Ohio State nav bar
The Ohio State University
- BuckeyeLink
- Find People
- Search Ohio State
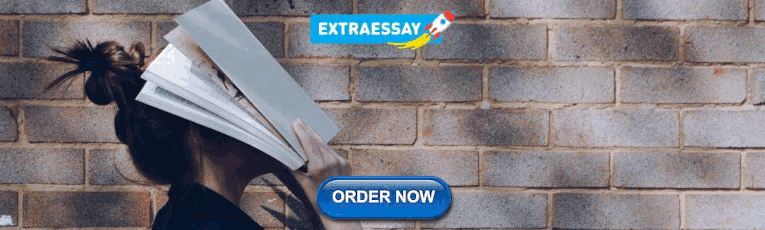
Research Questions & Hypotheses
Generally, in quantitative studies, reviewers expect hypotheses rather than research questions. However, both research questions and hypotheses serve different purposes and can be beneficial when used together.
Research Questions
Clarify the research’s aim (farrugia et al., 2010).
- Research often begins with an interest in a topic, but a deep understanding of the subject is crucial to formulate an appropriate research question.
- Descriptive: “What factors most influence the academic achievement of senior high school students?”
- Comparative: “What is the performance difference between teaching methods A and B?”
- Relationship-based: “What is the relationship between self-efficacy and academic achievement?”
- Increasing knowledge about a subject can be achieved through systematic literature reviews, in-depth interviews with patients (and proxies), focus groups, and consultations with field experts.
- Some funding bodies, like the Canadian Institute for Health Research, recommend conducting a systematic review or a pilot study before seeking grants for full trials.
- The presence of multiple research questions in a study can complicate the design, statistical analysis, and feasibility.
- It’s advisable to focus on a single primary research question for the study.
- The primary question, clearly stated at the end of a grant proposal’s introduction, usually specifies the study population, intervention, and other relevant factors.
- The FINER criteria underscore aspects that can enhance the chances of a successful research project, including specifying the population of interest, aligning with scientific and public interest, clinical relevance, and contribution to the field, while complying with ethical and national research standards.
- The P ICOT approach is crucial in developing the study’s framework and protocol, influencing inclusion and exclusion criteria and identifying patient groups for inclusion.
- Defining the specific population, intervention, comparator, and outcome helps in selecting the right outcome measurement tool.
- The more precise the population definition and stricter the inclusion and exclusion criteria, the more significant the impact on the interpretation, applicability, and generalizability of the research findings.
- A restricted study population enhances internal validity but may limit the study’s external validity and generalizability to clinical practice.
- A broadly defined study population may better reflect clinical practice but could increase bias and reduce internal validity.
- An inadequately formulated research question can negatively impact study design, potentially leading to ineffective outcomes and affecting publication prospects.
Checklist: Good research questions for social science projects (Panke, 2018)
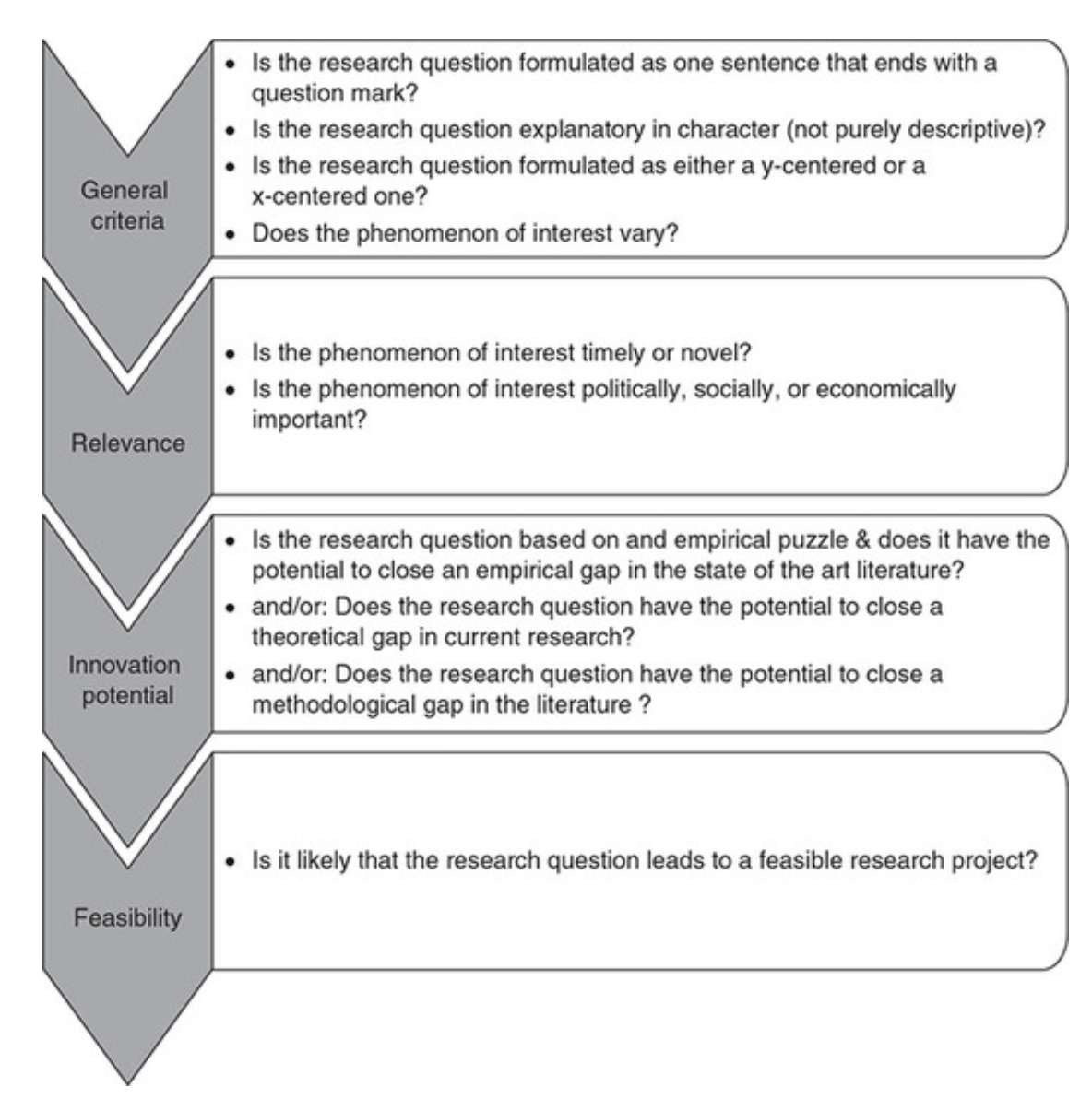
Research Hypotheses
Present the researcher’s predictions based on specific statements.
- These statements define the research problem or issue and indicate the direction of the researcher’s predictions.
- Formulating the research question and hypothesis from existing data (e.g., a database) can lead to multiple statistical comparisons and potentially spurious findings due to chance.
- The research or clinical hypothesis, derived from the research question, shapes the study’s key elements: sampling strategy, intervention, comparison, and outcome variables.
- Hypotheses can express a single outcome or multiple outcomes.
- After statistical testing, the null hypothesis is either rejected or not rejected based on whether the study’s findings are statistically significant.
- Hypothesis testing helps determine if observed findings are due to true differences and not chance.
- Hypotheses can be 1-sided (specific direction of difference) or 2-sided (presence of a difference without specifying direction).
- 2-sided hypotheses are generally preferred unless there’s a strong justification for a 1-sided hypothesis.
- A solid research hypothesis, informed by a good research question, influences the research design and paves the way for defining clear research objectives.
Types of Research Hypothesis
- In a Y-centered research design, the focus is on the dependent variable (DV) which is specified in the research question. Theories are then used to identify independent variables (IV) and explain their causal relationship with the DV.
- Example: “An increase in teacher-led instructional time (IV) is likely to improve student reading comprehension scores (DV), because extensive guided practice under expert supervision enhances learning retention and skill mastery.”
- Hypothesis Explanation: The dependent variable (student reading comprehension scores) is the focus, and the hypothesis explores how changes in the independent variable (teacher-led instructional time) affect it.
- In X-centered research designs, the independent variable is specified in the research question. Theories are used to determine potential dependent variables and the causal mechanisms at play.
- Example: “Implementing technology-based learning tools (IV) is likely to enhance student engagement in the classroom (DV), because interactive and multimedia content increases student interest and participation.”
- Hypothesis Explanation: The independent variable (technology-based learning tools) is the focus, with the hypothesis exploring its impact on a potential dependent variable (student engagement).
- Probabilistic hypotheses suggest that changes in the independent variable are likely to lead to changes in the dependent variable in a predictable manner, but not with absolute certainty.
- Example: “The more teachers engage in professional development programs (IV), the more their teaching effectiveness (DV) is likely to improve, because continuous training updates pedagogical skills and knowledge.”
- Hypothesis Explanation: This hypothesis implies a probable relationship between the extent of professional development (IV) and teaching effectiveness (DV).
- Deterministic hypotheses state that a specific change in the independent variable will lead to a specific change in the dependent variable, implying a more direct and certain relationship.
- Example: “If the school curriculum changes from traditional lecture-based methods to project-based learning (IV), then student collaboration skills (DV) are expected to improve because project-based learning inherently requires teamwork and peer interaction.”
- Hypothesis Explanation: This hypothesis presumes a direct and definite outcome (improvement in collaboration skills) resulting from a specific change in the teaching method.
- Example : “Students who identify as visual learners will score higher on tests that are presented in a visually rich format compared to tests presented in a text-only format.”
- Explanation : This hypothesis aims to describe the potential difference in test scores between visual learners taking visually rich tests and text-only tests, without implying a direct cause-and-effect relationship.
- Example : “Teaching method A will improve student performance more than method B.”
- Explanation : This hypothesis compares the effectiveness of two different teaching methods, suggesting that one will lead to better student performance than the other. It implies a direct comparison but does not necessarily establish a causal mechanism.
- Example : “Students with higher self-efficacy will show higher levels of academic achievement.”
- Explanation : This hypothesis predicts a relationship between the variable of self-efficacy and academic achievement. Unlike a causal hypothesis, it does not necessarily suggest that one variable causes changes in the other, but rather that they are related in some way.
Tips for developing research questions and hypotheses for research studies
- Perform a systematic literature review (if one has not been done) to increase knowledge and familiarity with the topic and to assist with research development.
- Learn about current trends and technological advances on the topic.
- Seek careful input from experts, mentors, colleagues, and collaborators to refine your research question as this will aid in developing the research question and guide the research study.
- Use the FINER criteria in the development of the research question.
- Ensure that the research question follows PICOT format.
- Develop a research hypothesis from the research question.
- Ensure that the research question and objectives are answerable, feasible, and clinically relevant.
If your research hypotheses are derived from your research questions, particularly when multiple hypotheses address a single question, it’s recommended to use both research questions and hypotheses. However, if this isn’t the case, using hypotheses over research questions is advised. It’s important to note these are general guidelines, not strict rules. If you opt not to use hypotheses, consult with your supervisor for the best approach.
Farrugia, P., Petrisor, B. A., Farrokhyar, F., & Bhandari, M. (2010). Practical tips for surgical research: Research questions, hypotheses and objectives. Canadian journal of surgery. Journal canadien de chirurgie , 53 (4), 278–281.
Hulley, S. B., Cummings, S. R., Browner, W. S., Grady, D., & Newman, T. B. (2007). Designing clinical research. Philadelphia.
Panke, D. (2018). Research design & method selection: Making good choices in the social sciences. Research Design & Method Selection , 1-368.
Have a language expert improve your writing
Run a free plagiarism check in 10 minutes, automatically generate references for free.
- Knowledge Base
- Methodology
- How to Write a Strong Hypothesis | Guide & Examples
How to Write a Strong Hypothesis | Guide & Examples
Published on 6 May 2022 by Shona McCombes .
A hypothesis is a statement that can be tested by scientific research. If you want to test a relationship between two or more variables, you need to write hypotheses before you start your experiment or data collection.
Table of contents
What is a hypothesis, developing a hypothesis (with example), hypothesis examples, frequently asked questions about writing hypotheses.
A hypothesis states your predictions about what your research will find. It is a tentative answer to your research question that has not yet been tested. For some research projects, you might have to write several hypotheses that address different aspects of your research question.
A hypothesis is not just a guess – it should be based on existing theories and knowledge. It also has to be testable, which means you can support or refute it through scientific research methods (such as experiments, observations, and statistical analysis of data).
Variables in hypotheses
Hypotheses propose a relationship between two or more variables . An independent variable is something the researcher changes or controls. A dependent variable is something the researcher observes and measures.
In this example, the independent variable is exposure to the sun – the assumed cause . The dependent variable is the level of happiness – the assumed effect .
Prevent plagiarism, run a free check.
Step 1: ask a question.
Writing a hypothesis begins with a research question that you want to answer. The question should be focused, specific, and researchable within the constraints of your project.
Step 2: Do some preliminary research
Your initial answer to the question should be based on what is already known about the topic. Look for theories and previous studies to help you form educated assumptions about what your research will find.
At this stage, you might construct a conceptual framework to identify which variables you will study and what you think the relationships are between them. Sometimes, you’ll have to operationalise more complex constructs.
Step 3: Formulate your hypothesis
Now you should have some idea of what you expect to find. Write your initial answer to the question in a clear, concise sentence.
Step 4: Refine your hypothesis
You need to make sure your hypothesis is specific and testable. There are various ways of phrasing a hypothesis, but all the terms you use should have clear definitions, and the hypothesis should contain:
- The relevant variables
- The specific group being studied
- The predicted outcome of the experiment or analysis
Step 5: Phrase your hypothesis in three ways
To identify the variables, you can write a simple prediction in if … then form. The first part of the sentence states the independent variable and the second part states the dependent variable.
In academic research, hypotheses are more commonly phrased in terms of correlations or effects, where you directly state the predicted relationship between variables.
If you are comparing two groups, the hypothesis can state what difference you expect to find between them.
Step 6. Write a null hypothesis
If your research involves statistical hypothesis testing , you will also have to write a null hypothesis. The null hypothesis is the default position that there is no association between the variables. The null hypothesis is written as H 0 , while the alternative hypothesis is H 1 or H a .
Hypothesis testing is a formal procedure for investigating our ideas about the world using statistics. It is used by scientists to test specific predictions, called hypotheses , by calculating how likely it is that a pattern or relationship between variables could have arisen by chance.
A hypothesis is not just a guess. It should be based on existing theories and knowledge. It also has to be testable, which means you can support or refute it through scientific research methods (such as experiments, observations, and statistical analysis of data).
A research hypothesis is your proposed answer to your research question. The research hypothesis usually includes an explanation (‘ x affects y because …’).
A statistical hypothesis, on the other hand, is a mathematical statement about a population parameter. Statistical hypotheses always come in pairs: the null and alternative hypotheses. In a well-designed study , the statistical hypotheses correspond logically to the research hypothesis.
Cite this Scribbr article
If you want to cite this source, you can copy and paste the citation or click the ‘Cite this Scribbr article’ button to automatically add the citation to our free Reference Generator.
McCombes, S. (2022, May 06). How to Write a Strong Hypothesis | Guide & Examples. Scribbr. Retrieved 6 May 2024, from https://www.scribbr.co.uk/research-methods/hypothesis-writing/
Is this article helpful?
Shona McCombes
Other students also liked, operationalisation | a guide with examples, pros & cons, what is a conceptual framework | tips & examples, a quick guide to experimental design | 5 steps & examples.
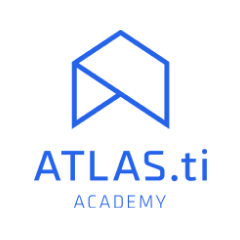
How to Write a Hypothesis: A Step-by-Step Guide
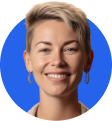
Introduction
An overview of the research hypothesis, different types of hypotheses, variables in a hypothesis, how to formulate an effective research hypothesis, designing a study around your hypothesis.
The scientific method can derive and test predictions as hypotheses. Empirical research can then provide support (or lack thereof) for the hypotheses. Even failure to find support for a hypothesis still represents a valuable contribution to scientific knowledge. Let's look more closely at the idea of the hypothesis and the role it plays in research.
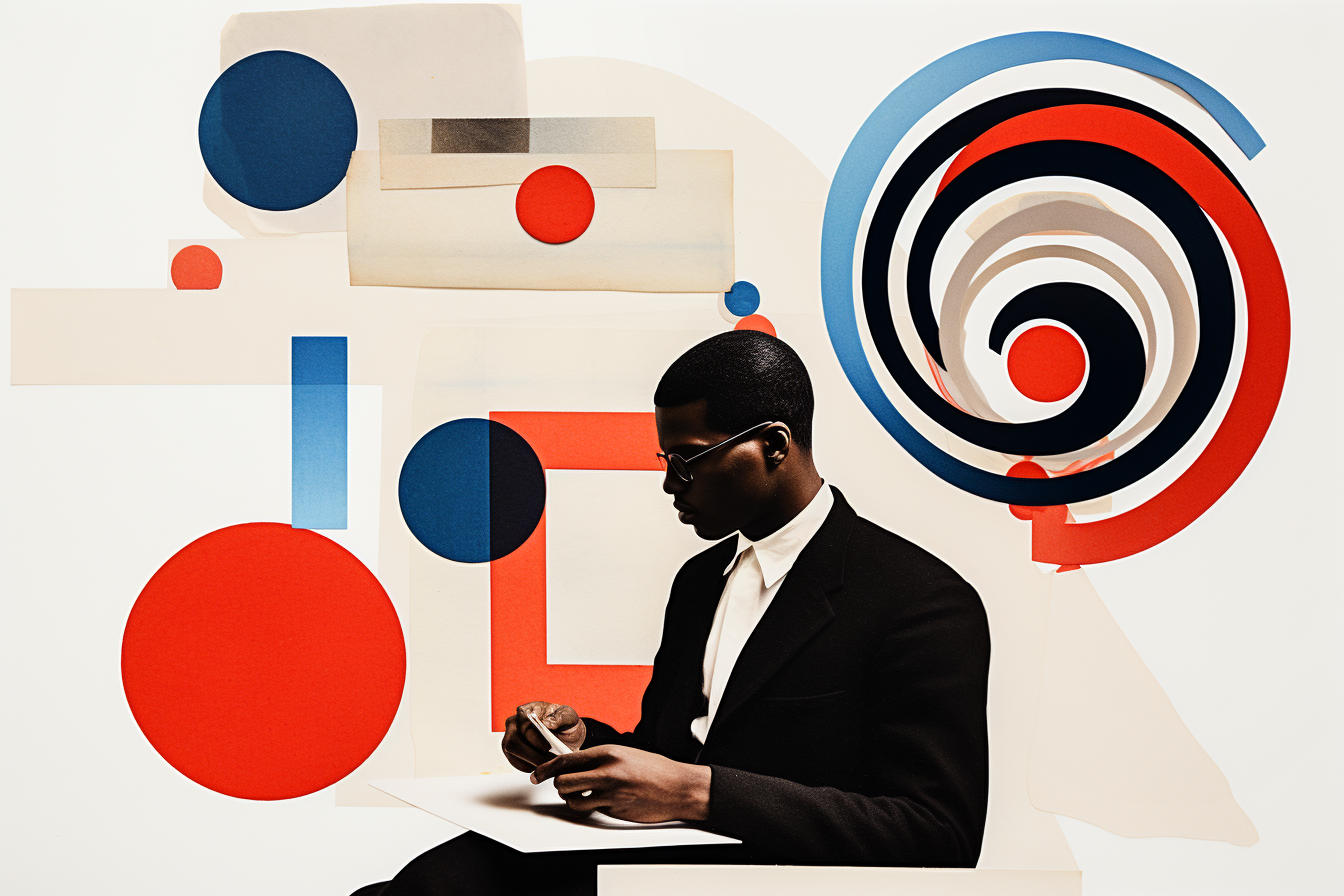
As much as the term exists in everyday language, there is a detailed development that informs the word "hypothesis" when applied to research. A good research hypothesis is informed by prior research and guides research design and data analysis , so it is important to understand how a hypothesis is defined and understood by researchers.
What is the simple definition of a hypothesis?
A hypothesis is a testable prediction about an outcome between two or more variables . It functions as a navigational tool in the research process, directing what you aim to predict and how.
What is the hypothesis for in research?
In research, a hypothesis serves as the cornerstone for your empirical study. It not only lays out what you aim to investigate but also provides a structured approach for your data collection and analysis.
Essentially, it bridges the gap between the theoretical and the empirical, guiding your investigation throughout its course.
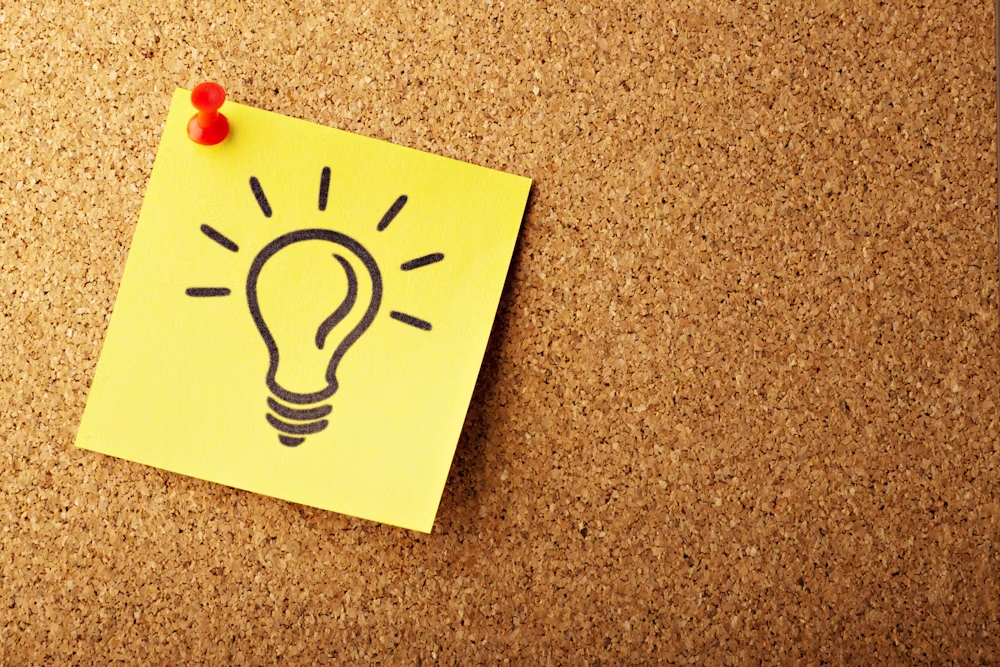
What is an example of a hypothesis?
If you are studying the relationship between physical exercise and mental health, a suitable hypothesis could be: "Regular physical exercise leads to improved mental well-being among adults."
This statement constitutes a specific and testable hypothesis that directly relates to the variables you are investigating.
What makes a good hypothesis?
A good hypothesis possesses several key characteristics. Firstly, it must be testable, allowing you to analyze data through empirical means, such as observation or experimentation, to assess if there is significant support for the hypothesis. Secondly, a hypothesis should be specific and unambiguous, giving a clear understanding of the expected relationship between variables. Lastly, it should be grounded in existing research or theoretical frameworks , ensuring its relevance and applicability.
