrained, constrained, and engineering design challenges, demonstrating its efficiency and effectiveness in diverse optimization scenarios.
3 Methodology Overview: Standardized GWO and Proposed Enhancements
This section offers an overview of the hunting behavior and the mathematical model that forms the foundation of the original GWO. Additionally, we introduce the IAGWO, our proposed enhancement to GWO. IAGWO integrates the PSO search mechanism, the IMF strategy for inertia weighting, and an adaptive strategy for updating positions. These additions aim to refine and boost the efficiency of the original GWO algorithm.
3.1 The standardized GWO
3.1.1 inspiration of grey wolf packs’ hunting activity behavior.
The GWO algorithm draws inspiration from the hunting behavior of grey wolf packs. It mathematically simulates the way a group of grey wolves hunts, encircles, and targets their prey while adhering to a well-defined social hierarchy. In this hierarchy, the pack is led by three primary wolves: the Alphas ( α ), Betas ( β ), and Deltas ( δ ), each playing a crucial role in guiding the pack's movements and decisions. These wolves are considered the leaders, showcasing significant leadership abilities. Below them are the Omega ( w ) wolves, who occupy a subordinate role and follow the directives of the leading wolves. This hierarchical structure, integral to the functioning of the GWO algorithm, is depicted in Fig. 1 .

Hierarchy of the grey Wolf Pack
3.1.2 Mathematical model: GWO
GWO simulates grey wolf leadership and hunting mechanisms by dividing grey wolves based on their characteristics into a leader, α , who rules over the entire grey wolf; a facilitator, β , who helps α to make decisions and replaces α when α dies; and an enforcer, δ , who follows α 's and β 's orders (Fan and Yu 2022 ). GWO searches for excellence by modeling the wolf hunting process. In addition to the social hierarchy of wolves, group hunting is another interesting social behavior of grey wolves. The main phases of grey wolf hunting are as follows: The Grey Wolf Optimizer algorithm mimics the hunting behavior of grey wolf packs. Initially, in the "tracking, chasing, and approaching prey" phase, each wolf searches for potential solutions in the solution space and adjusts its position through certain search strategies to get closer to possible candidate solutions. Subsequently, in the "chasing, surrounding, and harassing prey until it stops moving" phase, the wolf pack collaborates to try to corner the prey into a smaller area and prevent its escape, involving behaviors such as encircling and harassing the prey to prevent its escape. Finally, in the "attacking prey" phase, once the prey is cornered and unable to escape, the wolves concentrate their attack on the prey, gradually optimizing the position of candidate solutions through strategies such as linear or leap searches until finding the optimal solution or meeting specific optimization criteria. These three phases represent the Grey Wolf Optimization algorithm's process of searching, chasing, and optimizing in the solution space, analogous to the behavior of a grey wolf pack during hunting, progressing from search to attack, gradually optimizing and approaching the optimal solution.
Now, this paper shows the calculation steps of the basic grey Wolf Optimization algorithm and the pseudo-code as follows (Algorithm 1). The GWO algorithm process is as follows:
1) Each member is initialized using Eq. ( 1 ), determine the population size N , the maximum number of iterations M , the single grey wolf dimension dim , and ɑ , A and C ;
where, LB and UB are the lower and upper boundaries of the solution space, respectively. X represent the positions of the current solution. phi is a random number between [0,1].
2) Calculate the fitness value of each individual using the test function. Then, based on the magnitude of the fitness values, select the best-fit individual as the α -wolf, the second-best individual as the β -wolf, and the third-best individual as the δ -wolf;
3) The mathematical model of Wolf pack leader tracking prey is shown in Eq. ( 2 ), which calculates the traction direction of the entire pack according to the distance difference between the Wolf leader and the pack, that is, the movement direction information of the pack, can be calculated as shown in Eqs. ( 3 ) and ( 4 ). Update the current grey wolf position according to Eqs. ( 2 )– ( 4 ).
where D α , D β , and D δ denote the distance difference between α -wolf, β -wolf, and δ -wolf and other individuals, respectively. X α , X β , and X δ indicate the current positions of α -wolf, β -wolf, and δ -wolf respectively, X indicate the current positions C 1 , C 2 , and C 3 satisfy the constraints of Eq. ( 6 ). A 1 , A 2 , and A 3 are random vectors satisfying the constrain of Eq. ( 5 ); X 1 , X 2 and X 3 are the traction directions of the three leading wolves; and X ( t + 1) represents the next collective movement position of the wolf pack. As shown in Fig. 2 , the final orientation of the wolves in the search space will be randomly positioned within a circle defined by the locations of the α , β , and δ in the search space. This graphical representation illustrates how the wolves’ positions influence the movement and direction of the entire pack in the pursuit of their prey.
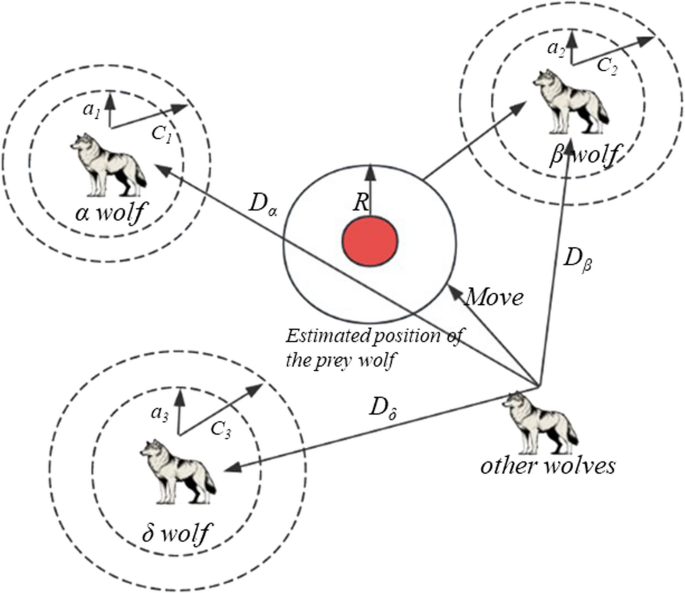
Position update of wolf groups in GWO algorithm
4) Update ɑ , A and C according to Eqs. ( 5 )–( 7 );
where, the parameter ɑ plays a crucial role in balancing global search and local exploration. Its value is set to decrease linearly from 2 to 0 over the course of the algorithm's iterations. Initially, a higher value of ɑ aids in the global convergence of the algorithm, guiding the wolf pack swiftly towards the region where the optimal solution might be found. As the algorithm progresses through its later iterations, the gradual decrease in the value of ɑ facilitates more refined exploration in the area of the optimal solution. This helps improve the convergence accuracy of the GWO algorithm, ensuring a more precise final result. r 1 and r 2 are random vectors and r 1 , r 2 ∈ [0, 1].
5) Update the positions of other individuals, calculate the updated fitness value based on the new position, and update the α -wolf, β -wolf, δ -wolf and global optimal solution, \(R\) represents the position vector of the optimization target;
6) Judge whether the specified stopping condition is reached (e.g., the maximum number of iterations is reached), if not, repeat steps 2 to 5. Otherwise, output the optimal result: the position of the α -wolf obtained at the end is the optimal solution, and the corresponding fitness value is the degree of superiority or inferiority of the optimal solution.
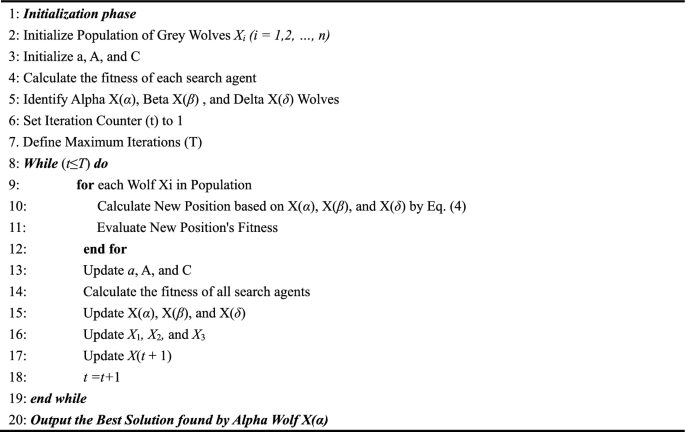
3.2 Improved grey wolf optimization algorithm
3.2.1 pso search mechanism.
The GWO (Grey Wolf Optimizer) exhibits a weak exploratory capability in its early stages and lacks diversity within the population, consequently resulting in suboptimal solution quality. In order to enhance exploration capabilities, improve population diversity (Hu et al. 2022 ), and increase the quality of solutions (Hakli and Kiran 2020 ), this study integrates the PSO, introducing a velocity concept to provide a new search mechanism for the GWO. The individual grey wolves are updated in terms of position during the early iterations, and the application in the velocity update introduces additional randomness. This prevents the algorithm from converging prematurely and encourages exploration of new areas, thereby increasing population diversity. By dynamically adjusting the velocity and position of each individual, this method may help in more effectively balancing global exploration and local exploitation. Leading to a wider search in the early stage of the iteration, this assists in identifying potential high-quality solutions. The computation Eq. ( 8 ) is as follows:
where t represents the current number of iterations, X and \({X}_{best}\) represent the positions of the current solution and the best-performing solution, respectively. \({v}_{rand}\left(t\right)\) is the velocity vector of the current solution at time t of iteration. phi is a random number between [0,1]. \({X}_{selfbest}\) is the best position vector in the history of the current solution.
In this study, at the start of each iteration, a PSO updating strategy is employed, along with the addition of extra randomness to stimulate a more extensive global search. This approach helps avoid local optimization and increases population diversity. This approach not only accumulates a more diverse and high-quality search experience for the GWO but also more effectively balances global exploration and local exploitation by dynamically adjusting the search behavior.
