Thesis Helpers

Find the best tips and advice to improve your writing. Or, have a top expert write your paper.
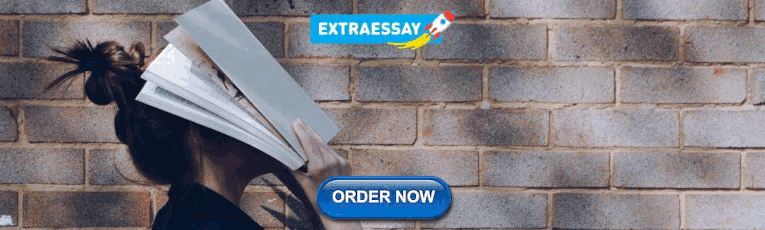
156 Hot Agriculture Research Topics For High Scoring Thesis
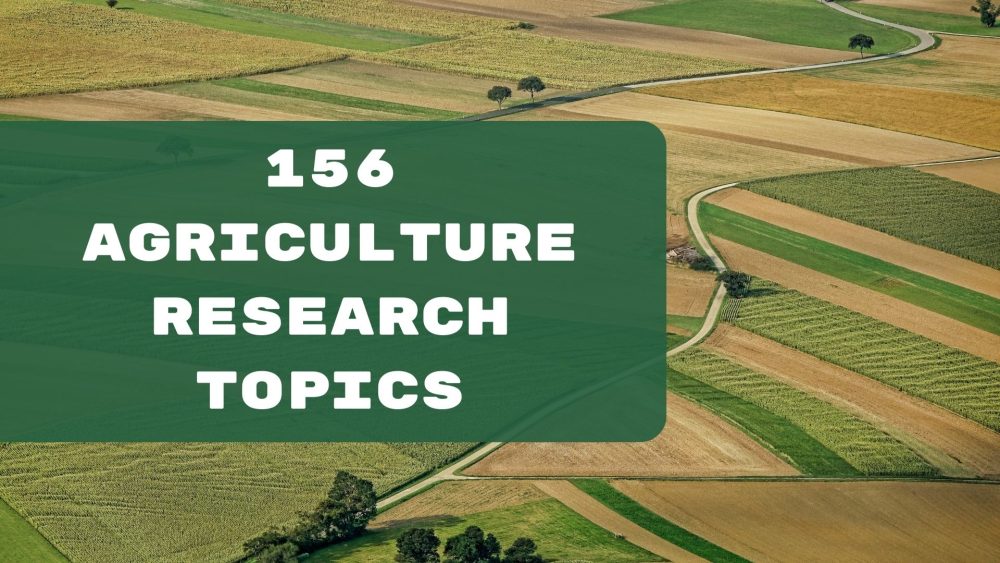
Are you preparing an agriculture research paper or dissertation on agriculture but stuck trying to pick the right topic? The title is very important because it determines how easy or otherwise the process of writing the thesis will be. However, this is never easy for many students, but you should not give up because we are here to offer some assistance. This post is a comprehensive list of the best 156 topics for agriculture projects for students. We will also outline what every part of a thesis should include. Keep reading and identify an interesting agriculture topic to use for your thesis paper. You can use the topics on agriculture as they are or change them a bit to suit your project preference.
What Is Agriculture?
Also referred to as farming, agriculture is the practice of growing crops and raising livestock. Agriculture extends to processing plants and animal products, their distribution and use. It is an essential part of local and global economies because it helps to feed people and supply raw materials for different industries.
The concept of agriculture is evolving pretty fast, with modern agronomy extending to complex technology. For example, plant breeding, agrochemicals, genetics, and relationship to emerging disasters, such as global warming, are also part of agriculture. For students studying agriculture, the diversity of the subject is a good thing, but it can also make selecting the right research paper, thesis, or dissertation topics a big challenge.
How To Write A Great Thesis: What Should You Include In Each Section?
If you are working on a thesis, it is prudent to start by understanding the main structure. In some cases, your college/ university professor or the department might provide a structure for it, but if it doesn’t, here is an outline:
- Thesis Topic This is the title of your paper, and it is important to pick something that is interesting. It should also have ample material for research.
- Introduction This takes the first chapter of a thesis paper, and you should use it to set the stage for the rest of the paper. This is the place to bring out the objective of the study, justification, and research problem. You also have to bring out your thesis statement.
- Literature Review This is the second chapter of a thesis statement and is used to demonstrate that you have comprehensively looked at what other scholars have done. You have to survey different resources, from books to journals and policy papers, on the topic under consideration.
- Methodology This chapter requires you to explain the methodology that was used for the study. It is crucial because the reader wants to know how you arrived at the results. You can opt to use qualitative, quantitative, or both methods.
- Results This chapter presents the results that you got after doing your study. Make sure to use different strategies, such as tables and graphs, to make it easy for readers to understand.
- Discussion This chapter evaluates the results gathered from the study. It helps the researcher to answer the main questions that he/she outlined in the first chapter. In some cases, the discussion can be merged with the results chapter.
- Conclusion This is the summary of the research paper. It demonstrates what the thesis contributed to the field of study. It also helps to approve or nullify the thesis adopted at the start of the paper.
Interesting Agriculture Related Topics
This list includes all the interesting topics in agriculture. You can take any topic and get it free:
- Food safety: Why is it a major policy issue for agriculture on the planet today?
- European agriculture in the period 1800-1900.
- What are the main food safety issues in modern agriculture? A case study of Asia.
- Comparing agri-related problems between Latin America and the United States.
- A closer look at the freedom in the countryside and impact on agriculture: A case study of Texas, United States.
- What are the impacts of globalisation on sustainable agriculture on the planet?
- European colonisation and impact on agriculture in Asia and Africa.
- A review of the top five agriculture technologies used in Israel to increase production.
- Water saving strategies and their impacts on agriculture.
- Homeland security: How is it related to agriculture in the United States?
- The impact of good agricultural practices on the health of a community.
- What are the main benefits of biotechnology?
- The Mayan society resilience: what was the role of agriculture?
Sustainable Agricultural Research Topics For Research
The list of topics for sustainable agriculture essays has been compiled by our editors and writers. This will impress any professor. Start writing now by choosing one of these topics:
- Cover cropping and its impact on agriculture.
- Agritourism in modern agriculture.
- review of the application of agroforestry in Europe.
- Comparing the impact of traditional agricultural practices on human health.
- Comparing equity in agriculture: A case study of Asia and Africa.
- What are the humane methods employed in pest management in Europe?
- A review of water management methods used in sustainable agriculture.
- Are the current methods used in agricultural production sufficient to feed the rapidly growing population?
- A review of crop rotation and its effects in countering pests in farming.
- Using sustainable agriculture to reduce soil erosion in agricultural fields.
- Comparing the use of organic and biological pesticides in increasing agricultural productivity.
- Transforming deserts into agricultural lands: A case study of Israel.
- The importance of maintaining healthy ecosystems in raising crop productivity.
- The role of agriculture in countering the problem of climate change.
Unique Agriculture Research Topics For Students
If students want to receive a high grade, they should choose topics with a more complicated nature.This list contains a variety of unique topics that can be used. You can choose from one of these options right now:
- Why large-scale farming is shifting to organic agriculture.
- What are the implications of groundwater pollution on agriculture?
- What are the pros and cons of raising factory farm chickens?
- Is it possible to optimise food production without using organic fertilisers?
- A review of the causes of declining agricultural productivity in African fields.
- The role of small-scale farming in promoting food sufficiency.
- The best eco-strategies for improving the productivity of land in Asia.
- Emerging concerns about agricultural production.
- The importance of insurance in countering crop failure in modern agriculture.
- Comparing agricultural policies for sustainable agriculture in China and India.
- Is agricultural technology advancing rapidly enough to feed the rapidly growing population?
- Reviewing the impact of culture on agricultural production: A case study of rice farming in Bangladesh.
Fun Agricultural Topics For Your Essay
This list has all the agricultural topics you won’t find anywhere else. It contains fun ideas for essay topics on agriculture that professors may find fascinating:
- Managing farm dams to support modern agriculture: What are the best practices?
- Native Americans’ history and agriculture.
- Agricultural methods used in Abu Dhabi.
- The history of agriculture: A closer look at the American West.
- What impacts do antibiotics have on farm animals?
- Should we promote organic food to increase food production?
- Analysing the impact of fish farming on agriculture: A case study of Japan.
- Smart farming in Germany: The impact of using drones in crop management.
- Comparing the farming regulations in California and Texas.
- Economics of pig farming for country farmers in the United States.
- Using solar energy in farming to reduce carbon footprint.
- Analysing the effectiveness of standards used to confine farm animals.
Technology And Agricultural Related Topics
As you can see, technology plays a significant role in agriculture today.You can now write about any of these technology-related topics in agriculture:
- A review of technology transformation in modern agriculture.
- Why digital technology is a game changer in agriculture.
- The impact of automation in modern agriculture.
- Data analysis and biology application in modern agriculture.
- Opportunities and challenges in food processing.
- Should artificial intelligence be made mandatory in all farms?
- Advanced food processing technologies in agriculture.
- What is the future of genetic engineering of agricultural crops?
- Is fertiliser a must-have for success in farming?
- Agricultural robots offer new hope for enhanced productivity.
- Gene editing in agriculture: Is it a benefit or harmful?
- Identify and trace the history of a specific technology and its application in agriculture today.
- What transformations were prompted by COVID-19 in the agricultural sector?
- Reviewing the best practices for pest management in agriculture.
- Analysing the impacts of different standards and policies for pest management in two countries of your choice on the globe.
Easy Agriculture Research Paper Topics
You may not want to spend too much time writing the paper. You have other things to accomplish. Look at this list of topics that are easy to write about in agriculture:
- Agricultural modernization and its impacts in third world countries.
- The role of human development in agriculture today.
- The use of foreign aid and its impacts on agriculture in Mozambique.
- The effect of hydroponics in agriculture.
- Comparing agriculture in the 20th and 21st centuries.
- Is it possible to engage in farming without water?
- Livestock owners should use farming methods that will not destroy forests.
- Subsistence farming versus commercial farming.
- Comparing the pros and cons of sustainable and organic agriculture.
- Is intensive farming the same as sustainable agriculture?
- A review of the leading agricultural practices in Latin America.
- Mechanisation of agriculture in Eastern Europe: A case study of Ukraine.
- Challenges facing livestock farming in Australia.
- Looking ahead: What is the future of livestock production for protein supply?
Emerging Agriculture Essay Topics
Emerging agriculture is an important part of modern life. Why not write an essay or research paper about one of these emerging agriculture topics?
- Does agriculture help in addressing inequality in society?
- Agricultural electric tractors: Is this a good idea?
- What ways can be employed to help Africa improve its agricultural productivity?
- Is education related to productivity in small-scale farming?
- Genome editing in agriculture: Discuss the pros and cons.
- Is group affiliation important in raising productivity in Centre Europe? A case study of Ukraine.
- The use of Agri-Nutrition programs to change gender norms.
- Mega-Farms: Are they the future of agriculture?
- Changes in agriculture in the next ten years: What should we anticipate?
- A review of the application of DNA fingerprinting in agriculture.
- Global market of agricultural products: Are non-exporters locked out of foreign markets for low productivity?
- Are production technologies related to agri-environmental programs more eco-efficient?
- Can agriculture support greenhouse mitigation?
Controversial Agricultural Project For Students
Our team of experts has searched for the most controversial topics in agriculture to write a thesis on. These topics are all original, so you’re already on your way towards getting bonus points from professors. However, the process of writing is sometimes not as easy as it seems, so dissertation writers for hire will help you to solve all the problems.
- Comparing the mechanisms of US and China agricultural markets: Which is better?
- Should we ban GMO in agriculture?
- Is vivisection a good application or a necessary evil?
- Agriculture is the backbone of modern Egypt.
- Should the use of harmful chemicals in agriculture be considered biological terror?
- How the health of our planet impacts the food supply networks.
- People should buy food that is only produced using sustainable methods.
- What are the benefits of using subsidies in agriculture? A case study of the United States.
- The agrarian protests: What were the main causes and impacts?
- What impact would a policy requiring 2/3 of a country to invest in agriculture have?
- Analysing the changes in agriculture over time: Why is feeding the world population today a challenge?
Persuasive Agriculture Project Topics
If you have difficulty writing a persuasive agricultural project and don’t know where to start, we can help. Here are some topics that will convince you to do a persuasive project on agriculture:
- What is the extent of the problem of soil degradation in the US?
- Comparing the rates of soil degradation in the United States and Africa.
- Employment in the agricultural sector: Can it be a major employer as the population grows?
- The process of genetic improvement for seeds: A case study of agriculture in Germany.
- The importance of potatoes in people’s diet today.
- Comparing sweet potato production in the US to China.
- What is the impact of corn production for ethanol production on food supply chains?
- A review of sustainable grazing methods used in the United States.
- Does urban proximity help improve efficiency in agriculture?
- Does agriculture create economic spillovers for local economies?
- Analysing the use of sprinkle drones in agriculture.
- The impact of e-commerce development on agriculture.
- Reviewing the agricultural policy in Italy.
- Climate change: What does it mean for agriculture in developed nations?
Advanced Agriculture Project Topics
A more difficult topic can help you impress your professor. It can earn you bonus points. Check out the latest list of advanced agricultural project topics:
- Analysing agricultural exposure to toxic metals: The case study of arsenic.
- Identifying the main areas for reforms in agriculture in the United States.
- Are developed countries obligated to help starving countries with food?
- World trade adjustments to emerging agricultural dynamics and climate change.
- Weather tracking and impacts on agriculture.
- Pesticides ban by EU and its impacts on agriculture in Asia and Africa.
- Traditional farming methods used to feed communities in winter: A case study of Mongolia.
- Comparing the agricultural policy of the EU to that of China.
- China grew faster after shifting from an agro to an industrial-based economy: Should more countries move away from agriculture to grow?
- What methods can be used to make agriculture more profitable in Africa?
- A comprehensive comparison of migratory and non-migratory crops.
- What are the impacts of mechanical weeding on soil structure and fertility?
- A review of the best strategies for restoring lost soil fertility in agricultural farmlands: A case study of Germany.
Engaging Agriculture Related Research Topics
When it comes to agriculture’s importance, there is so much to discuss. These engaging topics can help you get started in your research on agriculture:
- Agronomy versus horticultural crops: What are the main differences?
- Analysing the impact of climate change on the food supply networks.
- Meat processing laws in Germany.
- Plant parasites and their impacts in agri-production: A case study of India.
- Milk processing laws in Brazil.
- What is the extent of post-harvest losses on farming profits?
- Agri-supply chains and local food production: What is the relationship?
- Can insects help improve agriculture instead of harming it?
- The application of terraculture in agriculture: What are the main benefits?
- Vertical indoor farms.
- Should we be worried about the declining population of bees?
- Is organic food better than standard food?
- What are the benefits of taking fresh fruits and veggies?
- The impacts of over-farming on sustainability and soil quality.
Persuasive Research Topics in Agriculture
Do you need to write a paper on agriculture? Perfect! Here are the absolute best persuasive research topics in agriculture:
- Buying coffee produced by poor farmers to support them.
- The latest advances in drip irrigation application.
- GMO corn in North America.
- Global economic crises and impact on agriculture.
- Analysis of controversies on the use of chemical fertilisers.
- What challenges are facing modern agriculture in France?
- What are the negative impacts of cattle farms?
- A closer look at the economics behind sheep farming in New Zealand.
- The changing price of energy: How important is it for the local farms in the UK?
- A review of the changing demand for quality food in Europe.
- Wages for people working in agriculture.
Work With Experts To Get High Quality Thesis Paper
Once you pick the preferred topic of research, it is time to get down and start working on your thesis paper. If writing the paper is a challenge, do not hesitate to seek thesis help from our experts. We work with ENL writers who are educated in top universities. Therefore, you can trust them to carry out comprehensive research on your paper and deliver quality work to impress your supervisor. Students who come to us for assistance give a high rating to our writers after scoring top grades or emerging top in class. Our trustworthy experts can also help with other school assignments, thesis editing, and proofreading. We have simplified the process of placing orders so that every student can get assistance quickly and affordably. You only need to navigate to the ordering page to buy a custom thesis paper online.

Make PhD experience your own
Leave a Reply Cancel reply
Your email address will not be published. Required fields are marked *
Thank you for visiting nature.com. You are using a browser version with limited support for CSS. To obtain the best experience, we recommend you use a more up to date browser (or turn off compatibility mode in Internet Explorer). In the meantime, to ensure continued support, we are displaying the site without styles and JavaScript.
- View all journals
- Explore content
- About the journal
- Publish with us
- Sign up for alerts
- Perspective
- Published: 23 December 2021
On-Farm Experimentation to transform global agriculture
- Myrtille Lacoste ORCID: orcid.org/0000-0001-6557-1865 1 , 2 ,
- Simon Cook ORCID: orcid.org/0000-0003-0902-1476 1 , 3 ,
- Matthew McNee 4 ,
- Danielle Gale ORCID: orcid.org/0000-0003-3733-025X 1 ,
- Julie Ingram ORCID: orcid.org/0000-0003-0712-4789 5 ,
- Véronique Bellon-Maurel 6 , 7 ,
- Tom MacMillan ORCID: orcid.org/0000-0002-2893-6981 8 ,
- Roger Sylvester-Bradley 9 ,
- Daniel Kindred ORCID: orcid.org/0000-0001-7910-7676 9 ,
- Rob Bramley ORCID: orcid.org/0000-0003-0643-7409 10 ,
- Nicolas Tremblay ORCID: orcid.org/0000-0003-1409-4442 11 ,
- Louis Longchamps ORCID: orcid.org/0000-0002-4761-6094 12 ,
- Laura Thompson ORCID: orcid.org/0000-0001-5751-7869 13 ,
- Julie Ruiz ORCID: orcid.org/0000-0001-5672-2705 14 ,
- Fernando Oscar García ORCID: orcid.org/0000-0001-6681-0135 15 , 16 ,
- Bruce Maxwell 17 ,
- Terry Griffin ORCID: orcid.org/0000-0001-5664-484X 18 ,
- Thomas Oberthür ORCID: orcid.org/0000-0002-6050-9832 19 , 20 ,
- Christian Huyghe 21 ,
- Weifeng Zhang 22 ,
- John McNamara 23 &
- Andrew Hall ORCID: orcid.org/0000-0002-8580-6569 24
Nature Food volume 3 , pages 11–18 ( 2022 ) Cite this article
3817 Accesses
73 Citations
141 Altmetric
Metrics details
- Agriculture
- Science, technology and society
- Scientific community
- Sustainability
Restructuring farmer–researcher relationships and addressing complexity and uncertainty through joint exploration are at the heart of On-Farm Experimentation (OFE). OFE describes new approaches to agricultural research and innovation that are embedded in real-world farm management, and reflects new demands for decentralized and inclusive research that bridges sources of knowledge and fosters open innovation. Here we propose that OFE research could help to transform agriculture globally. We highlight the role of digitalization, which motivates and enables OFE by dramatically increasing scales and complexity when investigating agricultural challenges.