Understanding the types of hypotheses can greatly enhance how you construct and work with hypotheses. While all hypotheses serve the essential function of guiding your study, there are varying purposes among the types of hypotheses. In addition, all hypotheses stand in contrast to the null hypothesis, or the assumption that there is no significant relationship between the variables .
Here, we explore various kinds of hypotheses to provide you with the tools needed to craft effective hypotheses for your specific research needs. Bear in mind that many of these hypothesis types may overlap with one another, and the specific type that is typically used will likely depend on the area of research and methodology you are following.
Null hypothesis
The null hypothesis is a statement that there is no effect or relationship between the variables being studied. In statistical terms, it serves as the default assumption that any observed differences are due to random chance.
For example, if you're studying the effect of a drug on blood pressure, the null hypothesis might state that the drug has no effect.
Alternative hypothesis
Contrary to the null hypothesis, the alternative hypothesis suggests that there is a significant relationship or effect between variables.
Using the drug example, the alternative hypothesis would posit that the drug does indeed affect blood pressure. This is what researchers aim to prove.
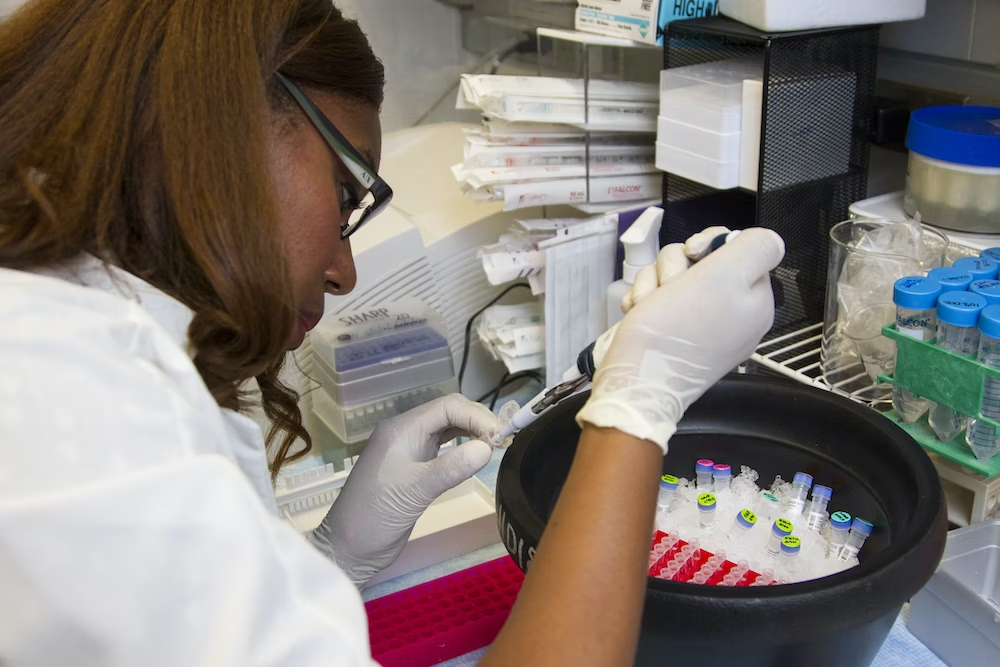
Simple hypothesis
A simple hypothesis makes a prediction about the relationship between two variables, and only two variables.
For example, "Increased study time results in better exam scores." Here, "study time" and "exam scores" are the only variables involved.
Complex hypothesis
A complex hypothesis, as the name suggests, involves more than two variables. For instance, "Increased study time and access to resources result in better exam scores." Here, "study time," "access to resources," and "exam scores" are all variables.
This hypothesis refers to multiple potential mediating variables. Other hypotheses could also include predictions about variables that moderate the relationship between the independent variable and dependent variable .
Directional hypothesis
A directional hypothesis specifies the direction of the expected relationship between variables. For example, "Eating more fruits and vegetables leads to a decrease in heart disease."
Here, the direction of heart disease is explicitly predicted to decrease, due to effects from eating more fruits and vegetables. All hypotheses typically specify the expected direction of the relationship between the independent and dependent variable, such that researchers can test if this prediction holds in their data analysis .
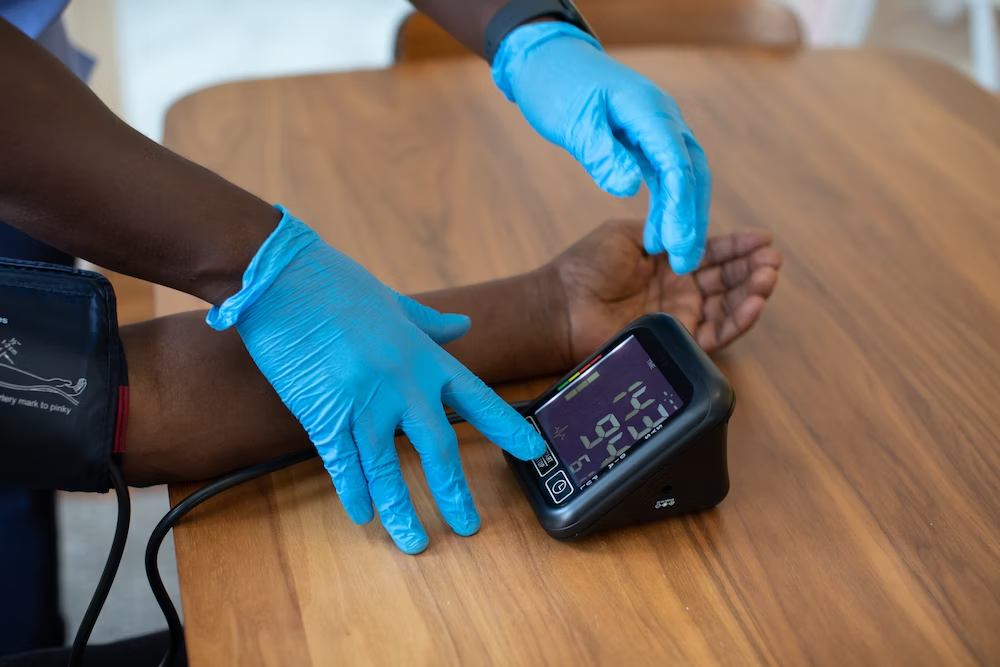
Statistical hypothesis
A statistical hypothesis is one that is testable through statistical methods, providing a numerical value that can be analyzed. This is commonly seen in quantitative research .
For example, "There is a statistically significant difference in test scores between students who study for one hour and those who study for two."
Empirical hypothesis
An empirical hypothesis is derived from observations and is tested through empirical methods, often through experimentation or survey data . Empirical hypotheses may also be assessed with statistical analyses.
For example, "Regular exercise is correlated with a lower incidence of depression," could be tested through surveys that measure exercise frequency and depression levels.
Causal hypothesis
A causal hypothesis proposes that one variable causes a change in another. This type of hypothesis is often tested through controlled experiments.
For example, "Smoking causes lung cancer," assumes a direct causal relationship.
Associative hypothesis
Unlike causal hypotheses, associative hypotheses suggest a relationship between variables but do not imply causation.
For instance, "People who smoke are more likely to get lung cancer," notes an association but doesn't claim that smoking causes lung cancer directly.
Relational hypothesis
A relational hypothesis explores the relationship between two or more variables but doesn't specify the nature of the relationship.
For example, "There is a relationship between diet and heart health," leaves the nature of the relationship (causal, associative, etc.) open to interpretation.
Logical hypothesis
A logical hypothesis is based on sound reasoning and logical principles. It's often used in theoretical research to explore abstract concepts, rather than being based on empirical data.
For example, "If all men are mortal and Socrates is a man, then Socrates is mortal," employs logical reasoning to make its point.
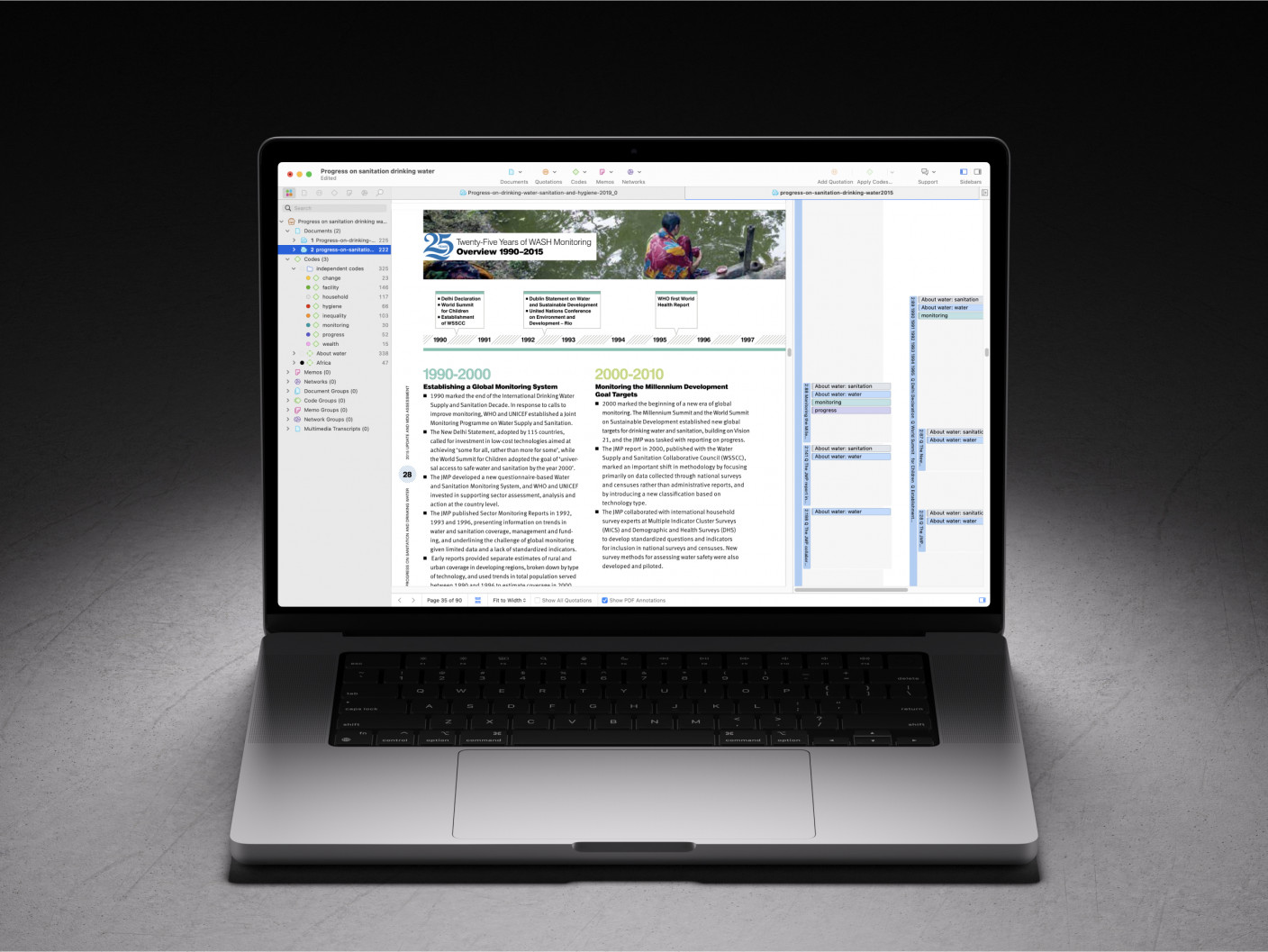
Let ATLAS.ti take you from research question to key insights
Get started with a free trial and see how ATLAS.ti can make the most of your data.
In any research hypothesis, variables play a critical role. These are the elements or factors that the researcher manipulates, controls, or measures. Understanding variables is essential for crafting a clear, testable hypothesis and for the stages of research that follow, such as data collection and analysis.
In the realm of hypotheses, there are generally two types of variables to consider: independent and dependent. Independent variables are what you, as the researcher, manipulate or change in your study. It's considered the cause in the relationship you're investigating. For instance, in a study examining the impact of sleep duration on academic performance, the independent variable would be the amount of sleep participants get.
Conversely, the dependent variable is the outcome you measure to gauge the effect of your manipulation. It's the effect in the cause-and-effect relationship. The dependent variable thus refers to the main outcome of interest in your study. In the same sleep study example, the academic performance, perhaps measured by exam scores or GPA, would be the dependent variable.
Beyond these two primary types, you might also encounter control variables. These are variables that could potentially influence the outcome and are therefore kept constant to isolate the relationship between the independent and dependent variables . For example, in the sleep and academic performance study, control variables could include age, diet, or even the subject of study.
By clearly identifying and understanding the roles of these variables in your hypothesis, you set the stage for a methodologically sound research project. It helps you develop focused research questions, design appropriate experiments or observations, and carry out meaningful data analysis . It's a step that lays the groundwork for the success of your entire study.
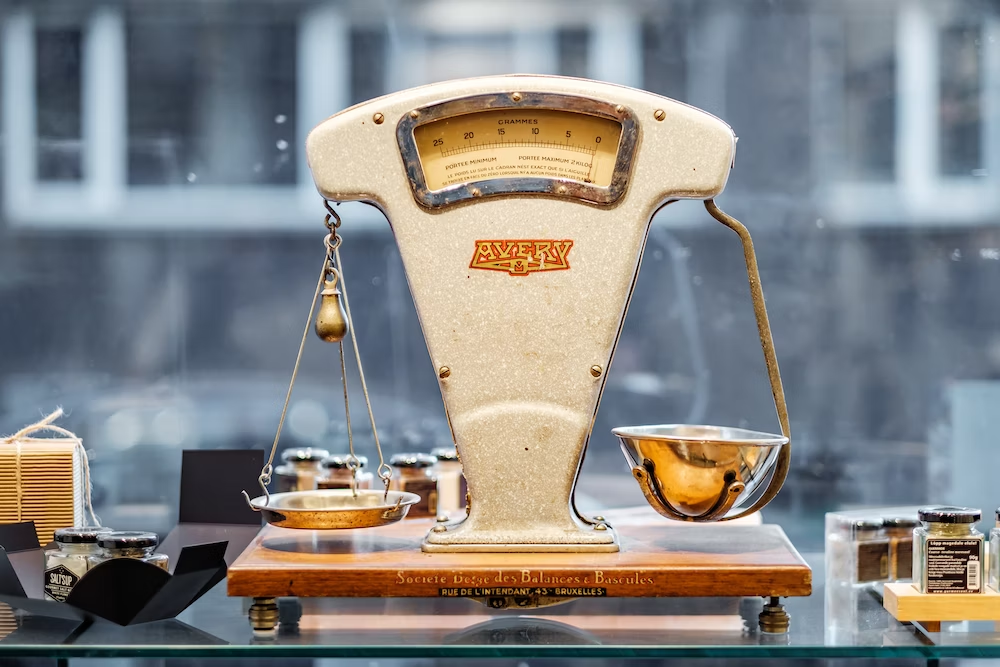
Crafting a strong, testable hypothesis is crucial for the success of any research project. It sets the stage for everything from your study design to data collection and analysis . Below are some key considerations to keep in mind when formulating your hypothesis:
- Be specific : A vague hypothesis can lead to ambiguous results and interpretations . Clearly define your variables and the expected relationship between them.
- Ensure testability : A good hypothesis should be testable through empirical means, whether by observation , experimentation, or other forms of data analysis.
- Ground in literature : Before creating your hypothesis, consult existing research and theories. This not only helps you identify gaps in current knowledge but also gives you valuable context and credibility for crafting your hypothesis.
- Use simple language : While your hypothesis should be conceptually sound, it doesn't have to be complicated. Aim for clarity and simplicity in your wording.
- State direction, if applicable : If your hypothesis involves a directional outcome (e.g., "increase" or "decrease"), make sure to specify this. You also need to think about how you will measure whether or not the outcome moved in the direction you predicted.
- Keep it focused : One of the common pitfalls in hypothesis formulation is trying to answer too many questions at once. Keep your hypothesis focused on a specific issue or relationship.
- Account for control variables : Identify any variables that could potentially impact the outcome and consider how you will control for them in your study.
- Be ethical : Make sure your hypothesis and the methods for testing it comply with ethical standards , particularly if your research involves human or animal subjects.
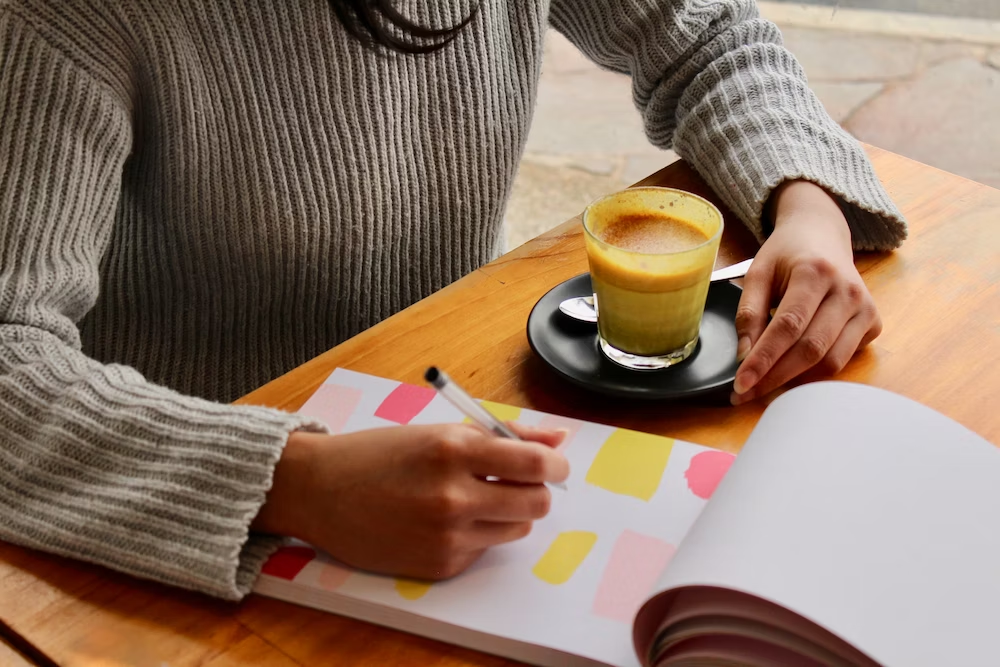
Designing your study involves multiple key phases that help ensure the rigor and validity of your research. Here we discuss these crucial components in more detail.
Literature review
Starting with a comprehensive literature review is essential. This step allows you to understand the existing body of knowledge related to your hypothesis and helps you identify gaps that your research could fill. Your research should aim to contribute some novel understanding to existing literature, and your hypotheses can reflect this. A literature review also provides valuable insights into how similar research projects were executed, thereby helping you fine-tune your own approach.
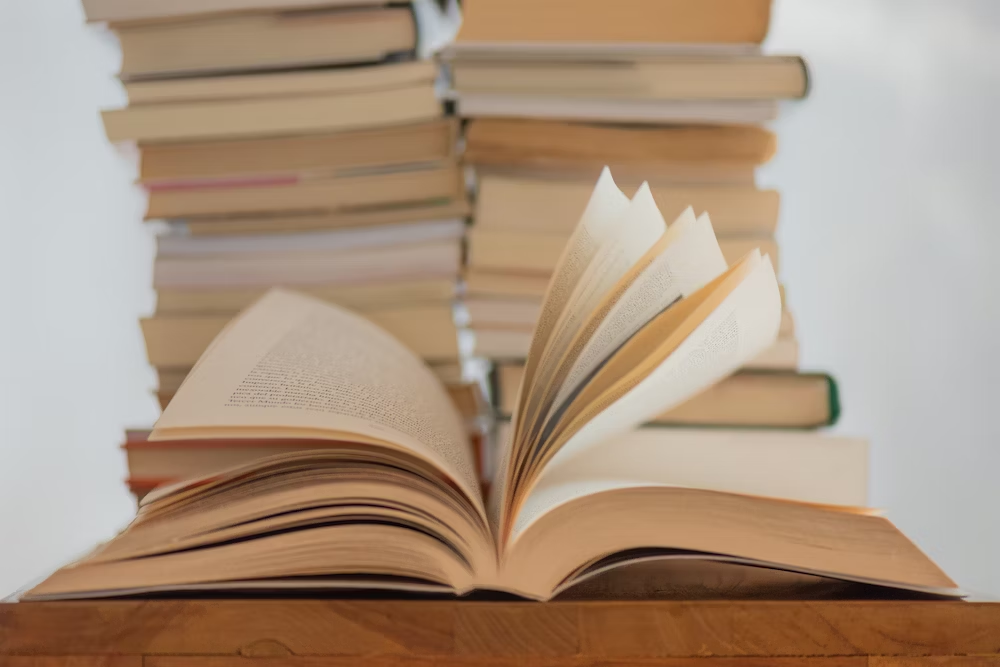
Research methods
Choosing the right research methods is critical. Whether it's a survey, an experiment, or observational study, the methodology should be the most appropriate for testing your hypothesis. Your choice of methods will also depend on whether your research is quantitative, qualitative, or mixed-methods. Make sure the chosen methods align well with the variables you are studying and the type of data you need.
Preliminary research
Before diving into a full-scale study, it’s often beneficial to conduct preliminary research or a pilot study . This allows you to test your research methods on a smaller scale, refine your tools, and identify any potential issues. For instance, a pilot survey can help you determine if your questions are clear and if the survey effectively captures the data you need. This step can save you both time and resources in the long run.
Data analysis
Finally, planning your data analysis in advance is crucial for a successful study. Decide which statistical or analytical tools are most suited for your data type and research questions . For quantitative research, you might opt for t-tests, ANOVA, or regression analyses. For qualitative research , thematic analysis or grounded theory may be more appropriate. This phase is integral for interpreting your results and drawing meaningful conclusions in relation to your research question.
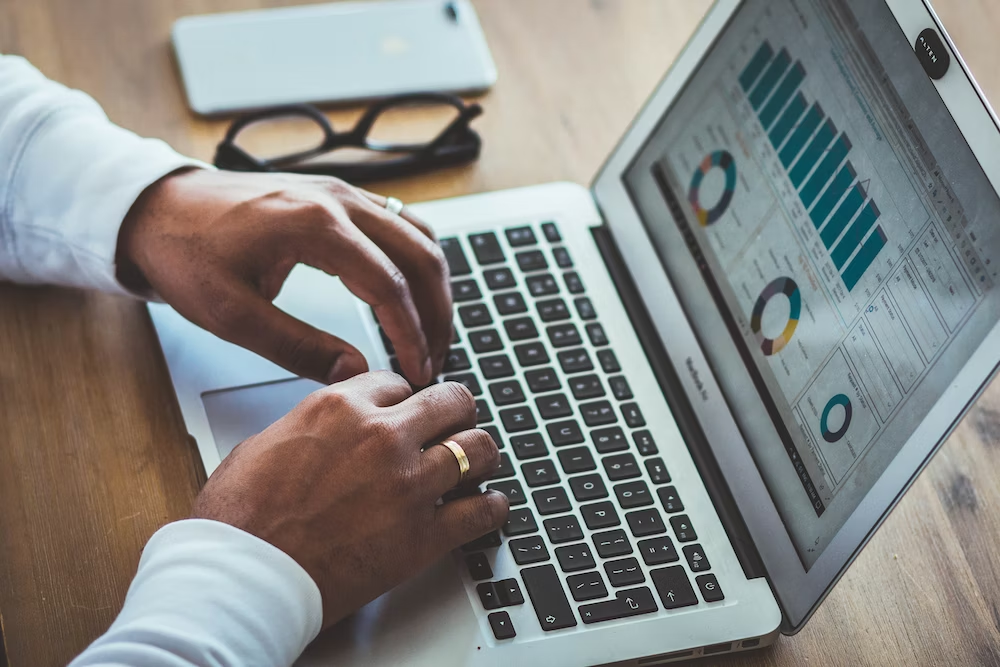
Turn data into evidence for insights with ATLAS.ti
Powerful analysis for your research paper or presentation is at your fingertips starting with a free trial.
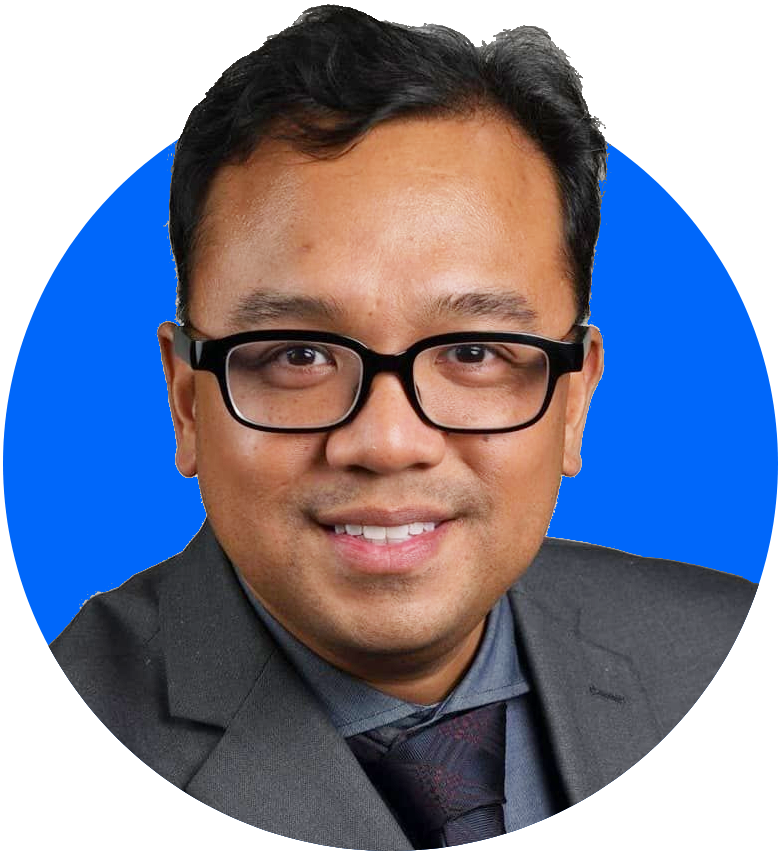
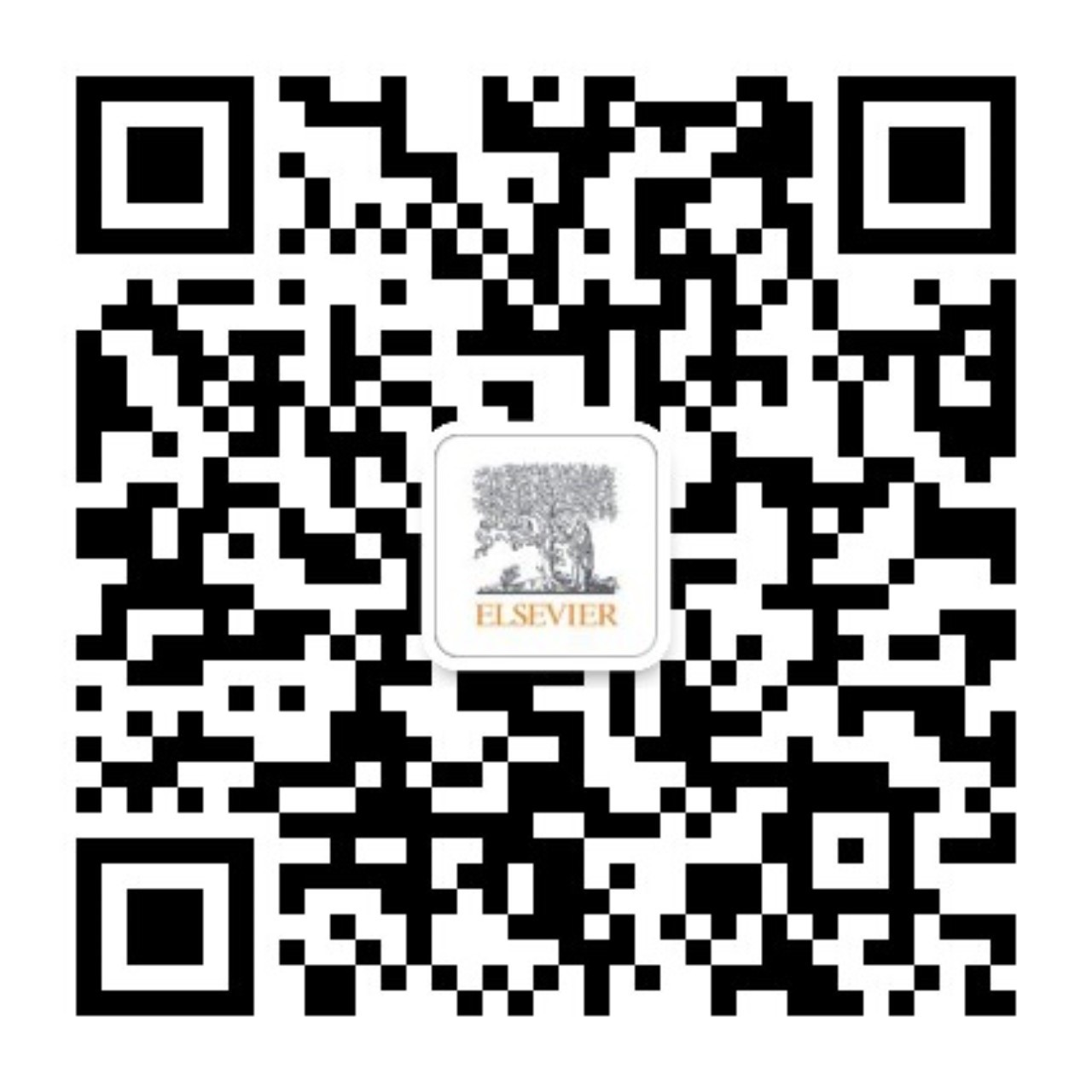
- Manuscript Preparation
What is and How to Write a Good Hypothesis in Research?
- 4 minute read
Table of Contents
One of the most important aspects of conducting research is constructing a strong hypothesis. But what makes a hypothesis in research effective? In this article, we’ll look at the difference between a hypothesis and a research question, as well as the elements of a good hypothesis in research. We’ll also include some examples of effective hypotheses, and what pitfalls to avoid.
What is a Hypothesis in Research?
Simply put, a hypothesis is a research question that also includes the predicted or expected result of the research. Without a hypothesis, there can be no basis for a scientific or research experiment. As such, it is critical that you carefully construct your hypothesis by being deliberate and thorough, even before you set pen to paper. Unless your hypothesis is clearly and carefully constructed, any flaw can have an adverse, and even grave, effect on the quality of your experiment and its subsequent results.
Research Question vs Hypothesis
It’s easy to confuse research questions with hypotheses, and vice versa. While they’re both critical to the Scientific Method, they have very specific differences. Primarily, a research question, just like a hypothesis, is focused and concise. But a hypothesis includes a prediction based on the proposed research, and is designed to forecast the relationship of and between two (or more) variables. Research questions are open-ended, and invite debate and discussion, while hypotheses are closed, e.g. “The relationship between A and B will be C.”
A hypothesis is generally used if your research topic is fairly well established, and you are relatively certain about the relationship between the variables that will be presented in your research. Since a hypothesis is ideally suited for experimental studies, it will, by its very existence, affect the design of your experiment. The research question is typically used for new topics that have not yet been researched extensively. Here, the relationship between different variables is less known. There is no prediction made, but there may be variables explored. The research question can be casual in nature, simply trying to understand if a relationship even exists, descriptive or comparative.
How to Write Hypothesis in Research
Writing an effective hypothesis starts before you even begin to type. Like any task, preparation is key, so you start first by conducting research yourself, and reading all you can about the topic that you plan to research. From there, you’ll gain the knowledge you need to understand where your focus within the topic will lie.
Remember that a hypothesis is a prediction of the relationship that exists between two or more variables. Your job is to write a hypothesis, and design the research, to “prove” whether or not your prediction is correct. A common pitfall is to use judgments that are subjective and inappropriate for the construction of a hypothesis. It’s important to keep the focus and language of your hypothesis objective.
An effective hypothesis in research is clearly and concisely written, and any terms or definitions clarified and defined. Specific language must also be used to avoid any generalities or assumptions.
Use the following points as a checklist to evaluate the effectiveness of your research hypothesis:
- Predicts the relationship and outcome
- Simple and concise – avoid wordiness
- Clear with no ambiguity or assumptions about the readers’ knowledge
- Observable and testable results
- Relevant and specific to the research question or problem
Research Hypothesis Example
Perhaps the best way to evaluate whether or not your hypothesis is effective is to compare it to those of your colleagues in the field. There is no need to reinvent the wheel when it comes to writing a powerful research hypothesis. As you’re reading and preparing your hypothesis, you’ll also read other hypotheses. These can help guide you on what works, and what doesn’t, when it comes to writing a strong research hypothesis.
Here are a few generic examples to get you started.
Eating an apple each day, after the age of 60, will result in a reduction of frequency of physician visits.
Budget airlines are more likely to receive more customer complaints. A budget airline is defined as an airline that offers lower fares and fewer amenities than a traditional full-service airline. (Note that the term “budget airline” is included in the hypothesis.
Workplaces that offer flexible working hours report higher levels of employee job satisfaction than workplaces with fixed hours.
Each of the above examples are specific, observable and measurable, and the statement of prediction can be verified or shown to be false by utilizing standard experimental practices. It should be noted, however, that often your hypothesis will change as your research progresses.
Language Editing Plus
Elsevier’s Language Editing Plus service can help ensure that your research hypothesis is well-designed, and articulates your research and conclusions. Our most comprehensive editing package, you can count on a thorough language review by native-English speakers who are PhDs or PhD candidates. We’ll check for effective logic and flow of your manuscript, as well as document formatting for your chosen journal, reference checks, and much more.
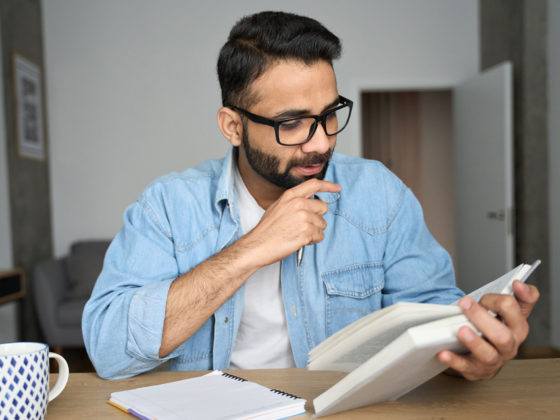
- Research Process
Systematic Literature Review or Literature Review?
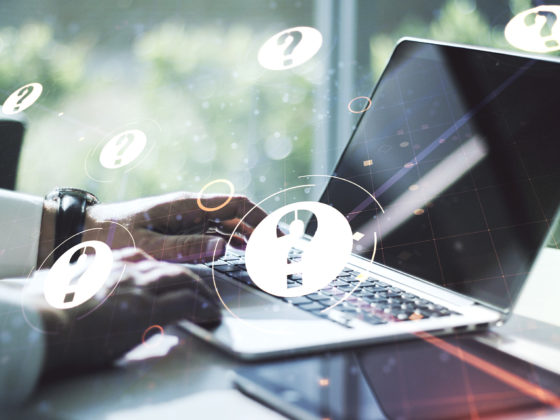
What is a Problem Statement? [with examples]
You may also like.
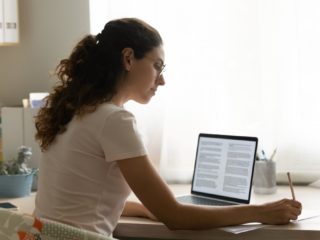
Make Hook, Line, and Sinker: The Art of Crafting Engaging Introductions
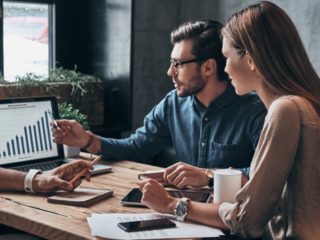
Can Describing Study Limitations Improve the Quality of Your Paper?
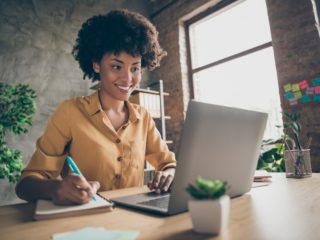
A Guide to Crafting Shorter, Impactful Sentences in Academic Writing
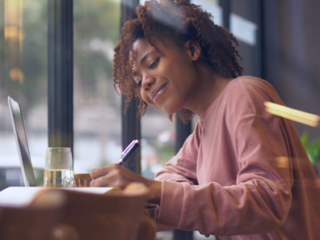
6 Steps to Write an Excellent Discussion in Your Manuscript
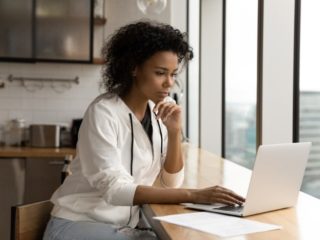
How to Write Clear and Crisp Civil Engineering Papers? Here are 5 Key Tips to Consider
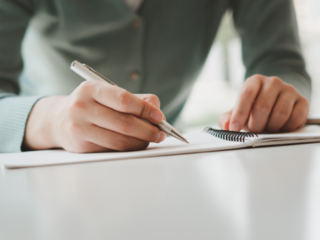
The Clear Path to An Impactful Paper: ②
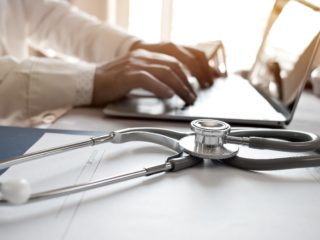
The Essentials of Writing to Communicate Research in Medicine
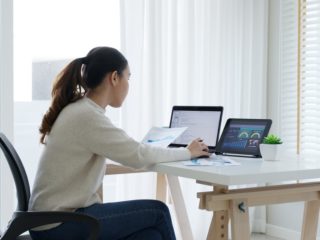
Changing Lines: Sentence Patterns in Academic Writing
Input your search keywords and press Enter.

