
Home > UGS > DISSERTATIONS > AAI3217563
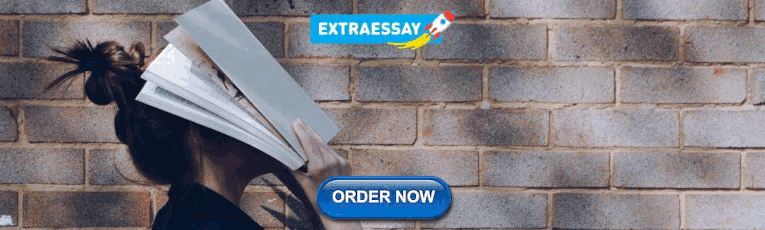
Customer relationship management: A content analysis of issues and best practices
Samit Chakravorti , Florida International University
This dissertation is a study of customer relationship management theory and practice. Customer Relationship Management (CRM) is a business strategy whereby companies build strong relationships with existing and prospective customers with the goal of increasing organizational profitability. It is also a learning process involving managing change in processes, people, and technology. CRM implementation and its ramifications are also not completely understood as evidenced by the high number of failures in CRM implementation in organizations and the resulting disappointments. The goal of this dissertation is to study emerging issues and trends in CRM, including the effect of computer software and the accompanying new management processes on organizations, and the dynamics of the alignment of marketing, sales and services, and all other functions responsible for delivering customers a satisfying experience. In order to understand CRM better a content analysis of more than a hundred articles and documents from academic and industry sources was undertaken using a new methodological twist to the traditional method. An Internet domain name (http://crm.fiu.edu) was created for the purpose of this research by uploading an initial one hundred plus abstracts of articles and documents onto it to form a knowledge database. Once the database was formed a search engine was developed to enable the search of abstracts using relevant CRM keywords to reveal emergent dominant CRM topics. The ultimate aim of this website is to serve as an information hub for CRM research, as well as a search engine where interested parties can enter CRM-relevant keywords or phrases to access abstracts, as well as submit abstracts to enrich the knowledge hub. Research questions were investigated and answered by content analyzing the interpretation and discussion of dominant CRM topics and then amalgamating the findings. This was supported by comparisons within and across individual, paired, and sets-of-three occurrences of CRM keywords in the article abstracts. Results show that there is a lack of holistic thinking and discussion of CRM in both academics and industry which is required to understand how the people, process, and technology in CRM impact each other to affect successful implementation. Industry has to get their heads around CRM and holistically understand how these important dimensions affect each other. Only then will organizational learning occur, and overtime result in superior processes leading to strong profitable customer relationships and a hard to imitate competitive advantage.
Subject Area
Marketing|Management|Business community
Recommended Citation
Chakravorti, Samit, "Customer relationship management: A content analysis of issues and best practices" (2006). ProQuest ETD Collection for FIU . AAI3217563. https://digitalcommons.fiu.edu/dissertations/AAI3217563
Since November 15, 2006
Advanced Search
- Notify me via email or RSS
- Florida International University
- FIU Libraries Home
- Data Management at FIU
- Collections
- Departments and Schools
- University Archives
- Expert Gallery
- Theses and Dissertations
Author Corner
- Author Toolbox
Home | About | FAQ | My Account | Accessibility Statement
Privacy Copyright
Artificial intelligence in customer relationship management: literature review and future research directions
Journal of Business & Industrial Marketing
ISSN : 0885-8624
Article publication date: 30 March 2022
Issue publication date: 19 December 2022
Due to the recent development of Big Data and artificial intelligence (AI) technology solutions in customer relationship management (CRM), this paper provides a systematic overview of the field, thus unveiling gaps and providing promising paths for future research.
Design/methodology/approach
A total of 212 peer-reviewed articles published between 1989 and 2020 were extracted from the Scopus database, and 2 bibliometric techniques were used: bibliographic coupling and keywords’ co-occurrence.
Outcomes of the bibliometric analysis enabled the authors to identify three main subfields of the AI literature within the CRM domain (Big Data and CRM as a database, AI and machine learning techniques applied to CRM activities and strategic management of AI–CRM integrations) and capture promising paths for future development for each of these subfields. This study also develops a three-step conceptual model for AI implementation in CRM, which can support, on one hand, scholars in further deepening the knowledge in this field and, on the other hand, managers in planning an appropriate and coherent strategy.
Originality/value
To the best of the authors’ knowledge, this study is the first to systematise and discuss the literature regarding the relationship between AI and CRM based on bibliometric analysis. Thus, both academics and practitioners can benefit from the study, as it unveils recent important directions in CRM management research and practices.
- Bibliometric analysis
- Research agenda
- Artificial intelligence
- Machine learning
- Customer relationship management
Ledro, C. , Nosella, A. and Vinelli, A. (2022), "Artificial intelligence in customer relationship management: literature review and future research directions", Journal of Business & Industrial Marketing , Vol. 37 No. 13, pp. 48-63. https://doi.org/10.1108/JBIM-07-2021-0332
Emerald Publishing Limited
Copyright © 2022, Cristina Ledro, Anna Nosella and Andrea Vinelli.
Published by Emerald Publishing Limited. This article is published under the Creative Commons Attribution (CC BY 4.0) licence. Anyone may reproduce, distribute, translate and create derivative works of this article (for both commercial and non-commercial purposes), subject to full attribution to the original publication and authors. The full terms of this licence may be seen at http://creativecommons.org/licences/by/4.0/legalcode
1. Introduction
Customer relationship management (CRM) activity involves collecting, managing and intelligently using data with the support of technology solutions to develop long-term customer relationships and exceptional customer experience (CX) ( Boulding et al. , 2005 ; Payne and Frow, 2005 ; Rababah, 2011 ). The data obtained from all customer contact points, if well managed, can support companies in generating personalised marketing responses, creating new ideas, tailoring products and services and, thus, delivering high customer value and gaining competitive advantage ( Kumar and Misra, 2021 ; Payne and Frow, 2005 ; Paquette, 2010 ). In the digital age, the increasing volume, velocity and variety of data, as well as their processing capacity, have led to new technology solutions, including the advancement of artificial intelligence (AI) techniques ( Brynjolfsson and McAfee, 2017 ). AI refers to a system’s ability to interpret a large quantity of data correctly, learn from such data and use these learnings to reach specific goals and tasks ( Kaplan and Haenlein, 2019 ).
Both companies that develop CRM systems and those that use CRM enjoy advances in AI technology solutions, which have become essential to survive in the CRM context (Pearson, 2019). In fact, new CRM features, such as personality insight services, website morphing, chatbot services, programmatic advertising and emotional, image and facial recognition technologies, require considerable data to be crunched in real time, which would be almost impossible to implement without AI’s advancements (Pearson, 2019).
Alongside the relevance of AI in the business world, academia also claims that AI is the next step towards a novel and more capable management of customer relations ( Kumar et al. , 2020 ; Lokuge et al. , 2020 ; Vignesh and Vasantha, 2019 ). As CRM “is the outcome of the continuing evolution and integration of marketing ideas and newly available data, technologies, and organisational forms” ( Boulding et al. , 2005 ), AI plays a fundamental role because AI solutions applied to CRM enable companies to better assimilate and analyse customer data ( Brynjolfsson and McAfee, 2017 ; Libai et al. , 2020 ), making them increasingly able to anticipate, plan and take advantage of upcoming opportunities ( Mishra and Mukherjee, 2019 ).
Despite AI becoming increasingly pervasive in managerial contexts, management scholars have provided little insights into AI during the past two decades ( Raisch and Krakowski, 2020 ). The AI literature has mainly evolved along two separate disciplines: computer science and operations research, whose scholars have mainly investigated operational tasks that machines can handle, and organisation and management research, where managerial tasks reserved for humans are analysed ( Raisch and Krakowski, 2020 ). Recently, the growing awareness of AI importance and the potential impact it might have on CRM have led to a large proliferation of publications, resulting in an accumulation of knowledge on the topic that is quite scattered and fragmented ( Schröder et al. , 2021 ). This is also attributed to the fact that there are several definitions of CRM, each looking at CRM from a different perspective as a strategy, a process or an information system ( Khodakarami and Chan, 2014 ; Richards and Jones, 2008 ). When dealing with AI–CRM relationship, these different perspectives drive different fields of knowledge, from business management to innovation science, causing advances in research to occur in isolated silos with few interdisciplinary exchanges ( Loureiro et al. , 2021 ). Furthermore, as CRM includes sales, marketing, service and operations activities, its interfunctionality has made the AI–CRM research even more fragmented in different business areas. On such grounds, it seems worth for both the business and academic worlds to systematise the literature on AI in CRM into a full body of structured knowledge, which can guide managers as well as inspire scholars’ future research.
Previous reviews in the field have focused on specific aspects, such as the challenges and applications of Big Data and AI on customer journey modelling ( Arco et al. , 2019 ; Chatterjee et al. , 2019 ), or the potential impacts of Big Data and AI, respectively, on the key success factors of CRM ( Zerbino et al. , 2018 ) and consumers’ decision-making ( Klaus and Zaichkowsky, 2020 ).
To the best of our knowledge, a wide-ranging review dedicated to mapping the literature concerning AI in the CRM domain is still lacking. Based on these premises, this paper aims to trace the state-of-the-art of AI in CRM and, thus, identify rising themes and promising paths for future research. For this purpose, the authors conduct a methodical, transparent and replicable review of AI in CRM, using bibliometric techniques to map the research field without subjective bias ( Zupic and Čater, 2015 ). In particular, the current study combines bibliographic coupling to analyse references and establish intellectual linkages among articles and keywords’ co-occurrence to comprehensively understand the leading keywords, allowing the construction of structural images of the research domain.
Our study contributes to advancing the domain of AI in CRM ( Donthu et al. , 2021 ), addressing new important directions for future research. Furthermore, it offers relevant insights to practitioners.
The rest of the paper is organized as follows. Section 2 describes the methodology, including the search strategy and data collection. Section 3 provides the results with data analysis and visualisation, whereas Section 4 discusses the main contributions and future research paths. Finally, Section 5 presents the conclusions, contributions and limitations of the study.
2. Methodology
To achieve the research goal, we performed a literature review combined with a bibliometric analysis. Bibliometrics is described as “the mathematical and statistical analysis of bibliographic records” ( Pritchard, 1969 ) and is used to establish intellectual linkages among articles and keywords, thus providing a big picture of rising trends and potential research opportunities ( Boyack and Klavans, 2010 ; Marchiori and Franco, 2020 ). Bibliometric techniques offer the advantage of introducing quantitative rigor compared to narrative literature reviews, which might be invalidated by the subjective bias of the researcher ( Tranfield et al. , 2003 ).
The first stage of the method concerns the search and collection of the articles to be analysed, which should truly represent the field of AI in CRM ( McCain, 1990 ), and the second stage employs several bibliometric analyses to map the field and identify the most important themes within it.
2.1 Search strategy and data collection
To identify the appropriate articles for our aim, we executed a search on the Scopus database by using keywords related to AI in CRM. Using the AND operator, we combined the search string for AI (“artificial intelligence” OR “AI” OR “machine learning” OR “deep learning” OR “Big Data”) with that for CRM (“customer relationship management” OR “CRM” OR “customer management” OR “customer experience” OR “CX” OR “customer journey”) in the title, abstract and keywords.
The definitions and motivations behind the choice of these keywords are listed as follows. Following Kumar et al. (2020) , we considered AI to be a generic term referring to a technology that can imitate humans and carry out tasks in an intelligent manner. The term Artificial Intelligence is a little bit loose, but it is essentially about using machine learning - and specifically deep learning - to enable applications ( Brynjolfsson and McAfee, 2017 ). Especially regarding AI in CRM, the prevalence of AI applications concerns machine learning (ML) and its successor technologies, particularly deep learning ( Libai et al. , 2020 ). Thus, as the terms “machine learning,” “deep learning” and “artificial intelligence” are related and often used interchangeably, we included all of them in the search string ( Borges et al. , 2020 ). In particular, we considered ML as a branch of AI that can learn from data, detect patterns and make decisions with minimal human intervention ( Kumar et al. , 2020 ) and deep learning as a technological evolution of ML that can learn from data as well as from its mistakes without human intervention ( Zaki, 2019 ). AI is often connected with the term Big Data ( Arco et al. , 2019 ), as Big Data is considered raw fuel of AI and significantly impacts AI capabilities and value creation ( Deshpande and Kumar, 2018 ; Saidulu and Sasikala, 2017 ). Thus, to exclude papers potentially related to AI, we also included “Big Data” in the search string.
Regarding the keywords used for CRM, given that CRM is linked and often interchanged with the terms “customer experience” and “customer journey”, we included the latter in the search string ( Buckley and Webster, 2016 ). CRM activities involve collecting and intelligently using data to build enduring customer relationships and a consistently superior CX by leveraging the comprehension of the customer journey ( Buckley and Webster, 2016 ; Lemon and Verhoef, 2016 ; Payne and Frow, 2005 ).
CRM and CX are often so much linked together that some companies (e.g. Oracle) see the management of CX as part of advanced CRM ( Lemon and Verhoef, 2016 ). However, CRM concerns planning, implementing, monitoring and improving customer relationships, whereas CX management mainly focusses on how to improve CX at a touchpoint level ( Holmlund et al. , 2020 ).
Similarly, customer journey literature and CRM are often interrelated, as CRM is considered by CRM providers (e.g. salesforce), as well as academics, as a source for customer journey mapping, allowing data centralisation and making them available to different touchpoints ( Payne and Frow, 2005 ). Moreover, given the speed of the current generation of servers and the sophistication of Big Data analytics tools, some have hypothesised that customer journey analytics will be the next CRM ( Fluss, 2017 ).
The search was performed in November 2020, and we obtained 1,032 articles. Filtering this initial data set out, English articles belonging to the Engineering and Business, Management and Accounting categories and articles and reviews underwent a double-blind peer-review process, and excluding duplicates, we finally retrieved 212 articles ( Figure 1 ). These articles ranged from 2001 (2 articles) to 2020 (68 articles, available in November). Only one article was published before 2001, in 1989. Figure 2 represents the temporal distribution of articles in the field of AI in CRM and shows a steep recent growth in the literature.
2.2 Bibliometric analysis: bibliographic coupling and keyword analysis
After identifying the articles focussed on the theme under investigation, we performed two bibliometric analysis – bibliographic coupling and keywords’ co-occurrence – to trace the state-of-the-art of AI in CRM contexts. VOS viewer version 1.6.15 was used to construct and display the bibliographic maps ( Van Eck and Waltman, 2010 ). The VOS viewer has already been used to review the literature on industrial marketing ( Valenzuela Fernandez et al. , 2019 ), information technology management ( Khan and Wood, 2015 ), the inter-connection between Big Data and business strategy ( Ciampi et al. , 2020 ) and Big Data and co-innovation ( Bresciani et al. , 2021 ) through bibliometric analyses. The 212-article data set and relative cited reference data were imported into VOS viewer. Before applying the bibliometric analysis, the completeness of the information within the data set was checked and missing cited reference data were added manually.
First, we conducted a bibliographic coupling of sampled articles to cluster papers based on shared references. This was achieved by counting how many times two articles cited the same references. Bibliographic coupling and co-citation analysis are the two most important science mapping techniques. A co-citation network is formed when two articles (nodes) are cited together by another document, whereas a bibliographic coupling network is formed when both articles (nodes) refer to a third document within their references, forming a link ( Van Eck and Waltman, 2020 ). While co-citation analysis focusses on older literature and bibliographic coupling focusses on more recent research, we opted for bibliographic coupling as we dealt with very recent papers ( Schröder et al. , 2021 ). In the bibliographic coupling network, each link has a strength, depicted by a positive numeric value; the higher this value, the stronger is the link ( Van Eck and Waltman, 2020 ). Thus, the more citations both articles have in common, the stronger is their bibliometric connection. Based on the bibliometric mapping performed by VOS viewer, 99 articles strongly connected within our data set were classified into clusters that likely addressed the same themes ( Waltman et al. , 2010 ; Schröder et al. , 2021 ).
Second, we analysed the keywords’ co-occurrence to discover the most important research topics and the conceptual structure underlying the field of AI in CRM at the time of conducting this study ( Callon et al. ,1983 ; Niknejad et al. , 2021 ). For this purpose, the number of sample articles in which two keywords appear together was counted. In particular, the keywords’ co-occurrence network is formed when the keywords (nodes) appear together, forming a link ( Van Eck and Waltman, 2020 ). Then, the most frequently related keywords were classified into clusters using bibliometric mapping.
To better interpret keyword mapping, we applied an overlay network visualisation, in which items appear in coloured scales with the “average publication year” and the “average normalized number of citations received by the article in which a keyword occurs” ( Van Eck and Waltman, 2020 ). These longitudinal visualisations enabled the assessment of the evolution of the conceptual structure of the research domain of AI in CRM. For the co-occurrence analysis, both the author keywords and index keywords of the sampled articles were considered. Before applying the bibliometric analysis, the extracted keywords were refined and standardised for 1,560 keywords ( Khan and Wood, 2015 ; Kim et al. , 2018 ). In particular, we removed synonyms and derivative words and standardised words with similar meanings. For example, “database”, “data-base” and “database system” were standardised into “database”. Moreover, terms separated by hyphens were standardised. For instance, “social-media” was grouped into “social media” and “omni-channel” into “omnichannel”. Abbreviations in brackets were considered as additional keywords. In addition, where appropriate, in the keywords containing “and” and “&”, these were removed and the keywords were separated.