This is a preview of subscription content, access via your institution
Access options
Access Nature and 54 other Nature Portfolio journals
Get Nature+, our best-value online-access subscription
24,99 € / 30 days
cancel any time
Subscribe to this journal
Receive 12 digital issues and online access to articles
111,21 € per year
only 9,27 € per issue
Buy this article
- Purchase on Springer Link
- Instant access to full article PDF
Prices may be subject to local taxes which are calculated during checkout
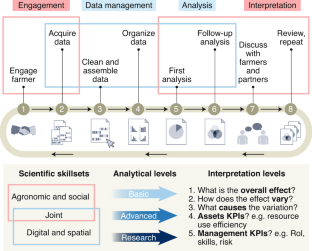
Similar content being viewed by others
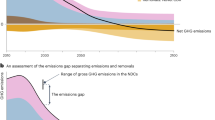
The carbon dioxide removal gap
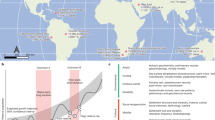
Frequent disturbances enhanced the resilience of past human populations
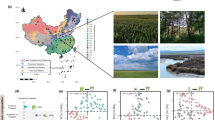
Land conversion to agriculture induces taxonomic homogenization of soil microbial communities globally
Data availability.
The authors declare that the data supporting the findings of this study are available within the paper and its Supplementary Information (sources of Figs. 1 – 3 ).
Whitfield, S., Challinor, A. J. & Rees, R. M. Frontiers in climate smart food systems: outlining the research space. Front. Sustain. Food Syst. 2 , https://doi.org/10.3389/fsufs.2018.00002 (2018).
Scoones, I. & Thompson, J. (eds) Farmer First Revisited: Innovation for Agricultural Research and Development 1st edn (Practical Action Publishing, 2009).
Stone, G. D. Towards a general theory of agricultural knowledge production: environmental, social, and didactic learning. Cult. Agric. Food Environ. 38 , 5–17 (2016).
Hansson, S. O. Farmers’ experiments and scientific methodology. Euro. J. Phil. Sci. 9 , 32 (2019).
Maat, H. & Glover, D. in Contested Agronomy: Agricultural Research in a Changing World (eds Sumberg, J. & Thompson, J.) 131–145 (Routledge, 2012).
Šūmane, S. et al. Local and farmers’ knowledge matters! How integrating informal and formal knowledge enhances sustainable and resilient agriculture. J. Rural Stud. 59 , 232–241 (2018).
Article Google Scholar
de Janvry, A., Sadoulet, E. & Rao, M. Adjusting Extension Models to the Way Farmers Learn Policy Brief No. 159 (FERDI, 2016).
Cross, R. & Ampt, P. Exploring agroecological sustainability: unearthing innovators and documenting a community of practice in Southeast Australia. Soc. Nat. Resour. 30 , 585–600 (2016).
Rickards, L., Alexandra, J., Jolley, C., Farhey, K. & Frewer, T. Review of Agricultural Extension (ACIAR, 2019).
MacMillan, T. & Benton, T. G. Engage farmers in research. Nature 509 , 25–27 (2014).
Article ADS CAS Google Scholar
Waters-Bayer, A. et al. Exploring the impact of farmer-led research supported by civil society organisations. Agric. Food Secur. 4 , 4 (2015).
Berthet, E. T. A., Barnaud, C., Girard, N., Labatut, J. & Martin, G. How to foster agroecological innovations? A comparison of participatory design methods. J. Environ. Plan. Manage. 59 , 280–301 (2015).
Cook, S. et al. An on-farm experimental philosophy for farmer-centric digital innovation. In 14th International Conference on Precision Agriculture (ISPA, 2018).
Cook, S. E., Cock, J., Oberthür, T. & Fisher, M. On-farm experimentation. Better Crops 97 , 17–20 (2013).
Google Scholar
Richardson, M. et al. Farmer research networks in principle and practice. Int. J. Agric. Sustain. https://doi.org/10.1080/14735903.2021.1930954 (2021).
Thompson, L. J. et al. Farmers as researchers: in‐depth interviews to discern participant motivation and impact. Agron. J. 111 , 2670–2680 (2019).
Sewell, A. M. et al. Hatching new ideas about herb pastures: learning together in a community of New Zealand farmers and agricultural scientists. Agric. Syst. 125 , 63–73 (2014).
Bramley, R. G. V., Lawes, R. & Cook, S. in Precision Agriculture for Sustainability and Environmental Protection (eds Oliver, M. A., Bishop, T. F. A. & Marchant, B. M.) 205–218 (Routledge, 2013).
Marchant, B. et al. Establishing the precision and robustness of farmers’ crop experiments. Field Crops Res. 230 , 31–45 (2019).
Briggs, J. Indigenous knowledge: a false dawn for development theory and practice? Progr. Dev. Stud. 13 , 231–243 (2013).
Caron, P., Biénabe, E. & Hainzelin, E. Making transition towards ecological intensification of agriculture a reality: the gaps in and the role of scientific knowledge. Curr. Opin. Environ. Sustain. 8 , 44–52 (2014).
Kool, H., Andersson, J. A. & Giller, K. E. Reproducibility and external validity of on-farm experimental research in Africa. Exp. Agric . 56 , 587–607 (2020).
de Roo, N., Andersson, J. A. & Krupnik, T. J. On-farm trials for development impact? The organisation of research and the scaling of agricultural technologies. Exp. Agric. 55 , 163–184 (2019).
Möhring, N. et al. Pathways for advancing pesticide policies. Nat. Food 1 , 535–540 (2020).
Sylvester-Bradley, R. et al. Agronōmics: transforming crop science through digital technologies. Adv. Anim. Biosci. 8 , 728–733 (2017).
Ruiz, J., Dumont, A. & Zingraff, V. in Penser le Gouvernement des Ressources Naturelles (eds Busca, D. & Lew, N.) 293–330 (Presses de l’Université Laval, 2019).
Fabregas, R., Kremer, M. & Schilbach, F. Realizing the potential of digital development: the case of agricultural advice. Science https://doi.org/10.1126/science.aay3038 (2019).
Dowd, A.-M. et al. The role of networks in transforming Australian agriculture. Nat. Clim. Change 4 , 558–563 (2014).
Article ADS Google Scholar
Klerkx, L., van Mierlo, B. & Leeuwis, C. in Farming Systems Research into the 21st Century: The New Dynamic (eds Darnhofer, I., Gibbon, D. & Dedieu, B.) 457–483 (Springer, 2012).
Ingram, J., Gaskell, P., Mills, J. & Dwyer, J. How do we enact co-innovation with stakeholders in agricultural research projects? Managing the complex interplay between contextual and facilitation processes. J. Rural Stud. 78 , 65–77 (2020).
Jackson, L. et al. Biodiversity and agricultural sustainagility: from assessment to adaptive management. Curr. Opin. Environ. Sustain. 2 , 80–87 (2010).
Laurent, A., Kyveryga, P., Makowski, D. & Miguez, F. A framework for visualization and analysis of agronomic field trials from on‐farm research networks. Agron. J. 111 , 2712–2723 (2019).
Kyveryga, P. M. On‐farm research: experimental approaches, analytical frameworks, case studies, and impact. Agron. J. 111 , 2633–2635 (2019).
Tremblay, N. in Precision Agriculture for Sustainability (ed. Stafford, J.) 145–168 (Burleigh Dodds Science Limited, 2019); https://doi.org/10.1201/9781351114592
Bullock, D. S. et al. The data‐intensive farm management project: changing agronomic research through on‐farm precision experimentation. Agron. J. 111 , 2736–2746 (2019).
Wyatt, J., Brown, T. & Carey, S. The next chapter in design for social innovation. Stanford Soc. Innov. Rev. 19 , 40–47 (2021).
Griffin, T. W., Fitzgerald, G. J., Lowenberg‐DeBoer, J. & Barnes, E. M. Modeling local and global spatial correlation in field‐scale experiments. Agron. J . https://doi.org/10.1002/agj2.20266 (2020).
Coudel, E., Tonneau, J.-P. & Rey-Valette, H. Diverse approaches to learning in rural and development studies: review of the literature from the perspective of action learning. Knowl. Manage. Res. Pract. 9 , 120–135 (2017).
Browning, D. M. et al. Emerging technological and cultural shifts advancing drylands research and management. Front. Ecol. Environ. 13 , 52–60 (2015).
Maxwell, B. et al. Can optimization associated with on-farm experimentation using site-specific technologies improve producer management decisions? In 14th International Conference on Precision Agriculture (2018).
Kindred, D. et al. Supporting and analysing on-farm nitrogen tramline trials so farmers, industry, agronomists and scientists can learn together. In 14th International Conference on Precision Agriculture (2018).
Oberthür, T. et al. Plantation intelligence applied oil palm operations: unlocking value by analysing commercial data. Planter 93 , 339–351 (2017).
Jin, H., Shuvo Bakar, K., Henderson, B. L., Bramley, R. G. V. & Gobbett, D. L. An efficient geostatistical analysis tool for on-farm experiments targeted at localised treatment. Biosys. Eng. 205 , 121–136 (2021).
Berthet, E. T., Hickey, G. M. & Klerkx, L. Opening design and innovation processes in agriculture: insights from design and management sciences and future directions. Agric. Syst. 165 , 111–115 (2018).
Curley, M. Twelve principles for open innovation 2.0. Nature 533 , 315–316 (2016).
Ryan, S. F. et al. The role of citizen science in addressing grand challenges in food and agriculture research. Proc. Biol. Sci . 285 , 20181977 (2018).
Herrero, M. et al. Innovation can accelerate the transition towards a sustainable food system. Nat. Food 1 , 266–272 (2020).
Fielke, S. J. et al. Conceptualising the DAIS: implications of the ‘digitalisation of agricultural innovation systems’ on technology and policy at multiple levels. NJAS 90–91 , 100296 (2019).
Cook, S., Jackson, E. L., Fisher, M. J., Baker, D. & Diepeveen, D. Embedding digital agriculture into sustainable Australian food systems: pathways and pitfalls to value creation. Int. J. Agric. Sustain. https://doi.org/10.1080/14735903.2021.1937881 (2021).
van Etten, J. et al. Crop variety management for climate adaptation supported by citizen science. Proc. Natl Acad. Sci. USA 116 , 4194–4199 (2019).
Ingram, J. & Maye, D. What are the implications of digitalisation for agricultural knowledge? Front. Sustain. Food Syst. 4 , https://doi.org/10.3389/fsufs.2020.00066 (2020).
McNee, M. Government Support for Farmer-Based Research in the Falkland Islands AAC Agenda 07.11.2019, Item 10 (Agricultural Advisory Committee, Falkland Islands Government, 2019).
Zhang, W. et al. Closing yield gaps in China by empowering smallholder farmers. Nature 537 , 671–674 (2016).
Lechenet, M., Dessaint, F., Py, G., Makowski, D. & Munier-Jolain, N. Reducing pesticide use while preserving crop productivity and profitability on arable farms. Nat. Plants 3 , 17008 (2017).
García, F. et al. La Red de Nutrición de la Región Crea Sur de Santa Fe: Resultados y Conclusiones de los Primeros Diez Años 2000-2009 (AACREA, 2010).
Posner, S. M., McKenzie, E. & Ricketts, T. H. Policy impacts of ecosystem services knowledge. Proc. Natl. Acad Sci. USA 113 , 1760–1765 (2016).
Moore, M.-L., Riddell, D. & Vocisano, D. Scaling out, scaling up, scaling deep. Strategies of non-profits in advancing systemic social innovation. J. Corp. Citizenship 58 , 67–84 (2015).
Payan, J.‐C. & Pichon, L. ApeX‐Vigne, Version 2020: Une Application Mobile Gratuite pour Faciliter le Suivi de la Croissance des Vignes et Estimer la Contrainte Hydrique (Institut Français de la Vigne et du Vin and Institut Agro, Montpellier SupAgro, 2020).
Samberg, L. H. A collaboration worth its weight in grain. Nature 537 , 624–625 (2016).
Download references
Acknowledgements
This study was funded by the Premier’s Agriculture and Food Fellowship Program of Western Australia. This Fellowship is a collaboration between Curtin and Murdoch Universities and the State Government. The Fellowship is the centrepiece of the Science and Agribusiness Connect initiative, made possible by the State Government’s Royalties for Regions program. Additional support was provided by the MAK’IT-FIAS Fellowship programme (Montpellier Advanced Knowledge Institute on Transitions – French Institutes for Advanced Study) co-funded by the University of Montpellier and the European Union’s Horizon 2020 Marie Skłodowska-Curie Actions (co-fund grant agreement no. 945408), the Digital Agriculture Convergence Lab #DigitAg (grant no. ANR-16-CONV-0004) supported by ANR/PIA, and the Elizabeth Creak Charitable Trust. Contributions toward enabling workshops were made by the USDA (USDA AFRI FACT Los Angeles 2017), the International Society for Precision Agriculture (ICPA Montreal 2018 OFE-C, On-Farm Experimentation Community), the National Key Research and Development Program of China (2016YFD0201303) and ADAS (Cambridge 2018), the European Conference for Precision Agriculture (ECPA Montpellier 2019) and the OECD Co-operative Research Program for ‘Biological resource management for sustainable agricultural systems – Transformational technologies and innovation’ towards ‘#OFE2021, the first Conference on farmer-centric On-Farm Experimentation – Digital Tools for a Scalable Transformative Pathway’. L. Tresh assisted with the design and preparation of Figs. 2 and 3. Members of the #OFE2021 Working Groups also contributed their experiences and insights.
Author information
Authors and affiliations.
Centre for Digital Agriculture, Curtin University, Perth, Western Australia, Australia
Myrtille Lacoste, Simon Cook & Danielle Gale
Montpellier Advanced Knowledge Institute on Transitions (MAK’IT), University of Montpellier, Montpellier, France
Myrtille Lacoste
Centre for Digital Agriculture, Murdoch University, Perth, Western Australia, Australia
Department of Agriculture, Falkland Islands Government, Stanley, Falkland Islands
Matthew McNee
Countryside and Community Research Institute, University of Gloucestershire, Cheltenham, UK
Julie Ingram
Technologies and methods for the agricultures of tomorrow (ITAP), University of Montpellier–National Research Institute for Agriculture, Food and Environment (INRAE)–L’Institut Agro, Montpellier, France
Véronique Bellon-Maurel
Digital Agriculture Convergence Lab (#DigitAg), National Research Institute for Agriculture, Food and Environment (INRAE), Montpellier, France
Centre for Effective Innovation in Agriculture, Royal Agricultural University, Cirencester, UK
Tom MacMillan
ADAS, Cambridge, UK
Roger Sylvester-Bradley & Daniel Kindred
Commonwealth Scientific and Industrial Research Organisation (CSIRO), Adelaide, South Australia, Australia
Rob Bramley
Saint-Jean-sur-Richelieu Research and Development Centre, Agriculture and Agri-Food Canada (AAFC), St-Jean-sur-Richelieu, Quebec, Canada
Nicolas Tremblay
School of Integrative Plant Science, Cornell University, Ithaca, NY, USA
Louis Longchamps
Institute of Agriculture and Natural Resources, University of Nebraska-Lincoln, Falls City, NE, USA
Laura Thompson
Watershed and Aquatic Ecosystem Interactions Research Centre (RIVE), Université du Québec à Trois-Rivières, Trois-Rivières, Québec, Canada
Latin America Southern Cone Group, International Plant Nutrition Institute (IPNI), Buenos Aires, Argentina
Fernando Oscar García
Faculty of Agricultural Sciences, National University of Mar del Plata, Balcarce, Argentina
Montana Institute on Ecosystems, Montana State University, Bozeman, MT, USA
Bruce Maxwell
Department of Agricultural Economics, Kansas State University, Manhattan, KS, USA
Terry Griffin
Southeast Asia Group, International Plant Nutrition Institute (IPNI), Penang, Malaysia
Thomas Oberthür
Business and Partnership Development, African Plant Nutrition Institute (APNI), Benguérir, Morocco
Scientific Direction of Agriculture, National Research Institute for Agriculture, Food and Environment (INRAE), Paris, France
Christian Huyghe
College of Resources and Environmental Sciences and National Academy of Agriculture Green Development, China Agricultural University, Beijing, China
Weifeng Zhang
National Animal Nutrition Program (NANP), United States Department of Agriculture (USDA), Pullman, WA, USA
John McNamara
Commonwealth Scientific and Industrial Research Organisation (CSIRO), Canberra, Australian Capital Territory, Australia
Andrew Hall
You can also search for this author in PubMed Google Scholar
Contributions
M.L. and S.C. developed the study concept. M.M., D.G., J.I., V.B.-M., T.M., R.S.-B. and A.H. contributed additional concept development. M.L. and D.G. obtained the data and prepared the results. M.L., M.M., L.T., D.K., F.O.G., B.M., V.B.-M., J.R., C.H. and W.Z. contributed data. M.L. wrote the manuscript with input from all other authors.
Corresponding author
Correspondence to Myrtille Lacoste .
Ethics declarations
Competing interests.
The authors declare no competing interests.
Additional information
Peer review information Nature Food thanks Carol Shennan, Petro Kyveryga, Nicolas Martin and the other, anonymous, reviewer(s) for their contribution to the peer review of this work.
Publisher’s note Springer Nature remains neutral with regard to jurisdictional claims in published maps and institutional affiliations.
Supplementary information
Supplementary information.
Sources for Figs. 1–3.
Rights and permissions
Reprints and permissions
About this article
Cite this article.
Lacoste, M., Cook, S., McNee, M. et al. On-Farm Experimentation to transform global agriculture. Nat Food 3 , 11–18 (2022). https://doi.org/10.1038/s43016-021-00424-4
Download citation
Received : 13 August 2020
Accepted : 05 November 2021
Published : 23 December 2021
Issue Date : January 2022
DOI : https://doi.org/10.1038/s43016-021-00424-4
Share this article
Anyone you share the following link with will be able to read this content:
Sorry, a shareable link is not currently available for this article.