How to Develop a Good Research Hypothesis
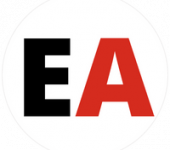
The story of a research study begins by asking a question. Researchers all around the globe are asking curious questions and formulating research hypothesis. However, whether the research study provides an effective conclusion depends on how well one develops a good research hypothesis. Research hypothesis examples could help researchers get an idea as to how to write a good research hypothesis.
This blog will help you understand what is a research hypothesis, its characteristics and, how to formulate a research hypothesis
Table of Contents
What is Hypothesis?
Hypothesis is an assumption or an idea proposed for the sake of argument so that it can be tested. It is a precise, testable statement of what the researchers predict will be outcome of the study. Hypothesis usually involves proposing a relationship between two variables: the independent variable (what the researchers change) and the dependent variable (what the research measures).
What is a Research Hypothesis?
Research hypothesis is a statement that introduces a research question and proposes an expected result. It is an integral part of the scientific method that forms the basis of scientific experiments. Therefore, you need to be careful and thorough when building your research hypothesis. A minor flaw in the construction of your hypothesis could have an adverse effect on your experiment. In research, there is a convention that the hypothesis is written in two forms, the null hypothesis, and the alternative hypothesis (called the experimental hypothesis when the method of investigation is an experiment).
Characteristics of a Good Research Hypothesis
As the hypothesis is specific, there is a testable prediction about what you expect to happen in a study. You may consider drawing hypothesis from previously published research based on the theory.
A good research hypothesis involves more effort than just a guess. In particular, your hypothesis may begin with a question that could be further explored through background research.
To help you formulate a promising research hypothesis, you should ask yourself the following questions:
- Is the language clear and focused?
- What is the relationship between your hypothesis and your research topic?
- Is your hypothesis testable? If yes, then how?
- What are the possible explanations that you might want to explore?
- Does your hypothesis include both an independent and dependent variable?
- Can you manipulate your variables without hampering the ethical standards?
- Does your research predict the relationship and outcome?
- Is your research simple and concise (avoids wordiness)?
- Is it clear with no ambiguity or assumptions about the readers’ knowledge
- Is your research observable and testable results?
- Is it relevant and specific to the research question or problem?
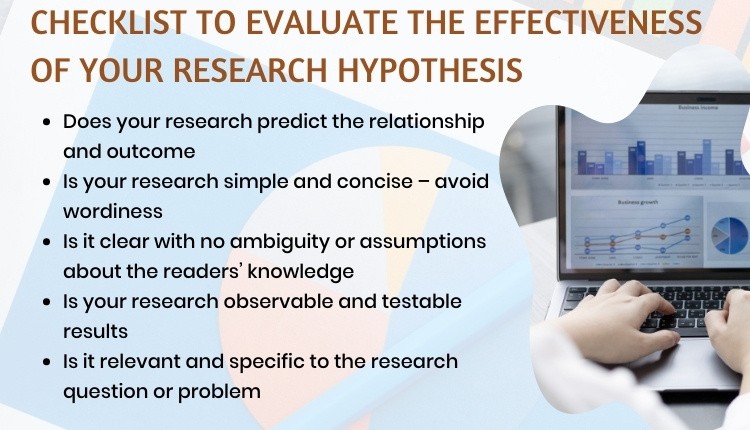
The questions listed above can be used as a checklist to make sure your hypothesis is based on a solid foundation. Furthermore, it can help you identify weaknesses in your hypothesis and revise it if necessary.
Source: Educational Hub
How to formulate a research hypothesis.
A testable hypothesis is not a simple statement. It is rather an intricate statement that needs to offer a clear introduction to a scientific experiment, its intentions, and the possible outcomes. However, there are some important things to consider when building a compelling hypothesis.
1. State the problem that you are trying to solve.
Make sure that the hypothesis clearly defines the topic and the focus of the experiment.
2. Try to write the hypothesis as an if-then statement.
Follow this template: If a specific action is taken, then a certain outcome is expected.
3. Define the variables
Independent variables are the ones that are manipulated, controlled, or changed. Independent variables are isolated from other factors of the study.
Dependent variables , as the name suggests are dependent on other factors of the study. They are influenced by the change in independent variable.
4. Scrutinize the hypothesis
Evaluate assumptions, predictions, and evidence rigorously to refine your understanding.
Types of Research Hypothesis
The types of research hypothesis are stated below:
1. Simple Hypothesis
It predicts the relationship between a single dependent variable and a single independent variable.
2. Complex Hypothesis
It predicts the relationship between two or more independent and dependent variables.
3. Directional Hypothesis
It specifies the expected direction to be followed to determine the relationship between variables and is derived from theory. Furthermore, it implies the researcher’s intellectual commitment to a particular outcome.
4. Non-directional Hypothesis
It does not predict the exact direction or nature of the relationship between the two variables. The non-directional hypothesis is used when there is no theory involved or when findings contradict previous research.
5. Associative and Causal Hypothesis
The associative hypothesis defines interdependency between variables. A change in one variable results in the change of the other variable. On the other hand, the causal hypothesis proposes an effect on the dependent due to manipulation of the independent variable.
6. Null Hypothesis
Null hypothesis states a negative statement to support the researcher’s findings that there is no relationship between two variables. There will be no changes in the dependent variable due the manipulation of the independent variable. Furthermore, it states results are due to chance and are not significant in terms of supporting the idea being investigated.
7. Alternative Hypothesis
It states that there is a relationship between the two variables of the study and that the results are significant to the research topic. An experimental hypothesis predicts what changes will take place in the dependent variable when the independent variable is manipulated. Also, it states that the results are not due to chance and that they are significant in terms of supporting the theory being investigated.
Research Hypothesis Examples of Independent and Dependent Variables
Research Hypothesis Example 1 The greater number of coal plants in a region (independent variable) increases water pollution (dependent variable). If you change the independent variable (building more coal factories), it will change the dependent variable (amount of water pollution).
Research Hypothesis Example 2 What is the effect of diet or regular soda (independent variable) on blood sugar levels (dependent variable)? If you change the independent variable (the type of soda you consume), it will change the dependent variable (blood sugar levels)
You should not ignore the importance of the above steps. The validity of your experiment and its results rely on a robust testable hypothesis. Developing a strong testable hypothesis has few advantages, it compels us to think intensely and specifically about the outcomes of a study. Consequently, it enables us to understand the implication of the question and the different variables involved in the study. Furthermore, it helps us to make precise predictions based on prior research. Hence, forming a hypothesis would be of great value to the research. Here are some good examples of testable hypotheses.
More importantly, you need to build a robust testable research hypothesis for your scientific experiments. A testable hypothesis is a hypothesis that can be proved or disproved as a result of experimentation.
Importance of a Testable Hypothesis
To devise and perform an experiment using scientific method, you need to make sure that your hypothesis is testable. To be considered testable, some essential criteria must be met:
- There must be a possibility to prove that the hypothesis is true.
- There must be a possibility to prove that the hypothesis is false.
- The results of the hypothesis must be reproducible.
Without these criteria, the hypothesis and the results will be vague. As a result, the experiment will not prove or disprove anything significant.
What are your experiences with building hypotheses for scientific experiments? What challenges did you face? How did you overcome these challenges? Please share your thoughts with us in the comments section.
Frequently Asked Questions
The steps to write a research hypothesis are: 1. Stating the problem: Ensure that the hypothesis defines the research problem 2. Writing a hypothesis as an 'if-then' statement: Include the action and the expected outcome of your study by following a ‘if-then’ structure. 3. Defining the variables: Define the variables as Dependent or Independent based on their dependency to other factors. 4. Scrutinizing the hypothesis: Identify the type of your hypothesis
Hypothesis testing is a statistical tool which is used to make inferences about a population data to draw conclusions for a particular hypothesis.
Hypothesis in statistics is a formal statement about the nature of a population within a structured framework of a statistical model. It is used to test an existing hypothesis by studying a population.
Research hypothesis is a statement that introduces a research question and proposes an expected result. It forms the basis of scientific experiments.
The different types of hypothesis in research are: • Null hypothesis: Null hypothesis is a negative statement to support the researcher’s findings that there is no relationship between two variables. • Alternate hypothesis: Alternate hypothesis predicts the relationship between the two variables of the study. • Directional hypothesis: Directional hypothesis specifies the expected direction to be followed to determine the relationship between variables. • Non-directional hypothesis: Non-directional hypothesis does not predict the exact direction or nature of the relationship between the two variables. • Simple hypothesis: Simple hypothesis predicts the relationship between a single dependent variable and a single independent variable. • Complex hypothesis: Complex hypothesis predicts the relationship between two or more independent and dependent variables. • Associative and casual hypothesis: Associative and casual hypothesis predicts the relationship between two or more independent and dependent variables. • Empirical hypothesis: Empirical hypothesis can be tested via experiments and observation. • Statistical hypothesis: A statistical hypothesis utilizes statistical models to draw conclusions about broader populations.