While bibliographic coupling looks at the background, keywords’ co-occurrences look at the content of the sampled articles. Thus, by combining the results of the keywords’ co-occurrence and bibliographic coupling, we recognised the thematic structure of the clusters. To perform this step, we mapped in a spreadsheet the relevant features of the sampled articles with reference to the following: article purpose, research questions/hypotheses, methodology, context, theoretical background, gaps in the literature, key findings and type of involved technology. Mapping these aspects supported us in identifying the theme that characterises each cluster and, at the same time, paved the way for identifying the gaps and research opportunities.
3.1 Bibliographic coupling
Table 1 shows that the article with the strongest link (29 citations and 92 total link strengths) is Zerbino et al. ’s (2018) , which studies Big Data-enabled CRM. Within the same line of enquiry, the highest link strength paper is Hallikainen et al. 's (2020) , which explores the use of Big Data analytics in CRM with a focus on B2B firms. These findings indicate that Big Data can be considered the cornerstone of AI applications in CRM systems ( Borges et al. , 2020 ; Deshpande and Kumar, 2018 ; Saidulu and Sasikala, 2017 ).
The article by Chatterjee et al. (2019) , ranking seventh in terms of total link strength within the sampled articles, is among the first to discuss approaches and challenges of AI–CRM integration, which is defined as a “hybrid modern system” required by firms to better analyse customers’ data strategically, improve their overall business process and ensure accurate decision-making without human intervention.
In addition, among the 10 articles with higher total link strengths, most are very recent as well as already heavily cited ( Table 1 ). Three of these articles look at the state-of-the-art and research opportunities of using text or image mining techniques in service research ( Villarroel Ordenes and Zhang, 2019 ) and, in particular, in CX management ( McColl-Kennedy et al. , 2019 ; Holmlund et al. , 2020 ). This evidence supports the increasing scholarly attention focussed on investigating AI techniques, such as text and image mining, and shows that applying these techniques to CX can offer significant insights in that matter.
The bibliographic coupling of the articles shows that papers are clustered around three main groups, depicted by three colours ( Figure 3 ). In particular, the node size is proportional to the total link strength of each article, whereas the line thickness represents the co-occurrence frequency of pairs. In addition, the position of a node gives insights into the nodes’ connections: the closeness of two articles indicates that they have several citations in common ( Marchiori and Franco, 2020 ).
The following two articles at the centre of the graph form the pillars in this research domain: Rust and Huang (2014) , a study on how the IT and service revolution are transforming marketing science by enhancing the ability to provide more personalised services and deepen customer relationships, and Liu et al. (2017) , a study on the use of linguistic-based text analytics (text mining and sentiment analysis) to derive latent brand topics and classify brand sentiments on social media.
Turning to the three formed clusters, the red cluster is the largest (38 articles), followed by the green and blue clusters (32 and 29 articles, respectively).
In the red cluster, articles deal with the management of Big Data and their impact on CRM ( Hallikainen et al. , 2020 ; Zerbino et al. , 2018 ), with a particular focus on knowledge management and information assets for, from and about customers ( Chatterjee et al. , 2020a , 2020b ; Del Vecchio et al. , 2020 ; Talón-Ballestero et al. , 2018 ).
In contrast, in the green cluster, there are articles proposing AI-based techniques that support CRM business activities, such as customers’ life event prediction ( De Caigny et al. , 2020a , 2020b ), customer churn prediction ( De Caigny et al. , 2020a , 2020b ), high-value customer identification ( Chang et al. , 2016 ; Chiang, 2019 ) and sentiment analysis ( Liu et al. , 2017 ; Mukherjee and Bala, 2017 ).
Finally, in the blue cluster, we find articles that deal with how to integrate Big Data insights into automated processes related to key customer touchpoints to improve customer value ( Spiess et al. , 2014 ) and how to use AI to deliver coherent streams of connections through different touchpoints for effective customer engagement ( Singh et al. , 2020 ). In the centre, still in blue, some articles examine how AI might affect the core characteristics of CRM ( Libai et al. , 2020 ) and improve operational efficiency and customer service ( Prentice and Nguyen, 2020 ), in particular with the use of bots ( Trivedi, 2019 ).
To further investigate the degree of connectivity among articles, we analysed the citations among them. Table 2 lists the articles according to the number of local citations (i.e. how many times an article cites or is cited by other articles within the sample) and global citations, which refers to the total citations for the article. As presented in Table 2 , global citations are remarkable, revealing how the issue of AI in the management of customer relationships is relevant, cross-cutting and draws the attention of academics in other research areas ( Niknejad et al. , 2021 ).
3.2 Keywords’ co-occurrence
Out of the dataset of 1,560 keywords, the top 55 keywords with at least 4 occurrences defined as the “number of articles in which a keyword occurs” were selected ( Van Eck and Waltman, 2020 ) ( Figure 4 ). The keyword’s number of occurrences defines the node size. The most frequently related keywords are classified into three clusters. The lines represent the connections among the keywords, and the colours identify the clusters to which the keywords belong. In addition, keywords closer to each other have a stronger relationship than farther keywords ( Van Eck and Waltman, 2020 ). Table 3 supports the results of Figure 4 by presenting the occurrences (weight) of the keywords among the different clusters.
If we look at the results of the cluster analysis, we can distinguish among three main conceptual streams in the academic discussion regarding AI and CRM, which are coherent with the previous three clusters identified with the bibliographic coupling analysis.
In the red cluster, the most frequently occurring keywords are Big Data , information management and social media. In addition, in this cluster, there are several keywords related to networks and data processing (such as internet, social media, social networking, database, mathematical models and computer software ). In particular, the strong link between Big Data and sentiment analysis highlights a rising interest in the applications of sentiment analysis on Big Data produced through telecommunication networks and social media.
In the green cluster, the most common keywords are machine learning, sales and marketing. In general, in this cluster, there are keywords of systems/techniques/models based on ML and AI (mainly related to classification and regression) bounded to keywords of business activities and practices related to CRM (such as sales, marketing, public relations, commerce and forecasting).
In the blue cluster, the most frequently occurring keywords are artificial intelligence, customer experience and customer relationship management, which are also strongly related. In general, in this cluster, there are keywords of data science (such as Big Data analytics, business intelligence, Internet of Things, data mining and data analysis ) related to keywords of customer-centric vision (such as customer relationship management, customer experience , customer journey, customer management and customer loyalty ).
We also examined the keywords’ co-occurrence by adopting a temporal perspective to trace the development of the conceptual structure of the field over time. For this purpose, we examined the average publication year of keywords ( Van Eck and Waltman, 2020 ). Figure 5 represents the overlay visualisation of the keywords’ co-occurrence network, where a colour scale indicates the average publication year of keywords. Figure 5 shows that the keywords corresponding to the red cluster are the oldest, whereas those in the blue cluster are the most recent ones, showing a very recent academic interest.
In addition, we examined the development of the conceptual structure of the field of AI in CRM, looking at the co-occurrence of keywords by considering the importance within the academic community. For this purpose, we considered the “average normalized number of citations received by the articles in which a keyword occurs” ( Van Eck and Waltman, 2020 ) ( Figure 6 ):
[…] the normalized number of citations of an article equals the number of citations of the article divided by the average number of citations of all articles published in the same year and included in the data that is provided to VOS viewer ( Van Eck and Waltman, 2020 ).
Thus, normalisation accounts for the fact that older articles have had more time to receive citations ( Van Eck and Waltman, 2020 ). In general, keywords within the blue cluster are the most cited, although with some exceptions.
Overall, the results of these analyses make it possible to draw some interesting insights about the rising trends within the field of AI in CRM.
Within the red cluster, the keyword with the most recent average publication year is Big Data (i.e. 2018), higher than that of artificial intelligence (i.e. 2015,8). Big Data also has a higher average normalised citation index than artificial intelligence (1,14 versus 0,97). This shows that the red cluster, despite being the oldest, is still evolving; thus, new research opportunities on information management when dealing with Big Data, as well as its impact on CRM, arise.
In addition, as shown in Figures 5 and 6 , keywords such as text mining, Big Data analytics, customer journey and Internet of Things are very recent, and the articles in which they occur are widely cited, meaning that they are strong rising themes within the field of AI in CRM.
Text mining, which appears in the green cluster, is an AI-powered technique for transforming unstructured text into structured data suitable for analysis or driving ML algorithms. Furthermore, it is strongly related to sales and deep learning. The former relationship highlights the rising trend of using text mining to spot and prevent decreasing sales ( McColl-Kennedy et al. , 2019 ), while the latter emphasises the increasing interest in deeper text analytics enabled by deep learning ( Ojo and Rizun, 2019 ). However, deep learning in the CRM domain is still in its infancy. The keyword deep learning occurred only seven times in the sample, with a paper average publication year of 2019,7.
The keyword of Big Data analytics belongs to the blue cluster and is strongly related to customer relationship management, customer experience and business intelligence . The differences among Big Data analytics, ML and AI applied to CRM should be stressed. Big Data analytics develop insights from data and information within CRM systems to support decision-making. ML optimises decision-making by creating predictions, whereas AI produces actions and makes decisions independently ( Grover et al. , 2018 ; Holmlund et al. , 2020 ).
The customer journey keyword also belongs to the blue cluster and is strongly related to artificial intelligence, customer experience and privacy , but the node size and position show that it has not yet caught much scholarly attention. However, Figures 5 and 6 show that the customer journey keyword is very recent, and the articles in which it occurs are widely cited, meaning that customer journey has become an important research topic unbound from CRM. In addition, the strong nearness between privacy and customer journey keywords depicts the nascent consideration of the privacy issue that necessarily occurs when AI is deployed in the interaction between brand and users ( Puntoni et al. , 2020 ).
The Internet of Things keyword is strongly related to artificial intelligence, customer relationship management and customer experience. The Internet of Things (IoT) powered by AI is dramatically transforming CX and CRM. While IoT deals with interacting devices through the internet, AI makes devices learn from their data and experience. IoT supports organisations to innovate CRM in terms of trackage of customers’ behaviour in real time and automation of data sourcing, enhancement of situational awareness, sensor-driven decision analytics for retailing and marketing and automated monitor and replying to the customer ( Lokuge et al. , 2020 ; Ng and Wakenshaw, 2017 ).
Finally, chatbot , an AI-enabled tool frequently used in organisations to facilitate processes, especially those related to after sales and personalisation ( Przegalinska et al. , 2019 ), has not yet captured much scholarly attention ( Figure 6 ).
4. Discussion
The results illustrate a sharp fit among the three clusters resulting from bibliographic coupling, which holds articles sharing common references, and the three clusters resulting from the keywords’ co-occurrence, which groups the most frequently related keywords. On this basis, combining the findings of bibliographic coupling and keywords’ co-occurrence, we identified three subfields of research on AI in CRM and captured promising paths for future development, as summarised in Figure 7 .
The first subfield of research, labelled “Big Data and CRM as a database”, considers CRM as a database on business prospects and customers and focusses on the information management of Big Data within CRM. This subfield is the oldest to be studied, even though it is not yet mature. Within this subfield, we identified two main themes: information management and social media.
Information management is concerned with the analytical part of CRM ( Buttle, 2009 ). It involves collecting, organising and using information related to customers and supports executives in developing insights into consumer preferences and behaviour ( Thakur and Chetty, 2019 ) . Given the growing interest in value creation from incorporating Big Data into CRM decisions, companies are recognising the value of data to obtain an increasing amount of detailed information about their customers and the power of Big Data analytics to improve the decision-making process ( Bernardino and Neves, 2016 ). However, the advent of Big Data has led to even more challenges as companies struggle to develop analytical capabilities, intended as abilities that organisations use for extracting useful information from data, which can support organisations’ ability to identify, attain and retain profitable customers ( Kumar et al. , 2020 ; Mikalef et al. , 2020 ; Wang and Feng, 2012 ). Investigating this issue is not only interesting for companies’ managers but also for all other entities who are involved in information management (e.g. data managers, data architects, regulators and suppliers) ( Kumar et al. , 2020 ).
The results also maintain a rising interest in social CRM (SCRM) ( Anshari et al. , 2015 ; Chang, 2018 ; El Fazziki et al. , 2017 ) and SCRM analysis ( He et al. , 2015 ). In today’s competitive business environment, companies increasingly need to listen to, as well as understand, customers’ expectations, opinions and conversations on social media networks and analyse them within a CRM system to obtain meaningful insights regarding their business opportunities. In this view, Del Vecchio et al. (2020) demonstrated how the integration of Big Data analytics and netnography is relevant for the development of an effective CRM strategy. However, the application of AI techniques to social network analysis approaches to transform the considerable data available on social media into actionable insights for CRM is still little explored in the literature, and fresh research along this perspective is more than welcome.
In addition, as companies are increasingly investing resources in Big Data and social media without completely acknowledging the return on these investments, scholars should deepen this issue, investigating how to measure first, and then maximise the return on Big Data applied to CRM and SCRM investments. In this context, scholars might supplement the performance methods usually used in marketing with those frequently used in information system research to improve the assessment of these investments ( Maklan et al. , 2015 ).
Findings have also proved a growing interest in the application of sentiment analysis on Big Data obtained through social media networks ( El Fazziki et al. , 2017 ; He et al. , 2015 ). Sentiment analysis can be a powerful weapon to increase customers’ vision not only outside the company but also within, exploiting CRM capabilities in collecting and analysing customer data. Organisations can use sentiment analysis to analyse verbal and textual exchanges with customers throughout the customer journey, from negotiation to post purchase, to request or after sale assistance, and new research along all these perspectives is recommended. In accordance with Kietzmann and Pitt (2020) , we also encourage academics to use AI to obtain value from the text and other contents created by companies and their customers.
The second subfield of research focuses on “ AI and ML techniques applied to CRM activities ” and in accordance with Wang and Hajli (2017) , we observe that this constantly growing body of research has mostly addressed the development, analysis and comparison of different AI and ML techniques. However, to completely benefit from AI and ML techniques within CRM systems, business organisations need to approach them from a strategic viewpoint rather than from a mere technical viewpoint ( Iansiti and Lakhani, 2020 ). In particular, CRM requirements, capabilities and practices must be reviewed, and their impacts on people’s behaviour and performance must be understood, ensuring that the new technological applications fit the organisational context and CRM strategy ( Catalan-Matamoros, 2012 ).
In addition, articles within this subfield compare innovative ML-enabled techniques, developed for specific functional applications, such as high-value customer identification, customer churn prediction and customer lifetime value prediction, with long-established techniques. In particular, the two most promising techniques identified in the study are text mining and deep learning . The fruitful areas of practical development of text mining are the prevention of sales decreases and derivation of latent brand topics. Areas of rising managerial and academic interest regarding the use of deep learning in CRM are opinion analysis, entity recognition and predictive modelling.
In fact, some studies have validated a specific technique in a real-world setting ( Spiess et al. , 2014 ; Chatterjee et al. , 2020a , 2020b ; Mogaji et al. , 2020 ); however, these examples are still few and isolated, and the literature does not yet provide an overarching big picture that presents and compares these new techniques and their applications. As the selection of a technique depends on many factors (e.g. sector, marketplace, level of personalisation or customer intrusion), it would be useful to provide an overview of the different techniques along with their features, application domains, requirements and outputs, thus providing guidelines to support managers to choose the most suitable technique that optimally uses the available data within a specific context.
Researchers can also advance the theory in the field by finding common patterns between techniques with different CRM-related purposes and applications or by identifying new techniques in business applications that can be adopted to carry out unconventional CRM-related activities. For instance, a convolutional neural network (CNN) has been adopted to analyse textual information ( De Caigny et al. , 2020a , 2020b ), but it can also be applied to a different data source (e.g. time-series data) or for a different purpose (e.g. sentiment and intent analyses of customer reviews). Therefore, future research can investigate the incorporation of time-series data into customer churn prediction models based on a CNN or of time-series data in sentiment and intent analyses of customers’ reviews based on a CNN.
The third subfield of research, labelled as “ Strategic management of AI-CRM integrations ”, looks at AI–CRM integration from a broader strategic viewpoint, rather than analysing specific technological applications. This subfield considers CRM as a tool that drives strategy through actionable insights, rather than as a database, and focusses on AI applied to CRM within a customer-centric vision. This third cluster contains the most recent papers, which start to debate AI considering the challenges, benefits and advantages it can provide to CRM processes and considering the required organisational, cultural and strategic changes. The shift in perspective from technology development to strategy advancement reflects the growing interest in conducting a renewed examination of how technology interacts with the CRM strategy. AI will have a disruptive impact on the strategy development process. For instance, AI can identify future events in the market, estimate product demand ( Arco et al. , 2019 ; Campbell et al. , 2020 ; Kumar et al. , 2020 ), implement a dynamic customer strategy ( Yi, 2018 ), optimise targeting decisions, customise messaging to specific target audiences and identify bestselling characteristics to address ( Kumar et al. , 2019 ). As other tasks will be redefined, AI will lead to several possibilities for CRM strategies and process innovations ( Tekic et al. , 2019 ). However, this literature is still in its infancy, with very few case studies. For instance, researchers can deeply investigate the strategic, operational and organisational changes that AI–CRM integration entail ( Wang and Hajli, 2017 ), how these changes will affect employees as well as customers ( Kumar et al. , 2020 ) and how AI–CRM integration will influence the success of CRM projects. In examining these aspects, scholars should broaden their perspective and consider hybrid organisational systems, which include both humans and AI, exploring their interactive behaviours ( Raisch and Krakowski, 2020 ).