Provided by the Springer Nature SharedIt content-sharing initiative
This article is cited by
Can machine learning models provide accurate fertilizer recommendations.
- Takashi S. T. Tanaka
- Gerard B. M. Heuvelink
- David S. Bullock
Precision Agriculture (2024)
Digital strategies for nitrogen management in grain production systems: lessons from multi-method assessment using on-farm experimentation
- A. F. Colaço
- B. M. Whelan
- R. A. Lawes
Fully Bayesian economically optimal design for a spatially varying coefficient linear stochastic plateau model over multiple years
- Davood Poursina
- B. Wade Brorsen
Stochastic Environmental Research and Risk Assessment (2024)
Analytic transparency is key for reproducibility of agricultural research
- Gudeta W. Sileshi
CABI Agriculture and Bioscience (2023)
Pesticide reduction amidst food and feed security concerns in Europe
- Kevin Schneider
- Jesus Barreiro-Hurle
- Emilio Rodriguez-Cerezo
Nature Food (2023)
Quick links
- Explore articles by subject
- Guide to authors
- Editorial policies
Sign up for the Nature Briefing: Anthropocene newsletter — what matters in anthropocene research, free to your inbox weekly.

Agricultural Research: Applications and Future Orientations
- Reference work entry
- First Online: 01 January 2020
- Cite this reference work entry
- Naser Valizadeh Ph.D. Student 6 &
- Masoud Bijani Assistant Professor 7
Part of the book series: Encyclopedia of the UN Sustainable Development Goals ((ENUNSDG))
106 Accesses
1 Citations
Agricultural research methodology
Agricultural research can be broadly defined as any research activity aimed at improving productivity and quality of crops by their genetic improvement, better plant protection, irrigation, storage methods, farm mechanization, efficient marketing, and a better management of resources (Loebenstein and Thottappilly 2007 ).
Introduction
The objective of this document is to provide a tool to understand aspects and future orientations of agricultural research. It begins with an overview of the concept and/or definition of agricultural research. It then focuses on the role of agricultural research in achieving the goals of 2030 Agenda, different types of agricultural researched, systemic research methodology in agriculture, and finally different kinds of use for agricultural research.
The Concept and Definition of Agricultural Research
Finding answers for questions about unknown phenomena in the agricultural area is the key to agricultural...
This is a preview of subscription content, log in via an institution to check access.
Access this chapter
- Available as PDF
- Read on any device
- Instant download
- Own it forever
- Available as EPUB and PDF
- Durable hardcover edition
- Dispatched in 3 to 5 business days
- Free shipping worldwide - see info
Tax calculation will be finalised at checkout
Purchases are for personal use only
Institutional subscriptions
Aboelela SW, Larson E, Bakken S, Carrasquillo O, Formicola A, Glied SA, Haas J, Gebbie KM (2007) Defining interdisciplinary research: conclusions from a critical review of the literature. Health Serv Res 42(1–1):329–346
Article Google Scholar
Alrøe HF, Kristensen ES (2002) Towards a systemic research methodology in agriculture: rethinking the role of values in science. Agric Hum Values 19(1):3–23
Anastasios M, Koutsouris A, Konstadinos M (2010) Information and communication technologies as agricultural extension tools: a survey among farmers in West Macedonia, Greece. J Agric Educ Ext 16(3):249–263
Bijani M, Ghazani E, Valizadeh N, Fallah Haghighi N (2017) Pro-environmental analysis of farmers’ concerns and behaviors towards soil conservation in central district of Sari County, Iran. Int Soil Water Conserv Res 5(1):43–49
Google Scholar
Borg WR, Gall MD, Gall JP (1963) Educational research: an introduction. Longmans, New York & London p 704
Damalas CA, Georgiou EB, Theodorou MG (2006) Pesticide use and safety practices among Greek tobacco farmers: a survey. Int J Environ Health Res 16(5):339–348
Delavar A (2017) Research methods in psychology and educational sciences. Virayesh Publishing, Tehran. In Persian
Ebrahimi Sarcheshmeh E, Bijani M, Sadighi H (2018) Adoption behavior towards the use of nuclear technology in agriculture: A causal analysis. Technol Soc 54(2018):175–182
Fallah Haghighi N, Bijani M, Parhizkar M (2019) An analysis of major social obstacles affecting human resource development in Iran. J Hum Behav Soc Environ 29(3):372–388
Feder G, Just RE, Zilberman D (1985) Adoption of agricultural innovations in developing countries: a survey. Econ Dev Cult Chang 33(2):255–298
Fleischer DN, Christie CA (2009) Evaluation use: results from a survey of US American Evaluation Association members. Am J Eval 30(2):158–175
Food and Agriculture Organization of the United Nations (FAO) (2017) Food and agriculture – driving action across the 2030 agenda for sustainable development, Rome. https://www.fao.org/3/a-i7454e.pdf
Gibbons M, Limoges C, Nowotny H, Schwartzman S, Trow M (1994) The new production of knowledge. The dynamics of science and research in contemporary societies. Sage, London
Guba EG, Lincoln YS (1994) Competing paradigms in qualitative research. In NK Denzin, YS Lincoln (Eds), Handbook of qualitative research, pp 105–117. London: Sage
Habashiani R (2011) Qanat: a sustainable groundwater supply system. Master’s thesis, School of Arts and Social Science, James Cook University, Queensland
Habibpour Gatabi K, Safari Shali R (2013) Comprehensive manual for using SPSS in survey researches. Looyeh Publications, Tehran
Henry GT, Mark MM (2003) Toward an agenda for research on evaluation. N Dir Eval 97:69–80
Iman MT (2009) Paradigmatic foundations of quantitative and qualitative research methods in humanities. Research Institute of Hawzah and University, Qom. In Persian
Khoursandi-Taskouh A (2009) Typological diversity in interdisciplinary education and research. J Interdiscip Stud Humanit 1(4):57–83
Lekka-Kowalik A (2010) Why science cannot be value-free. Sci Eng Ethics 16(1):33–41
Lockheed ME, Jamison T, Lau LJ (1980) Farmer education and farm efficiency: a survey. Econ Dev Cult Chang 29(1):37–76
Loebenstein G, Thottappilly G (2007) The mission of agricultural research. In: Loebenstein G, Thottappilly G (eds) Agricultural research management. Springer, Dordrecht, pp 3–7
Chapter Google Scholar
Madani K (2014) Water management in Iran: what is causing the looming crisis? J Environ Stud Sci 4(4):315–328
Majidi F, Bijani M, Abbasi E (2017) Pathology of scientific articles publishing in the field of agriculture as perceived by faculty members and Ph. D. students (The case of colleges of agriculture at Public Universities, Iran). J Agric Sci Technol 19:1469–1484
Malekian A, Hayati D, Aarts N (2017) Conceptualizations of water security in the agricultural sector: perceptions, practices, and paradigms. J Hydrol 544:224–232
Mennatizadeh M, Zamani G (2016) Water ethics: theoretical analysis of moral development theories. Indian J Fundam Appl Life Sci 6:413–428
Mohammadi-Mehr S, Bijani M, Abbasi E (2018) Factors affecting the aesthetic behavior of villagers towards the natural environment: The case of Kermanshah province, Iran. J Agric Sci Technol 20(7):1353–1367
Morales FJ (2007) The mission and evolution of international agricultural research in developing countries. In: Loebenstein G, Thottappilly G (eds) Agricultural research management. Springer, Dordrecht, pp 9–36
Patton MQ (2008) Utilization focused evaluation, 4th edn. Sage, Thousand Oaks
Popa F, Guillermin M, Dedeurwaerdere T (2015) A pragmatist approach to transdisciplinarity in sustainability research: from complex systems theory to reflexive science. Futures 65:45–56
Raeisi AA, Bijani M, Chizari M (2018) The mediating role of environmental emotions in transition from knowledge to sustainable behavior toward exploit groundwater resources in Iran’s agriculture. Int Soil Water Conserv Res 6(2):143–152
Rosenthal R, Rosnow RL (1991) Essentials of behavioral research: methods and data analysis. McGraw-Hil, Boston
Schensul SL, Schensul JJ, LeCompte MD (2012) Initiating ethnographic research: a mixed methods approach, vol 2. AltaMira Press, London
Shadish WR, Cook TD, Leviton LC (1991) Foundations of program evaluation: theories of practice. Sage, Newbury Park
Shahvali M (2013) Explanation of transcendental innovation system for sustainability. In: The proceedings of the Iranian and Islamic pattern of development, pp 1245–1267
Shahvali M, Amiri Ardakani M (2011) Research methodology for agricultural indigenous knowledge. Agricultural Research, Education, and Extension Organization, Tehran
Shiri S, Bijani M, Chaharsoughi Amin H, Noori H, Soleymanifard A (2011) Effectiveness evaluation of the axial plan of wheat from expert supervisors’ view in Ilam province. World Appl Sci J 14(11):1724–1729
Valizadeh N, Bijani M, Abbasi E (2016) Pro-environmental analysis of farmers’ participatory behavior toward conservation of surface water resources in southern sector of Urmia Lake’s catchment area. Iran Agric Ext Educ J 11(2):183–201. In Persian
Valizadeh N, Bijani M, Abbasi E (2018a) Farmers’ active participation in water conservation: insights from a survey among farmers in Southern Regions of West Azerbaijan Province, Iran. J Agric Sci Technol 20(5):895–910
Valizadeh N, Bijani M, Abbasi E, Ganguli S (2018b) The role of time perspective in predicting Iranian farmers’ participatory-based water conservation attitude and behavior. J Hum Behav Soc Environ 28:992
Weiss CH, Murphy-Graham E, Birkeland S (2005) An alternate route to policy influence: how evaluations affect D.A.R.E. Am J Eval 26(1):12–30
Yazdanpanah M, Hayati D, Hochrainer-Stigler S, Zamani GHH (2014) Understanding farmers’ intention and behavior regarding water conservation in the Middle-East and North Africa: a case study in Iran. J Environ Manag 135:63–72
Zamani GHH (2016) Human liability theory: ethical approach towards agriculture and environment. Iran Agric Ext Educ J 12(1):149–163
Zanoli R, Krell R (1999) Research methodologies in organic farming. Proceedings. REU technical series. FAO, Rome
Download references
Author information
Authors and affiliations.
Department of Agricultural Extension and Education, School of Agriculture, Shiraz University, Shiraz, Iran
Naser Valizadeh Ph.D. Student
Department of Agricultural Extension and Education, College of Agriculture, Tarbiat Modares University (TMU), Tehran, Iran
Masoud Bijani Assistant Professor
You can also search for this author in PubMed Google Scholar
Corresponding author
Correspondence to Masoud Bijani Assistant Professor .
Editor information
Editors and affiliations.
European School of Sustainability Science and Research, Hamburg University of Applied Sciences, Hamburg, Germany
Walter Leal Filho
Center for Neuroscience and Cell Biology, Institute for Interdisciplinary Research, University of Coimbra, Coimbra, Portugal
Anabela Marisa Azul
Faculty of Engineering and Architecture, The University of Passo Fundo, Passo Fundo, Brazil
Luciana Brandli
Istinye University, Istanbul, Turkey
Pinar Gökçin Özuyar
International Centre for Thriving, University of Chester, Chester, UK
Rights and permissions
Reprints and permissions
Copyright information
© 2020 Springer Nature Switzerland AG
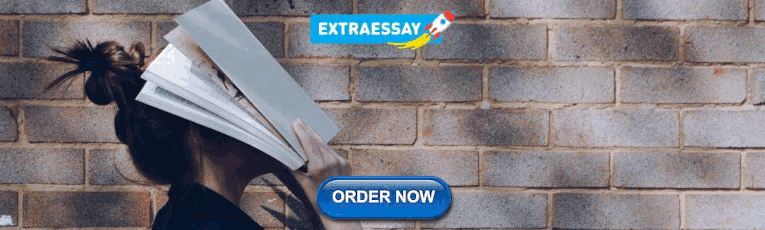
About this entry
Cite this entry.
Valizadeh, N., Bijani, M. (2020). Agricultural Research: Applications and Future Orientations. In: Leal Filho, W., Azul, A.M., Brandli, L., Özuyar, P.G., Wall, T. (eds) Zero Hunger. Encyclopedia of the UN Sustainable Development Goals. Springer, Cham. https://doi.org/10.1007/978-3-319-95675-6_5
Download citation
DOI : https://doi.org/10.1007/978-3-319-95675-6_5
Published : 04 June 2020
Publisher Name : Springer, Cham
Print ISBN : 978-3-319-95674-9
Online ISBN : 978-3-319-95675-6
eBook Packages : Earth and Environmental Science Reference Module Physical and Materials Science Reference Module Earth and Environmental Sciences
Share this entry
Anyone you share the following link with will be able to read this content:
Sorry, a shareable link is not currently available for this article.
Provided by the Springer Nature SharedIt content-sharing initiative
- Publish with us
Policies and ethics
- Find a journal
- Track your research
- Search Menu
- Browse content in Arts and Humanities
- Browse content in Archaeology
- Anglo-Saxon and Medieval Archaeology
- Archaeological Methodology and Techniques
- Archaeology by Region
- Archaeology of Religion
- Archaeology of Trade and Exchange
- Biblical Archaeology
- Contemporary and Public Archaeology
- Environmental Archaeology
- Historical Archaeology
- History and Theory of Archaeology
- Industrial Archaeology
- Landscape Archaeology
- Mortuary Archaeology
- Prehistoric Archaeology
- Underwater Archaeology
- Urban Archaeology
- Zooarchaeology
- Browse content in Architecture
- Architectural Structure and Design
- History of Architecture
- Residential and Domestic Buildings
- Theory of Architecture
- Browse content in Art
- Art Subjects and Themes
- History of Art
- Industrial and Commercial Art
- Theory of Art
- Biographical Studies
- Byzantine Studies
- Browse content in Classical Studies
- Classical History
- Classical Philosophy
- Classical Mythology
- Classical Literature
- Classical Reception
- Classical Art and Architecture
- Classical Oratory and Rhetoric
- Greek and Roman Papyrology
- Greek and Roman Epigraphy
- Greek and Roman Law
- Greek and Roman Archaeology
- Late Antiquity
- Religion in the Ancient World
- Digital Humanities
- Browse content in History
- Colonialism and Imperialism
- Diplomatic History
- Environmental History
- Genealogy, Heraldry, Names, and Honours
- Genocide and Ethnic Cleansing
- Historical Geography
- History by Period
- History of Emotions
- History of Agriculture
- History of Education
- History of Gender and Sexuality
- Industrial History
- Intellectual History
- International History
- Labour History
- Legal and Constitutional History
- Local and Family History
- Maritime History
- Military History
- National Liberation and Post-Colonialism
- Oral History
- Political History
- Public History
- Regional and National History
- Revolutions and Rebellions
- Slavery and Abolition of Slavery
- Social and Cultural History
- Theory, Methods, and Historiography
- Urban History
- World History
- Browse content in Language Teaching and Learning
- Language Learning (Specific Skills)
- Language Teaching Theory and Methods
- Browse content in Linguistics
- Applied Linguistics
- Cognitive Linguistics
- Computational Linguistics
- Forensic Linguistics
- Grammar, Syntax and Morphology
- Historical and Diachronic Linguistics
- History of English
- Language Evolution
- Language Reference
- Language Acquisition
- Language Variation
- Language Families
- Lexicography
- Linguistic Anthropology
- Linguistic Theories
- Linguistic Typology
- Phonetics and Phonology
- Psycholinguistics
- Sociolinguistics
- Translation and Interpretation
- Writing Systems
- Browse content in Literature
- Bibliography
- Children's Literature Studies
- Literary Studies (Romanticism)
- Literary Studies (American)
- Literary Studies (Asian)
- Literary Studies (European)
- Literary Studies (Eco-criticism)
- Literary Studies (Modernism)
- Literary Studies - World
- Literary Studies (1500 to 1800)
- Literary Studies (19th Century)
- Literary Studies (20th Century onwards)
- Literary Studies (African American Literature)
- Literary Studies (British and Irish)
- Literary Studies (Early and Medieval)
- Literary Studies (Fiction, Novelists, and Prose Writers)
- Literary Studies (Gender Studies)
- Literary Studies (Graphic Novels)
- Literary Studies (History of the Book)
- Literary Studies (Plays and Playwrights)
- Literary Studies (Poetry and Poets)
- Literary Studies (Postcolonial Literature)
- Literary Studies (Queer Studies)
- Literary Studies (Science Fiction)
- Literary Studies (Travel Literature)
- Literary Studies (War Literature)
- Literary Studies (Women's Writing)
- Literary Theory and Cultural Studies
- Mythology and Folklore
- Shakespeare Studies and Criticism
- Browse content in Media Studies
- Browse content in Music
- Applied Music
- Dance and Music
- Ethics in Music
- Ethnomusicology
- Gender and Sexuality in Music
- Medicine and Music
- Music Cultures
- Music and Media
- Music and Religion
- Music and Culture
- Music Education and Pedagogy
- Music Theory and Analysis
- Musical Scores, Lyrics, and Libretti
- Musical Structures, Styles, and Techniques
- Musicology and Music History
- Performance Practice and Studies
- Race and Ethnicity in Music
- Sound Studies
- Browse content in Performing Arts
- Browse content in Philosophy
- Aesthetics and Philosophy of Art
- Epistemology
- Feminist Philosophy
- History of Western Philosophy
- Metaphysics
- Moral Philosophy
- Non-Western Philosophy
- Philosophy of Language
- Philosophy of Mind
- Philosophy of Perception
- Philosophy of Science
- Philosophy of Action
- Philosophy of Law
- Philosophy of Religion
- Philosophy of Mathematics and Logic
- Practical Ethics
- Social and Political Philosophy
- Browse content in Religion
- Biblical Studies
- Christianity
- East Asian Religions
- History of Religion
- Judaism and Jewish Studies
- Qumran Studies
- Religion and Education
- Religion and Health
- Religion and Politics
- Religion and Science
- Religion and Law
- Religion and Art, Literature, and Music
- Religious Studies
- Browse content in Society and Culture
- Cookery, Food, and Drink
- Cultural Studies
- Customs and Traditions
- Ethical Issues and Debates
- Hobbies, Games, Arts and Crafts
- Lifestyle, Home, and Garden
- Natural world, Country Life, and Pets
- Popular Beliefs and Controversial Knowledge
- Sports and Outdoor Recreation
- Technology and Society
- Travel and Holiday
- Visual Culture
- Browse content in Law
- Arbitration
- Browse content in Company and Commercial Law
- Commercial Law
- Company Law
- Browse content in Comparative Law
- Systems of Law
- Competition Law
- Browse content in Constitutional and Administrative Law
- Government Powers
- Judicial Review
- Local Government Law
- Military and Defence Law
- Parliamentary and Legislative Practice
- Construction Law
- Contract Law
- Browse content in Criminal Law
- Criminal Procedure
- Criminal Evidence Law
- Sentencing and Punishment
- Employment and Labour Law
- Environment and Energy Law
- Browse content in Financial Law
- Banking Law
- Insolvency Law
- History of Law
- Human Rights and Immigration
- Intellectual Property Law
- Browse content in International Law
- Private International Law and Conflict of Laws
- Public International Law
- IT and Communications Law
- Jurisprudence and Philosophy of Law
- Law and Politics
- Law and Society
- Browse content in Legal System and Practice
- Courts and Procedure
- Legal Skills and Practice
- Primary Sources of Law
- Regulation of Legal Profession
- Medical and Healthcare Law
- Browse content in Policing
- Criminal Investigation and Detection
- Police and Security Services
- Police Procedure and Law
- Police Regional Planning
- Browse content in Property Law
- Personal Property Law
- Study and Revision
- Terrorism and National Security Law
- Browse content in Trusts Law
- Wills and Probate or Succession
- Browse content in Medicine and Health