3.2.2 IMF inertia weighting strategy
Inverse Multiquadric Function is a decreasing function based on the principle of inverse multiple squares. It is often used as a regularization method in neural networks, such as a kernel function in support vector machines (Hu et al. 1998 ; Rathan et al. 2023). In accordance with the characteristics of the IMF, this paper incorporates it into the population position update mechanism within the framework of the GWO as delineated in Eq. ( 3 ). The IMF inertia weight ω , along with the revised formulae for the wolf pack updating process, are elucidated in Eqs. ( 9 )–( 10 ).
where, the parameter groups [a, b, c, d] are taken as [0.6, 0.02, 0.05, 0.3] and the graph of ω is shown in Fig. 3 . As indicated by Fig. 3 , during the early to mid-phases of the algorithm's iteration, the inertia weight ω is set to a higher value. This larger influence of the α -wolf, β -wolf, and δ -wolf on the updated positions is beneficial for the pack to quickly converge towards the optimal solution, effectively preventing the waste of search resources due to blind searching and thus enhancing the quality of the pack. As the development progresses to the mid and late stages and the pack becomes densely concentrated, if the higher-ranking wolves get trapped in a local optimum, the lower-ranking wolves led by them are also unable to escape this local optimum. At this juncture, the value of ω should be reduced to a lower level, thereby enlarging the pack's autonomous search capability and avoiding premature convergence.
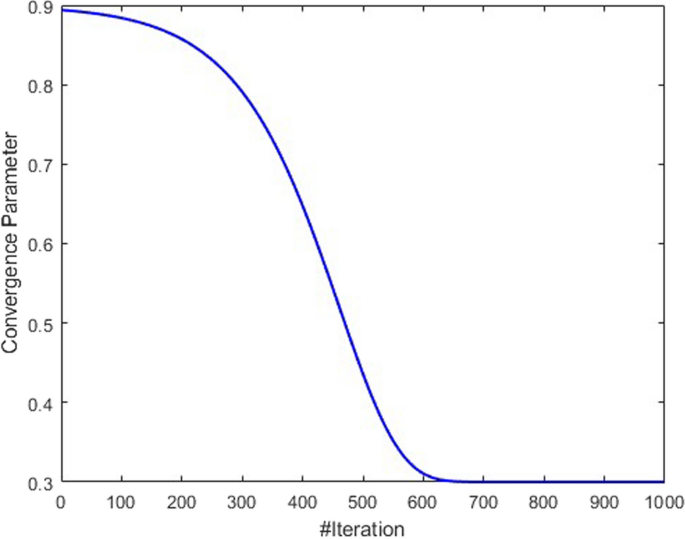
IMF Inertia Weight Graph
3.2.3 Adaptive updating mechanism
The population updating mechanism based on IMF inertia weight effectively reduces the density of population clustering to a certain extent. However, due to the intrinsic dynamics of the GWO, the newly generated wolf packs are still inevitably concentrated and migrate towards the positions directed by the α -wolf, β -wolf, and δ -wolf during the iterative process. In response to this, the present study defines the aggregation coefficient as the ratio of an individual's fitness value to the average population fitness value, which serves to quantify the degree of divergence between the current solution and the optimal solution. In minimization problems, the smaller the fitness value, the better the solution. A smaller aggregation coefficient indicates a more favorable current solution, thus allowing for minor updates in the vicinity of the individual's current position. Conversely, a larger aggregation coefficient suggests a poor location of the individual, warranting a significant perturbation to facilitate a jump to other positions. Based on this analysis, this paper introduces a Sigmoid function to construct the adaptive updating amplitude of the population under different aggregation coefficients, as depicted in Eqs. ( 11 )-( 12 ).
where f i represents the fitness value of the i th individual, and f ave denotes the average fitness value of the population. θ is the exponential coefficient, which is taken as 0.5 in this paper.
In comparison to the standard GWO, the IAGWO brings several significant advancements. Firstly, it introduces a novel search mechanism by incorporating velocity concepts. This addition helps in preventing premature convergence and allows for a more thorough exploration of the search space. The integration of velocity updates also adds an element of randomness, which in turn increases the diversity within the population of solutions. Moreover, the implementation of the IMF inertia weight strategy in IAGWO improves the balance between exploring the global search space and exploiting local solutions. This strategic enhancement significantly boosts the convergence speed of the algorithm. Furthermore, IAGWO differentiates itself from the standard GWO through its adaptive updating mechanism. This mechanism combines the aggregation coefficient with the Sigmoid function, enhancing the algorithm's ability to switch between broad search patterns and detailed solution refinement. This results in improved performance in maintaining diversity and achieving faster convergence rates. This adaptive approach enables IAGWO to search and optimize more efficiently within the solution space of the problem. For an in-depth comprehension of the workings of IAGWO, the procedural flow is visually depicted in Fig. 4 , its pseudocode is meticulously detailed in Algorithm 2, and the proposed IAGWO workflow(Chauhan & Yadav 2023b ) is shown in Fig. 5 .
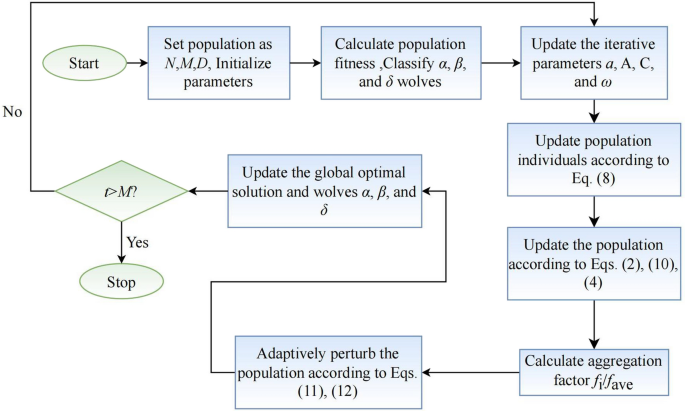
Implementation Process for IAGWO
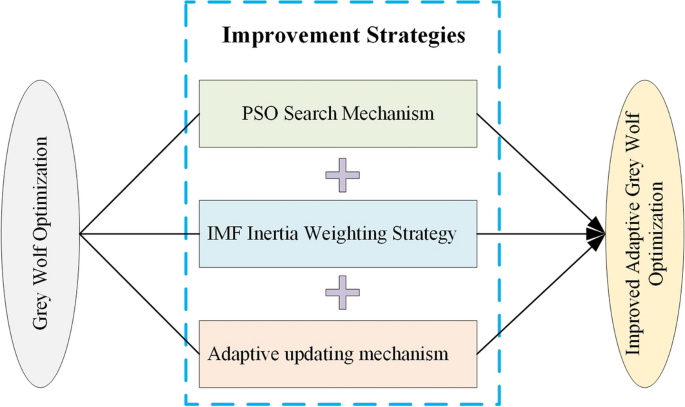
Working procedure of the proposed IAGWO algorithm
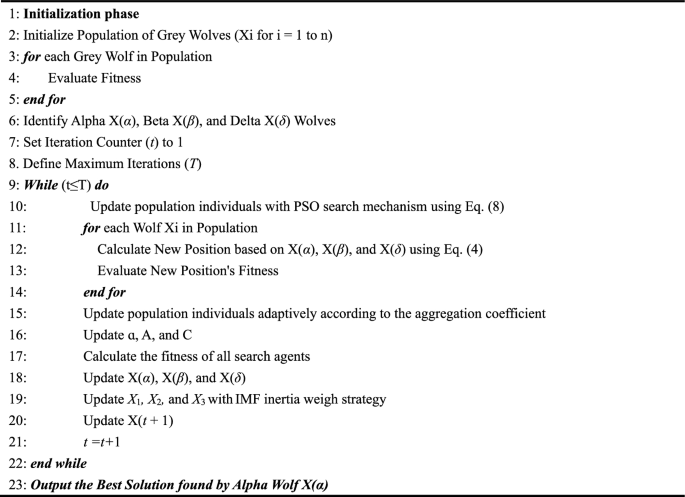
3.3 Time complexity analysis
CEC17 (Competition on Evolutionary Computation) defines algorithm complexity as a measure of the computational resources required by an algorithm to solve a given problem instance. This section explains the computational complexity of IAGWO. The complexity of IAGWO is primarily influenced by two main factors: the initialization of solutions and the execution of the algorithm's core functions. These core functions involve calculating fitness functions and updating solutions. The computational complexity is determined by considering several variables: the count of solutions \(\left(N\right)\) , the upper limit of iterations \((T)\) , and the problem's dimension \((D)\) being tackled. Specifically, the complexity of initializing solutions in the IAGWO algorithm can be represented as \(O(N)\) , indicating the order of complexity in relation to the number of solutions. This gives an understanding of the computational resources required for the initial setup phase of the algorithm. This means that as the number of solutions \(N\) increases, the computational complexity of the initial solution of the algorithm will also increase accordingly. This represents the order of complexity for the initial setup phase of the algorithm. The time complexity of the original GWO algorithm is \(O(T\times N\times D)\) . IAGWO modifies this with Eqs. ( 8 ), ( 9 ), and ( 10 )–( 11 ), including enhancements to population diversity using the PSO position updating strategy, integration of IMF weights to reduce the excessive influence of higher-level wolves on lower-level ones, and the introduction of a population adaptive update based on the sigmoid function. The PSO position updating strategy requires calculations for each individual and each dimension, with a complexity of \(O(T\times N\times D)\) . The update from Eq. ( 10 ) is independent of population size and search dimensions, correlating only with the maximum number of iterations, resulting in a time complexity of \(O(T)\) . The time complexity for Eq. ( 11 ) is \(O(N\times D)\) . Consequently, the overall time complexity of IAGWO is \(O(\text{IAGWO})=O\left(T\times N\times D\right)+O\left(T\right)+O\left(N\times D\right)=O(T\times N\times D)\) , consistent with the original algorithm.
4 Results and comprehensive analysis
The simulation for this study was carried out on a Windows 11 platform, operating on a 64-bit system. The analysis was performed using MATLAB 2023b, running on a machine equipped with an AMD Ryzen 7 4800H CPU at 2.30 GHz and 16 GB of RAM.