In addition, we identify three main themes that can be addressed in the future: customer journey, chatbots and IoT.
Customer journey mapping and customer decision journey might be impacted by the fresh knowledge made available by AI, characterised by higher accuracy, suitability and timeliness, thus leaving room for investigation ( Lemon and Verhoef, 2016 ). AI can allow a deeper dive into customer decision journeys, identifying an opportunity for an intervention or change ( Lemon and Verhoef, 2016 ) as, for instance, automatically identifying potential anomalies in customer behaviour or negative sentiments. As AI will infer customer behaviours, trends and preferences ( Marinchak et al. , 2018 ), privacy issues will become a priority. Chatterjee et al. (2020a , 2020b ) are among the first to investigate the adoption of AI-integrated CRM systems from a privacy perspective, paving the way for an interesting path for future research. To the best of our knowledge, no study has yet deeply investigated risks, regulatory strategies and customer protection policies concerning AI applications in CRM systems.
Another promising research theme concerns chatbots, which are increasingly used in customer service to tackle requests or complaints, even though customers are still perceiving some risks in their use. Recently, academics have started exploring the implementation processes, impacts, drivers and challenges of chatbot application in marketing ( Sujata et al. , 2019 ) and CRM ( Anjali Daisy, 2020 ), providing insights to reduce the customers’ perceived risk in its use ( Trivedi, 2019 ). However, no studies have yet investigated the ethical issues beyond conversational service automation platform construction (chatbots). In addition, chatbots used together with other technologies, such as smartphones, virtual assistants and augmented reality, will increase the omnichannel strategy intricacy ( Wilson-Nash et al. , 2020 ), and AI will help to predict the optimal combination of channels to reach customers ( Hopkinson and Singhal, 2018 ). The use of AI to deliver coherent streams of interaction across diverse touchpoints is another relevant research issue that calls for further research ( Singh et al. , 2020 ).
Another rising theme is IoT, which is considered a key disruptive technology for CRM in the future ( Lokuge et al. , 2020 ). Allowing the collection of customers’ real-time data, IoT makes the relationship with CRM intriguing. How firms might use IoT to design and build exceptional CX, how IoT can bring CRM to a higher level, the so-called “CRM of everything” and how real data can generate insights to take actions are some of the open questions that need to be researched ( Abu Ghazaleh and Zabadi, 2020 ; Lokuge et al. , 2020 ).
Taking an overarching perspective, the reviewed articles contribute to advancing knowledge in the field of AI in CRM by categorising phenomena, providing intellectual insights or developing frameworks to create an overall understanding. However, papers that advance theories formulating propositions or test theories remain scant. Thus, we wish future contributions to expand the theoretical reference background to better comprehend how AI is shaping CRM.
In this light, more research that elaborates strategic management theories on pivotal decisions concerning AI–CRM integration is required. For example, in terms of making or buying decisions, both the resource-based view (RBV) theory ( Barney, 1991 ) and the transaction cost economics (TCE) ( Williamson, 1979 ) theory can serve as theoretical lenses to evaluate whether AI–CRM integration should be developed in-house or outsourced. In particular, following RBV, the AI application should be implemented internally if it is considered a fundamental capability for developing knowledge in managing customer relationships and maintaining competitive advantage ( Grover et al. , 2018 ; Zerbino et al. , 2018 ). Following the TCE theory, considerations related to asset specificity, opportunism, frequency of transaction and environmental/behavioural uncertainty should be taken into account when deciding between developing AI internally or outsourcing. Turning now to the decision on the level of AI automation–augmentation, management scholars should examine the extent to which AI results influence human decision-making within CRM strategies to clarify the real impact of different levels of AI automation–augmentation on CRM performance. To date, in most cases, within the CRM domain, the final decision still remains with humans. However, the more AI applications are automated, the more they will lead the decision-making process. In this view, humans should interact with AI algorithms to drive the CRM decision-making process. For example, AI analyses consumers’ past behaviours to transfer the most promising sales opportunities to vendors. Based on this issue, the prospect theory, which maintains that human decision-making depends on choosing among options that can themselves rest on biased judgments ( Kahneman et al. , 1979 ), can be adopted as a theoretical perspective to examine the influence of AI on human decision-making. Some may fear that the automation afforded by AI technologies will replace humans, whereas others state that they will elevate employees’ roles, which will allow them to invest their time in creative tasks rather than in mere operating processes ( Campbell et al. , 2020 ). What is certain is that humans will have less and less interface with unprocessed data, and their tasks will increasingly be impacted by AI ( Rust, 2020 ). Thus, future research should also investigate what humans’ new tasks will be, what capabilities a company must have to remain competitive and how humans will interact with AI. Furthermore, humans should be able to transparently identify the logic behind a given decision and to verify the morality of the action; thus, AI must be programmed with a rule-based system of ethics. However, we observed a lack of studies on ethics applied to AI–CRM integration. Future studies can also grasp ethical issues by using the lens of different pre-existing ethical theories, such as deontological, utilitarian or virtue ethics ( Manna and Nath, 2021 ) and, thus, help managers find the right balance between ethical concern consideration and AI application effectiveness.
Finally, only a few studies have used a longitudinal perspective for analysing how AI–CRM integration projects are implemented and develop over time; thus, we recommend that future studies should adopt a process theory approach in investigating this subject.
5. Conclusion
Answering the call of Raisch and Krakowski (2020) to develop comprehensive perspectives on the AI debate in management, this study identifies and describes three subfields that shape and characterise this literature within the CRM domain: Big Data and CRM as a database, AI and ML techniques applied to CRM activities and strategic management of AI–CRM integrations.
The findings suggest that CRM is evolving from a data-driven strategy to an AI-driven strategy ( Colson, 2019 ). In addition, very recently, scholars have been approaching the topic with a broader strategic perspective to harness the power of AI to improve CRM, rather than only investigating specific technological applications to maximise the operational efficiency or CX within a single CRM activity. However, further development of the two subfields “Big Data and CRM as a database” and “AI and ML techniques applied to CRM activities” might be beneficial to support the growth of the third subfield.
Based on our findings, we developed a conceptual model ( Figure 8 ) that integrates the identified subfields and proposes a three-step strategy for AI implementation in CRM:
information management of Big Data;
technology investigation of AI and ML techniques applied to CRM activities; and
AI-driven business transformation.
The model sets out the initiatives and actions that should be deployed in each step to achieve an AI-driven CRM strategy. For this purpose, managers need to set the strategic goals of business transformation from the beginning and start by creating a unique customer data platform from which new information can be integrated into CRM decisions. Subsequently, they can investigate specific algorithms meant to solve narrow business challenges related to customer relationships; in this context, managers need to reflect upon new employees’ competencies, keeping in mind the strategic, operational and organisational changes. Finally, once specific applications have been successfully implemented, managers can move to the last step, where an overarching AI-driven CRM strategy is fully realised. The model can assist executives and managers in identifying the appropriate and consistent business strategy for the effective integration of AI into CRM systems.
This study makes several significant contributions to theory and practice. First, from an academic viewpoint, this study traces the development of research on AI in CRM, depicting the main subfields that have characterised the recent evolution of this fragmented literature. These findings are underpinned by a robust literature review and bibliometric techniques that allow mapping of the research field without subjective bias. Accordingly, this study empowers scholars to gain a one-step overview of AI in CRM and positions their intended contributions within this field (Donthu et al. , 2021). Second, this study outlines the main underdeveloped issues on this topic and addresses avenues for future novel research. The results will enable academics from various domains (e.g. Big Data, AI, customer relationship and marketing management) to work further to develop a common understanding of the relationship between AI and CRM, which will ultimately advance knowledge and benefit organisations. Third, this study develops a three-step conceptual model for AI implementation in CRM, which might support scholars in further deepening the knowledge in this field, also through empirical analysis. The model is far from encompassing all actions for an effective implementation; consequently, further research is required to enrich it and make it contingent to different business contexts.
From a managerial viewpoint, this study offers insights for organisations and managers willing to enable CRM systems to take advantage of the opportunities offered by AI, providing guidelines to capture the main directions along which AI–CRM integration is evolving and managerial practices to make this integration forceful and productive. The proposed model serves as a guideline tool for executives and managers to plan an appropriate and consistent strategy for AI implementation in CRM and improve efficiency in the information management of Big Data, technology investigation of AI and ML techniques and AI-driven business transformation. In addition, the model can provide practical knowledge for organisations to conduct a self-introspection of their strategy and assess if they are missing actions for an effective implementation of AI into CRM systems.
Despite these contributions, our study has some limitations that can also offer avenues for extending research. First, limitations are mainly related to the shortcomings of citing behaviour, because bibliographic coupling does not capture the objectives or motivations that guided the authors in citing prior articles ( Vogel and Güttel, 2013 ; Soranzo et al. , 2016 ). Furthermore, articles with more references are over-weighted as they probably present more intersections with the references of other articles ( Agostini and Nosella, 2019 ). Second, future work can operationalise and test the proposed conceptual model, identifying possible moderators, mediators and controlling factors to build a comprehensive and thorough understanding.
In conclusion, we hope that our study can be a source of inspiration for future studies to advance knowledge of AI in the CRM domain, as it provides useful knowledge to design and publish fresh research and highlights emerging themes that can offer significant theoretical and empirical contributions to the field of CRM and beyond.
Search and selection of articles considered in this study
Temporal distribution of the filtered initial data set
Bibliographic coupling network, with a minimum of one citation
Keywords’ co-occurrence network, with a minimum of four occurrences
Overlay visualisation – average publication year
Source: (colour printing)
Overlay visualisation – average normalised citations
Promising paths for future research related to AI in CRM
Three-step strategy for AI implementation in CRM
More related articles in the data set (based on total link strength)
Top 10 most local cited articles
Occurrences of keywords
The table divides 55 top keywords into the three clusters to which they belong and presents the occurrences (weight) of each keyword from 4
Abu Ghazaleh , M. and Zabadi , A.M. ( 2020 ), “ Promoting a revamped CRM through internet of things and big data: an AHP-based evaluation ”, International Journal of Organizational Analysis , Vol. 28 No. 1 , pp. 66 - 91 .
Agostini , L. and Nosella , A. ( 2019 ), “ Inter-organizational relationships involving SMEs: a bibliographic investigation into the state of the art ”, Long Range Planning , Vol. 52 No. 1 , pp. 1 - 31 .
Anjali Daisy , S. ( 2020 ), “ Stimulus of chatbot with customer relationship pipeline ”, Journal of Advanced Research in Dynamical and Control Systems , Vol. 12 No. 5 , pp. 885 - 889 , Institute of Advanced Scientific Research, Inc., SASTRA Deemed University, India .
Anshari , M. , Alas , Y. , Yunus , N. , Sabtu , N.I. and Hamid , M.H. ( 2015 ), “ Social customer relationship management and student empowerment in online learning systems ”, International Journal of Electronic Customer Relationship Management , Vol. 9 Nos 2/3 , pp. 104 - 121 .
Arco , M.D. , Presti , L.L. , Marino , V. and Resciniti , R. ( 2019 ), “ Embracing AI and big data in customer journey mapping: from literature review to a theoretical framework ”, Innovative Marketing , Vol. 15 No. 4 , pp. 102 - 115 .
Barney , J. ( 1991 ), “ Firm resources and sustained competitive advantage ”, Journal of Management , Vol. 17 No. 1 , pp. 99 - 120 .
Bernardino , J. and Neves , P.C. ( 2016 ), “ Decision-making with big data using open source business intelligence systems ”, in Rahman , H. (Eds), Human Development and Interaction in the Age of Ubiquitous Technology , IGI Global , Hershey, PA , pp. 120 - 147 .
Borges , A.F.S. , Laurindo , F.J.B. , Spínola , M.M. , Gonçalves , R.F. and Mattos , C.A. ( 2020 ), “ The strategic use of artificial intelligence in the digital era: systematic literature review and future research directions ”, International Journal of Information Management , Vol. 57 , p. 102225 , Elsevier, No. August .
Boulding , W. , Staelin , R. , Ehret , M. and Johnston , W.J. ( 2005 ), “ A customer relationship management roadmap: what is known, potential pitfalls, and where to go ”, Journal of Marketing , Vol. 69 No. 4 , pp. 155 - 166 , No. November 2015 .
Boyack , K.W. and Klavans , R. ( 2010 ), “ Co-citation analysis, bibliographic coupling, and direct citation: which citation approach represents the research front most accurately? ”, Journal of the American Society for Information Science and Technology , Vol. 61 No. 12 , pp. 2389 - 2404 .
Bresciani , S. , Ciampi , F. , Meli , F. and Ferraris , A. ( 2021 ), “ Using big data for co-innovation processes: mapping the field of data-driven innovation, proposing theoretical developments and providing a research agenda ”, International Journal of Information Management , Vol. 60 , p. 102347 , Elsevier Ltd, 20 March .
Brynjolfsson , B.Y.E. and McAfee , A. ( 2017 ), “ Artificial intelligence for real ”, Harvard Business Review , Vol. 1 , pp. 1 - 31 , July .
Buckley , R. and Webster , S. ( 2016 ), “ Fintech in developing countries: charting new customer journeys ”, Journal of Financial Transformation , Vol. 44 , pp. 151 - 159 .
Buttle , F. ( 2009 ), Customer Relationship Management: Concepts and Technologies , 4th ed. , Routledge, Taylor and Francis Group , Abingdon, Oxon; New York, NY .
Callon , M. , Courtial , J.P. , Turner , W.A. and Bauin , S. ( 1983 ), “ From translations to problematic networks: an introduction to co-word analysis ”, Social Science Information , Vol. 22 No. 2 , pp. 191 - 235 .
Campbell , C. , Sands , S. , Ferraro , C. , Tsao , H.-Y.J. and Mavrommatis , A. ( 2020 ), “ From data to action: how marketers can leverage AI ”, Business Horizons , Vol. 63 No. 2 , pp. 227 - 243 .
Catalan-Matamoros , D. ( 2012 ), “ An overview to customer relationship management ”, in Catalan-Matamoros , D. (Eds), Advances in Customer Relationship Management , IntechOpen , London .
Chang , J.W. , Lee , M.C. and Wang , T.I. ( 2016 ), “ Integrating a semantic-based retrieval agent into case-based reasoning systems: a case study of an online bookstore ”, Computers in Industry , Vol. 78 , pp. 29 - 42 .
Chang , V. ( 2018 ), “ A proposed social network analysis platform for big data analytics ”, Technological Forecasting and Social Change , Vol. 130 , pp. 57 - 68 .
Chatterjee , S. , Ghosh , S.K. , Chaudhuri , R. and Nguyen , B. ( 2019 ), “ Are CRM systems ready for AI integration?: a conceptual framework of organizational readiness for effective AI-CRM integration ”, The Bottom Line , Vol. 32 No. 2 , pp. 144 - 157 .
Chatterjee , S. , Ghosh , S.K. and Chaudhuri , R. ( 2020a ), “ Knowledge management in improving business process: an interpretative framework for successful implementation of AI–CRM–KM system in organizations ”, Business Process Management Journal , Vol. 26 No. 6 , pp. 1261 - 1281 , doi: 10.1108/BPMJ-05-2019-0183 .
Chatterjee , S. , Ghosh , S.K. , Chaudhuri , R. and Chaudhuri , S. ( 2020b ), “ Adoption of AI-integrated CRM system by Indian industry: from security and privacy perspective ”, Information & Computer Security , Vol. 29 No. 1 , pp. 1 - 24 , doi: 10.1108/ICS-02-2019-0029 , Emerald Group Publishing Ltd. , Department of Computer Science and Engineering, Indian Institute of Technology, Kharagpur, India .
Chiang , W.Y. ( 2019 ), “ Establishing high value markets for data-driven customer relationship management systems: an empirical case study ”, Kybernetes , Vol. 48 No. 3 , pp. 650 - 662 .
Ciampi , F. , Marzi , G. , Demi , S. and Faraoni , M. ( 2020 ), “ The big data-business strategy interconnection: a grand challenge for knowledge management. a review and future perspectives ”, Journal of Knowledge Management , Vol. 24 No. 5 , pp. 1157 - 1176 .
Colson , E. ( 2019 ), “ What AI-Driven decision making looks like ”, Harvard Business Review , available at: https://hbr.org/2019/07/what-ai-driven-decision-making-looks-like ( accessed 20 December 2021 ).
De Caigny , A. , Coussement , K. and De Bock , K.W. ( 2020a ), “ Leveraging fine-grained transaction data for customer life event predictions ”, Decision Support Systems , Vol. 130 , p. 113232 , doi: 10.1016/j.dss.2019.113232 .
De Caigny , A. , Coussement , K. , De Bock , K.W. and Lessmann , S. ( 2020b ), “ Incorporating textual information in customer churn prediction models based on a convolutional neural network ”, International Journal of Forecasting , Vol. 36 No. 4 , pp. 1563 - 1578 , doi: 10.1016/j.ijforecast.2019.03.029 .
