Have a language expert improve your writing
Run a free plagiarism check in 10 minutes, automatically generate references for free.
- Knowledge Base
- Methodology
- How to Write a Strong Hypothesis | Guide & Examples
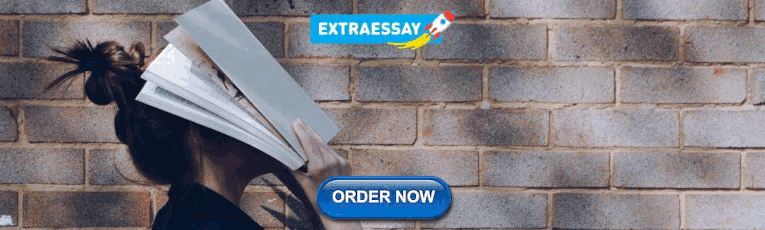
How to Write a Strong Hypothesis | Guide & Examples
Published on 6 May 2022 by Shona McCombes .
A hypothesis is a statement that can be tested by scientific research. If you want to test a relationship between two or more variables, you need to write hypotheses before you start your experiment or data collection.
Table of contents
What is a hypothesis, developing a hypothesis (with example), hypothesis examples, frequently asked questions about writing hypotheses.
A hypothesis states your predictions about what your research will find. It is a tentative answer to your research question that has not yet been tested. For some research projects, you might have to write several hypotheses that address different aspects of your research question.
A hypothesis is not just a guess – it should be based on existing theories and knowledge. It also has to be testable, which means you can support or refute it through scientific research methods (such as experiments, observations, and statistical analysis of data).
Variables in hypotheses
Hypotheses propose a relationship between two or more variables . An independent variable is something the researcher changes or controls. A dependent variable is something the researcher observes and measures.
In this example, the independent variable is exposure to the sun – the assumed cause . The dependent variable is the level of happiness – the assumed effect .
Prevent plagiarism, run a free check.
Step 1: ask a question.
Writing a hypothesis begins with a research question that you want to answer. The question should be focused, specific, and researchable within the constraints of your project.
Step 2: Do some preliminary research
Your initial answer to the question should be based on what is already known about the topic. Look for theories and previous studies to help you form educated assumptions about what your research will find.
At this stage, you might construct a conceptual framework to identify which variables you will study and what you think the relationships are between them. Sometimes, you’ll have to operationalise more complex constructs.
Step 3: Formulate your hypothesis
Now you should have some idea of what you expect to find. Write your initial answer to the question in a clear, concise sentence.
Step 4: Refine your hypothesis
You need to make sure your hypothesis is specific and testable. There are various ways of phrasing a hypothesis, but all the terms you use should have clear definitions, and the hypothesis should contain:
- The relevant variables
- The specific group being studied
- The predicted outcome of the experiment or analysis
Step 5: Phrase your hypothesis in three ways
To identify the variables, you can write a simple prediction in if … then form. The first part of the sentence states the independent variable and the second part states the dependent variable.
In academic research, hypotheses are more commonly phrased in terms of correlations or effects, where you directly state the predicted relationship between variables.
If you are comparing two groups, the hypothesis can state what difference you expect to find between them.
Step 6. Write a null hypothesis
If your research involves statistical hypothesis testing , you will also have to write a null hypothesis. The null hypothesis is the default position that there is no association between the variables. The null hypothesis is written as H 0 , while the alternative hypothesis is H 1 or H a .
Hypothesis testing is a formal procedure for investigating our ideas about the world using statistics. It is used by scientists to test specific predictions, called hypotheses , by calculating how likely it is that a pattern or relationship between variables could have arisen by chance.
A hypothesis is not just a guess. It should be based on existing theories and knowledge. It also has to be testable, which means you can support or refute it through scientific research methods (such as experiments, observations, and statistical analysis of data).
A research hypothesis is your proposed answer to your research question. The research hypothesis usually includes an explanation (‘ x affects y because …’).
A statistical hypothesis, on the other hand, is a mathematical statement about a population parameter. Statistical hypotheses always come in pairs: the null and alternative hypotheses. In a well-designed study , the statistical hypotheses correspond logically to the research hypothesis.
Cite this Scribbr article
If you want to cite this source, you can copy and paste the citation or click the ‘Cite this Scribbr article’ button to automatically add the citation to our free Reference Generator.
McCombes, S. (2022, May 06). How to Write a Strong Hypothesis | Guide & Examples. Scribbr. Retrieved 15 April 2024, from https://www.scribbr.co.uk/research-methods/hypothesis-writing/
Is this article helpful?
Shona McCombes
Other students also liked, operationalisation | a guide with examples, pros & cons, what is a conceptual framework | tips & examples, a quick guide to experimental design | 5 steps & examples.
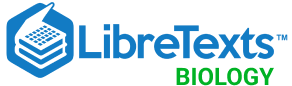
- school Campus Bookshelves
- menu_book Bookshelves
- perm_media Learning Objects
- login Login
- how_to_reg Request Instructor Account
- hub Instructor Commons
- Download Page (PDF)
- Download Full Book (PDF)
- Periodic Table
- Physics Constants
- Scientific Calculator
- Reference & Cite
- Tools expand_more
- Readability
selected template will load here
This action is not available.
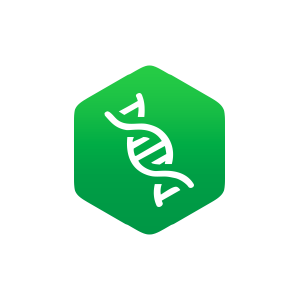
1.1: Scientific Investigation
- Last updated
- Save as PDF
- Page ID 6252
Chances are you've heard of the scientific method. But what exactly is the scientific method?
Is it a precise and exact way that all science must be done? Or is it a series of steps that most scientists generally follow, but may be modified for the benefit of an individual investigation?
The Scientific Method
"We also discovered that science is cool and fun because you get to do stuff that no one has ever done before." In the article Blackawton bees, published by eight to ten year old students: Biology Letters (2010) http://rsbl.royalsocietypublishing.org/content/early/2010/12/18/rsbl.2010.1056.abstract .
There are basic methods of gaining knowledge that are common to all of science. At the heart of science is the scientific investigation, which is done by following the scientific method . A scientific investigation is a plan for asking questions and testing possible answers. It generally follows the steps listed in Figure below . See http://www.youtube.com/watch?v=KZaCy5Z87FA for an overview of the scientific method.
Steps of a Scientific Investigation. A scientific investigation typically has these steps. Scientists often develop their own steps they follow in a scientific investigation. Shown here is a simplification of how a scientific investigation is done.
Making Observations
A scientific investigation typically begins with observations. You make observations all the time. Let’s say you take a walk in the woods and observe a moth, like the one in Figure below, resting on a tree trunk. You notice that the moth has spots on its wings that look like eyes. You think the eye spots make the moth look like the face of an owl.
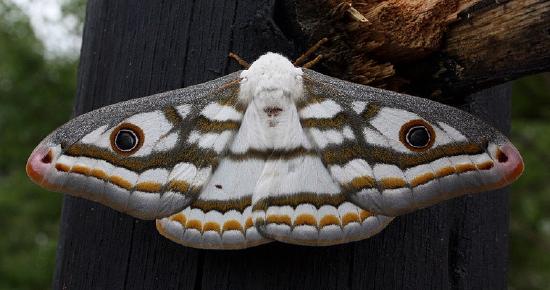
Figure 2: Marbled emperor moth Heniocha dyops in Botswana. (CC-SA-BY-4.0; Charlesjsharp ).
Does this moth remind you of an owl?
Asking a Question
Observations often lead to questions. For example, you might ask yourself why the moth has eye spots that make it look like an owl’s face. What reason might there be for this observation?
Forming a Hypothesis
The next step in a scientific investigation is forming a hypothesis . A hypothesis is a possible answer to a scientific question, but it isn’t just any answer. A hypothesis must be based on scientific knowledge, and it must be logical. A hypothesis also must be falsifiable. In other words, it must be possible to make observations that would disprove the hypothesis if it really is false. Assume you know that some birds eat moths and that owls prey on other birds. From this knowledge, you reason that eye spots scare away birds that might eat the moth. This is your hypothesis.
Testing the Hypothesis
To test a hypothesis, you first need to make a prediction based on the hypothesis. A prediction is a statement that tells what will happen under certain conditions. It can be expressed in the form: If A occurs, then B will happen. Based on your hypothesis, you might make this prediction: If a moth has eye spots on its wings, then birds will avoid eating it.
Next, you must gather evidence to test your prediction. Evidence is any type of data that may either agree or disagree with a prediction, so it may either support or disprove a hypothesis. Evidence may be gathered by an experiment . Assume that you gather evidence by making more observations of moths with eye spots. Perhaps you observe that birds really do avoid eating moths with eye spots. This evidence agrees with your prediction.
Drawing Conclusions
Evidence that agrees with your prediction supports your hypothesis. Does such evidence prove that your hypothesis is true? No; a hypothesis cannot be proven conclusively to be true. This is because you can never examine all of the possible evidence, and someday evidence might be found that disproves the hypothesis. Nonetheless, the more evidence that supports a hypothesis, the more likely the hypothesis is to be true.