4.1 Test functions and parameter settings
In this paper, the CEC 2017 (Dim = 30) (Mallipeddi and Suganthan 2010 ), CEC 2020 (Dim = 10 and 20) (Liang et al. 2019 ), and CEC 2022 (Dim = 10 and 20) (Ahrari et al. 2022) test suites were employed to evaluate the performance of the proposed IAGWO algorithm. The test suite for evaluating algorithms covers four different functional types: single-modal, multimodal, mixed, and combined. These different types of test suites are designed to comprehensively evaluate the performance and applicability of algorithms. Additionally, for assessing the scalability of the IAGWO algorithm, we employed the CEC 2013 Large-scale Global Optimization suite (800-dimensional) for simulation analysis (Li et al. 2013 ). The suite contains 15 highly complex reference functions that are grouped into four groups: fully separable, partially additively separable, overlapping, and completely indivisible. These different types of benchmark functions provide a comprehensive experimental framework for evaluating the scalability of optimization algorithms, so that we can more accurately evaluate the performance of IAGWO algorithm on different types of problems.
4.2 Comparison with other algorithms and parameter settings
The performance of the Improved Adaptive Grey Wolf Optimization (IAGWO) is benchmarked against 12 well-known algorithms, grouped into three categories for comparison:
High-citation algorithms: These include the Gravitational Search Algorithm (GSA) (Rashedi et al. 2009 ), Dolphin Echolocation Optimization (DMO) (Kaveh and Farhoudi 2013 ), Whale Optimization Algorithm (WOA) (Mirjalili and Lewis 2016 ), and Harris Hawks Optimization (HHO) (Tripathy et al. 2022 ).
Advanced algorithms: This category includes Combined Particle Swarm Optimization and Gravitational Search Algorithm (CPSOGSA), Crow Optimization Algorithm (COA) (Jia et al. 2023 ), African Vulture Optimization Algorithm (AVOA) (Abdollahzadeh et al. 2021 ), Optical Microscope Algorithm (OMA) (Cheng and Sholeh 2023 ), and Adaptive Artificial Electric Field Algorithm (iAEFA) (Chauhan and Yadav 2023a ).
GWO and its variants: This includes the original Grey Wolf Optimization (GWO), the Adaptive GWO (AGWO) (Meidani et al. 2022 ), the Enhanced GWO (ENGWO)(Mohammed et al. 2024 )and the Revised GWO (RGWO) (Banaie-Dezfouli et al. 2021 ).
Table 1 offers a comprehensive summary of the parameters for 14 different MH algorithms. For each of these algorithms, 30 independent runs were conducted, with each run limited to a maximum of 500 iterations and population size is set to 30 and a maximum of 30,000 evaluations. The outcomes of these runs were meticulously recorded, capturing the average values (denoted as Ave) and the standard deviations (Std) for each algorithm. To facilitate an easy comparison of their performance, the table highlights the best results among these 14 algorithms by formatting them in bold text. This highlighting method provides a clear visual indicator of which algorithms performed most effectively under the given testing conditions.
4.3 Qualitative assessment of IAGWO
4.3.1 exploring convergence patterns.
To verify the convergence performance of IAGWO, we plotted its convergence performance evaluation on the 30-dimensional CEC2017 test functions, as shown in Fig. 6 . It presents the corresponding results of different test functions in the form of nine images involving three instances selected from the same suite. In the presentation of images, the first column distinctly illustrates the two-dimensional profiles of the reference functions being analyzed. The visuals presented in the first column accurately depict the characteristics and contours of each function being optimized. These graphical representations offer a clear understanding of the challenges and intricacies of each function. In the second column, images depict the final positions of the search agents at the end of the optimization process. Within these visuals, the optimal solution's location is distinctly marked with a red dot. This not only illustrates the end point of the search agents' journey but also visually highlights the spot where they successfully identified the most favorable solution. This layout effectively communicates the results of the optimization process, making it easier to comprehend the behavior and efficacy of the search agents in navigating the solution space. This layout offers a clear and informative view of both the nature of the functions and the outcomes of the optimization process. By observing the second column of images, we can clearly find that the search agent is close to the optimal solution in most cases, which fully reflects the powerful ability of IAGWO algorithm in the process of exploration and development. In addition, the third column image accurately tracks the change of the average fitness value during the iteration. Initially high, these values decrease and stabilize after 100 iterations, albeit with minor fluctuations. These fluctuations are normal in complex optimization problems, indicate ongoing detailed searches for improvement and the maintenance of population diversity to prevent premature convergence to local optima. The fourth column reveals the search agents' trajectories in the first dimension, there were marked fluctuations in the early iterations, which then leveled off, and then fluctuations again at intervals, which leveled off again, signifying a balance between exploration and exploitation. Finally, the convergence curve, smooth for unimodal functions, suggests optimal values are achievable through iteration. For multimodal functions, however, the step-like curve reflects the need for continual avoidance of local optima to reach global optima. These four metrics collectively affirm IAGWO's robust convergence.
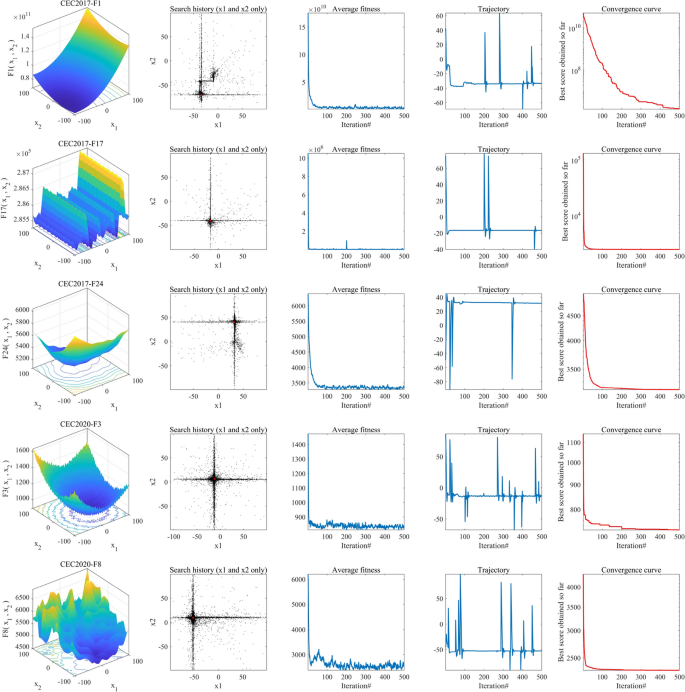
The convergence behavior of IAGWO
4.3.2 Analyzing the diversity of population
In optimization algorithms, the importance of population diversity is a matter of balance. Moderate population diversity can help the algorithm avoid falling into local optima, thereby increasing search space coverage and global search capability, improving convergence speed, and the quality of optimization results. However, excessive population diversity may lead to overly dispersed search, making it difficult for the algorithm to explore local regions deeply, thereby reducing convergence speed and the quality of final solutions. Therefore, when designing optimization algorithms, it is necessary to consider a balance between population diversity and search efficiency. This can be achieved through appropriate parameter settings or suitable strategies to maintain population diversity, thus effectively solving optimization problems. A population with high diversity indicates significant differences among individuals, allowing for broader exploration in the search space and avoiding premature convergence to local optima. Hence, maintaining good population diversity is a crucial objective in metaheuristic algorithms. Typically, we use Eq. ( 13 ) and Eq. ( 14 ) to measure the population diversity of the algorithm. This calculation method was proposed by Morrison in 2004. Where, \({I}_{C}\) represents the moment of inertia, \({x}_{id}\) denotes the ith search agent's value in the \({d}^{th}\) dimension at iteration t . Furthermore, \({c}_{d}\) represents the spread of the population from its center of mass, denoted by ' c ', in every iteration, as illustrated in Eq. ( 14 ) (Fu et al. 2023a , b).
Figure 7 displays the comparative experimental outcomes regarding population diversity for both IAGWO and GWO. The measurement of population diversity is conducted through \({I}_{C}\) . Observations from Fig. 7 reveal that IAGWO demonstrates an initial marked increase in diversity during the early phases of iteration, which then transitions to a state of relative stability at an elevated level. This indicates an increase in the variance among individuals within the IAGWO population during the early iterations, effectively exploring a vast search space. As iterations progress, the population diversity tends to stabilize, which aids in averting premature convergence to local optima. The minor fluctuation are normal and beneficial for the algorithm to adapt to dynamically changing search spaces and prevent premature convergence. In contrast, GWO shows insufficient population diversity, highlighting IAGWO’s effectiveness in maintaining diversity, crucial for exploring complex search spaces and avoiding local optima. These experimental outcomes demonstrate IAGWO's substantial potential in optimization.
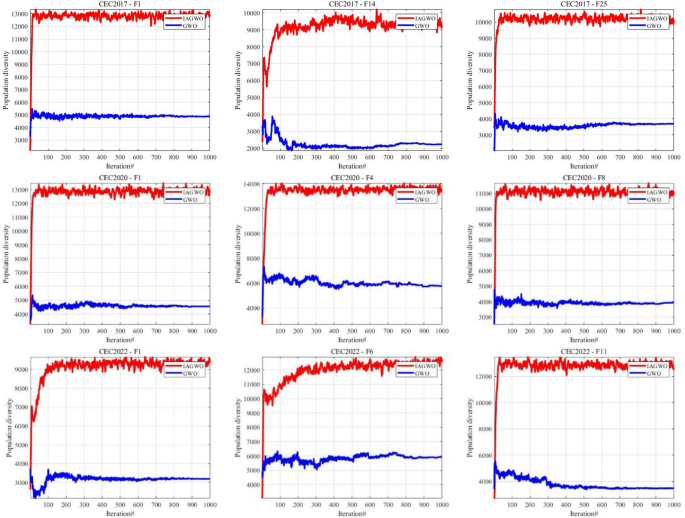
The population diversity of IAGWO and GWO
4.3.3 Exploration and exploitation analysis
In optimization algorithms, managing the balance between exploration and exploitation is key for optimal performance (Saka et al. 2016 ). Exploration involves searching through the solution space, while exploitation focuses on refining known good solutions. This section deals with quantifying the extent of exploration and exploitation in the algorithm. To do this, we use Eq. ( 15 ) to calculate the percentage of exploration and Eq. ( 16 ) for the percentage of exploitation. Additionally, the parameter \(Div\left(t\right)\) used for measuring dimension diversity is calculated using Eq. ( 17 ). The parameter \({\rm Div}_{max}\) reflects the peak diversity noted throughout the entire course of iterations,, which is essential for understanding how broadly and effectively the algorithm explores the solution space(Li et al. 2023 ) (Nadimi-Shahraki et al. 2023 ).