Del Vecchio , P. , Mele , G. , Passiante , G. , Vrontis , D. and Fanuli , C. ( 2020 ), “ Detecting customers knowledge from social media big data: toward an integrated methodological framework based on netnography and business analytics ”, Journal of Knowledge Management , Vol. 24 No. 4 , pp. 799 - 821 .
Deshpande , A. and Kumar , M. ( 2018 ), “ Artificial intelligence for big data ”, Journal of Chemical Information and Modeling , Vol. 53 No. 9 , pp. 1689 - 1699 .
Donthu , N. , Kumar , S. , Mukherjee , D. , Pandey , N. and Lim , W.M. ( 2021 ), “ How to conduct a bibliometric analysis: an overview and guidelines ”, Journal of Business Research , Vol. 133 , pp. 285 - 296 , No. March .
El Fazziki , A. , Ennaji , F.Z. , Sadiq , A. , Benslimane , D. and Sadgal , M. ( 2017 ), “ A multi-agent based social crm framework for extracting and analysing opinions ”, Journal of Engineering Science and Technology , Vol. 12 No. 8 , pp. 2154 - 2174 .
Fluss , D. ( 2017 ), “ Will customer journey analysis be the next CRM ”, CRM Magazine , available at: www.destinationcrm.com/Articles/Columns-Departments/Reality-Check/Will-Customer-Journey-Analytics-Be-the-Next-CRM-117384.aspx ( accessed 20 December 2021 ).
Grover , V. , Chiang , R.H.L. , Liang , T.-P. and Zhang , D. ( 2018 ), “ Creating strategic business value from big data analytics: a research framework ”, Journal of Management Information Systems , Vol. 35 No. 2 , pp. 388 - 423 .
Hallikainen , H. , Savimäki , E. and Laukkanen , T. ( 2020 ), “ Fostering B2B sales with customer big data analytics ”, Industrial Marketing Management , Vol. 86 , pp. 90 - 98 .
He , W. , Wu , H. , Yan , G. , Akula , V. and Shen , J. ( 2015 ), “ A novel social media competitive analytics framework with sentiment benchmarks ”, Information & Management , Vol. 52 No. 7 , pp. 801 - 812 .
Holmlund , M. , Van Vaerenbergh , Y. , Ciuchita , R. , Ravald , A. , Sarantopoulos , P. , Ordenes , F.V. and Zaki , M. ( 2020 ), “ Customer experience management in the age of big data analytics: a strategic framework ”, Journal of Business Research , Vol. 116 , pp. 356 - 365 , doi: 10.1016/j.jbusres.2020.01.022 , No. August .
Hopkinson , P.J. and Singhal , A. ( 2018 ), “ Exploring the use of AI to manage customers’ relationships ”, in 51st Academy of Marketing Conference , University of Stirling, United Kingdom , pp. 1 - 7 .
Iansiti , M. and Lakhani , K.R. ( 2020 ), Competing in the Age of AI: Strategy and Leadership When Algorithms and Networks Run the World , Harvard Business Review Press , Boston .
Kahneman , D. , Tversky , A. and Tversky , A. ( 1979 ), “ Prospect theory: an analysis of decision under risk ”, Econometrica , Vol. 47 No. 2 , pp. 263 - 292 .
Kaplan , A. and Haenlein , M. ( 2019 ), “ Siri, Siri, in my hand: who’s the fairest in the land? On the interpretations, illustrations, and implications of artificial intelligence ”, Business Horizons , Vol. 62 No. 1 , pp. 15 - 25 , “Kelley School of Business, Indiana University” .
Khan , G.F. and Wood , J. ( 2015 ), “ Information technology management domain: emerging themes and keyword analysis ”, Scientometrics , Vol. 105 No. 2 , pp. 959 - 972 , Springer Netherlands .
Khodakarami , F. and Chan , Y.E. ( 2014 ), “ Exploring the role of customer relationship management (CRM) systems in customer knowledge creation ”, Information & Management , Vol. 51 No. 1 , pp. 27 - 42 .
Kietzmann , J. and Pitt , L.F. ( 2020 ), “ Computerized content analysis of online data – opportunities for marketing scholars and practitioners ”, European Journal of Marketing , Vol. 54 No. 3 , pp. 473 - 477 .
Kim , Y. , Jang , S.N. and Lee , J.L. ( 2018 ), “ Co-occurrence network analysis of keywords in geriatric frailty ”, Journal of Korean Academy of Community Health Nursing , Vol. 29 No. 4 , pp. 429 - 439 .
Klaus , P. and Zaichkowsky , J. ( 2020 ), “ AI voice bots: a services marketing research agenda ”, Journal of Services Marketing , Vol. 34 No. 3 , pp. 389 - 398 .
Kumar , M. and Misra , M. ( 2021 ), “ Evaluating the effects of CRM practices on organizational learning, its antecedents and level of customer satisfaction ”, Journal of Business & Industrial Marketing , Vol. 36 No. 1 , pp. 164 - 176 .
Kumar , V. , Rajan , B. , Venkatesan , R. and Lecinski , J. ( 2019 ), “ Understanding the role of artificial intelligence in personalized engagement marketing ”, California Management Review , Vol. 61 No. 4 , pp. 135 - 155 .
Kumar , V. , Ramachandran , D. and Kumar , B. ( 2020 ), “ Influence of new-age technologies on marketing: a research agenda ”, Journal of Business Research , Vol. 125 , pp. 864 - 877 , doi: 10.1016/j.jbusres.2020.01.007 , No. March .
Lemon , K.N. and Verhoef , P.C. ( 2016 ), “ Understanding customer experience throughout the customer journey ”, Journal of Marketing , Vol. 80 No. 6 , pp. 69 - 96 .
Libai , B. , Bart , Y. , Gensler , S. , Hofacker , C.F. , Kaplan , A. , Kötterheinrich , K. and Kroll , E.B. ( 2020 ), “ Brave new world? On AI and the management of customer relationships ”, Journal of Interactive Marketing , Vol. 51 , pp. 44 - 56 , doi: 10.1016/j.intmar.2020.04.002 .
Liu , X. , Burns , A.C. and Hou , Y. ( 2017 ), “ An investigation of brand-related user-generated content on twitter ”, Journal of Advertising , Vol. 46 No. 2 , pp. 236 - 247 .
Lokuge , S. , Sedera , D. , Kumar , S. , Ariyachandra , T. and Ravi , V. ( 2020 ), “ The next wave of CRM innovation: implications for research, teaching, and practice ”, Communications of the Association for Information Systems , Vol. 46 , pp. 560 - 583 .
Loureiro , S.M.C. , Guerreiro , J. and Tussyadiah , I. ( 2021 ), “ Artificial intelligence in business: state of the art and future research agenda ”, Journal of Business Research , Vol. 129 , pp. 911 - 926 , doi: 10.1016/j.jbusres.2020.11.001 , No. May .
McCain , K.W. ( 1990 ), “ Mapping authors in intellectual space: a technical overview ”, Journal of the American Society for Information Science , Vol. 41 No. 6 , p. 433 .
McColl-Kennedy , J.R. , Zaki , M. , Lemon , K.N. , Urmetzer , F. and Neely , A. ( 2019 ), “ Gaining customer experience insights that matter ”, Journal of Service Research , Vol. 22 No. 1 , pp. 8 - 26 .
Maklan , S. , Peppard , J. and Klaus , P. ( 2015 ), “ Show me the money improving our understanding of how organizations generate return from technology-led marketing change ”, European Journal of Marketing , Vol. 49 Nos 3/4 , pp. 561 - 595 .
Manna , R. and Nath , R. ( 2021 ), “ The problem of moral agency in artificial intelligence ”, paper presented at IEEE Conference on Norbert Wiener in the 21st Century: Being Human in a Global Village , 22-25 July 2021 , CEG Campus, Anna University Chennai , doi: 10.1109/21CW48944.2021.9532549 .
Marchiori , D. and Franco , M. ( 2020 ), “ Knowledge transfer in the context of inter-organizational networks: foundations and intellectual structures ”, Journal of Innovation & Knowledge , Vol. 5 No. 2 , pp. 130 - 139 .
Marinchak , C.M. , Forrest , E. and Hoanca , B. ( 2018 ), “ Artificial intelligence: redefining marketing management and the customer experience ”, International Journal of E-Entrepreneurship and Innovation , Vol. 8 No. 2 , pp. 14 - 24 .
Mikalef , P. , Krogstie , J. , Pappas , I.O. and Pavlou , P. ( 2020 ), “ Exploring the relationship between big data analytics capability and competitive performance: the mediating roles of dynamic and operational capabilities ”, Information & Management , Vol. 57 No. 2 , North-Holland .
Mishra , N. and Mukherjee , S. ( 2019 ), “ Effect of artificial intelligence on customer relationship management of amazon in Bangalore ”, International Journal of Management , Vol. 10 No. 4 , pp. 168 - 172 .
Mogaji , E. , Soetan , T.O. and Kieu , T.A. ( 2020 ), “ The implications of artificial intelligence on the digital marketing of financial services to vulnerable customers ”, Australasian Marketing Journal , Vol. 29 No. 3 , pp. 235 - 242 , doi: 10.1016/j.ausmj.2020.05.003 .
Mukherjee , S. and Bala , P.K. ( 2017 ), “ Detecting sarcasm in customer tweets: an NLP based approach ”, Industrial Management & Data Systems , Vol. 117 No. 6 , pp. 1109 - 1126 .
Ng , I.C.L. and Wakenshaw , S.Y.L. ( 2017 ), “ The internet-of-things: review and research directions ”, International Journal of Research in Marketing , Vol. 34 No. 1 , pp. 3 - 21 , doi: 10.1016/j.ijresmar.2016.11.003 .
Niknejad , N. , Ismail , W. , Bahari , M. , Hendradi , R. and Salleh , A.Z. ( 2021 ), “ Mapping the research trends on blockchain technology in food and agriculture industry: a bibliometric analysis ”, Environmental Technology & Innovation , Vol. 21 , p. 101272 .
Ojo , A. and Rizun , N. ( 2019 ), “ Enabling deeper linguistic-based text analytics – construct development for the criticality of negative service experience ”, IEEE Access , Vol. 7 , pp. 169217 - 169256 , doi: 10.1109/ACCESS.2019.2947593 .
Paquette , S. ( 2010 ), “ Customer knowledge management ”, in Schwartz , D. and Te’eni , D. (Eds), Encyclopedia of Knowledge Management , IGI Global , Hershey, PA , pp. 175 - 184 .
Payne , A. and Frow , P. ( 2005 ), “ A strategic framework for customer relationship management ”, Journal of Marketing , Vol. 69 No. 4 , pp. 167 - 176 .
Pearson , A. ( 2019 ), “ Personalisation the artificial intelligence way ”, Journal of Digital and Social Media Marketing , Vol. 7 No. 3 , pp. 245 - 269 .
Prentice , C. and Nguyen , M. ( 2020 ), “ Engaging and retaining customers with AI and employee service ”, Journal of Retailing and Consumer Services , Vol. 56 , p. 102186 , doi: 10.1016/j.jretconser.2020.102186 .
Pritchard , A. ( 1969 ), “ Statistical bibliography or bibliometrics? ”, Journal of Documentation , Vol. 25 No. 4 , pp. 348 - 349 .
Przegalinska , A. , Ciechanowski , L. , Stroz , A. , Gloor , P. and Mazurek , G. ( 2019 ), “ In bot we trust: a new methodology of chatbot performance measures ”, Business Horizons , Vol. 62 No. 6 , pp. 785 - 797 .
Puntoni , S. , Reczek , R.W. , Giesler , M. and Botti , S. ( 2020 ), “ Consumers and artificial intelligence: an experiential perspective ”, Journal of Marketing , Vol. 85 No. 1 , pp. 131 - 151 , doi: 10.1177/0022242920953847 , SAGE Publications Ltd .
Rababah , K. ( 2011 ), “ Customer relationship management (CRM) processes from theory to practice: the pre-implementation plan of CRM system ”, International Journal of e-Education, e-Business, e-Management and e-Learning , Vol. 1 No. 1 , pp. 22 - 27 .
Raisch , S. and Krakowski , S. ( 2020 ), “ Artificial intelligence and management: the automation-augmentation paradox ”, Academy of Management Review , Vol. 46 No. 1 , pp. 1 - 48 .
Richards , K.A. and Jones , E. ( 2008 ), “ Customer relationship management: finding value drivers ”, Industrial Marketing Management , Vol. 37 No. 2 , pp. 120 - 130 .
Rust , R.T. ( 2020 ), “ The future of marketing ”, International Journal of Research in Marketing , Vol. 37 No. 1 , pp. 15 - 26 , Elsevier B.V .
Rust , R.T. and Huang , M.H. ( 2014 ), “ The service revolution and the transformation of marketing science ”, Marketing Science , Vol. 33 No. 2 , pp. 206 - 221 .
Saidulu , D. and Sasikala , R. ( 2017 ), “ Machine learning and statistical approaches for big data: issues, challenges and research directions ”, International Journal of Applied Engineering Research , Vol. 12 No. 21 , pp. 11691 - 11699 .
Schröder , K.B. , Tiberius , V. , Bouncken , R. and Kraus , S. ( 2021 ), “ Strategic entrepreneurship: mapping a research field ”, International Journal of Entrepreneurial Behavior & Research , Vol. 27 No. 3 , pp. 753 - 776 , doi: 10.1108/IJEBR-11-2020-0798 .
Singh , J. , Nambisan , S. , Bridge , R.G. and Brock , J.K.-U. ( 2020 ), “ One-Voice strategy for customer engagement ”, Journal of Service Research , Vol. 24 No. 1 , pp. 42 - 65 , doi: 10.1177/1094670520910267 .
Soranzo , B. , Nosella , A. and Filippini , R. ( 2016 ), “ Managing firm patents: a bibliometric investigation into the state of the art ”, Journal of Engineering and Technology Management , Vol. 42 , pp. 15 - 30 .
Spiess , J. , T’Joens , Y. , Dragnea , R. , Spencer , P. and Philippart , L. ( 2014 ), “ Using big data to improve customer experience and business performance ”, Bell Labs Technical Journal , Vol. 18 No. 4 , pp. 3 - 17 .
Sujata , J. , Nikita , M. and Shubham , S. ( 2019 ), “ Applications of chatbots in marketing: use cases, impacts, challenges and drivers ”, International Journal of Advanced Trends in Computer Science and Engineering , Vol. 8 No. 1.6 , pp. 195 - 200 .
Talón-Ballestero , P. , González-Serrano , L. , Soguero-Ruiz , C. , Muñoz-Romero , S. and Rojo-Álvarez , J.L. ( 2018 ), “ Using big data from customer relationship management information systems to determine the client profile in the hotel sector ”, Tourism Management , Vol. 68 , pp. 187 - 197 .
Tekic , Z. , Cosic , I. and Katalinic , B. ( 2019 ), “ Manufacturing and the rise of artificial intelligence: innovation challenges ”, Annals of DAAAM and Proceedings of the International DAAAM Symposium , Vol. 30 No. 1 , pp. 192 - 196 .
Thakur , S. and Chetty , P. ( 2019 ), “ How Payne’s five process model can help to improve CRM? ”, Project Guru , available at: www.projectguru.in/paynes-five-process-model-crm-strategy/ ( accessed 11 March 2022 ).
Tranfield , D. , Denyer , D. and Smart , P. ( 2003 ), “ Towards a methodology for developing evidence-Informed management knowledge by means of systematic review ”, British Journal of Management , Vol. 14 No. 3 , pp. 207 - 222 , doi: 10.1111/1467-8551.00375 .
Trivedi , J. ( 2019 ), “ Examining the customer experience of using banking chatbots and its impact on brand love: the moderating role of perceived risk ”, Journal of Internet Commerce , Vol. 18 No. 1 , pp. 91 - 111 .
Valenzuela Fernandez , L.M. , Nicolas , C. , Merigó , J.M. and Arroyo-Cañada , F.J. ( 2019 ), “ Industrial marketing research: a bibliometric analysis (1990-2015) ”, Journal of Business & Industrial Marketing , Vol. 34 No. 3 , pp. 550 - 560 , doi: 10.1108/JBIM-07-2017-0167 .
Van Eck , N.J. and Waltman , L. ( 2010 ), “ Software survey: VOSviewer, a computer program for bibliometric mapping ”, Scientometrics , Vol. 84 No. 2 , pp. 523 - 538 .
Van Eck , N.J. and Waltman , L. ( 2020 ), “ Manual for VOSviewer version 1.6.15 .
Vignesh , R. and Vasantha , S. ( 2019 ), “ Significant developments of artificial intelligence in Indian ecommerce industry in 2019 ”, International Journal of Scientific and Technology Research , Vol. 8 No. 12 , pp. 2746 - 2748 .
Villarroel Ordenes , F. and Zhang , S. ( 2019 ), “ From words to pixels: text and image mining methods for service research ”, Journal of Service Management , Vol. 30 No. 5 , pp. 593 - 620 .
Vogel , R. and Güttel , W.H. ( 2013 ), “ The dynamic capability view in strategic management: a bibliometric review ”, International Journal of Management Reviews , Vol. 15 No. 4 , pp. 426 - 446 .
Waltman , L. , Van Eck , N.J. and Noyons , E.C.M. ( 2010 ), “ A unified approach to mapping and clustering of bibliometric networks ”, Journal of Informetrics , Vol. 4 No. 4 , pp. 629 - 635 .