Wow! You really simplified your explanation that even dummies would find it easy to comprehend. Thank you so much.
Thanks a lot for your valuable guidance.
I enjoy reading the post. Hypotheses are actually an intrinsic part in a study. It bridges the research question and the methodology of the study.
Useful piece!
This is awesome.Wow.
It very interesting to read the topic, can you guide me any specific example of hypothesis process establish throw the Demand and supply of the specific product in market
Nicely explained
It is really a useful for me Kindly give some examples of hypothesis
It was a well explained content ,can you please give me an example with the null and alternative hypothesis illustrated
clear and concise. thanks.
So Good so Amazing
Good to learn
Thanks a lot for explaining to my level of understanding
Explained well and in simple terms. Quick read! Thank you
It awesome. It has really positioned me in my research project
Rate this article Cancel Reply
Your email address will not be published.
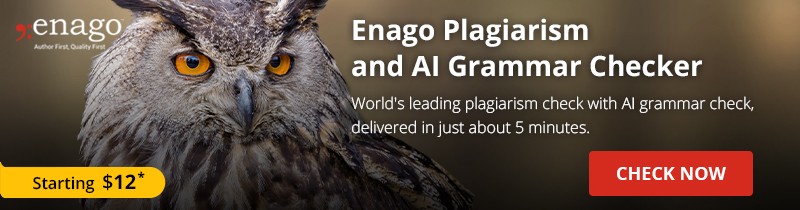
Enago Academy's Most Popular Articles
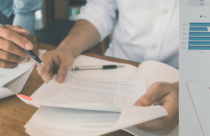
- Reporting Research
Choosing the Right Analytical Approach: Thematic analysis vs. content analysis for data interpretation
In research, choosing the right approach to understand data is crucial for deriving meaningful insights.…
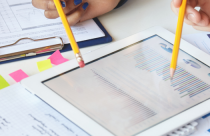
Comparing Cross Sectional and Longitudinal Studies: 5 steps for choosing the right approach
The process of choosing the right research design can put ourselves at the crossroads of…
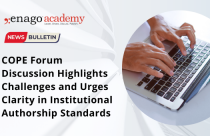
- Industry News
COPE Forum Discussion Highlights Challenges and Urges Clarity in Institutional Authorship Standards
The COPE forum discussion held in December 2023 initiated with a fundamental question — is…
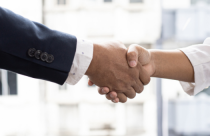
- Career Corner
Unlocking the Power of Networking in Academic Conferences
Embarking on your first academic conference experience? Fear not, we got you covered! Academic conferences…
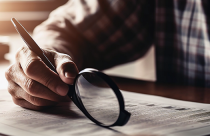
Research Recommendations – Guiding policy-makers for evidence-based decision making
Research recommendations play a crucial role in guiding scholars and researchers toward fruitful avenues of…
Choosing the Right Analytical Approach: Thematic analysis vs. content analysis for…
Comparing Cross Sectional and Longitudinal Studies: 5 steps for choosing the right…
How to Design Effective Research Questionnaires for Robust Findings

Sign-up to read more
Subscribe for free to get unrestricted access to all our resources on research writing and academic publishing including:
- 2000+ blog articles
- 50+ Webinars
- 10+ Expert podcasts
- 50+ Infographics
- 10+ Checklists
- Research Guides
We hate spam too. We promise to protect your privacy and never spam you.
I am looking for Editing/ Proofreading services for my manuscript Tentative date of next journal submission:
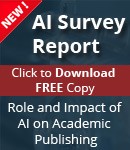
As a researcher, what do you consider most when choosing an image manipulation detector?
How to write a research hypothesis
Last updated
19 January 2023
Reviewed by
Miroslav Damyanov
Start with a broad subject matter that excites you, so your curiosity will motivate your work. Conduct a literature search to determine the range of questions already addressed and spot any holes in the existing research.
Narrow the topics that interest you and determine your research question. Rather than focusing on a hole in the research, you might choose to challenge an existing assumption, a process called problematization. You may also find yourself with a short list of questions or related topics.
Use the FINER method to determine the single problem you'll address with your research. FINER stands for:
I nteresting
You need a feasible research question, meaning that there is a way to address the question. You should find it interesting, but so should a larger audience. Rather than repeating research that others have already conducted, your research hypothesis should test something novel or unique.
The research must fall into accepted ethical parameters as defined by the government of your country and your university or college if you're an academic. You'll also need to come up with a relevant question since your research should provide a contribution to the existing research area.
This process typically narrows your shortlist down to a single problem you'd like to study and the variable you want to test. You're ready to write your hypothesis statements.
Make research less tedious
Dovetail streamlines research to help you uncover and share actionable insights
- Types of research hypotheses
It is important to narrow your topic down to one idea before trying to write your research hypothesis. You'll only test one problem at a time. To do this, you'll write two hypotheses – a null hypothesis (H0) and an alternative hypothesis (Ha).
You'll come across many terms related to developing a research hypothesis or referring to a specific type of hypothesis. Let's take a quick look at these terms.
Null hypothesis
The term null hypothesis refers to a research hypothesis type that assumes no statistically significant relationship exists within a set of observations or data. It represents a claim that assumes that any observed relationship is due to chance. Represented as H0, the null represents the conjecture of the research.
Alternative hypothesis
The alternative hypothesis accompanies the null hypothesis. It states that the situation presented in the null hypothesis is false or untrue, and claims an observed effect in your test. This is typically denoted by Ha or H(n), where “n” stands for the number of alternative hypotheses. You can have more than one alternative hypothesis.
Simple hypothesis
The term simple hypothesis refers to a hypothesis or theory that predicts the relationship between two variables - the independent (predictor) and the dependent (predicted).
Complex hypothesis
The term complex hypothesis refers to a model – either quantitative (mathematical) or qualitative . A complex hypothesis states the surmised relationship between two or more potentially related variables.
Directional hypothesis
When creating a statistical hypothesis, the directional hypothesis (the null hypothesis) states an assumption regarding one parameter of a population. Some academics call this the “one-sided” hypothesis. The alternative hypothesis indicates whether the researcher tests for a positive or negative effect by including either the greater than (">") or less than ("<") sign.
Non-directional hypothesis
We refer to the alternative hypothesis in a statistical research question as a non-directional hypothesis. It includes the not equal ("≠") sign to show that the research tests whether or not an effect exists without specifying the effect's direction (positive or negative).
Associative hypothesis
The term associative hypothesis assumes a link between two variables but stops short of stating that one variable impacts the other. Academic statistical literature asserts in this sense that correlation does not imply causation. So, although the hypothesis notes the correlation between two variables – the independent and dependent - it does not predict how the two interact.
Logical hypothesis
Typically used in philosophy rather than science, researchers can't test a logical hypothesis because the technology or data set doesn't yet exist. A logical hypothesis uses logic as the basis of its assumptions.
In some cases, a logical hypothesis can become an empirical hypothesis once technology provides an opportunity for testing. Until that time, the question remains too expensive or complex to address. Note that a logical hypothesis is not a statistical hypothesis.
Empirical hypothesis
When we consider the opposite of a logical hypothesis, we call this an empirical or working hypothesis. This type of hypothesis considers a scientifically measurable question. A researcher can consider and test an empirical hypothesis through replicable tests, observations, and measurements.
Statistical hypothesis
The term statistical hypothesis refers to a test of a theory that uses representative statistical models to test relationships between variables to draw conclusions regarding a large population. This requires an existing large data set, commonly referred to as big data, or implementing a survey to obtain original statistical information to form a data set for the study.
Testing this type of hypothesis requires the use of random samples. Note that the null and alternative hypotheses are used in statistical hypothesis testing.
Causal hypothesis
The term causal hypothesis refers to a research hypothesis that tests a cause-and-effect relationship. A causal hypothesis is utilized when conducting experimental or quasi-experimental research.
Descriptive hypothesis
The term descriptive hypothesis refers to a research hypothesis used in non-experimental research, specifying an influence in the relationship between two variables.
- What makes an effective research hypothesis?
An effective research hypothesis offers a clearly defined, specific statement, using simple wording that contains no assumptions or generalizations, and that you can test. A well-written hypothesis should predict the tested relationship and its outcome. It contains zero ambiguity and offers results you can observe and test.
The research hypothesis should address a question relevant to a research area. Overall, your research hypothesis needs the following essentials:
Hypothesis Essential #1: Specificity & Clarity
Hypothesis Essential #2: Testability (Provability)
- How to develop a good research hypothesis
In developing your hypothesis statements, you must pre-plan some of your statistical analysis. Once you decide on your problem to examine, determine three aspects:
the parameter you'll test
the test's direction (left-tailed, right-tailed, or non-directional)
the hypothesized parameter value
Any quantitative research includes a hypothesized parameter value of a mean, a proportion, or the difference between two proportions. Here's how to note each parameter:
Single mean (μ)
Paired means (μd)
Single proportion (p)
Difference between two independent means (μ1−μ2)
Difference between two proportions (p1−p2)
Simple linear regression slope (β)
Correlation (ρ)
Defining these parameters and determining whether you want to test the mean, proportion, or differences helps you determine the statistical tests you'll conduct to analyze your data. When writing your hypothesis, you only need to decide which parameter to test and in what overarching way.
The null research hypothesis must include everyday language, in a single sentence, stating the problem you want to solve. Write it as an if-then statement with defined variables. Write an alternative research hypothesis that states the opposite.
- What is the correct format for writing a hypothesis?
The following example shows the proper format and textual content of a hypothesis. It follows commonly accepted academic standards.
Null hypothesis (H0): High school students who participate in varsity sports as opposed to those who do not, fail to score higher on leadership tests than students who do not participate.
Alternative hypothesis (H1): High school students who play a varsity sport as opposed to those who do not participate in team athletics will score higher on leadership tests than students who do not participate in athletics.
The research question tests the correlation between varsity sports participation and leadership qualities expressed as a score on leadership tests. It compares the population of athletes to non-athletes.
- What are the five steps of a hypothesis?
Once you decide on the specific problem or question you want to address, you can write your research hypothesis. Use this five-step system to hone your null hypothesis and generate your alternative hypothesis.
Step 1 : Create your research question. This topic should interest and excite you; answering it provides relevant information to an industry or academic area.
Step 2 : Conduct a literature review to gather essential existing research.
Step 3 : Write a clear, strong, simply worded sentence that explains your test parameter, test direction, and hypothesized parameter.
Step 4 : Read it a few times. Have others read it and ask them what they think it means. Refine your statement accordingly until it becomes understandable to everyone. While not everyone can or will comprehend every research study conducted, any person from the general population should be able to read your hypothesis and alternative hypothesis and understand the essential question you want to answer.
Step 5 : Re-write your null hypothesis until it reads simply and understandably. Write your alternative hypothesis.
What is the Red Queen hypothesis?
Some hypotheses are well-known, such as the Red Queen hypothesis. Choose your wording carefully, since you could become like the famed scientist Dr. Leigh Van Valen. In 1973, Dr. Van Valen proposed the Red Queen hypothesis to describe coevolutionary activity, specifically reciprocal evolutionary effects between species to explain extinction rates in the fossil record.
Essentially, Van Valen theorized that to survive, each species remains in a constant state of adaptation, evolution, and proliferation, and constantly competes for survival alongside other species doing the same. Only by doing this can a species avoid extinction. Van Valen took the hypothesis title from the Lewis Carroll book, "Through the Looking Glass," which contains a key character named the Red Queen who explains to Alice that for all of her running, she's merely running in place.
- Getting started with your research
In conclusion, once you write your null hypothesis (H0) and an alternative hypothesis (Ha), you’ve essentially authored the elevator pitch of your research. These two one-sentence statements describe your topic in simple, understandable terms that both professionals and laymen can understand. They provide the starting point of your research project.
Editor’s picks
Last updated: 11 January 2024
Last updated: 15 January 2024
Last updated: 25 November 2023
Last updated: 12 May 2023
Last updated: 30 April 2024
Last updated: 18 May 2023
Last updated: 10 April 2023
Latest articles
Related topics, .css-je19u9{-webkit-align-items:flex-end;-webkit-box-align:flex-end;-ms-flex-align:flex-end;align-items:flex-end;display:-webkit-box;display:-webkit-flex;display:-ms-flexbox;display:flex;-webkit-flex-direction:row;-ms-flex-direction:row;flex-direction:row;-webkit-box-flex-wrap:wrap;-webkit-flex-wrap:wrap;-ms-flex-wrap:wrap;flex-wrap:wrap;-webkit-box-pack:center;-ms-flex-pack:center;-webkit-justify-content:center;justify-content:center;row-gap:0;text-align:center;max-width:671px;}@media (max-width: 1079px){.css-je19u9{max-width:400px;}.css-je19u9>span{white-space:pre;}}@media (max-width: 799px){.css-je19u9{max-width:400px;}.css-je19u9>span{white-space:pre;}} decide what to .css-1kiodld{max-height:56px;display:-webkit-box;display:-webkit-flex;display:-ms-flexbox;display:flex;-webkit-align-items:center;-webkit-box-align:center;-ms-flex-align:center;align-items:center;}@media (max-width: 1079px){.css-1kiodld{display:none;}} build next, decide what to build next.

Users report unexpectedly high data usage, especially during streaming sessions.

Users find it hard to navigate from the home page to relevant playlists in the app.

It would be great to have a sleep timer feature, especially for bedtime listening.

I need better filters to find the songs or artists I’m looking for.
Log in or sign up
Get started for free

What Is A Research (Scientific) Hypothesis? A plain-language explainer + examples
By: Derek Jansen (MBA) | Reviewed By: Dr Eunice Rautenbach | June 2020
If you’re new to the world of research, or it’s your first time writing a dissertation or thesis, you’re probably noticing that the words “research hypothesis” and “scientific hypothesis” are used quite a bit, and you’re wondering what they mean in a research context .
“Hypothesis” is one of those words that people use loosely, thinking they understand what it means. However, it has a very specific meaning within academic research. So, it’s important to understand the exact meaning before you start hypothesizing.
Research Hypothesis 101
- What is a hypothesis ?
- What is a research hypothesis (scientific hypothesis)?
- Requirements for a research hypothesis
- Definition of a research hypothesis
- The null hypothesis
What is a hypothesis?
Let’s start with the general definition of a hypothesis (not a research hypothesis or scientific hypothesis), according to the Cambridge Dictionary:
Hypothesis: an idea or explanation for something that is based on known facts but has not yet been proved.
In other words, it’s a statement that provides an explanation for why or how something works, based on facts (or some reasonable assumptions), but that has not yet been specifically tested . For example, a hypothesis might look something like this:
Hypothesis: sleep impacts academic performance.
This statement predicts that academic performance will be influenced by the amount and/or quality of sleep a student engages in – sounds reasonable, right? It’s based on reasonable assumptions , underpinned by what we currently know about sleep and health (from the existing literature). So, loosely speaking, we could call it a hypothesis, at least by the dictionary definition.
But that’s not good enough…
Unfortunately, that’s not quite sophisticated enough to describe a research hypothesis (also sometimes called a scientific hypothesis), and it wouldn’t be acceptable in a dissertation, thesis or research paper . In the world of academic research, a statement needs a few more criteria to constitute a true research hypothesis .
What is a research hypothesis?
A research hypothesis (also called a scientific hypothesis) is a statement about the expected outcome of a study (for example, a dissertation or thesis). To constitute a quality hypothesis, the statement needs to have three attributes – specificity , clarity and testability .
Let’s take a look at these more closely.
Need a helping hand?
Hypothesis Essential #1: Specificity & Clarity
A good research hypothesis needs to be extremely clear and articulate about both what’ s being assessed (who or what variables are involved ) and the expected outcome (for example, a difference between groups, a relationship between variables, etc.).
Let’s stick with our sleepy students example and look at how this statement could be more specific and clear.
Hypothesis: Students who sleep at least 8 hours per night will, on average, achieve higher grades in standardised tests than students who sleep less than 8 hours a night.
As you can see, the statement is very specific as it identifies the variables involved (sleep hours and test grades), the parties involved (two groups of students), as well as the predicted relationship type (a positive relationship). There’s no ambiguity or uncertainty about who or what is involved in the statement, and the expected outcome is clear.
Contrast that to the original hypothesis we looked at – “Sleep impacts academic performance” – and you can see the difference. “Sleep” and “academic performance” are both comparatively vague , and there’s no indication of what the expected relationship direction is (more sleep or less sleep). As you can see, specificity and clarity are key.
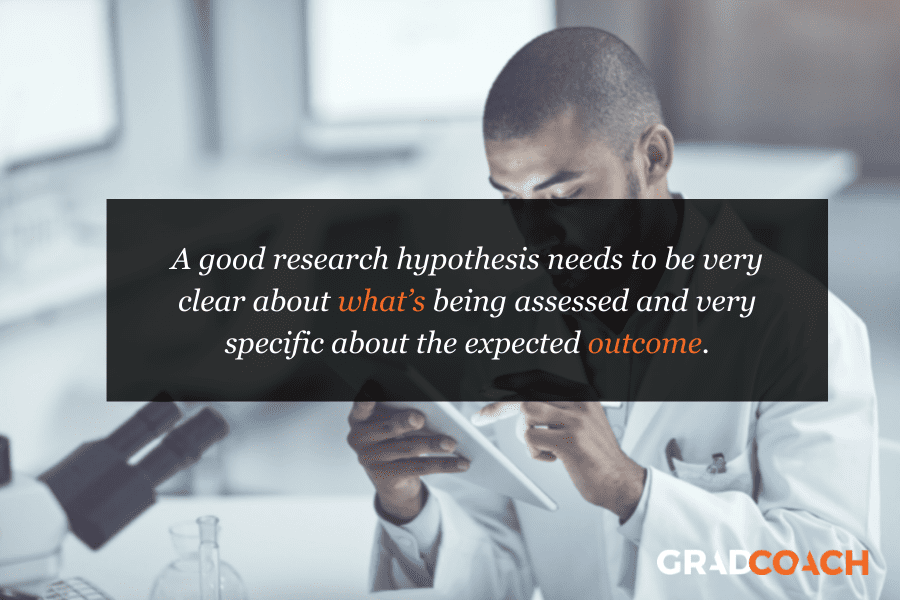
Hypothesis Essential #2: Testability (Provability)
A statement must be testable to qualify as a research hypothesis. In other words, there needs to be a way to prove (or disprove) the statement. If it’s not testable, it’s not a hypothesis – simple as that.
For example, consider the hypothesis we mentioned earlier:
Hypothesis: Students who sleep at least 8 hours per night will, on average, achieve higher grades in standardised tests than students who sleep less than 8 hours a night.
We could test this statement by undertaking a quantitative study involving two groups of students, one that gets 8 or more hours of sleep per night for a fixed period, and one that gets less. We could then compare the standardised test results for both groups to see if there’s a statistically significant difference.
Again, if you compare this to the original hypothesis we looked at – “Sleep impacts academic performance” – you can see that it would be quite difficult to test that statement, primarily because it isn’t specific enough. How much sleep? By who? What type of academic performance?
So, remember the mantra – if you can’t test it, it’s not a hypothesis 🙂
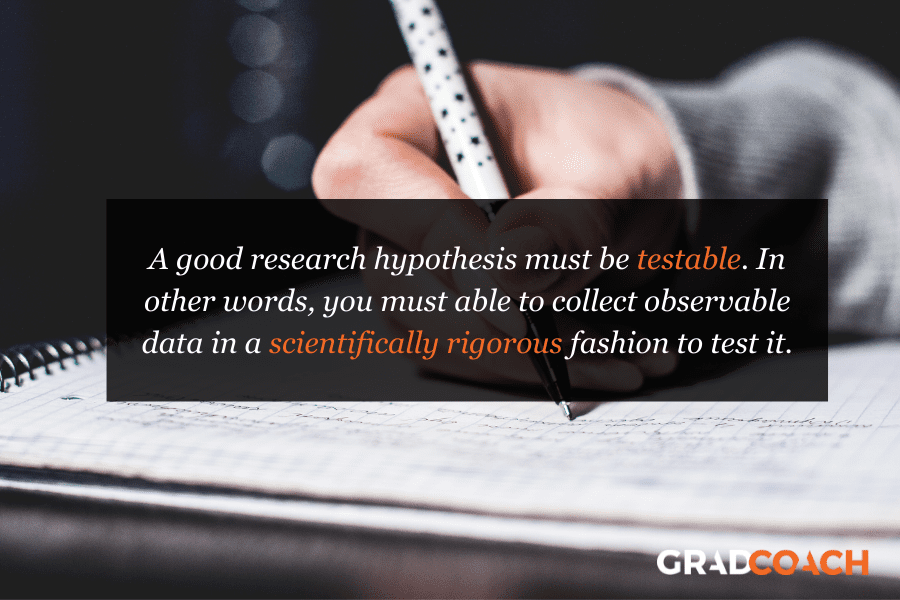
Defining A Research Hypothesis
You’re still with us? Great! Let’s recap and pin down a clear definition of a hypothesis.
A research hypothesis (or scientific hypothesis) is a statement about an expected relationship between variables, or explanation of an occurrence, that is clear, specific and testable.
So, when you write up hypotheses for your dissertation or thesis, make sure that they meet all these criteria. If you do, you’ll not only have rock-solid hypotheses but you’ll also ensure a clear focus for your entire research project.
What about the null hypothesis?
You may have also heard the terms null hypothesis , alternative hypothesis, or H-zero thrown around. At a simple level, the null hypothesis is the counter-proposal to the original hypothesis.
For example, if the hypothesis predicts that there is a relationship between two variables (for example, sleep and academic performance), the null hypothesis would predict that there is no relationship between those variables.
At a more technical level, the null hypothesis proposes that no statistical significance exists in a set of given observations and that any differences are due to chance alone.
And there you have it – hypotheses in a nutshell.
If you have any questions, be sure to leave a comment below and we’ll do our best to help you. If you need hands-on help developing and testing your hypotheses, consider our private coaching service , where we hold your hand through the research journey.
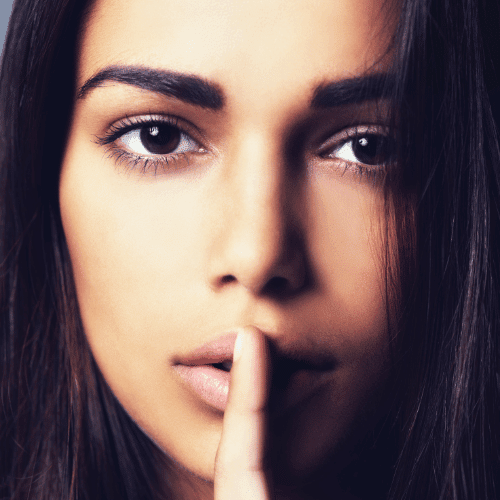
Psst... there’s more!
This post was based on one of our popular Research Bootcamps . If you're working on a research project, you'll definitely want to check this out ...
You Might Also Like:
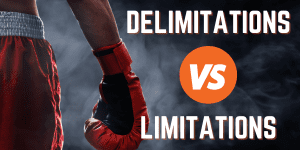
16 Comments
Very useful information. I benefit more from getting more information in this regard.
Very great insight,educative and informative. Please give meet deep critics on many research data of public international Law like human rights, environment, natural resources, law of the sea etc
In a book I read a distinction is made between null, research, and alternative hypothesis. As far as I understand, alternative and research hypotheses are the same. Can you please elaborate? Best Afshin
This is a self explanatory, easy going site. I will recommend this to my friends and colleagues.
Very good definition. How can I cite your definition in my thesis? Thank you. Is nul hypothesis compulsory in a research?
It’s a counter-proposal to be proven as a rejection
Please what is the difference between alternate hypothesis and research hypothesis?
It is a very good explanation. However, it limits hypotheses to statistically tasteable ideas. What about for qualitative researches or other researches that involve quantitative data that don’t need statistical tests?
In qualitative research, one typically uses propositions, not hypotheses.
could you please elaborate it more
I’ve benefited greatly from these notes, thank you.
This is very helpful
well articulated ideas are presented here, thank you for being reliable sources of information
Excellent. Thanks for being clear and sound about the research methodology and hypothesis (quantitative research)
I have only a simple question regarding the null hypothesis. – Is the null hypothesis (Ho) known as the reversible hypothesis of the alternative hypothesis (H1? – How to test it in academic research?
this is very important note help me much more
Trackbacks/Pingbacks
- What Is Research Methodology? Simple Definition (With Examples) - Grad Coach - […] Contrasted to this, a quantitative methodology is typically used when the research aims and objectives are confirmatory in nature. For example,…
Submit a Comment Cancel reply
Your email address will not be published. Required fields are marked *
Save my name, email, and website in this browser for the next time I comment.
- Print Friendly
- Bipolar Disorder
- Therapy Center
- When To See a Therapist
- Types of Therapy
- Best Online Therapy
- Best Couples Therapy
- Best Family Therapy
- Managing Stress
- Sleep and Dreaming
- Understanding Emotions
- Self-Improvement
- Healthy Relationships
- Student Resources
- Personality Types
- Guided Meditations
- Verywell Mind Insights
- 2024 Verywell Mind 25
- Mental Health in the Classroom
- Editorial Process
- Meet Our Review Board
- Crisis Support
How to Write a Great Hypothesis
Hypothesis Definition, Format, Examples, and Tips
Kendra Cherry, MS, is a psychosocial rehabilitation specialist, psychology educator, and author of the "Everything Psychology Book."
:max_bytes(150000):strip_icc():format(webp)/IMG_9791-89504ab694d54b66bbd72cb84ffb860e.jpg)
Amy Morin, LCSW, is a psychotherapist and international bestselling author. Her books, including "13 Things Mentally Strong People Don't Do," have been translated into more than 40 languages. Her TEDx talk, "The Secret of Becoming Mentally Strong," is one of the most viewed talks of all time.
:max_bytes(150000):strip_icc():format(webp)/VW-MIND-Amy-2b338105f1ee493f94d7e333e410fa76.jpg)
Verywell / Alex Dos Diaz
- The Scientific Method
Hypothesis Format
Falsifiability of a hypothesis.
- Operationalization
Hypothesis Types
Hypotheses examples.
- Collecting Data
A hypothesis is a tentative statement about the relationship between two or more variables. It is a specific, testable prediction about what you expect to happen in a study. It is a preliminary answer to your question that helps guide the research process.
Consider a study designed to examine the relationship between sleep deprivation and test performance. The hypothesis might be: "This study is designed to assess the hypothesis that sleep-deprived people will perform worse on a test than individuals who are not sleep-deprived."
At a Glance
A hypothesis is crucial to scientific research because it offers a clear direction for what the researchers are looking to find. This allows them to design experiments to test their predictions and add to our scientific knowledge about the world. This article explores how a hypothesis is used in psychology research, how to write a good hypothesis, and the different types of hypotheses you might use.
The Hypothesis in the Scientific Method
In the scientific method , whether it involves research in psychology, biology, or some other area, a hypothesis represents what the researchers think will happen in an experiment. The scientific method involves the following steps:
- Forming a question
- Performing background research
- Creating a hypothesis
- Designing an experiment
- Collecting data
- Analyzing the results
- Drawing conclusions
- Communicating the results
The hypothesis is a prediction, but it involves more than a guess. Most of the time, the hypothesis begins with a question which is then explored through background research. At this point, researchers then begin to develop a testable hypothesis.
Unless you are creating an exploratory study, your hypothesis should always explain what you expect to happen.
In a study exploring the effects of a particular drug, the hypothesis might be that researchers expect the drug to have some type of effect on the symptoms of a specific illness. In psychology, the hypothesis might focus on how a certain aspect of the environment might influence a particular behavior.
Remember, a hypothesis does not have to be correct. While the hypothesis predicts what the researchers expect to see, the goal of the research is to determine whether this guess is right or wrong. When conducting an experiment, researchers might explore numerous factors to determine which ones might contribute to the ultimate outcome.
In many cases, researchers may find that the results of an experiment do not support the original hypothesis. When writing up these results, the researchers might suggest other options that should be explored in future studies.
In many cases, researchers might draw a hypothesis from a specific theory or build on previous research. For example, prior research has shown that stress can impact the immune system. So a researcher might hypothesize: "People with high-stress levels will be more likely to contract a common cold after being exposed to the virus than people who have low-stress levels."
In other instances, researchers might look at commonly held beliefs or folk wisdom. "Birds of a feather flock together" is one example of folk adage that a psychologist might try to investigate. The researcher might pose a specific hypothesis that "People tend to select romantic partners who are similar to them in interests and educational level."
Elements of a Good Hypothesis
So how do you write a good hypothesis? When trying to come up with a hypothesis for your research or experiments, ask yourself the following questions:
- Is your hypothesis based on your research on a topic?
- Can your hypothesis be tested?
- Does your hypothesis include independent and dependent variables?
Before you come up with a specific hypothesis, spend some time doing background research. Once you have completed a literature review, start thinking about potential questions you still have. Pay attention to the discussion section in the journal articles you read . Many authors will suggest questions that still need to be explored.
How to Formulate a Good Hypothesis
To form a hypothesis, you should take these steps:
- Collect as many observations about a topic or problem as you can.
- Evaluate these observations and look for possible causes of the problem.
- Create a list of possible explanations that you might want to explore.
- After you have developed some possible hypotheses, think of ways that you could confirm or disprove each hypothesis through experimentation. This is known as falsifiability.
In the scientific method , falsifiability is an important part of any valid hypothesis. In order to test a claim scientifically, it must be possible that the claim could be proven false.
Students sometimes confuse the idea of falsifiability with the idea that it means that something is false, which is not the case. What falsifiability means is that if something was false, then it is possible to demonstrate that it is false.
One of the hallmarks of pseudoscience is that it makes claims that cannot be refuted or proven false.
The Importance of Operational Definitions
A variable is a factor or element that can be changed and manipulated in ways that are observable and measurable. However, the researcher must also define how the variable will be manipulated and measured in the study.
Operational definitions are specific definitions for all relevant factors in a study. This process helps make vague or ambiguous concepts detailed and measurable.
For example, a researcher might operationally define the variable " test anxiety " as the results of a self-report measure of anxiety experienced during an exam. A "study habits" variable might be defined by the amount of studying that actually occurs as measured by time.
These precise descriptions are important because many things can be measured in various ways. Clearly defining these variables and how they are measured helps ensure that other researchers can replicate your results.
Replicability
One of the basic principles of any type of scientific research is that the results must be replicable.
Replication means repeating an experiment in the same way to produce the same results. By clearly detailing the specifics of how the variables were measured and manipulated, other researchers can better understand the results and repeat the study if needed.
Some variables are more difficult than others to define. For example, how would you operationally define a variable such as aggression ? For obvious ethical reasons, researchers cannot create a situation in which a person behaves aggressively toward others.
To measure this variable, the researcher must devise a measurement that assesses aggressive behavior without harming others. The researcher might utilize a simulated task to measure aggressiveness in this situation.
Hypothesis Checklist
- Does your hypothesis focus on something that you can actually test?
- Does your hypothesis include both an independent and dependent variable?
- Can you manipulate the variables?
- Can your hypothesis be tested without violating ethical standards?
The hypothesis you use will depend on what you are investigating and hoping to find. Some of the main types of hypotheses that you might use include:
- Simple hypothesis : This type of hypothesis suggests there is a relationship between one independent variable and one dependent variable.
- Complex hypothesis : This type suggests a relationship between three or more variables, such as two independent and dependent variables.
- Null hypothesis : This hypothesis suggests no relationship exists between two or more variables.
- Alternative hypothesis : This hypothesis states the opposite of the null hypothesis.
- Statistical hypothesis : This hypothesis uses statistical analysis to evaluate a representative population sample and then generalizes the findings to the larger group.
- Logical hypothesis : This hypothesis assumes a relationship between variables without collecting data or evidence.
A hypothesis often follows a basic format of "If {this happens} then {this will happen}." One way to structure your hypothesis is to describe what will happen to the dependent variable if you change the independent variable .
The basic format might be: "If {these changes are made to a certain independent variable}, then we will observe {a change in a specific dependent variable}."
A few examples of simple hypotheses:
- "Students who eat breakfast will perform better on a math exam than students who do not eat breakfast."
- "Students who experience test anxiety before an English exam will get lower scores than students who do not experience test anxiety."
- "Motorists who talk on the phone while driving will be more likely to make errors on a driving course than those who do not talk on the phone."
- "Children who receive a new reading intervention will have higher reading scores than students who do not receive the intervention."
Examples of a complex hypothesis include:
- "People with high-sugar diets and sedentary activity levels are more likely to develop depression."
- "Younger people who are regularly exposed to green, outdoor areas have better subjective well-being than older adults who have limited exposure to green spaces."
Examples of a null hypothesis include:
- "There is no difference in anxiety levels between people who take St. John's wort supplements and those who do not."
- "There is no difference in scores on a memory recall task between children and adults."
- "There is no difference in aggression levels between children who play first-person shooter games and those who do not."
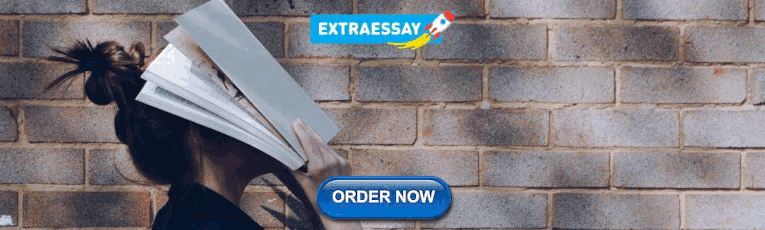
Examples of an alternative hypothesis:
- "People who take St. John's wort supplements will have less anxiety than those who do not."
- "Adults will perform better on a memory task than children."
- "Children who play first-person shooter games will show higher levels of aggression than children who do not."
Collecting Data on Your Hypothesis
Once a researcher has formed a testable hypothesis, the next step is to select a research design and start collecting data. The research method depends largely on exactly what they are studying. There are two basic types of research methods: descriptive research and experimental research.
Descriptive Research Methods
Descriptive research such as case studies , naturalistic observations , and surveys are often used when conducting an experiment is difficult or impossible. These methods are best used to describe different aspects of a behavior or psychological phenomenon.
Once a researcher has collected data using descriptive methods, a correlational study can examine how the variables are related. This research method might be used to investigate a hypothesis that is difficult to test experimentally.
Experimental Research Methods
Experimental methods are used to demonstrate causal relationships between variables. In an experiment, the researcher systematically manipulates a variable of interest (known as the independent variable) and measures the effect on another variable (known as the dependent variable).
Unlike correlational studies, which can only be used to determine if there is a relationship between two variables, experimental methods can be used to determine the actual nature of the relationship—whether changes in one variable actually cause another to change.
The hypothesis is a critical part of any scientific exploration. It represents what researchers expect to find in a study or experiment. In situations where the hypothesis is unsupported by the research, the research still has value. Such research helps us better understand how different aspects of the natural world relate to one another. It also helps us develop new hypotheses that can then be tested in the future.
Thompson WH, Skau S. On the scope of scientific hypotheses . R Soc Open Sci . 2023;10(8):230607. doi:10.1098/rsos.230607
Taran S, Adhikari NKJ, Fan E. Falsifiability in medicine: what clinicians can learn from Karl Popper [published correction appears in Intensive Care Med. 2021 Jun 17;:]. Intensive Care Med . 2021;47(9):1054-1056. doi:10.1007/s00134-021-06432-z
Eyler AA. Research Methods for Public Health . 1st ed. Springer Publishing Company; 2020. doi:10.1891/9780826182067.0004
Nosek BA, Errington TM. What is replication ? PLoS Biol . 2020;18(3):e3000691. doi:10.1371/journal.pbio.3000691
Aggarwal R, Ranganathan P. Study designs: Part 2 - Descriptive studies . Perspect Clin Res . 2019;10(1):34-36. doi:10.4103/picr.PICR_154_18
Nevid J. Psychology: Concepts and Applications. Wadworth, 2013.
By Kendra Cherry, MSEd Kendra Cherry, MS, is a psychosocial rehabilitation specialist, psychology educator, and author of the "Everything Psychology Book."
- Privacy Policy

Home » Quantitative Research – Methods, Types and Analysis
Quantitative Research – Methods, Types and Analysis
Table of Contents

Quantitative Research
Quantitative research is a type of research that collects and analyzes numerical data to test hypotheses and answer research questions . This research typically involves a large sample size and uses statistical analysis to make inferences about a population based on the data collected. It often involves the use of surveys, experiments, or other structured data collection methods to gather quantitative data.
Quantitative Research Methods