Figure 8 depicts the results of the experiments conducted. It shows that for various function types, as the number of iterations progresses, GWO consistently demonstrates a higher rate of exploration and a comparatively lower rate of exploitation. In contrast, IAGWO shows a changing pattern, with exploration decreasing and exploitation increasing as iterations progress. This observation suggests that GWO tends towards a broad search across the entire space, with less focus on local search and weaker performance in thoroughly exploiting the optimal regions found. In comparison, IAGWO demonstrates the ability to dynamically adjust its search strategy. This implies that the algorithm initially identifies potential good solution areas through extensive exploration and then finely tunes these solutions in the later stages through focused exploitation, potentially enhancing both the efficiency of the algorithm and the quality of solutions. Overall, while GWO shows commendable exploration capabilities, it lacks effective exploitation. In contrast, IAGWO effectively strikes a balance between exploration and exploitation. This balance is well-maintained across a variety of benchmark functions, showcasing IAGWO's adaptability and efficiency in different optimization scenarios. This attribute is particularly important as it ensures the algorithm can thoroughly search the solution space while also honing in on the most promising solutions.
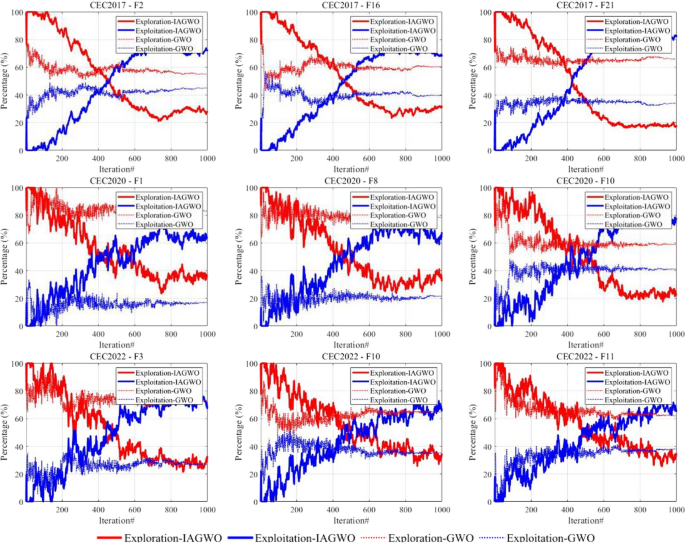
The exploration and exploitation of IAGWO and GWO
4.3.4 Ablation experiments
In this section, a detailed analysis is conducted on the impact of three proposed improvement strategies on the GWO. These strategies include the PSO position updating mechanism, the introduction of IMF inertia weight strategy, and the adoption of a Sigmoid adaptive updating strategy. Based on these improvements, three new algorithm variants are named: PGWO for the PSO search mechanism, IGWO for the IMF inertia weight, and SGWO for the Sigmoid adaptive updating strategy. According to the experimental results in Fig. 9 , all three strategies significantly enhance the convergence accuracy and speed of GWO, with IAGWO showing particularly notable performance.
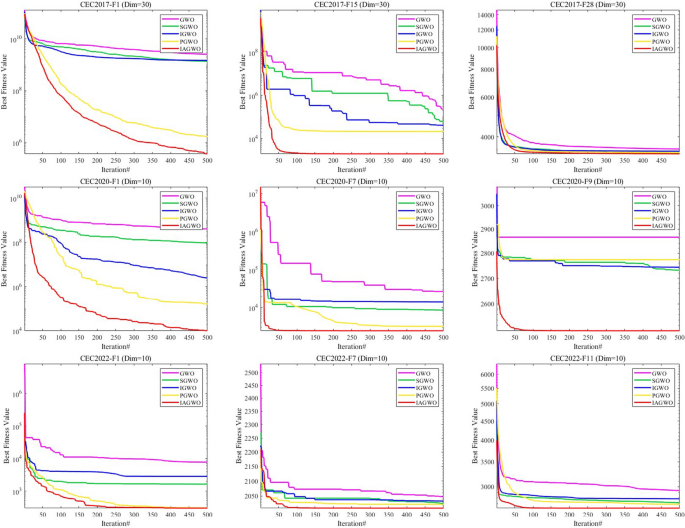
Comparison of different improvement strategies
Specifically, when dealing with unimodal and multimodal functions, the results of PGWO and IAGWO are relatively consistent, showing a more significant improvement over GWO compared to SGWO and IGWO. However, when dealing with more complex hybrid modal functions, the enhancement of PGWO on GWO diminishes, while the IAGWO algorithm, integrating all three strategies, continues to exhibit exceptional optimization performance. Overall, the IAGWO algorithm successfully overcomes challenges of local optima and premature convergence, significantly boosting the algorithm's convergence speed and accuracy. These findings provide valuable insights for the further development and application of the GWO.
4.4 Quantitative evaluation
In this section, the efficacy of IAGWO is scrutinized using a series of test suites: CEC 2017, CEC 2020, and CEC 2022. Moreover, its proficiency in handling large-scale problems is assessed with the CEC 2013 suite. To clearly compare performance, the best results among the algorithms are highlighted in bold in the tables. The parameters are standardized with a population size of 100, a maximum iteration limit of 500, and a total of 30 independent runs. The performance outcomes are systematically presented in Tables 2 to 7 , which illustrate the average values (Ave) and the standard deviations (Std) for each competing algorithm. A thorough statistical analysis is conducted to highlight the superiority of IAGWO. This includes an initial evaluation represented by three indicators (W|T|L) in the first line of the results, denoting the algorithms' performance as best (win), comparable (tie), or least effective (loss) for specific functions. The second row compiles the mean performance of all algorithms, while the third row offers insights into the overall standings through the final Friedman ranking. The tables distinctly highlight the top results, emphasizing their significance. Furthermore, the comparative analysis of the convergence curves for each algorithm is depicted in Fig. 10 . This visual representation aids in understanding the progression and efficiency of each algorithm in finding optimal solutions over the course of iterations. This detailed evaluation underscores the robustness and adaptability of IAGWO in varied optimization contexts.
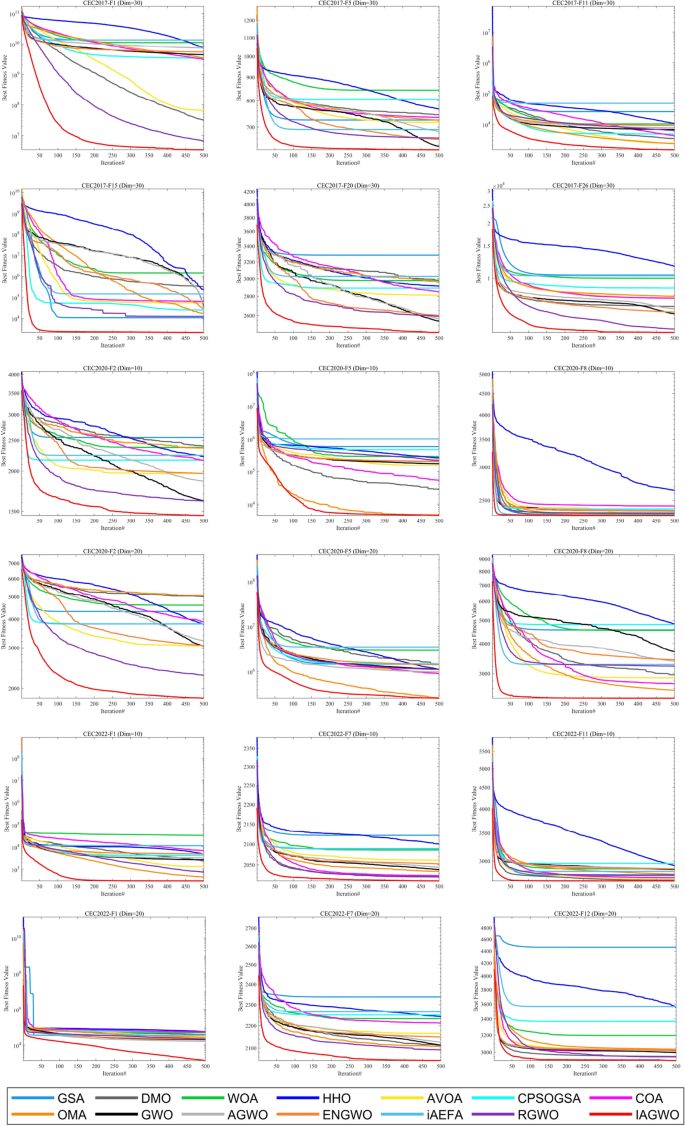
Convergence curves of different algorithms
4.4.1 Assessing performance with CEC 2017 test suite
This section examines the efficacy of IAGWO using the CEC 2017 test suite with a dimensionality of 30, as detailed in Table 2 . The results are quite telling: IAGWO recorded the highest number of best performances, leading in 16 out of the 30 functions tested. Notably, it did not register as the least effective in any of the functions. In terms of statistical standing, IAGWO's Friedman mean ranking is 3.00, earning it the top position. Further, a diverse range of functions from CEC 2017 (Dim = 30) were chosen for a more comprehensive evaluation. The comparative analysis of the convergence trends, depicted in Fig. 10 , reveals that IAGWO consistently achieved the quickest convergence rate and maintained the highest level of accuracy in convergence. These results underscore IAGWO's exceptional proficiency in both global exploration and local exploitation. Collectively, these findings solidify the effectiveness and superiority of IAGWO as an optimization tool.