- Browse content in Allied Health Professions
- Arts Therapies
- Clinical Science
- Dietetics and Nutrition
- Occupational Therapy
- Operating Department Practice
- Physiotherapy
- Radiography
- Speech and Language Therapy
- Browse content in Anaesthetics
- General Anaesthesia
- Neuroanaesthesia
- Clinical Neuroscience
- Browse content in Clinical Medicine
- Acute Medicine
- Cardiovascular Medicine
- Clinical Genetics
- Clinical Pharmacology and Therapeutics
- Dermatology
- Endocrinology and Diabetes
- Gastroenterology
- Genito-urinary Medicine
- Geriatric Medicine
- Infectious Diseases
- Medical Toxicology
- Medical Oncology
- Pain Medicine
- Palliative Medicine
- Rehabilitation Medicine
- Respiratory Medicine and Pulmonology
- Rheumatology
- Sleep Medicine
- Sports and Exercise Medicine
- Community Medical Services
- Critical Care
- Emergency Medicine
- Forensic Medicine
- Haematology
- History of Medicine
- Browse content in Medical Skills
- Clinical Skills
- Communication Skills
- Nursing Skills
- Surgical Skills
- Browse content in Medical Dentistry
- Oral and Maxillofacial Surgery
- Paediatric Dentistry
- Restorative Dentistry and Orthodontics
- Surgical Dentistry
- Medical Ethics
- Medical Statistics and Methodology
- Browse content in Neurology
- Clinical Neurophysiology
- Neuropathology
- Nursing Studies
- Browse content in Obstetrics and Gynaecology
- Gynaecology
- Occupational Medicine
- Ophthalmology
- Otolaryngology (ENT)
- Browse content in Paediatrics
- Neonatology
- Browse content in Pathology
- Chemical Pathology
- Clinical Cytogenetics and Molecular Genetics
- Histopathology
- Medical Microbiology and Virology
- Patient Education and Information
- Browse content in Pharmacology
- Psychopharmacology
- Browse content in Popular Health
- Caring for Others
- Complementary and Alternative Medicine
- Self-help and Personal Development
- Browse content in Preclinical Medicine
- Cell Biology
- Molecular Biology and Genetics
- Reproduction, Growth and Development
- Primary Care
- Professional Development in Medicine
- Browse content in Psychiatry
- Addiction Medicine
- Child and Adolescent Psychiatry
- Forensic Psychiatry
- Learning Disabilities
- Old Age Psychiatry
- Psychotherapy
- Browse content in Public Health and Epidemiology
- Epidemiology
- Public Health
- Browse content in Radiology
- Clinical Radiology
- Interventional Radiology
- Nuclear Medicine
- Radiation Oncology
- Reproductive Medicine
- Browse content in Surgery
- Cardiothoracic Surgery
- Gastro-intestinal and Colorectal Surgery
- General Surgery
- Neurosurgery
- Paediatric Surgery
- Peri-operative Care
- Plastic and Reconstructive Surgery
- Surgical Oncology
- Transplant Surgery
- Trauma and Orthopaedic Surgery
- Vascular Surgery
- Browse content in Science and Mathematics
- Browse content in Biological Sciences
- Aquatic Biology
- Biochemistry
- Bioinformatics and Computational Biology
- Developmental Biology
- Ecology and Conservation
- Evolutionary Biology
- Genetics and Genomics
- Microbiology
- Molecular and Cell Biology
- Natural History
- Plant Sciences and Forestry
- Research Methods in Life Sciences
- Structural Biology
- Systems Biology
- Zoology and Animal Sciences
- Browse content in Chemistry
- Analytical Chemistry
- Computational Chemistry
- Crystallography
- Environmental Chemistry
- Industrial Chemistry
- Inorganic Chemistry
- Materials Chemistry
- Medicinal Chemistry
- Mineralogy and Gems
- Organic Chemistry
- Physical Chemistry
- Polymer Chemistry
- Study and Communication Skills in Chemistry
- Theoretical Chemistry
- Browse content in Computer Science
- Artificial Intelligence
- Computer Architecture and Logic Design
- Game Studies
- Human-Computer Interaction
- Mathematical Theory of Computation
- Programming Languages
- Software Engineering
- Systems Analysis and Design
- Virtual Reality
- Browse content in Computing
- Business Applications
- Computer Security
- Computer Games
- Computer Networking and Communications
- Digital Lifestyle
- Graphical and Digital Media Applications
- Operating Systems
- Browse content in Earth Sciences and Geography
- Atmospheric Sciences
- Environmental Geography
- Geology and the Lithosphere
- Maps and Map-making
- Meteorology and Climatology
- Oceanography and Hydrology
- Palaeontology
- Physical Geography and Topography
- Regional Geography
- Soil Science
- Urban Geography
- Browse content in Engineering and Technology
- Agriculture and Farming
- Biological Engineering
- Civil Engineering, Surveying, and Building
- Electronics and Communications Engineering
- Energy Technology
- Engineering (General)
- Environmental Science, Engineering, and Technology
- History of Engineering and Technology
- Mechanical Engineering and Materials
- Technology of Industrial Chemistry
- Transport Technology and Trades
- Browse content in Environmental Science
- Applied Ecology (Environmental Science)
- Conservation of the Environment (Environmental Science)
- Environmental Sustainability
- Environmentalist Thought and Ideology (Environmental Science)
- Management of Land and Natural Resources (Environmental Science)
- Natural Disasters (Environmental Science)
- Nuclear Issues (Environmental Science)
- Pollution and Threats to the Environment (Environmental Science)
- Social Impact of Environmental Issues (Environmental Science)
- History of Science and Technology
- Browse content in Materials Science
- Ceramics and Glasses
- Composite Materials
- Metals, Alloying, and Corrosion
- Nanotechnology
- Browse content in Mathematics
- Applied Mathematics
- Biomathematics and Statistics
- History of Mathematics
- Mathematical Education
- Mathematical Finance
- Mathematical Analysis
- Numerical and Computational Mathematics
- Probability and Statistics
- Pure Mathematics
- Browse content in Neuroscience
- Cognition and Behavioural Neuroscience
- Development of the Nervous System
- Disorders of the Nervous System
- History of Neuroscience
- Invertebrate Neurobiology
- Molecular and Cellular Systems
- Neuroendocrinology and Autonomic Nervous System
- Neuroscientific Techniques
- Sensory and Motor Systems
- Browse content in Physics
- Astronomy and Astrophysics
- Atomic, Molecular, and Optical Physics
- Biological and Medical Physics
- Classical Mechanics
- Computational Physics
- Condensed Matter Physics
- Electromagnetism, Optics, and Acoustics
- History of Physics
- Mathematical and Statistical Physics
- Measurement Science
- Nuclear Physics
- Particles and Fields
- Plasma Physics
- Quantum Physics
- Relativity and Gravitation
- Semiconductor and Mesoscopic Physics
- Browse content in Psychology
- Affective Sciences
- Clinical Psychology
- Cognitive Psychology
- Cognitive Neuroscience
- Criminal and Forensic Psychology
- Developmental Psychology
- Educational Psychology
- Evolutionary Psychology
- Health Psychology
- History and Systems in Psychology
- Music Psychology
- Neuropsychology
- Organizational Psychology
- Psychological Assessment and Testing
- Psychology of Human-Technology Interaction
- Psychology Professional Development and Training
- Research Methods in Psychology
- Social Psychology
- Browse content in Social Sciences
- Browse content in Anthropology
- Anthropology of Religion
- Human Evolution
- Medical Anthropology
- Physical Anthropology
- Regional Anthropology
- Social and Cultural Anthropology
- Theory and Practice of Anthropology
- Browse content in Business and Management
- Business Ethics
- Business Strategy
- Business History
- Business and Technology
- Business and Government
- Business and the Environment
- Comparative Management
- Corporate Governance
- Corporate Social Responsibility
- Entrepreneurship
- Health Management
- Human Resource Management
- Industrial and Employment Relations
- Industry Studies
- Information and Communication Technologies
- International Business
- Knowledge Management
- Management and Management Techniques
- Operations Management
- Organizational Theory and Behaviour
- Pensions and Pension Management
- Public and Nonprofit Management
- Strategic Management
- Supply Chain Management
- Browse content in Criminology and Criminal Justice
- Criminal Justice
- Criminology
- Forms of Crime
- International and Comparative Criminology
- Youth Violence and Juvenile Justice
- Development Studies
- Browse content in Economics
- Agricultural, Environmental, and Natural Resource Economics
- Asian Economics
- Behavioural Finance
- Behavioural Economics and Neuroeconomics
- Econometrics and Mathematical Economics
- Economic History
- Economic Systems
- Economic Methodology
- Economic Development and Growth
- Financial Markets
- Financial Institutions and Services
- General Economics and Teaching
- Health, Education, and Welfare
- History of Economic Thought
- International Economics
- Labour and Demographic Economics
- Law and Economics
- Macroeconomics and Monetary Economics
- Microeconomics
- Public Economics
- Urban, Rural, and Regional Economics
- Welfare Economics
- Browse content in Education
- Adult Education and Continuous Learning
- Care and Counselling of Students
- Early Childhood and Elementary Education
- Educational Equipment and Technology
- Educational Strategies and Policy
- Higher and Further Education
- Organization and Management of Education
- Philosophy and Theory of Education
- Schools Studies
- Secondary Education
- Teaching of a Specific Subject
- Teaching of Specific Groups and Special Educational Needs
- Teaching Skills and Techniques
- Browse content in Environment
- Applied Ecology (Social Science)
- Climate Change
- Conservation of the Environment (Social Science)
- Environmentalist Thought and Ideology (Social Science)
- Natural Disasters (Environment)
- Social Impact of Environmental Issues (Social Science)
- Browse content in Human Geography
- Cultural Geography
- Economic Geography
- Political Geography
- Browse content in Interdisciplinary Studies
- Communication Studies
- Museums, Libraries, and Information Sciences
- Browse content in Politics
- African Politics
- Asian Politics
- Chinese Politics
- Comparative Politics
- Conflict Politics
- Elections and Electoral Studies
- Environmental Politics
- European Union
- Foreign Policy
- Gender and Politics
- Human Rights and Politics
- Indian Politics
- International Relations
- International Organization (Politics)
- International Political Economy
- Irish Politics
- Latin American Politics
- Middle Eastern Politics
- Political Behaviour
- Political Economy
- Political Institutions
- Political Methodology
- Political Communication
- Political Philosophy
- Political Sociology
- Political Theory
- Politics and Law
- Public Policy
- Public Administration
- Quantitative Political Methodology
- Regional Political Studies
- Russian Politics
- Security Studies
- State and Local Government
- UK Politics
- US Politics
- Browse content in Regional and Area Studies
- African Studies
- Asian Studies
- East Asian Studies
- Japanese Studies
- Latin American Studies
- Middle Eastern Studies
- Native American Studies
- Scottish Studies
- Browse content in Research and Information
- Research Methods
- Browse content in Social Work
- Addictions and Substance Misuse
- Adoption and Fostering
- Care of the Elderly
- Child and Adolescent Social Work
- Couple and Family Social Work
- Developmental and Physical Disabilities Social Work
- Direct Practice and Clinical Social Work
- Emergency Services
- Human Behaviour and the Social Environment
- International and Global Issues in Social Work
- Mental and Behavioural Health
- Social Justice and Human Rights
- Social Policy and Advocacy
- Social Work and Crime and Justice
- Social Work Macro Practice
- Social Work Practice Settings
- Social Work Research and Evidence-based Practice
- Welfare and Benefit Systems
- Browse content in Sociology
- Childhood Studies
- Community Development
- Comparative and Historical Sociology
- Economic Sociology
- Gender and Sexuality
- Gerontology and Ageing
- Health, Illness, and Medicine
- Marriage and the Family
- Migration Studies
- Occupations, Professions, and Work
- Organizations
- Population and Demography
- Race and Ethnicity
- Social Theory
- Social Movements and Social Change
- Social Research and Statistics
- Social Stratification, Inequality, and Mobility
- Sociology of Religion
- Sociology of Education
- Sport and Leisure
- Urban and Rural Studies
- Browse content in Warfare and Defence
- Defence Strategy, Planning, and Research
- Land Forces and Warfare
- Military Administration
- Military Life and Institutions
- Naval Forces and Warfare
- Other Warfare and Defence Issues
- Peace Studies and Conflict Resolution
- Weapons and Equipment

- < Previous chapter
- Next chapter >
5 5 Experimental design: agricultural field experiments and clinical trials
- Published: August 2011
- Cite Icon Cite
- Permissions Icon Permissions
This chapter discusses the statistical approach to experimental design, with an emphasis on the core concepts of randomization and blocking. It focuses initially on agricultural field experiments, but also describes the basic elements of a type of medical research investigation known as a clinical trial.
Signed in as
Institutional accounts.
- GoogleCrawler [DO NOT DELETE]
- Google Scholar Indexing
Personal account
- Sign in with email/username & password
- Get email alerts
- Save searches
- Purchase content
- Activate your purchase/trial code
Institutional access
- Sign in with a library card Sign in with username/password Recommend to your librarian
- Institutional account management
- Get help with access
Access to content on Oxford Academic is often provided through institutional subscriptions and purchases. If you are a member of an institution with an active account, you may be able to access content in one of the following ways:
IP based access
Typically, access is provided across an institutional network to a range of IP addresses. This authentication occurs automatically, and it is not possible to sign out of an IP authenticated account.
Sign in through your institution
Choose this option to get remote access when outside your institution. Shibboleth/Open Athens technology is used to provide single sign-on between your institution’s website and Oxford Academic.
- Click Sign in through your institution.
- Select your institution from the list provided, which will take you to your institution's website to sign in.
- When on the institution site, please use the credentials provided by your institution. Do not use an Oxford Academic personal account.
- Following successful sign in, you will be returned to Oxford Academic.
If your institution is not listed or you cannot sign in to your institution’s website, please contact your librarian or administrator.
Sign in with a library card
Enter your library card number to sign in. If you cannot sign in, please contact your librarian.
Society Members
Society member access to a journal is achieved in one of the following ways:
Sign in through society site
Many societies offer single sign-on between the society website and Oxford Academic. If you see ‘Sign in through society site’ in the sign in pane within a journal:
- Click Sign in through society site.
- When on the society site, please use the credentials provided by that society. Do not use an Oxford Academic personal account.
If you do not have a society account or have forgotten your username or password, please contact your society.
Sign in using a personal account
Some societies use Oxford Academic personal accounts to provide access to their members. See below.
A personal account can be used to get email alerts, save searches, purchase content, and activate subscriptions.
Some societies use Oxford Academic personal accounts to provide access to their members.
Viewing your signed in accounts
Click the account icon in the top right to:
- View your signed in personal account and access account management features.
- View the institutional accounts that are providing access.
Signed in but can't access content
Oxford Academic is home to a wide variety of products. The institutional subscription may not cover the content that you are trying to access. If you believe you should have access to that content, please contact your librarian.
For librarians and administrators, your personal account also provides access to institutional account management. Here you will find options to view and activate subscriptions, manage institutional settings and access options, access usage statistics, and more.
Our books are available by subscription or purchase to libraries and institutions.
- About Oxford Academic
- Publish journals with us
- University press partners
- What we publish
- New features
- Open access
- Rights and permissions
- Accessibility
- Advertising
- Media enquiries
- Oxford University Press
- Oxford Languages
- University of Oxford
Oxford University Press is a department of the University of Oxford. It furthers the University's objective of excellence in research, scholarship, and education by publishing worldwide
- Copyright © 2024 Oxford University Press
- Cookie settings
- Cookie policy
- Privacy policy
- Legal notice
This Feature Is Available To Subscribers Only
Sign In or Create an Account
This PDF is available to Subscribers Only
For full access to this pdf, sign in to an existing account, or purchase an annual subscription.
TEXTBOOK OF AGRICULTURAL STATISTICS
14 design of experiments, 14.1 introduction.
Design of Experiments is an integral component of agricultural research. A scientifically designed experiment is a valuable tool in advancement in gaining new knowledge and technology development. “It is the effective use of the tools of statistical design of experiments that paved the way for the green revolution” – these words of the father of green revolution in India, Dr. M.S. Swaminathan, itself shows how important is Design and analysis of experiments as well as statistical science is for agricultural experiments. A carefully designed experiment is able to answer all the queries of a researcher with accuracy and reliability with efficient use of available resources of the experimenters. Thus, for successful experimentation, it is highly desirable that scientists and researchers of scientific disciplines, including agricultural sciences, understand the basic principles of designing an experiment and analysis of resultant data from the completed experiment. It may be emphasized that a researcher should always consult a statistician before, during and after experimentation, if he is not convinced enough about using a design for his experiment or an analysis technique for his data.
Any scientific investigation involves formulation of certain assertions (or hypotheses) whose validity is examined through the data generated from an experiment conducted for the purpose. The term ’ experiment ’is defined as the systematic procedure carried out under controlled conditions in order to discover an unknown effect, to test or establish a hypothesis, or to illustrate a known effect.
Experiments can be designed in many different ways to collect information. Design of experiments (DOE) is a systematic method to determine the relationship between factors affecting a process and the output of that process. In other words, it is used to find cause-and-effect relationships.