Wang , Y. and Feng , H. ( 2012 ), “ Customer relationship management capabilities: measurement, antecedents and consequences ”, Management Decision , Vol. 50 No. 1 , pp. 115 - 129 .
Wang , Y. and Hajli , N. ( 2017 ), “ Exploring the path to big data analytics success in healthcare ”, Journal of Business Research , Vol. 70 , pp. 287 - 299 , Elsevier Inc .
Williamson , O.E. ( 1979 ), “ Transaction-cost economics: the governance of contractual relations ”, The Journal of Law and Economics , Vol. 22 No. 2 , pp. 233 - 261 .
Wilson-Nash , C. , Goode , A. and Currie , A. ( 2020 ), “ Introducing the socialbot: a novel touchpoint along the young adult customer journey ”, European Journal of Marketing , Vol. 54 No. 10 , pp. 2621 - 2643 , doi: 10.1108/EJM-07-2019-0555 .
Yi , Z. ( 2018 ), “ The application of clustering in customer management based on big data ”, IPPTA: Quarterly Journal of Indian Pulp and Paper Technical Association , Vol. 30 No. 8 , pp. 718 - 722 , Indian Pulp and Paper Technical Association, Sichuan University of Media and Communications, Xinxiang, Henan, 611745, China .
Zaki , M. ( 2019 ), “ Digital transformation: harnessing digital technologies for the next generation of services ”, Journal of Services Marketing , Vol. 33 No. 4 , pp. 429 - 435 .
Zerbino , P. , Aloini , D. , Dulmin , R. and Mininno , V. ( 2018 ), “ Big data-enabled customer relationship management: a holistic approach ”, Information Processing & Management , Vol. 54 No. 5 , pp. 818 - 846 .
Zupic , I. and Čater , T. ( 2015 ), “ Bibliometric methods in management and organization ”, Organizational Research Methods , Vol. 18 No. 3 , pp. 429 - 472 , doi: 10.1177/1094428114562629 .
Acknowledgements
This research is linked to the fund cod. VERB_SID19_01 of the University of Padua.
Corresponding author
About the authors.
Cristina Ledro is a PhD student in Management Engineering and Real Estate Economics at the Department of Management and Engineering of the University of Padova, Italy. She holds a master’s degree in Management Engineering from the University of Padova. Her research focus is within the area of customer relationship management. Her research interests include customer experience, value creation, performance measurement and digital technologies.
Anna Nosella , PhD, is Full Professor of Business Strategy at the Department of Management and Engineering of the University of Padova. Her research interest focusses on innovation management, dynamic capabilities and strategies. Her papers have been published in Technovation , Long Range Planning , Management Decision , Journal of Engineering and Technology Management , Journal of Business Research , International Journal of Human Resource Management , Strategic Organization .
Andrea Vinelli , PhD, is a Professor of Operations and Supply Chain Management and Service Operations Management at the Department of Management and Engineering at the University of Padova, Italy. Director of the MBA Programme at the CUOA Business School, Italy. His research and consulting interests lie in the areas of operations strategies, supply networks, servitisation and digital operating models, with specific expertise in the fashion industry and sustainability.
Related articles
We’re listening — tell us what you think, something didn’t work….
Report bugs here
All feedback is valuable
Please share your general feedback
Join us on our journey
Platform update page.
Visit emeraldpublishing.com/platformupdate to discover the latest news and updates
Questions & More Information
Answers to the most commonly asked questions here
Academia.edu no longer supports Internet Explorer.
To browse Academia.edu and the wider internet faster and more securely, please take a few seconds to upgrade your browser .
Enter the email address you signed up with and we'll email you a reset link.
- We're Hiring!
- Help Center
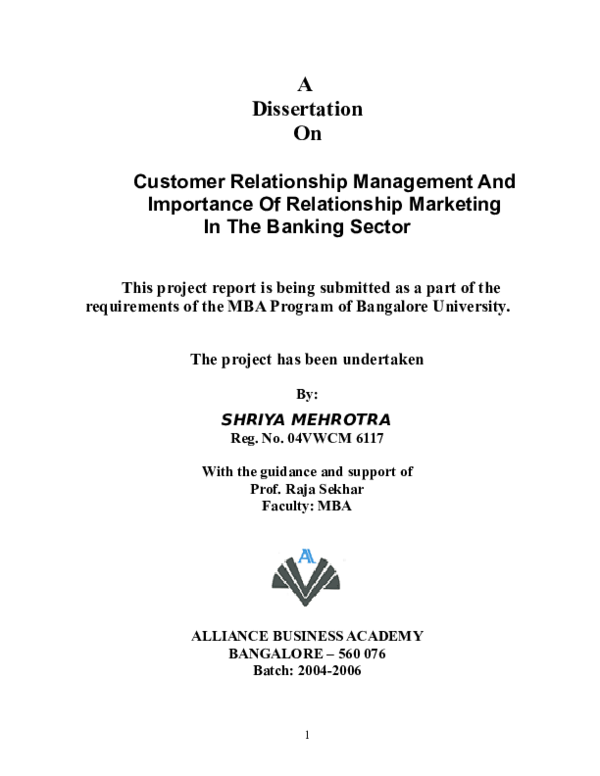
A Dissertation On Customer Relationship Management And Importance Of Relationship Marketing In The Banking Sector

Related Papers
anfas hanan
Customer Relationship Management concept is tendency of banking sector to establish and maintain long-term relationships with customers in order to provide value for customers and banks. This concept allows bank to identify, segment, communicate and build long-term relationships with customers on individual basis. In today's business environment, banks have aim to identify customers and to adjust offer to meet customer`s needs, in order to maximize profits. Using modern technologies, Customer Relationship Management is becoming a method to maintain existing structure and development of high quality customer base. It involves development of marketing strategy through a better understanding of the entire customer base, understanding needs and attitudes of customers, as well as more efficient consideration of profitability and added value that each customer have for the bank. The aim of research, presented in this paper, is to assess to benefits of introducing Customer Relationship Management concept in banking sector, by defining strategies, adjustment of organizational structure, culture and internal processes with help of modern technology. The paper presents methods of measuring success of Customer Relationship Management concept and problems which banks have when implementing a new business philosophy.
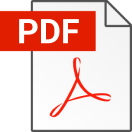
Dr. Asif Perwej
Namadhila Shapaka
International Journal of Marketing Studies
Dadson Awunyo-Vitor
Umut Kaplan
JMRA Publication
Banks play a vital role in the socioeconomic development of our country. Banks offer several facilities to enhance the standard of living of our citizens. Today customer is the king of the market. To achieve the competitive advantage, Indian banks have to focus on the customer. In modern era, each and every bank is realizing the importance of relationship with customer to survive in the competitive world. CRM is focus on creating, satisfy and retaining customer through uncompromising services. In India there is large number of public sector banks but there market position is not so good as compared to private sectors. Proper CRM strategy can be used by these banks to survive effectively in this competitive world. Life long relationship with customers is the key to success for any bank. Customer Relationship Management is very important for the growth and profitability of banks in the present age. So, in this paper I have discussed the Role of Customer Relationship Management (CRM) in the Indian banking Sector.
service per mobil purwokerto
Service Per Mobil Purwokerto
Service PER Mobil Purwokerto Berpengalaman. Bengkel Kaki Kaki Mobil Arum Sari Purwokerto siap membantu anda dalam mengatasi masalah pada kaki-kaki atau Shockbreaker mobil & menghilangkan bunyi – bunyi Bising pada kendaraan roda empat anda. Banyak dari kita yang tidak sadar akan keselamatan ketika kita mengendarai kendaraan kita. Hanya karena satu masalah Kaki kaki atau shockbreaker saja keselamatan kita jadi terancam. Salah satunya ketika mobil anda mengalami kendala yang menurut mereka biasa saja, justru itu dapat mengancam keselamatan kita sendiri apa lagi ketika kita sedang dalam perjalanan.
Hyperfine Interactions
Mohammed Saad
Paediatrics and Child Health
Lauri Van der poel
Medical care
Tabitha Kung
RELATED PAPERS
Twenty-Fourth AAAI Conference on Artificial …
Ankit Sharma
International Journal of Pregnancy & Child Birth
Haley Milot
Espiral Estudios sobre Estado y sociedad
Zeyda Rodríguez
IFIP Advances in Information and Communication Technology
Miguel Miranda
andika dwiputra
Journal of Tropical Upland Resources (J. Trop. Upland Res.)
Dwiyana Habsary
Open Access Emergency Medicine
Rusmin Syukur
Engineering Mechanics Institute 2019 Conference, Book of Abstracts
Michele Zecchini
Archives of Disease in Childhood
Tsvetomir Loukanov
Soraida Cándida Acosta Brooks
PS: Political Science & Politics
Suzanne Scoggins
Sustainability
Salem Alkhalaf
Nursing Ethics
İbrahim Başağaoğlu
Revista Portuguesa de Cardiologia
Sofia Cabral
The Lindenwood Review: a journal of literary prose
Ofelia Montelongo
Proceedings of the 12th international conference on Supercomputing - ICS '98
Richard Brent
Molecular Pharmaceutics
Ram Sundar Mahato
Economy of eastern Croatia – yesterday, today, tommorow
Mirta Šodić
Archaeological and Anthropological Sciences
Thomas Sutikna
LUTS: Lower Urinary Tract Symptoms
Hussein Nasef
Energy and Buildings
Jelena Srebric
- We're Hiring!
- Help Center
- Find new research papers in:
- Health Sciences
- Earth Sciences
- Cognitive Science
- Mathematics
- Computer Science
- Academia ©2024
The CRM behaviour theory – Managing corporate customer relationships in service industries
- Original Article
- Published: 14 December 2010
- Volume 17 , pages 155–173, ( 2010 )
Cite this article
- Michael Labus 1 &
- Merlin Stone
29k Accesses
10 Citations
Explore all metrics
This article illustrates a Grounded Theory-based approach towards the discovery of the CRM Behaviour Theory. The CRM Behaviour Theory represents seven inter-related perspectives of Customer Relationship Management (CRM) relating to managing corporate customer relationships in service industries such as telecommunications. To gain a fresh perspective on CRM, an amended Glaserian Grounded Theory-based research methodology is proposed. This involved 52 personal interviews with service providers and their corporate clients. The results suggested taking a holistic view on CRM holons (a holon is defined as a system that is a whole system itself as well as being part of other systems) comprising seven interrelated pattern-like views. Leadership and an integrated approach are found to be critical, but not software. Software-centred approaches fail to deliver long-term results because of their exclusion of ‘soft’ issues, in particular organisational culture.
Similar content being viewed by others
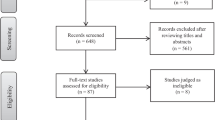
Reactions towards organizational change: a systematic literature review
Khai Wah Khaw, Alhamzah Alnoor, … Nadia A. Atshan
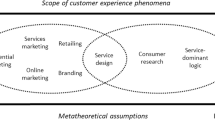
Customer experience: fundamental premises and implications for research
Larissa Becker & Elina Jaakkola
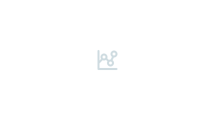
Change Management: From Theory to Practice
Jeffrey Phillips & James D. Klein
Avoid common mistakes on your manuscript.
INTRODUCTION
Much research has been conducted on Customer Relationship Management (CRM) since its academic emergence in 1997. 1 After more than a decade, CRM for corporate clients in service industries such as telecommunications has been neglected in both academic and professional domains – often regarded as ‘quick fix’ technology projects. 2 Many CRM approaches focus on mass markets and on related CRM initiatives such as retention and churn management.
CRM academics’ theoretical writings are often unrelated to business realities, consisting of overly abstract CRM models (Figure 3). CRM practitioners writing about the topic often address the success rate of technology-focused CRM software projects. 3 However, CRM for corporate clients is very complex, in particular in service industries such as telecommunications. A tentative complexity analysis carried out during this research project uncovered more than 1400 CRM ‘hard’ activities for marketing, sales, customer service and billing. However, CRM for corporate clients is not just related to ‘hard’ factors. ‘Soft’ issues prevail in the business-to-business (B2B) world, where clients are known personally by supplier staff. CRM studies (Figure 3) and CRM market data 4 propose a narrow view focusing just on CRM strategy, processes and systems. However, bearing in mind the actual complexity of CRM for corporate clients, perhaps Einstein 5 described the best approach: ‘Everything should be made as simple as possible, but not simpler’.
Notwithstanding the progress in CRM project success rates 6 and wider academic propositions, 7 , 8 , 9 CRM for corporate clients has never been fully explored, 10 embracing both ‘hard’ and ‘soft’ factors. 11 This is the role of this article.
Evolution of research questions
Our research questions (RQs) evolved over time as an iterative, non-linear process (2002, 2004, 2007) in order to answer ‘what you specifically want to understand by doing your study’. 12 In conclusion, we were trying to answer the major research question:
‘What are the evolved characteristics and behaviours of the holistic management of corporate customer relationships for telecoms carriers?’
This involved answering these further research questions:
RQ1: How are corporate customer relationships managed in the telecoms industry and what CRM models evolve?
RQ2: How do corporate clients behave and how are their requirements aligned with CRM models?
RQ3: What are the characteristics of performance management (PM) in business customers’ CRM?
RQ4: What are the inter-model, overall relationships of evolved holistic CRM model dimensions?
Interviewees’ understanding of CRM
As an introduction to the ‘real world’(pp. 116), Figure 1 presents an example of CRM for corporate clients in service industries, based on a 12-hour diary of a typical CRM ‘working day’ – extracted from the analysis of interview transcripts carried out by our research study. This diary reminds us of CRM complexity in theory and practice. Interviewees identify the gaps between claim and reality.
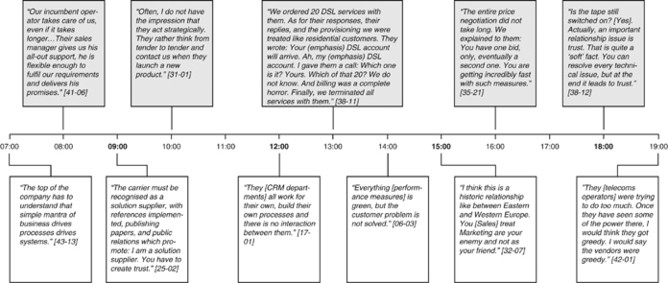
Diary of CRM claims and realities in service industries.
At the beginning of interviews, interview participants were asked to provide a short definition of their understanding about CRM. The objective was to ensure a mutual understanding of the terms used during the course of conduction of the interviews. Definitions varied between interviewees, depending on their expertise domain or personal background.
Here are some of the definitions of CRM reported by interview participants:
‘CRM is a philosophy and a strategy conception. And leaders shape the design of the relationship with our clients’. Director of quality assurance of a telecoms operator
‘For most sales people CRM is a piece of software deterring them from doing their job’. Key account manager for a fixed-network operator
‘CRM to me is: I am using CRM as a single system which provides you with a complete view of the customer’. Senior director for applications development of a metropolitan service provider
THE MISINTERPRETATION OF CRM IN THE LITERATURE
As indicated in the introduction section, significant research has been conducted on CRM since its academic emergence in 1997. However, the entirety of complex CRM has still not been properly explored. Instead, focused frameworks, for example, on CRM processes and technology, prevail, 13 , 14 categorising CRM as technology-centric. Even though scholars point out the neglect of causal interrelatedness between CRM model dimensions, 15 they still ‘follow’ the same theoretical path as models criticised. The end result is explicit similarities of CRM frameworks propagating fundamental modelling errors ( Figure 2 ). Shifting the perspectives towards academic analyses of professional CRM project failure, CRM scholars seem to reflect and learn very little from the identified causes of CRM failure – for example, the neglect of organisational change. On the other hand, many CRM managers ‘assume, that more CRM technology is better’. 16 By contrast, some scholars 17 describe CRM as a customer-centric business philosophy. What is more, Salomann et al 18 point out that 48 per cent measure (hardly or not at all) CRM process performance and that corporate customers are neglected.
Contextual analysis of inward-centred CRM worldviews.
CRM in service industries
To better understand CRM for corporate clients, we need to identify the main differences between CRM for corporate clients and for consumers – the complexity of CRM for corporate clients. This is confirmed by Webster 19 who argues that marketing for corporate clients, as one element of CRM, can be characterised by the complexity of products and purchase processes. Coviello and Brodie 20 recognise that ‘marketing appears to be relational in B2B [business-to-business] firms and more transactional in consumer firms’ although there are key similarities, such as the approach to market planning. Gummesson 21 adds that B2B CRM consists of ongoing business, whereas CRM for consumers focuses on everyday transactions. There is no active management of service-level agreements for consumers. Understanding corporate clients’ CRM demands an understanding of the organisational Theory of the Firm, 22 as ‘an interplay of technology, social structure, culture, and physical structure’. 23 A better elaboration of dynamic perspectives of CRM is needed to improve understanding of CRM causalities, that is, Systems Theory 24 and Systems Thinking. 25 CRM in service industries requires an understanding of principal service characteristics, which are virtually always neglected or ignored in the CRM literature. We also need to consider whether, from the point of view of CRM analysis, management of services differs from manufacturing management. A tentative answer is given by Normann 26 who claims: ‘Yes and no’. Services can be evaluated and offered like nominal economic goods. 27 Thus, both services and manufacturing goods can be classified as products. 28 Second, Sasser et al 29 recognise that services cannot be stocked, Levitt 30 explores that service ‘production’ takes place in the field, whereas Normann emphasises that the customer is ‘a participant in the production of the service … [and ] … it is also necessary to ‘manage’ them as part of the production’.