4.4.2 Assessing performance with CEC 2020 test suite
This section is dedicated to evaluating 13 algorithms with the utilization of the CEC 2020 test suite, which includes tests with dimensions of 10 and 20. The outcomes of this evaluation are systematically presented in Table 3 and Table 4 . On the CEC 2020 tests, IAGWO mirrors the impressive results observed in the CEC 2017 suite, achieving the highest number of best performances while not being the least effective in any function. To provide a visual representation of these results, representative functions are chosen to illustrate the convergence curves, as depicted in Fig. 10 . IAGWO consistently shows the quickest convergence speed and the highest accuracy in convergence, reaffirming its efficiency. Additionally, it's important to note the contrasting performance of GWO on the CEC 2020 suite. Despite its lower ranking in the Friedman rankings, indicating a comparatively poor performance, its improved variants, namely AGWO, ENGWO, and RGWO, show marked improvements. Remarkably, RGWO secures the second-highest ranking, closely following IAGWO, underscoring the substantial research value in enhancing the GWO algorithm. A comprehensive statistical analysis among the 13 algorithms tested places IAGWO at the forefront in the Friedman rankings. This achievement highlights its superiority not only over the original GWO but also over other well-regarded algorithms. These results collectively demonstrate the robustness and effectiveness of IAGWO in a competitive algorithmic landscape.
4.4.3 Assessing performance with CEC 2022 test suite
This section is dedicated to a thorough evaluation of the proposed IAGWO and 12 other comparative algorithms, utilizing the CEC 2022 test suite. The primary objective of this evaluation is to gauge the exploration and exploitation capabilities of these algorithms and assess their proficiency in avoiding local optima traps. The experiments are conducted under 10-dimensional and 20-dimensional scenarios, with corresponding results displayed in Tables 5 and 6 , respectively. IAGWO ranks first in Friedman mean ranking in both dimensional settings, with ranking values of 1.75 and 2.25 respectively. Similarly, while GWO shows subpar performance, its variants enhance GWO's performance, emphasizing the research significance of GWO. The analysis of results depicted in Fig. 10 leads to a conclusive observation that IAGWO successfully evades getting stuck in local optima and avoids premature convergence. These findings serve not just as a testament to the excellence and robustness of IAGWO, but they also highlight its substantial performance benefits and the capability to yield enhanced solutions. This analysis underscores IAGWO's effectiveness in navigating complex optimization landscapes, further establishing its potential as a superior tool in optimization tasks.
4.4.4 Scalability evaluation using the CEC 2013 test suite
In real-world scenarios, solving optimization problems often requires adjusting multiple parameters at once. To test the scalability of the IAGWO for high-dimensional problems, we utilized the CEC 2013 suite for large-scale global optimization. The results of this testing are detailed in Table 7 . This suite includes 15 highly complex test functions, each with up to 1000 dimensions, providing a robust challenge for assessing algorithmic performance. In our experiments, IAGWO was compared with 12 other algorithms. The population size was fixed at 100, and we limited the maximum number of iterations to 10 for each run. After conducting 30 independent runs for each algorithm, IAGWO achieved a Friedman mean rank value of 2.63. This score signifies a higher level of performance relative to the other algorithms in the competition. The findings from these experiments demonstrate that the IAGWO algorithm has significant scalability, effectively handling complex, high-dimensional optimization challenges. This capability distinguishes IAGWO from other algorithms, highlighting its suitability for practical, large-scale optimization applications.
4.5 Wilcoxon rank sum test
This study utilizes the non-parametric Wilcoxon rank sum test (Wilcoxon 1945 ) to conduct comparative performance assessments of various algorithms, setting the significance level at 0.05. To succinctly represent the performance of IAGWO relative to its competitors, the symbols “ + / = /-” are used to denote whether IAGWO is superior to, equivalent to, or inferior to the competing algorithms. As shown in Table 8 , these statistical results clearly indicate significant performance differences between IAGWO and other competing algorithms in most cases. Specifically, the statistical data show the following comparative results: 344/0/46、119/0/11、111/13/6、150/0/6、152/0/4 and 175/0/5. The analysis presented above demonstrates that the IAGWO method, as introduced in this study, shows exceptional overall performance when compared to the traditional GWO and other rival algorithms, thereby underscoring its distinct advantages.
4.6 Time comparison analysis of IAGWO and GWO
Building on the findings from previous chapters, it's clear that IAGWO significantly surpasses the original GWO in terms of overall performance. In this section, we focus on a more detailed comparison, specifically looking at the computational costs of both algorithms, with a particular emphasis on the differences in computational time. To facilitate this comparison, we standardized the settings for both IAGWO and GWO. For this evaluation, the population size was configured to 50, the maximum iterations were limited to 1000, and each algorithm underwent 30 independent runs. Table 9 presents the total time (in seconds) each algorithm took to complete all 30 runs. This data provides a clear basis for comparing the efficiency of the two algorithms in terms of how long they take to execute, offering insights into their time-based performance efficiency.
Analysis of the experimental data on the CEC 2017 test suit (Dim = 30) indicates that under the same experimental parameters, IAGWO and GWO perform almost equally in terms of execution time on unimodal functions and some simpler multimodal functions, but when dealing with more complex multimodal and hybrid functions, IAGWO generally consumes significantly less computational time than GWO. This suggests that in handling highly complex problems, IAGWO demonstrates greater computational efficiency. Compared to the original GWO, IAGWO's improved search strategies are more efficient, possessing better global search capabilities or faster local convergence speeds. Overall, IAGWO not only excels in benchmark tests but also exhibits higher computational efficiency and better adaptability when addressing more complex optimization problems that may arise in practical applications.
However, on the CEC 2020 test suite (Dim = 10 and 20) and CEC 2022 test suite (Dim = 10 and 20), IAGWO generally exhibits higher computational times compared to GWO. This may indicate that the types of problems or characteristics included in CEC 2020 are not entirely compatible with the strategies of IAGWO, leading to a higher computational load.
4.7 Evaluating performance against CEC 2014 and CEC 2017 competition-winners
This section evaluates the performance of the proposed IAGWO using the CEC 2014 test suite with a dimensionality of 30 (Liang et al. 2013 ) and CEC 2017 (Dim = 30) test suites. Additionally, we compare the performance of IAGWO with the competition-winners of these two suites in previous CEC competitions, including L-SHADE (Tanabe & Fukunaga 2014 ) and AL-SHADE (Li et al. 2022 ) from CEC 2014, and LSHADE-SPACMA (Mohamed, Hadi, Fattouh, & Jambi, 2017) and LSHADE-cnEpSin (Awad, Ali, & Suganthan, 2017) from CEC 2017. In the experimental setup, the population size is fixed at 30, the maximum iterations are limited to 500, and a total of 30 independent runs are performed.
Table 10 presents the results from testing IAGWO using the CEC 2014 suite. In these tests, IAGWO surpassed other algorithms in six different scenarios, though it showed slightly weaker performance in one. Notably, IAGWO achieved a Friedman mean ranking value of 1.71, which places it second after L-SHADE but ahead of AL-SHADE. Table 11 focuses on the performance of IAGWO in the CEC 2017 suite. Here, IAGWO showed strong results in 8 of the test cases, but its performance was less impressive in 10 others. In terms of the Friedman mean ranking, IAGWO scored 1.99, which is slightly better than LSHADE-SPACMA, but not quite as good as LSHADE-cnEpSin. These results provide a detailed comparison of IAGWO's performance relative to other algorithms in these specific test environments.
Combining experimental outcomes, IAGWO can be positioned as a high-performing optimizer in test functions. These results not only demonstrate IAGWO's strong capability in handling different types of optimization problems but also indicate its competitive standing against existing top-tier algorithms. These findings emphasize the potential application value of IAGWO in the field of evolutionary computing and optimization. This simultaneously demonstrates the effectiveness of the three improvement strategies we introduced: the PSO Search Mechanism, the IMF Inertia Weighting Strategy, and the Adaptive Updating Mechanism, enhancing the optimization performance of the algorithm.
4.8 IAGWO for 19 engineering design challenges
The specific constrained handling technique used in engineering design challenges is called "constraint relaxation." Constraint relaxation involves temporarily easing or loosening certain constraints within the design problem to explore alternative solutions. This allows designers to generate a wider range of potential solutions without being overly restricted by strict constraints. Once various solutions have been identified, designers can then reintroduce and refine the constraints to ensure that the final design meets all necessary requirements. Intelligent optimization algorithms can efficiently explore the design space and uncover potential solutions. By integrating constraint relaxation techniques, these algorithms can dynamically handle constraints during the search process, allowing for a broader exploration of the design space and enhancing the efficiency of finding optimal solutions.
In this section, the proficiency of IAGWO is meticulously evaluated through a set of 19 engineering design challenges (EDC) sourced from the CEC 2020 real-world optimization benchmarks, as outlined by Kumar et al., 2020., 2020. A concise summary of these engineering challenges is presented in Table 12 , which includes key details such as their dimensions ( D ), the count of inequality constraints ( g ), equality constraints ( h ), and the known optimal cost ( f min ). The evaluation parameters are defined as follows: a population size of 50, a maximum of 1000 iterations, and 30 independent runs for each challenge.
Table 13 is dedicated to enumerating the performance metrics of IAGWO. This table encompasses various metrics including the best cost achieved (Best), the average cost (Ave), the cost's standard deviation (Std), and performance symbols (W|T|L), representing the number of wins, ties, and losses, respectively. Additionally, the evaluation includes a comprehensive analysis of the mean performance of all the algorithms involved in the testing. It also presents a ranking of these methods, providing a clear and structured comparison of their overall effectiveness and highlights instances where IAGWO achieves optimal results.
The statistical analysis drawn from these results clearly demonstrates IAGWO's superior ability in solving these real-world engineering design challenges, effectively outshining other methods. In terms of overall effectiveness, other algorithms like OMA, DMO, RGWO, and ENGWO trail behind IAGWO. This comprehensive analysis accentuates the robustness and efficacy of IAGWO.