DOE is a structured approach for conducting experiments. Mainly aims at
Reliability
Replicability
14.2 A simple example

Figure 14.1: Poultry manure, cow dung and coirpith compost
You have decided to conduct an experiment. Consider you as a layman with no knowledge in design of experiments. So, you have selected 3 potted plants for the experiment. 3 organic manures are applied to the potted plants.

Figure 14.2: Treatments given to potted plants as shown

Figure 14.3: Yield observed from the plants
So, based on your experiment, can you say the poultry manure is the best?
What if somebody repeats this experiment somewhere and the results are different?
What about the variance due to experimental error?
What if the experimenter wants to show that poultry manure is the best? So he has allotted healthy plant to poultry manure.
Can an experiment like this has validity?
Can we make a conclusion from this experiment?
Answer to this question gives the importance of proper designing of experiments. Nobody in the scientific fraternity is going to accept your above experiment. Your experiment has validity only if its validity is proved by statistical theories. All these issues can be well taken care off by proper designing of experiments. After discussing the basic principles of design, it will be shown, how the above experiment looks like after proper designing.
Design of experiment means how to design an experiment. In the sense that how the observations or measurements should be obtained to answer a query in a valid, efficient and economical way. The designing of experiment and the analysis of obtained data are inseparable. If the experiment is designed properly keeping in mind the question, then the data generated is valid and proper analysis of data provides the valid statistical inferences. If the experiment is not well designed, the validity of the statistical inferences is questionable and may be invalid.
14.3 Importance of DoE
Reduce, control and provides an estimate of the experimental Error
Gives a structured approach
It reduce cost of experiment with considerable reliability
Produces statistically valid results
Allows to accommodate changes
Reduce complexity
Improves accountability
14.4 Characteristics of a good design
Provides unbiased estimates of the factor effects and associated uncertainties
Enables the experimenter to detect important differences
Includes the plan for analysis and reporting of the results
Gives results that are easy to interpret
Permits conclusions that have wide validity
Minimal resource usage
Is as simple as possible
Statistical design of experiments refers to the process of planning the experiment so that appropriate data will be collected and analyzed by statistical methods, resulting in valid and objective conclusions. The statistical approach to experimental design is necessary if we wish to draw meaningful conclusions from the data. When the problem involves data that are subject to experimental errors, statistical methods are the only objective approach to analysis.
Creation of controlled conditions is the main characteristic feature of experimentation and DOE specifies the nature of control over the operations in experiments. Proper designing ensures that the assumptions required for appropriate interpretations of data are satisfied thus increasing the accuracy and sensitivity of results.
There are two aspects to any experimental problem: the design of the experiment and the statistical analysis of the data. These two subjects are closely related because the method of analysis depends directly on the design employed.

Figure 14.4: DoE and Statistical analysis
14.5 Brief history
The statistical principles underlying design of experiments were pioneered by R. A. Fisher in the 1920s and 1930s at Rothamsted Experimental Station, an agricultural research station around fourty kilometres north of London. Fisher had shown the way on how to draw valid conclusions from field experiments where nuisance variables such as temperature, soil conditions, and rainfall are present. He introduced the concept of analysis of variance (ANOVA) for partitioning the variation present in data (a) due to attributable factors, and (b) due to chance factors. The methodologies he and his colleague Frank Yates developed are now widely used. Their methodologies have a profound impact on agricultural sciences research.
Though the experimental design was initially introduced in an agricultural context, the method has been applied successfully in the industry since the 1940s. George Box and his co-workers developed experimental design procedures for optimizing chemical processes, particularly response surface designs for chemical and process industries.
Recently, experimental designs are also being used in clinical trials. This evolved in the 1960s when medical advances were previously based on unreliable data. For example, doctors used to examine a few patients and publish papers based on such data. The biases resulting from these kinds of studies became known. This led to a move toward making the randomized double-blind clinical trial the standard for approval of any new product, medical device, or procedure. The scientific application of the valid designing and analysis following proper statistical methods became very important in clinical trials.
More recently the experimental design techniques have started gaining popularity in the area of computer-aided design and engineering using computer/simulation models including applications in manufacturing industries.
14.6 Some terms involved
14.6.1 treatments.
The term treatments is used to denote the different objects , methods or processes among which comparison is made. For example, if an experimenter wants to identify which among the objects/methods/process is the best based on an experiment; then this objects/methods/process is called the treatment. More clearly anything that you are about to compare in an experiment is known as the treatment.
Some examples of treatments are different kinds of fertilizer in agronomic experiments, different irrigation methods or levels of irrigation, different fungicides in pest management experiments , doses of different drugs or chemicals in laboratory experiments, different varieties of crops, different pesticides, grazing systems for animals, different tree species in agro-forestry experiments, different concentrations of a solute in chemical experiments etc.
14.6.2 Control
A control treatment is a standard treatment that is used as a baseline or basis of comparison for the other treatments. This control treatment might be the treatment which is currently in use, or it might be a no treatment at all. For example, a study of new pesticides could use a standard pesticide as a control treatment, or an experiment involving fertilizers may have one treatment as no fertilizers at all. In clinical trials, a control treatment is generally a placebo.
14.6.3 Experimental units
Experimental units are the subjects or objects on which the treatments are applied. For example, plots of land receiving fertilizer, groups of animals receiving different feeds, or batches of chemicals receiving different temperatures, pots in glasshouse experiments, Petri dishes or tissues to culture bacteria or micro-organisms in laboratory experiments, etc
14.6.4 Response
Responses are measurable outcomes, which are observed after applying a treatment to an experimental unit. Alternatively, the response is what we measure to find out what happened in the experiment. In an experiment, there may be more than one response. Some examples of responses are grain yield or straw yield, nitrogen content in plants or biomass of plants, quality parameters of the produce, percentage of plants infested by disease, weight gain by animals, etc.
14.6.5 Factors
Factors are the variables whose influence on a response variable is being studied in the experiment. If only one factor is being studied in an experiment then such an experiment is called a single factor experiment. If more than one factor is being studied simultaneously in an experiment, then such an experiment is called multi-factor or factorial experiment. The term factor is commonly used in the case of factorial experiments. For example, temperature and concentration of chemicals in a chemical experiment are two factors, Nitrogen, Phosphorus and Potassium fertilizers are three factors in an agronomic experiment.Dose and time of application of a chemical formulation are two factors in a laboratory experiment.
14.6.6 Factor levels
The term factor levels or a simply levels is used to denote the values or settings that a factor takes in a factorial experiment. For example, doses of a nitrogenous fertilizer as 0 kg/ha, 30 kg/ ha, 80 kg/ha are three levels of the factor fertilizer . 10%, 20%, 30%, 40% concentration of a solute in a solution are four levels of the factor solute in a laboratory experiment. Presence of polythene sheet on the surface of soil or its absence could be two levels of factor management practice in water management study.
14.6.7 Observational Unit
An observational unit is a unit on which the response variables are measured. Observational units are often the same as experimental units, but this may not be true always. The mistake of confusing observational unit with experimental unit leads to pseudo-replication as discussed in a paper by ( Hurlbert 1984 ) . Consider an experiment to investigate the effects of ultraviolet (UV) levels on the growth of smolt. The experiment is conducted in two tanks where one tank receives high levels of UV light and the other tank receives no UV light. Fish are placed in each tank and at the end of the experiment growths of the individual fish are measured. In this experiment, the tanks are the experimental units but the observational units are the smolts. The treatments, presence and absence of UV light, are applied to the tanks and not to individual fish but a whole group of fish are simultaneously exposed to the UV radiation. Here any tank effect is completely confounded with the treatment effect and cannot be separated. Another example is that inorganic fertilizers are applied to plots in a field containing some plants. At the time of harvest, all the plants in the plot are not harvested. Only a sample of plants is harvested. In this case once again the plot is the experimental unit to which fertilizers are applied but the observational units are the plants sampled.
14.7 Experimental error
To explain experimental error consider the example given by ( Gomez and Gomez 1984 ) . Consider a plant breeder who wishes to compare the yield of a new rice variety A to that of a standard variety B of known and tested properties. He lays out two plots of equal size, side by side, and sows one to variety A and the other to variety B. Grain yield for each plot is then measured and the variety with higher yield is judged as better. Despite the simplicity and common-sense appeal of the procedure just outlined, it has one important flaw. It presumes that any difference between the yields of the two plots is caused by the varieties and nothing else. This certainly is not true. Even if the same variety were planted on both plots, the yield would differ. Other factors, such as soil fertility, moisture, and damage by insects, diseases, and birds also affect rice yields. Because these other factors affect yields, a satisfactory evaluation of the two varieties must involve a procedure that can separate varietal difference from other sources of variation. That is, the plant breeder must be able to design an experiment that allows him to decide whether the difference observed is caused by varietal difference or by other factors.
The logic behind the decision is simple. Two rice varieties planted in two adjacent plots will be considered different in their yielding ability only if the observed yield difference is larger than that expected, if both plots were planted to the same variety.
Hence, the researcher needs to know not only the yield difference between plots planted to different varieties, but also the yield difference between plots planted to the same variety. The difference among experimental plots treated alike is called experimental error . This error is the primary basis for deciding whether an observed difference is real or just due to chance. Clearly, every experiment must be designed to have a measure of the experimental error.
Response from all experimental units receiving the same treatment may not be same even under similar conditions. These variations in responses may be due to various reasons. Other factors like heterogeneity of soil, climatic factors and genetic differences, etc also may cause variations (known as extraneous factors).
Definition: The variations in response caused by extraneous factors are known as experimental error .
Our aim of designing an experiment will be to minimize the experimental error.
14.8 Basic principles of design
There are three basic principles of designing an experiment namely randomization, replication and local control (blocking).
14.8.1 Randomization
Randomization means random assignment of conditions to study or treatments to the subjects or experimental units. The principle of randomization involves the allocation of treatment to experimental units at random to avoid any bias in the experiment resulting from the influence of some extraneous unknown factor that may affect the experiment.
In the development of analysis of variance (ANOVA), we assume that the errors are random and independent. In turn, the observations also become random through randomization.
The observations are independent and are identically distributed as normal variate is an important assumption in hypothesis testing problems involving test statistics F (Snedecor’s F) and t (Student’s t). This is the major purpose of randomization.
Randomization forms the basis of a valid experiment but replication is also needed for the validity of the experiment. If the randomization process is such that every experimental unit has an equal chance of receiving each treatment, it is called complete randomization .
Consider an example where suppose you want to randomly allot 3 treatments to 3 experimental units. How will you do this? It is very easy; just label all the units from 1 to 3. Make a lot of equal size labelling 1,2 and 3. Put these labels in a bowl pick it with eyes closed. Now if 1 comes; first treatment is alloted to 1st unit. This is a very simple technique of randomization. Random number tables or computer generated random numbers can also be used.

Figure 14.5: Taking a lot from a bowl is also a procees of randomization
14.8.2 Replication
In the replication principle, any treatment is repeated a number of times to obtain a valid and more reliable estimate than which is possible with one observation only. Replication provides an efficient way of increasing the precision of an experiment. The precision increases with the increase in the number of observations. Replication provides more observations when the same treatment is used, so it increases precision.
Replication enables the experimenter to obtain a valid estimate of the experimental error. Estimate of experimental error permits statistical inference; for example, performing tests of significance or obtaining confidence interval, etc. If there is no replication, then the researcher would not be able to estimate the experimental error. And as will be seen in the later Chapters, it is against this estimated experimental error the null hypotheses are tested.

Figure 14.6: Treatments alloted to four plots, replication of each treatment is 2
The results from the experiment is shown Figure 1.7. The yield in kg per plot is given in bracket.

Figure 14.7: The yield in kg per plot is given in bracket
In the experiment, the experimental error can be estimated as \(\frac{\left( 6 - 8 \right)^{2} + {(5 - 4)}^{2}}{2} = \frac{4 + 1}{2} = 2.5\) ; here the denominator 2 is the number of replications.
This can be also calculated as the square of difference of observation from corresponding treatment mean, here the mean of A is \(\frac{6 + 8}{2} = 7\) ; the mean of B is \(\frac{5 + 4}{2} = 4.5\) . The sum of the square of difference of each observation from treatment mean is taken as shown below \(\left( 6 - 7 \right)^{2} + \left( 8 - 7 \right)^{2} + \ \left( 5 - 4.5 \right)^{2} + \ {(4 - 4.5)}^{2} = 2.5\)
Thus, replication helps to estimate experimental error. Increasing the size of the experiment or increasing the replication also helps to increase the precision of estimating the pairwise differences among the treatment effects. . Replication provides an efficient way of increasing the precision of an experiment. The precision increases with the increase in the number of observations. Replication provides more observations when the same treatment is used, so it increases precision.
14.8.3 Local control (error control)
A good experiment incorporates all possible means of minimizing the experimental error; because ability to detect experimental error increases as the size of experimental error decreases. By putting experimental units that are as similar as possible together in the same group (commonly referred to as a block) and by assigning all treatments into each block separately and independently, variation among blocks can be measured and removed from experimental error. In field experiments where substantial variation within an experimental field can be expected, significant reduction in experimental error is usually achieved with the use of proper blocking.
The replication is used with local control to reduce the experimental error. For example, if the experimental units are divided into different groups such that they are homogeneous within the blocks, then the variation among the blocks is eliminated and ideally, the error component will contain the variation due to the treatments only. This will, in turn, increase the efficiency.
You have a field experiment with 4 treatments and 5 replications. Consider a field with fertility gradient from left to right as shown in figure 1.8.

Figure 14.8: A field with fertility gradient from left to right
Homogeneity can be achieved by dividing the field in to groups as shown in figure 1.9. Now each vertical strips can be considered as a block. Plots are formed with in each block, where each treatment is allotted randomly. Here randomization is performed with in blocks. You can see that in this example replication is equal to number of blocks, which is equal to 5. Randomization is achieved with in blocks. Local control is achieved by grouping treatments in homogeneous blocks, where fertilizer gradient is same. This is a typical example of Randomized Block Design (RBD), which will be discussed in chapter

Figure 14.9: Plots are grouped into blocks
14.9 Other methods of error control
14.9.1 border effect.
Plants which are in the outer areas or the borders of the plot will get the influence of the treatment that is applied in the adjacent plot, this may alter the response of the character of interest in these plants (for example yield of these plants may be higher), this phenomenon is called as border effect. For example, if in a plot a particular fertilizer is applied as treatment and in the adjacent one another fertilizer is applied, due to seepage plants in the boarder areas will have the influence of the fertilizer in the adjacent plot, this may affect the yield or some other attributes of the border plants. Usually while taking observations, these border plants are discarded.
14.9.2 Proper Plot Technique
It is essential that all other factors, which are not treatments should be maintained uniformly for all experimental units. For example, in field experiments, it is required that all other factors such as soil nutrients, solar energy, plant population, pest incidence, and an almost infinite number of other environmental factors are maintained uniformly for all plots in the experiment. This requirement is impossible to satisfy, however to ensure that variability among experimental plots is minimum, some important sources of variability are taken care off using a good plot technique. For field experiments with crops, some important sources of variability considered among plots treated alike are soil heterogeneity, competition effects, and mechanical errors.
14.9.3 Data Analysis
Proper choice of data analysis helps in controlling error, where blocking is not so effective. Covariance analysis is most commonly used for this purpose. By measuring one or more covariates- the characters whose functional relationships to the character of primary interest are known, the analysis of covariance (ANCOVA) can reduce the variability among experimental units by adjusting their values to a common value of the covariates.
For example, in an animal feeding trial, the initial weight of the animals usually differs. Using this initial weight as the covariate, final weight after the animals are subjected to various feeds (i.e., treatments) can be adjusted to the values that would have been attained had all experimental animals started with the same body weight. Or, in a rice field experiment where rats damaged some of the test plots, covariance analysis with rat damage as the covariate can adjust plot yields to the levels that they should have been with no rat damage in any plot.
186 Agriculture Essay Topics & Research Questions + Examples
Are you looking for the best agriculture topics to write about? You’re at the right place! StudyCorgi has prepared a list of important agriculture research topics. On this page, any student can find essay questions and project ideas on various agricultural issues, such as food safety, genetically engineered crops, and sustainable farming practices.
👨🌾 TOP 7 Agriculture Research Topics – 2024
🏆 best essay topics on agriculture, 🎓 most interesting agriculture topics for college students, 👍 good agriculture research topics & essay examples, 💡 cool agricultural research topics for high school students, ❓ research questions about agriculture, 🔎 current agriculture research paper topics, 📝 agriculture argumentative essay topics, 🗣️ agriculture topics for speech.
- Agriculture and Its Role in Economic Development
- Globalization Impact on Sustainable Agriculture
- Food Safety Issues in Modern Agriculture
- Agricultural Biotechnology and Its Pros and Cons
- Agriculture: Personal Field Visit
- Commercial Agriculture, Its Role and Definition
- Agricultural Influences on the Developing Civil Society
- In Support of Robotics Use in Agriculture Robotic technologies have vast potential to be used in the agricultural sector due to the multi-dimensional nature of their applications and the possibilities for ongoing improvement.
- The Impact of Pesticides’ Use on Agriculture Pesticides are mostly known for their adverse effects and, therefore, have a mostly negative connotation when discussed among general audiences.
- Agriculture and Food in Ancient Greece The paper states that agricultural practices and goods from Greece extended to neighboring countries in the Mediterranean as the dominance increased.
- Agriculture the Backbone of Ancient Egypt’s Economy In pre-industrial societies, agriculture was the backbone of most economies. This is true in ancient times and very much evident in ancient Egypt.
- Soil: The Essential Aspect of Agriculture Soil is an integral part of human life as it determines one’s quality of life. The health of the soil is reduced by erosion and degradation due to human activities.
- Hunting and Gathering Versus Agricultural Society The hunting and gathering society is considered the most equitable of all seven types, while the agricultural community gives rise to the development of civilization.
- Industry and Agriculture: Use of Technology Industry and agriculture are among the areas that have experienced a vast rise in effectiveness and performance quality due to the integration of new types of technology into them.
- Population Growth and Agriculture in the Future The current industrial agriculture needs to be advanced and developed in combination with sustainable agricultural practices.
- Agricultural Role in African Development Diao et al. attempt to determine the role of agriculture in overcoming the challenge of poverty in rural areas of Africa compared to alternative theories of economic growth.
- Improving Stress Resistance in Agricultural Crops The essay suggests that stress-resistant crops are needed to ensure yield stability under stress conditions and to minimize the environmental impacts of crop production.
- History of Agricultural Technology Development Agricultural technologies were majorly developed during the Medieval period to ensure sufficient product yields for growing populations around the world.
- Food and Agriculture of Ancient Greece The concepts of agriculture and cuisine both have a deep connection to Greek history, culture, development, and social trends.