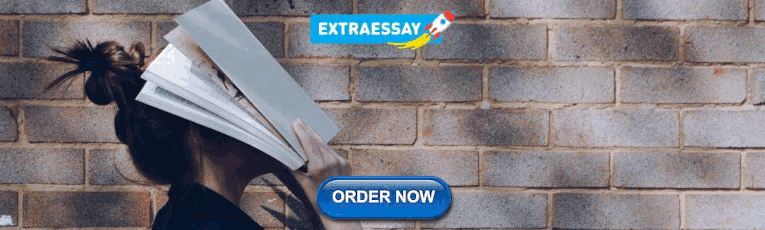
CRM – Not just software
In the past, CRM was often seen as a ‘quick fix’ IT project proposal implemented by consultancies. According to Bergeron, CRM ‘was born around 1997’. Mack et al 31 claim that CRM evolved from total quality management in the 1980s. Schmitt 32 identifies the origin in the customer orientation movement in 1990s, whereas Newell 33 recognises the strategic and technological focus of CRM. Auer 34 states that US software vendors took up Relationship Marketing (RM) to market CRM systems. Bruhn refers to the continued usage of Relationship Marketing terminology, whereas Payne and Frow 15 claim that CRM has ‘its roots in RM’ (p. 85). Later, Payne addresses the significance of change management in achieving positive CRM outcomes. Therefore, CRM thinking has evolved over the last decade, but there are differences of opinion as to how.
Our analysis of CRM literature revealed the emergence of three de-coupled ‘C-R-M Schools of Thought’ corresponding with the evolution of CRM. The separation of C-R-M is based on two analytical reflections: First, an ethnographic analysis 35 of CRM: A ‘customer’ has a ‘relationship’ that is ‘managed’. Per se , this implies that clients – not ‘suppliants’, a terminology used by an interviewee of a corporate customer – are the starting point of CRM, rather than ‘ R ’ or the even prevailing end point ‘ M ’. Moreover, it implies that a customer has a relationship (‘ R ’) with personally known supplier staff – relationships with software would raise a rather different set of issues. Finally, it implies managers ‘ M ’ caring about the relationship (‘ R ’) with their customers (‘ C ’) rather than the opposite.
Second, the separation of C-R-M is additionally based on the allocation of scrutinised C-R-M models to global cultural traits 36 clusters by applying the analysis principles of Grounded Theory (GT).
The iterative end result of this comprehensive analysis is: There is no unifying CRM modelling embracing the unifying CRM holism, but three distinct, geographically allocatable, partly overlapping, dis-unified CRM ideologies representing characteristic thinking about CRM as follows:
Managerial C-R-M School of Thought (relationships are monitored)
Process, IT and Implementation C-R-M School of Thought (relationships are technology-centred)
Markets and Stakeholder C-R-M School of Thought (relationships are stakeholder-centred)
A typical example of this absence of a unified CRM approach is the Strategic Framework for CRM and the CRM Value Chain, 37 from the markets and stakeholder-centred C-R-M School of Thought: Both these models focus on markets and stakeholder, but propose different CRM viewpoints. There is some overlap with other ‘C-R-M Schools of Thought’, for example, Buttle's CRM Value Chain addresses implementation aspects as well. However, in our view a comprehensive description of CRM as a whole is not proposed by one particular School of Thought. In our view, contemporary C-R-M science is therefore weak, despite its volume. Ngai 38 identified 105 CRM articles published in 2002. Parkinson's 39 view that ‘the progress of science varies inversely with the number of journals published’ underlies Figure 3 . By elaborating the nine C-R-M models, we can see that progress corresponds with dates of C-R-M publications. We believe that we need a fundamentally different research methodology, avoiding existing C-R-M propositions in order to avoid being ‘contaminated’.
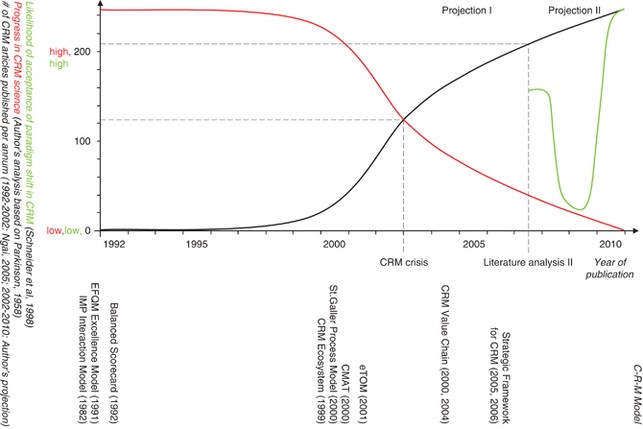
Diminishing progress of C-R-M science and likelihood of paradigm shift.
GROUNDED RESEARCH METHODOLOGY – ENABLING A FRESH PERSPECTIVE ON CRM
Research methodology.
The requirements for a (CRM) publication are that the contribution to knowledge is original, relevant, communicable and trustworthy. Particular trustworthiness aspects include the credibility, controllability, validity and reliability of the contribution to knowledge. 40 To address the unexplored holism of CRM, a qualitative research methodology 41 emerged. A pre-condition for the understanding of this study is the tension from applying and advancing GT) principles for CRM in the telecoms industry – the fundamental paradigm controversy between GT founders Glaser and Strauss and Corbin. 42 This resulted in the requirement for an amended GT research methodology that can be positioned 43 , 44 in the constructivism paradigm by drawing a line to postpositivism epistemology. 45 The meaning to our CRM research is: Findings are expected to being objective, derived from actual, subjective CRM experience incorporating human values. On the basis of this, a GT methodology was designed incorporating two major principles: 46 , 47
Existing literature theories are not reviewed, but theories are discovered.
Theoretical elements are developed through coding procedures based on constant comparison of instances of data.
However, the paradigm controversy of Strauss and Corbin's evolved GT is positioned towards relativism (that is, multiple CRM realities exist) versus Glaser's ‘real’ realities, and virtually disregarded any discussion about vital GT modelling gaps and about a significant dilemma with regard to this study on CRM research. This is for two reasons:
The Glaserian position does not (intentionally) include a modelling ontology required for this research, but very useful coding families such as the ‘bread and butter’ 48 coding family ‘Six Cs’ (causes, context, contingencies, consequences, covariances conditions).
Strauss's position includes modelling propositions, but these are not useful for this CRM research confirming Urquhart's 49 GT experience of having ‘broken apart (from) Strauss’ and Corbin's paradigm’.
These modelling gaps were addressed by iterative, normative GT research methodology amendments and included patterns, 50 holons 51 and abstract modelling. 52 A pattern approach enabled the authors to identify CRM problems and solutions, that is, to answer the RQs (Introduction section). Constructing holons equipped us to explore dedicated CRM viewpoints and inter-linkages between them, that is, particularly to address RQ4 (What are the inter-model, overall relationships of evolved holistic CRM model dimensions?). Moreover, meta-modelling amendments provided an unambiguous classification of categories and properties representing abstract entities of CRM experiences, for example, ‘inconsistent design of CRM actions’ as stated by corporate client: ‘We were treated like residential customers … and billing as a complete horror’. The end result is the assembly of a grounded CRM behaviour theory as presented in Figure 4 .
Assembly of the CRM behaviour theory development.
RESEARCH METHOD
Our grounded research method comprises three major components, adapted from Miles and Huberman 41 :
data collection including fieldwork preparation, that is, sampling method and broad fieldwork questions;
data reduction buttressed on amended GT principles; and
data display, that is, theory writing succeeded by the verification of findings.
Data collection
‘Lightly’ semi-structured interviews were the best way of discovering a CRM theory. These semi-structured interviews contained several broad, open fieldwork questions. Wengraf 53 suggests that by applying this type of interview the (CRM) theory is likely to be discovered without reinventing the wheel or closing off the conceptual innovation. The unit of analysis was expected to be the marketing, sales, customer service and billing departments of service providers in mature telecoms markets in Europe, America and Asia/Pacific. In corporate clients, Information Technology (IT) departments of various industries, for example banking or publishing, typically purchasing Next Generation Services (NGS) such as broadband connections from telecoms best fitted the qualitative sampling. Fifty-two semi-structured depth interviews were conducted by a single interviewer from different organisational perspectives, of which 70 per cent were face-to-face interviews. The total elapsed time for data collection and analysis was 18 months, involving 46 600 miles (75 000 km) of global travel. The distribution is presented in Figure 5 .
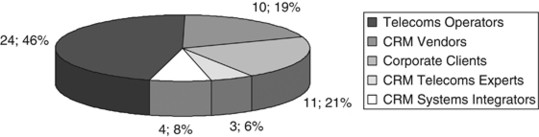
Distribution of interviewees’ organisations.
Data reduction
Collecting large amounts of interview data (a total of 596 pages of interview transcripts) requires much data management, recording and use of qualitative analysis software. This included preservation of raw data. NVivo and Decision Explorer software packages were used together with text processing for detailed code descriptions. Our grounded data analysis implied a set of coding procedures to analyse the meaning of interviewees’ experience, for example, memos, open and selective coding, as well as theoretical coding. Miles and Huberman define coding as the analysis of transcripts: not the words themselves but their meaning, 41 that is, we derived meaning from interviewees’ statements rather than just rephrasing them.
We needed reliability and validity in data for the research to be valid. 54 To address the question ‘Can one trust the results?’, we scrutinised and derived three meaningful criteria, as well as a consideration about the generalisability, as follows:
Internal postpositivism reliability (IPPR): 86 per cent intra-checker reliability. IPPR implied that interviewees were shown the results, and asked to what extent their CRM experience was reflected – rather than the widely used but entirely misjudging ‘do your peer student a favour’ feedback approach.
Constructionism (derive meaning from subjective interviews) transparency: A thorough documentation of executing GT coding procedures, including 596 pages of written interview transcripts.
Glaserian trust-building criteria: A rigorous layman's judgement of 4 months. This process involved four peer researchers and three CRM professional experts who judged the seeing and trusting the ‘big picture’ of the CRM findings.
Generalisability of results: Glaserian 55 criterion of theory ‘modifiability over time’ by applying the findings in other and wider contextual environments.
As a reflective epilogue on ‘doing Grounded Theory’, we refer to Einstein 56 who recognises: ‘There is no logical path to these laws. Only intuition, resting on sympathetic understanding of experience [which is helped by a feeling for the order lying behind the appearance], can reach them’.
FINDINGS – THE DISCOVERY OF THE CRM BEHAVIOUR THEORY
Structural approach to findings’ presentment.
On the basis of the methodology approach, qualitative data were gathered from both telecoms operators and their corporate clients to answer the RQs. We were trying to answer the major RQ:
This involved answering these further RQs:
RQ3: What are the characteristics of PM in business customers’ CRM?
Bearing in mind the requirement for a communicable and systematic findings’ structure, as well as non-hierarchical, multiple model perspectives, Figure 6 presents our approach and illustrates the sequence and linkages with the RQs:
Logical macro-structure of fieldwork findings.
Key findings overview
The purpose of this paragraph is to present key issues and propositions that emerged from the grounded data analysis, and to present a visual overview of fieldwork findings.
Interview participants stated that isolation in CRM, and particularly the complexity of CRM for corporate customers in the telecoms industry, requires a multi-perspective model. Five major CRM issues were revealed:
CRM managers have no common approach to CRM and lack a coherent and long-term direction in CRM.
CRM departments think and work in isolation and in parallel.
CRM processes and systems are designed in isolation and inconsistently managed.
Corporate customers are dissatisfied with inconsistent treatment and do not trust telecoms operators.
PM in CRM is network-centric focused and neglects corporate clients’ perceptions.
These central issues limit the ability of telecoms operators to manage corporate customer relationships. Five central propositions emerged to address the issues:
Leaders are willing to serve the CRM organisation and have genuine motives.
Create a motivating CRM culture with open communication and mutual trust.
Telecoms operators deliver a preferred customer experience to their corporate clients and sell services honestly and personally.
Corporate clients choose trusted, reliable and fast telecoms operators for their NGS solutions.
Outside-in PM addressed customer-centric ‘soft’ and ‘hard’ measures and inter-departmental key performance indicators (KPIs).
On the basis of the findings, detailed causal maps were designed that are presented throughout this part of the thesis. The conceptual CRM theory model overview is presented as follows:
Key findings statement
On the basis of the presentation of issues and propositions and the conceptual overview shown in Figure 7 , the key finding of this research is:
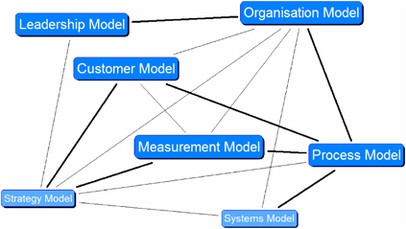
Conceptual overview for CRM in the telecoms industry.
‘A holistic CRM behaviour theory for telecoms operators and their relationships with corporate clients consisting of seven causally interrelated, problem-solution founded, non-hierarchical CRM perspectives based holons’.
SEVEN HOLISTIC CRM MODEL PERSPECTIVES
The seven models illustrate a systematically structured, non-hierarchical CRM approach for corporate clients in the telecoms industry and are as follows:
CRM Leadership model
This model deals with the behaviour of CRM managers in the telecoms industry. It is a central model consisting of 44 concepts (that is, properties and categories). The CRM leadership view addresses the interplay of self-centric progress (that is, personal objectives diametrical to CRM objectives), diversified CRM approaches and operational CRM problem solving with genuine, customer-centric motives and relevant leadership tasks and roles in CRM. CRM managers are at the centre of change activities and their different behaviours are analysed. Figure 7 briefly shows that mutual linkages with the organisation and strategy models were revealed at various management levels.
CRM Strategy model
This model addresses the long-term planning of CRM actions and different types of strategy behaviour. The strategy perspective plays a vital role in the overall CRM model, although it consists of 21 categories and properties, only. This viewpoint discusses the causal behaviour of non-aligned CRM programmes, CRM project failure, as well as understanding corporate clients’ requirements and personal sales of NGS. The improvement of CRM initiatives forms the analytical centre of the model. The model is mutually related to the customer and measurement views in particular.
CRM Organisation model
The organisation model discusses people-related behaviour in sales, marketing customer services and billing departments of telecoms operators. It is a central model, and consists of 44 concepts. The model deals with isolated thinking and working in CRM and the concepts required to address such issues. The conceptual focus is on structural changes towards interwoven customer-centric teams operating in trusted atmospheres. As can be seen in Figure 7 , the model is closely linked with CRM processes, the customer perspective and the leadership model in various organisational CRM units.
CRM Process model
The process model deals with the execution of CRM programmes via operational activities in sales, marketing, customer service and billing. It is a central model consisting of 26 concepts, and reflects issues of unsatisfactory corporate customer experience and missing customer information handshakes. The design of an outside-in CRM process approach is at the model centre. The process model view is closely linked with organisation, systems, measurement and customer models.
CRM Systems model
The systems model addresses CRM software application-related behaviour in the telecoms industry. The model consists of 34 categories and properties and plays a vital role in automating CRM processes. It deals with monolithic and isolated CRM installations and their causal relationships with CRM systems architecture, dynamic CRM applications and embedded PM capabilities. The systems model is closely linked with the process model, in particular.
CRM Customer model
The customer model addresses the outside-in perspective of corporate customers about CRM. It is a central model linking external with internal CRM perspectives, and it consists of 34 categories and properties. It deals with inconsistent, standardised treatment of clients and missing long-term CRM commitment. The conceptual centre is formed by designing preferred methods of CRM interaction and NGS provider independence. There is a very close linkage with the strategy model as illustrated in Figure 7 .
CRM Measurement model
This model deals with CRM performance management in the telecoms industry. Comprising 38 concepts, it is a central model and defines a bracket of internal and external model perspectives. It deals with the causal behaviour of isolated CRM measurement of both telecoms operators and their corporate clients and the lack of customer-relevant indicators in relation to conceptual elements. These concepts include a holistically connected framework from the outside-in. As presented in Figure 7 , the model is closely linked with strategy and processes.
On the basis of the presentation of key findings, the next methodological step is the grounded allocation of actually relevant literature.
Locating the CRM Behaviour Theory to Relevant C-R-M Literature
This paragraph addresses the discussion of findings in the context of relevant literature. A set of five key differences with our findings was revealed:
Isolation, the key problem of all seven CRM perspectives is often neglected in CRM literature, 57 although there was evidence in the fieldwork that separate execution of CRM activities has inter-model affects.
CRM culture and related ‘people business’ aspects of CRM for corporate clients emerged as the central CRM discussion perspective related to addressing isolation issues. However, the fieldwork findings demonstrated little similarities with the CRM literature, where a customer-centric motivating CRM culture was concerned.
PM requirements for CRM revealed by this fieldwork such as ‘measuring the right things’ and PM as a measurement theme in itself are disregarded in the CRM literature. 58 A focus on ‘hard’ or financial measures for processes and internally directed benchmarks prevails.