5 Summary and future directions
In this study, we introduced an enhanced version of the GWO, aiming to tackle its inherent limitations and elevate its efficacy for addressing contemporary optimization challenges. The original GWO, while promising, exhibited deficiencies, notably in its convergence speed and its adaptability to intricate, high-dimensional problem landscapes. To fortify its capabilities, we embarked on an innovative path, culminating in the birth of an enhanced variant dubbed the Improved Adaptive Grey Wolf Optimizer (IAGWO). Central to our enhancement strategy was the infusion of concepts borrowed from Particle Swarm Optimization (PSO), introducing a velocity component to expedite convergence. This integration of velocity mechanics injected dynamism into the algorithm, enabling it to traverse solution spaces with greater agility. Moreover, a novel search mechanism was devised, augmenting the algorithm's exploration and exploitation capabilities to navigate complex problem domains more efficiently. In addition to these fundamental alterations, we devised novel strategies for Inertia Weighting and Position Updating, leveraging Nonlinear Inertia Weighting for Intermediary Fitness (IMF) and employing Sigmoid adaptive techniques. These refinements were meticulously crafted to synergize with the core algorithm, amplifying its prowess in navigating diverse optimization landscapes with finesse.
To validate the prowess of IAGWO, rigorous experimentation ensued, wherein 52 test functions sourced from prestigious benchmark suites were scrutinized. Comparative analysis against eight prominent Metaheuristic (MH) algorithms, including the original GWO and three of its variants, underscored IAGWO's supremacy in terms of convergence speed and solution precision. Furthermore, the algorithm's mettle was tested against 15 formidable large-scale global optimization challenges, affirming its adeptness in grappling with high-dimensional complexities. The litmus test for IAGWO's efficacy extended to competitive arenas, where it stood toe-to-toe against previous champions of the renowned CEC competitions across various iterations. Notably, IAGWO's performance surpassed expectations, firmly establishing its dominance and resilience in the face of formidable adversaries. Beyond the realm of academia, the real-world applicability of IAGWO was validated through its deployment in 19 diverse engineering design challenges. Here, its versatility and competitive edge shone brightly, outperforming established algorithms and offering tangible solutions to practical problems.
Despite its commendable achievements, the journey of IAGWO is far from over. While it has emerged as a potent force in the optimization landscape, ongoing efforts are directed towards fine-tuning its computational efficiency. Time comparison analyses have revealed areas for optimization, particularly concerning computational overhead in certain test suites. Future endeavors will thus focus on streamlining computational complexity without compromising search efficacy, ensuring that IAGWO remains at the forefront of optimization methodologies. Looking ahead, the horizon for IAGWO is brimming with promise. Beyond academic benchmarks, its utility extends to a myriad of real-world applications, ranging from feature extraction to operations research, classification, and logistical challenges. As we embark on this journey, our aim is clear: to harness the full potential of IAGWO in unraveling the complexities of the modern world, one optimization problem at a time.
Availability of data and materials
Enquiries about data availability should be directed to the authors.
Abdel-Basset M, Mohamed R, Jameel M, Abouhawwash M (2023) Nutcracker optimizer: A novel nature-inspired metaheuristic algorithm for global optimization and engineering design problems. Knowl Based Syst 262:110248
Article Google Scholar
Abdollahzadeh B, Gharehchopogh FS, Mirjalili S (2021) African vultures optimization algorithm: A new nature-inspired metaheuristic algorithm for global optimization problems. Comput Ind Eng 158:107408
Abdullah JM, Ahmed T (2019) Fitness dependent optimizer: inspired by the bee swarming reproductive process. IEEE Access 7:43473–43486
Abualigah L, Yousri D, Abd Elaziz M, Ewees AA, Al-qaness MAA, Gandomi AH (2021) Aquila Optimizer: A novel meta-heuristic optimization algorithm. Comput Ind Eng 157:107250
Abushawish, A., & Jarndal, A. 2021. Hybrid GWOCS optimization based parameter extraction method applied to GaN devices. Paper presented at the 2021 IEEE International Midwest Symposium on Circuits and Systems (MWSCAS).
Ahrari, A., Elsayed, S., Sarker, R., Essam, D., & Coello, C. A. C. 2022. Problem Definition and Evaluation Criteria for the CEC’2022 Competition on Dynamic Multimodal Optimization. Paper presented at the Proceedings of the IEEE World Congress on Computational Intelligence (IEEE WCCI 2022), Padua, Italy.
Aldosari F, Abualigah L, Almotairi KH (2022) A normal distributed dwarf Mongoose Optimization Algorithm for global optimization and data clustering applications. Symmetry 14(5):1021
Ambika V, Lim S-J (2022) Hybrid image embedding technique using Steganographic Signcryption and IWT-GWO methods. Microprocess Microsyst 95:104688
Awad, N. H., Ali, M. Z., & Suganthan, P. N. 2017. Ensemble sinusoidal differential covariance matrix adaptation with Euclidean neighborhood for solving CEC2017 benchmark problems. In: Paper presented at the 2017 IEEE congress on evolutionary computation (CEC).
Bäck T, Schwefel H-P (1993) An overview of evolutionary algorithms for parameter optimization. Evol Comput 1(1):1–23
Banaie-Dezfouli M, Nadimi-Shahraki MH, Beheshti Z (2021) R-GWO: Representative-based grey wolf optimizer for solving engineering problems. Appl Soft Comput 106:107328
Bayraktar, Z., Komurcu, M., & Werner, D. H. 2010. Wind Driven Optimization (WDO): A novel nature-inspired optimization algorithm and its application to electromagnetics . Paper presented at the 2010 IEEE antennas and propagation society international symposium.
Biabani F, Shojaee S, Hamzehei-Javaran S (2022) A new insight into metaheuristic optimization method using a hybrid of PSO, GSA, and GWO. Structures 44:1168–1189
Chauhan, D., & Yadav, A. 2024b. A Comprehensive Survey on Artificial Electric Field Algorithm: Theories and Applications. Archives of Computational Methods in Engineering.
Chauhan D, Shivani, & Cheng, R. (2024) Competitive Swarm Optimizer: a decade survey. Swarm Evol Comput 87:101543
Chauhan D, Yadav A (2023a) An Adaptive Artificial Electric Field Algorithm for Continuous Optimization Problems 40(9):e13380
Google Scholar
Chauhan D, Yadav A (2023b) Optimizing the parameters of hybrid active power filters through a comprehensive and dynamic multi-swarm gravitational search algorithm. Eng Appl Artif Intell 123:106469
Chauhan D, Yadav A (2024a) An archive-based self-adaptive artificial electric field algorithm with orthogonal initialization for real-parameter optimization problems. Appl Soft Comput 150:111109
Chen W, Wang H, Liu Z, Jiang K (2023) Time-energy-jerk optimal trajectory planning for high-speed parallel manipulator based on quantum-behaved particle swarm optimization algorithm and quintic B-spline. Eng Appl Artif Intell 126:107223
Cheng M-Y, Sholeh MN (2023) Optical microscope algorithm: A new metaheuristic inspired by microscope magnification for solving engineering optimization problems. Knowl-Based Syst 279:110939
Cuong-Le T, Minh H-L, Sang-To T, Khatir S, Mirjalili S, Abdel Wahab M (2022) A novel version of grey wolf optimizer based on a balance function and its application for hyperparameters optimization in deep neural network (DNN) for structural damage identification. Eng Fail Anal 142:106829
Deng W, Xu J, Gao X-Z, Zhao H (2022) An enhanced MSIQDE algorithm with novel multiple strategies for global optimization problems. IEEE Trans Syst Man Cybern Syst 52(3):1578–1587
Dorigo M, Birattari M, Stützle T (2006) Ant colony optimization. Computational Intelligence Magazine, IEEE 1:28–39
Eskandar H, Sadollah A, Bahreininejad A, Hamdi M (2012) Water cycle algorithm—a novel metaheuristic optimization method for solving constrained engineering optimization problems. Comput Struct 110:151–166
Fan X, Yu M (2022) Coverage optimization of WSN based on improved grey wolf optimizer. Comput Sci 49:628–631
Fu H, Shi H, Xu Y, Shao J (2022) Research on gas outburst prediction model based on multiple strategy fusion improved snake optimization algorithm with temporal convolutional network. IEEE Access 10:117973–117984
Fu S, Huang H, Ma C, Wei J, Li Y, Fu Y (2023a) Improved dwarf mongoose optimization algorithm using novel nonlinear control and exploration strategies. Expert Syst Appl 233:120904
Fu S, Li K, Huang H, Ma C, Fan Q, Zhu Y (2024a) Red-billed blue magpie optimizer: a novel metaheuristic algorithm for 2D/3D UAV path planning and engineering design problems. Artif Intell Rev 57(6):134
Fu Y, Liu D, Chen J, He L (2024b) Secretary bird optimization algorithm: a new metaheuristic for solving global optimization problems. Artif Intell Rev 57(5):123
Garg V, Deep K, Bansal S (2023) Improved Teaching Learning Algorithm with Laplacian operator for solving nonlinear engineering optimization problems. Eng Appl Artif Intell 124:106549
Guo H-W, Sang H-Y, Zhang X-J, Duan P, Li J-Q, Han Y-Y (2023) An effective fruit fly optimization algorithm for the distributed permutation flowshop scheduling problem with total flowtime. Eng Appl Artif Intell 123:106347
Gupta, S., & Deep, K. 2017. Hybrid Grey Wolf Optimizer with Mutation Operator. Paper presented at the International Conference on Soft Computing for Problem Solving (SocProS), Indian Inst Technol Bhubaneswar, Bhubaneswar, INDIA.