- Agriculture: Application of Information Technology IT application in agriculture has contributed to food security in most modern communities. Farming has become easier than before as new inventions are made.
- Virtual Water Trade and Savings in Agriculture This essay discusses the savings associated with virtual water trade in agriculture and touches on the effects of a shift to local agricultural production on global water savings.
- Food Safety: A Policy Issue in Agriculture Today Food safety constitutes proper preparation, storage and preservation of all foods. Markets are increasingly calling for improvement in the quality and safety standards of food crops.
- Sustainable Agriculture Against Food Insecurity The paper argues sustainable agriculture is one way to reduce food insecurity without harming the planet because the number of resources is currently decreasing.
- The Neolithic Era: Architecture and Agriculture The improvements to agriculture, society, architecture, and culture made during the Neolithic period had an undeniable impact on aspects of the world.
- Agricultural Technology Implementation by Medieval Europeans and West Africans The paper examines how West Africans and Medieval Europeans were affected by their corresponding climates and why their methods were unique to their respective locations.
- Agriculture Development and Related Theories There are two main domestication models used to describe the development of agriculture: unconscious and conscious.
- Agricultural Traditions of Canadians In Canada there is a very good agricultural education, so young people can get higher education in agriculture and use it on their own farms.
- Sharecropping. History of Racial Agriculture Sharecropping became a variation of racialized agriculture, that which has negative impact on the capabilities of the black population to generate and pass down wealth.
- Climate Changes Impact on Agriculture and Livestock The project evaluates the influences of climate changes on agriculture and livestock in different areas in the Kingdom of Saudi Arabia.
- Agriculture in Honduras: Existing Challenges and Possible Solutions This paper tackles the issue of existing challenges and possible solutions to the problems of agriculture in Honduras.
- Repeasantization: Impact on Agriculture The repeasantization led to fundamental changes that created a new system of agriculture that is still relevant today.
- Colonialism and Economic Development of Africa Through Agriculture The colonial period is characterized by the exploitation of the agricultural sector in Africa to make a profit and provide Western countries with raw materials.
- The Big History of Civilizations – Origins of Agriculture: Video Analysis This paper aims to analyze the origins of agriculture – what was a foraging economy and way of life like, as well as compare foragers and farmers.
- Agriculture and Food Production in the Old Kingdom
- Agriculture and the Transition to the Market in Asia
- Agrarian Reform and Subsistence Agriculture in Russia
- Agriculture, Nutrition, and the Green Revolution in Bangladesh
- Agriculture Business and Management
- Agriculture, Horticulture, and Ancient Egypt
- Agriculture and Food Production in the Old Kingdom of Egypt
- Administrative and Transaction-Related Costs of Subsidising Agriculture
- Agriculture and Economic Growth in Argentina, 1913-84
- Agriculture and Economic Development in Brazil, 1960-1995
- Agriculture and Greenhouse Gas Cap-And-Trade
- Croatian Agriculture Towards World Market Liberalization
- Adapting Credit Risk Models to Agriculture
- Agriculture and European Union Enlargement
- Agriculture and Food Security in Pakistan
- Cash Flows and Financing in Texas Agriculture
- Current Problems With Indian Agriculture
- Agriculture and Its Drain on California
- Agriculture and the Economic Life of India
- Agriculture and Global Climate Stabilization
- Achieving Regional Growth Dynamics in African Agriculture
- Agriculture and Non-agricultural Liberalization in the Millennium Round
- Corporate Agriculture and Modern Times
- Agriculture and Rural Employment Agricultural in Bolivia
- Climatic Fluctuations and the DI¤Usion of Agriculture
- Agriculture Global Market Briefing
- Agriculture and the Industrial Revolution of the Late 1700s
- Agriculture and Animal Husbandry in Ecuador
- Biofuels, Agriculture, and Climate Change
- Aggregate Technical Efficiency and Water Use in U.S. Agriculture
- Market Revolution: Agriculture and Global Trade In the era of traders, the vast land area and rich natural resources created many economic opportunities. Most people lived in rural areas and were engaged in agriculture.
- Agriculture, Water, and Food Security in Tanzania This paper evaluates the strategies applicable to the development and further maintenance of agriculture, water, and food security in Tanzania.
- The Australian Agriculture Company’s Financial Analysis The Australian Agriculture Company shows a positive sign for investment due to its financial analysis indicating company resilience and strong prospects of growth.
- Governmental Price Control in Agricultural Sector The consequences of real-life governmental price control are the evolutionary nature of transformations in the agricultural sector.
- The Agriculture Industry’s Digital Transformation This study seeks to explore the dynamics of digital technology in agriculture over the past two decades, focusing on the perspectives and perceptions of the farmers.
- Aspects of Pesticide Use in Agriculture This paper investigates socio-environmental factors connected with pesticide use in agriculture and food production. It has a destructive impact on the environment
- Agriculture-Led Food Crops and Cash Crops in Tanzania This paper aims to explore the contributions of the agriculture sector in Tanzania to the country’s industrialization process by using recent data about its food and cash crops.
- Cuisine and Agriculture of Ancient Greece There are many reasons for modern students to investigate the development of cuisine and agriculture in Ancient Greece.
- Agriculture and Food Safety in the United States Agriculture in the United States has grown progressively centralized. The shortcomings in the 2018 U.S. farm legislation resulted in multiple challenges in the food system.
- Sustainable Agriculture and Future Perspectives Sustainable agriculture is essential to the earth’s environment. When farmers take care of their land and crops, they are taking care of environmental sustainability.
- Agricultural Adaptation to Changing Environments The paper discusses the impact of climate change on agriculture in Canada. This phenomenon is real and has affected the industry over at least the last three decades.
- Trade Peculiarities in Food and Agriculture Food trading is a peculiar area, as food is the basis for surviving the population. The one who controls food production and trading routes, also controls all populations.
- Multinational Agricultural Manufacturing Companies’ Standardization & Adaptation The most popular approaches that multinational companies use to serve their customers from various countries are standardization and adaptation.
- Impacts of Climate Change on Agriculture and Food This paper will examine four aspects of climate change: variation in the rainfall pattern, water levels, drought, temperature, and heatwaves.
- Canadian Laws Regarding Agricultural Sector The unions in Canada are the concept over which there has been an excessive dispute involving court proceedings and questioning the constitutional rights of citizens.
- Food Additives Use in Agriculture in the United States Food additives in agriculture become a debatable issue because their benefits do not always prevail over such shortages like health issues and environmental concerns.
- Radio-Frequency Identification in Healthcare and Agriculture Specifically, radio-frequency identification (RFID) has gained traction due to its ability to transmit data over distance.
- Mechanism of US Agricultural Market The fact that lower interest rates increased the number of potential customers for real estate in the 2000s shows that housing prices should have increased.
- A Biological Terror Attack in Agriculture The United States is highly vulnerable to terror attacks of biological nature in agriculture yet such an occurrence can cripple the economy.
- The Economics of Race, Agriculture and Environment This research paper is going to answer the question; do public policies reduce or enhance racial inequality in agricultural and environmental affairs?
- Impact of Bioterrorism on the U.S Agriculture System The paper describes that the term bioterrorism has several definitions depending upon the origin of the attack but in general terms, it refers to any form of terrorist attack.
- Impacts of Genetic Engineering of Agricultural Crops In present days the importance of genetic engineering grew due to the innovations in biotechnologies and Sciences.
- The Effects of Genetic Modification of Agricultural Products Discussion of the threat to the health of the global population of genetically modified food in the works of Such authors as Jane Brody and David Ehrenfeld.
- Climate Change and Its Potential Impact on Agriculture and Food Supply The global food supply chain has been greatly affected by the impact of global climate change. There are, however, benefits as well as drawbacks to crop production.
- Agriculture and Mayan Society Resilience The Yucatan peninsula had a vast landscape which was good for agriculture thus making agriculture to be the main economic base for the Mayans.
- Homeland Security in Agriculture and Health Sectors Lack of attention to the security and protection of the agricultural sector in the U.S. economy can create a serious threat to the health and safety of the population.
- Water Savings and Virtual Trade in Agriculture Water trade in agriculture is not a practice that is unique to the modern generation. The practice was common long before the emergence of the Egyptian Empire.
- Virtual Water Trade of Agricultural Products Virtual water trade is a concept associated with globalization and the global economy. Its rise was motivated by growing water scarcity in arid areas around the world.
- Virtual Water Savings and Trade in Agriculture The idea of virtual water was initially created as a method for assessing how water-rare nations could offer food, clothing, and other water-intensive products to their residents.
- European Invasion and Agriculture in the Caribbean The early invasion of the Europeans in the Caribbean did not prompt the employment of the slave trade in the agricultural activities until the development of the sugar plantations.
- Freedom in American Countryside and Agriculture This paper portrays how freedom has been eliminated in the countryside by the state agriculture department, and whether the farmer has a moral right to do his farming practices.
- Agricultural Problems in Venezuela Agriculture has been greatly underdeveloped in Venezuela, yet it is a country that has vital minerals and resources required for the global economy.
- America’s Agriculture in the Period of 1865-1938 This paper analyzes America’s contribution in prevention of natural calamities, decline of soil quality, promotion of production outlay and provision of sufficient food.
- Capital Taxes and Agriculture
- Canadian Trade With the Chinese Agriculture Market
- Agriculture and Its Impact on Economic Development
- Bacteriocins From the Rhizosphere Microbiome From an Agriculture Perspective
- Agriculture and Its Impact on Financial Institutions
- Agriculture, Fisheries, and Food in the Irish Economy
- Adoption and Economic Impact of Site-Specific Technologies in U.S. Agriculture
- Cash Rents and Land Values in U.S. Agriculture
- Crises and Structural Change in Australian Agriculture
- Biotechnology and Its Application in Agriculture
- Alternative Policies for Agriculture in Europe
- Agriculture and Food Security in Asia by 2030
- Agriculture and Coping Climate Change in Nepal
- Agriculture and Ethiopia’s Economic Transformation
- Culture: Agriculture and Egalitarian Social
- Adaptation, Climate Change, Agriculture, and Water
- Agriculture and the Literati in Colonial Bengal, 1870 to 1940
- Agriculture and Barley Farming Taro
- Agriculture and Agricultural Inputs Markets
- Agriculture and Environmental Challenges
- Challenges for Sustainable Agriculture in India
- Agriculture and German Reunification
- Agriculture and Tourism Relationship in Malaysia Tourism
- 21st Century Rural America: New Horizons for U.S. Agriculture
- Canadian Agriculture and the Canadian Agricultural Industry
- California Agriculture Dimensions and Issues
- Advancements and the Development of Agriculture in Ancient Greece and Rome
- Agriculture and Early Industrial Revolution
- Aztec: Agriculture and Habersham County
- Agriculture and Current Deforestation Practices
- How Has Agriculture Changed From Early Egypt, Greece, and Rome to the Present?
- What Are the Advantages of Using Pesticides on Agriculture?
- Are Digital Technologies for the Future of Agriculture?
- How Did Agriculture Change Our Society?
- Does Agriculture Help Poverty and Inequality Reduction?
- Can Agriculture Prosper Without Increased Social Capital?
- Are Mega-Farms the Future of Global Agriculture?
- How Can African Agriculture Adapt to Climate Change?
- Does Agriculture Really Matter for Economic Growth in Developing Countries?
- Can Conservation Agriculture Save Tropical Forests?
- How Can Sustainable Agriculture Be Better for Americans?
- Are U.S. and European Union Agriculture Policies Becoming More Similar?
- Should Pollution Reductions Count as Productivity Gains for Agriculture?
- Can Market Access Help African Agriculture?
- How Does Genetic Engineering Affect Agriculture?
- Does Individualization Help Productivity of Transition Agriculture?
- Can Spot and Contract Markets Co-Exist in Agriculture?
- How Has Biotechnology Changed Agriculture Throughout the Years?
- Does Trade Policy Impact Food and Agriculture Global Value Chain Participation of Sub-Saharan African Countries?
- Can Sustainable Agriculture Feed Africa?
- How Can Multifunctional Agriculture Support a Transition to a Green Economy in Africa?
- Does Urban Agriculture Enhance Dietary Diversity?
- How Did Government Policy, Technology, and Economic Conditions Affect Agriculture?
- Can the Small Dairy Farm Remain Competitive in US Agriculture?
- What Are the Main Changes in French Agriculture Since 1945 and What Challenges Does It Face Today?
- How Can Marketing Theory Be Applied to Policy Design to Deliver Sustainable Agriculture in England?
- Will African Agriculture Survive Climate Change?
- How Has Agriculture Changed Civilizations?
- Does Urban Agriculture Improve Food Security?
- Can US and Great Plains Agriculture Compete in the World Market?
- The effect of climate change on crop yields and food security.
- Sustainable agricultural practices for soil health.
- Precision agriculture techniques and applications.
- The impact of genetically engineered organisms on crop yields and safety.
- The benefits of agroforestry systems for the environment.
- Current challenges in water management in agriculture.
- The environmental impact of organic farming.
- The potential of urban agriculture to address food insecurity.
- Food waste in the agricultural supply chain.
- Comparing the effectiveness of aquaponic and hydroponic systems.
- Organic vs. conventional farming.
- Can regenerative agriculture combat climate change?
- Agricultural subsidies: pros and cons.
- Should harmful pesticides be banned to protect pollinators?
- Should arable land be used for biofuels or food production?
- Do patent protections of seeds hinder agricultural innovation?
- Agricultural robots: increased efficiency or displaced rural labor?
- Should GMO labeling be mandatory?
- Do the benefits of pesticides outweigh their potential health harms?
- Is it unsustainable to grow water-intensive crops in arid regions?
- The economics of organic farming.
- The need for climate-adaptive crops.
- The role of bees in agriculture and threats to their survival.
- Smart agriculture: transforming farming with data and connectivity.
- The journey of food in modern agricultural supply chains.
- The role of agri-tech startups in agricultural innovation.
- Youth in agriculture: inspiring the next generation of farmers.
- Why should we shift to plant-based meat alternatives?
- The importance of preserving indigenous agricultural practices.
- Smart irrigation systems: optimizing water use in agriculture.
Cite this post
- Chicago (N-B)
- Chicago (A-D)
StudyCorgi. (2022, March 1). 186 Agriculture Essay Topics & Research Questions + Examples. https://studycorgi.com/ideas/agriculture-essay-topics/
"186 Agriculture Essay Topics & Research Questions + Examples." StudyCorgi , 1 Mar. 2022, studycorgi.com/ideas/agriculture-essay-topics/.
StudyCorgi . (2022) '186 Agriculture Essay Topics & Research Questions + Examples'. 1 March.
1. StudyCorgi . "186 Agriculture Essay Topics & Research Questions + Examples." March 1, 2022. https://studycorgi.com/ideas/agriculture-essay-topics/.
Bibliography
StudyCorgi . "186 Agriculture Essay Topics & Research Questions + Examples." March 1, 2022. https://studycorgi.com/ideas/agriculture-essay-topics/.
StudyCorgi . 2022. "186 Agriculture Essay Topics & Research Questions + Examples." March 1, 2022. https://studycorgi.com/ideas/agriculture-essay-topics/.
These essay examples and topics on Agriculture were carefully selected by the StudyCorgi editorial team. They meet our highest standards in terms of grammar, punctuation, style, and fact accuracy. Please ensure you properly reference the materials if you’re using them to write your assignment.
This essay topic collection was updated on January 21, 2024 .
How to Conduct Research on Your Farm or Ranch
Common research designs for farmers.
or call (301) 779-1007 to order.
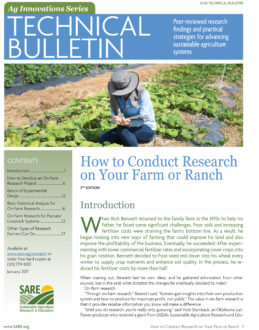
Completely Randomized Design
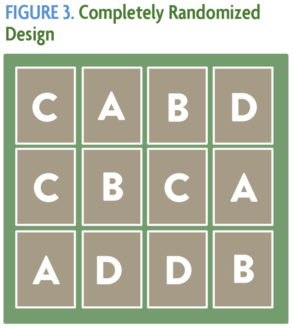
The completely randomized design works best in tightly controlled situations and very uniform conditions. A farmer wants to study the effects of four different fertilizers (A, B, C, D) on corn productivity. Three replicates of each treatment are assigned randomly to 12 plots.
The simplest experimental layout is a completely randomized design (Figure 3). This layout works best in tightly controlled situations and very uniform conditions. For this reason, the completely randomized design is not commonly used in field experiments. You can use it if you are working with a very uniform field, in a greenhouse or growth chamber, or if you have no idea about the variability in your field. The statistical analysis of completely randomized designs is not covered in this publication.
Paired Comparison
As the name implies, the paired comparison is used to compare the effect of two different treatments assigned randomly within blocks. Each block contains two plots—one plot of each treatment—and blocks are replicated four to six times across the field. Typically, plots run the length of the field and are one or two tractor widths in order to facilitate management. Figure 4 shows the layout for a typical paired comparison experiment.
In collecting yield data or other samples from the field, measurements are generally taken from the center rows of a plot in order to avoid any “edge effects.” You can use this design to evaluate any pair of treatments: comparing two varieties, growing the crop with and without starter fertilizer, comparing two rates of fertilizer application, comparing the timing of nutrient application, or using two different cover crop treatments, for example. The paired comparison is a type of randomized block design, but it is usually classified on its own since we use a simplified statistical analysis, the t-test, to analyze the data when compared to the standard randomized complete block design (described next). The t-test will help you determine whether the difference you observe in two treatments is due to natural variation or is a real difference. It is described in the section, Using the t-Test to Compare Two Treatments .
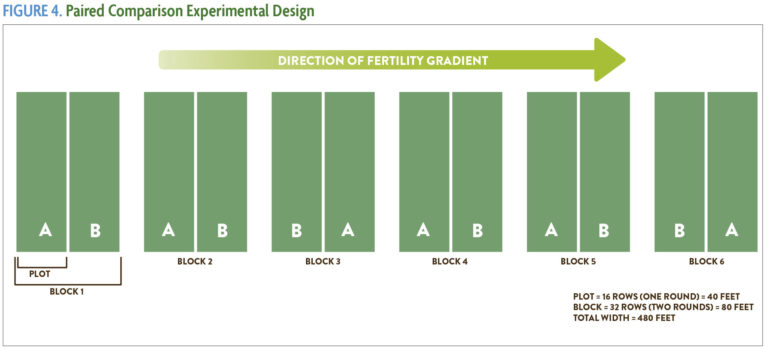
The paired comparison is used to study two treatments. Each treatment should still be replicated several times, generally in blocks that should be set up to account for any known field variability. Randomize treatments within each block. Harvest only the middle rows of each plot (e.g., eight middle rows). Adapted from Anderson (1993).