Communicating Results
The last step in a scientific investigation is communicating what you have learned with others. This is a very important step because it allows others to test your hypothesis. If other researchers get the same results as yours, they add support to the hypothesis. However, if they get different results, they may disprove the hypothesis.
When scientists share their results, they should describe their methods and point out any possible problems with the investigation. For example, while you were observing moths, perhaps your presence scared birds away. This introduces an error into your investigation. You got the results you predicted (the birds avoided the moths while you were observing them), but not for the reason you hypothesized. Other researchers might be able to think of ways to avoid this error in future studies.
The Scientific Method Made Easy explains scientific method: http://www.youtube.com/watch?v=zcavPAFiG14 (9:55).
As you view The Scientific Method Made Easy, focus on these concepts: the relationship between evidence, conclusions and theories, the "ground rules" of scientific research, the steps in a scientific procedure, the meaning of the "replication of results," the meaning of "falsifiable," the outcome when the scientific method is not followed.
Discovering the Scientific Method
A summery video of the scientific method, using the identification of DNA structure as an example, is shown in this video by MIT students: https://www.youtube.com/watch?v=5eDNgeEUtMg .
Why I do Science
Dan Costa, Ph.D. is a professor of Biology at the University of California, Santa Cruz, and has been studying marine life for well over 40 years. He is a leader in using satellite tags, time and depth recorders and other sophisticated electronic tags to gather information about the amazing depths to which elephant seals dive, their migration routes and how they use oceanographic features to hunt for prey as far as the international dateline and the Alaskan Aleutian Islands. In the following KQED video, Dr. Costa discusses why he is a scientist: http://science.kqed.org/quest/video/why-i-do-science-dan-costa/ .
- At the heart of science is the scientific investigation, which is done by following the scientific method. A scientific investigation is a plan for asking questions and testing possible answers.
- A scientific investigation typically begins with observations. Observations often lead to questions.
- A hypothesis is a possible logical answer to a scientific question, based on scientific knowledge.
- A prediction is a statement that tells what will happen under certain conditions.
- Evidence is any type of data that may either agree or disagree with a prediction, so it may either support or disprove a hypothesis. Conclusions may be formed from evidence.
- The last step in a scientific investigation is the communication of results with others.
Explore More
Explore more i.
Use this resource to answer the questions that follow.
- Steps of the Scientific Method at http://www.sciencebuddies.org/science-fair-projects/project_scientific_method.shtml#overviewofthescientificmethod .
- Describe what is means to "Ask a Question."
- Describe what it means to "Construct a Hypothesis."
- How does a scientist conduct a fair test?
- What does a scientist do if the hypothesis is not supported?
Explore More II
- SciMeth Matching at http://www.studystack.com/matching-2497 .
- Outline the steps of a scientific investigation.
- What is a scientific hypothesis? What characteristics must a hypothesis have to be useful in science?
- Give an example of a scientific question that could be investigated with an experiment. Then give an example of question that could not be investigated.
- Can a hypothesis be proven true? Why or why not?
- Why do scientists communicate their results?
If you're seeing this message, it means we're having trouble loading external resources on our website.
If you're behind a web filter, please make sure that the domains *.kastatic.org and *.kasandbox.org are unblocked.
To log in and use all the features of Khan Academy, please enable JavaScript in your browser.
Biology library
Course: biology library > unit 1, the scientific method.
- Controlled experiments
- The scientific method and experimental design
Introduction
- Make an observation.
- Ask a question.
- Form a hypothesis , or testable explanation.
- Make a prediction based on the hypothesis.
- Test the prediction.
- Iterate: use the results to make new hypotheses or predictions.
Scientific method example: Failure to toast
1. make an observation..
- Observation: the toaster won't toast.
2. Ask a question.
- Question: Why won't my toaster toast?
3. Propose a hypothesis.
- Hypothesis: Maybe the outlet is broken.
4. Make predictions.
- Prediction: If I plug the toaster into a different outlet, then it will toast the bread.
5. Test the predictions.
- Test of prediction: Plug the toaster into a different outlet and try again.
- If the toaster does toast, then the hypothesis is supported—likely correct.
- If the toaster doesn't toast, then the hypothesis is not supported—likely wrong.
Logical possibility
Practical possibility, building a body of evidence, 6. iterate..
- Iteration time!
- If the hypothesis was supported, we might do additional tests to confirm it, or revise it to be more specific. For instance, we might investigate why the outlet is broken.
- If the hypothesis was not supported, we would come up with a new hypothesis. For instance, the next hypothesis might be that there's a broken wire in the toaster.
Want to join the conversation?
- Upvote Button navigates to signup page
- Downvote Button navigates to signup page
- Flag Button navigates to signup page

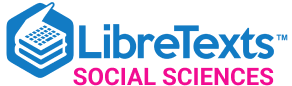
- school Campus Bookshelves
- menu_book Bookshelves
- perm_media Learning Objects
- login Login
- how_to_reg Request Instructor Account
- hub Instructor Commons
- Download Page (PDF)
- Download Full Book (PDF)
- Periodic Table
- Physics Constants
- Scientific Calculator
- Reference & Cite
- Tools expand_more
- Readability
selected template will load here
This action is not available.
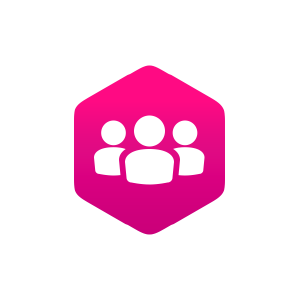
2.4: Developing a Hypothesis
- Last updated
- Save as PDF
- Page ID 16104

Learning Objectives
- Distinguish between a theory and a hypothesis.
- Discover how theories are used to generate hypotheses and how the results of studies can be used to further inform theories.
- Understand the characteristics of a good hypothesis.
Theories and Hypotheses
Before describing how to develop a hypothesis it is important to distinguish between a theory and a hypothesis. A theory is a coherent explanation or interpretation of one or more phenomena. Although theories can take a variety of forms, one thing they have in common is that they go beyond the phenomena they explain by including variables, structures, processes, functions, or organizing principles that have not been observed directly. Consider, for example, Zajonc’s theory of social facilitation and social inhibition. He proposed that being watched by others while performing a task creates a general state of physiological arousal, which increases the likelihood of the dominant (most likely) response. So for highly practiced tasks, being watched increases the tendency to make correct responses, but for relatively unpracticed tasks, being watched increases the tendency to make incorrect responses. Notice that this theory—which has come to be called drive theory—provides an explanation of both social facilitation and social inhibition that goes beyond the phenomena themselves by including concepts such as “arousal” and “dominant response,” along with processes such as the effect of arousal on the dominant response.
Outside of science, referring to an idea as a theory often implies that it is untested—perhaps no more than a wild guess. In science, however, the term theory has no such implication. A theory is simply an explanation or interpretation of a set of phenomena. It can be untested, but it can also be extensively tested, well supported, and accepted as an accurate description of the world by the scientific community. The theory of evolution by natural selection, for example, is a theory because it is an explanation of the diversity of life on earth—not because it is untested or unsupported by scientific research. On the contrary, the evidence for this theory is overwhelmingly positive and nearly all scientists accept its basic assumptions as accurate. Similarly, the “germ theory” of disease is a theory because it is an explanation of the origin of various diseases, not because there is any doubt that many diseases are caused by microorganisms that infect the body.
A hypothesis , on the other hand, is a specific prediction about a new phenomenon that should be observed if a particular theory is accurate. It is an explanation that relies on just a few key concepts. Hypotheses are often specific predictions about what will happen in a particular study. They are developed by considering existing evidence and using reasoning to infer what will happen in the specific context of interest. Hypotheses are often but not always derived from theories. So a hypothesis is often a prediction based on a theory but some hypotheses are a-theoretical and only after a set of observations have been made, is a theory developed. This is because theories are broad in nature and they explain larger bodies of data. So if our research question is really original then we may need to collect some data and make some observation before we can develop a broader theory.
Theories and hypotheses always have this if-then relationship. “ If drive theory is correct, then cockroaches should run through a straight runway faster, and a branching runway more slowly, when other cockroaches are present.” Although hypotheses are usually expressed as statements, they can always be rephrased as questions. “Do cockroaches run through a straight runway faster when other cockroaches are present?” Thus deriving hypotheses from theories is an excellent way of generating interesting research questions.
But how do researchers derive hypotheses from theories? One way is to generate a research question using the techniques discussed in this chapter and then ask whether any theory implies an answer to that question. For example, you might wonder whether expressive writing about positive experiences improves health as much as expressive writing about traumatic experiences. Although this question is an interesting one on its own, you might then ask whether the habituation theory—the idea that expressive writing causes people to habituate to negative thoughts and feelings—implies an answer. In this case, it seems clear that if the habituation theory is correct, then expressive writing about positive experiences should not be effective because it would not cause people to habituate to negative thoughts and feelings. A second way to derive hypotheses from theories is to focus on some component of the theory that has not yet been directly observed. For example, a researcher could focus on the process of habituation—perhaps hypothesizing that people should show fewer signs of emotional distress with each new writing session.