Quantitative Research Methods are as follows:
Descriptive Research Design
Descriptive research design is used to describe the characteristics of a population or phenomenon being studied. This research method is used to answer the questions of what, where, when, and how. Descriptive research designs use a variety of methods such as observation, case studies, and surveys to collect data. The data is then analyzed using statistical tools to identify patterns and relationships.
Correlational Research Design
Correlational research design is used to investigate the relationship between two or more variables. Researchers use correlational research to determine whether a relationship exists between variables and to what extent they are related. This research method involves collecting data from a sample and analyzing it using statistical tools such as correlation coefficients.
Quasi-experimental Research Design
Quasi-experimental research design is used to investigate cause-and-effect relationships between variables. This research method is similar to experimental research design, but it lacks full control over the independent variable. Researchers use quasi-experimental research designs when it is not feasible or ethical to manipulate the independent variable.
Experimental Research Design
Experimental research design is used to investigate cause-and-effect relationships between variables. This research method involves manipulating the independent variable and observing the effects on the dependent variable. Researchers use experimental research designs to test hypotheses and establish cause-and-effect relationships.
Survey Research
Survey research involves collecting data from a sample of individuals using a standardized questionnaire. This research method is used to gather information on attitudes, beliefs, and behaviors of individuals. Researchers use survey research to collect data quickly and efficiently from a large sample size. Survey research can be conducted through various methods such as online, phone, mail, or in-person interviews.
Quantitative Research Analysis Methods
Here are some commonly used quantitative research analysis methods:
Statistical Analysis
Statistical analysis is the most common quantitative research analysis method. It involves using statistical tools and techniques to analyze the numerical data collected during the research process. Statistical analysis can be used to identify patterns, trends, and relationships between variables, and to test hypotheses and theories.
Regression Analysis
Regression analysis is a statistical technique used to analyze the relationship between one dependent variable and one or more independent variables. Researchers use regression analysis to identify and quantify the impact of independent variables on the dependent variable.
Factor Analysis
Factor analysis is a statistical technique used to identify underlying factors that explain the correlations among a set of variables. Researchers use factor analysis to reduce a large number of variables to a smaller set of factors that capture the most important information.
Structural Equation Modeling
Structural equation modeling is a statistical technique used to test complex relationships between variables. It involves specifying a model that includes both observed and unobserved variables, and then using statistical methods to test the fit of the model to the data.
Time Series Analysis
Time series analysis is a statistical technique used to analyze data that is collected over time. It involves identifying patterns and trends in the data, as well as any seasonal or cyclical variations.
Multilevel Modeling
Multilevel modeling is a statistical technique used to analyze data that is nested within multiple levels. For example, researchers might use multilevel modeling to analyze data that is collected from individuals who are nested within groups, such as students nested within schools.
Applications of Quantitative Research
Quantitative research has many applications across a wide range of fields. Here are some common examples:
- Market Research : Quantitative research is used extensively in market research to understand consumer behavior, preferences, and trends. Researchers use surveys, experiments, and other quantitative methods to collect data that can inform marketing strategies, product development, and pricing decisions.
- Health Research: Quantitative research is used in health research to study the effectiveness of medical treatments, identify risk factors for diseases, and track health outcomes over time. Researchers use statistical methods to analyze data from clinical trials, surveys, and other sources to inform medical practice and policy.
- Social Science Research: Quantitative research is used in social science research to study human behavior, attitudes, and social structures. Researchers use surveys, experiments, and other quantitative methods to collect data that can inform social policies, educational programs, and community interventions.
- Education Research: Quantitative research is used in education research to study the effectiveness of teaching methods, assess student learning outcomes, and identify factors that influence student success. Researchers use experimental and quasi-experimental designs, as well as surveys and other quantitative methods, to collect and analyze data.
- Environmental Research: Quantitative research is used in environmental research to study the impact of human activities on the environment, assess the effectiveness of conservation strategies, and identify ways to reduce environmental risks. Researchers use statistical methods to analyze data from field studies, experiments, and other sources.
Characteristics of Quantitative Research
Here are some key characteristics of quantitative research:
- Numerical data : Quantitative research involves collecting numerical data through standardized methods such as surveys, experiments, and observational studies. This data is analyzed using statistical methods to identify patterns and relationships.
- Large sample size: Quantitative research often involves collecting data from a large sample of individuals or groups in order to increase the reliability and generalizability of the findings.
- Objective approach: Quantitative research aims to be objective and impartial in its approach, focusing on the collection and analysis of data rather than personal beliefs, opinions, or experiences.
- Control over variables: Quantitative research often involves manipulating variables to test hypotheses and establish cause-and-effect relationships. Researchers aim to control for extraneous variables that may impact the results.
- Replicable : Quantitative research aims to be replicable, meaning that other researchers should be able to conduct similar studies and obtain similar results using the same methods.
- Statistical analysis: Quantitative research involves using statistical tools and techniques to analyze the numerical data collected during the research process. Statistical analysis allows researchers to identify patterns, trends, and relationships between variables, and to test hypotheses and theories.
- Generalizability: Quantitative research aims to produce findings that can be generalized to larger populations beyond the specific sample studied. This is achieved through the use of random sampling methods and statistical inference.
Examples of Quantitative Research
Here are some examples of quantitative research in different fields:
- Market Research: A company conducts a survey of 1000 consumers to determine their brand awareness and preferences. The data is analyzed using statistical methods to identify trends and patterns that can inform marketing strategies.
- Health Research : A researcher conducts a randomized controlled trial to test the effectiveness of a new drug for treating a particular medical condition. The study involves collecting data from a large sample of patients and analyzing the results using statistical methods.
- Social Science Research : A sociologist conducts a survey of 500 people to study attitudes toward immigration in a particular country. The data is analyzed using statistical methods to identify factors that influence these attitudes.
- Education Research: A researcher conducts an experiment to compare the effectiveness of two different teaching methods for improving student learning outcomes. The study involves randomly assigning students to different groups and collecting data on their performance on standardized tests.
- Environmental Research : A team of researchers conduct a study to investigate the impact of climate change on the distribution and abundance of a particular species of plant or animal. The study involves collecting data on environmental factors and population sizes over time and analyzing the results using statistical methods.
- Psychology : A researcher conducts a survey of 500 college students to investigate the relationship between social media use and mental health. The data is analyzed using statistical methods to identify correlations and potential causal relationships.
- Political Science: A team of researchers conducts a study to investigate voter behavior during an election. They use survey methods to collect data on voting patterns, demographics, and political attitudes, and analyze the results using statistical methods.
How to Conduct Quantitative Research
Here is a general overview of how to conduct quantitative research:
- Develop a research question: The first step in conducting quantitative research is to develop a clear and specific research question. This question should be based on a gap in existing knowledge, and should be answerable using quantitative methods.
- Develop a research design: Once you have a research question, you will need to develop a research design. This involves deciding on the appropriate methods to collect data, such as surveys, experiments, or observational studies. You will also need to determine the appropriate sample size, data collection instruments, and data analysis techniques.
- Collect data: The next step is to collect data. This may involve administering surveys or questionnaires, conducting experiments, or gathering data from existing sources. It is important to use standardized methods to ensure that the data is reliable and valid.
- Analyze data : Once the data has been collected, it is time to analyze it. This involves using statistical methods to identify patterns, trends, and relationships between variables. Common statistical techniques include correlation analysis, regression analysis, and hypothesis testing.
- Interpret results: After analyzing the data, you will need to interpret the results. This involves identifying the key findings, determining their significance, and drawing conclusions based on the data.
- Communicate findings: Finally, you will need to communicate your findings. This may involve writing a research report, presenting at a conference, or publishing in a peer-reviewed journal. It is important to clearly communicate the research question, methods, results, and conclusions to ensure that others can understand and replicate your research.
When to use Quantitative Research
Here are some situations when quantitative research can be appropriate:
- To test a hypothesis: Quantitative research is often used to test a hypothesis or a theory. It involves collecting numerical data and using statistical analysis to determine if the data supports or refutes the hypothesis.
- To generalize findings: If you want to generalize the findings of your study to a larger population, quantitative research can be useful. This is because it allows you to collect numerical data from a representative sample of the population and use statistical analysis to make inferences about the population as a whole.
- To measure relationships between variables: If you want to measure the relationship between two or more variables, such as the relationship between age and income, or between education level and job satisfaction, quantitative research can be useful. It allows you to collect numerical data on both variables and use statistical analysis to determine the strength and direction of the relationship.
- To identify patterns or trends: Quantitative research can be useful for identifying patterns or trends in data. For example, you can use quantitative research to identify trends in consumer behavior or to identify patterns in stock market data.
- To quantify attitudes or opinions : If you want to measure attitudes or opinions on a particular topic, quantitative research can be useful. It allows you to collect numerical data using surveys or questionnaires and analyze the data using statistical methods to determine the prevalence of certain attitudes or opinions.
Purpose of Quantitative Research
The purpose of quantitative research is to systematically investigate and measure the relationships between variables or phenomena using numerical data and statistical analysis. The main objectives of quantitative research include:
- Description : To provide a detailed and accurate description of a particular phenomenon or population.
- Explanation : To explain the reasons for the occurrence of a particular phenomenon, such as identifying the factors that influence a behavior or attitude.
- Prediction : To predict future trends or behaviors based on past patterns and relationships between variables.
- Control : To identify the best strategies for controlling or influencing a particular outcome or behavior.
Quantitative research is used in many different fields, including social sciences, business, engineering, and health sciences. It can be used to investigate a wide range of phenomena, from human behavior and attitudes to physical and biological processes. The purpose of quantitative research is to provide reliable and valid data that can be used to inform decision-making and improve understanding of the world around us.
Advantages of Quantitative Research
There are several advantages of quantitative research, including:
- Objectivity : Quantitative research is based on objective data and statistical analysis, which reduces the potential for bias or subjectivity in the research process.
- Reproducibility : Because quantitative research involves standardized methods and measurements, it is more likely to be reproducible and reliable.
- Generalizability : Quantitative research allows for generalizations to be made about a population based on a representative sample, which can inform decision-making and policy development.
- Precision : Quantitative research allows for precise measurement and analysis of data, which can provide a more accurate understanding of phenomena and relationships between variables.
- Efficiency : Quantitative research can be conducted relatively quickly and efficiently, especially when compared to qualitative research, which may involve lengthy data collection and analysis.
- Large sample sizes : Quantitative research can accommodate large sample sizes, which can increase the representativeness and generalizability of the results.
Limitations of Quantitative Research
There are several limitations of quantitative research, including:
- Limited understanding of context: Quantitative research typically focuses on numerical data and statistical analysis, which may not provide a comprehensive understanding of the context or underlying factors that influence a phenomenon.
- Simplification of complex phenomena: Quantitative research often involves simplifying complex phenomena into measurable variables, which may not capture the full complexity of the phenomenon being studied.
- Potential for researcher bias: Although quantitative research aims to be objective, there is still the potential for researcher bias in areas such as sampling, data collection, and data analysis.
- Limited ability to explore new ideas: Quantitative research is often based on pre-determined research questions and hypotheses, which may limit the ability to explore new ideas or unexpected findings.
- Limited ability to capture subjective experiences : Quantitative research is typically focused on objective data and may not capture the subjective experiences of individuals or groups being studied.
- Ethical concerns : Quantitative research may raise ethical concerns, such as invasion of privacy or the potential for harm to participants.
About the author
Muhammad Hassan
Researcher, Academic Writer, Web developer
You may also like

Questionnaire – Definition, Types, and Examples

Case Study – Methods, Examples and Guide

Observational Research – Methods and Guide

Qualitative Research Methods

Explanatory Research – Types, Methods, Guide

Survey Research – Types, Methods, Examples
- USC Libraries
- Research Guides
Organizing Your Social Sciences Research Paper
- Quantitative Methods
- Purpose of Guide
- Design Flaws to Avoid
- Independent and Dependent Variables
- Glossary of Research Terms
- Reading Research Effectively
- Narrowing a Topic Idea
- Broadening a Topic Idea
- Extending the Timeliness of a Topic Idea
- Academic Writing Style
- Applying Critical Thinking
- Choosing a Title
- Making an Outline
- Paragraph Development
- Research Process Video Series
- Executive Summary
- The C.A.R.S. Model
- Background Information
- The Research Problem/Question
- Theoretical Framework
- Citation Tracking
- Content Alert Services
- Evaluating Sources
- Primary Sources
- Secondary Sources
- Tiertiary Sources
- Scholarly vs. Popular Publications
- Qualitative Methods
- Insiderness
- Using Non-Textual Elements
- Limitations of the Study
- Common Grammar Mistakes
- Writing Concisely
- Avoiding Plagiarism
- Footnotes or Endnotes?
- Further Readings
- Generative AI and Writing
- USC Libraries Tutorials and Other Guides
- Bibliography
Quantitative methods emphasize objective measurements and the statistical, mathematical, or numerical analysis of data collected through polls, questionnaires, and surveys, or by manipulating pre-existing statistical data using computational techniques . Quantitative research focuses on gathering numerical data and generalizing it across groups of people or to explain a particular phenomenon.
Babbie, Earl R. The Practice of Social Research . 12th ed. Belmont, CA: Wadsworth Cengage, 2010; Muijs, Daniel. Doing Quantitative Research in Education with SPSS . 2nd edition. London: SAGE Publications, 2010.
Need Help Locating Statistics?
Resources for locating data and statistics can be found here:
Statistics & Data Research Guide
Characteristics of Quantitative Research
Your goal in conducting quantitative research study is to determine the relationship between one thing [an independent variable] and another [a dependent or outcome variable] within a population. Quantitative research designs are either descriptive [subjects usually measured once] or experimental [subjects measured before and after a treatment]. A descriptive study establishes only associations between variables; an experimental study establishes causality.
Quantitative research deals in numbers, logic, and an objective stance. Quantitative research focuses on numeric and unchanging data and detailed, convergent reasoning rather than divergent reasoning [i.e., the generation of a variety of ideas about a research problem in a spontaneous, free-flowing manner].
Its main characteristics are :
- The data is usually gathered using structured research instruments.
- The results are based on larger sample sizes that are representative of the population.
- The research study can usually be replicated or repeated, given its high reliability.
- Researcher has a clearly defined research question to which objective answers are sought.
- All aspects of the study are carefully designed before data is collected.
- Data are in the form of numbers and statistics, often arranged in tables, charts, figures, or other non-textual forms.
- Project can be used to generalize concepts more widely, predict future results, or investigate causal relationships.
- Researcher uses tools, such as questionnaires or computer software, to collect numerical data.
The overarching aim of a quantitative research study is to classify features, count them, and construct statistical models in an attempt to explain what is observed.
Things to keep in mind when reporting the results of a study using quantitative methods :
- Explain the data collected and their statistical treatment as well as all relevant results in relation to the research problem you are investigating. Interpretation of results is not appropriate in this section.
- Report unanticipated events that occurred during your data collection. Explain how the actual analysis differs from the planned analysis. Explain your handling of missing data and why any missing data does not undermine the validity of your analysis.
- Explain the techniques you used to "clean" your data set.
- Choose a minimally sufficient statistical procedure ; provide a rationale for its use and a reference for it. Specify any computer programs used.
- Describe the assumptions for each procedure and the steps you took to ensure that they were not violated.
- When using inferential statistics , provide the descriptive statistics, confidence intervals, and sample sizes for each variable as well as the value of the test statistic, its direction, the degrees of freedom, and the significance level [report the actual p value].
- Avoid inferring causality , particularly in nonrandomized designs or without further experimentation.
- Use tables to provide exact values ; use figures to convey global effects. Keep figures small in size; include graphic representations of confidence intervals whenever possible.
- Always tell the reader what to look for in tables and figures .
NOTE: When using pre-existing statistical data gathered and made available by anyone other than yourself [e.g., government agency], you still must report on the methods that were used to gather the data and describe any missing data that exists and, if there is any, provide a clear explanation why the missing data does not undermine the validity of your final analysis.
Babbie, Earl R. The Practice of Social Research . 12th ed. Belmont, CA: Wadsworth Cengage, 2010; Brians, Craig Leonard et al. Empirical Political Analysis: Quantitative and Qualitative Research Methods . 8th ed. Boston, MA: Longman, 2011; McNabb, David E. Research Methods in Public Administration and Nonprofit Management: Quantitative and Qualitative Approaches . 2nd ed. Armonk, NY: M.E. Sharpe, 2008; Quantitative Research Methods. Writing@CSU. Colorado State University; Singh, Kultar. Quantitative Social Research Methods . Los Angeles, CA: Sage, 2007.
Basic Research Design for Quantitative Studies
Before designing a quantitative research study, you must decide whether it will be descriptive or experimental because this will dictate how you gather, analyze, and interpret the results. A descriptive study is governed by the following rules: subjects are generally measured once; the intention is to only establish associations between variables; and, the study may include a sample population of hundreds or thousands of subjects to ensure that a valid estimate of a generalized relationship between variables has been obtained. An experimental design includes subjects measured before and after a particular treatment, the sample population may be very small and purposefully chosen, and it is intended to establish causality between variables. Introduction The introduction to a quantitative study is usually written in the present tense and from the third person point of view. It covers the following information:
- Identifies the research problem -- as with any academic study, you must state clearly and concisely the research problem being investigated.
- Reviews the literature -- review scholarship on the topic, synthesizing key themes and, if necessary, noting studies that have used similar methods of inquiry and analysis. Note where key gaps exist and how your study helps to fill these gaps or clarifies existing knowledge.
- Describes the theoretical framework -- provide an outline of the theory or hypothesis underpinning your study. If necessary, define unfamiliar or complex terms, concepts, or ideas and provide the appropriate background information to place the research problem in proper context [e.g., historical, cultural, economic, etc.].
Methodology The methods section of a quantitative study should describe how each objective of your study will be achieved. Be sure to provide enough detail to enable the reader can make an informed assessment of the methods being used to obtain results associated with the research problem. The methods section should be presented in the past tense.
- Study population and sampling -- where did the data come from; how robust is it; note where gaps exist or what was excluded. Note the procedures used for their selection;
- Data collection – describe the tools and methods used to collect information and identify the variables being measured; describe the methods used to obtain the data; and, note if the data was pre-existing [i.e., government data] or you gathered it yourself. If you gathered it yourself, describe what type of instrument you used and why. Note that no data set is perfect--describe any limitations in methods of gathering data.
- Data analysis -- describe the procedures for processing and analyzing the data. If appropriate, describe the specific instruments of analysis used to study each research objective, including mathematical techniques and the type of computer software used to manipulate the data.
Results The finding of your study should be written objectively and in a succinct and precise format. In quantitative studies, it is common to use graphs, tables, charts, and other non-textual elements to help the reader understand the data. Make sure that non-textual elements do not stand in isolation from the text but are being used to supplement the overall description of the results and to help clarify key points being made. Further information about how to effectively present data using charts and graphs can be found here .
- Statistical analysis -- how did you analyze the data? What were the key findings from the data? The findings should be present in a logical, sequential order. Describe but do not interpret these trends or negative results; save that for the discussion section. The results should be presented in the past tense.
Discussion Discussions should be analytic, logical, and comprehensive. The discussion should meld together your findings in relation to those identified in the literature review, and placed within the context of the theoretical framework underpinning the study. The discussion should be presented in the present tense.
- Interpretation of results -- reiterate the research problem being investigated and compare and contrast the findings with the research questions underlying the study. Did they affirm predicted outcomes or did the data refute it?
- Description of trends, comparison of groups, or relationships among variables -- describe any trends that emerged from your analysis and explain all unanticipated and statistical insignificant findings.
- Discussion of implications – what is the meaning of your results? Highlight key findings based on the overall results and note findings that you believe are important. How have the results helped fill gaps in understanding the research problem?
- Limitations -- describe any limitations or unavoidable bias in your study and, if necessary, note why these limitations did not inhibit effective interpretation of the results.
Conclusion End your study by to summarizing the topic and provide a final comment and assessment of the study.
- Summary of findings – synthesize the answers to your research questions. Do not report any statistical data here; just provide a narrative summary of the key findings and describe what was learned that you did not know before conducting the study.
- Recommendations – if appropriate to the aim of the assignment, tie key findings with policy recommendations or actions to be taken in practice.
- Future research – note the need for future research linked to your study’s limitations or to any remaining gaps in the literature that were not addressed in your study.
Black, Thomas R. Doing Quantitative Research in the Social Sciences: An Integrated Approach to Research Design, Measurement and Statistics . London: Sage, 1999; Gay,L. R. and Peter Airasain. Educational Research: Competencies for Analysis and Applications . 7th edition. Upper Saddle River, NJ: Merril Prentice Hall, 2003; Hector, Anestine. An Overview of Quantitative Research in Composition and TESOL . Department of English, Indiana University of Pennsylvania; Hopkins, Will G. “Quantitative Research Design.” Sportscience 4, 1 (2000); "A Strategy for Writing Up Research Results. The Structure, Format, Content, and Style of a Journal-Style Scientific Paper." Department of Biology. Bates College; Nenty, H. Johnson. "Writing a Quantitative Research Thesis." International Journal of Educational Science 1 (2009): 19-32; Ouyang, Ronghua (John). Basic Inquiry of Quantitative Research . Kennesaw State University.
Strengths of Using Quantitative Methods
Quantitative researchers try to recognize and isolate specific variables contained within the study framework, seek correlation, relationships and causality, and attempt to control the environment in which the data is collected to avoid the risk of variables, other than the one being studied, accounting for the relationships identified.
Among the specific strengths of using quantitative methods to study social science research problems:
- Allows for a broader study, involving a greater number of subjects, and enhancing the generalization of the results;
- Allows for greater objectivity and accuracy of results. Generally, quantitative methods are designed to provide summaries of data that support generalizations about the phenomenon under study. In order to accomplish this, quantitative research usually involves few variables and many cases, and employs prescribed procedures to ensure validity and reliability;
- Applying well established standards means that the research can be replicated, and then analyzed and compared with similar studies;
- You can summarize vast sources of information and make comparisons across categories and over time; and,
- Personal bias can be avoided by keeping a 'distance' from participating subjects and using accepted computational techniques .
Babbie, Earl R. The Practice of Social Research . 12th ed. Belmont, CA: Wadsworth Cengage, 2010; Brians, Craig Leonard et al. Empirical Political Analysis: Quantitative and Qualitative Research Methods . 8th ed. Boston, MA: Longman, 2011; McNabb, David E. Research Methods in Public Administration and Nonprofit Management: Quantitative and Qualitative Approaches . 2nd ed. Armonk, NY: M.E. Sharpe, 2008; Singh, Kultar. Quantitative Social Research Methods . Los Angeles, CA: Sage, 2007.
Limitations of Using Quantitative Methods
Quantitative methods presume to have an objective approach to studying research problems, where data is controlled and measured, to address the accumulation of facts, and to determine the causes of behavior. As a consequence, the results of quantitative research may be statistically significant but are often humanly insignificant.
Some specific limitations associated with using quantitative methods to study research problems in the social sciences include:
- Quantitative data is more efficient and able to test hypotheses, but may miss contextual detail;
- Uses a static and rigid approach and so employs an inflexible process of discovery;
- The development of standard questions by researchers can lead to "structural bias" and false representation, where the data actually reflects the view of the researcher instead of the participating subject;
- Results provide less detail on behavior, attitudes, and motivation;
- Researcher may collect a much narrower and sometimes superficial dataset;
- Results are limited as they provide numerical descriptions rather than detailed narrative and generally provide less elaborate accounts of human perception;
- The research is often carried out in an unnatural, artificial environment so that a level of control can be applied to the exercise. This level of control might not normally be in place in the real world thus yielding "laboratory results" as opposed to "real world results"; and,
- Preset answers will not necessarily reflect how people really feel about a subject and, in some cases, might just be the closest match to the preconceived hypothesis.
Research Tip
Finding Examples of How to Apply Different Types of Research Methods
SAGE publications is a major publisher of studies about how to design and conduct research in the social and behavioral sciences. Their SAGE Research Methods Online and Cases database includes contents from books, articles, encyclopedias, handbooks, and videos covering social science research design and methods including the complete Little Green Book Series of Quantitative Applications in the Social Sciences and the Little Blue Book Series of Qualitative Research techniques. The database also includes case studies outlining the research methods used in real research projects. This is an excellent source for finding definitions of key terms and descriptions of research design and practice, techniques of data gathering, analysis, and reporting, and information about theories of research [e.g., grounded theory]. The database covers both qualitative and quantitative research methods as well as mixed methods approaches to conducting research.
SAGE Research Methods Online and Cases
- << Previous: Qualitative Methods
- Next: Insiderness >>
- Last Updated: May 9, 2024 11:05 AM
- URL: https://libguides.usc.edu/writingguide
Research Paper Guide
Quantitative Research

Understanding Quantitative Research - Types & Data Collection Techniques
13 min read
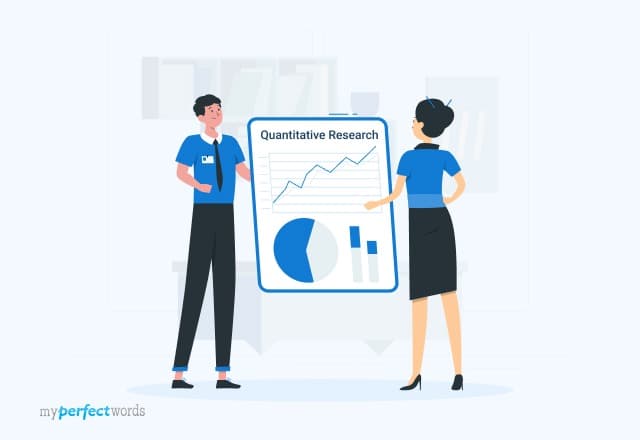
People also read
Research Paper Writing - A Step by Step Guide
Research Paper Examples - Free Sample Papers for Different Formats!
Guide to Creating Effective Research Paper Outline
Interesting Research Paper Topics for 2024
Research Proposal Writing - A Step-by-Step Guide
How to Start a Research Paper - 7 Easy Steps
How to Write an Abstract for a Research Paper - A Step by Step Guide
Writing a Literature Review For a Research Paper - A Comprehensive Guide
Qualitative Research - Methods, Types, and Examples
8 Types of Qualitative Research - Overview & Examples
Qualitative vs Quantitative Research - Learning the Basics
200+ Engaging Psychology Research Paper Topics for Students in 2024
Learn How to Write a Hypothesis in a Research Paper: Examples and Tips!
20+ Types of Research With Examples - A Detailed Guide
230+ Sociology Research Topics & Ideas for Students
How to Cite a Research Paper - A Complete Guide
Excellent History Research Paper Topics- 300+ Ideas
A Guide on Writing the Method Section of a Research Paper - Examples & Tips
How To Write an Introduction Paragraph For a Research Paper: Learn with Examples
Crafting a Winning Research Paper Title: A Complete Guide
Writing a Research Paper Conclusion - Step-by-Step Guide
Writing a Thesis For a Research Paper - A Comprehensive Guide
How To Write A Discussion For A Research Paper | Examples & Tips
How To Write The Results Section of A Research Paper | Steps & Examples
Writing a Problem Statement for a Research Paper - A Comprehensive Guide
Finding Sources For a Research Paper: A Complete Guide
A Guide on How to Edit a Research Paper
200+ Ethical Research Paper Topics to Begin With (2024)
300+ Controversial Research Paper Topics & Ideas - 2024 Edition
150+ Argumentative Research Paper Topics For You - 2024
How to Write a Research Methodology for a Research Paper
Ever had a tough time with quantitative research? You're not alone!
Quantitative research is the process of collecting and analyzing numerical data to understand and study various phenomena using statistical methods. Many find this tedious process tricky.
But don't worry!
Our complete guide is here to guide you through the important steps and tricks to handle this challenge with confidence. We've even added some examples to make it easier.
So, let's dive in and learn together!

- 1. Quantitative Research Definition - What is Quantitative Research?
- 2. Data Collection in Quantitative Research
- 3. Data Analysis in Quantitative Research
- 4. Types of Quantitative Research Methods for Students and Researchers
- 5. Types of Data Collection Methodologies in Quantitative Research
- 6. Quantitative vs. Qualitative Research
- 7. Advantages and Strengths of Quantitative Research
- 8. Disadvantages and Weaknesses of Quantitative Research
Quantitative Research Definition - What is Quantitative Research?
Quantitative research involves gathering and studying numerical data. Its applications include identifying trends, making forecasts, testing cause-and-effect links, and drawing broader conclusions applicable to larger groups.
In this method, researchers employ tools such as surveys, experiments, and observations to gather data. Whereas in qualitative research, you deal with non-numeric data, such as text, video, or audio.
Quantitative research is extensively applied in natural and social sciences, including biology, chemistry, psychology, economics, sociology, and marketing, among others.
Characteristics of Quantitative Research
Here are some distinct quantitative research characteristics:
- Large Sample Sizes: Quantitative studies often involve larger sample sizes, allowing for more robust statistical analyses and generalizability of findings.
- Statistical Analysis: Statistical techniques and tools are extensively used to analyze data, unveiling patterns, relationships, and significance.
- Objective and Replicable: Quantitative research aims for objectivity and replicability. Other researchers should be able to conduct the same study and obtain similar results.
- Closed-Ended Questions: Surveys and questionnaires typically use closed-ended questions with predefined response options, making data analysis more straightforward.
- Quantifiable Variables: Researchers identify and measure variables that can be quantified, such as age, income, or test scores, for precise analysis.
- Hypothesis Testing: It often involves testing hypotheses and making inferences about populations based on sample data.
- Cross-Sectional or Longitudinal: Studies can be cross-sectional (data collected at a single point in time) or longitudinal (data collected over an extended period).
- Generalizability: Quantitative research seeks to generalize findings from a sample to a larger population, provided the sample is representative.
These characteristics make quantitative research different from qualitative research.
Data Collection in Quantitative Research
Data collection is the systematic process of gathering information for research purposes. It is a critical starting point, ensuring that the information gathered is relevant, accurate, and comprehensive.
- Structured Instruments - Quantitative research typically employs structured instruments like surveys and questionnaires. These tools ensure consistency in data gathering by posing the same set of questions to each participant.
- Sampling Methods - Researchers use various sampling techniques, such as random sampling, stratified sampling, or convenience sampling, to select a representative group from the target population.
- Objective Observation - Data collection often involves objective observations of phenomena. This may include recording numerical data, such as counting occurrences or measuring attributes.
- Experimental Control - In experimental research, control over variables is essential. Researchers manipulate one or more variables to observe their impact on the outcome, maintaining control over external factors.
Data Analysis in Quantitative Research
Data analysis is the second important aspect of quantitative research. After collecting the data, the data is analyzed with statistical methods. When analyzing, it is important that the results are relevant and related to the objective and aim of the research.
Below are some common statistical analysis methods that are used to analyze the collected data.
- SWOT Analysis - It stands for Strengths, Weaknesses, Opportunities, and Threats. Businesses use this kind of analysis to evaluate their performance and develop appropriate strategies.
- Conjoint Analysis - This kind of analysis helps businesses to identify how customers make difficult purchasing decisions. The businesses involved in direct sales and purchases know this and use the analysis to make the decisions.
- Cross-tabulation - A preliminary statistical analysis helps understand patterns, trends, and relationships between the various factors of the research.
- TURF Analysis - It stands for Totally Unduplicated Reach and Frequency Analysis. It is conducted to collect and analyze the data and responses of a chosen or favored target group.
Afterward, other methods like inferential statistics could be used to gather the results.
Types of Quantitative Research Methods for Students and Researchers
‘What are the four types of quantitative research?’
Quantitative research has four distinct types, and all four of them are regarded as primary research methods. Primary quantitative research is more common and useful than secondary research methods.
It is mainly because, in them, the researcher collects the data directly. He does not depend on previous research and collects the data from scratch.
Below are the four types of quantitative research methods.
Survey Research
This type of research is conducted through means of online surveys, online polls, and questionnaires. A group of people is chosen for the survey, and the method is used by big and small organizations and companies. They use it to understand their customers better.
Ideally, the survey is done through face-to-face meetings and interviews. Now, it is conducted through various online methodologies. Below are the common types of surveys.
- Cross-Sectional Survey - This research is conducted on a selected group of people at a certain point in time. The researcher evaluates several things. The selected group of people has similarities in all aspects except the ones chosen by the researcher. This kind of research is used for industries like retail, small-scale businesses, and healthcare industries.
- Longitudinal Survey - This research is based on observing a specific group of people for a set duration. The duration could be days, months, or even years. The researcher observes the change in behavior of the selected group of people.
This kind of research is used in the fields of applied sciences, medicine, and marketing.
Correlational Research
Correlational research is conducted to identify the relationship between two entities. These entities must be closely related and have a significant impact on each other.
This research is conducted to identify, evaluate, and understand the correlation between the variables and how they depend on each other.
The researchers use mathematical and statistical methods to understand this correlation. Some factors that they consider include relationships, trends, and patterns between these variables.
Sometimes, the researchers make changes in one of the variables to notice the effect on the other one.
Causal-comparative Research
This research is also known as quasi-experimental research. It is based on the cause and effect relationship between the two variables. Here, one of the variables is dependent on the other one, but the other one is independent. The researcher does not change the independent variable.
The research is not limited to statistical analysis only but includes other groups and variables also. The research could be conducted on the variables, no matter the kind of relationship they have. The statistical analysis method is used to acquire the results.
Experimental Research
This kind of research is based on proving or contradicting a theory or statement. It is also known as true experimentation and is usually focused on single or multiple theories.
The respective theory is not proven yet, and the research method is commonly used in natural sciences.
There could be some theories involved in this research. Due to this, it is more common in social sciences.
Types of Data Collection Methodologies in Quantitative Research
After determining the kind of research, finding the right data collection method is the most important step. Data could be collected through both the sampling and surveys and polls method.
Sampling Data Collection Method
In quantitative research, two types of sampling methods are used: probability and non-probability sampling.
1. Probability Sampling
The data is collected by sifting some individuals from the general population and creating samples. The individuals, data samples are chosen randomly and without any particular selection criteria.
Probability sampling is further divided into the following kinds.
- Simple Random Sampling - This kind of data selection is the simplest one, and the participants are chosen randomly. This kind of sampling is conducted on a large population.
- Stratified Random Sampling - In this sampling, the population is divided into several groups and strata. The participants for the research are chosen randomly from those groups.
- Cluster Sampling - In cluster sampling, the population is divided into several clusters based on geography and demography.
- Systematic Sampling - In this, the samples from the population are chosen at regular intervals. These intervals are predefined, and usually, they are calculated based on the population or size of the target sample.
2. Non-Probability Sampling
In this kind of data collection, the researcher uses his knowledge and experience to choose the samples. The researcher is involved and has a set of criteria. Due to this, not all individuals have the chance to be selected for the research.
Below are the main types of non-probability sampling frameworks.
- Convenience Sampling - These kinds of samples are probably the easiest to obtain. They are chosen only because they are the easiest ones to obtain. They are usually closer to the researcher, and these samples are easy to work with because there are no rigid parameters.
- Consecutive Sampling - This is similar to convenience sampling, but the researcher could choose a specific group of people for his research. The researcher could repeat the process with other groups of samples.
- Quota Sampling - The researchers select some specific elements based on the researcher’s target personalities and traits. Based on this, different individuals in the groups have equal chances of getting selected.
- Snowball Sampling - This kind of sampling is done on a target audience or a chosen group that is difficult to contact. In this, the chosen group is difficult to put together.
- Judgemental Sampling - This kind of sample is built based on the researcher’s skills, experience, and preferences.
Survey and Polls Data Collection Method
After the sample or group is chosen, the researcher could use polls or surveys to collect the required research data.
In this kind of research, the data is collected from a selected group of people. The data is used to identify new trends and collect information about different things and topics. Through the survey, the researcher could reach a wider population.
Based on the time allocated for the research, it could be used to collect more information and data.
When creating questions and options for the survey, the researchers use four measurement scales or criteria. These four parameters include nominal, interval, ordinal, and ratio measurement scales. Without them, no multiple-choice questions could be created.
The questions used for the survey must be close-ended. These could be a mix of different kinds of questions, and the responses could be analyzed through different rating scales.
After creating the survey, the next thing is to distribute it. Below are some of the commonly used survey distribution methods.
- Email - The most common method of distributing the survey is email management software to dispense the survey to your selected participants.
- Buying the Respondents - This is also a quite famous and widely used survey distribution method. Select the respondent and have him respond to the survey. Since the respondents would be knowledgeable, they will help in maximizing the results.
- Embedding the Survey on a Website - This is a great way of getting more responses and targeted results. Embedding the survey on a website works because the researcher is at the right place and close to the brand.
- Social Distribution - Distributing the survey through a social media platform helps collect more responses from the right audience.
- QR Code - The survey is stored in the QR code, and it is printed in magazines or on business cards.
- SMS Survey - It is the most convenient way of collecting more responses and data.
Like surveys, polls are also used to collect the data. It also has close-ended quantitative research questions, and election and exit polls are commonly used in this survey.
Quantitative vs. Qualitative Research
Quantitative and qualitative research are major kinds of research. They are mainly used in the subjects that follow detailed research patterns. How does it differ from quantitative research?
Below is a detailed comparison of the two kinds of research.
Want to know more about the differences between these types of research? Check out this extensive read on qualitative vs. quantitative research to get more insights!
Advantages and Strengths of Quantitative Research
Quantitative research offers several advantages to researchers. Some of the main reasons why researchers use this kind of research are discussed below:
- The Data Can Be Replicated - The research and study could be replicated. The data collection methods and definitions of the concepts are clear and easy to understand.
- The Results Can Be Compared Easily - The same study could be conducted in different cultural settings and sample groups. The results could also be compared statistically.
- Usage of Large Samples - Data and information from large samples could be processed and analyzed using different research procedures.
- Hypothesis Could be Tested - The researcher could use formal hypothesis testing. He could report the data collection, research variables, research predictions, and testing techniques before forecasting and establishing any conclusion.
- The Data Collection is Quick - The data could be collected easily and from a wider population. The usage of statistical methods and conducting and analyzing results is also easy and to the point.
- The Data Analysis is Inclusive - Quantitative data and research offer a wider population for sampling. They could be analyzed through research and analysis procedures.
Due to all of these advantages, researchers prefer using this kind of research method. It is easy to sample, collect, and analyze data and repeat the procedure easily.
Disadvantages and Weaknesses of Quantitative Research
Despite the benefits for the researchers, quantitative research design has some limitations. It may not be suitable for more complex and detailed kinds of topics.
Below are some common quantitative research limitations.
- Superficial - since the research includes limited and precise research samples. In quantitative research, the research is presented in numbers. They could be explained in detail through qualitative data and research.
- Limited Focus - the focus is narrow and limited, and the researcher would have to ignore other relevant and important variables.
- Biased Structure - structural biases could exist and affect sampling methods, data collection, and measurement results.
- Lack of Proper Conditions - sometimes, quantitative research may not include other important factors to collect the data.
Due to these reasons, quantitative research is not an ideal choice for detailed kinds of research. For them, qualitative research works better.
To help you further, we have added some useful examples of quantitative research here.
Quantitative Research Examples
Below are some helpful quantitative research examples to help you understand it better.
Sample Quantitative Research
Quantitative Research Example for Students
Now that you've got the hang of how to do quantitative research and why it's valuable, you're all set to begin your research journey.
The qualitative research method shows the idea and perception of your targeted audience. However, not every student is able to choose the right approach while writing a research paper. It requires a thorough understanding of both qualitative research and quantitative research methods.
This is where the professional help from MyPerfectWords.com comes in. We offer custom essay help with your academic assignments at affordable rates.
Contact our customer support and place " write my research paper " order today!