Randomized Complete Block
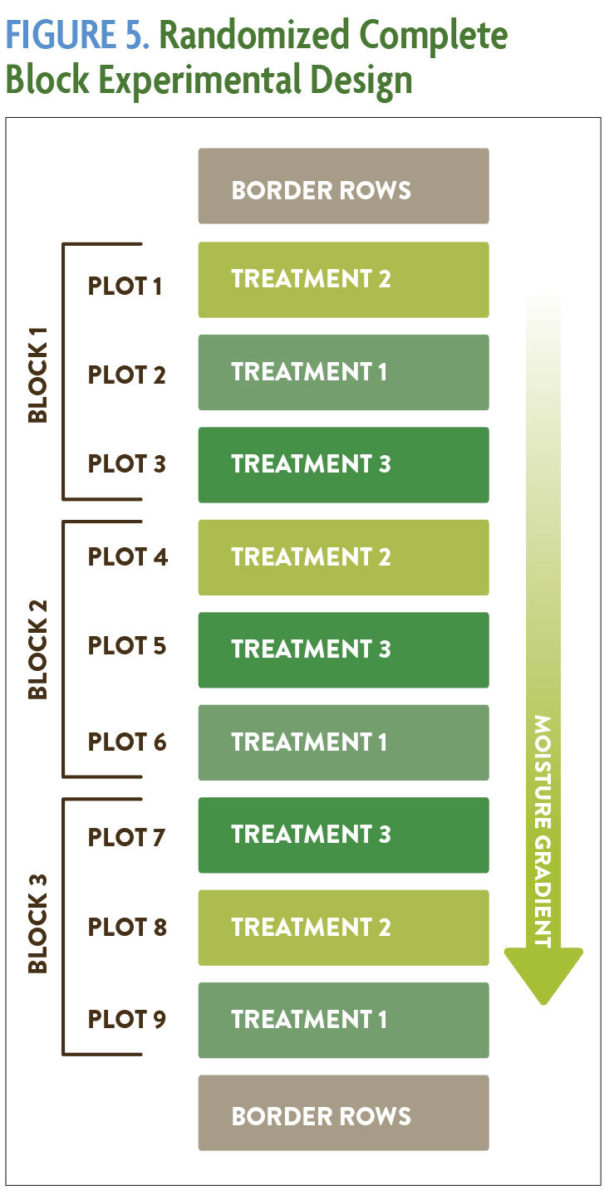
A randomized complete block experiment. Adapted from Nielsen (2010).
The randomized complete block design is used to evaluate three or more treatments. As with the paired comparison, blocking and the orientation of plots helps to address the problem of field variability as described earlier (Figure 3). Each block contains a complete set of treatments, and the treatments are randomized within each block. Four to six replications of a “complete block” are sufficient for most on-farm research projects. Figure 5 shows a schematic of a randomized complete block design with three treatments. The statistical test known as analysis of variance (ANOVA) is used to analyze the data from a randomized complete block experiment.
The split-plot design is for experiments that look at how different sets of treatments interact with each other. It is also used when one of the treatment factors needs more replication or when it is difficult to change the level of one of the factors. For example, in a cover crop study, it may be most convenient due to machinery limitations to plant cover crops in larger areas (the main plots) and then impose other treatments such as fertilizer rates in the sub-plots. In this design, main treatments are overlaid with another set of sub-treatments. Though fairly easy to set up in the field, a split-plot experiment will usually take up a larger area and be more complex to implement, manage and analyze. Given the greater number of treatments and the interaction component, using ANOVA for the split-plot design is also more complex than with the paired comparison or the randomized complete block. It is best to work with someone who has expertise in this type of research design when setting up a split-plot experiment. An example of a split-plot design is shown in Figure 6.
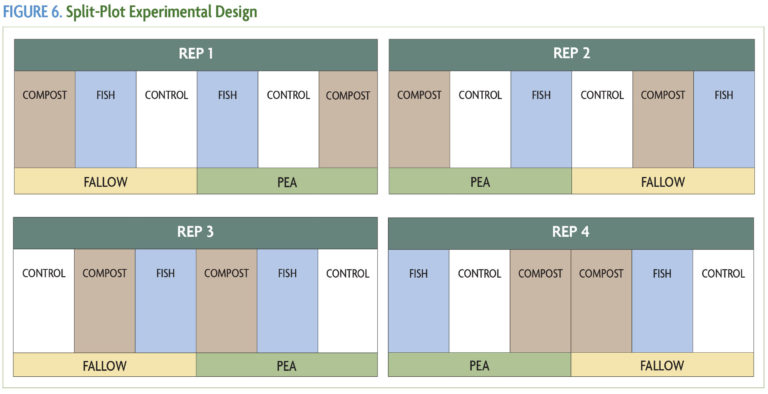
In split-plot design, one treatment (the main plot—fallow or pea) is split further into another treatment (sub-plots) of interest. Here, compost and fish fertilizer are compared to a no-treatment control. Main plots are sometimes decided by field machinery limitations, such as the pea planter used to plant a larger area, with compost and fish emulsion applied to smaller areas. Adapted from Sooby (2001).
Users agree that automated translations may not effectively convert the intended design, meaning, and/or context of the website, may not translate images or PDF content, and may not take into account regional language differences. Any person that uses the translated site does so at that person’s own risk. UGA Extension is not responsible for any damages, costs, liability, or risk associated with any use, functionality, and/or content of the website translations.
For more information, visit the Language Translation page.
Designing Research and Demonstration Tests for Farmers' Fields
Richard F. Davis, Extension Nematologist Glen H. Harris, Extension Agronomist Phillip M. Roberts, Extension Entomologist Greg E. MacDonald, Extension Agronomist
Selecting Treatments
Replication, randomization, experimental designs, data collection, collecting unbiased data, statistical calculations.
As farmers and farm advisers, you make many management choices during a growing season. For example, you may have to decide which cultivar to plant, which herbicide to use, how frequently to apply a fungicide, and what rate of nematicide to use. Often the information needed to make the best decision is available to you, but when it is not available you can frequently compare the options by conducting your own small experiments. Your experiments can be just as valid as any university study if you follow a few important principles of experimental design.
All experiments have certain things in common, so designing an experiment usually includes the following steps. You must decide what question you want to have answered. This is the goal, or objective, of the experiment. The goal of the experiment will dictate what to include in the experiment to help you answer your question. The individual things that you wish to test in your experiment are called "treatments" and the physical areas to which the treatments are applied are called "plots." Then you need to decide how the treatments should be physically arranged in the field. Technically, this is what is called the "experimental design."
Experiments answer your original question by allowing you to make unbiased comparisons among the treatments you selected. You will need some way to evaluate how well each treatment worked to make comparisons among treatments. The information you collect to help you make those comparisons (such as yield, insect counts, or disease severity) is called "data." Finally, you need an objective way to evaluate the data. This is usually done through statistical analysis.
The objective, or purpose, of the study will determine the treatments included in an experiment. Writing down the test objectives is helpful because this forces you to define them precisely. A test may have more than one objective, although multiple objectives should be closely related.
The selection of treatments is usually logical if you can define the purpose of the study; all treatments necessary to address the test's objective should be included. For example, if the purpose were to determine which of five fungicides works the best, then the treatments would include all five of those fungicides. If the purpose were to determine if any of the five fungicides works better than your current choice, then the treatments would include the five fungicides plus the fungicide you currently use. Accurately stating the purpose of the test before the treatments are applied in the field is critical. After the treatments have begun, it will be too late to add other treatments to answer the question you really wanted to address.
The selection of treatments and the experimental design get more complicated as the question you are trying to answer gets more complex. It is common to want to test in the same experiment two (or more) things that influence crop production. For example, you may want to test chicken litter as a fertilizer and test five corn hybrids to maximize yield. The specific questions addressed in this case are:
- What effect does chicken litter have on corn production?
- What effect do the hybrids have on corn production?
- Does chicken litter have the same effect on each hybrid?
The third question may not be as obvious as the first two, but it will always be asked or implied if you are testing two or more factors in the same experiment. In this example, you have to determine what the effect of chicken litter is on each hybrid and then compare those effects to each other. To do that, the treatment list must include each hybrid without chicken litter and each hybrid with chicken litter (a total of 10 treatments). With this list of treatments, you can make the comparisons necessary to answer our three questions. This example employs a "factorial arrangement of treatments" that will be discussed in more detail in a later section.
One final note about treatment selection involves including additional treatments to provide a relative measure of effect. Comparing the yield of five new corn hybrids does little good if you cannot tell how those yields compare with the hybrids you already grow. You should include at least one hybrid with which you are already familiar (often called a "standard" treatment) to provide a relative measure of how well the new hybrids produce. If you wish to test a new nematicide, you should include a treatment with the currently used nematicide and a treatment with no nematicide as a basis for comparison. Such treatments are known as "checks" or "controls." Without the proper controls, you will not be able to say that the new nematicide worked better than the currently used nematicide or even that the new nematicide worked better than no nematicide! The questions you wish the experiment to answer should indicate what treatments should be included as controls.
It is frequently desirable to have both a positive and a negative control in an experiment. The negative control helps you determine if the treatments being tested work better than some minimal treatment (or nothing) and positive controls help you determine if the treatments being tested work better than the current standard practice. You may have several control treatments in an experiment if you currently have several viable options from which to choose. For example, if you currently can choose either of two fungicides to control a leafspot problem, you may wish to include them both as controls in your experiment when you test new products. You do not have to include all currently available options as controls for the experiment to be useful, but you can.
In an experiment, replication means that individual treatments (such as each of the five pesticides being tested in an experiment) have been applied to more than one plot. Replication is necessary because all test plots are not identical, and that leads to variation in the data you collect; you will not get exactly the same results from two plots that received the same treatment. You can take steps to minimize the effect of variation if it has an identifiable cause, but there will always be some variation among plots that cannot be controlled. In statistical terms, uncontrolled variation is called experimental error. The purpose of replication is to allow you to make a more accurate estimate of how each treatment performed even though there is uncontrolled variation in the experiment. This can best be shown in an example.
Suppose you have 10 rose bushes and you want to test if a new fungicide will protect the bushes from black spot, a fungal leaf disease. You could pick five plants to leave untreated as a control and spray the other five with the fungicide. Later, when black spot is evident on the leaves, you count the number of diseased spots on each plant and compare the two treatments. The five untreated plants have 26, 21, 19, 25, and 23 infected spots (a treatment mean, or average, of 22.8 spots per plant), and the fungicide treated plants have 20, 15, 18, 21, and 20 spots (a mean of 18.8). Statistical analysis indicates that the fungicide did in fact reduce the number of infected sites, but you would not be able to determine that if you only had one treated and one untreated plot. Think about the following examples based on the data above. If you only had two plants and the untreated plant had 26 spots and the treated plant had 15 spots, it may seem easy to determine that the fungicide reduced disease. But what if the untreated plant had 19 spots and the treated plant had 21 spots? You might conclude that the fungicide did not work or even that it increased disease! Adequate replication can minimize this problem.
It is common when you have several replications of each treatment to have data like that in the rose example above: The treatment means are different but individual measurements may overlap. In this example, the lowest measurement from the untreated plants was 19, and the highest measurement from the fungicide treated plants was 21, but the treatment means were 22.8 for the untreated plants and 18.8 for the treated plants. Replication of treatments increases your ability to detect differences in treatment means. Having more replications allows you to identify (statistically) smaller differences in treatment means than you could identify with fewer replications.
The number of replications that you need is influenced by the biology of what you are testing, how close together the treatment means are, and how much variation exists within a treatment. For field tests in plant pathology, nematology, weed science, soil fertility studies, and entomology, a minimum of four replications is suggested, but five or six replications are much better. If treatment means are close together or variation is relatively large among the plots that received the same treatment, then you may need more replications to detect differences among treatments.
Just as the data may vary within a replicated treatment, the results may vary among experiments if the whole experiment is repeated. This can happen because of different weather conditions, different disease or insect pressure, or many other factors beyond your control. This does not mean that the results of a single experiment are not valid, but it does make it dangerous to draw conclusions from a single experiment. The one set of results you have may indicate treatment differences, but if you repeated the test several times you might not see those treatment differences again. If the test is repeated (and that means you cannot change any of the treatments) and you get similar results, then you can be much more confident that your conclusions are correct.
Randomization in an experiment means that the treatments are assigned to plots with no discernable pattern to the assignments. The reason randomization is important is that the positioning of treatments within the block may affect their performance. One example of this is an experiment testing five corn hybrids (labeled 1 through 5) in which you plant the hybrids in the same order in each block: 1, 2, 3, 4, then 5 (see figure 1 ). If hybrid 2 is naturally much taller than the others, it can slightly shade the hybrids planted next to it (hybrids 1 and 3) and unfairly make them look a little bit worse than they would look if they were not planted next to hybrid 2. Another example is a field in which soil fertility gets progressively lower as you cross the field from east to west so that productivity is reduced as you go from one side of the field to the other. If two corn hybrids are planted side by side but within a block, hybrid 1 is always planted on the east side of hybrid 2, then hybrid 1 is always planted in slightly more fertile soil and therefore has an unfair advantage.
In both of the preceding examples, randomization could have prevented the unintentional bias because the arrangement of the treatments would have been different within each block. Because you cannot anticipate all the influences that may introduce bias into a test, ALL experiments should be randomized. There are many ways to randomize treatments within a block, but the simplest is literally to pull the numbers out of a hat. Assign each treatment a number, write the numbers on individual pieces of paper, mix the slips of paper up, and then select the slips one at a time without looking at them first. The order in which the numbers are drawn is the order in which they will be arranged in a block. Repeat these steps for each block in the experiment.
If you wish to use the experiment as a demonstration plot (such as for a field day), it is common that one block not be truly randomized. This is done so that particular treatments can be seen side-by-side to facilitate comparisons and highlight differences for casual observers. Though it is better to randomize all blocks and not intentionally arrange treatments, arranging the treatments in one block is unlikely to affect the test's results as long as the other blocks are truly randomized.
A plot, the area to which an individual treatment is applied, can be any size, including a single plant growing in a pot or 5 acres or more of a field. Before you can apply treatments to your test area, you must decide how large your plots should be. Although there is a lot of subjectivity in selecting plot size, there are some important considerations including the equipment to be used in planting, harvesting, and treatment application; how much space is available for use in the experiment and how many other treatments there are; and the biology of what you are studying. Accommodating equipment and space concerns makes it easier to conduct the test. Accommodating biological concerns reduces the chances of overlooking differences among treatments. Equipment and space considerations are usually easy to identify, but biological considerations are not always obvious.
If you have equipment to plant, harvest, and apply treatments to four rows at a time, then the logical plot width would be some multiple of four rows (four rows, eight rows, 12 rows, etc.). Any other width (such as six rows) would make it more difficult to conduct the experiment. The length that plots should be is more flexible than plot width. For example, if you plan to weigh the harvest from each plot, the scales you have may influence the length plots should be. If you have scales that are designed for weighing hundreds of pounds, your plots should be large enough to provide a harvest weight that can be accurately determined by your equipment, and increasing the length of plots is an easy way to do that. Also, the length of your plots may be adjusted so that all of your plots (all replications of all treatments) will fit into the area available for your test. If you have a large area for your test, space may not be an important consideration.
To accommodate biological considerations, you should answer two questions:
- How large a plot is needed to observe the biological effect (disease severity, insect damage, weed frequency, nematode population levels, etc.) that you are studying?
- How large a plot is needed to minimize the influence of a treatment (chemical application, etc.) on the plots next to it?
By answering these questions, you can determine the minimum plot size necessary to get useful data from the experiment.
To get an accurate measurement of the effect of pest management treatments, the plot must be large enough to account for uneven initial distribution of the pest (pathogen, insect, weed, etc.). Some areas may start with the pest present, but the pest may occur in other areas only after it has spread from its initial location. This is very important for pests that spread very slowly (such as most soilborne organisms).
Some diseases and pests are highly mobile and spread very rapidly (such as many insects). In an insect management trial, measuring the effect of a treatment can be very difficult if your plots are too small because the insects that you see in the plot may have simply spread from the plot next to it. To minimize this problem, you can increase your plot size and then collect data from the middle section of the plot. For example, you might have an eight-row plot but only collect data from the middle four rows. The rows from which you do not collect data are often referred to as "buffer rows" because they buffer the effect of the neighboring plots. If you do not use buffer rows when they are needed, you may fail to detect differences among treatments and incorrectly conclude that many treatments were ineffective. Buffer rows are frequently used when there is uncertainty whether treatments can influence nearby rows.
A similar concept involves the use of border rows along the edges of your test area. A significant "border effect" commonly exists at the edge of a field where the plants may grow differently than plants not at the edge. Although you may be able to minimize this problem with blocking, it is often better to eliminate the problem by not using the rows at the edge of a field in your experiment.
Once the plots are large enough to be representative of a much larger area, further increasing plot size will not significantly improve the accuracy of the results. For example, in an experiment testing fungicides for control of white mold, or stem rot, in peanut, a four-row-wide by 100-foot-long plot should be just as good as an eight-row-wide by 400-foot-long plot. Plots that are larger than necessary take more field space and may increase the amount of work required for an experiment, but they usually will not adversely affect the test results unless the plots get so large that the plots within a block are no longer uniform. Plots that are too small may prevent the accurate assessment of treatment effects. If the space available for an experiment is limiting, having more replications is usually more beneficial than having larger plots as long as your plot size allows accurate assessment of treatment effects.
Completely Randomized Design
The completely randomized design is the simplest experimental design. In this design, treatments are replicated but not blocked, which means that the treatments are assigned to plots in a completely random manner (as in the left side of figure 2 ). This design is appropriate if the entire test area is homogeneous (uniform in every way that can influence the results). Unfortunately, it is rare that you can ever be confident of a test site's uniformity, so a completely randomized design is rarely used in field tests. The completely randomized design is used more commonly in greenhouse tests, though blocking is often useful even in the more controlled environment of a greenhouse.
Randomized Complete Block Design
The randomized complete block design is the most commonly used design in agricultural field research. In this design, treatments are both replicated and blocked, which means that plots are arranged into blocks and then treatments are assigned to plots within a block in a random manner (as in the right side of figure 2 ). This design is most effective if you can identify the patterns of non-uniformity in a field such as changing soil types, drainage patterns, fertility gradients, direction of insect migration into a field, etc. If you cannot identify the potential sources of variation, you should still use this design for field research but make your blocks as square as possible. This usually will keep plots within a block as uniform as possible even if you cannot predict the variation among plots.