Gupta S, Deep K (2020) Enhanced leadership-inspired grey wolf optimizer for global optimization problems. Engineering with Computers 36(4):1777–1800
Hakli H, Kiran MS (2020) An improved artificial bee colony algorithm for balancing local and global search behaviors in continuous optimization. Int J Mach Learn Cybern 11(9):2051–2076
Havaei P, Sandidzadeh MA (2023) Multi-objective train speed profile determination for automatic train operation with conscious search: a new optimization algorithm, a comprehensive study. Eng Appl Artif Intell 119:105756
Hayyolalam V, Kazem AAP (2020) Black widow optimization algorithm: a novel meta-heuristic approach for solving engineering optimization problems. Eng Appl Artif Intell 87:103249
Heidari AA, Mirjalili S, Faris H, Aljarah I, Mafarja M, Chen H (2019) Harris hawks optimization: Algorithm and applications. Futur Gener Comput Syst 97:849–872
Hu X-G, Ho T-S, Rabitz H (1998) The collocation method based on a generalized inverse multiquadric basis for bound-state problems. Comput Phys Commun 113(2–3):168–179
Hu G, Du B, Wang X, Wei G (2022) An enhanced black widow optimization algorithm for feature selection. Knowl Based Syst 235:107638
Jangir P, Jangir N (2018) A new Non-Dominated Sorting Grey Wolf Optimizer (NS-GWO) algorithm: Development and application to solve engineering designs and economic constrained emission dispatch problem with integration of wind power. Eng Appl Artif Intell 72:449–467
Jia H, Peng X, Lang C (2021) Remora optimization algorithm. Expert Syst Appl 185:115665
Jia H, Rao H, Wen C, Mirjalili S (2023) Crayfish optimization algorithm. Artif Intell Rev 56(Suppl 2):1919–1979
Kaveh A, Farhoudi N (2013) A new optimization method: Dolphin echolocation. Adv Eng Softw 59:53–70
Kennedy, J., & Eberhart, R. 1995a. Particle swarm optimization. Paper presented at the Proceedings of ICNN'95 - International Conference on Neural Networks.
Kennedy, J., & Eberhart, R. 1995b. Particle swarm optimization (PSO) . Paper presented at the Proc. IEEE international conference on neural networks, Perth, Australia.
Li X, Tang K, Omidvar MN, Yang Z, Qin K, China H (2013) Benchmark functions for the CEC 2013 special session and competition on large-scale global optimization. Gene 7(33):8
Li Y, Han T, Zhou H, Tang S, Zhao H (2022) A novel adaptive L-SHADE algorithm and its application in UAV swarm resource configuration problem. Inf Sci 606:350–367
Li K, Huang H, Fu S, Ma C, Fan Q, Zhu Y (2023) A multi-strategy enhanced northern goshawk optimization algorithm for global optimization and engineering design problems. Comput Methods Appl Mech Eng 415:116199
Article MathSciNet Google Scholar
Liang JJ, Qu BY, Suganthan PN (2013) Problem definitions and evaluation criteria for the CEC 2014 special session and competition on single objective real-parameter numerical optimization. Comput Intell Lab 635(2):1–32
Liang J-J, Qu B, Gong D, Yue C (2019) Problem definitions and evaluation criteria for the CEC 2019 special session on multimodal multiobjective optimization. Zhengzhou University, Computational Intelligence Laboratory, pp 1–26
Liu Z, He L, Yuan L, Zhang H (2022) Path Planning of Mobile Robot Based on TGWO Algorithm. Hsi-Chiao Tung Ta Hsueh/J. Xi’an Jiaotong Univ 56:49–60
Liu Y, Jiang Y, Zhang X, Pan Y, Wang J (2023) An improved grey wolf optimizer algorithm for identification and location of gas emission. J Loss Prev Process Ind 82:105003
Liu, Y. Y., Sun, J. H., Yu, H. Y., Wang, Y. Y., & Zhou, X. K. 2020. An Improved Grey Wolf Optimizer Based on Differential Evolution and OTSU Algorithm. Applied Sciences-Basel, 10(18).
Luo Y, Qin Q, Hu Z, Zhang Y (2023) Path planning for unmanned delivery robots based on EWB-GWO algorithm. Sensors 23(4):1867
Mallipeddi R, Suganthan PN (2010) Problem definitions and evaluation criteria for the CEC 2010 competition on constrained real-parameter optimization. Nanyang Technological University, Singapore 24:1–17
Meidani K, Hemmasian A, Mirjalili S, Barati Farimani A (2022) Adaptive grey wolf optimizer. Neural Comput Appl 34(10):7711–7731
Meng X, Jiang J, Wang H (2021) AGWO: Advanced GWO in multi-layer perception optimization. Expert Syst Appl 173:114676
Mirjalili S (2016) SCA: a sine cosine algorithm for solving optimization problems. Knowl Based Syst 96:120–133
Mirjalili S, Lewis A (2016) The whale optimization algorithm. Adv Eng Softw 95:51–67
Mirjalili S, Mirjalili SM, Lewis A (2014) Grey wolf optimizer. Adv Eng Softw 69:46–61
Mohakud, R., & Dash, R. 2022. Skin cancer image segmentation utilizing a novel EN-GWO based hyper-parameter optimized FCEDN. J. King Saud Univ. - Comput. Inf. Sci., 34(10): 9889–9904.
Mohamed, A. W., Hadi, A. A., Fattouh, A. M., & Jambi, K. M. 2017. LSHADE with semi-parameter adaptation hybrid with CMA-ES for solving CEC 2017 benchmark problems. Paper presented at the 2017 IEEE Congress on evolutionary computation (CEC).
Mohammed H, Rashid T (2023) FOX: a FOX-inspired optimization algorithm. Appl Intell 53(1):1030–1050
Mohammed H, Abdul Z, Hamad Z (2024) Enhancement of GWO for solving numerical functions and engineering problems. Neural Comput Appl 36(7):3405–3413
Muthiah-Nakarajan V, Noel MM (2016) Galactic Swarm Optimization: a new global optimization metaheuristic inspired by galactic motion. Appl Soft Comput 38:771–787
Nadimi-Shahraki MH, Taghian S, Mirjalili S (2021) An improved grey wolf optimizer for solving engineering problems. Expert Syst Appl 166:113917
Nadimi-Shahraki MH, Taghian S, Mirjalili S, Zamani H, Bahreininejad A (2022) GGWO: Gaze cues learning-based grey wolf optimizer and its applications for solving engineering problems. J Comput Sci 61:101636
Nadimi-Shahraki MH, Taghian S, Zamani H, Mirjalili S, Elaziz MA (2023) MMKE: Multi-trial vector-based monkey king evolution algorithm and its applications for engineering optimization problems. PLoS ONE 18(1):e0280006
Pan W-T (2012) A new Fruit Fly Optimization Algorithm: Taking the financial distress model as an example. Knowl Based Syst 26:69–74
Rashedi E, Nezamabadi-Pour H, Saryazdi S (2009) GSA: A Gravitational Search Algorithm. Inf Sci 179(13):2232–2248
Rathan, S., Shah, D., Kumar, T. H., & Charan, K. S. 2023. Adaptive IQ and IMQ-RBFs for solving Initial Value Problems: Adam-Bashforth and Adam-Moulton methods. arXiv preprint arXiv:2302.06113.
Said R, Elarbi M, Bechikh S, Coello Coello CA, Said LB (2023) Discretization-based feature selection as a bilevel optimization problem. IEEE Trans Evol Comput 27(4):893–907
Saka MP, Hasançebi O, Geem ZW (2016) Metaheuristics in structural optimization and discussions on harmony search algorithm. Swarm Evol Comput 28:88–97
Şenel FA, Gökçe F, Yüksel AS, Yiğit T (2019) A novel hybrid PSO–GWO algorithm for optimization problems. Eng Comput 35(4):1359–1373
Shayanfar H, Gharehchopogh FS (2018) Farmland fertility: A new metaheuristic algorithm for solving continuous optimization problems. Appl Soft Comput 71:728–746
Shehadeh HA (2023) Chernobyl disaster optimizer (CDO): a novel meta-heuristic method for global optimization. Neural Comput Appl 35(15):10733–10749
Singh, S., & Bansal, J. C. 2022b. Mutation-driven grey wolf optimizer with modified search mechanism. Expert Systems with Applications, 194.
Singh S, Bansal JC (2022a) Mutation-driven grey wolf optimizer with modified search mechanism. Expert Syst Appl 194:116450
Soliman MA, Hasanien HM, Turky RA, Muyeen SM (2022) Hybrid African vultures–grey wolf optimizer approach for electrical parameters extraction of solar panel models. Energy Rep 8:14888–14900
Storn R, Price K (1997) Differential evolution–a simple and efficient heuristic for global optimization over continuous spaces. J Global Optim 11(4):341–359
Tanabe, R., & Fukunaga, A. S. 2014. Improving the search performance of SHADE using linear population size reduction. Paper presented at the 2014 IEEE congress on evolutionary computation (CEC).
Tripathy, B., Reddy Maddikunta, P. K., Pham, Q.-V., Gadekallu, T. R., Dev, K., Pandya, S., & ElHalawany, B. M. 2022. Harris hawk optimization: a survey onvariants and applications. Computational Intelligence and Neuroscience, 2022.
Wang, Q., Xu, J., Zhang, W., Mao, M., Wei, Z., Wang, L., Cui, C., Zhu, Y., & Ma, J. 2018. Research progress on vanadium-based cathode materials for sodium ion batteries. J. Mater. Chem. A Mater. Energy Sustain., 6(19): 8815–8838.
Wei, G. 2012. Study on Genetic Algorithm and Evolutionary Programming. Paper presented at the 2nd IEEE International Conference on Parallel, Distributed and Grid Computing (PDGC), Jaypee Univ Informat Technol (JUIT), Waknaghat, INDIA.
Wilcoxon F (1945) Individual comparisons by ranking methods. Biom Bull 1(6):80–83
Wolpert DH, Macready WG (1997) No free lunch theorems for optimization. IEEE Trans Evol Comput 1(1):67–82
Xia X, Fu X, Zhong S, Bai Z, Wang Y (2023) Gravity particle swarm optimization algorithm for solving shop visit balancing problem for repairable equipment. Eng Appl Artif Intell 117:105543
Xue, J., & Shen, B. 2022. Dung beetle optimizer: a new meta-heuristic algorithm for global optimization. Journal of Supercomputing.
Xue J, Shen B (2020) A novel swarm intelligence optimization approach: sparrow search algorithm. Systems Science & Control Engineering 8:22–34
Yang, X.-S. 2009. Firefly algorithms for multimodal optimization. Paper presented at the International symposium on stochastic algorithms.