Write Essay Within 60 Seconds!

Nova Allison is a Digital Content Strategist with over eight years of experience. Nova has also worked as a technical and scientific writer. She is majorly involved in developing and reviewing online content plans that engage and resonate with audiences. Nova has a passion for writing that engages and informs her readers.

Paper Due? Why Suffer? That’s our Job!
Keep reading
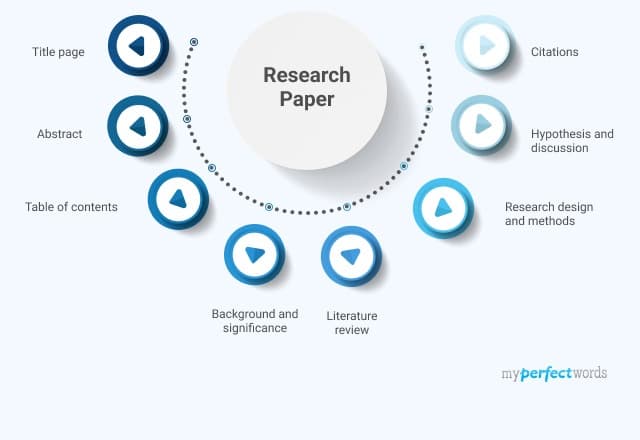
- Open access
- Published: 08 May 2024
Measurement and analysis of change in research scholars’ knowledge and attitudes toward statistics after PhD coursework
- Mariyamma Philip 1
BMC Medical Education volume 24 , Article number: 512 ( 2024 ) Cite this article
55 Accesses
Metrics details
Knowledge of statistics is highly important for research scholars, as they are expected to submit a thesis based on original research as part of a PhD program. As statistics play a major role in the analysis and interpretation of scientific data, intensive training at the beginning of a PhD programme is essential. PhD coursework is mandatory in universities and higher education institutes in India. This study aimed to compare the scores of knowledge in statistics and attitudes towards statistics among the research scholars of an institute of medical higher education in South India at different time points of their PhD (i.e., before, soon after and 2–3 years after the coursework) to determine whether intensive training programs such as PhD coursework can change their knowledge or attitudes toward statistics.
One hundred and thirty research scholars who had completed PhD coursework in the last three years were invited by e-mail to be part of the study. Knowledge and attitudes toward statistics before and soon after the coursework were already assessed as part of the coursework module. Knowledge and attitudes towards statistics 2–3 years after the coursework were assessed using Google forms. Participation was voluntary, and informed consent was also sought.
Knowledge and attitude scores improved significantly subsequent to the coursework (i.e., soon after, percentage of change: 77%, 43% respectively). However, there was significant reduction in knowledge and attitude scores 2–3 years after coursework compared to the scores soon after coursework; knowledge and attitude scores have decreased by 10%, 37% respectively.
The study concluded that the coursework program was beneficial for improving research scholars’ knowledge and attitudes toward statistics. A refresher program 2–3 years after the coursework would greatly benefit the research scholars. Statistics educators must be empathetic to understanding scholars’ anxiety and attitudes toward statistics and its influence on learning outcomes.
Peer Review reports
A PhD degree is a research degree, and research scholars submit a thesis based on original research in their chosen field. Doctor of Philosophy (PhD) degrees are awarded in a wide range of academic disciplines, and the PhD students are usually referred as research scholars. A comprehensive understanding of statistics allows research scholars to add rigour to their research. This approach helps them evaluate the current practices and draw informed conclusions from studies that were undertaken to generate their own hypotheses and to design, analyse and interpret complex clinical decisions. Therefore, intensive training at the beginning of the PhD journey is essential, as intensive training in research methodology and statistics in the early stages of research helps scholars design and plan their studies efficiently.
The University Grants Commission of India has taken various initiatives to introduce academic reforms to higher education institutions in India and mandated in 2009 that coursework be treated as a prerequisite for PhD preparation and that a minimum of four credits be assigned to one or more courses on research methodology, which could cover areas such as quantitative methods, computer applications, and research ethics. UGC also clearly states that all candidates admitted to PhD programmes shall be required to complete the prescribed coursework during the initial two semesters [ 1 ]. National Institute of Mental Health and Neurosciences (NIMHANS) at Bangalore, a tertiary care hospital and medical higher education institute in South India, that trains students in higher education in clinical fields, also introduced coursework in the PhD program for research scholars from various backgrounds, such as basic, behavioral and neurosciences, as per the UGC mandate. Research scholars undertake coursework programs soon after admission, which consist of several modules that include research methodology and statistical software training, among others.
Most scholars approach a course in statistics with the prejudice that statistics is uninteresting, demanding, complex or involve much mathematics and, most importantly, it is not relevant to their career goals. They approach statistics with considerable apprehension and negative attitudes, probably because of their inability to grasp the relevance of the application of the methods in their fields of study. This could be resolved by providing sufficient and relevant examples of the application of statistical techniques from various fields of medical research and by providing hands-on experience to learn how these techniques are applied and interpreted on real data. Hence, research methodology and statistical methods and the application of statistical methods using software have been given much importance and are taught as two modules, named Research Methodology and Statistics and Statistical Software Training, at this institute of medical higher education that trains research scholars in fields as diverse as basic, behavioural and neurosciences. Approximately 50% of the coursework curriculum focused on these two modules. Research scholars were thus given an opportunity to understand the theoretical aspects of the research methodology and statistical methods. They were also given hands-on training on statistical software to analyse the data using these methods and to interpret the findings. The coursework program was designed in this specific manner, as this intensive training would enable the research scholars to design their research studies more effectively and analyse their data in a better manner.
It is important to study attitudes toward statistics because attitudes are known to impact the learning process. Also, most importantly, these scholars are expected to utilize the skills in statistics and research methods to design research projects or guide postgraduate students and research scholars in the near future. Several authors have assessed attitudes toward statistics among various students and examined how attitudes affect academic achievement, how attitudes are correlated with knowledge in statistics and how attitudes change after a training program. There are studies on attitudes toward statistics among graduate [ 2 , 3 , 4 ] and postgraduate [ 5 ] medical students, politics, sociology, ( 6 – 7 ) psychology [ 8 , 9 , 10 ], social work [ 11 ], and management students [ 12 ]. However, there is a dearth of related literature on research scholars, and there are only two studies on the attitudes of research scholars. In their study of doctoral students in education-related fields, Cook & Catanzaro (2022) investigated the factors that contribute to statistics anxiety and attitudes toward statistics and how anxiety, attitudes and plans for future research use are connected among doctoral students [ 13 ]. Another study by Sohrabi et al. (2018) on research scholars assessed the change in knowledge and attitude towards teaching and educational design of basic science PhD students at a Medical University after a two-day workshop on empowerment and familiarity with the teaching and learning principles [ 14 ]. There were no studies that assessed changes in the attitudes or knowledge of research scholars across the PhD training period or after intensive training programmes such as PhD coursework. Even though PhD coursework has been established in institutes of higher education in India for more than a decade, there are no published research on the effectiveness of coursework from Indian universities or institutes of higher education.
This study aimed to determine the effectiveness of PhD coursework and whether intensive training programs such as PhD coursework can influence the knowledge and attitudes toward statistics of research scholars. Additionally, it would be interesting to know if the acquired knowledge could be retained longer, especially 2–3 years after the coursework, the crucial time of PhD data analysis. Hence, this study compares the scores of knowledge in statistics and attitude toward statistics of the research scholars at different time points of their PhD training, i.e., before, soon after and 2–3 years after the coursework.
Participants
This is an observational study of single group with repeated assessments. The institute offers a three-month coursework program consisting of seven modules, the first module is ethics; the fifth is research methodology and statistics; and the last is neurosciences. The study was conducted in January 2020. All research scholars of the institute who had completed PhD coursework in the last three years were considered for this study ( n = 130). Knowledge and attitudes toward statistics before and soon after the coursework module were assessed as part of the coursework program. They were collected on the first and last day of the program respectively. The author who was also the coordinator of the research methodology and statistics module of the coursework have obtained the necessary permission to use the data for this study. The scholars invited to be part of the study by e-mail. Knowledge and attitude towards statistics 2–3 years after the coursework were assessed online using Google forms. They were also administered a semi structured questionnaire to elicit details about the usefulness of coursework. Participation was voluntary, and consent was also sought online. The confidentiality of the data was assured. Data were not collected from research scholars of Biostatistics or from research scholars who had more than a decade of experience or who had been working in the institute as faculty, assuming that their scores could be higher and could bias the findings. This non funded study was reviewed and approved by the Institute Ethics Committee.
Instruments
Knowledge in Statistics was assessed by a questionnaire prepared by the author and was used as part of the coursework evaluation. The survey included 25 questions that assessed the knowledge of statistics on areas such as descriptive statistics, sampling methods, study design, parametric and nonparametric tests and multivariate analyses. Right answers were assigned a score of 1, and wrong answers were assigned a score of 0. Total scores ranged from 0 to 25. Statistics attitudes were assessed by the Survey of Attitudes toward Statistics (SATS) scale. The SATS is a 36-item scale that measures 6 domains of attitudes towards statistics. The possible range of scores for each item is between 1 and 7. The total score was calculated by dividing the summed score by the number of items. Higher scores indicate more positive attitudes. The SAT-36 is a copyrighted scale, and researchers are allowed to use it only with prior permission. ( 15 – 16 ) The author obtained permission for use in the coursework evaluation and this study. A semi structured questionnaire was also used to elicit details about the usefulness of coursework.
Statistical analysis
Descriptive statistics such as mean, standard deviation, number and percentages were used to describe the socio-demographic data. General Linear Model Repeated Measures of Analysis of variance was used to compare knowledge and attitude scores across assessments. Categorical data from the semi structured questionnaire are presented as percentages. All the statistical tests were two-tailed, and a p value < 0.05 was set a priori as the threshold for statistical significance. IBM SPSS (28.0) was used to analyse the data.
One hundred and thirty research scholars who had completed coursework (CW) in the last 2–3 years were considered for the study. These scholars were sent Google forms to assess their knowledge and attitudes 2–3 years after coursework. 81 scholars responded (62%), and 4 scholars did not consent to participate in the study. The data of 77 scholars were merged with the data obtained during the coursework program (before and soon after CW). Socio-demographic characteristics of the scholars are presented in Table 1 .
The age of the respondents ranged from 23 to 36 years, with an average of 28.7 years (3.01), and the majority of the respondents were females (65%). Years of experience (i.e., after masters) before joining a PhD programme ranged from 0.5 to 9 years, and half of them had less than three years of experience before joining the PhD programme (median-3). More than half of those who responded were research scholars from the behavioural sciences (55%), while approximately 30% were from the basic sciences (29%).
General Linear Model Repeated Measures of Analysis of variance was used to compare the knowledge and attitude scores of scholars before, soon after and 2–3 after the coursework (will now be referred as “later the CW”), and the results are presented below (Table 2 ; Fig. 1 ).
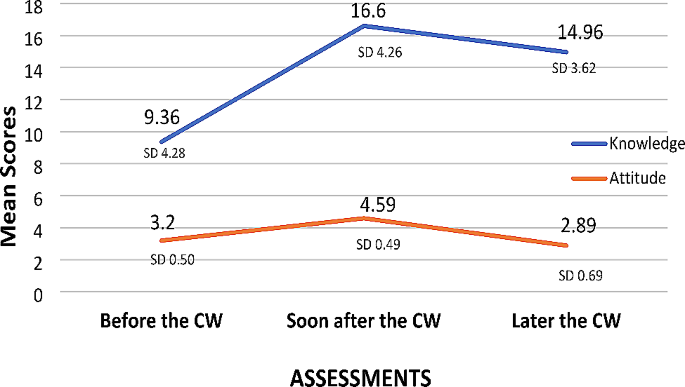
Comparison of knowledge and attitude scores across the assessments. Later the CW – 2–3 years after the coursework
The scores for knowledge and attitude differed significantly across time. Scores of knowledge and attitude increased soon after the coursework; the percentage of change was 77% and 43% respectively. However, significant reductions in knowledge and attitude scores were observed 2–3 years after the coursework compared to scores soon after the coursework. The reduction was higher for attitude scores; knowledge and attitude scores have decreased by 10% and 37% respectively. The change in scores across assessments is evident from the graph, and clearly the effect size is higher for attitude than knowledge.
The scores of knowledge or attitude before the coursework did not significantly differ with respect to gender or age or were not correlated with years of experience. Hence, they were not considered as covariates in the above analysis.
A semi structured questionnaire with open ended questions was also administered to elicit in-depth information about the usefulness of the coursework programme, in which they were also asked to self- rate their knowledge. The data were mostly categorical or narratives. Research scholars’ self-rated knowledge scores (on a scale of 0–10) also showed similar changes; knowledge improved significantly and was retained even after the training (Fig. 2 ).
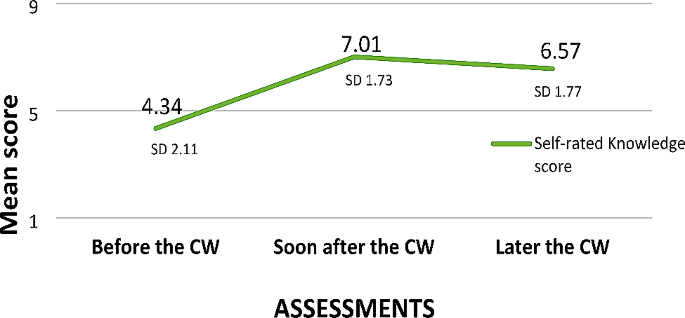
Self-rated knowledge scores of research scholars over time. Later the CW – 2–3 years after the coursework
The response to the question “ How has coursework changed your attitude toward statistics?”, is presented in Fig. 3 . The responses were Yes, positively, Yes - Negatively, No change – still apprehensive, No change – still appreciate, No change – still hate statistics. The majority of the scholars (70%) reported a positive change in their attitude toward statistics. Moreover, none of the scholars reported negative changes. Approximately 9% of the scholars reported that they were still apprehensive about statistics or hate statistics after the coursework.
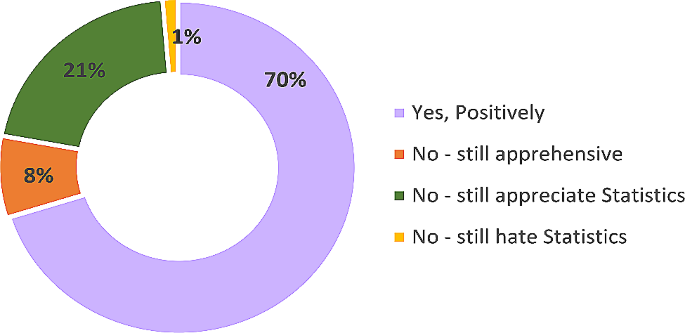
How has coursework changed your attitude toward statistics?
Those scholars who reported that they were apprehensive about statistics or hate statistics noted the complexity of the subject, lack of clarity, improper instructions and fear of mathematics as major reasons for their attitude. Some responses are listed below.
“The statistical concepts were not taught in an understandable manner from the UG level” , “I am weak in mathematical concepts. The equations and formulae in statistics scare me”. “Lack of knowledge about the importance of statistics and fear of mathematical equations”. “The preconceived notion that Statistics is difficult to learn” . “In most of the places, it is not taught properly and conceptual clarity is not focused on, and because of this an avoidance builds up, which might be a reason for the negative attitude”.
Majority of the scholars (92%) felt that coursework has helped them in their PhD, and they were happy to recommend it for other research scholars (97%). The responses of the scholars to the question “ How was coursework helpful in your PhD journey ?”, are listed below.
“Course work gave a fair idea on various things related to research as well as statistics” . “Creating the best design while planning methodology, which is learnt form course work, will increase efficiency in completing the thesis, thereby making it faster”. “Course work give better idea of how to proceed in many areas like literature search, referencing, choosing statistical methods, and learning about research procedures”. “Course work gave a good idea of research methodology, biostatistics and ethics. This would help in writing a better protocol and a better thesis”. “It helps us to plan our research well and to formulate, collect and plan for analysis”. “It makes people to plan their statistical analysis well in advance” .
This study evaluated the effectiveness of the existing coursework programme in an institution of higher medical education, and investigated whether the coursework programme benefits research scholars by improving their knowledge of statistics and attitudes towards statistics. The study concluded that the coursework program was beneficial for improving scholars’ knowledge about statistics and attitudes toward statistics.
Unlike other studies that have assessed attitudes toward statistics, the study participants in this study were research scholars. Research scholars need extensive training in statistics, as they need to apply statistical tests and use statistical reasoning in their research thesis, and in their profession to design research projects or their future student dissertations. Notably, no studies have assessed the attitudes or knowledge of research scholars in statistics either across the PhD training period or after intensive statistics training programs. However, the findings of this study are consistent with the findings of a study that compared the knowledge and attitudes toward teaching and education design of PhD students after a two-day educational course and instructional design workshop [ 14 ].
Statistics educators need not only impart knowledge but they should also motivate the learners to appreciate the role of statistics and to continue to learn the quantitative skills that is needed in their professional lives. Therefore, the role of learners’ attitudes toward statistics requires special attention. Since PhD coursework is possibly a major contributor to creating a statistically literate research community, scholars’ attitudes toward statistics need to be considered important and given special attention. Passionate and engaging statistics educators who have adequate experience in illustrating relatable examples could help scholars feel less anxious and build competence and better attitudes toward statistics. Statistics educators should be aware of scholars’ anxiety, fears and attitudes toward statistics and about its influence on learning outcomes and further interest in the subject.
Strengths and limitations
Analysis of changes in knowledge and attitudes scores across various time points of PhD training is the major strength of the study. Additionally, this study evaluates the effectiveness of intensive statistical courses for research scholars in terms of changes in knowledge and attitudes. This study has its own limitations: the data were collected through online platforms, and the nonresponse rate was about 38%. Ability in mathematics or prior learning experience in statistics, interest in the subject, statistics anxiety or performance in coursework were not assessed; hence, their influence could not be studied. The reliability and validity of the knowledge questionnaire have not been established at the time of this study. However, author who had prepared the questionnaire had ensured questions from different areas of statistics that were covered during the coursework, it has also been used as part of the coursework evaluation. Despite these limitations, this study highlights the changes in attitudes and knowledge following an intensive training program. Future research could investigate the roles of age, sex, mathematical ability, achievement or performance outcomes and statistics anxiety.
The study concluded that a rigorous and intensive training program such as PhD coursework was beneficial for improving knowledge about statistics and attitudes toward statistics. However, the significant reduction in attitude and knowledge scores after 2–3 years of coursework indicates that a refresher program might be helpful for research scholars as they approach the analysis stage of their thesis. Statistics educators must develop innovative methods to teach research scholars from nonstatistical backgrounds. They also must be empathetic to understanding scholars’ anxiety, fears and attitudes toward statistics and to understand its influence on learning outcomes and further interest in the subject.
Data availability
The data that support the findings of this study are available from the corresponding author upon request.
UGC Regulations on Minimum Standards and Procedure for the award of, M.Phil/Ph D, Degree R. 2009. Ugc.ac.in. [cited 2023 Oct 26]. https://www.ugc.ac.in/oldpdf/regulations/mphilphdclarification.pdf .
Althubaiti A. Attitudes of medical students toward statistics in medical research: Evidence from Saudi Arabia. J Stat Data Sci Educ [Internet]. 2021;29(1):115–21. https://doi.org/10.1080/10691898.2020.1850220 .
Hannigan A, Hegarty AC, McGrath D. Attitudes towards statistics of graduate entry medical students: the role of prior learning experiences. BMC Med Educ [Internet]. 2014;14(1):70. https://doi.org/10.1186/1472-6920-14-70 .
Hasabo EA, Ahmed GEM, Alkhalifa RM, Mahmoud MD, Emad S, Albashir RB et al. Statistics for undergraduate medical students in Sudan: associated factors for using statistical analysis software and attitude toward statistics among undergraduate medical students in Sudan. BMC Med Educ [Internet]. 2022;22(1):889. https://doi.org/10.1186/s12909-022-03960-0 .
Zhang Y, Shang L, Wang R, Zhao Q, Li C, Xu Y et al. Attitudes toward statistics in medical postgraduates: measuring, evaluating and monitoring. BMC Med Educ [Internet]. 2012;12(1):117. https://doi.org/10.1186/1472-6920-12-117 .
Bechrakis T, Gialamas V, Barkatsas A. Survey of attitudes towards statistics (SATS): an investigation of its construct validity and its factor structure invariance by gender. Int J Theoretical Educational Pract. 2011;1(1):1–15.
Google Scholar
Khavenson T, Orel E, Tryakshina M. Adaptation of survey of attitudes towards statistics (SATS 36) for Russian sample. Procedia Soc Behav Sci [Internet]. 2012; 46:2126–9. https://doi.org/10.1016/j.sbspro.2012.05.440 .
Coetzee S, Van Der Merwe P. Industrial psychology students’ attitudes towards statistics. J Industrial Psychol. 2010;36(1):843–51.
Francesca C, Primi C. Assessing statistics attitudes among College Students: Psychometric properties of the Italian version of the Survey of attitudes toward statistics (SATS). Learn Individual Differences. 2009;2:309–13.
Counsell A, Cribbie RA. Students’ attitudes toward learning statistics with R. Psychol Teach Rev [Internet]. 2020;26(2):36–56. https://doi.org/10.53841/bpsptr.2020.26.2.36 .
Yoon E, Lee J. Attitudes toward learning statistics among social work students: Predictors for future professional use of statistics. J Teach Soc Work [Internet]. 2022;42(1):65–81. https://doi.org/10.1080/08841233.2021.2014018 .
Melad AF. Students’ attitude and academic achievement in statistics: a Correlational Study. J Posit School Psychol. 2022;6(2):4640–6.
Cook KD, Catanzaro BA. Constantly Working on My Attitude Towards Statistics! Education Doctoral Students’ Experiences with and Motivations for Learning Statistics. Innov High Educ. 2023; 48:257–84. https://doi.org/10.1007/s10755-022-09621-w .
Sohrabi Z, Koohestani HR, Nahardani SZ, Keshavarzi MH. Data on the knowledge, attitude, and performance of Ph.D. students attending an educational course (Tehran, Iran). Data Brief [Internet]. 2018; 21:1325–8. https://doi.org/10.1016/j.dib.2018.08.081 .
Chau C, Stevens J, Dauphine T. Del V. A: The development and validation of the survey of attitudes toward statistics. Educ Psychol Meas. 1995;(5):868–75.
Student attitude surveys. and online educational consulting [Internet]. Evaluationandstatistics.com. [cited 2023 Oct 26]. https://www.evaluationandstatistics.com/ .
Download references
Acknowledgements
The author would like to thank the participants of the study and peers and experts who examined the content of the questionnaire for their time and effort.
This research did not receive any grants from funding agencies in the public, commercial, or not-for-profit sectors.
Author information
Authors and affiliations.
Department of Biostatistics, Dr. M.V. Govindaswamy Centre, National Institute of Mental Health and Neurosciences (NIMHANS), Bangalore, 560 029, India
Mariyamma Philip
You can also search for this author in PubMed Google Scholar
Contributions
Mariyamma Philip: Conceptualization, Methodology, Validation, Investigation, Writing- Original draft, Reviewing and Editing.
Corresponding author
Correspondence to Mariyamma Philip .
Ethics declarations
Ethics approval and consent to participate.
This study used data already collected data (before and soon after coursework). The data pertaining to knowledge and attitude towards statistics 2–3 years after coursework were collected from research scholars through the online survey platform Google forms. The participants were invited to participate in the survey through e-mail. The study was explained in detail, and participation in the study was completely voluntary. Informed consent was obtained online in the form of a statement of consent. The confidentiality of the data was assured, even though identifiable personal information was not collected. This non-funded study was reviewed and approved by NIMHANS Institute Ethics Committee (No. NIMHANS/21st IEC (BS&NS Div.)
Consent for publication
Not applicable because there is no personal information or images that could lead to the identification of a study participant.
Competing interests
The authors declare no competing interests.
Additional information
Publisher’s note.
Springer Nature remains neutral with regard to jurisdictional claims in published maps and institutional affiliations.
Rights and permissions
Open Access This article is licensed under a Creative Commons Attribution 4.0 International License, which permits use, sharing, adaptation, distribution and reproduction in any medium or format, as long as you give appropriate credit to the original author(s) and the source, provide a link to the Creative Commons licence, and indicate if changes were made. The images or other third party material in this article are included in the article’s Creative Commons licence, unless indicated otherwise in a credit line to the material. If material is not included in the article’s Creative Commons licence and your intended use is not permitted by statutory regulation or exceeds the permitted use, you will need to obtain permission directly from the copyright holder. To view a copy of this licence, visit http://creativecommons.org/licenses/by/4.0/ . The Creative Commons Public Domain Dedication waiver ( http://creativecommons.org/publicdomain/zero/1.0/ ) applies to the data made available in this article, unless otherwise stated in a credit line to the data.
Reprints and permissions
About this article
Cite this article.
Philip, M. Measurement and analysis of change in research scholars’ knowledge and attitudes toward statistics after PhD coursework. BMC Med Educ 24 , 512 (2024). https://doi.org/10.1186/s12909-024-05487-y
Download citation
Received : 27 October 2023
Accepted : 29 April 2024
Published : 08 May 2024
DOI : https://doi.org/10.1186/s12909-024-05487-y
Share this article
Anyone you share the following link with will be able to read this content:
Sorry, a shareable link is not currently available for this article.
Provided by the Springer Nature SharedIt content-sharing initiative
- Knowledge of statistics
- Attitude towards statistics
- PhD coursework
- Research scholars
BMC Medical Education
ISSN: 1472-6920
- Submission enquiries: [email protected]
- General enquiries: [email protected]
How patients with chronic disease create value in online health communities? A mixed methods study from social technical perspective
- Published: 08 May 2024
Cite this article
- Jiaxin Xue 1 &
- Zhaohua Deng ORCID: orcid.org/0000-0002-7744-7818 1
Explore all metrics
Online health communities can help patients with chronic diseases to better self-manage their health and provide an effective channel for doctor-patient and patient-patient health value co-creation. However, fewer studies have explored the factors influencing chronic disease patients’ participation in health value co-creation in online health communities from a comprehensive perspective. By combining a mixed method of qualitative and quantitative research, this study established a research model based on the socio-technical systems theory to systematically explore the factors influencing the participation of patients with chronic diseases in health value co-creation. Data were collected from patients with chronic diseases who had used an online health community and partial least square-structural equation modelling was used to test the data. The results revealed that the impact of interactivity on information and emotional support, as well as the impact of social support and service convenience on health value co-creation intention, were significant. The moderating effect of service convenience on the impact of emotional support on health value co-creation has also been validated. Originality: This study provides insights to further understand the psychological characteristics of patients with chronic diseases and optimize the use of online health platforms. From a health management perspective, this study promotes an understanding of the online behavioral characteristics of patients with chronic diseases.
This is a preview of subscription content, log in via an institution to check access.
Access this article
Price includes VAT (Russian Federation)
Instant access to the full article PDF.
Rent this article via DeepDyve
Institutional subscriptions
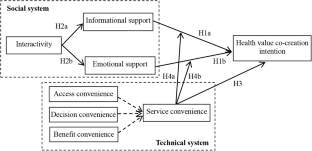
Similar content being viewed by others
Value Co-Creation Activities Role in Patient Well-Being in Online Healthcare Communities
Why People Are Willing to Provide Social Support in Online Health Communities: Evidence from Social Exchange Perspective
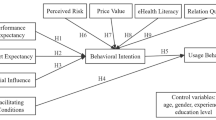
What drives the adoption of online health communities? An empirical study from patient-centric perspective
Holman H, Lorig K (2000) Patients as partners in managing chronic disease. Partnership is a prerequisite for effective and efficient health care. BMJ 320(7234):526–527
Article Google Scholar
Son J, Kim Y, Zhou S (2022) Alerting patients via health information system considering trust-dependent patient adherence. Inform Technol Manage 23(4):245–269. https://doi.org/10.1007/s10799-021-00350-8
De Angelis G, Wells GA, Davies B, King J, Shallwani SM, McEwan J, Cavallo S, Brosseau L (2018) The use of social media among health professionals to facilitate chronic disease self-management with their patients: a systematic review. Digit Health 4:2055207618771416–2055207618771416. https://doi.org/10.1177/2055207618771416
Kim J, Chung K (2020) Knowledge-based hybrid decision model using neural network for nutrition management. Inform Technol Manage 21(1):29–39. https://doi.org/10.1007/s10799-019-00300-5
Gu D, Li M, Yang X, Gu Y, Zhao Y, Liang C, Liu H (2023) An analysis of cognitive change in online mental health communities: a textual data analysis based on post replies of support seekers. Inf Process Manag 60(2). https://doi.org/10.1016/j.ipm.2022.103192
iiMedia Research (2021) Research Report on the Development of Internet Hospital Industry in China, 2021. https://max.book118.com/html/2021/1115/8031014037004037.shtm
Centola D, van de Rijt A (2015) Choosing your network: social preferences in an online health community. Special Issue: Social Networks Health Mental Health 125:19–31. https://doi.org/10.1016/j.socscimed.2014.05.019
Kivits J (2006) Informed patients and the internet: a mediated context for consultations with health professionals. J Health Psychol 11(2):269–282
Gu D, Deng S, Zheng Q, Liang C, Wu J (2019) Impacts of case-based health knowledge system in hospital management: the mediating role of group effectiveness. Inf Manag 56(8). https://doi.org/10.1016/j.im.2019.04.005
Clarke N, Dunne S, Coffey L, Sharp L, Desmond D, O’Conner J, O’Sullivan E, Timon C, Cullen C, Gallagher P (2021) Health literacy impacts self-management, quality of life and fear of recurrence in head and neck cancer survivors. J Cancer Surviv 15(6):855–865. https://doi.org/10.1007/s11764-020-00978-5
Shirazi F, Wu Y, Hajli A, Zadeh AH, Lin X (2021) Value co-creation in online healthcare communities. Technol Forecast Soc Chang 167(2):120665
Peng Y, Wu T, Chen Z, Deng Z (2022) Value Cocreation in Health Care: systematic review. J Med Internet Res 24(3). https://doi.org/10.2196/33061
Porter M, Lee T (2013) The strategy that will fix Health Care. Harvard Business Rev 91(12):24–24
Google Scholar
Mccoll-Kennedy JR, Vargo SL, Dagger TS, Sweeney JC, Kasteren YV (2012) Health Care customer value Cocreation Practice styles. J Service Res 15(4):370–389
Camacho N, Landsman V, Stremersch S (2009) The connected customer: the changing nature of consumer and business markets. Routledge Academic
Keeling DI, Laing A, Newholm T (2015) Health communities as Permissible Space: supporting negotiation to Balance asymmetries. Psychol Mark, 32(3)
Pinho N, Beirao G, Patricio L, Fisk R (2014) Understanding value co-creation in complex services with many actors. J SERVICE Manage 25(4):470–493. https://doi.org/10.1108/JOSM-02-2014-0055
Normann R, Ramirez R (1993) From value chain to value constellation: Designing interactive strategy. Harvard Business Rev 71(4):65–77
Sheth JN, Uslay C (2007) Implications of the revised definition of marketing: from exchange to value creation. J Public Policy Mark 26(2):302–307. https://doi.org/10.1509/jppm.26.2.302
Michie S, Miles J, Weinman J (2003) Patient-centredness in chronic illness: what is it and does it matter? Patient Educ Couns 51(3):197–206. https://doi.org/10.1016/S0738-3991(02)00194-5
Johnson G, Ambrose P (2006) Neo-tribes: the power and potential of online communities in health care. Commun ACM 49(1):107–113. https://doi.org/10.1145/1107458.1107463
Johansson V, Islind A, Lindroth T, Angenete E, Gellerstedt M (2021) Online communities as a driver for patient empowerment: systematic review. J Med Internet Res 23(2). https://doi.org/10.2196/19910
Yan Z, Wang T, Chen Y, Zhang H (2016) Knowledge sharing in online health communities: a social exchange theory perspective. Inf Manag 53(5):643–653. https://doi.org/10.1016/j.im.2016.02.001
Andersen KN, Medaglia R, Henriksen HZ (2012) Social media in public health care: impact domain propositions. Social Media Government - Selections 12th Annual Int Conf Digit Government Res (Dg O2011) 29(4):462–469. https://doi.org/10.1016/j.giq.2012.07.004
Sarah VO, Annouk L, Dominik M (2018) Value co-creation in online healthcare communities: the impact of patients’ reference frames on cure and care. Psychology & Marketing
Luo N, Zhang M, Liu W (2015) The effects of value co-creation practices on building harmonious brand community and achieving brand loyalty on social media in China. Comput Hum Behav 48:492–499
Tajvidi M, Wang YC, Hajli N, Love PED (2021) Brand value Co-creation in social commerce: The role of interactivity, social support, and relationship quality. Computers in Human Behavior, 115. https://doi.org/10.1016/j.chb.2017.11.006
Hsu C-L, Chen M-C (2018) How gamification marketing activities motivate desirable consumer behaviors: focusing on the role of brand love. Comput Hum Behav 88:121–133. https://doi.org/10.1016/j.chb.2018.06.037
Chen C-F, Wang J-P (2016) Customer participation, value co-creation and customer loyalty – a case of airline online check-in system. Comput Hum Behav 62:346–352. https://doi.org/10.1016/j.chb.2016.04.010
Hsieh PL (2015) Encounters in an online brand community: development and validation of a Metric for Value Co-creation by customers. Cyberpsychology Behav Social Netw 18(5):286–295
Trist E (1981) The evolution of Sociotechnical Systems. Ontario Ministry of Labour
Bostrom RP, Heinen JS (1977) MIS problems and failures: A socio-technical perspective, part II: the application of socio-technical theory. MIS Q 1(4):11–28
Appelbaum SH (1997) Socio-technical systems theory: an intervention strategy for organizational development. Manag Decis 35(6):452–463
Hyer NL, Brown KA, Zimmerman S (1999) A socio-technical systems approach to cell design: case study and analysis. J Oper Manag 17(2):179–203
Wu T, Deng Z, Chen Z, Zhang D, Wu X, Wang R (2019) Predictors of patients’ loyalty toward doctors on web-based health communities: cross-sectional study. J Med Internet Res, 21(9), e14484
Chai S, Kim M (2009) A socio-technical approach to knowledge contribution behavior: an empirical investigation of social networking sites users. Int J Inf Manag 29(2):118–126
Choi SY, Kang YS, Lee H (2008) The effects of socio-technical enablers on knowledge sharing: an exploratory examination. J Inform Sci 34(5):742–754
Wan J, Lu Y, Wang B, Zhao L (2017) How attachment influences users’ willingness to donate to content creators in social media: A socio-technical systems perspective. Inf Manag 54(7):837–850
Zhang M, Liu Y, Wang Y, Zhao L (2022) How to retain customers: understanding the role of trust in live streaming commerce with a socio-technical perspective. Comput Hum Behav 127. https://doi.org/10.1016/j.chb.2021.107052
Tortorella G, Fogliatto F, Kumar M, Gonzalez V, Pepper M (2023) Effect of industry 4.0 on the relationship between socio-technical practices and workers’ performance. J Manuf Technol Manage 34(1):44–66. https://doi.org/10.1108/JMTM-04-2022-0173
Lombardo G, Mordonini M, Tomaiuolo M (2021) Adoption of Social Media in Socio-Technical systems: a Survey. INFORMATION 12(3). https://doi.org/10.3390/info12030132
Leimeister J, Ebner W, Krcmar H (2005) Design, implementation, and evaluation of trust-supporting components in virtual communities for patients. J Manage Inform Syst 21(4):101–135. https://doi.org/10.1080/07421222.2005.11045825
Xue J, Deng Z, Wu T, Chen Z (2023) Patient distrust toward doctors in online health communities: integrating distrust construct model and social-technical systems theory. Inform Technol PEOPLE 36(4):1414–1438. https://doi.org/10.1108/ITP-03-2021-0197
Pew Research Center (2013) Health online 2013. https://www.pewinternet.org/~/media//Files/Reports/PIP_HealthOnline.pdf
Chen Y, Xu Y (2021) Exploring the Effect of Social Support and Empathy on user Engagement in Online Mental Health communities. Int J Environ Res Public Health 18(13). https://doi.org/10.3390/ijerph18136855
Wang Y, Bao S, Chen Y (2023) How does social media use influence the mental health of pancreatic cancer patients: a chain mediating effect of online social support and psychological resilience. Front PUBLIC HEALTH 11. https://doi.org/10.3389/fpubh.2023.1166776
Audrain-Pontevia A, Menvielle L (2018) Do online health communities enhance patient-physician relationship? An assessment of the impact of social support and patient empowerment. HEALTH Serv Manage Res 31(3):154–162. https://doi.org/10.1177/0951484817748462
Califf C, Sarker S, Sarker S, DARK SIDES OF TECHNOSTRESS: A MIXED-METHODS STUDY INVOLVING HEALTHCARE IT (2020) MIS Q 44(2):809–856. https://doi.org/10.25300/MISQ/2020/14818 . THE BRIGHT AND
Hou T, Luo X, Ke D, Cheng X (2022) Exploring different appraisals in deviant sharing behaviors: a mixed-methods study. J Bus Res 139:496–509. https://doi.org/10.1016/j.jbusres.2021.09.066
Plowman D, Baker L, Beck T, Kulkarni M, Solansky S, Travis D (2007) Radical change accidentally: the emergence and amplification of small change. Acad Manag J 50(3):515–543
Wunderlich P, Veit D, Sarker S (2019) ADOPTION OF SUSTAINABLE TECHNOLOGIES: A MIXED-METHODS STUDY OF GERMAN HOUSEHOLDS. MIS Q 43(2):673–. https://doi.org/10.25300/MISQ/2019/12112
Kim S, Graham S, Ahn S, Olson M, Card D, Kessler M, DeVasto D, Roberts L, Bubacy F (2016) Correcting biased Cohen’s Kappa in NVivo. COMMUNICATION METHODS MEASURES 10(4):217–232. https://doi.org/10.1080/19312458.2016.1227772
Zhaohua D, Zihao D, Guorui F, Bin W, Weiguo (Patrick) F, Shan L (2023) More is better? Understanding the effects of online interactions on patients health anxiety. Journal of the Association for Information Science and Technology , 74(11): 1243–1264
Thoits PA (1982) Conceptual, methodological, and theoretical problems in studying social support as a buffer against life stress. J Health Soc Behav 23(2):145–159
Madjar N (2011) Emotional and informational support from different sources and employee creativity. J Occup Organizational Psychol 81(1):83–100
Liang TP, Ho YT, Li YW, Turban E (2011) What drives Social Commerce: the role of Social Support and Relationship Quality. Int J Electron Commer 16(2):69–90
Yan L, Tan Y (2014) Feeling blue? Go online: an empirical study of Social Support among patients. Inform Syst Res 25(4):690–709
Taylor S, Sherman D, Kim H, Jarcho J, Takagi K, Dunagan M (2004) Culture and Social Support who seeks it and why? J Personality Social Psychol 87(3):354–362
Brodie RJ, Ilic A, Juric B, Hollebeek L (2013) Consumer engagement in a virtual brand community: an exploratory analysis. J Bus Res 66(1):105–114
Josefsson U (2005) Coping with illness online: the case of patients’ online communities. Inform Soc 21(2):143–153. https://doi.org/10.1080/01972240590925357
Hajli MN, Shanmugam M, Hajli A, Khani AH, Wang Y (2014) Health care development: integrating transaction cost theory with social support theory. Inf Health Social Care 40(4):334–344
Dennis CL (2003) Peer support within a health care context: a concept analysis. Int J Nurs Stud 40(3):321–332
Barrett M, Oborn E, Orlikowski W (2017) Creating value in online communities: the sociomaterial configuring of strategy, platform and stakeholder engagement. Oper Res 57(5–6):525–528
Jadad A, Enkin M, Glouberman S, Groff P, Stern A (2006) Are virtual communities good for our health? They seem to be good at managing chaotic information—and may have other virtues too. BMJ-BRITISH Med J 332(7547):925–926. https://doi.org/10.1136/bmj.332.7547.925
Saenger C, Thomas VL, Johnson JW (2013) Consumption-focused self‐expression word of Mouth: a New Scale and its role in Consumer Research. Psychol Mark, 30(11)
Lindsay S, Smith S, Bellaby P, Baker R (2009) The health impact of an online heart disease support group: a comparison of moderated versus unmoderated support. Health Education Research
Gross BL (1987) Time scarcity: interdisciplinary perspectives and implications for consumer behavior. Res Consumer Behav 2(2):1–54
Srinivasan SS, Anderson R, Ponnavolu K (2002) Customer loyalty in e-commerce: An exploration of its antecedents and consequences
Nah FH, Davis S (2002) HCI research issues in E-commerce. J Electron Commer Res
Tarafdar M, Zhang J (2008) Determinants of Reach and Loyalty—A study of website performance and implications for website design. J Comput Inform Syst XLVIII(2):16–24
Berry LL, Seiders K, Grewal D (2002) Understanding Service Convenience. J Mark 66(3):1–17
Roy S, Shekhar V, Lassar W, Chen T (2018) Customer engagement behaviors: the role of service convenience, fairness and quality. J RETAILING CONSUMER Serv 44:293–304. https://doi.org/10.1016/j.jretconser.2018.07.018
Davenport TH, Prusak L (1998) Working knowledge: how organizations manage what they know. Harvard Business
Lam L, Chuang A, Wong C, Zhu J (2019) A typology of three-way interaction models: applications and suggestions for Asian management research. ASIA Pac J Manage 36(1):1–16. https://doi.org/10.1007/s10490-018-9577-9
Farquhar J, Rowley J (2009) Convenience: a services perspective. Mark THEORY 9(4):425–438. https://doi.org/10.1177/1470593109346894
Chen Z, Chen Q, Hu M (2021) Health and Healthcare in China: An Editorial Introduction. The Chinese Economy, 1–3. https://doi.org/10.1080/10971475.2021.1996549
China Internet Network Information Center (2023), August 28 The 52th Statistical Reports on Internet Development in China
Liu S, Xiao WY, Fang C, Zhang X, Lin JB (2020) Social support, belongingness, and value co-creation behaviors in online health communities. Telematics Inform 50:101398. https://doi.org/10.1016/j.tele.2020.101398
Zhang X, Liu S, Chen X, Wang L, Gao BJ, Zhu Q (2018) Health information privacy concerns, antecedents, and information disclosure intention in online health communities. Inf Manag 55(4):482–493. https://doi.org/10.1016/j.im.2017.11.003
Chen H-Y, Boore JRP (2010) Translation and back-translation in qualitative nursing research: methodological review. J Clin Nurs 19(1–2):234–239. https://doi.org/10.1111/j.1365-2702.2009.02896.x
Becker J-M, Klein K, Wetzel M (2012) Hierarchical latent variable models in PLS-SEM: guidelines for using reflective-formative type model. Long Range Plann 45(5–6):359–394
James TL, Wallace L, Deane JK, ORGANISMIC INTEGRATION THEORY TO EXPLORE THE ASSOCIATIONS BETWEEN USERS’ EXERCISE MOTIVATIONS AND FITNESS TECHNOLOGY FEATURE SET USE (2019) MIS Q 43(1):287–. https://doi.org/10.25300/misq/2019/14128 . USING
Anderson JC, Gerbing DW (1988) Structural equation modeling in practice: a review and recommended two-step approach. Psychol Bull 103(3):411–423
Fornell C, Larcker FD (1981) Structural equation models with unobservable variables and measurement error: Algebra and statistics. J Mark Res 18(3):382–388
Churchill GA (1979) A paradigm for developing Better Measures of Marketing Constructs. J Mark Res 16(1):12–27
Mathwick C, Malhotra N, Rigdon E (2001) Experiential value: conceptualization, measurement and application in the catalog and internet shopping environment☆11☆This article is based upon the first author’s doctoral dissertation completed while at Georgia Institute of Technology. J Retail 77(1):39–56. https://doi.org/10.1016/S0022-4359(00)00045-2
Chan TKH, Cheung CMK, Wong RYM (2019) Cyberbullying on Social networking sites: the crime opportunity and affordance perspectives. J Manage Inform Syst 36(2):574–609. https://doi.org/10.1080/07421222.2019.1599500
Richardson HA, Simmering MJ, Sturman MC (2009) A tale of three perspectives examining Post Hoc Statistical Techniques for Detection and Correction of Common Method Variance. Organizational Res Methods 12(4):762–800. https://doi.org/10.1177/1094428109332834
Cenfetelli RT, Bassellier G (2009) Interpretation of the Formative Measurement in Information Systems Research. MIS Q 33(4):689–707
Prahalad CK, Ramaswamy V (2004) Co-creating unique value with customers. Strategy Leadersh 32(3):4–9. https://doi.org/10.1108/10878570410699249
Zhao J, Wang T, Fan X (2015) Patient value co-creation in online health communities social identity effects on customer knowledge contributions and membership continuance intentions in online health communities. J SERVICE Manage 26(1):72–96. https://doi.org/10.1108/JOSM-12-2013-0344
See-To EWK, Ho KKW (2014) Value co-creation and purchase intention in social network sites: the role of electronic word-of-mouth and trust – a theoretical analysis. Comput Hum Behav 31:182–189
Yu CH, Tsai CC, Wang Y, Lai KK, Tajvidi M (2018) Towards building a value co-creation circle in social commerce. Computers in Human Behavior, S074756321830181X
Liu W, Fan X, Ji R, Jiang Y (2020) Perceived Community support, users’ interactions, and Value Co-creation in Online Health Community: the moderating effect of Social Exclusion. Int J Environ Res Public Health 17(1). https://doi.org/10.3390/ijerph17010204
Lee AR, Kim KK (2018) Customer benefits and value co-creation activities in corporate social networking services. Behav Inform Technol 37(7):675–692. https://doi.org/10.1080/0144929X.2018.1474252
Carranza R, Díaz E, Muoz SC, Navarro MC (2020) e-Banking adoption: an opportunity for customer value co-creation. Front Psychol 11(621248):1–10
Guo L, Zhang M, Wang Y (2016) Effects of customers’ psychological characteristics on their engagement behavior in company social networks. Social Behav Personality: Int J, 44(10)
Lin L, Huang Z, Othman B, Luo Y (2020) Let’s make it better: an updated model interpreting international student satisfaction in China based on PLS-SEM approach. PLoS ONE, 15(7), e0233546
Lv X, Zhang R, Li Q (2021) Value co-destruction: the influence of failed interactions on members’ behaviors in online travel communities. Comput Hum Behav, 122(106829)
Zhao J, Jing F (2016) The influence of Online Brand Community Climate on the customer Innovation Behavior. J Manage Sci, 47(3), e7649
Sharma S, Conduit J, Hill S (2017) Hedonic and eudaimonic well-being outcomes from co-creation roles: a study of vulnerable customers. J Serv Mark 31(4–5):397–411. https://doi.org/10.1108/JSM-06-2016-0236
Osei-Frimpong K, Wilson A, Lemke F (2018) Patient co-creation activities in healthcare service delivery at the micro level: the influence of online access to healthcare information. Technol Forecast Soc Chang 126:14–27. https://doi.org/10.1016/j.techfore.2016.04.009
Caic M, Odekerken-Schroder G, Mahr D (2018) Service robots: Value co-creation and co-destruction in elderly care networks. J SERVICE Manage 29(2):178–205. https://doi.org/10.1108/JOSM-07-2017-0179
Davey J, Gronroos C (2019) Health service literacy: complementary actor roles for transformative value co-creation. J Serv Mark 33(6):687–701. https://doi.org/10.1108/JSM-09-2018-0272
Download references
This work was supported by the National Natural Science Foundation of China [No. 71971092 & No. 72271102], and The National Social Science Fund of China [No. 22VRC153].
Author information
Authors and affiliations.
School of Management, Huazhong University of Science and Technology, 1037 Luoyu Road, Hongshan District, Wuhan, Hubei Province, China
Jiaxin Xue & Zhaohua Deng
You can also search for this author in PubMed Google Scholar
Corresponding author
Correspondence to Zhaohua Deng .
Ethics declarations
Conflict of interest.
The authors have no relevant financial or non-financial interests to disclose.
Additional information
Publisher’s note.
Springer Nature remains neutral with regard to jurisdictional claims in published maps and institutional affiliations.
Appendix A. Literature review on value co-creation (except healthcare)
Appendix b. literature review on value co-creation (healthcare), appendix c. definition and items of constructs, appendix d. multicollinearity test.
By running the entire model as a basic regression onto the dependent variable (Health value co-creation intention), we checked the variance inflation factors (VIF) to test for multicollinearity. As shown in Table D, we find that the VIF scores are all below the conservative threshold of 3.3 [ 89 ], so we believe that multicollinearity is not a problem for this model.
Rights and permissions
Springer Nature or its licensor (e.g. a society or other partner) holds exclusive rights to this article under a publishing agreement with the author(s) or other rightsholder(s); author self-archiving of the accepted manuscript version of this article is solely governed by the terms of such publishing agreement and applicable law.
Reprints and permissions
About this article
Xue, J., Deng, Z. How patients with chronic disease create value in online health communities? A mixed methods study from social technical perspective. Inf Technol Manag (2024). https://doi.org/10.1007/s10799-024-00424-3
Download citation
Accepted : 20 April 2024
Published : 08 May 2024
DOI : https://doi.org/10.1007/s10799-024-00424-3
Share this article
Anyone you share the following link with will be able to read this content:
Sorry, a shareable link is not currently available for this article.
Provided by the Springer Nature SharedIt content-sharing initiative
- Online health community
- Value co-creation
- Chronic disease patients
- Socio-technical systems theory
- Find a journal
- Publish with us
- Track your research
- Skip to main content
- Skip to FDA Search
- Skip to in this section menu
- Skip to footer links