Among the very best hypotheses are those that distinguish between competing theories. For example, Norbert Schwarz and his colleagues considered two theories of how people make judgments about themselves, such as how assertive they are (Schwarz et al., 1991) [1] . Both theories held that such judgments are based on relevant examples that people bring to mind. However, one theory was that people base their judgments on the number of examples they bring to mind and the other was that people base their judgments on how easily they bring those examples to mind. To test these theories, the researchers asked people to recall either six times when they were assertive (which is easy for most people) or 12 times (which is difficult for most people). Then they asked them to judge their own assertiveness. Note that the number-of-examples theory implies that people who recalled 12 examples should judge themselves to be more assertive because they recalled more examples, but the ease-of-examples theory implies that participants who recalled six examples should judge themselves as more assertive because recalling the examples was easier. Thus the two theories made opposite predictions so that only one of the predictions could be confirmed. The surprising result was that participants who recalled fewer examples judged themselves to be more assertive—providing particularly convincing evidence in favor of the ease-of-retrieval theory over the number-of-examples theory.
Theory Testing
The primary way that scientific researchers use theories is sometimes called the hypothetico-deductive method (although this term is much more likely to be used by philosophers of science than by scientists themselves). A researcher begins with a set of phenomena and either constructs a theory to explain or interpret them or chooses an existing theory to work with. He or she then makes a prediction about some new phenomenon that should be observed if the theory is correct. Again, this prediction is called a hypothesis. The researcher then conducts an empirical study to test the hypothesis. Finally, he or she reevaluates the theory in light of the new results and revises it if necessary. This process is usually conceptualized as a cycle because the researcher can then derive a new hypothesis from the revised theory, conduct a new empirical study to test the hypothesis, and so on. As Figure 2.2 shows, this approach meshes nicely with the model of scientific research in psychology presented earlier in the textbook—creating a more detailed model of “theoretically motivated” or “theory-driven” research.
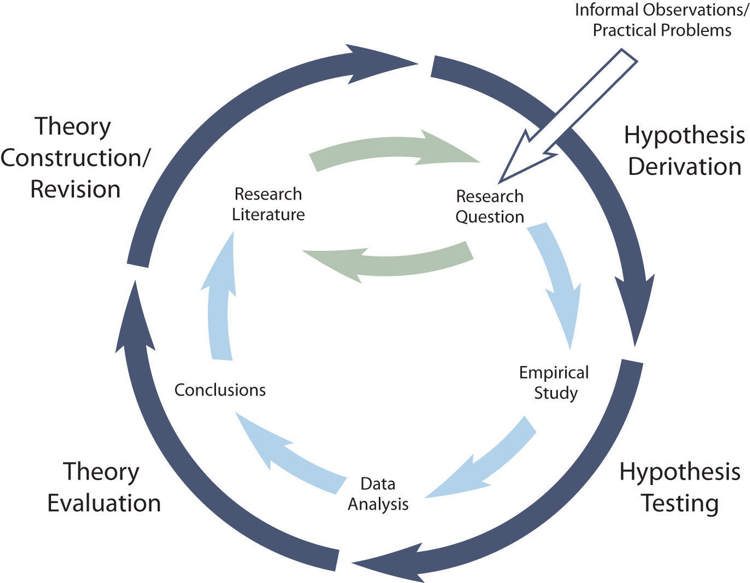
As an example, let us consider Zajonc’s research on social facilitation and inhibition. He started with a somewhat contradictory pattern of results from the research literature. He then constructed his drive theory, according to which being watched by others while performing a task causes physiological arousal, which increases an organism’s tendency to make the dominant response. This theory predicts social facilitation for well-learned tasks and social inhibition for poorly learned tasks. He now had a theory that organized previous results in a meaningful way—but he still needed to test it. He hypothesized that if his theory was correct, he should observe that the presence of others improves performance in a simple laboratory task but inhibits performance in a difficult version of the very same laboratory task. To test this hypothesis, one of the studies he conducted used cockroaches as subjects (Zajonc, Heingartner, & Herman, 1969) [2] . The cockroaches ran either down a straight runway (an easy task for a cockroach) or through a cross-shaped maze (a difficult task for a cockroach) to escape into a dark chamber when a light was shined on them. They did this either while alone or in the presence of other cockroaches in clear plastic “audience boxes.” Zajonc found that cockroaches in the straight runway reached their goal more quickly in the presence of other cockroaches, but cockroaches in the cross-shaped maze reached their goal more slowly when they were in the presence of other cockroaches. Thus he confirmed his hypothesis and provided support for his drive theory. (Zajonc also showed that drive theory existed in humans (Zajonc & Sales, 1966) [3] in many other studies afterward).
Incorporating Theory into Your Research
When you write your research report or plan your presentation, be aware that there are two basic ways that researchers usually include theory. The first is to raise a research question, answer that question by conducting a new study, and then offer one or more theories (usually more) to explain or interpret the results. This format works well for applied research questions and for research questions that existing theories do not address. The second way is to describe one or more existing theories, derive a hypothesis from one of those theories, test the hypothesis in a new study, and finally reevaluate the theory. This format works well when there is an existing theory that addresses the research question—especially if the resulting hypothesis is surprising or conflicts with a hypothesis derived from a different theory.
To use theories in your research will not only give you guidance in coming up with experiment ideas and possible projects, but it lends legitimacy to your work. Psychologists have been interested in a variety of human behaviors and have developed many theories along the way. Using established theories will help you break new ground as a researcher, not limit you from developing your own ideas.
Characteristics of a Good Hypothesis
There are three general characteristics of a good hypothesis. First, a good hypothesis must be testable and falsifiable . We must be able to test the hypothesis using the methods of science and if you’ll recall Popper’s falsifiability criterion, it must be possible to gather evidence that will disconfirm the hypothesis if it is indeed false. Second, a good hypothesis must be logical. As described above, hypotheses are more than just a random guess. Hypotheses should be informed by previous theories or observations and logical reasoning. Typically, we begin with a broad and general theory and use deductive reasoning to generate a more specific hypothesis to test based on that theory. Occasionally, however, when there is no theory to inform our hypothesis, we use inductive reasoning which involves using specific observations or research findings to form a more general hypothesis. Finally, the hypothesis should be positive. That is, the hypothesis should make a positive statement about the existence of a relationship or effect, rather than a statement that a relationship or effect does not exist. As scientists, we don’t set out to show that relationships do not exist or that effects do not occur so our hypotheses should not be worded in a way to suggest that an effect or relationship does not exist. The nature of science is to assume that something does not exist and then seek to find evidence to prove this wrong, to show that really it does exist. That may seem backward to you but that is the nature of the scientific method. The underlying reason for this is beyond the scope of this chapter but it has to do with statistical theory.
Key Takeaways
- A theory is broad in nature and explains larger bodies of data. A hypothesis is more specific and makes a prediction about the outcome of a particular study.
- Working with theories is not “icing on the cake.” It is a basic ingredient of psychological research.
- Like other scientists, psychologists use the hypothetico-deductive method. They construct theories to explain or interpret phenomena (or work with existing theories), derive hypotheses from their theories, test the hypotheses, and then reevaluate the theories in light of the new results.
- Practice: Find a recent empirical research report in a professional journal. Read the introduction and highlight in different colors descriptions of theories and hypotheses.
- Schwarz, N., Bless, H., Strack, F., Klumpp, G., Rittenauer-Schatka, H., & Simons, A. (1991). Ease of retrieval as information: Another look at the availability heuristic. Journal of Personality and Social Psychology, 61 , 195–202.
- Zajonc, R. B., Heingartner, A., & Herman, E. M. (1969). Social enhancement and impairment of performance in the cockroach. Journal of Personality and Social Psychology, 13 , 83–92.
- Zajonc, R.B. & Sales, S.M. (1966). Social facilitation of dominant and subordinate responses. Journal of Experimental Social Psychology, 2 , 160-168.
Module 1: Introduction to Biology
Experiments and hypotheses, learning outcomes.
- Form a hypothesis and use it to design a scientific experiment
Now we’ll focus on the methods of scientific inquiry. Science often involves making observations and developing hypotheses. Experiments and further observations are often used to test the hypotheses.
A scientific experiment is a carefully organized procedure in which the scientist intervenes in a system to change something, then observes the result of the change. Scientific inquiry often involves doing experiments, though not always. For example, a scientist studying the mating behaviors of ladybugs might begin with detailed observations of ladybugs mating in their natural habitats. While this research may not be experimental, it is scientific: it involves careful and verifiable observation of the natural world. The same scientist might then treat some of the ladybugs with a hormone hypothesized to trigger mating and observe whether these ladybugs mated sooner or more often than untreated ones. This would qualify as an experiment because the scientist is now making a change in the system and observing the effects.
Forming a Hypothesis
When conducting scientific experiments, researchers develop hypotheses to guide experimental design. A hypothesis is a suggested explanation that is both testable and falsifiable. You must be able to test your hypothesis, and it must be possible to prove your hypothesis true or false.