Blocking refers to physically grouping treatments together in an experiment to minimize unexplained variation in the data you collect (referred to as experimental error). This allows the statistical analysis to identify treatment differences that would otherwise be obscured by too much unexplained variation in the experiment. Variation in an experiment can be divided into two types: variation for which you can account in the statistical analysis and variation that is unexplained. The goal in blocking is to allow you to measure the variation among blocks and then remove that variation from the statistical comparison of treatment means. If you can anticipate causes of variation, you can block the treatments to minimize variation within each block and remove some variation from the statistical analysis. The mathematics of how blocking allows you to reduce unexplained variation is beyond the scope of this bulletin.
In the most common experimental designs, a block will contain one plot of each treatment in the experiment. If an experiment has five treatments, then each block will contain five plots, with each plot receiving a different treatment. When a block contains one plot of each treatment, then each block represents one replication of each treatment. For this reason, blocks are frequently referred to as "replications" or "reps," but the concept of blocking should not be confused with the concept of replication; replication and blocking serve different purposes. In agricultural research, field plots are almost always blocked even when no obvious differences are present in the field. It is much better to block when you did not really need to than not to block when you should have blocked.
Blocking is a very powerful tool that is most effective if you can anticipate sources of variation before you begin an experiment. For example, in a herbicide trial, one side of a field may have a history of more severe weed problems. If you just scattered your treatments randomly through the field, a lot of the variation in the data you collected could be due to the increased weed pressure on one side of the field. Such variation would make it difficult to determine how well each treatment worked. Because you know one side of the field will have more weeds, you can remove that source of variation from the statistical analysis by blocking and improve your chances of identifying differences among treatments.
The process of blocking follows a logical sequence. First, you determine that there is something (weeds, drainage, sun/shadow, water, soil type, etc.) that is not uniform throughout the experimental area (field, greenhouse, etc.) that may influence whatever you are measuring (yield, plant height, etc.). Then you can arrange your treatments into blocks so that the area within each block is as uniform as possible (see figure 2 ). Though the area within a block should be relatively uniform, there may be large differences among the blocks, but that is what makes blocking effective. Your goal is to maximize the differences among blocks while minimizing the differences within a block.
The shape of the blocks is not important as long as the plots within a block are as uniform as possible. Ideally, the only differences among plots within a block should be due to the treatments. Blocks in field experiments are usually square or rectangular, but they may be any shape. Blocks in the same experiment do not have to be the same shape; the shape of individual blocks will be determined by variation in the field that you are trying to minimize. If you are not sure what shape your blocks should be, square or nearly square blocks are usually a safe choice.
Blocks may be arranged through the field in many ways. If the field is wide enough, an easy way to arrange blocks is to place them side-by-side all the way down the field (see figure 3 ). But blocks do not have to be contiguous and may be scattered through the field in any way that is convenient for you.
Factorial Arrangement of Treatments
A factorial arrangement of treatments is not an experimental design, though you will often hear it referred to as a factorial design or a factorial experiment. A factorial arrangement of treatments means that the experiment is testing two or more factors at the same time, and that the experiment includes all combinations of all factors. The term "factor" is used to describe a group of treatments that have something in common. Fungicides, sources of nitrogen, or corn hybrids could be considered factors in an experiment. Factors may be defined broadly or narrowly in different experiments. All herbicides may be grouped as a factor in one experiment, but pre-plant and post-plant herbicides may be treated as separate factors in another experiment. A single-factor experiment tests one factor at a time; a two-factor experiment tests two factors at once.
Most simple on-farm experiments are single-factor experiments (in a Completely Randomized or Randomized Complete Block design) and compare things such as crop varieties or herbicides, but it is sometimes useful to test two or more factors at once. For example, a two-factor experiment would allow you to compare the yields five corn hybrids at three planting dates. This accomplishes three things at once:
- It allows you to compare the corn hybrids with each other.
- It allows you to evaluate the effect of planting date.
- It allows you to determine if varying the planting date changes the relative performance of the hybrids (e.g. one hybrid may only perform well if planted early).
The first two could be done in separate single-factor experiments, but the third can only be achieved by having both factors in a single experiment. This becomes especially important if one factor can have a significant influence on the effect of the other factor. For example, you might test soybean varieties as one factor and nematicides as another factor. If a few varieties have good nematode resistance but others do not, they may appear equally good when effective nematicides are used but varieties with resistance would appear much better when nematicides are not used. In cases like this, the effect of one factor (variety) is strongly influenced by the other factor (nematicide). When one factor influences the effect of the other factor, there is said to be a significant interaction between the two factors. It can be very important to know if there is an interaction between factors, because if there is an interaction, you can make predictions or recommendations based on the results of single-factor experiments ONLY when all other factors are at the same levels they were at in the experiment. If you change some factor not included in the experiment, the results from your single-factor experiment may no longer be valid.
With a factorial arrangement of treatments, all values (or levels) of each factor must be paired with all levels of the other factors. If you have two nematicides and five soybean varieties, then your treatment list must include each variety with each nematicide for a total of 10 treatments. This would be referred to as a "two by five factorial" to denote how many factors were present in the experiment and how many levels of each factor were used. The number of treatments increases quickly when you add more levels for a factor (if you used three nematicides instead of two, you would have 15 treatments instead of 10), so choose your levels carefully or the experiment can get too large to manage.
A factorial arrangement of treatments can be a very powerful tool, but because the number of treatments can get very large it is best used when some reason exists to believe that the factors may influence each other and have a significant interaction. If there is no suspicion that the factors may influence each other, it is frequently easier and more thorough to test the factors in separate experiments. A factorial arrangement of treatments can be used with a completely randomized experimental design or a randomized complete block design. The top half of figure 4 shows a factorial arrangement of treatments in a randomized complete block design.
Split-Plot Experimental Design
A split-plot experimental design is a special design that is sometimes used with factorial arrangements of treatments. This design usually is used when an experiment has at least two factors and some constraint prevents you from randomizing the treatments into a randomized complete block design. Such a constraint may be based on equipment limitations or on biological considerations. For example, the equipment you have may make it difficult to put out a soil fumigant in randomized complete blocks, but you may be able to put out the fumigant so that all treatments within a block that get the fumigant will be clustered together rather than scattered throughout the block. You can use a split-plot experimental design to work around this limitation as long as you are able to randomize the other factors. There are other situations when this design is appropriate, but a constraint on randomization is the most likely to occur.
Suppose you want to test the effect of five fungicides to control Cylindrocladium Black Rot on two varieties of peanut. In this test, you would have a 2x5 factorial arrangement of treatments: The two factors would be varieties (2 levels of this factor) and fungicides (5 levels of this factor). Because a factorial arrangement of treatments is not an experimental design, you still have to select an experimental design that best meets your needs. If you are able to randomize varieties and fungicides within a block, then you should pick a randomized complete block design. If there is some reason why you cannot completely randomize the treatments within each block, then you may be able to use a split-plot design to work around that limitation. For example, you may have a six-row planter but only enough space in the field to put out four-row plots. To resolve this dilemma, you could plant all of the plots that have the same peanut variety together within a block and then randomize the five fungicide treatments within each peanut variety.
In split-plot designs, the terms "whole plots" and "sub-plots" refer to the plots into which the factors are randomized. As the names imply, whole plots are subdivided into subplots. In figure 4 , a whole plot would be the areas designated with A or B, and the subplots, the subdivisions within the whole plots, are designated 1, 2, 3, 4, or 5. In this example, A and B could represent two varieties (two levels of one factor) and the numbers could represent different fungicides (five levels of a second factor). Each whole plot serves as a block for the subplot treatments.
To assign treatments in a split-plot design, start by identifying where each block will be. Then randomize the whole plot treatments within each block. The whole plot treatments will be the treatment that you are unable to randomize into a randomized complete block design. The subplot treatments can then be randomized within each whole plot treatment (see figure 4 ).
You can collect an almost infinite amount of data in any experiment, but not all of it will be useful. Proper planning will ensure that you collect the right data to address your test's objective. The "right" data to collect can usually be determined by examining the stated purposes of the experiment. For example, if the objective of a peanut leafspot fungicide trial is "to evaluate the ability of five fungicides to reduce leafspot incidence and severity," then collecting data on leafspot incidence and severity and peanut yield should seem obvious. Collecting data on rainfall and temperature, which strongly influence leafspots on peanut, may be worthwhile because it can help you explain your results. But collecting data on soil physical properties does not seem to be related to the objective. It is useful to ask yourself, "How can this data be used?" If you have trouble answering that question, then collecting that data may be a waste of time. It is much more common for people to collect too little data than to collect too much data.
Deciding what data to collect is only part of the process. You also have to decide when to collect that data and if you need to collect the same type of data on more than one occasion. For example, in a nematicide trial, it is not sufficient to collect nematode population data at harvest; you must also collect data at planting to ensure that the plots started out equal. It is usually a good idea to collect nematode population data in the middle of the season because even in effective treatments nematode populations can sometimes increase to the level of the untreated control by the end of the season. The biology of the organisms involved will determine when and how frequently data should be collected.
So, how much data is enough? The answer is "enough data to fully address the test's objective." If you understand the biology of the organisms involved and how your data addresses the test objective, then you should be able to tell if you are collecting enough data.
You should take photographs of any differences among treatments that are easily visible. To most farmers, a picture is more convincing than a graph or data table.
It is critically important to collect unbiased data. The only way to ensure this is to collect data without knowing what the treatment was in that plot. That would be difficult to do if the treatment were written on a stake in front of each plot. It is beneficial to use some type of code on the plot stakes so that you have to decode the stake number to determine what the treatment was. You can make up any code you like just so long as the person collecting the data cannot tell from the plot stake what the treatment was. For example, you can number the plots sequentially (1, 2, 3, etc.) and have a sheet of paper listing what treatment was applied to plot 1, plot 2, etc. When you collect the data, you write down your observation for plot 1 and later look at your list to see what treatment was in that plot.
If you know what treatment was in a plot, or which plots were the untreated controls, your evaluations (disease severity ratings, insect damage ratings, etc.) may inadvertently be influenced. Your subconscious may slightly increase the ratings for untreated plots and decrease it for the plots with treatments that you think should work well. You will probably not even be aware that it is happening, but these subtle influences can change the data enough to affect your ultimate conclusions from the test. If you do not collect unbiased data, you cannot be certain that your conclusions are correct.
After collecting data from a properly designed experiment, you will usually need to analyze the data with appropriate statistical calculations. Statistical analysis may not be necessary if treatment differences are very large and consistent; treatment means may then be sufficient. Statistical analysis is beyond the scope of this publication. Proper statistical analysis can be done if your experiment was designed according to the principles outlined in this publication, but proper analysis can be complicated greatly if these principles were not followed.
It is probably best for you to seek help in making statistical calculations. If your experiment was properly designed, Extension specialists and other scientists may be willing to help you with the statistics if you involve them early in the process. They can also check your proposed design for flaws and omissions. If you want to do the work yourself some simple statistics can be calculated by hand, but most people will make the calculations with the help of computer software. Specialized statistical software is available, but most spreadsheet software can calculate simple statistics.
The following checklist can be used in designing an experiment. These items may be addressed in any order.
- Determine the objective of the test.
- Select treatments to address the objective. Consider including positive and negative controls.
- Determine what data should be collected, and when it should be collected, to address the objective.
- Select the number of replications to use. Consider four replications a minimum.
- Determine how big individual plots will be.
- Select an experimental design.
- Determine how blocks should be arranged in the field.
- Randomize treatments within blocks.
Properly designing and implementing a field trial may seem complex the first time, but it is really a logical process that should not be intimidating. You may need help the first time you design a trial to ensure that you are not overlooking something important, but if you learn the principles involved in the process, you should quickly gain confidence in your ability to conduct experiments on your own.
Status and Revision History Published on May 01, 1999 Published on Feb 09, 2009 Published on Apr 28, 2009 Published with Full Review on Apr 25, 2012 Published with Full Review on Jan 30, 2017
Have a question?
Related publications.
- Whitefly-Transmitted Plant Viruses in South Georgia (B 1507)
- Common Tomato Diseases in Georgia (B 1285)
- 2023 Vegetable Extension and Research Report (AP 113-5)
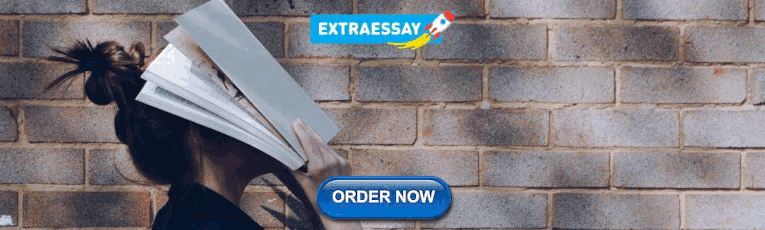
IMAGES
VIDEO
COMMENTS
Analysing the impact of fish farming on agriculture: A case study of Japan. Smart farming in Germany: The impact of using drones in crop management. Comparing the farming regulations in California and Texas. Economics of pig farming for country farmers in the United States.
Experimental research on these transformations is incipient and creates new challenges. 3. Methodological adjustments. Taking the experimental approach from the lab to the field has to confront challenges specific to agriculture which is simultaneously a dimension of nature, a sector of economic activity, and a way of life.
The query returned 3955 documents published from 1940 but with a steep and constant increase in numbers of publications from 1980. The five most frequent journals were Field Crops Research (157 articles), Experimental Agriculture (118), Agronomy Journal (102), Agricultural Systems (88), and Acta Horticulturae (85).. 3.1 Analysis of the structure of the corpus: Seven thematic clusters related ...
With a focus on the tropical and sub-tropical regions of the world, Experimental Agriculture publishes the results of original research on field, plantation and herbage crops grown for food or feed, or for industrial purposes, and on farming systems, including livestock and people. It reports experimental work designed to explain how crops respond to the environment in biological and physical ...
On-farm experimentation (OFE) is an effective approach that brings agricultural stakeholders to support farmers' own management decisions for agricultural innovation, with digitalization playing ...
Field Experiments in Developing Country Agriculture437. nontraded goods, a time constraint for own family labor, and a budget constraint over traded goods. An important aspect of agricultural production is its variability, due to both predictable seasonality and annual stochastic weather realizations or other shocks.
Without trying to be complete, we discuss several promising developments in experimental. research. 2. Technology adoption. A key challenge facing farmers and policy ma kers concerns technology ...
An example is experimentation with flood resilient new. Methodological adjustments. Taking the experimental approach from the lab to the field has to confront challenges specific to agriculture which is simultaneously a dimension of nature, a sector of economic activity, and a way of life.
Definition. Agricultural research can be broadly defined as any research activity aimed at improving productivity and quality of crops by their genetic improvement, better plant protection, irrigation, storage methods, farm mechanization, efficient marketing, and a better management of resources (Loebenstein and Thottappilly 2007 ).
Experimental Agriculture. ISSN: 0014-4797 (Print) , 1469-4441 (Online) Frequency: 1 volume per year. With a focus on the tropical and sub-tropical regions of the world, Experimental Agriculture publishes the results of original research on field, plantation and herbage crops grown for food or feed, or for industrial purposes, and on farming ...
This chapter discusses the statistical approach to experimental design, with an emphasis on the core concepts of randomization and blocking. It focuses initially on agricultural field experiments, but also describes the basic elements of a type of medical research investigation known as a clinical trial.
A multi discipline "Data-Intensive Farm Management" project is focused on developing a research framework to conduct randomized agronomic field trials testing multiple factors in farmers' fields using precision agriculture technologies (Bullock et al., 2019). Currently the framework is being tested across nine U.S. states, and in ...
The aim of this study is to analyze the dynamics of the research on the application of experimental economics in agriculture on a global level. Thus, a literature review has been carried out for the period between the years 2000 and 2020 based on a bibliometric study. The main results show that there has been a growing use of experimental ...
Engineering and agronomy aspects of a long-term precision agriculture field experiment. USDA-ARS?s Scientific Manuscript database. Much research has been conducted on specific precision agriculture tools and implementation strategies, but little has been reported on long-term evaluation of integrated precision agriculture field experiments.In 2004 our research team developed and initiated a ...
14.1 Introduction. Design of Experiments is an integral component of agricultural research. A scientifically designed experiment is a valuable tool in advancement in gaining new knowledge and technology development. "It is the effective use of the tools of statistical design of experiments that paved the way for the green revolution ...
Over the last two decades, experimental economics has been gaining relevance in the research of a wide range of issues related to agriculture. In turn, the agricultural activity provides an excellent field of study within which to validate the use of instruments employed by experimental economics. The aim of this study is to analyze the dynamics of the research on the application of ...
This essay discusses the savings associated with virtual water trade in agriculture and touches on the effects of a shift to local agricultural production on global water savings. Food Safety: A Policy Issue in Agriculture Today. Food safety constitutes proper preparation, storage and preservation of all foods.
Principles of Experimental design. • To explore new technologies, crops and new areas of production. • To develop a basic understanding of the factors that control production. • To develop ...
Basics of Experimental Design. or call (301) 779-1007 to order. The previous section summarized the 10 steps for developing and implementing an on-farm research project. In steps 1 through 3, you wrote out your research question and objective, developed a hypothesis, and figured out what you will observe and measure in the field.
US Department of Agriculture. This site is maintained by SARE Outreach for the SARE program and is based upon work supported by the National Institute of Food and Agriculture, U.S. Department of Agriculture, under award No. 2021-38640-34723.SARE Outreach operates under cooperative agreements with the University of Maryland to develop and disseminate information about sustainable agriculture.
The randomized complete block design is the most commonly used design in agricultural field research. In this design, treatments are both replicated and blocked, which means that plots are arranged into blocks and then treatments are assigned to plots within a block in a random manner (as in the right side of figure 2). This design is most ...
A sample of each organic input was taken and N content determined (Tab le 3); then the amount of organics to be applied, equivalent to 30 or 60 kg N, was determined (for the treatments
The results indicated that the simulation results closely matched the experimental data when the number of sub-spheres in the bonded-particle model was equal to or more than 70, as measured by the standard deviation. ... This example will serve as a valuable reference point for future field studies. ... Agriculture 2024, 14(5), 752; https://doi ...
PDF | First published on 1984( in Farsi ) have seven chapters and 467 pages | Find, read and cite all the research you need on ResearchGate