The .gov means it’s official. Federal government websites often end in .gov or .mil. Before sharing sensitive information, make sure you're on a federal government site.
The site is secure. The https:// ensures that you are connecting to the official website and that any information you provide is encrypted and transmitted securely.
U.S. Food and Drug Administration
- Search
- Menu
- Vaccines, Blood & Biologics
- Science & Research (Biologics)
Development of Quantitative Assays to Evaluate the Safety of Cell Substrates and Vaccines
Keith Peden, PhD
Office of Vaccines Research and Review Division of Viral Products Laboratory of DNA Viruses
Dr. Keith Peden obtained his Ph.D. from the MRC Mammalian Genome Unit, Department of Zoology, University of Edinburgh, UK. He then carried out post-doctoral research in the Department of Molecular Biology, University of Edinburgh, UK, and at the Department of Molecular Biology and Genetics with Dan Nathans at the Johns Hopkins University School of Medicine, Baltimore. Following postdoctoral training, Dr. Peden spent a year at the Pasteur Institute (1988/89) with Luc Montagnier working on HIV. The work involved generating infectious molecular clones of HIV-1 and HIV-2 and initiating studies on the viral accessory genes. He then spent 5 years at the NIH continuing work on HIV-1 and HIV-2 before moving to CBER/FDA in 1994.
Dr. Peden’s research at CBER first focused on HIV but turned to addressing perceived safety issues with the use of tumorigenic cell substrates for the manufacture of vaccines against viral diseases. Currently, he is Chief of the Laboratory of DNA Viruses in the Division of Viral Products, Office of Vaccines Research and Review, CBER, FDA, where the major focus of his research continues to address safety issues associated with cell substrates for vaccine production. More recently, he has been developing of micro-neutralization assays to assess the effectiveness of vaccines. The overall aims of both areas of research are to facilitate the introduction of effective vaccines against viral diseases.
General Overview
Viral vaccines are produced in animal cells called “cell substrates.” Therefore, the characteristics of these cell substrates affect the quality of the vaccine. Our division regulates vaccines against infectious diseases caused by viruses, and our research program is directed at both developing new tools that could be used to evaluate the safety of cell substrates used to produce viral vaccines and developing methods to evaluate the effectiveness of vaccines.
A number of vaccines protect the public against viral diseases such as polio, influenza, measles, mumps, rubella, varicella, smallpox, and rotavirus. These vaccines, which are made in monkey, chick, or human cell substrates, are safe and effective. However, not all vaccines can be manufactured in current cell substrates for two reasons: 1) the vaccine viruses may not be able to grow in these cells; 2) the yield of virus may be too low for the product to be commercially viable. Nevertheless, because new vaccines are required to combat emerging and re-emerging infectious viruses (e.g., pandemic influenza viruses, Ebola virus, MERS coronavirus, Zika virus, and Lassa virus) and also against HIV/AIDS, new cell substrates are necessary.
The reason that only a limited variety of cell substrates are used is that scientists in the 1950s concluded that cell lines established from human tumors should not be used to make preventative vaccines. The rationale for this decision was based on the concern that if a cell substrate were derived from a human tumor, or was shown experimentally to be able to form a tumor in an animal (i.e., the cells were tumorigenic), then components from those cells could be present in vaccines manufactured in them and these components might be able to induce cancer or other diseases in recipients of the vaccines.
However, the growth of some viruses requires the use of these kinds of cells, and as a consequence, using these cells to develop new vaccines remains of interest. Over the past several decades, researchers have learned much about how cancer develops, and this knowledge has allowed FDA to reopen the question about the safety concerns with using tumorigenic cells for vaccine production and to address these concerns in a mechanistic and data-driven manner. Our laboratory is developing new approaches, using rodent models as well as in vitro studies, to investigate whether the use of tumorigenic cells or cells derived from human tumors for vaccine production poses safety concerns to the recipients of those vaccines.
An additional component of our work is developing assays that can measure neutralizing antibodies induced by vaccines and that can be adapted to high throughput. Because most vaccines owe their effectiveness to eliciting neutralizing antibodies, which are proteins that block infection and/or production of the virus, determining whether neutralizing antibodies are induced following vaccination is an important component of determining whether the vaccine is effective. Additionally, developing novel neutralization assays might aid the evaluation of the effectiveness of vaccines and thus facilitate their licensure.
Scientific Overview
The variety of cell substrates used to manufacture licensed vaccines has been limited to primary avian or monkey cells, human diploid cells, and the VERO cell line. This repertoire is insufficient for the production of the next generation of vaccines. All mammalian cell substrates being evaluated are neoplastic, since they are immortal, and some are tumorigenic. The fear that components from the cell substrate could induce cancer in vaccine recipients was the main reason that tumorigenic cells were originally prohibited for vaccine manufacture in the 1950s. Several expert panels have concluded that the major concerns with using these types of cells are the potential presence of adventitious agents and the unavoidable presence of small quantities of residual cell substrate DNA in vaccines. Our laboratory is developing assays to evaluate the safety of novel cell substrates with respect to the latter issue, namely, whether residual cellular DNA could pose a safety risk. The potential risk from DNA comes from its known biological activities: cell-substrate DNA may encode an infectious genome (the infectivity activity of DNA), and DNA may encode dominant activated oncogenes that could transform the vaccine recipient’s cells to become cancerous (the oncogenic activity of DNA). To evaluate DNA oncogenicity, we are developing in vivo assays to quantify the oncogenicity of DNA. These assays involve identifying sensitive rodent systems that can detect oncogenic activity of positive-control expression plasmids for dominant oncogenes. If such rodent systems can be identified, then DNA from the cell substrate will be assessed for its oncogenic activity in these systems. These studies could allow us to estimate the oncogenic risks from DNA. The resulting estimates will be conservative, since they will be based on the most sensitive assays available.
A second project is to identify the mechanism by which cells become tumorigenic. Many mammalian cell substrates (VERO, MDCK, CHO) were derived from primary cells by passage in culture. These cells became immortal, i.e., capable of indefinite passaging, but sometimes with further passaging they become tumorigenic. We are investigating the mechanism by which this occurs, in order to determine whether there is a risk from using tumorigenic cells for vaccine production.
A third project is to develop quantitative high-throughput micro-neutralization assays to detect neutralizing antibodies to viruses. Because most licensed vaccines are effective through eliciting neutralizing antibodies, methods that can quantify these antibodies are critical to vaccine development. When a human pathogenic virus cannot be propagated under standard laboratory conditions and requires high containment (e.g., Ebola, Lassa, and Nipah viruses), and even for viruses that require BSL-3 containment (e.g., SARS-CoV, MERS-CoV, and the recently isolated SARS-CoV-2), evaluating neutralizing antibodies against such viruses is complicated. We have been exploring alternative methodologies that can be used in standard BSL-2 laboratories to quantify neutralizing antibodies, such as using replication-competent hybrid viruses. In addition, the hybrid viruses can themselves be used to study the biology of the original virus and also to generate reagents for the development of vaccines.
Important Links
Innovation and Regulatory Science - Research Summary: FDA develops rapid and sensitive assay to assess antibody response to Ebola virus vaccine without using the virus
Publications
- PLoS One 2023 Dec 7;18(12):e0293406 GLI1+ perivascular, renal, progenitor cells: The likely source of spontaneous neoplasia that created the AGMK1-9T7 cell line. Lewis AM Jr, Foseh G, Tu W, Peden K, Akue A, KuKuruga M, Rotroff D, Lewis G, Mazo I, Bauer SR
- Biologicals 2023 Nov;84:101724 Evaluating the sensitivity of newborn rats and newborn hamsters to oncogenic DNA. Sheng-Fowler L, Tu W, Phy K, Macauley J, Lanning L, Lewis AM Jr, Peden K
- Curr Top Microbiol Immunol 2022;440:187-205 Regulatory considerations on the development of mRNA vaccines. Naik R, Peden K
- PLoS One 2022 Oct 24;17(10):e0275394 The AGMK1-9T7 cell model of neoplasia: evolution of DNA copy-number aberrations and miRNA expression during transition from normal to metastatic cancer cells. Lewis AM Jr, Thomas R, Breen M, Peden K, Teferedegne B, Foseh G, Motsinger-Reif A, Rotroff D, Lewis G
- J Virol 2022 Sep;96(18):e0116621 Enhanced in vitro and in vivo potency of a T cell epitope in the Ebola virus glycoprotein following amino acid replacement at HLA-A*02:01 binding positions. Chabot S, Gimie Y, Obeid K, Kim J, Meseda CA, Konduru K, Kaplan G, Sheng Fowler L, Weir JP, Peden K, Major ME
- Vaccines 2021 Jan 23;9(2):81 Development of mRNA vaccines: scientific and regulatory issues. Knezevic I, Liu MA, Peden K, Zhou T, Kang HN
- NPJ Vaccines 2020 Jun 18;5:52 WHO informal consultation on the guidelines for evaluation of the quality, safety, and efficacy of DNA vaccines, Geneva, Switzerland, December 2019. Sheets R, Kang HN, Meyer H, Knezevic I, Sheets R, Kang HN, Meyer H, Knezevic I, Abwao E, Alali M, Aprea P, Bae C, Blades CDRZ, Boyer J, Broderick KE, Duffy P, Farnsworth A, Gangakhedkar J, Gutsch D, Hafiz RA, Jackson N, Kaslow D, Khan AS, Ledgerwood J, Liu MA, Maslow J, Nkansah E, Park Y, Patel A, Peden K, Racine T, Rose N, Roy P, Song M, Wei W
- Vaccine 2020 Feb 18;38(8):1869-80 The science of vaccine safety: summary of meeting at Wellcome Trust. Plotkin SA, Offit PA, DeStefano F, Larson HJ, Arora NK, Zuber PLF, Fombonne E, Sejvar J, Lambert PH, Hviid A, Halsey N, Garcon N, Peden K, Pollard AJ, Markowitz LE, Glanz J
- NPJ Vaccines 2019 Oct 14;4:42 Harmonization of Zika neutralization assays by using the WHO International Standard for anti-Zika virus antibody. Mattiuzzo G, Knezevic I, Hassall M, Ashall J, Myhill S, Faulkner V, Hockley J, Rigsby P, Wilkinson DE, Page M, collaborative study participants
- Vaccine X 2019 Apr 11;(1):100004 Responsiveness to basement membrane extract as a possible trait for tumorigenicity characterization. Murata H, Omeir R, Tu W, Lanning L, Phy K, Foseh G, Lewis AM Jr, Peden K
- Vaccine 2017 Oct 4;35(41):5481-6 Development of a micro-neutralization assay for ebolaviruses using a replication-competent vesicular stomatitis hybrid virus and a quantitative PCR readout. Lee SS, Phy K, Peden K, Sheng-Fowler L
- Vaccine 2015 Dec 16;33(51):7254-61 RT-qPCR-based microneutralization assay for human cytomegalovirus using fibroblasts and epithelial cells. Wang X, Peden K, Murata H
- Chromosome Res 2015 Dec;23(4):663-80 A novel canine kidney cell line model for the evaluation of neoplastic development: karyotype evolution associated with spontaneous immortalization and tumorigenicity. Omeir R, Thomas R, Teferedegne B, Williams C, Foseh G, Macauley J, Brinster L, Beren J, Peden K, Breen M, Lewis AM Jr
ORIGINAL RESEARCH article
Empowering pro-environmental behavior in tourists through digital media: the influence of eco-guilt and empathy with nature.
- 1 College of Landscape Architecture and Tourism, Hebei Agricultural University, Baoding, China
- 2 Business School, Guangzhou College of Technology and Business, Guangzhou, China
In the digital economy era, leveraging digital media to foster tourists’ pro-environmental behavioral intention (TPEBI) has become crucial in the field of sustainable tourism. While existing studies have mainly focused on the driving mechanism of TPEBI within physical tourism contexts, the correlation between digital media information sharing and TPEBI remains unclear. Our study employs the cognitive-affective-conative framework to construct a theoretical model, considering eco-guilt and empathy with nature as mediating variables. It aims to explore the influencing mechanism of destination environmental information sharing through digital media on TPEBI from a presence perspective. Thereby, two scenario experiments were designed: Study 1 examined the impact of different formats of destination environmental threat information presentation on digital media on the sense of presence, while Study 2 explored the influencing mechanism of presence on TPEBI based on the conclusions of Study 1. Results indicate that (1) vivid and visible presentation formats of destination environmental threat information on digital media enhance individuals’ sense of presence; (2) sense of presence positively influences TPEBI; and (3) eco-guilt and empathy with nature mediate between presence and TPEBI. These findings not only contribute to theoretical and empirical research on digital media information sharing in sustainable tourism but also offer guidance for governments and tourism destinations to effectively stimulate TPEBI through digital media, achieve the sustainable development of destinations.
1 Introduction
The ecological environment stands as a cornerstone for the sustainable development of tourism destinations. However, the exponential surge in tourist arrivals has exacerbated the damage inflicted by tourism activities on destination ecosystems, posing significant challenges to sustainable development ( Lee et al., 2021 ). Hence, it becomes paramount to educate tourists about their responsibilities and foster responsible behavior throughout their travels. Typically, tourism unfolds in unique environments and within constrained timeframes. The influence of daily moral norms on tourists may be limited, while the repercussions of violating social norms are relatively minor, often leading to uncivilized behavior ( Zhang et al., 2019 ; Li and Chen, 2021 ). In recent years, media exposure has highlighted instances of improper conduct such as littering, trampling vegetation, and defacing landmarks by tourists in destinations. Consequently, discerning the primary factors influencing tourists’ pro-environmental behavioral intention (TPEBI) has emerged as a pivotal endeavor for the sustainable development of tourism destinations ( Wang G. Q. et al., 2023 ).
Existing studies on the influencing mechanism of TPEBI primarily concentrate on the field tourism context, emphasizing aspects such as travel experience ( Lin and Lee, 2020 ; Lee and Jan, 2023 ), destination psychological ownership ( Qiu et al., 2022 ), and environmental commitment ( Tang et al., 2022 ) as antecedent variables. However, they often overlook factors pertinent to the digital virtual context, hindering the widespread dissemination of environmental education. In light of the rapid proliferation of the Internet and intelligent terminal devices, individuals now possess the ability to share information regarding environmental threats in tourism destinations on digital media platforms at any time and from any location ( Meng et al., 2023 ). This trend has the potential to draw greater attention to destination environmental issues. Nevertheless, the specific types of environmental information presentation formats (such as text-based, image-based, or video-based) that are most effective in capturing individuals’ attention toward ecological concerns in tourism destinations and subsequently stimulating their TPEBI remain unclear. Addressing these pertinent issues is imperative.
Several studies have indicated a close relationship between the presentation format of information in virtual environments and the sense of presence ( Verhagen et al., 2014 ). The sense of presence, as an individual’s sensory experience within a media environment, reflects the extent to which individuals perceives themselves in the virtual setting ( Ou et al., 2014 ). With the rapid advancements in mobile Internet and digital technology, the sense of presence finds widespread application in areas such as live-streamed shopping, information dissemination, and online education ( Xu et al., 2021 ; Yao and Jia, 2021 ; Guo et al., 2023 ). Vonkeman et al. (2017) found that in online environments, the more vivid and interactive the presentation format of media information, the higher the sense of presence experienced by individuals. Furthermore, presence plays a crucial role in understanding attitudes and behaviors in virtual environments. Scholars have extensively explored the relationship between presence and individual behavioral decision-making, yielding significant insights. For example, Chen (2023) confirmed the positive impact of virtual presence on consumers’ online shopping intentions using the Stimulus-Organism-Response paradigm. Additionally, Yao and Jia (2021) explored the influence of presence on impulse travel intentions within the digital media context. It is evident that the sense of presence plays a pivotal role in mediating the relationship between the presentation format of media information and user behavioral intentions. However, the precise correlation mechanism of presence between digital media environmental information sharing and TPEBI remains elusive.
In light of this, our study constructs a mediation model based on the cognitive-affective-conative framework, incorporating eco-guilt and empathy with nature (affective factor). It aims to explore the influencing mechanism of destination environmental information sharing through digital media on TPEBI from the perspective of presence (cognitive factor). The main contributions of our study are as follows: (1) Examining the differences in the impact of various environmental threat information presentation formats via digital media on presence, which enriches our understanding of the formation mechanism of presence. (2) Clarifying that digital media serves as an effective pathway to stimulate TPEBI, which expands research on the driving mechanism of TPEBI from the traditional field tourism context to the digital virtual context. (3) Revealing the influencing mechanism of presence on TPEBI, which expands the explanatory power of presence to the domain of responsible tourism behavior, offering a fresh perspective for comprehending the relationship between digital media information sharing and individual behavior decision-making. (4) Verifying the mediating effect of eco-guilt and empathy with nature between presence and TPEBI, which provides a new theoretical framework for studying the influencing mechanism between presence and individual behavioral intention.
2 Literature review
2.1 digital media information sharing.
With the rapid advancement of digital technology and the widespread use of instant communication tools, various digital media platforms (such as Facebook, WeChat, Weibo, etc.) have permeated every aspect of social life, profoundly influencing and reshaping people’s consumption patterns, communication habits, collaborative efforts, and other behaviors ( Liu et al., 2024 ). In the realm of tourism, digital media plays a pivotal role, subtly shaping and influencing tourists’ behavioral decisions ( Yao and Jia, 2021 ). Existing studies on the impact of digital media information sharing on tourists predominantly focus on its effects on travel intentions ( Yao and Jia, 2021 ; Hussain et al., 2024 ), tourism experiences ( Jamshidi et al., 2023 ), destination image ( Liu et al., 2024 ), with more attention devoted to analyzing the features of the shared information ( Jamshidi et al., 2023 ). However, in reality, digital media information encompasses various presentation formats such as text, images, and videos, each potentially exerting different influences on tourists ( Yao and Jia, 2021 ). Therefore, our study seeks to explore the disparities in the impact of different environmental information presentation formats on individual attitudes and behavioral decisions within the context of digital media.
2.2 Presence
The concept of presence originates from telepresence, denoting the extent to which individuals perceive reality within a virtual environment ( Lombard and Jones, 2007 ). When an individual’s perception of the virtual environment surpasses that of the physical environment, a sense of presence ensues ( Pelet et al., 2017 ). Scholars often categorize presence into two dimensions: spatial presence and social presence. Spatial presence, also referred to as physical presence, pertains to the authenticity of an individual’s perception of the physical environment constructed by virtual information, indicating a sense of immersion. Whereas, social presence relates to individuals’ perception of interaction within a virtual environment, emphasizing a feeling of companionship ( Ou et al., 2014 ). In this study, presence primarily denotes the immersive feeling individuals experience when browsing destination environmental information on digital media, thereby aligning with spatial presence. As individuals’ perception of the authenticity of the media environment, presence holds significant implications for understanding their perception of media information and subsequent behavioral decision-making. Scholars have highlighted that within the context of tourism live-streamed shopping, factors such as information quality and source credibility can enhance individuals’ sense of presence, thereby stimulating their willingness to continue viewing ( Sang et al., 2023 ). Similarly, when individuals browse tourism information shared via social media, they also experience a sense of presence, with higher perceived presence correlating with a greater likelihood of impulse travel intentions ( Yao and Jia, 2021 ). However, whether individuals experience a sense of presence when perusing destination environmental information through digital media, and whether this resultant presence can further stimulate TPEBI, remain unresolved questions.
2.3 Tourists’ pro-environmental behavioral intention
Tourists’ pro-environmental behavior refers to actions undertaken by tourists within the tourism environment that contribute to promoting environmental sustainable development ( Lee et al., 2013 ). Previous studies have demonstrated that TPEBI plays a pivotal role in enhancing environmental quality and fostering the sustainable development of tourism destinations ( He et al., 2022 ). Consequently, understanding the driving mechanisms behind TPEBI has become a critical topic in sustainable tourism research. Within the tourism context, tourists often prioritize hedonic experiences and may overlook the environmental impact of their actions, making it challenging for them to voluntarily assume responsibility for environmental protection ( Liu et al., 2021 ). Additionally, the unconventional nature of tourism environments weakens the influence of moral norms on tourists, diminishing their sense of responsibility during travel ( Li and Chen, 2021 ). Consequently, instances of irresponsible behavior such as littering and scribbling are prevalent, resulting in direct or indirect adverse effects on tourist destinations ( Cheng and Chen, 2022 ). Given this backdrop, stimulating TPEBI has become a pressing issue. While existing research has predominantly explored the influencing mechanisms of TPEBI within the field tourism context, scant attention has been paid to factors associated with the digital virtual context. With the proliferation of mobile Internet and digital technology, the influence of digital media on individual attitudes and behaviors has become increasingly significant. Therefore, it is imperative to investigate the substantial impact of digital media on TPEBI.
2.4 Eco-guilt
Guilt, characterized by a negative emotional response triggered by an individual’s recognition that their behavior violates social or moral norms and leads to negative consequences for society or others, Tangney and Dearing (2002) often motivates prosocial actions ( Dahl et al., 2003 ; Lunardo and Saintives, 2018 ). According to cognitive dissonance theory, guilt arises from the discrepancy between one’s actions and moral standards, prompting individuals to engage in compensatory behaviors to alleviate the resulting psychological discomfort ( Festinger, 1957 ; Theotokis and Manganari, 2015 ). Given the significant role of guilt in stimulating prosocial behavior, scholars have extensively investigated its relationship with various formats of positive conduct. For example, Hassan and Rahman (2023) discovered that guilt can deter unethical consumption behaviors. Similarly, Wang and Wu (2016) revealed that emotions like guilt prompt individuals to undertake compensatory actions, fostering a willingness to engage in sustainable consumption, particularly for energy-saving products. In recent years, amidst escalating concerns over issues such as global warming and environmental degradation, researchers have begun to explore the integration of guilt into the realm of environmental behavior. Some studies indicate that eco-guilt, as a kind of negative emotion arising when individuals perceive their responsibility for environmental consequences, can spur environmental protection actions, with its impact often surpassing that of positive emotions ( Moore and Yang, 2020 ; Shipley and van Riper, 2022 ). While scholars have extensively discussed the relationship between eco-guilt and pro-environmental behaviors, there has been comparatively less focus on the driving mechanism of eco-guilt on TPEBI within the tourism context ( Bahja and Hancer, 2021 ). Given the escalating prominence of environmental issues in tourist destinations and the pivotal role of tourists in shaping the sustainable development of these locales, it becomes imperative to explore the influencing mechanism of eco-guilt on TPEBI.
2.5 Empathy with nature
Empathy refers to the ability to put oneself in the other person’s position to identify with and understand another person’s emotions, thoughts, and experiences, essentially putting oneself in their shoes ( Hogan, 1969 ). As ecological and environmental issues have become increasingly prominent, Tam (2013) expanded the scope of empathy to encompass the relationship between humans and nature, introducing the notion of empathy with nature. This concept suggests that empathy with nature entails an individual’s ability to empathize with the situation in nature. Individuals with a strong empathic connection to nature are better equipped to recognize and comprehend environmental challenges faced by nature, thereby fostering their sense of environmental responsibility ( Liu, 2023 ). Empathy with nature not only enables individuals to gain profound insights into various environmental issues but also strengthens the emotional bond between humans and nature, motivating individuals to pay attention to and address environmental problems ( Tam, 2013 ). Numerous studies have empirically demonstrated the relationship between empathy with nature and individual pro-environmental behaviors. For example, Wang L. T. et al. (2023) argue that individuals who empathize with nature are more inclined to take effective action to address environmental issues. Similarly, Ienna et al. (2022) found that individuals with a higher capacity to empathize with nature exhibit more positive environmental attitudes and are more willing to engage in pro-environmental behaviors. Therefore, our study contends that empathy with nature serves as a crucial perspective for investigating the driving factors of pro-environmental behavior, warranting empirical analysis of the relationship between empathy with nature and pro-environmental behavior.
3 Research hypotheses
3.1 digital media information sharing and presence.
Some scholars have noted that the formats of information presentation in digital media, including text, images, and videos, are closely linked to the sense of presence, with each form having a distinct impact on individuals’ presence ( Yao and Jia, 2021 ). Generally, media information that is more vivid and interactive tends to facilitate a stronger sense of presence ( Vonkeman et al., 2017 ). Yao and Jia (2021) highlighted that compared to text-based information, both image-based and video-based information are more likely to evoke a sense of presence in individuals. Similarly, Yu et al. (2017) found that presentation formats with enhanced visibility and interactivity can lead online consumers to experience a heightened sense of presence, thereby influencing their recommendation and purchase intentions. Verhagen et al. (2014) , focusing on the online shopping context, confirmed that different product presentation formats exert varying impacts on presence, noting that virtual mirrors induce a higher sense of presence compared to 360-spin rotation and static images. These findings suggest that information with enhanced visibility and vividness tends to augment an individual’s sense of presence. Therefore, based on the above discussions, our study posits the following hypothesis:
H1: Video-based information presented by digital media evokes a higher sense of presence compared to image-based information, and the sense of presence induced by image-based information is higher than that induced by text-based information.
3.2 Presence and tourists’ pro-environmental behavioral intention
Presence, defined as the extent to which individuals perceive reality within virtual environments, significantly influences individual attitudes and behavioral decisions ( Ou et al., 2014 ). In recent years, the academic community has increasingly focused on the relationship between presence and individual behavioral intention within the realms of virtual reality and digital technology. Sang et al. (2023) discovered that heightened presence experienced while viewing tourism live-streamed shopping content correlates with a greater likelihood of viewers continuing to watch. Similarly, Yao and Jia (2021) observed that stronger presence when browsing tourism information on social media corresponds to a greater impulse to travel. Scholars have also investigated the relationship between presence and individual behavioral intention in the online shopping context. For instance, Xu et al. (2021) found that the sense of presence induced by individuals watching online live-streamed shopping positively influences their purchase intention. Additionally, Dong et al. (2023) highlighted the significance of presence in live e-commerce, where it serves as a crucial incentive for consumers to experience flow and develop impulse consumption intentions. Building on this literature, our study posits that a higher sense of presence experienced by individuals when browsing destination environmental threat information through digital media facilitates a deeper understanding of the destination’s ecological environment, thereby stimulating TPEBI. Therefore, the following hypothesis is proposed:
H2: Presence positively influences TPEBI.
3.3 Mediating role of eco-guilt
According to the cognitive appraisal theory ( Lazarus, 1991 ), individuals engage in cognitive evaluation in response to external stimuli, leading to emotional responses and subsequent coping behaviors, thereby forming an “evaluation-emotion-behavioral response” path relationship. According to this theory, when individuals browse information concerning threats to the ecological environment of tourism destinations via digital media, they undergo cognitive evaluation of the immersive experience, subsequently experiencing feelings of guilt regarding the ecological environment. This guilt then activates their pro-environmental behavioral intentions, thus facilitating the “evaluation-emotion-behavioral response” pathway. Existing studies have also proved the mediating role of affective emotions between individual cognitive evaluation (perceived tourism impact) and behavioral response (support for tourism) on the basis of cognitive appraisal theory ( Munanura et al., 2023 ). Additionally, according to the stimulus-organism-response theory, individuals’ emotions are influenced by external stimuli, leading to corresponding behavioral responses ( Mehrabian and Russell, 1974 ). In this study, the sense of presence induced by individuals browsing destination environmental threat information through digital media serves as an external stimulus. This sense of presence further triggers individuals’ eco-guilt (organism), subsequently stimulating their pro-environmental behavioral intentions (behavioral response). Compared to individuals’ direct observation of environmental issues in the field, the immersive experience generated by viewing media information tends to evoke stronger feelings of guilt, worry, and anxiety regarding environmental issues, thus reducing the psychological distance between individuals and the ecological environment ( Wang et al., 2017 ), and consequently stimulating their pro-environmental behavioral intentions ( Lange and Dewitte, 2022 ). Based on the aforementioned discussion, our study proposes the following hypotheses:
H3: Presence positively affects eco-guilt.
H4: Eco-guilt positively affects TPEBI.
H5: Eco-guilt plays a mediating role between presence and TPEBI.
3.4 Mediating role of empathy with nature
The empathy-altruism hypothesis proposed by Batson (1987) provides a theoretical basis for revealing the intermediary transmission mechanism between presence and pro-environmental behavior. According to the theory, individuals encountering information regarding destination environmental threats through digital media are likely to experience emotional resonance with the environmental pollution and ecological damage faced by those destinations. This emotional resonance, in turn, activates their willingness to engage in behaviors aimed at solving environmental problems. Moreover, following the cognitive-affective-conative theoretical framework ( Mischel and Shoda, 1995 ), the sense of presence (cognitive factor) generated when individuals browse destination environmental threat information through digital media can promote their empathy with nature (emotional factor), and consequently stimulate their pro-environmental behavioral intentions. Therefore, the cognitive-affective-conative framework provides a theoretical basis for elucidating the mediating mechanism between presence and TPEBI. Empirical analyses in existing studies have corroborated the mediating role of empathy between presence and individual prosocial behaviors ( Kandaurova and Lee, 2019 ; Lee and Li, 2023 ). Therefore, our study posits that the presence experienced by individuals when browsing destination environmental threat information through digital media can elicit empathy toward nature. This empathy with nature, in turn, further stimulates their willingness to engage in pro-environmental behavior. Based on these premises, the study proposes the following hypotheses:
H6: Presence positively affects empathy with nature.
H7: Empathy with nature positively affects TPEBI.
H8: Empathy with nature plays a mediating role between presence and TPEBI.
In summary, drawing from a review of literature and practical research, our study constructs a theoretical model (as shown in Figure 1 ) to empirically investigate the influencing mechanism of destination environmental threat information on digital media on TPEBI.
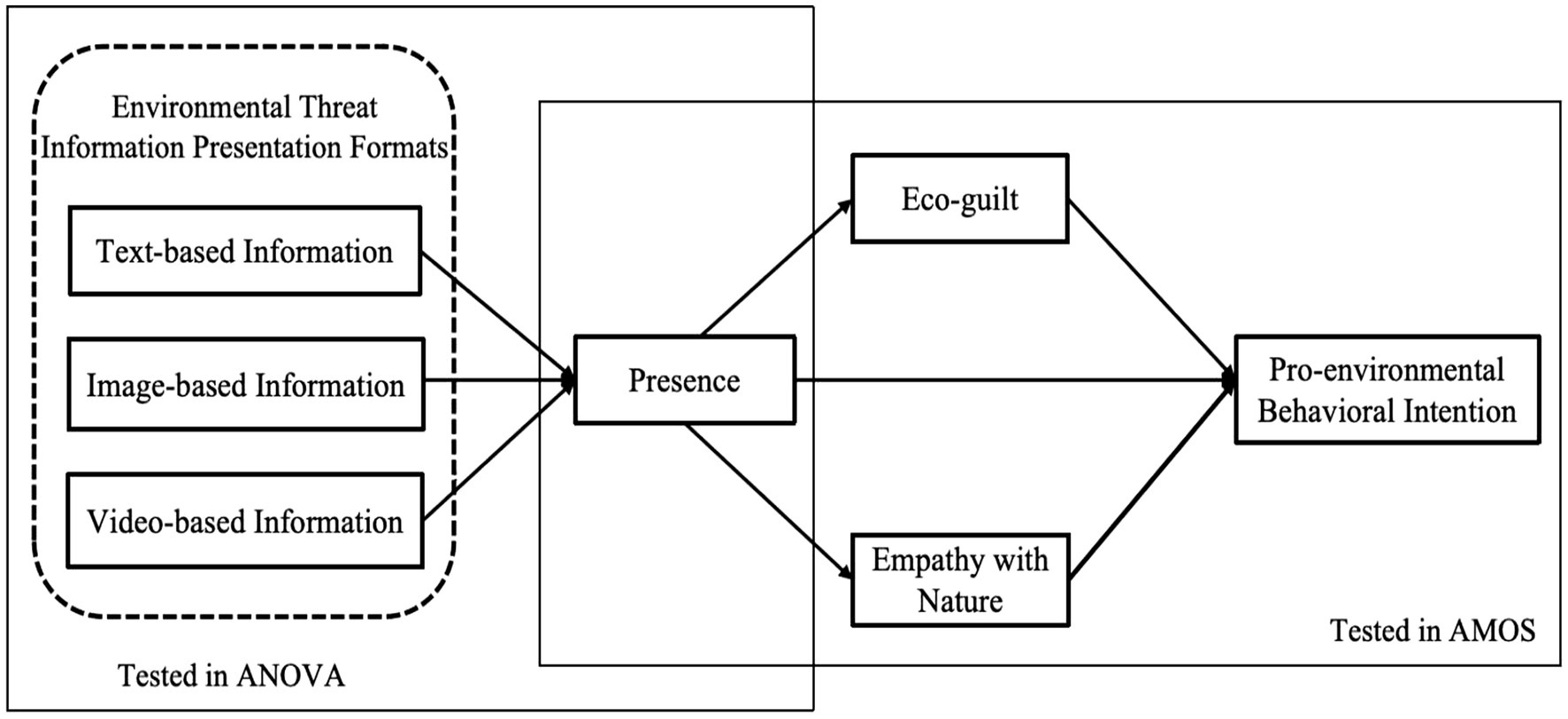
Figure 1 . Theoretical model.
In Study 1, a scenario experiment was conducted to examine the impact of various formats of environmental threat information presented via digital media on individuals’ sense of presence. Our study selects Chinese digital media users as the research object. There are a large number of digital media users in China, and their behaviors and attitudes are deeply influenced by digital media. In this context, it is more necessary and urgent to explore how to enhance Chinese users’ pro-environmental behavior through digital media. Participants were recruited through Credamo, a prominent Chinese online research platform, and were offered incentives as a token of appreciation for their participation. To reflect the primary methods through which digital media presents destination environmental information, the study selected three presentation formats-text, images, and videos-depicting environmental threats on Weibo, a widely influential digital media platform in China. To eliminate the bias stemming from participants’ preconceived notions about the destination, the experiment’s design concealed the destination’s name. The manipulation materials for the independent variables consisted of three groups: Text-based information group: Participants were exposed to written descriptions of the destination’s environmental threats. Image-based information group: Participants viewed visual representations illustrating the ecological threats faced by the destination. Video-based information group: Participants watched videos depicting the environmental threats present at the destination.
4.2 Pre-test and measures
To assess the effectiveness of the independent variable manipulation and the validity of measurement items, a pre-test was conducted. The pre-test was mainly carried out in the classroom. Three classes were selected and the experimental materials were randomly allocated among the classes. In this pre-test, 72 participants were randomly assigned to one of three experimental scenarios. They were then instructed to read the corresponding stimulus material, complete measures of scenario authenticity and presence, and provide demographic information. The measurement item for scenario authenticity was adapted from Dabholkar (1994) , with the specific item being “in real life, the above scenario may happen.” The measurement items of presence were adapted from Xu et al. (2021) and Yin et al. (2023) , comprising four items. These items assessed the participants’ feelings when browsing destination environmental threat information through digital media, including statements like “I have a feeling of being there,” “I feel that the tourism destination is in front of me,” “I feel that I am in the real world created by digital media,” and “I can really perceive the existence of environmental threats in the destination.” A total of 68 valid questionnaires were collected from the pre-experiment (21 in G text , 23 in G image and 24 in G video ). Among the participants, 41.1% were male and 58.9% were female. And they were mainly between 18 and 24. One-way analysis of variance (ANOVA) was performed, revealing that the degree of presence reported by participants exposed to video-based information was significantly higher than that reported by those exposed to image-based and text-based information (M text = 2.83, SD = 0.48; M image = 3.37, SD = 0.37; M video = 4.21, SD = 0.47; p < 0.001). This result indicates successful manipulation of the independent variable. Furthermore, reliability analysis demonstrated that Cronbach’s α value for the presence measurement items was 0.887, exceeding the threshold of 0.7, indicating good reliability. Thus, these measures were deemed suitable for use in the formal experiments.
4.3 Procedure
Through the Chinese online research platform Credamo, 180 participants were recruited to partake in the formal experiment, with the participants randomly assigned to three groups (60 in G text , 60 in G image and 60 in G video ). Prior to the commencement of the experiment, the research team thoroughly explained the experiment requirements and relevant instructions to the participants, emphasizing the anonymity of the process. Participants were then asked to complete a questionnaire based on their genuine reactions to browsing the experimental material. The experimental stimulus materials, the authenticity test of the scenario, and the measurement methods for the sense of presence were consistent with those utilized in the pre-test. To ensure the experiment’s alignment with the context of digital media usage, questionnaires were administered online. Additionally, a question pertaining to the ecological environment information of the destination featured in the experimental materials was incorporated into the questionnaire. Only questionnaires with complete browsing of the experimental materials and accurately filled responses were considered valid. Following the removal of incomplete questionnaires, 161 valid questionnaires were obtained (51 in G text , 56 in G image , and 54 in G video ). Among the participants, 45.8% were male and 54.2% were female; 73.1% were aged 35 or below, 18.7% were between 36 and 45, and 8.2% were over 45. Furthermore, the majority of participants held a college degree or higher (94.3%).
4.4 Results
The results of the scenario authenticity test indicated that more than 95 percent (98.4 percent) of participants, perceived the scenarios as realistic. Additionally, the reliability analysis revealed a Cronbach’s α coefficient of 0.848, greater than 0.7, for the measurement items, indicating good reliability. In this study, one-way ANOVA analysis was conducted to assess the impact of the three types of information presentation on the sense of presence. The ANOVA analysis treated presence as the dependent variable and the presentation form of digital media information as the independent variable. The results unveiled significant differences in the influence of the three formats of information presentation on the sense of presence [ F (2, 158) = 534.03, p < 0.001]. As depicted in Figure 2 , the presence reported by participants in the video group was significantly higher than that in the image group (M image = 3.62, SD = 0.25; M video = 4.38, SD = 0.26; p < 0.001) and text group (M text = 2.55, SD = 0.34; M video = 4.38, SD = 0.26; p < 0.001). Moreover, the presence in the image group was significantly higher than that in the text group (M text = 2.55, SD = 0.34; M image = 3.62, SD = 0.25; p < 0.001). These findings indicate that compared to text and image presentations, conveying environmental threats information through short videos can significantly enhance individuals’ sense of immersion. Thus, Hypothesis H1 is supported.
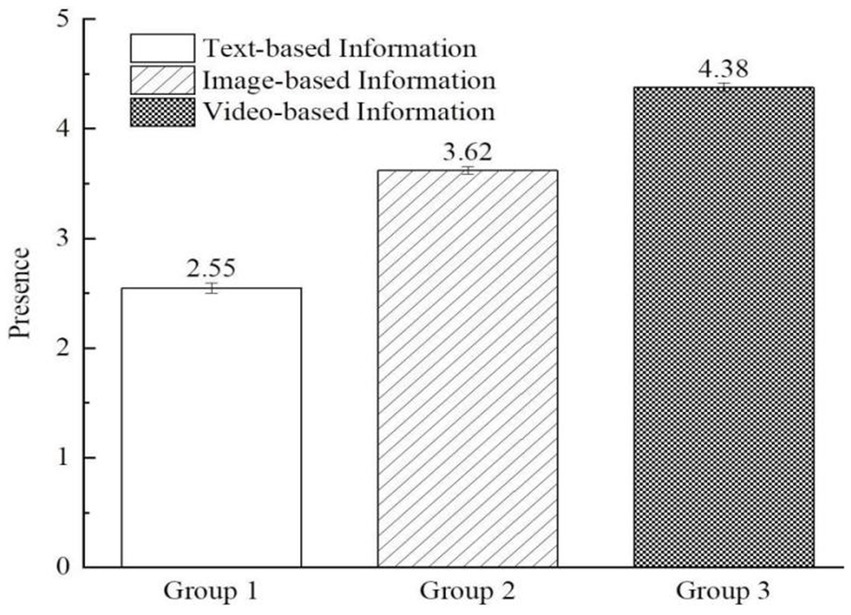
Figure 2 . The impact of digital media information presentation formats on presence (study 1).
5.1 Design and measures
Study 2, aims to investigate the mechanism through which presence influences TPEBI. The experimental materials and design are the same as those in Study 1. A total of 366 participants were recruited through the Credamo online research platform and randomly assigned to one of three experimental groups (G text , G image , and G video ). Participants were then asked to browse the experimental materials and complete various measurement items, including scenario authenticity, presence, eco-guilt, empathy with nature, and pro-environmental behavioral intention, and demographic information. The measurement items for scenario authenticity and presence were consistent with those used in Study 1. Additionally, the measurement items for eco-guilt, empathy with nature, and pro-environmental behavioral intention were adapted from existing scales. To ensure the applicability of the English scale in the Chinese context, our study adopted the back-translation method to carry out translational work. For eco-guilt, three measurement items were utilized, adapted from Bahja and Hancer (2021) and Onwezen et al. (2013) . Specific items are: “I will feel guilty,” “I will feel ashamed,” and “I will feel uneasy if I do not pay attention to ecological protection when traveling.” Empathy with nature was measured using three items adapted from scales designed by Li et al. (2019) and Liu (2023) . These items are: “I can perceive the pain suffered by the animals and plants in the destination,” “I can imagine the difficult situation of the animals and plants in the destination.” and “I care and sympathize with the animals and plants in the destination.” Pro-environmental behavioral intention was assessed using three items, mainly referring to Cheng and Chen (2022) and Zhao et al. (2020) . Specific items are: “I will abide by relevant policies and not destroy the ecological environment of the destination,” “I will prevent the behavior of damaging the ecological environment of the destination,” and “I will participate in activities to protect the ecological environment of the destination.” After removing invalid questionnaires (with confusing or non-serious answers), 329 valid questionnaires were obtained, with sample sizes of 106, 109, and 114 in the text, image, and video groups, respectively. The demographic distribution included 43.2% male and 56.8% female participants, with 67.3% aged 35 or below, 23.6% between 36 and 45, and 9.1% over 45. The majority of participants had a college degree or higher (91.8%). On observation of the demographic characteristics it was found that it mostly comprised young participants, who are more familiar with digital media platforms and have strong acceptance of new technologies.Therefore, the characteristics of the sample in our study are in line with the reality that the majority of digital media users in China are young people, indicating that the survey sample is representative.