For example, Michael observes that maple trees lose their leaves in the fall. He might then propose a possible explanation for this observation: “cold weather causes maple trees to lose their leaves in the fall.” This statement is testable. He could grow maple trees in a warm enclosed environment such as a greenhouse and see if their leaves still dropped in the fall. The hypothesis is also falsifiable. If the leaves still dropped in the warm environment, then clearly temperature was not the main factor in causing maple leaves to drop in autumn.
In the Try It below, you can practice recognizing scientific hypotheses. As you consider each statement, try to think as a scientist would: can I test this hypothesis with observations or experiments? Is the statement falsifiable? If the answer to either of these questions is “no,” the statement is not a valid scientific hypothesis.
Practice Questions
Determine whether each following statement is a scientific hypothesis.
Air pollution from automobile exhaust can trigger symptoms in people with asthma.
- No. This statement is not testable or falsifiable.
- No. This statement is not testable.
- No. This statement is not falsifiable.
- Yes. This statement is testable and falsifiable.
Natural disasters, such as tornadoes, are punishments for bad thoughts and behaviors.
a: No. This statement is not testable or falsifiable. “Bad thoughts and behaviors” are excessively vague and subjective variables that would be impossible to measure or agree upon in a reliable way. The statement might be “falsifiable” if you came up with a counterexample: a “wicked” place that was not punished by a natural disaster. But some would question whether the people in that place were really wicked, and others would continue to predict that a natural disaster was bound to strike that place at some point. There is no reason to suspect that people’s immoral behavior affects the weather unless you bring up the intervention of a supernatural being, making this idea even harder to test.
Testing a Vaccine
Let’s examine the scientific process by discussing an actual scientific experiment conducted by researchers at the University of Washington. These researchers investigated whether a vaccine may reduce the incidence of the human papillomavirus (HPV). The experimental process and results were published in an article titled, “ A controlled trial of a human papillomavirus type 16 vaccine .”
Preliminary observations made by the researchers who conducted the HPV experiment are listed below:
- Human papillomavirus (HPV) is the most common sexually transmitted virus in the United States.
- There are about 40 different types of HPV. A significant number of people that have HPV are unaware of it because many of these viruses cause no symptoms.
- Some types of HPV can cause cervical cancer.
- About 4,000 women a year die of cervical cancer in the United States.
Practice Question
Researchers have developed a potential vaccine against HPV and want to test it. What is the first testable hypothesis that the researchers should study?
- HPV causes cervical cancer.
- People should not have unprotected sex with many partners.
- People who get the vaccine will not get HPV.
- The HPV vaccine will protect people against cancer.
Experimental Design
You’ve successfully identified a hypothesis for the University of Washington’s study on HPV: People who get the HPV vaccine will not get HPV.
The next step is to design an experiment that will test this hypothesis. There are several important factors to consider when designing a scientific experiment. First, scientific experiments must have an experimental group. This is the group that receives the experimental treatment necessary to address the hypothesis.
The experimental group receives the vaccine, but how can we know if the vaccine made a difference? Many things may change HPV infection rates in a group of people over time. To clearly show that the vaccine was effective in helping the experimental group, we need to include in our study an otherwise similar control group that does not get the treatment. We can then compare the two groups and determine if the vaccine made a difference. The control group shows us what happens in the absence of the factor under study.
However, the control group cannot get “nothing.” Instead, the control group often receives a placebo. A placebo is a procedure that has no expected therapeutic effect—such as giving a person a sugar pill or a shot containing only plain saline solution with no drug. Scientific studies have shown that the “placebo effect” can alter experimental results because when individuals are told that they are or are not being treated, this knowledge can alter their actions or their emotions, which can then alter the results of the experiment.
Moreover, if the doctor knows which group a patient is in, this can also influence the results of the experiment. Without saying so directly, the doctor may show—through body language or other subtle cues—their views about whether the patient is likely to get well. These errors can then alter the patient’s experience and change the results of the experiment. Therefore, many clinical studies are “double blind.” In these studies, neither the doctor nor the patient knows which group the patient is in until all experimental results have been collected.
Both placebo treatments and double-blind procedures are designed to prevent bias. Bias is any systematic error that makes a particular experimental outcome more or less likely. Errors can happen in any experiment: people make mistakes in measurement, instruments fail, computer glitches can alter data. But most such errors are random and don’t favor one outcome over another. Patients’ belief in a treatment can make it more likely to appear to “work.” Placebos and double-blind procedures are used to level the playing field so that both groups of study subjects are treated equally and share similar beliefs about their treatment.
The scientists who are researching the effectiveness of the HPV vaccine will test their hypothesis by separating 2,392 young women into two groups: the control group and the experimental group. Answer the following questions about these two groups.
- This group is given a placebo.
- This group is deliberately infected with HPV.
- This group is given nothing.
- This group is given the HPV vaccine.
- a: This group is given a placebo. A placebo will be a shot, just like the HPV vaccine, but it will have no active ingredient. It may change peoples’ thinking or behavior to have such a shot given to them, but it will not stimulate the immune systems of the subjects in the same way as predicted for the vaccine itself.
- d: This group is given the HPV vaccine. The experimental group will receive the HPV vaccine and researchers will then be able to see if it works, when compared to the control group.
Experimental Variables
A variable is a characteristic of a subject (in this case, of a person in the study) that can vary over time or among individuals. Sometimes a variable takes the form of a category, such as male or female; often a variable can be measured precisely, such as body height. Ideally, only one variable is different between the control group and the experimental group in a scientific experiment. Otherwise, the researchers will not be able to determine which variable caused any differences seen in the results. For example, imagine that the people in the control group were, on average, much more sexually active than the people in the experimental group. If, at the end of the experiment, the control group had a higher rate of HPV infection, could you confidently determine why? Maybe the experimental subjects were protected by the vaccine, but maybe they were protected by their low level of sexual contact.
To avoid this situation, experimenters make sure that their subject groups are as similar as possible in all variables except for the variable that is being tested in the experiment. This variable, or factor, will be deliberately changed in the experimental group. The one variable that is different between the two groups is called the independent variable. An independent variable is known or hypothesized to cause some outcome. Imagine an educational researcher investigating the effectiveness of a new teaching strategy in a classroom. The experimental group receives the new teaching strategy, while the control group receives the traditional strategy. It is the teaching strategy that is the independent variable in this scenario. In an experiment, the independent variable is the variable that the scientist deliberately changes or imposes on the subjects.
Dependent variables are known or hypothesized consequences; they are the effects that result from changes or differences in an independent variable. In an experiment, the dependent variables are those that the scientist measures before, during, and particularly at the end of the experiment to see if they have changed as expected. The dependent variable must be stated so that it is clear how it will be observed or measured. Rather than comparing “learning” among students (which is a vague and difficult to measure concept), an educational researcher might choose to compare test scores, which are very specific and easy to measure.
In any real-world example, many, many variables MIGHT affect the outcome of an experiment, yet only one or a few independent variables can be tested. Other variables must be kept as similar as possible between the study groups and are called control variables . For our educational research example, if the control group consisted only of people between the ages of 18 and 20 and the experimental group contained people between the ages of 30 and 35, we would not know if it was the teaching strategy or the students’ ages that played a larger role in the results. To avoid this problem, a good study will be set up so that each group contains students with a similar age profile. In a well-designed educational research study, student age will be a controlled variable, along with other possibly important factors like gender, past educational achievement, and pre-existing knowledge of the subject area.
What is the independent variable in this experiment?
- Sex (all of the subjects will be female)
- Presence or absence of the HPV vaccine
- Presence or absence of HPV (the virus)
List three control variables other than age.
What is the dependent variable in this experiment?
- Sex (male or female)
- Rates of HPV infection
- Age (years)
Contribute!
Improve this page Learn More
- Revision and adaptation. Authored by : Shelli Carter and Lumen Learning. Provided by : Lumen Learning. License : CC BY-NC-SA: Attribution-NonCommercial-ShareAlike
- Scientific Inquiry. Provided by : Open Learning Initiative. Located at : https://oli.cmu.edu/jcourse/workbook/activity/page?context=434a5c2680020ca6017c03488572e0f8 . Project : Introduction to Biology (Open + Free). License : CC BY-NC-SA: Attribution-NonCommercial-ShareAlike
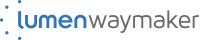

How to Develop a Good Research Hypothesis
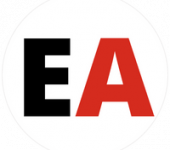
The story of a research study begins by asking a question. Researchers all around the globe are asking curious questions and formulating research hypothesis. However, whether the research study provides an effective conclusion depends on how well one develops a good research hypothesis. Research hypothesis examples could help researchers get an idea as to how to write a good research hypothesis.
This blog will help you understand what is a research hypothesis, its characteristics and, how to formulate a research hypothesis
Table of Contents
What is Hypothesis?