The dynamic behavioural aspects discovered in our fieldwork are almost excluded in the literature focusing on conceptual 59 or static views, neglecting causal relationships.
An integrative perspective on PM in CRM (CRMQUAL) incorporating the measurement of CRM culture, the conceptual separation of processes and systems measures, as well as non-hierarchical (that is, non-successive, unranked) feedback loops is not covered by the literature – in particular Kaplan and Norton's strategy maps.
Some parts of the CRM literature showed four key similarities, but relevant literature actually varies and does not show a single key criterion of similarity:
The literature partly recognises the ‘cross-functional nature’ of relationships. This has been confirmed by our fieldwork, which shows that a functional perspective (that is, Marketing, Sales, Customer Service, as well as Billing) would destroy behavioural understanding rather than support it. In fact, a functional perspective (that is, Marketing, Sales, Customer Service and Billing) was dissolved and concepts transformed into seven dedicated CRM views.
Payne and Frow 15 indicate that isolation is a CRM issue, although no systematic approach is proposed as we suggest. Lovelock 60 proposes a service quality gap model. The approach offers a partly similar problem awareness related to CRM, but falls short concerning some ‘soft’ issues such as motivation, and particularly managerial willingness to support CRM.
CRM culture is often addressed in the managerial School of Thought. This means that there is a contradiction in the CRM literature. However, Homburg and Sieben 61 propose a customer-centric culture model that confirms the relevance of CRM culture and its impact on processes as well as the interaction with corporate clients.
PM in CRM revealed novel similarities. IMP Group suggests measures related to corporate clients’ requirements such as trust, reliability and flexibility, while Woodcock's proposition for a collective PM approach in CRM indicated that a lack of customer behaviour measures means valuable PM insights are disregarded.
The seven CRM perspectives were mapped against the literature, revealing fresh perspectives on CRM, as illustrated in Figure 8 .
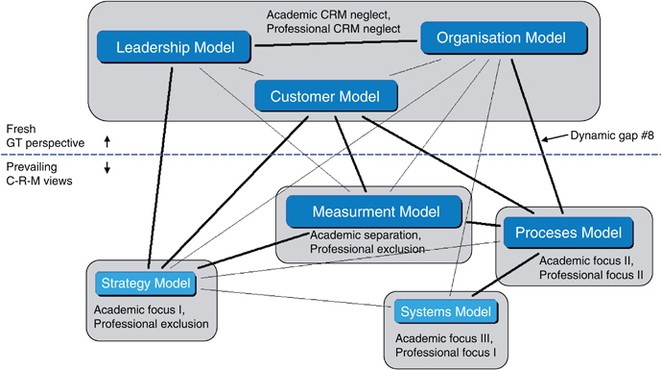
Emerged differences and similarities of findings with the C-R-M literature.
CONCLUSIONS AND IMPLICATIONS FOR ACADEMIC AND PROFESSIONAL CRM EXPERTS
Analytical reflective conclusions from interviewees’ perspectives.
There is significant support for modelling actual CRM experience and episodes (fourth interviewee, 2007). The relevance of an outside-in perspective of corporate customers is confirmed: ‘I discussed your model with my colleagues. Our [corporate customer] experience is entirely reflected … the results are clearly understandable and logically structured’ (thirty-fifth Interviewee, 2007). This confirms Rigby et al , who suggest incorporating customer requirements in order to learn from previous CRM experiences. The seven dedicated CRM perspectives and their inter-linkages are confirmed. The fifteenth interviewee (2007) investigated the CRM strategy model and suggested that ‘Marketing is not needed in B2B, but a “fine” NGS solution image’. This indicates the balanced functional viewpoint of the CRM Behaviour Theory rather than focus just on marketing. The comprehensiveness of the theory was understood by CRM professionals (for example, fourteenth interviewee, 2006, 2007). This argument is supported by the result of an intra-checker reliability analysis of 86 per cent.
However, the CRM Behaviour Theory is criticised by interviewees and peer scholars who provided their rigorous feedback. Criticism concerns:
‘Heavyweight’ reading requires comprehensive attention.
Complexity is criticised by less experienced academic researchers.
It is said to be hard to understand quickly Grounded Influence Diagrams, owing to the large number of figures and proxies provided (thirty-fifth interviewee, 2007), although the thirty-fifth interviewee (ibid.) adds: ‘Once you are really acquainted with the subject, everything is understandable’. Moreover, the fourteenth interviewee (2007) adds that ‘it required 20 hours to read and to feedback on the organisation and measurement models’. Perhaps this means that both vast CRM experience and comprehensive academic analytic capabilities may be needed to understand the CRM Behaviour Theory. However, a limiting software issue in Decision Explorer is closely related: The numbering of visual models, in particular proxies that cannot be modelled as one but consecutively numbered representations, only. This limits the efficiency of reading Grounded Influence Diagrams, which was confirmed by interviewees reflecting on the findings.
With regard to the second major issue, findings are criticised by academic scholars who are inexperienced in modelling. In our view, this reflects the comprehensiveness of long-term CRM transformation projects. Conclusions revolve around the confirmation of results, as well as the cognition that a differentiated research methodology surfaces actual CRM issues and solutions. As a logical next step, conclusions on ‘doing’ GT are explored.
Conclusive suitability of the amended GT research methodology
The purpose of this paragraph is to evaluate the suitability of the amended GT research methodology approach to addressing the RQs. GT certainly facilitated a ‘fresh’ perspective on CRM ( Figure 8 ) and the exploration of characteristics and behaviour of CRM from multiple viewpoints. However, the author was still contaminated, that is, influenced, by misleading, irrelevant C-R-M literature at the beginning (14 February 2005) of the evolution of tentative broad fieldwork questions. As discussed in the methodology section, a set of three normative amendments was developed to address existing GT modelling gaps. GT coding principles enabled the discovery of 242 CRM concepts grounded in 52 semi-structured interviews. Nevertheless, the author recognises that constructionism research, which is based on subjective experience, is not free of bias, 42 and interpreting beliefs had to be suspended in order to bring to the surface theoretical CRM concepts. The evaluation confirms the suitability of GT to explore CRM for corporate clients in the telecoms industry and to enable a paradigm shift in CRM.
We experienced significant resistance while uncovering the CRM Behaviour Theory. GT research seems to uncover basic methodological issues, for example, in cognitive mapping. We learnt one important lesson: Perhaps Einstein 56 best describes the methodological meaning of this GT methodology: ‘We cannot solve our problems with the same thinking we used when we created them’.
Conclusions on explicit and implicit contribution to knowledge: An instance of a general relationship behaviour theory?
The purpose of this paragraph is to draw conclusions on the contribution to knowledge from explicit and implicit viewpoints. Explicit perspectives are characterised by four evaluation criteria: originality, credibility, communicability and relevance. 62 This is constituted in the fundamental questions of ‘what’, ‘how’ and ‘why’. 63 By contrast, implicit viewpoints emerged from cognitive holistic reflection and peer discourses. To fulfil these criteria, we conducted an almost ‘endoscopic’ analysis of 6-month end-to-end results’ testing and reflection. One particular criterion, originality, stands out: we are very much aware that the theory discovered is not based on entirely new concepts, but an analytic result of grounded data collection and data analysis based on an amended GT methodology. Hence, as for the generalisability of results, the fieldwork was only in the telecoms industry and the model as a whole was developed in this context and not evaluated or used in other industries. Any indications about the generalisability of the outcomes are not grounded in an empirical process. However, CRM is not a specific discipline developed for the telecoms industry, but marketing, sales, customer service and billing exist in almost every industrial context.
IMPLICATIONS
Implications for professional and academic crm stakeholders.
On the basis of the above conclusions, the next logical step is to explore applicable implications for a set of five relevant stakeholders of our research: CRM researchers, CRM lecturers and corporate customers, CRM employees as well as CRM leaders so as to ground our research in the professional domain. However, considering the research problem that uncovered implicit, hidden attitudes in CRM, a holistic understanding of attitude-behaviour implications is needed. Referring to Kleinke, 64 an approach relevant to our research appears to be the application of ‘traditional attitude’ theories 65 , 66 enhanced by results changes 67 as follows:
Implications for CRM researchers: Internalise critical, independent grounded thinking beyond CRM
The purpose of this paragraph is to explore implications of this thesis for CRM researchers. The literature section unveiled academic CRM issues related to over-simplification and error propagation of theoretical CRM propositions. Perhaps, Einstein's 68 résumé best reflects upon this contemporary problem: ‘It is also vital to a valuable education that independent critical thinking be developed … a development that is greatly jeopardised’. The author witnessed eccentric hegemony and jealousy among scholars in the literature. Indeed, a good comparison of such behavioural issues to CRM leaders is stated by an interviewee as follows: ‘I am lucrative and you are empty-headed’. As a result, an attitude shift is needed as follows: ‘Be willing to develop critical, independent academic thinking in CRM’. This is based on the awareness of one's research paradigm and the refusal to uncritically accept CRM literature. The behavioural change implies the conduct of research independent from prominent academic institutions to address error propagation. The result of behavioural change is ‘rewarded’ with the discovery of innovative, integral CRM models and an in-depth understanding of the multidimensionality of CRM. One important side aspect is the philosophic cognition of research methodologies and understanding of grand scholars such as Einstein.
Implications for CRM lecturers: Integrate CRM issues for prospective professionals and stimulate intellectual activities
The purpose of this paragraph is to explore implications of this thesis for CRM lecturers. CRM lecturers often do not teach the ‘right things’ to prospective CRM professionals but refer to technology, abstract and misleading CRM case studies or ‘proven models’. This is further confirmed by discourses with three peers and 24 semesters of own experience as a research student. However, this seems to be a rather specific issue related to CRM as a discipline of applied sciences. Perhaps Einstein best describes the situation: ‘Numerous are the academic chairs, but rare are wise and noble teachers’. Hence, an attitude change is needed as follows: ‘Think beyond academic CRM disciplines and stimulate intellectual activities of students’. This is based on the awareness of the principal CRM modelling gaps such as sequential or hierarchical thinking. The implied change of behaviour focalises on teaching inter-disciplinary, grounded thinking and acting in CRM rather than another process automation lecture. Such lecturing is supported by the development of CRM examples from own experience rather than reprocessing abstract CRM case studies. The result is a stimulus of holistic intellectual activities of CRM students and the preparation of prospective CRM professionals for complex realities CRM ‘out there’. Perhaps the realistic outcome is recognised by Einstein: ‘Small is the number of people who see with their eyes and think with their minds’.
Implications for corporate customers: Improve purchasing conditions and become provider-independent
The purpose of this paragraph is to explore implications of the thesis for corporate clients and their relationships with telecoms operators. CRM issues identified, such as inconsistent treatment, are the ‘rule’ rather than exception in the telecoms industry. Corporate clients should not mistakenly assume that CRM issues are just related to their individual experiences with telecommunications operators. Therefore, as an implication, a change in attitude appears to be a logical, sound measure towards independence from suppliers, that is, engaging more than one telecoms operator and increasing competition among them. The implied change of attitude is: ‘Corporate clients are independent from telecoms operators’, that is, they can select NGS from a small pool of operators. However, attitude changes take effort and time, typically become very personal and require the willingness to feedback and learn. On the basis of such an attitude change, in particular CRM problem awareness, the behavioural change of negotiation conditions for the purchase of NGS solutions appear as follows: ‘You [operator] have one bid, only’. This is supported by systematically measuring ‘soft’ CRM aspects such as trust replacing ‘gut feeling’. The result is independence from suppliers, although a caring dialogue supports the long-term perspective of the relationship with telecoms operators.
Implications for CRM employees: Think honestly from outside-in corporate customer viewpoints and across CRM boundaries
The purpose of this paragraph is to explore implications of this thesis on CRM employees in the telecoms industry. Employee issues in CRM are related to thinking and working in isolated, artificial boundaries. Perhaps a good example is NGS sales behaviour, which has been described by a service delivery engineer of a mobile operator as ‘sell and forget’ accompanied by a ‘treat marketing as your enemy’ attitude. Therefore, a change in CRM employee attitude is the logical implication of the thesis that appears as follows: ‘Think honestly from outside-in, about corporate customers’ viewpoints and across CRM boundaries’. This is based on the trusting of other CRM employees in an open atmosphere. The behavioural change involves the adaptation and execution of CRM processes from an outside-in corporate customer experience to address, for example, the problem that the ‘left hand does not know what the right hand is doing’ conduct as expressed by the thirty-eighth interviewee in the fifth quotation [38–05]. Such a conduct is supported by designing measuring inter-departmental KPIs and the listening to and interacting with corporate clients. However, CRM employees should not imitate behavioural issues related to CRM leaders, but become ‘men of value’. 68 The result is the ability to operate in motivated, customer-centric teams and interwoven CRM processes and the linking of disconnected CRM and PM. One important side effect is the understanding of corporate clients.
Implications for CRM leaders: Be willing to serve the CRM organisation, understand corporate customers and manage complexity
The purpose of this final paragraph is to explore implications of this thesis for CRM leaders in the telecoms industry. A figurative sense of Einstein's cognition is required: the issue in CRM leadership is not intellect but ‘character’. Of 17 interviewees asked about CRM leadership 12 reported that a focus on self-centred personal progress of CRM managers was closely related to the lack of a common CRM approach among leaders. Such behaviour affects the entirety of CRM; to put it mildly, CRM problems start at the top and return to the top. Therefore, a change in CRM leadership attitude is the logical implication of the thesis. The implied change of attitude is: ‘Be willing to serve the CRM organisation and corporate clients’. This is based on a genuine desire to lead CRM, a thoughtful CRM leadership style and the trusting of CRM employees. Our findings support both bringing to the surface CRM leadership problems and solutions, as well as the awareness of the consequences. The behavioural change implies performing CRM leadership tasks such as understanding corporate customers and employee requirements rather than just solving operations issues. Talking to corporate clients and employees personally and openly reinforces the change, accompanied by measuring the ‘right things’ in CRM and a step-by-step change. The result is the ability to manage CRM complexity in the long run rather than just understanding CRM technology. Moreover, the behavioural change enables CRM leaders to understand ‘hard’ and ‘soft’ corporate customer CRM requirements.
Epilogue: A redefinition of CRM
The purpose of this paragraph is to critically rethink the actual meaning of CRM to the authors as it emerged after the fieldwork. A rigorous, retrospective comparison with the stereotypical software-centred working definition of CRM defined before the grounded fieldwork reveals that the understanding of CRM was nothing more than a contaminated, derivative re-processing of prevailing CRM definitions. Indeed, we were just rephrasing other scholar's thinking without understanding the meaning of CRM in the complex real world of business. The end result was a redefinition of CRM.
‘Customer Relationship Management (CRM) for corporate customers in the telecoms industry is a long-term customer- and total quality management-centred business philosophy to address the two core CRM issues of, firstly, self-centric leadership behaviour in CRM and, secondly, working and thinking in isolation in Marketing, Sales, Customer Service and Billing “silos”. CRM is based on seven causally interrelated, non-hierarchical perspectives that emerged from, and are grounded in, actual CRM experience rather than abstract metaphors of information technology-centricity. At the centre is the outside-in understanding and integration of “hard” and “soft” corporate customer CRM requirements. The transformational CRM nucleus is a personal attitude change of CRM leaders, to become willing to serve the CRM organisation and corporate customers, enabling a motivating CRM culture to “glue” prevailing isolation. CRM change is supported by a seven-dimensional, problem-solution-founded CRM dashboard to address existing fragmented and misleading measurement in CRM. The emerging CRM holism enables the understanding of the actual complexity of CRM for business customers and the effective management of CRM, as well as the recognition of behavioural CRM patterns in a “people business” environment’.
Further research – Significant research-related work to be addressed
The purpose of this paragraph is to explore other research subjects that emerged from data analysis and collective reflection. The most obvious research subjects are explored exemplarily, now.
First, applying and testing the CRM Behaviour Theory in other (service) industries appears the most obvious step. Perhaps the trust-centred financial service industry is a good candidate, particularly given the current issues with financial services. What is clear from our research is that researchers should directly interview corporate clients to grasp a multi-perspective understanding of CRM behaviour. Front and back-office employees should also be interviewed, in particular contact centre customer service representatives (CSRs). Scholars should approach CSRs independently from their managers in order to ensure an open interview atmosphere. Of course, CRM managers should also be interviewed. Perhaps the most relevant advice is: first, let interviewees ‘tell their stories’, and second let concepts emerge while coding interview transcripts.
Second, based on research topic number one, we intend to address the research topic ‘Discovery of the General Relationship Behaviour Theory (GRBT)’ by tentatively adapting our research into a general context, that is, other service industries, non-service industries and particularly society as an ‘ultimate general’ context.
Third, we propose to revisit this research in 2014. This particular date is based on the idea of the diminishing progress of science, according to which concepts change ‘in time and space’ after 7 years.
REFERENCES AND NOTES
Bergeron, B. (2002) Essentials of CRM: A Guide to Customer Service Relationship Management. New York: John Wiley & Sons, p. 2.
Google Scholar
Bruhn, M. (2001) Relationship Marketing: Das Management von Kundenbeziehungen [ Relationship Marketing: Management of Customer Relationships ]. Munich, Germany: Verlag Franz Vahlen, p. VI.