5.2 Reliability and validity analysis
The reliability of the measurement tools was assessed using Cronbach’s α coefficient. The results indicated that the Cronbach’s α values for presence, eco-guilt, empathy with nature, and pro-environmental behavioral intention were 0.919, 0.892, 0.890, and 0.904, respectively. All of these values exceeded the threshold of 0.7, demonstrating good reliability for each scale.
The validity of the measurement scale was assessed by examining both convergent validity and discriminant validity through confirmatory factor analysis using AMOS 28.0 software. Table 1 presents the results. The standardized factor loadings for all measured items ranged from 0.806 to 0.894, with composite reliability (CR) values ranging from 0.891 to 0.920, all exceeding the threshold of 0.7. Additionally, the average variance extracted (AVE) values ranged from 0.732 to 0.760, all surpassing the recommended threshold of 0.5. These findings indicate strong convergent validity for all scales.
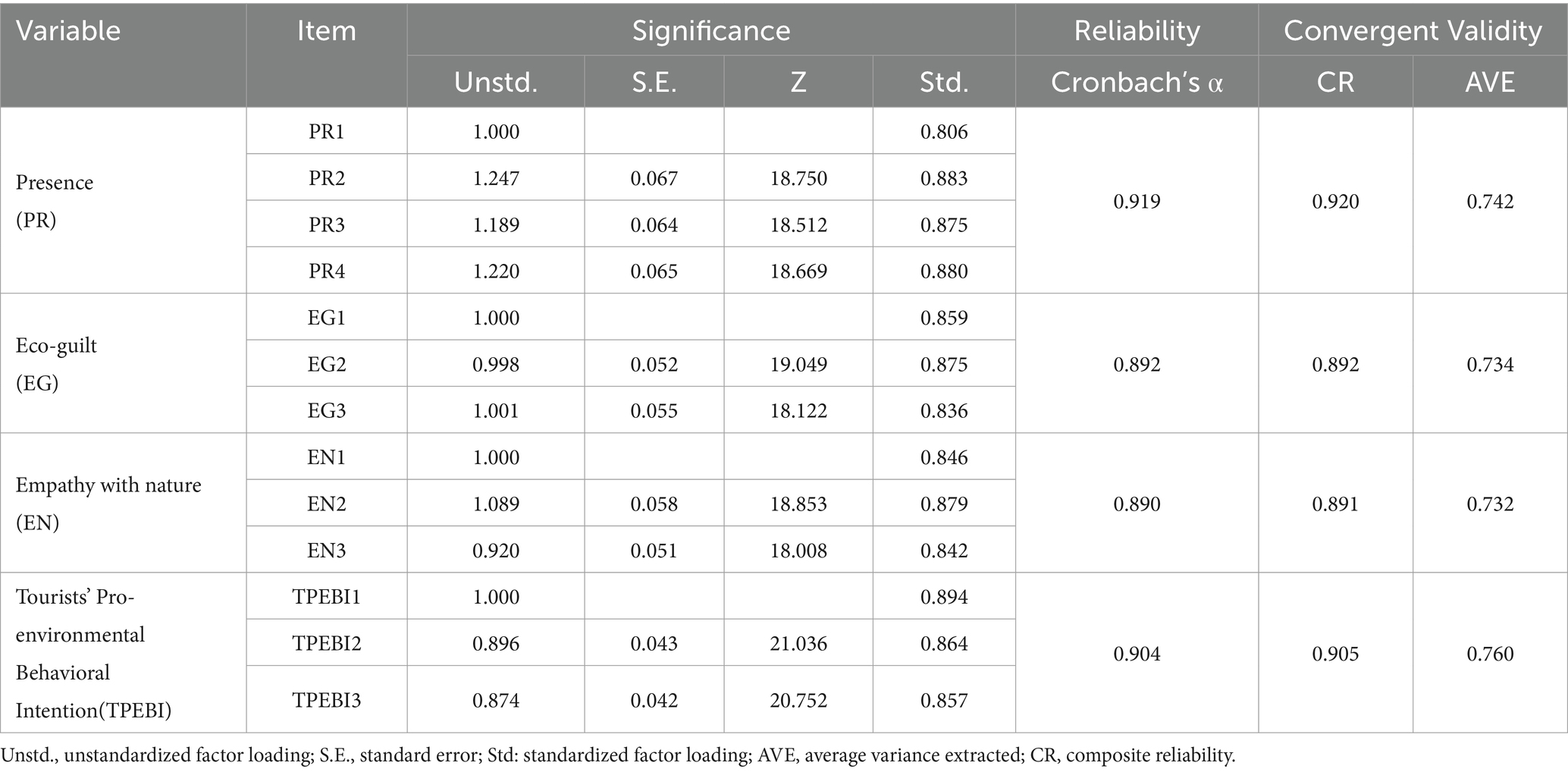
Table 1 . Reliability and convergent validity test.
The discriminant validity of the measurement scale was assessed by comparing the square root value of AVE of each variable with the correlation coefficient between that variable and other variables. The results are presented in Table 2 . It was found that the square root value of AVE for each variable exceeded the correlation coefficient between variables, demonstrating satisfactory discriminant validity for all scales.
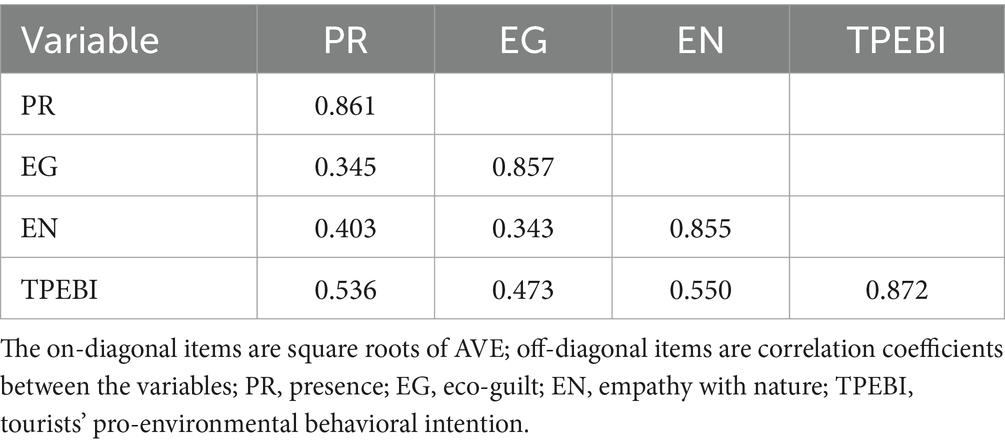
Table 2 . Discriminant validity test.
5.3 Hypotheses testing
5.3.1 relationship between the destination environmental threat information on digital media and the sense of presence.
To further corroborate the findings of Study 1, we conducted a one-way ANOVA analysis to examine the difference in the impact of different presentation formats of destination environmental threat information on digital media on individual sense of presence. The results revealed significant differences in the influence of various information presentation formats on individual presence [ F (2, 326) = 592.325, p < 0.001]. As depicted in Figure 3 , the sense of presence in the video group significantly surpassed that in the image and text groups (M text = 2.29, SD = 0.45; M image = 3.27, SD = 0.36; M video = 4.22, SD = 0.43; p < 0.001), thereby further affirming Hypothesis H1. Furthermore, our study further examined the differences in the effects of distinct information presentation formats on eco-guilt, empathy with nature, and pro-environmental behavioral intention. The results demonstrated significant differences in the levels of eco-guilt [ F (2, 326) = 20.575, p < 0.001], empathy with nature [ F (2, 326) = 26.637, p < 0.001], and pro-environmental behavioral intention [ F (2, 326) = 51.606, p < 0.001] induced by varied information presentation formats, with video-based information eliciting higher responses compared to image-based and text-based information.
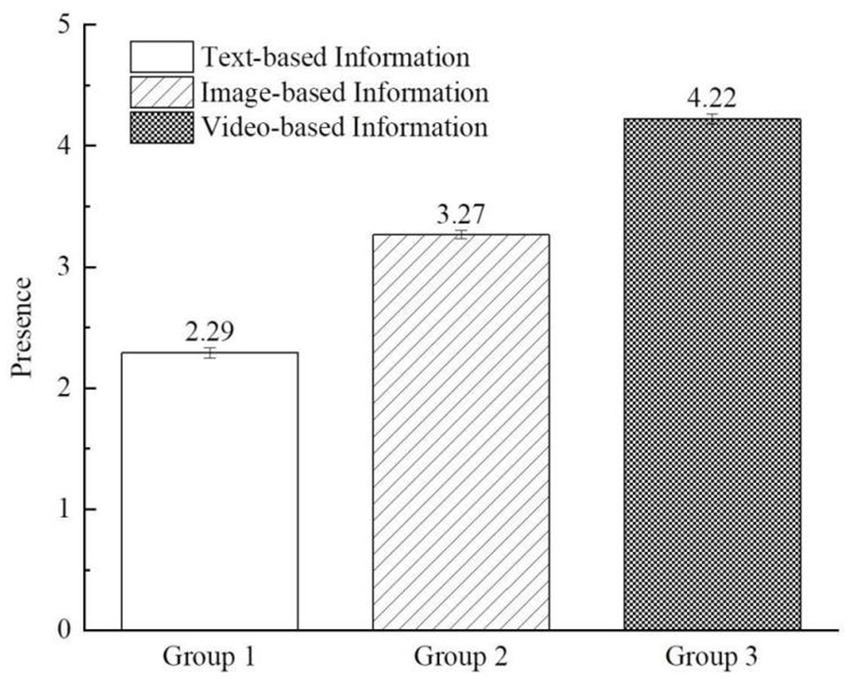
Figure 3 . The impact of digital media information presentation formats on presence (study 2).
5.3.2 Relationship between presence and tourists’ pro-environmental behavioral intention
Before conducting the main effect test, we used AMOS 28.0 software to analyze the fit index of the structural model. The results indicated a good fit degree (X 2 /DF = 0.990, GFI = 0.974, CFI = 0.959, NFI = 0.981, RMSEA = 0.011), suggesting that the structural model was suitable for further analysis. The results of main effect test based on the structural equation model showed that the sense of presence exhibited a positive promoting effect on TPEBI [ β = 0.411, BC 95% CI = (0.284, 0.552), p < 0.001], supporting Hypothesis H2. Additionally, presence demonstrated significant positive effects on both eco-guilt [ β = 0.488, BC 95% CI = (0.338, 0.641), p < 0.01] and empathy with nature [ β = 0.525, BC 95% CI = (0.393, 0.665), p < 0.001]. Both eco-guilt [ β = 0.276, BC 95% CI = (0.174, 0.376), p < 0.001] and empathy with nature [ β = 0.412, BC 95% CI = (0.299, 0.527), p < 0.01] were found to further stimulate TPEBI. These findings suggest that eco-guilt and empathy with nature may mediate the relationship between presence and TPEBI. Therefore, Hypotheses H3, H4, H5, H6, H7, and H8 are preliminarily supported.
5.3.3 Mediating effect test
The bootstrapping method was employed to further verify the mediating effect of eco-guilt and empathy with nature between presence and TPEBI, with 5,000 iterations and a confidence level set to 95%. The results indicate that presence indirectly influences TPEBI through eco-guilt [ β = 0.135, BC 95% CI = (0.078, 0.211), p < 0.01] and empathy with nature [ β = 0.216, BC 95% CI = (0.148, 0.309), p < 0.001] (refer to Table 3 ). This finding suggests that eco-guilt and empathy with nature indeed play a mediating role between presence and TPEBI. Consequently, Hypotheses H3, H4, H5, H6, H7, and H8 are further corroborated. Considering the direct positive promoting effect of presence on TPEBI [ β = 0.411, BC 95% CI = (0.284, 0.552), p < 0.001], it can be inferred that eco-guilt and empathy with nature partially mediate the relationship between presence and TPEBI.
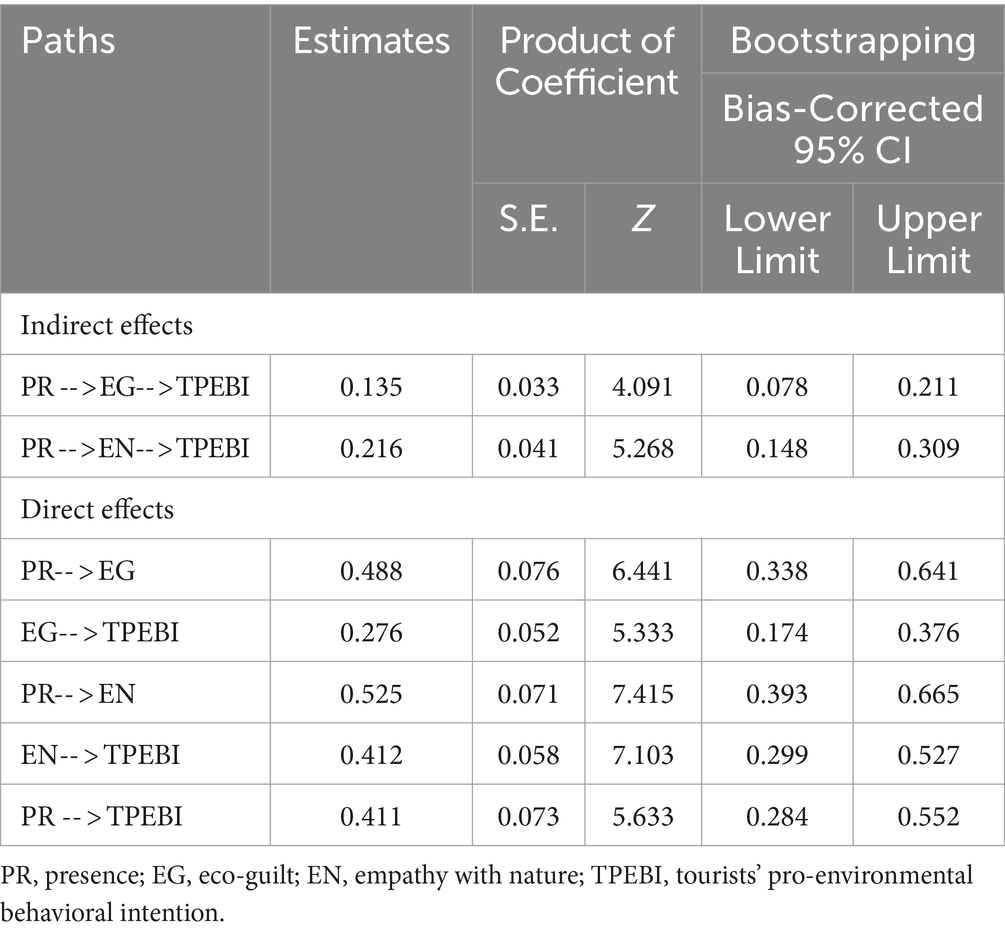
Table 3 . Indirect and direct effects analysis.
6 Discussion and conclusion
Based on the perspective of the sense of presence, our study, through a combination of scenario experiments and questionnaire surveys, has examined the difference in the impact of various formats of destination environmental threat information presented by digital media on individual presence. Additionally, it has elucidated the influencing pathway of presence on TPEBI. The specific conclusions drawn are as follows:
First, different formats of destination environmental threat information presented by digital media exhibit significant differences in their impact on individuals’ sense of presence. Video-based information elicits the highest level of presence, followed by image-based information, while text-based information has the lowest impact. This finding underscores the notion that the more immersive the presentation form of environmental threat information in digital media, the more likely it is to engender a sense of immersion in individuals. This result further corroborates the research findings of Yao and Jia (2021) regarding the relationship between information sharing and presence in digital media. Second, presence emerges as a pivotal inducement to trigger TPEBI, highlighting its crucial role in driving individuals to engage in pro-environmental behavior. Specifically, when individuals peruse information concerning threats to the destination’s ecological environment on digital media, the sense of presence serves to diminish the psychological distance between them and the destination’s ecological environment. Consequently, they become more inclined to care about and address environmental issues, ultimately fostering a willingness to protect the ecological environment of destinations. Third, presence not only directly fosters TPEBI but also indirectly influences it through eco-guilt and empathy with nature. Specifically, information regarding threats to the destination’s ecological environment, presented with vividness and visibility on digital media, can evoke individuals’ immersive experiences, subsequently stimulating their emotional responses of eco-guilt and empathy with nature. This, in turn, enables individuals to empathize with the vulnerability of the destination’s ecological environment, propelling them toward pro-environmental behavior. It is evident that eco-guilt and empathy with nature serve as significant emotional driving mechanisms effectively fostering individuals’ engagement in pro-environmental behavior.
6.1 Theoretical implications
First, amidst the escalating environmental challenges in tourist destinations, the exploration of the driving forces behind TPEBI has garnered considerable attention. Existing studies predominantly delve into the formation mechanisms of TPEBI within the context of on-site tourism experiences, encompassing factors such as destination image, tourism encounters, and environmental commitment, among others ( He et al., 2018 ; Tang et al., 2022 ; Lee and Jan, 2023 ). However, scant attention has been paid to the influence of virtual environments, such as digital media, on TPEBI ( Han et al., 2023 ). Building upon existing research, our study empirically scrutinized the presentation formats of destination environmental threat information on digital media and the ensuing influence of presence on TPEBI. This conclusion broadened the scope of inquiry established by Shang et al. (2021) regarding the nexus between information dissemination on digital platforms and individual behavioral intentions, thereby further enriching the literature on the determinants of TPEBI ( Tu and Ma, 2022 ; Li et al., 2023a ).
Second, existing studies examining the impact of presence on individual behavioral decision-making primarily concentrate on contexts such as live-streamed shopping and online education ( Xu et al., 2021 ; Guo et al., 2023 ), with limited discussion on the influencing mechanism of presence on individual pro-environmental behavioral intention from an ecological perspective. Our study extends the observed effects of presence to the realm of individual pro-environmental behavioral intention, offering a novel lens for comprehending the interplay between digital media information dissemination and individual behavioral decision-making. In doing so, it broadens the scope of the influence of presence, thus contributing to a deeper understanding of the relationship between digital media and human behavior ( Amin et al., 2021 ; Shu et al., 2023 ; Li et al., 2023b ).
Finally, existing research on the correlation mechanism between presence and individual behavioral decision-making is relatively scarce. Our study attempts to explore a novel mediating mechanism in the formation of TPEBI by investigating the mediating effect of eco-guilt and empathy with nature between presence and TPEBI. This enriches the research findings of Shu et al. (2023) that presence can indirectly influence individuals’ behavioral decisions by shaping their emotional experiences. By providing a new analytical framework for understanding the influencing path between presence and behavioral intention, our study contributes to the broader theoretical discussion on the correlation mechanism between digital media use and individual behavioral decision-making ( Rajarathinam et al., 2022 ; Choi and Noh, 2023 ).
6.2 Practical implications
First, the accurate and transparent dissemination of information regarding destination environmental threats through digital media serves as a pivotal means to foster TPEBI. As environmental challenges in tourism destinations escalate, stakeholders such as governments and tourism authorities must leverage platforms like WeChat, Weibo, and TikTok to conduct comprehensive environmental education initiatives. By enhancing public awareness of environmental issues and the detrimental effects of irresponsible behavior on destination ecosystems, individuals are more likely to engage in pro-environmental actions. Additionally, it is imperative for governments, tourism authorities, and media outlets to effectively communicate and supervise the dissemination of information regarding threats to destination environments through digital channels, thus highlighting the consequences of environmental degradation. Furthermore, empowering individuals with the necessary skills to participate in environmental conservation efforts can bolster their willingness to address and mitigate environmental challenges in destinations, fostering a culture of environmentally responsible behavior.
Additionally, our study underscores the significant impact of different presentation formats of destination environmental threat information on individuals’ sense of presence and pro-environmental behavioral intentions. Notably, the more immersive and visually compelling the digital media presentations are, the greater the individual’s sense of presence and inclination toward pro-environmental action. These findings offer valuable theoretical insights for governmental bodies, tourism authorities, and other stakeholders seeking to leverage digital media for environmental advocacy. When disseminating information about the environmental challenges facing tourism destinations through digital platforms, it is essential for relevant entities to enhance management and intervention in information presentation formats to ensure the efficacy of environmental messaging and educational efforts. Additionally, the public should be encouraged to share high-quality digital media content aimed at promoting environmental protection in destinations, thereby bolstering their awareness of environmental issues and sense of responsibility. Specifically, information regarding threats to the ecological integrity of tourism destinations should be conveyed through digital media using engaging formats such as videos and images, with the integration of cutting-edge technologies like 3D, virtual reality (VR), and augmented reality (AR) to enhance visual immersion. Moreover, efforts should be made to guide the public in sharing content that highlights the environmental damage incurred by destinations, utilizing short videos or images, while implementing effective management and monitoring mechanisms to filter out any harmful or misleading content. Furthermore, digital technologies should be harnessed to authentically depict the environmental challenges faced by destinations, vividly illustrating the adverse consequences of environmental degradation in a visually compelling manner.
Furthermore, our study reveals that the sense of presence indirectly influences TPEBI through eco-guilt, highlighting the importance of leveraging emotional factor of eco-guilt and stimulate responsible pro-environmental behavior among tourists. On the one hand, with the rapid development of digital technologies such as VR, AR and artificial intelligence, government and tourism destinations should employ real-time monitoring systems to track threats and ecological damage to tourism destinations. By leveraging digital platforms, scenes depicting environmental degradation and its severe consequences can be effectively conveyed to tourists, evoking a sense of guilt about the state of the destination’s environment and subsequently motivating them toward pro-environment behavioral intentions. On the other hand, relevant agencies can also stimulate tourists’ eco-guilt through various communication channels, including public lectures, educational campaigns, and on-site exhibitions. For instance, issues pertaining to ecological damage and environmental pollution, along with their detrimental effects, can be disseminated via popular digital media platforms such as WeChat, Weibo, and TikTok.
Finally, our study found that empathy with nature plays a mediating role between presence and TPEBI, highlighting the importance of enhancing tourists’ empathy with nature to foster pro-environmental behavior. To this end, various strategies can be implemented to deepen tourists’ connection with the natural environment. For example, relevant authorities should leverage digital media platforms to share the current state of the ecological environment in destinations, allowing the public to gain a deeper understanding of the fragility of the ecological environment. Additionally, engaging and informative activities focused on human-nature relationships can be popularized, utilizing creative short videos to underscore the importance of harmonious coexistence between humans and nature. Moreover, anthropomorphic representations of the tourist landscape can be designed to evoke empathy among tourists. Utilizing VR, AR, and other digital technologies, immersive experiences can be created to vividly depict the plight of animals and plants in tourism destinations, thereby enhancing tourists’ empathy with nature.
6.3 Limitations and future research directions
First, it’s important to acknowledge that our study primarily relies on self-report. Considering that pro-environmental behavioral intention is a positive pro-social behavioral intention, the participants may have a tendency to meet social expectations when filling out questionnaires, thus affecting the authenticity of research conclusions to a certain extent. Future studies could incorporate a wider range of data collection methods to enhance the robustness of the measurement tools and improve the overall scientific rigor of the research. Second, our study focuses solely on examining the determinants of TPEBI, without delving into the factors influencing the translation of pro-environmental behavioral intention into actual pro-environmental behavior. Future studies can further explore the mechanism between TPEBI and their pro-environmental behavior, so as to further expand the research findings. Finally, our study only discusses the mediating role of eco-guilt and empathy-with nature between presence and TPEBI. Future studies can explore other mediating mechanisms and boundary conditions between presence and TPEBI.
Data availability statement
The raw data supporting the conclusions of this article will be made available by the authors, without undue reservation.
Ethics statement
Ethical review and approval was not required for the study on human participants in accordance with the local legislation and institutional requirements. Written informed consent from the [patients/ participants OR patients/participants legal guardian/next of kin] was not required to participate in this study in accordance with the national legislation and the institutional requirements.
Author contributions
XC: Conceptualization, Data curation, Formal analysis, Methodology, Software, Supervision, Visualization, Writing – original draft, Funding acquisition. Z-fC: Conceptualization, Data curation, Funding acquisition, Investigation, Project administration, Resources, Supervision, Writing – review & editing. H-jY: Data curation, Investigation, Methodology, Project administration, Resources, Supervision, Visualization, Writing – review & editing.
The author(s) declare that financial support was received for the research, authorship, and/or publication of this article. This work was supported by the following agency: Humanities and Social Science Foundation of Ministry of Education of China (grant number: 22YJC860003).
Conflict of interest
The authors declare that the research was conducted in the absence of any commercial or financial relationships that could be construed as a potential conflict of interest.
Publisher’s note
All claims expressed in this article are solely those of the authors and do not necessarily represent those of their affiliated organizations, or those of the publisher, the editors and the reviewers. Any product that may be evaluated in this article, or claim that may be made by its manufacturer, is not guaranteed or endorsed by the publisher.
Amin, M., Ryu, K., Cobanoglu, C., and Nizam, A. (2021). Determinants of online hotel booking intentions: website quality, social presence, affective commitment, and e-trust. J. Hosp. Market. Manag. 30, 845–870. doi: 10.1080/19368623.2021.1899095
Crossref Full Text | Google Scholar
Bahja, F., and Hancer, M. (2021). Eco-guilt in tourism: do tourists intend to behave environmentally friendly and still revisit. J. Destin. Mark. Manage. 20:100602. doi: 10.1016/j.jdmm.2021.100602
Batson, C. D. (1987). Prosocial motivation: is it ever truly altruistic. Adv. Exp. Soc. Psychol. 20, 65–122. doi: 10.1016/S0065-2601(08)60412-8
Chen, M. J. (2023). Antecedents and outcomes of virtual presence in online shopping: a perspective of SOR (stimulus-organism-response) paradigm. Electron. Mark. 33:58. doi: 10.1007/s12525-023-00674-z
Cheng, Z., and Chen, X. (2022). The effect of tourism experience on tourists’ environmentally responsible behavior at cultural heritage sites: the mediating role ofcultural attachment. Sustain. For. 14:565. doi: 10.3390/su14010565
Choi, D. H., and Noh, G. Y. (2023). The impact of social media on preventive behavior during the COVID-19 outbreak in South Korea: the roles of social norms and self-efficacy. SAGE Open 13:21582440231184969. doi: 10.1177/21582440231184969
PubMed Abstract | Crossref Full Text | Google Scholar
Dabholkar, P. A. (1994). Incorporating choice into an attitudinal framework: analyzing models of mental comparison processes. J. Consum.Res. 21, 100–118. doi: 10.1086/209385
Dahl, D. W., Honea, H., and Manchanda, R. V. (2003). The nature of self-reported guilt in consumption contexts. Market. Lett. 14, 159–171. doi: 10.1023/A:1027492516677
Dong, W., Wang, Y., and Qin, J. (2023). An empirical study on impulse consumption intention of livestreaming e-commerce: the mediating effect of flow experience and the moderating effect of time pressure. Front. Psychol. 13:1019024. doi: 10.3389/fpsyg.2022.1019024
Festinger, L. (1957). A theory of cognitive dissonance . Stanford, CA: Stanford University Press.
Google Scholar
Guo, P. Y., Saab, N., Ren, D. L., and Admiraal, W. (2023). The community of inquiry perspective on teachers? Role and students? Evaluations of online project-based learning. Online. Learn. 26, 259–280. doi: 10.24059/olj.v26i4.3193
Han, W., Wang, Y., Zhang, S., and Jiang, Y. Y. (2023). Internalizing social norms to promote pro-environmental behavior: Chinese tourists on social media. J. China Tour. Res. 19, 443–466. doi: 10.1080/19388160.2022.2085835
Hassan, S. M., and Rahman, Z. (2023). Curbing unethical consumer behaviour: the role of religiosity, consumer ethical beliefs and anticipated guilt. Int. J. Ethics. Syst . doi: 10.1108/IJOES-06-2022-0127
He, X., Cheng, J., Swanson, S. R., Su, L., and Hu, D. (2022). The effect of destination employee service quality on tourist environmentally responsible behavior: a moderated mediation model incorporating environmental commitment, destination social responsibility and motive attributions. Tour. Manag. 90:104470. doi: 10.1016/j.tourman.2021.104470
He, X., Hu, D., Swanson, S. R., Su, L., and Chen, X. (2018). Destination perceptions, relationship quality, and tourist environmentally responsible behavior. Tour. Manag. Perspect. 28, 93–104. doi: 10.1016/j.tmp.2018.08.001
Hogan, R. (1969). Development of an empathy scale. J. Consult. Clin. Psych. 33, 307–316. doi: 10.1037/h0027580
Hussain, T., Wang, D., and Li, B. (2024). Exploring the impact of social media on tourist behavior in rural mountain tourism during the COVID-19 pandemic: the role of perceived risk and community participation. Acta Psychol. 242:104113. doi: 10.1016/j.actpsy.2023.104113
Ienna, M., Rofe, A., Gendi, M., Douglas, H. E., Kelly, M., Hayward, M. W., et al. (2022). The relative role of knowledge and empathy inpredicting pro-environmental attitudes and behavior. Sustain. For. 14:4622. doi: 10.3390/su14084622
Jamshidi, D., Rousta, A., and Shafei, R. (2023). Social media destination information features and destination loyalty: does perceived coolness and memorable tourism experiences matter. Curr. Issues. Tour. 26, 407–428. doi: 10.1080/13683500.2021.2019204
Kandaurova, M., and Lee, S. H. (2019). The effects of virtual reality (vr) on charitable giving: the role of empathy, guilt, responsibility, and social exclusion. J. Bus. Res. 100, 571–580. doi: 10.1016/j.jbusres.2018.10.027
Lange, F., and Dewitte, S. (2022). The work for environmental protection task: a consequential web-based procedure for studying pro-environmental behavior. Behav. Res. Methods 54, 133–145. doi: 10.3758/s13428-021-01617-2
Lazarus, R. S. (1991). Emotion and adaptation . New York: Oxford Press.
Lee, T. H., and Jan, F. H. (2023). How do smart tourism experiences affect visitors’ environmentally responsible behavior? Influence analysis of nature-based tourists in Taiwan. J. Hosp. Tour. Manag. 55, 1–10. doi: 10.1016/j.jhtm.2023.02.016
Lee, T. H., Jan, F. H., and Yang, C. C. (2013). Conceptualizing and measuring environmentally responsible behaviors from the perspective of community-based tourists. Tour. Manag. 36, 454–468. doi: 10.1016/j.tourman.2012.09.012
Lee, H. M., and Li, B. J. (2023). So far yet so near: exploring the effects of immersion, presence, and psychological distance on empathy and prosocial behavior. Int. J. Hum. Comput. St. 76:103042. doi: 10.1016/j.ijhcs.2023.103042
Lee, S., Park, H., Kim, K. H., and Lee, C. K. (2021). A moderator of destination social responsibility for tourists’ pro-environmental behaviors in the VIP model. J. Destin. Mark. Manage. 20:100610. doi: 10.1016/j.jdmm.2021.100610
Li, T., and Chen, Y. (2021). The obstacles to building a self-management system: exploring deviant tourists’ anger reactions to the intervention of others. J. Hosp. Tour. Manag. 47, 303–311. doi: 10.1016/j.jhtm.2021.04.004
Li, T., Liao, C. M., Law, R., and Zhang, M. (2023b). An integrated model of destination attractiveness and tourists’ environmentally responsible behavior: the mediating effect of place attachment. Behav. Sci. 13:264. doi: 10.3390/bs13030264
Li, W., Yin, C., Tang, W., Li, X., Yang, D., and Zhang, Y. (2019). Place attachment and pro-environmental behaviors of bird-watching tourists:taking natural empathy and perception of environmental education as mediating variables. Economic. Geo. 39, 215–224. doi: 10.15957/j.cnki.jjdl.2019.01.026
Li, Q., Zhao, C., and Cheng, R. (2023a). How the characteristics of live-streaming environment affect consumer purchase intention: the mediating role of presence and perceived trust. Ieee. Access. 11, 123977–123988. doi: 10.1109/ACCESS.2023.3330324
Lin, Y. H., and Lee, T. H. (2020). How do recreation experiences affect visitors’ environmentally responsible behavior? Evidence from recreationists visiting ancient trails in Taiwan. J. Sustain. Tour. 28, 705–726. doi: 10.1080/09669582.2019.1701679
Liu, J. X. (2023). The influence of narrative transportation on university students’ environmental intentions: a serial mediation of empathy with nature and environmental attitudes. J. Clean. Prod. 431:139763. doi: 10.1016/j.jclepro.2023.139763
Liu, J., Wang, C. H., and Zhang, T. T. (2024). Exploring social media affordances in tourist destination image formation: a study on China’s rural tourism destination. Tourism. Manage. 101:104843. doi: 10.1016/j.tourman.2023.104843
Liu, J., Zhao, Y. C., and Jang, S. (2021). Understanding beach tourists’ environmentally responsible behaviors: an extended value-attitude-behavior model. J. Travel Tour. Mark. 38, 696–709. doi: 10.1080/10548408.2021.1985036
Lombard, M., and Jones, M. T. (2007). Identifying the (tele)presence literature. PsychNology. J. 5, 197–206. doi: 10.2139/ssrn.2078211
Lunardo, R., and Saintives, C. (2018). Coping with the ambivalent emotions of guilt and pride in the service context. J. Serv. Mark. 32, 360–370. doi: 10.1108/JSM-01-2017-0003
Mehrabian, A., and Russell, J. A. (1974). An approach to environmental Psycholog . Cambridge: The MIT Press.
Meng, Y., Chung, D., and Zhang, A. (2023). The effect of social media environmental information exposure on the intention to participate in proenvironmental behavior. PLoS One 18:e0294577. doi: 10.1371/journal.pone.0294577
Mischel, W., and Shoda, Y. (1995). A cognitive-affective system theory of personality: Reconceptualizing situations, dispositions, dynamics, and invariance in personality structure. Psychol. Rev. 102, 246–268. doi: 10.1037/0033-295X.102.2.246
Moore, M. M., and Yang, J. Z. (2020). Using eco-guilt to motivate environmental behavior change. Environ. Commun. 14, 522–536. doi: 10.1080/17524032.2019.1692889
Munanura, I. E., Parada, J. A., and Kline, J. D. (2023). A cognitive appraisal theory perspective of residents’ support for tourism. J. Ecotour. , 1–20. doi: 10.1080/14724049.2023.2198169
Onwezen, M. C., Antonides, G., and Bartels, J. (2013). The norm activation model: an exploration of the functions of anticipated pride and guilt in pro-environmental behaviour. J. Econ. Psychol. 39, 141–153. doi: 10.1016/j.joep.2013.07.005
Ou, C. X., Pavlou, P. A., and Davison, R. (2014). Swift guanxi in online marketplaces the role of computer-mediated communication technologies. Mis. Quart. 38, 209–230. doi: 10.25300/MISQ/2014/38.1.10
Pelet, J. É., Ettis, S., and Cowart, K. (2017). Optimal experience of flow enhanced by telepresence: evidence from social media use. Inform. Manag. 54, 115–128. doi: 10.1016/j.im.2016.05.001
Qiu, H. L., Wang, X. Z., Morrison, A. M., Kelly, C., and Wei, W. (2022). From ownership to responsibility: extending the theory of planned behavior to predict tourist environmentally responsible behavioral intentions. J. Sustain. Tour. , 1–24. doi: 10.1080/09669582.2022.2116643
Rajarathinam, K. K., Rathinam, L., and Parayitam, S. (2022). A moderated mediation model of the effect of social media advertising on consumer buying behaviour: evidence from India. Inter. J. Internet. Market. Advert. 17, 48–76. doi: 10.1504/IJIMA.2022.10050165
Sang, W. Y., Ji, S. Y., Pu, B., and Phau, I. (2023). The effect of tourism live streaming information quality and source credibility on users’ continuous viewing intention: an application of elaboration likelihood model. Asia. Pac. J. Tour. Res. 28, 177–190. doi: 10.1080/10941665.2023.2217954
Shang, Y., Mehmood, K., Iftikhar, Y., Aziz, A., Tao, X., and Shi, L. (2021). Energizing intention to visit ruraldestinations: how social media disposition and social media use boost tourism through information publicity. Front. Psychol. 12:782461. doi: 10.3389/fpsyg.2021.782461
Shipley, N. J., and van Riper, C. J. (2022). Pride and guilt predict pro-environmental behavior: a meta-analysis of correlational and experimental evidence. J. Environ. Psychol. 79:101753. doi: 10.1016/j.jenvp.2021.101753
Shu, B. Y., Wu, W. X., and Li, M. L. (2023). “Preview to positive”: field visit intention as consequences of presence in tourism short videos: the role of optimism bias. Asia. Pac. J. Tour. Res. 28, 763–776. doi: 10.1080/10941665.2023.2264971
Tam, K. P. (2013). Dispositional empathy with nature. J. Environ. Psychol. 35, 92–104. doi: 10.1016/j.jenvp.2013.05.004
Tang, H., Ma, Y., and Ren, J. (2022). Influencing factors and mechanism of tourists’pro-environmental behavior: empirical analysis of the CAC-MOA integration model. Front. Psychol. 13:1060404. doi: 10.3389/fpsyg.2022.1060404
Tangney, J., and Dearing, R. (2002). Shame and guilt . New York, NY: Guilford Press.
Theotokis, A., and Manganari, E. (2015). The impact of choice architecture on sustainable consumer behavior: the role of guilt. J. Bus. Ethics 131, 423–437. doi: 10.1007/s10551-014-2287-4
Tu, H. W., and Ma, J. F. (2022). Does positive contact between residents and tourists stimulate tourists’ environmentally responsible behavior? The role of gratitude and boundary conditions. J. Travel Res. 61, 1774–1790. doi: 10.1177/00472875211048938
Verhagen, T., Vonkeman, C., Feldberg, F., and Verhagen, P. (2014). Present it like it is here: creating local presence to improve online product experiences. Comput. Hum. Behav. 39, 270–280. doi: 10.1016/j.chb.2014.07.036
Vonkeman, C., Verhagen, T., and van Dolen, W. (2017). Role of local presence in online impulse buying. Inform. Manag. 54, 1038–1048. doi: 10.1016/j.im.2017.02.008
Wang, Y. F.Petrina, S., and Feng, F. (2017). Village-virtual immersive language learning and gaming environment: immersion and presence. Brit. J. Educ. Technol. 48,431–450. doi: 10.1111/bjet.12388
Wang, L. T., Sheng, G. H., She, S. X., and Xu, J. Q. (2023). Impact of empathy with nature on pro-environmental behaviour. Int. J. Consum. Stud. 47, 652–668. doi: 10.1111/ijcs.12856
Wang, J. M., and Wu, L. C. (2016). The impact of emotions on the intention of sustainable consumption choices: evidence from a big city in an emerging country. J. Clean. Prod. 126, 325–336. doi: 10.1016/j.jclepro.2016.03.119
Wang, G. Q., Yao, Y. B., Ren, L. P., Zhang, S., and Zhu, M. Y. (2023). Examining the role of generativity on tourists’ environmentally responsible behavior: an inter-generational comparison. J. Hosp. Tour. Manag. 57, 303–314. doi: 10.1016/j.jhtm.2023.10.008
Xu, X. Y., Huang, D., and Shang, X. Y. (2021). Social presence or physical presence? Determinants of purchasing behaviour in tourism live-streamed shopping. Tour. Manag. Perspect. 40:100917. doi: 10.1016/j.tmp.2021.100917
Yao, Y., and Jia, G. (2021). The effect of social media travel experience sharing on potential tourists’ impulsive travel intention: based on presence perspective. Nankai. Bus. Review. 24, 72–82.
Yin, J. L., Huang, Y. H., and Ma, Z. Z. (2023). Explore the feeling of presence and purchase intention in livestream shopping: A flow-based model. J. Theor. Appl. El. Comm. 18, 237–256. doi: 10.3390/jtaer18010013
Yu, X., Xu, Z., and Guo, W. (2017). Research on the influence of online product information presentation on customers’ behavior intention. Inform. Studies. 40, 80–84. doi: 10.16353/j.cnki.1000-7490.2017.10.015
Zhang, C. X., Pearce, P., and Chen, G. H. (2019). Not losing our collective face: social identity and Chinese tourists’ reflections on uncivilised behaviour. Tourism manage. 73, 71–82. doi: 10.1016/j.tourman.2019.01.020
Zhao, X., Wang, X., and Ji, L. (2020). Evaluating the effect of anticipated emotion on forming environmentally responsible behavior in heritage tourism: developing an extended model of norm activation theory. Asia. Pac. J. Tour. Res. 25, 1185–1198. doi: 10.1080/10941665.2020.1837892
Keywords: digital media information sharing, presence, eco-guilt, empathy with nature, tourists’ pro-environmental behavioral intention
Citation: Chen X, Cheng Z-f and Yang H-j (2024) Empowering pro-environmental behavior in tourists through digital media: the influence of eco-guilt and empathy with nature. Front. Psychol . 15:1387817. doi: 10.3389/fpsyg.2024.1387817
Received: 18 February 2024; Accepted: 26 April 2024; Published: 09 May 2024.
Reviewed by:
Copyright © 2024 Chen, Cheng and Yang. This is an open-access article distributed under the terms of the Creative Commons Attribution License (CC BY) . The use, distribution or reproduction in other forums is permitted, provided the original author(s) and the copyright owner(s) are credited and that the original publication in this journal is cited, in accordance with accepted academic practice. No use, distribution or reproduction is permitted which does not comply with these terms.
*Correspondence: Zhen-feng Cheng, [email protected]
Disclaimer: All claims expressed in this article are solely those of the authors and do not necessarily represent those of their affiliated organizations, or those of the publisher, the editors and the reviewers. Any product that may be evaluated in this article or claim that may be made by its manufacturer is not guaranteed or endorsed by the publisher.
The Federal Register
The daily journal of the united states government, request access.
Due to aggressive automated scraping of FederalRegister.gov and eCFR.gov, programmatic access to these sites is limited to access to our extensive developer APIs.
If you are human user receiving this message, we can add your IP address to a set of IPs that can access FederalRegister.gov & eCFR.gov; complete the CAPTCHA (bot test) below and click "Request Access". This process will be necessary for each IP address you wish to access the site from, requests are valid for approximately one quarter (three months) after which the process may need to be repeated.
An official website of the United States government.
If you want to request a wider IP range, first request access for your current IP, and then use the "Site Feedback" button found in the lower left-hand side to make the request.
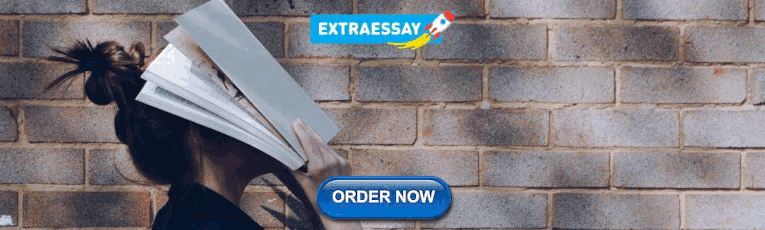
IMAGES
VIDEO
COMMENTS
Developing a hypothesis (with example) Step 1. Ask a question. Writing a hypothesis begins with a research question that you want to answer. The question should be focused, specific, and researchable within the constraints of your project. Example: Research question.
The primary research question should originate from the hypothesis, not the data, and be established before starting the study. Formulating the research question and hypothesis from existing data (e.g., a database) can lead to multiple statistical comparisons and potentially spurious findings due to chance.
DEVELOPING HYPOTHESES & RESEARCH QUESTIONS. The first hypothesis. provides a simple statement of association between Y and X. Nothing is indicated about the association that would allow the researcher to determine which variable, Y or X, would tend to cause the other variable to change in value. The second hypothesis.
INTRODUCTION. Scientific research is usually initiated by posing evidenced-based research questions which are then explicitly restated as hypotheses.1,2 The hypotheses provide directions to guide the study, solutions, explanations, and expected results.3,4 Both research questions and hypotheses are essentially formulated based on conventional theories and real-world processes, which allow the ...
Step 5: Phrase your hypothesis in three ways. To identify the variables, you can write a simple prediction in if … then form. The first part of the sentence states the independent variable and the second part states the dependent variable. If a first-year student starts attending more lectures, then their exam scores will improve.
3. Simple hypothesis. A simple hypothesis is a statement made to reflect the relation between exactly two variables. One independent and one dependent. Consider the example, "Smoking is a prominent cause of lung cancer." The dependent variable, lung cancer, is dependent on the independent variable, smoking. 4.
Aim for clarity and simplicity in your wording. State direction, if applicable: If your hypothesis involves a directional outcome (e.g., "increase" or "decrease"), make sure to specify this. You also need to think about how you will measure whether or not the outcome moved in the direction you predicted.
It seeks to explore and understand a particular aspect of the research subject. In contrast, a research hypothesis is a specific statement or prediction that suggests an expected relationship between variables. It is formulated based on existing knowledge or theories and guides the research design and data analysis. 7.
Primarily, a research question, just like a hypothesis, is focused and concise. But a hypothesis includes a prediction based on the proposed research, and is designed to forecast the relationship of and between two (or more) variables. Research questions are open-ended, and invite debate and discussion, while hypotheses are closed, e.g.
The steps to write a research hypothesis are: 1. Stating the problem: Ensure that the hypothesis defines the research problem. 2. Writing a hypothesis as an 'if-then' statement: Include the action and the expected outcome of your study by following a 'if-then' structure. 3.
Hypotheses are the testable statements linked to your research question. Hypotheses bridge the gap from the general question you intend to investigate (i.e., the research question) to concise statements of what you hypothesize the connection between your variables to be. For example, if we were studying the influence of mentoring relationships ...
Step 2: Conduct a literature review to gather essential existing research. Step 3: Write a clear, strong, simply worded sentence that explains your test parameter, test direction, and hypothesized parameter. Step 4: Read it a few times. Have others read it and ask them what they think it means.
Most quantitative research falls into one or more of these three categories. The most rigorous form of quantitative research follows from a test of a theory (see Chapter 3) and the specification of research questions or hypotheses that are included in the theory. The independent and dependent variables must be measured sepa-rately.
Formulating Hypotheses for Different Study Designs. Generating a testable working hypothesis is the first step towards conducting original research. Such research may prove or disprove the proposed hypothesis. Case reports, case series, online surveys and other observational studies, clinical trials, and narrative reviews help to generate ...
A research hypothesis (also called a scientific hypothesis) is a statement about the expected outcome of a study (for example, a dissertation or thesis). To constitute a quality hypothesis, the statement needs to have three attributes - specificity, clarity and testability. Let's take a look at these more closely.
A hypothesis is a tentative statement about the relationship between two or more variables. It is a specific, testable prediction about what you expect to happen in a study. It is a preliminary answer to your question that helps guide the research process. Consider a study designed to examine the relationship between sleep deprivation and test ...
Researchers use experimental research designs to test hypotheses and establish cause-and-effect relationships. ... Here is a general overview of how to conduct quantitative research: Develop a research question: The first step in conducting quantitative research is to develop a clear and specific research question. This question should be based ...
The research hypothesis is central to all research endeavors, whether qualitative or quantitative, exploratory or explanatory. At its most basic, the research hypothesis states what the researcher expects to find - it is the tentative answer to the research question that guides the entire study. Developing testable research hypotheses takes ...
As a consequence, the results of quantitative research may be statistically significant but are often humanly insignificant. Some specific limitations associated with using quantitative methods to study research problems in the social sciences include: Quantitative data is more efficient and able to test hypotheses, but may miss contextual detail;
Due to these reasons, quantitative research is not an ideal choice for detailed kinds of research. For them, qualitative research works better. To help you further, we have added some useful examples of quantitative research here. Quantitative Research Examples. Below are some helpful quantitative research examples to help you understand it better.
Background Knowledge of statistics is highly important for research scholars, as they are expected to submit a thesis based on original research as part of a PhD program. As statistics play a major role in the analysis and interpretation of scientific data, intensive training at the beginning of a PhD programme is essential. PhD coursework is mandatory in universities and higher education ...
In our study, qualitative research, including interviews, coding, and results analysis, was used to explore the antecedents affecting users' intention to co-creating health values in OHCs and to develop hypotheses accordingly. The quantitative study tested the proposed hypotheses and models. 3.1 Data collection
A good research question is essential to guide your research paper, dissertation, or thesis. All research questions should be: Focused on a single problem or issue. Researchable using primary and/or secondary sources. Feasible to answer within the timeframe and practical constraints. Specific enough to answer thoroughly.
Our division regulates vaccines against infectious diseases caused by viruses, and our research program is directed at both developing new tools that could be used to evaluate the safety of cell ...
3 Research hypotheses 3.1 Digital media information sharing and presence. Some scholars have noted that the formats of information presentation in digital media, including text, images, and videos, are closely linked to the sense of presence, with each form having a distinct impact on individuals' presence (Yao and Jia, 2021).
Soybean (Glycine max) is a vital food crop, serving as a major source of high-quality protein for human and animal consumption. Stem diameter is one of the primary determinants of the stem lodging resistance of a given plant, but there has been relatively little research to date focused on genes associated with this trait. To address this gap in the literature, 207 chromosome segment ...
In response to comments received during that earlier rulemaking, we considered, and declined, the option to establish a qualitative standard alone in lieu of a quantitative microbial quality requirement for pre-harvest agricultural water. 80 FR 74354 at 74443. However, since 2015, new scientific findings as well as findings from FDA outbreak ...