Hypothesis is an assumption or an idea proposed for the sake of argument so that it can be tested. It is a precise, testable statement of what the researchers predict will be outcome of the study. Hypothesis usually involves proposing a relationship between two variables: the independent variable (what the researchers change) and the dependent variable (what the research measures).
What is a Research Hypothesis?
Research hypothesis is a statement that introduces a research question and proposes an expected result. It is an integral part of the scientific method that forms the basis of scientific experiments. Therefore, you need to be careful and thorough when building your research hypothesis. A minor flaw in the construction of your hypothesis could have an adverse effect on your experiment. In research, there is a convention that the hypothesis is written in two forms, the null hypothesis, and the alternative hypothesis (called the experimental hypothesis when the method of investigation is an experiment).
Characteristics of a Good Research Hypothesis
As the hypothesis is specific, there is a testable prediction about what you expect to happen in a study. You may consider drawing hypothesis from previously published research based on the theory.
A good research hypothesis involves more effort than just a guess. In particular, your hypothesis may begin with a question that could be further explored through background research.
To help you formulate a promising research hypothesis, you should ask yourself the following questions:
- Is the language clear and focused?
- What is the relationship between your hypothesis and your research topic?
- Is your hypothesis testable? If yes, then how?
- What are the possible explanations that you might want to explore?
- Does your hypothesis include both an independent and dependent variable?
- Can you manipulate your variables without hampering the ethical standards?
- Does your research predict the relationship and outcome?
- Is your research simple and concise (avoids wordiness)?
- Is it clear with no ambiguity or assumptions about the readers’ knowledge
- Is your research observable and testable results?
- Is it relevant and specific to the research question or problem?
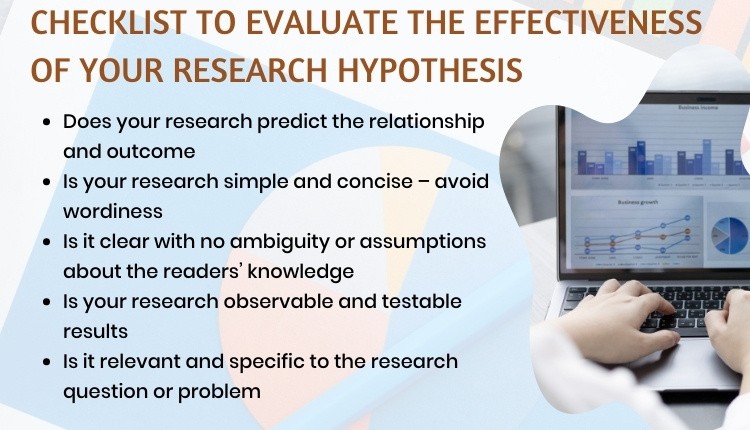
The questions listed above can be used as a checklist to make sure your hypothesis is based on a solid foundation. Furthermore, it can help you identify weaknesses in your hypothesis and revise it if necessary.
Source: Educational Hub
How to formulate a research hypothesis.
A testable hypothesis is not a simple statement. It is rather an intricate statement that needs to offer a clear introduction to a scientific experiment, its intentions, and the possible outcomes. However, there are some important things to consider when building a compelling hypothesis.
1. State the problem that you are trying to solve.
Make sure that the hypothesis clearly defines the topic and the focus of the experiment.
2. Try to write the hypothesis as an if-then statement.
Follow this template: If a specific action is taken, then a certain outcome is expected.
3. Define the variables
Independent variables are the ones that are manipulated, controlled, or changed. Independent variables are isolated from other factors of the study.
Dependent variables , as the name suggests are dependent on other factors of the study. They are influenced by the change in independent variable.
4. Scrutinize the hypothesis
Evaluate assumptions, predictions, and evidence rigorously to refine your understanding.
Types of Research Hypothesis
The types of research hypothesis are stated below:
1. Simple Hypothesis
It predicts the relationship between a single dependent variable and a single independent variable.
2. Complex Hypothesis
It predicts the relationship between two or more independent and dependent variables.
3. Directional Hypothesis
It specifies the expected direction to be followed to determine the relationship between variables and is derived from theory. Furthermore, it implies the researcher’s intellectual commitment to a particular outcome.
4. Non-directional Hypothesis
It does not predict the exact direction or nature of the relationship between the two variables. The non-directional hypothesis is used when there is no theory involved or when findings contradict previous research.
5. Associative and Causal Hypothesis
The associative hypothesis defines interdependency between variables. A change in one variable results in the change of the other variable. On the other hand, the causal hypothesis proposes an effect on the dependent due to manipulation of the independent variable.
6. Null Hypothesis
Null hypothesis states a negative statement to support the researcher’s findings that there is no relationship between two variables. There will be no changes in the dependent variable due the manipulation of the independent variable. Furthermore, it states results are due to chance and are not significant in terms of supporting the idea being investigated.
7. Alternative Hypothesis
It states that there is a relationship between the two variables of the study and that the results are significant to the research topic. An experimental hypothesis predicts what changes will take place in the dependent variable when the independent variable is manipulated. Also, it states that the results are not due to chance and that they are significant in terms of supporting the theory being investigated.
Research Hypothesis Examples of Independent and Dependent Variables
Research Hypothesis Example 1 The greater number of coal plants in a region (independent variable) increases water pollution (dependent variable). If you change the independent variable (building more coal factories), it will change the dependent variable (amount of water pollution).
Research Hypothesis Example 2 What is the effect of diet or regular soda (independent variable) on blood sugar levels (dependent variable)? If you change the independent variable (the type of soda you consume), it will change the dependent variable (blood sugar levels)
You should not ignore the importance of the above steps. The validity of your experiment and its results rely on a robust testable hypothesis. Developing a strong testable hypothesis has few advantages, it compels us to think intensely and specifically about the outcomes of a study. Consequently, it enables us to understand the implication of the question and the different variables involved in the study. Furthermore, it helps us to make precise predictions based on prior research. Hence, forming a hypothesis would be of great value to the research. Here are some good examples of testable hypotheses.
More importantly, you need to build a robust testable research hypothesis for your scientific experiments. A testable hypothesis is a hypothesis that can be proved or disproved as a result of experimentation.
Importance of a Testable Hypothesis
To devise and perform an experiment using scientific method, you need to make sure that your hypothesis is testable. To be considered testable, some essential criteria must be met:
- There must be a possibility to prove that the hypothesis is true.
- There must be a possibility to prove that the hypothesis is false.
- The results of the hypothesis must be reproducible.
Without these criteria, the hypothesis and the results will be vague. As a result, the experiment will not prove or disprove anything significant.
What are your experiences with building hypotheses for scientific experiments? What challenges did you face? How did you overcome these challenges? Please share your thoughts with us in the comments section.
Frequently Asked Questions
The steps to write a research hypothesis are: 1. Stating the problem: Ensure that the hypothesis defines the research problem 2. Writing a hypothesis as an 'if-then' statement: Include the action and the expected outcome of your study by following a ‘if-then’ structure. 3. Defining the variables: Define the variables as Dependent or Independent based on their dependency to other factors. 4. Scrutinizing the hypothesis: Identify the type of your hypothesis
Hypothesis testing is a statistical tool which is used to make inferences about a population data to draw conclusions for a particular hypothesis.
Hypothesis in statistics is a formal statement about the nature of a population within a structured framework of a statistical model. It is used to test an existing hypothesis by studying a population.
Research hypothesis is a statement that introduces a research question and proposes an expected result. It forms the basis of scientific experiments.
The different types of hypothesis in research are: • Null hypothesis: Null hypothesis is a negative statement to support the researcher’s findings that there is no relationship between two variables. • Alternate hypothesis: Alternate hypothesis predicts the relationship between the two variables of the study. • Directional hypothesis: Directional hypothesis specifies the expected direction to be followed to determine the relationship between variables. • Non-directional hypothesis: Non-directional hypothesis does not predict the exact direction or nature of the relationship between the two variables. • Simple hypothesis: Simple hypothesis predicts the relationship between a single dependent variable and a single independent variable. • Complex hypothesis: Complex hypothesis predicts the relationship between two or more independent and dependent variables. • Associative and casual hypothesis: Associative and casual hypothesis predicts the relationship between two or more independent and dependent variables. • Empirical hypothesis: Empirical hypothesis can be tested via experiments and observation. • Statistical hypothesis: A statistical hypothesis utilizes statistical models to draw conclusions about broader populations.

Wow! You really simplified your explanation that even dummies would find it easy to comprehend. Thank you so much.
Thanks a lot for your valuable guidance.
I enjoy reading the post. Hypotheses are actually an intrinsic part in a study. It bridges the research question and the methodology of the study.
Useful piece!
This is awesome.Wow.
It very interesting to read the topic, can you guide me any specific example of hypothesis process establish throw the Demand and supply of the specific product in market
Nicely explained
It is really a useful for me Kindly give some examples of hypothesis
It was a well explained content ,can you please give me an example with the null and alternative hypothesis illustrated
clear and concise. thanks.
So Good so Amazing
Good to learn
Thanks a lot for explaining to my level of understanding
Explained well and in simple terms. Quick read! Thank you
It awesome. It has really positioned me in my research project
Rate this article Cancel Reply
Your email address will not be published.