Yapici H, Cetinkaya N (2019) A new meta-heuristic optimizer: Pathfinder algorithm. Appl Soft Comput 78:545–568
Yu X, Jiang N, Wang X, Li M (2023) A hybrid algorithm based on grey wolf optimizer and differential evolution for UAV path planning. Expert Syst Appl 215:119327
Yuan Y, Ren J, Wang S, Wang Z, Mu X, Zhao W (2022) Alpine skiing optimization: A new bio-inspired optimization algorithm. Adv Eng Softw 170:103158
Zhang, M. J., Long, D. Y., Wang, X., Yu, L. Z., Wu, J. W., Li, D. H., Yang, J., & Ieee. 2019. Improved Grey Wolf Algorithm Based on Nonlinear Control Parameter Strategy. Paper presented at the Chinese Automation Congress (CAC), Hangzhou, PEOPLES R CHINA.
Zhou Y, He X, Chen Z, Jiang S (2022) A neighborhood regression optimization algorithm for computationally expensive optimization problems. IEEE Trans Cybern 52(5):3018–3031
Download references
Acknowledgements
There is no acknowledgement involved in this work.
This work was supported by Natural Science Foundation of Tianjin Municipality (21JCYBJC00110) and China Postdoctoral Science Foundation (2023M731803).
Author information
Authors and affiliations.
College of Artificial Intelligence, Nankai University, Tianjin, 300350, China
Mingyang Yu, Jing Xu, Weiyun Liang, Yu Qiu & Sixu Bao
Department of Biological and Chemical Sciences, New York Institute of Technology, New York, 11568-8000, USA
You can also search for this author in PubMed Google Scholar
Contributions
MY: conceptualization, methodology, writing—original draft, formal analysis, data curation, writing—review & editing, software. WL: visualization, formal analysis, writing—review & editing. JX: conceptualization, resources, supervision, formal analysis. YQ: software, writing—review & editing, resources. SB: methodology, visualization resources, software. LT: visualization resources.
Corresponding author
Correspondence to Jing Xu .
Ethics declarations
Competing interests.
The authors have no relevant financial or non-financial interests to disclose.
Ethical approval
His article does not contain any studies with human participants or animals performed by any of the authors.
Informed consent
This article does not contain any studies with human participants. So informed consent is not applicable here.
Additional information
Publisher's note.
Springer Nature remains neutral with regard to jurisdictional claims in published maps and institutional affiliations.
Rights and permissions
Open Access This article is licensed under a Creative Commons Attribution 4.0 International License, which permits use, sharing, adaptation, distribution and reproduction in any medium or format, as long as you give appropriate credit to the original author(s) and the source, provide a link to the Creative Commons licence, and indicate if changes were made. The images or other third party material in this article are included in the article's Creative Commons licence, unless indicated otherwise in a credit line to the material. If material is not included in the article's Creative Commons licence and your intended use is not permitted by statutory regulation or exceeds the permitted use, you will need to obtain permission directly from the copyright holder. To view a copy of this licence, visit http://creativecommons.org/licenses/by/4.0/ .
Reprints and permissions
About this article
Yu, M., Xu, J., Liang, W. et al. Improved multi-strategy adaptive Grey Wolf Optimization for practical engineering applications and high-dimensional problem solving. Artif Intell Rev 57 , 277 (2024). https://doi.org/10.1007/s10462-024-10821-3
Download citation
Accepted : 28 May 2024
Published : 05 September 2024
DOI : https://doi.org/10.1007/s10462-024-10821-3
Share this article
Anyone you share the following link with will be able to read this content:
Sorry, a shareable link is not currently available for this article.
Provided by the Springer Nature SharedIt content-sharing initiative
- Grey Wolf Optimization algorithm
- Search mechanism
- Inverse multiquadratic function
- Adaptive strategy
- Engineering design challenges
- Find a journal
- Publish with us
- Track your research
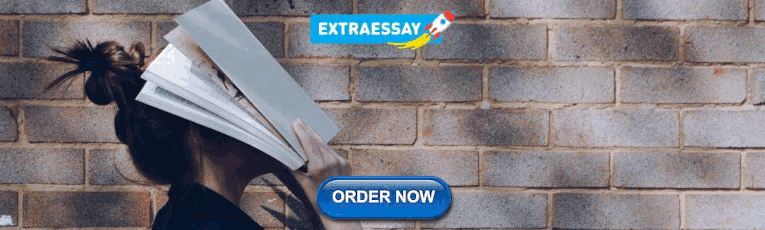
IMAGES
VIDEO
COMMENTS
The eight steps to practical problem solving also include the Plan, Do, Check and Act (PDCA) cycle. Steps one through five are the planning process. The doing is found in step six. Step seven is the checking . Step eight involves acting out the results of the new standard. This practical problem solving can be powerful tool to issues facing ...
Practical Problem Solving is both a process and a skill that you develop over time to solve problems quickly and achieve goals. This process provides teams with a framework for solving problems, allowing them to quickly define, diagnose, and resolve issues. Additionally, because this process involves root cause analysis, follow-up, and ...
Overview. Toyota practical problem solving consists of the steps as listed below. Note that sometimes you have a step more if you decide to split a step into two. Clarify the Problem. Break Down the Problem. Set a Target. Root-Cause Analysis. Develop Countermeasures and Implement. Monitor Process and Results.
Problem-solving is an important skill to develop because life will always throw you curveballs. Being able to respond to these problems with flexibility and calmness will generate much better results than if you respond to the problem with resistance or avoidance. Also, research has shown that increasing problem-solving skills through problem-solving therapy is beneficial for several physical ...
1. Define the problem. Diagnose the situation so that your focus is on the problem, not just its symptoms. Helpful problem-solving techniques include using flowcharts to identify the expected steps of a process and cause-and-effect diagrams to define and analyze root causes.. The sections below help explain key problem-solving steps.
Facilitator's Guide. PPS Overview. An overview of the 8 steps to Practical Problem Solving. A step by step guide to presenting Gemba Academy's 5S course to lean learners in all positions. This guide includes key points, discussion items, quiz questions and answers, and helpful tips. Using Gemba Academy's online learning content, any ...
The 8-Step Practical Problem Solving Process: The eight steps: Clarifying the problem and describing the current situation. Breaking down a big problem into smaller, more specific problems and studying the process. Setting a challenging yet achievable target. Analyzing the root cause. Creating a plan to remove the root cause through multiple ...
An argument is a set of statements that claims that one or more of those statements, called the premises, support another of them, called the conclusion. Thus, every argument claims that its premises support its conclusion. logically strong argument, according to Hughes, is one "whose premises, if true, support its conclusion.".
Problem-solving is an important component of any business or organization. It entails identifying, analyzing, and resolving problems in order to improve processes, drive results, and foster a culture of continuous improvement. A3 Problem solving is one of the most effective problem-solving methodologies. A3 Problem solving is a structured and systematic approach to problem-solving that ...
Problem-solving is a mental process that involves discovering, analyzing, and solving problems. The ultimate goal of problem-solving is to overcome obstacles and find a solution that best resolves the issue. The best strategy for solving a problem depends largely on the unique situation. In some cases, people are better off learning everything ...
1. The first step in the process has us clarifying the problem. In other words, we must clearly describe the current situation, while going to see with our own eyes in order to get the facts. 2. The second step of the process has us breaking the big vague problem down to its smaller, more specific problems. 3.
7. Solution evaluation. 1. Problem identification. The first stage of any problem solving process is to identify the problem (s) you need to solve. This often looks like using group discussions and activities to help a group surface and effectively articulate the challenges they're facing and wish to resolve.
A3 8 Step Practical Problem Solving (PPS) is a structured and effective problem-solving process used by individuals and teams to solve challenging, medium term, business and operational problems, originally pioneered by Toyota. Learn about the 8-step process, including clarifying the problem, containment, analysing & breaking it down, target ...
The problem-solving process typically includes the following steps: Identify the issue: Recognize the problem that needs to be solved. Analyze the situation: Examine the issue in depth, gather all relevant information, and consider any limitations or constraints that may be present. Generate potential solutions: Brainstorm a list of possible ...
Practical Problem Solving. Practical Problem Solving (PPS) is a Lean methodology initially developed in the Toyota factory that expands on the PDCA cycle (Plan, Do, Check, Act) and turns problem-solving into a systematic eight-step process. Practical Problem Solving allows organizations to estab ...
Key Learning Points from Video. 1 - "Go and See" at the workplace - grasp the real situation. 2 - Follow Structured Scientific Method - not guessing. In Practical Problem Solving it is 8 Steps. 3 - Follow Plan - Do - Check - Act an approach of rapid trials and tests to prove out theories and learn. 4 - Take a step towards ...
Practical problem-solving. This 8-step method centers around solving problems in a team setting, though it can be adapted to individual use: Clarify the problem. Ensure all your team members are on the same page by discussing the problem and landing on a definition. Break down the problem.
https://gembaacademy.com | The Gemba Academy Practical Problem Solving course explains the exact 8 step problem solving process used by these lean exemplars ...
Brainstorm options to solve the problem. Select an option. Create an implementation plan. Execute the plan and monitor the results. Evaluate the solution. Read more: Effective Problem Solving Steps in the Workplace. 2. Collaborative. This approach involves including multiple people in the problem-solving process.
Problem-solving is a vital skill for coping with various challenges in life. This webpage explains the different strategies and obstacles that can affect how you solve problems, and offers tips on how to improve your problem-solving skills. Learn how to identify, analyze, and overcome problems with Verywell Mind.
Men tended to act more independently. If you're going to solve a problem quickly, involve those around you and share the glory. 4. They know how to explain the problem and solution effectively. A ...
This study aims to improve mathematics problem-solving skills and learning achievement of students by developing learning media on YouTube and Learning Blogs. The experiment is conducted by using two groups of learners, the Control group and the experiment group. Each of them has 19 Junior High School students. The topic is statistics material.
While Sunday is the start of the week, especially for Christians, Monday is often the day where people really have to lock into their tasks, start problem-solving, and deal with the realities of the week. It is easy to focus on Christ on the Sabbath, as it is a day set aside to rest and develop one's relationship with the Savior.
The Grey Wolf Optimization (GWO) is a highly effective meta-heuristic algorithm leveraging swarm intelligence to tackle real-world optimization problems. However, when confronted with large-scale problems, GWO encounters hurdles in convergence speed and problem-solving capabilities. To address this, we propose an Improved Adaptive Grey Wolf Optimization (IAGWO), which significantly enhances ...