Gartner Group. (2003) Building Business Benefits from CRM. Stamford, CT: Gartner Group.
Mertz, S. (2008) CRM Software Market to Grow 14 Percent in 2008. Stamford, CT: Gartner Group.
Einstein, A. (1934) On the method of theoretical physics. Philosophy of Science 1: 32.
Article Google Scholar
Band, W. (2007) The Forrester Wave: Enterprise CRM Suites. Cambridge, MA: Forrester.
Buttle, F. (2000) The CRM Value Chain. Sydney: Macquarie University, also published in Marketing Business , 2001, February, pp. 52–55.
Payne, A. (2006) Handbook of CRM: Achieving Excellence through Customer Management. Burlington, MA: Butterworth-Heinemann.
EFQM. (1991) European Quality Management Forum. European Quality Management Forum. Paris: European Foundation for Quality Management.
IMP Group. (2002) An interaction approach. In: H. Hakansson (ed.) International Marketing and Purchasing of Industrial Goods. Chichester, UK: Wiley & Sons.
Glaser, B.G. (1998) Doing Grounded Theory: Issues and Discussions. Mill Valley, CA: Sociology Press, p. 31.
Maxwell, J.A. (2005) Qualitative Research Design: An Interactive Approach. Thousand Oaks, CA: Sage Publications, p. 65.
Österle, H., Schmid, R.E. and Bach, V. (2000) Mit customer relationship management zum Prozessportal [CRM and process portals]. In: H. Österle and V. Bach (eds.) Customer Relationship Management in der Praxis: Erfolgreiche Wege zu Kundenzentrierten Lösungen [ CRM in Practice: Successful Approaches of Customer-centric Solutions ]. Berlin, Germany: Springer.
Shahnam, E. (1999) The customer relationship management ecosystem. The Journal of Customer Loyalty 11: 22–29.
Payne, A. and Frow, P. (2005) Customer relationship management: From strategy to implementation, ANZMAC 2005 Conference: Business Interaction, Relationships and Networks. Perth, Australia: ANZMAC, p. 15.
Rigby, D.K., Reichheld, F.F. and Schefter, P. (2002) Avoid the four perils of CRM. Harvard Business Review 80: 101–107.
Winkelmann, P. (2002) Kundenbindung – richtig dosiert [Customer retention]. Marketing Journal 5: 41–45.
Salomann, H., Dous, M., Kolbe, L. and Brenner, W. (2005) Customer Relationship Management Survey: Status Quo and Future Challenges. St. Gallen, CH: Institute of Information Management, University of St. Gallen.
Webster, F.E. (1978) Is industrial marketing coming of age? In: T.V. Bonoma and G. Zaltman (eds.) Review of Marketing . Chicago, IL: American Marketing Association, pp. 138–159.
Coviello, N.E. and Brodie, R.J. (2001) Contemporary marketing practices of consumer and business-to-business firms: How different are they? Journal of Business & Industrial Marketing 16: 382–400.
Gummesson, E. (2004) Return on relationship (ROR): The value of relationship marketing and CRM in business-to-business contexts. Journal of Business & Industrial Marketing 19: 136–148.
Cohen, K.J. and Cyert, R.M. (1966) Theory of the Firm. Southern Economic Journal 32: 362–363.
Hatch, M.J. (1997) Organization Theory: Modern, Symbolic and Postmodern Perspectives. Oxford: Oxford University Press, p. 15.
Bertalanffy, L. (1950) An outline of general systems theory. British Journal for the Philosophy of Science 1: 139–164.
Senge, P. (1990) The Fifth Discipline: The Art and Practice of the Learning Organization. New York: Doubleday.
Normann, R. (1991) Service Management: Strategy and Leadership in Service Business. Chichester, UK: John Wiley & Sons, pp. 14–15.
Kühnapfel, J.B. (1995) Telekommunikations-Marketing: Design von Vermarktungskonzepten auf Basis des erweiterten Dienstleistungsmarketing. Wiesbaden, DE: Gabler.
Book Google Scholar
Scheuch, F. (1989) Marketing. Munich, Germany: Vahlen.
Sasser, E.W., Olsen, P.R. and Wykoff, D.D. (1978) Management of Service Operations. Boston, MA: Alleyn & Bacon.
Levitt, T. (1972) Production-line approach to service. Harvard Business Review 50(September–October): 41–52.
Mack, O., Mayo, M.C. and Khare, A. (2005) A strategic approach for successful CRM: A European perspective. Problems and Perspectives in Management 2: 98–106.
Schmitt, B.H. (2003) Customer Experience Management: A Revolutionary Approach to Connecting with Your Customers. New Jersey: John Wiley & Sons.
Newell, F. (2003) Why CRM Does Not Work. Princeton, NJ: Bloomberg Press.
Auer, C. (2004) Performance Measurement für Customer Relationship Management: Controlling des IKT-basierten Kundenbeziehungsmanagement [ Performance Management for IS-based CRM ]. Wiesbaden, DE: Deutscher Unversitaets-Verlag.
Lecompte, M. and Goetz, J. (1982) Problems of reliability and validity in ethnographic research. Review of Educational Research 52: 31–60.
Hofstede, G. (2007) Cultural dimensions, http://www.geert-hofstede.com , accessed 13 April 2007.
Buttle, F. (2004) Customer Relationship Management: Concepts and Tools. Burlington, MA: Elsevier Butterworth-Heinemann.
Ngai, E.W.T. (2005) Customer relationship management research (1992–2002). Marketing Intelligence & Planning 23: 582–605.
Parkinson, C.N. (1958) Parkinson's Law or the Pursuit of Progress. London: John Murray.
Whetten, D.A. (1989) What constitutes a theoretical contribution? Academy of Management Review 14: 490–495.
Miles, M.B. and Huberman, A.M. (1994) Qualitative Data Analysis: An Expanded Sourcebook. Thousand Oaks, CA: Sage Publications, p. 56.
Strauss, A. and Corbin, J. (1990, 1998) Basics of Qualitative Research: Techniques and Procedures for Developing Grounded Theory. Thousand Oaks, CA: Sage Publications.
Klein, H.K. and Myers, D.D. (1999) A set of principles for conducting and evaluating interpretive studies in information systems. MIS Quarterly 23(1): 67–94. Special Issue on Intensive Research.
Easterby-Smith, M., Thorpe, R. and Lowe, A. (2002) Management Research: An Introduction. London: Sage Publications.
Guba, E.G. and Lincoln, Y.S. (2005) Paradigmatic controversies, contradictions, and emerging confluences. In: N.K. Denzin and Y.S. Lincoln (eds.) The Sage Handbook of Qualitative Research, 3rd edn. Thousand Oaks, CA: Sage Publications.
Glaser, B.G. and Strauss, A.L. (1967) The Discovery of Grounded Theory: Strategies for Qualitative Research. New York: Aldine de Gruyter.
Dey, I. (1999) Grounding Grounded Theory: Guidelines for Qualitative Inquiry. San Diego, CA: Academic Press.
Glaser, B.G. (1978) Theoretical Sensitivity: Advances in the Methodology of Grounded Theory. Mill Valley, CA: Sociology Press, p. 74.
Urquhart, C. (2001) An encounter with grounded theory: Tackling the practical and philosophical issues. In: E.M. Trauth (ed.) Qualitative Research in IS: Issues and Trends. London: Idea Group Publishing, p. 115.
Alexander, C., Ishikawa, S., Silverstein, M., Jacobson, I. and Fiksdahl-King, S.A. (1977) A Pattern Language – Towns, Buildings, Construction. New York, NY: Oxford University Press.
Koestler, A. (1975, c1967) The Ghost in the Machine. London: Pan Books.
OMG. (2003) Unified Modelling Language Specification, Version 1.5. Needham, MA: Object Management Group.
Wengraf, T. (2001) Qualitative Research Interviewing: Biographic Narrative and Semi-structured Methods. London: Sage Publications, pp. 61–93.
Silverman, D. (2000) Doing Qualitative Research: A Practical Handbook. London: Sage Publications.
Glaser, B.G. (1992) Basics of Grounded Theory Analysis: Emergence vs Forcing. Mill Valley, CA: Sociology Press, p. 116.
Einstein, A. (1918) Principles of Research. Berlin, Germany: Max Planck Institut. Berlin, Physical Society.
Kaplan, R.S. and Norton, D.P. (1992) The balanced scorecard – Measures that drive performance. Harvard Business Review 70 (January–February): 71–79.
Woodcock, N. (2000) Does how customers are managed impact on business performance? Interactive Marketing 1: 375–389.
Telemanagement Forum. (2001, 2005) Enhanced Telecom Operations Map (eTOM): The Business Process Framework for the Information and Communications Services Industry, GB921, V6.1. Morristown, NJ: TeleManagement Forum.
Lovelock, C. (1984, 2006) Services Marketing: People, Technology, Strategy. Englewood Cliffs, NJ: Prentice Hall.
Homburg, C. and Sieben, F.G. (2001) Customer relationship management (CRM) – Strategische Ausrichtung statt IT-getriebenem Aktivismus [CRM – Strategic alignment instead of IT-driven short-term actions]. In: M. Bruhn and C. Homburg (eds.) Handbuch Kundenbindungsmanagement: Grundlagen – Konzepte – Erfahrungen [ Handbook Customer Retention: Basics – Concepts – Experiences ]. Wiesbaden, DE: Gabler.
Taxen, L. (2003) A Framework for the Coordination of Complex Systems’ Development. Linköping, Sweden: Department of Computer and Information Science, Linköpings universitet.
Kleinke, C.L. (1984) Two models for conceptualizing the attitude-behavior relationship. Human Relations 37: 333–350.
Mcguire, W.J. (1969) The nature of attitude and attitude change. In: G. Lindzey and E. Aronson (eds.) The Handbook of Social Psychology. Reading, MA: Addison-Wesley.
Oskamp, S. (1977) Attitudes and Opinions. New Jersey: Prentice-Hall.
Bem, D.J. (1972) Self-perception theory. In: L. Berkowitz (ed.) Advances in Experimental Social Psychology. New York: Academic Press.
Einstein, A. (1954) Ideas and Opinions. New York: Random House, p. 28.
Download references
Author information
Authors and affiliations.
Angler Street 7, Munich, 80339, Germany
Michael Labus
You can also search for this author in PubMed Google Scholar
Corresponding author
Correspondence to Michael Labus .
Rights and permissions
Reprints and permissions
About this article
Labus, M., Stone, M. The CRM behaviour theory – Managing corporate customer relationships in service industries. J Database Mark Cust Strategy Manag 17 , 155–173 (2010). https://doi.org/10.1057/dbm.2010.17
Download citation
Received : 22 September 2010
Revised : 22 September 2010
Published : 14 December 2010
Issue Date : 01 September 2010
DOI : https://doi.org/10.1057/dbm.2010.17
Share this article
Anyone you share the following link with will be able to read this content:
Sorry, a shareable link is not currently available for this article.
Provided by the Springer Nature SharedIt content-sharing initiative
- Customer Relationship Management (CRM)
- CRM software
- CRM leadership
- CRM culture
- systems thinking
- grounded theory
- Find a journal
- Publish with us
- Track your research

Finished Papers
Courtney Lees

Customer Reviews
Final Paper

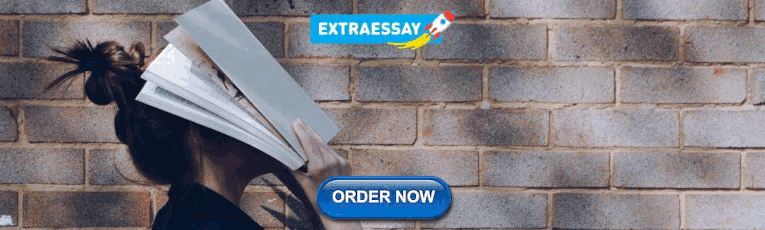
IMAGES
VIDEO
COMMENTS
CRM is a customer-service-oriented tool, placing the customer's voice at the centre of the company's efforts, thus clarifying the positive connection between CRM and consumer loyalty (Morvay, Citation 2008) through a powerful services module. In other words, customer loyalty is at the very core of economic sustainability, which leads us to ...
Master's Thesis Digital Business and Management Master of Business Administration Vaasa, 2022 . MASTER'S THESIS ... (CRM) on an organisation's performance with the case study of Dangote Flour mill. CRM (Customer Relationship Management) is widely recognized as a successful method for gathering, evaluating, and transforming important ...
This dissertation is a study of customer relationship management theory and practice. Customer Relationship Management (CRM) is a business strategy whereby companies build strong relationships with existing and prospective customers with the goal of increasing organizational profitability. It is also a learning process involving managing change in processes, people, and technology.
This article aims to review the literature on customer relationship management (CRM) research. This review article analyses the trends in CRM research, popular research topics and tools used in ...
This Dissertation is brought to you for free and open access by the Walden Dissertations and Doctoral Studies Collection at ScholarWorks. It has been ... Management (CRM) systems by 2017, it is critical that leaders understand the impact of CRM on their bottom line. The purpose of this correlational study was to investigate
The three dimensions that have been frequently used in prior studies that address the impact of CRM on customer satisfaction and customer loyalty are service quality (SQ), service access (SA), and ...
PDF | Customer relationship management (CRM) is a combination of people, processes and technology that seeks to understand a company's customers. ... Thesis PDF Available. CUSTOMER RELATIONSHIP ...
thesis in industrial engineering at Luleå University of Technology. The work was assigned by IBM in Sweden, and one of their service departments. The area of research has been interesting and this past time has given us a lot of new experiences and knowledge. ... a CRM strategy, they are supposed to focus on the relationships with their ...
1. Introduction. Customer relationship management (CRM) activity involves collecting, managing and intelligently using data with the support of technology solutions to develop long-term customer relationships and exceptional customer experience (CX) (Boulding et al., 2005; Payne and Frow, 2005; Rababah, 2011).The data obtained from all customer contact points, if well managed, can support ...
This Dissertation is brought to you for free and open access by the Walden Dissertations and Doctoral Studies ... (CRM), employee retention, and service revenue. Data were analyzed for 80 leaders and managers working in Vietnam's industrial service field. The results of the
CRM is a research field in Marketing, Business and Management as well as in IT and IS (cf. [Ngai 2005]). A focus on published research articles may result in a bias in the findings, since research is also reported in conference papers, masters and doctoral dissertations, textbooks and unpublished working papers. Paulissen et al. [2007] and
(2011) said that the success of CRM is determined by four main factors: technology, people, process, and knowledge and insight. CRM practices are the values and strategies or relationship marketing with particular emphasis on customer relationships turned into practical application - satisfying customers ultimately.
Empirical papers were maximum in number, whereas case studies were least. Primary studies were more than secondary studies. In review papers, the maximum times CRM and electronic customer relationship management (E-CRM) were taken as a basis for reviewing. The further article has classifications within primary studies and review papers.
Student thesis, Master degree (one year), 15 HE ... CRM is connected to relationship marketing and the concerned principles of this field (Parvatiyar & Jagdish, 2001). The role of IT and AI in today's world is becoming indispensable for business firms especially marketing functions. According to Hall (2019), AI marketing is basically using ...
According to one industry view, CRM consists of: Helping an enterprise to enable its marketing departments to identify and target. their best customers, manage marketing campaigns and generate ...
CRM is focus on creating, satisfy and retaining customer through uncompromising services. In India there is large number of public sector banks but there market position is not so good as compared to private sectors. Proper CRM strategy can be used by these banks to survive effectively in this competitive world.
1.1.1 CRM In Banking Sector 13 1.1.2 CRM Strategies 15 1.1.3 CRM - A Powerful Tool 17 1.1.4 Need Of CRM In Banks 18 1.2 Organization Profile 1.2.1 SBI Introduction 19 1.2.2 History Of SBI 23 1.2.3 Logo And Slogans 25 1,3 Objective of the Study 26 2 Literature Review 2.1 Trends in CRM 27 2.2 Features Of CRM 30
The purpose of this paragraph is to explore implications of this thesis on CRM employees in the telecoms industry. Employee issues in CRM are related to thinking and working in isolated, artificial boundaries. Perhaps a good example is NGS sales behaviour, which has been described by a service delivery engineer of a mobile operator as 'sell ...
BACHELOR THESIS Customer Relationship Management From a Buyer's Perspective in a B2B Relationship Thomas Axelsson Jonathan Olausson Luleå University of Technology Bachelor thesis Marketing Department of Business Administration and Social Sciences Division of Business Administration and Management 2007:208 - ISSN: 1402-1773 - ISRN: LTU-CUPP--07 ...
At Essayswriting, it all depends on the timeline you put in it. Professional authors can write an essay in 3 hours, if there is a certain volume, but it must be borne in mind that with such a service the price will be the highest. The cheapest estimate is the work that needs to be done in 14 days. Then 275 words will cost you $ 10, while 3 ...
A Study On Customer R elationship Management P ractices In Banking Sector. Shailja Pal. Research Scholar. Deparme nt of Commerce and Business Administration. Unvers y of Allahabad. , Allahabad ...
HubSpot, Inc.'s investment thesis is focused on revenue growth rates. ... Essentially, they are a CRM platform, like Salesforce , but aimed at small and medium-sized businesses.
Customer relationship management (CRM) is a successful marketing strategy, which has been proven to aid business performance, customer satisfaction and customer retention (Abu Kasim and Minai ...