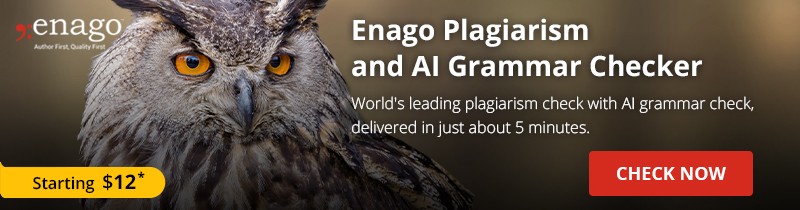
Enago Academy's Most Popular Articles
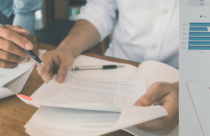
- Reporting Research
Choosing the Right Analytical Approach: Thematic analysis vs. content analysis for data interpretation
In research, choosing the right approach to understand data is crucial for deriving meaningful insights.…
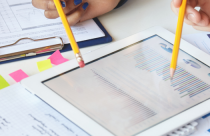
Comparing Cross Sectional and Longitudinal Studies: 5 steps for choosing the right approach
The process of choosing the right research design can put ourselves at the crossroads of…
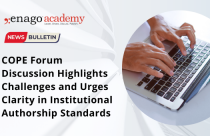
- Industry News
COPE Forum Discussion Highlights Challenges and Urges Clarity in Institutional Authorship Standards
The COPE forum discussion held in December 2023 initiated with a fundamental question — is…
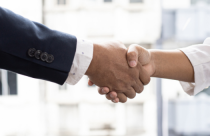
- Career Corner
Unlocking the Power of Networking in Academic Conferences
Embarking on your first academic conference experience? Fear not, we got you covered! Academic conferences…
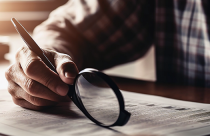
Research Recommendations – Guiding policy-makers for evidence-based decision making
Research recommendations play a crucial role in guiding scholars and researchers toward fruitful avenues of…
Choosing the Right Analytical Approach: Thematic analysis vs. content analysis for…
Comparing Cross Sectional and Longitudinal Studies: 5 steps for choosing the right…
How to Design Effective Research Questionnaires for Robust Findings

Sign-up to read more
Subscribe for free to get unrestricted access to all our resources on research writing and academic publishing including:
- 2000+ blog articles
- 50+ Webinars
- 10+ Expert podcasts
- 50+ Infographics
- 10+ Checklists
- Research Guides
We hate spam too. We promise to protect your privacy and never spam you.
I am looking for Editing/ Proofreading services for my manuscript Tentative date of next journal submission:
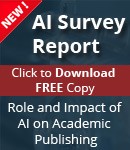
What should universities' stance be on AI tools in research and academic writing?
- Bipolar Disorder
- Therapy Center
- When To See a Therapist
- Types of Therapy
- Best Online Therapy
- Best Couples Therapy
- Best Family Therapy
- Managing Stress
- Sleep and Dreaming
- Understanding Emotions
- Self-Improvement
- Healthy Relationships
- Student Resources
- Personality Types
- Guided Meditations
- Verywell Mind Insights
- 2023 Verywell Mind 25
- Mental Health in the Classroom
- Editorial Process
- Meet Our Review Board
- Crisis Support
How to Write a Great Hypothesis
Hypothesis Definition, Format, Examples, and Tips
Kendra Cherry, MS, is a psychosocial rehabilitation specialist, psychology educator, and author of the "Everything Psychology Book."
:max_bytes(150000):strip_icc():format(webp)/IMG_9791-89504ab694d54b66bbd72cb84ffb860e.jpg)
Amy Morin, LCSW, is a psychotherapist and international bestselling author. Her books, including "13 Things Mentally Strong People Don't Do," have been translated into more than 40 languages. Her TEDx talk, "The Secret of Becoming Mentally Strong," is one of the most viewed talks of all time.
:max_bytes(150000):strip_icc():format(webp)/VW-MIND-Amy-2b338105f1ee493f94d7e333e410fa76.jpg)
Verywell / Alex Dos Diaz
- The Scientific Method
Hypothesis Format
Falsifiability of a hypothesis.
- Operationalization
Hypothesis Types
Hypotheses examples.
- Collecting Data
A hypothesis is a tentative statement about the relationship between two or more variables. It is a specific, testable prediction about what you expect to happen in a study. It is a preliminary answer to your question that helps guide the research process.
Consider a study designed to examine the relationship between sleep deprivation and test performance. The hypothesis might be: "This study is designed to assess the hypothesis that sleep-deprived people will perform worse on a test than individuals who are not sleep-deprived."
At a Glance
A hypothesis is crucial to scientific research because it offers a clear direction for what the researchers are looking to find. This allows them to design experiments to test their predictions and add to our scientific knowledge about the world. This article explores how a hypothesis is used in psychology research, how to write a good hypothesis, and the different types of hypotheses you might use.
The Hypothesis in the Scientific Method
In the scientific method , whether it involves research in psychology, biology, or some other area, a hypothesis represents what the researchers think will happen in an experiment. The scientific method involves the following steps:
- Forming a question
- Performing background research
- Creating a hypothesis
- Designing an experiment
- Collecting data
- Analyzing the results
- Drawing conclusions
- Communicating the results
The hypothesis is a prediction, but it involves more than a guess. Most of the time, the hypothesis begins with a question which is then explored through background research. At this point, researchers then begin to develop a testable hypothesis.
Unless you are creating an exploratory study, your hypothesis should always explain what you expect to happen.
In a study exploring the effects of a particular drug, the hypothesis might be that researchers expect the drug to have some type of effect on the symptoms of a specific illness. In psychology, the hypothesis might focus on how a certain aspect of the environment might influence a particular behavior.
Remember, a hypothesis does not have to be correct. While the hypothesis predicts what the researchers expect to see, the goal of the research is to determine whether this guess is right or wrong. When conducting an experiment, researchers might explore numerous factors to determine which ones might contribute to the ultimate outcome.
In many cases, researchers may find that the results of an experiment do not support the original hypothesis. When writing up these results, the researchers might suggest other options that should be explored in future studies.
In many cases, researchers might draw a hypothesis from a specific theory or build on previous research. For example, prior research has shown that stress can impact the immune system. So a researcher might hypothesize: "People with high-stress levels will be more likely to contract a common cold after being exposed to the virus than people who have low-stress levels."
In other instances, researchers might look at commonly held beliefs or folk wisdom. "Birds of a feather flock together" is one example of folk adage that a psychologist might try to investigate. The researcher might pose a specific hypothesis that "People tend to select romantic partners who are similar to them in interests and educational level."
Elements of a Good Hypothesis
So how do you write a good hypothesis? When trying to come up with a hypothesis for your research or experiments, ask yourself the following questions:
- Is your hypothesis based on your research on a topic?
- Can your hypothesis be tested?
- Does your hypothesis include independent and dependent variables?
Before you come up with a specific hypothesis, spend some time doing background research. Once you have completed a literature review, start thinking about potential questions you still have. Pay attention to the discussion section in the journal articles you read . Many authors will suggest questions that still need to be explored.
How to Formulate a Good Hypothesis
To form a hypothesis, you should take these steps:
- Collect as many observations about a topic or problem as you can.
- Evaluate these observations and look for possible causes of the problem.
- Create a list of possible explanations that you might want to explore.
- After you have developed some possible hypotheses, think of ways that you could confirm or disprove each hypothesis through experimentation. This is known as falsifiability.
In the scientific method , falsifiability is an important part of any valid hypothesis. In order to test a claim scientifically, it must be possible that the claim could be proven false.
Students sometimes confuse the idea of falsifiability with the idea that it means that something is false, which is not the case. What falsifiability means is that if something was false, then it is possible to demonstrate that it is false.
One of the hallmarks of pseudoscience is that it makes claims that cannot be refuted or proven false.
The Importance of Operational Definitions
A variable is a factor or element that can be changed and manipulated in ways that are observable and measurable. However, the researcher must also define how the variable will be manipulated and measured in the study.
Operational definitions are specific definitions for all relevant factors in a study. This process helps make vague or ambiguous concepts detailed and measurable.
For example, a researcher might operationally define the variable " test anxiety " as the results of a self-report measure of anxiety experienced during an exam. A "study habits" variable might be defined by the amount of studying that actually occurs as measured by time.
These precise descriptions are important because many things can be measured in various ways. Clearly defining these variables and how they are measured helps ensure that other researchers can replicate your results.
Replicability
One of the basic principles of any type of scientific research is that the results must be replicable.
Replication means repeating an experiment in the same way to produce the same results. By clearly detailing the specifics of how the variables were measured and manipulated, other researchers can better understand the results and repeat the study if needed.
Some variables are more difficult than others to define. For example, how would you operationally define a variable such as aggression ? For obvious ethical reasons, researchers cannot create a situation in which a person behaves aggressively toward others.
To measure this variable, the researcher must devise a measurement that assesses aggressive behavior without harming others. The researcher might utilize a simulated task to measure aggressiveness in this situation.
Hypothesis Checklist
- Does your hypothesis focus on something that you can actually test?
- Does your hypothesis include both an independent and dependent variable?
- Can you manipulate the variables?
- Can your hypothesis be tested without violating ethical standards?
The hypothesis you use will depend on what you are investigating and hoping to find. Some of the main types of hypotheses that you might use include:
- Simple hypothesis : This type of hypothesis suggests there is a relationship between one independent variable and one dependent variable.
- Complex hypothesis : This type suggests a relationship between three or more variables, such as two independent and dependent variables.
- Null hypothesis : This hypothesis suggests no relationship exists between two or more variables.
- Alternative hypothesis : This hypothesis states the opposite of the null hypothesis.
- Statistical hypothesis : This hypothesis uses statistical analysis to evaluate a representative population sample and then generalizes the findings to the larger group.
- Logical hypothesis : This hypothesis assumes a relationship between variables without collecting data or evidence.
A hypothesis often follows a basic format of "If {this happens} then {this will happen}." One way to structure your hypothesis is to describe what will happen to the dependent variable if you change the independent variable .
The basic format might be: "If {these changes are made to a certain independent variable}, then we will observe {a change in a specific dependent variable}."
A few examples of simple hypotheses:
- "Students who eat breakfast will perform better on a math exam than students who do not eat breakfast."
- "Students who experience test anxiety before an English exam will get lower scores than students who do not experience test anxiety."
- "Motorists who talk on the phone while driving will be more likely to make errors on a driving course than those who do not talk on the phone."
- "Children who receive a new reading intervention will have higher reading scores than students who do not receive the intervention."
Examples of a complex hypothesis include:
- "People with high-sugar diets and sedentary activity levels are more likely to develop depression."
- "Younger people who are regularly exposed to green, outdoor areas have better subjective well-being than older adults who have limited exposure to green spaces."
Examples of a null hypothesis include:
- "There is no difference in anxiety levels between people who take St. John's wort supplements and those who do not."
- "There is no difference in scores on a memory recall task between children and adults."
- "There is no difference in aggression levels between children who play first-person shooter games and those who do not."
Examples of an alternative hypothesis:
- "People who take St. John's wort supplements will have less anxiety than those who do not."
- "Adults will perform better on a memory task than children."
- "Children who play first-person shooter games will show higher levels of aggression than children who do not."
Collecting Data on Your Hypothesis
Once a researcher has formed a testable hypothesis, the next step is to select a research design and start collecting data. The research method depends largely on exactly what they are studying. There are two basic types of research methods: descriptive research and experimental research.
Descriptive Research Methods
Descriptive research such as case studies , naturalistic observations , and surveys are often used when conducting an experiment is difficult or impossible. These methods are best used to describe different aspects of a behavior or psychological phenomenon.
Once a researcher has collected data using descriptive methods, a correlational study can examine how the variables are related. This research method might be used to investigate a hypothesis that is difficult to test experimentally.
Experimental Research Methods
Experimental methods are used to demonstrate causal relationships between variables. In an experiment, the researcher systematically manipulates a variable of interest (known as the independent variable) and measures the effect on another variable (known as the dependent variable).
Unlike correlational studies, which can only be used to determine if there is a relationship between two variables, experimental methods can be used to determine the actual nature of the relationship—whether changes in one variable actually cause another to change.
The hypothesis is a critical part of any scientific exploration. It represents what researchers expect to find in a study or experiment. In situations where the hypothesis is unsupported by the research, the research still has value. Such research helps us better understand how different aspects of the natural world relate to one another. It also helps us develop new hypotheses that can then be tested in the future.
Thompson WH, Skau S. On the scope of scientific hypotheses . R Soc Open Sci . 2023;10(8):230607. doi:10.1098/rsos.230607
Taran S, Adhikari NKJ, Fan E. Falsifiability in medicine: what clinicians can learn from Karl Popper [published correction appears in Intensive Care Med. 2021 Jun 17;:]. Intensive Care Med . 2021;47(9):1054-1056. doi:10.1007/s00134-021-06432-z
Eyler AA. Research Methods for Public Health . 1st ed. Springer Publishing Company; 2020. doi:10.1891/9780826182067.0004
Nosek BA, Errington TM. What is replication ? PLoS Biol . 2020;18(3):e3000691. doi:10.1371/journal.pbio.3000691
Aggarwal R, Ranganathan P. Study designs: Part 2 - Descriptive studies . Perspect Clin Res . 2019;10(1):34-36. doi:10.4103/picr.PICR_154_18
Nevid J. Psychology: Concepts and Applications. Wadworth, 2013.
By Kendra Cherry, MSEd Kendra Cherry, MS, is a psychosocial rehabilitation specialist, psychology educator, and author of the "Everything Psychology Book."
- Privacy Policy
Buy Me a Coffee

Home » What is a Hypothesis – Types, Examples and Writing Guide
What is a Hypothesis – Types, Examples and Writing Guide
Table of Contents

Definition:
Hypothesis is an educated guess or proposed explanation for a phenomenon, based on some initial observations or data. It is a tentative statement that can be tested and potentially proven or disproven through further investigation and experimentation.
Hypothesis is often used in scientific research to guide the design of experiments and the collection and analysis of data. It is an essential element of the scientific method, as it allows researchers to make predictions about the outcome of their experiments and to test those predictions to determine their accuracy.
Types of Hypothesis
Types of Hypothesis are as follows:
Research Hypothesis
A research hypothesis is a statement that predicts a relationship between variables. It is usually formulated as a specific statement that can be tested through research, and it is often used in scientific research to guide the design of experiments.
Null Hypothesis
The null hypothesis is a statement that assumes there is no significant difference or relationship between variables. It is often used as a starting point for testing the research hypothesis, and if the results of the study reject the null hypothesis, it suggests that there is a significant difference or relationship between variables.
Alternative Hypothesis
An alternative hypothesis is a statement that assumes there is a significant difference or relationship between variables. It is often used as an alternative to the null hypothesis and is tested against the null hypothesis to determine which statement is more accurate.
Directional Hypothesis
A directional hypothesis is a statement that predicts the direction of the relationship between variables. For example, a researcher might predict that increasing the amount of exercise will result in a decrease in body weight.
Non-directional Hypothesis
A non-directional hypothesis is a statement that predicts the relationship between variables but does not specify the direction. For example, a researcher might predict that there is a relationship between the amount of exercise and body weight, but they do not specify whether increasing or decreasing exercise will affect body weight.
Statistical Hypothesis
A statistical hypothesis is a statement that assumes a particular statistical model or distribution for the data. It is often used in statistical analysis to test the significance of a particular result.
Composite Hypothesis
A composite hypothesis is a statement that assumes more than one condition or outcome. It can be divided into several sub-hypotheses, each of which represents a different possible outcome.
Empirical Hypothesis
An empirical hypothesis is a statement that is based on observed phenomena or data. It is often used in scientific research to develop theories or models that explain the observed phenomena.
Simple Hypothesis
A simple hypothesis is a statement that assumes only one outcome or condition. It is often used in scientific research to test a single variable or factor.
Complex Hypothesis
A complex hypothesis is a statement that assumes multiple outcomes or conditions. It is often used in scientific research to test the effects of multiple variables or factors on a particular outcome.
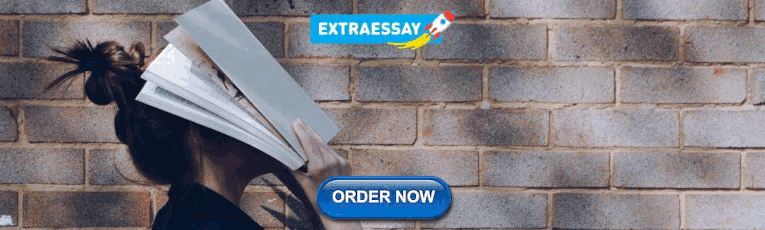
Applications of Hypothesis
Hypotheses are used in various fields to guide research and make predictions about the outcomes of experiments or observations. Here are some examples of how hypotheses are applied in different fields:
- Science : In scientific research, hypotheses are used to test the validity of theories and models that explain natural phenomena. For example, a hypothesis might be formulated to test the effects of a particular variable on a natural system, such as the effects of climate change on an ecosystem.
- Medicine : In medical research, hypotheses are used to test the effectiveness of treatments and therapies for specific conditions. For example, a hypothesis might be formulated to test the effects of a new drug on a particular disease.
- Psychology : In psychology, hypotheses are used to test theories and models of human behavior and cognition. For example, a hypothesis might be formulated to test the effects of a particular stimulus on the brain or behavior.
- Sociology : In sociology, hypotheses are used to test theories and models of social phenomena, such as the effects of social structures or institutions on human behavior. For example, a hypothesis might be formulated to test the effects of income inequality on crime rates.
- Business : In business research, hypotheses are used to test the validity of theories and models that explain business phenomena, such as consumer behavior or market trends. For example, a hypothesis might be formulated to test the effects of a new marketing campaign on consumer buying behavior.
- Engineering : In engineering, hypotheses are used to test the effectiveness of new technologies or designs. For example, a hypothesis might be formulated to test the efficiency of a new solar panel design.
How to write a Hypothesis
Here are the steps to follow when writing a hypothesis:
Identify the Research Question
The first step is to identify the research question that you want to answer through your study. This question should be clear, specific, and focused. It should be something that can be investigated empirically and that has some relevance or significance in the field.
Conduct a Literature Review
Before writing your hypothesis, it’s essential to conduct a thorough literature review to understand what is already known about the topic. This will help you to identify the research gap and formulate a hypothesis that builds on existing knowledge.
Determine the Variables
The next step is to identify the variables involved in the research question. A variable is any characteristic or factor that can vary or change. There are two types of variables: independent and dependent. The independent variable is the one that is manipulated or changed by the researcher, while the dependent variable is the one that is measured or observed as a result of the independent variable.
Formulate the Hypothesis
Based on the research question and the variables involved, you can now formulate your hypothesis. A hypothesis should be a clear and concise statement that predicts the relationship between the variables. It should be testable through empirical research and based on existing theory or evidence.
Write the Null Hypothesis
The null hypothesis is the opposite of the alternative hypothesis, which is the hypothesis that you are testing. The null hypothesis states that there is no significant difference or relationship between the variables. It is important to write the null hypothesis because it allows you to compare your results with what would be expected by chance.
Refine the Hypothesis
After formulating the hypothesis, it’s important to refine it and make it more precise. This may involve clarifying the variables, specifying the direction of the relationship, or making the hypothesis more testable.
Examples of Hypothesis
Here are a few examples of hypotheses in different fields:
- Psychology : “Increased exposure to violent video games leads to increased aggressive behavior in adolescents.”
- Biology : “Higher levels of carbon dioxide in the atmosphere will lead to increased plant growth.”
- Sociology : “Individuals who grow up in households with higher socioeconomic status will have higher levels of education and income as adults.”
- Education : “Implementing a new teaching method will result in higher student achievement scores.”
- Marketing : “Customers who receive a personalized email will be more likely to make a purchase than those who receive a generic email.”
- Physics : “An increase in temperature will cause an increase in the volume of a gas, assuming all other variables remain constant.”
- Medicine : “Consuming a diet high in saturated fats will increase the risk of developing heart disease.”
Purpose of Hypothesis
The purpose of a hypothesis is to provide a testable explanation for an observed phenomenon or a prediction of a future outcome based on existing knowledge or theories. A hypothesis is an essential part of the scientific method and helps to guide the research process by providing a clear focus for investigation. It enables scientists to design experiments or studies to gather evidence and data that can support or refute the proposed explanation or prediction.
The formulation of a hypothesis is based on existing knowledge, observations, and theories, and it should be specific, testable, and falsifiable. A specific hypothesis helps to define the research question, which is important in the research process as it guides the selection of an appropriate research design and methodology. Testability of the hypothesis means that it can be proven or disproven through empirical data collection and analysis. Falsifiability means that the hypothesis should be formulated in such a way that it can be proven wrong if it is incorrect.
In addition to guiding the research process, the testing of hypotheses can lead to new discoveries and advancements in scientific knowledge. When a hypothesis is supported by the data, it can be used to develop new theories or models to explain the observed phenomenon. When a hypothesis is not supported by the data, it can help to refine existing theories or prompt the development of new hypotheses to explain the phenomenon.
When to use Hypothesis
Here are some common situations in which hypotheses are used:
- In scientific research , hypotheses are used to guide the design of experiments and to help researchers make predictions about the outcomes of those experiments.
- In social science research , hypotheses are used to test theories about human behavior, social relationships, and other phenomena.
- I n business , hypotheses can be used to guide decisions about marketing, product development, and other areas. For example, a hypothesis might be that a new product will sell well in a particular market, and this hypothesis can be tested through market research.
Characteristics of Hypothesis
Here are some common characteristics of a hypothesis:
- Testable : A hypothesis must be able to be tested through observation or experimentation. This means that it must be possible to collect data that will either support or refute the hypothesis.
- Falsifiable : A hypothesis must be able to be proven false if it is not supported by the data. If a hypothesis cannot be falsified, then it is not a scientific hypothesis.
- Clear and concise : A hypothesis should be stated in a clear and concise manner so that it can be easily understood and tested.
- Based on existing knowledge : A hypothesis should be based on existing knowledge and research in the field. It should not be based on personal beliefs or opinions.
- Specific : A hypothesis should be specific in terms of the variables being tested and the predicted outcome. This will help to ensure that the research is focused and well-designed.
- Tentative: A hypothesis is a tentative statement or assumption that requires further testing and evidence to be confirmed or refuted. It is not a final conclusion or assertion.
- Relevant : A hypothesis should be relevant to the research question or problem being studied. It should address a gap in knowledge or provide a new perspective on the issue.
Advantages of Hypothesis
Hypotheses have several advantages in scientific research and experimentation:
- Guides research: A hypothesis provides a clear and specific direction for research. It helps to focus the research question, select appropriate methods and variables, and interpret the results.
- Predictive powe r: A hypothesis makes predictions about the outcome of research, which can be tested through experimentation. This allows researchers to evaluate the validity of the hypothesis and make new discoveries.
- Facilitates communication: A hypothesis provides a common language and framework for scientists to communicate with one another about their research. This helps to facilitate the exchange of ideas and promotes collaboration.
- Efficient use of resources: A hypothesis helps researchers to use their time, resources, and funding efficiently by directing them towards specific research questions and methods that are most likely to yield results.
- Provides a basis for further research: A hypothesis that is supported by data provides a basis for further research and exploration. It can lead to new hypotheses, theories, and discoveries.
- Increases objectivity: A hypothesis can help to increase objectivity in research by providing a clear and specific framework for testing and interpreting results. This can reduce bias and increase the reliability of research findings.
Limitations of Hypothesis
Some Limitations of the Hypothesis are as follows:
- Limited to observable phenomena: Hypotheses are limited to observable phenomena and cannot account for unobservable or intangible factors. This means that some research questions may not be amenable to hypothesis testing.
- May be inaccurate or incomplete: Hypotheses are based on existing knowledge and research, which may be incomplete or inaccurate. This can lead to flawed hypotheses and erroneous conclusions.
- May be biased: Hypotheses may be biased by the researcher’s own beliefs, values, or assumptions. This can lead to selective interpretation of data and a lack of objectivity in research.
- Cannot prove causation: A hypothesis can only show a correlation between variables, but it cannot prove causation. This requires further experimentation and analysis.
- Limited to specific contexts: Hypotheses are limited to specific contexts and may not be generalizable to other situations or populations. This means that results may not be applicable in other contexts or may require further testing.
- May be affected by chance : Hypotheses may be affected by chance or random variation, which can obscure or distort the true relationship between variables.
About the author
Muhammad Hassan
Researcher, Academic Writer, Web developer
You may also like

Data Collection – Methods Types and Examples

Delimitations in Research – Types, Examples and...

Research Process – Steps, Examples and Tips

Research Design – Types, Methods and Examples

Institutional Review Board – Application Sample...

Evaluating Research – Process, Examples and...
Learn How To Write A Hypothesis For Your Next Research Project!

Undoubtedly, research plays a crucial role in substantiating or refuting our assumptions. These assumptions act as potential answers to our questions. Such assumptions, also known as hypotheses, are considered key aspects of research. In this blog, we delve into the significance of hypotheses. And provide insights on how to write them effectively. So, let’s dive in and explore the art of writing hypotheses together.
Table of Contents
What is a Hypothesis?
A hypothesis is a crucial starting point in scientific research. It is an educated guess about the relationship between two or more variables. In other words, a hypothesis acts as a foundation for a researcher to build their study.
Here are some examples of well-crafted hypotheses:
- Increased exposure to natural sunlight improves sleep quality in adults.
A positive relationship between natural sunlight exposure and sleep quality in adult individuals.
- Playing puzzle games on a regular basis enhances problem-solving abilities in children.
Engaging in frequent puzzle gameplay leads to improved problem-solving skills in children.
- Students and improved learning hecks.
S tudents using online paper writing service platforms (as a learning tool for receiving personalized feedback and guidance) will demonstrate improved writing skills. (compared to those who do not utilize such platforms).
- The use of APA format in research papers.
Using the APA format helps students stay organized when writing research papers. Organized students can focus better on their topics and, as a result, produce better quality work.
The Building Blocks of a Hypothesis
To better understand the concept of a hypothesis, let’s break it down into its basic components:
- Variables . A hypothesis involves at least two variables. An independent variable and a dependent variable. The independent variable is the one being changed or manipulated, while the dependent variable is the one being measured or observed.
- Relationship : A hypothesis proposes a relationship or connection between the variables. This could be a cause-and-effect relationship or a correlation between them.
- Testability : A hypothesis should be testable and falsifiable, meaning it can be proven right or wrong through experimentation or observation.
Types of Hypotheses
When learning how to write a hypothesis, it’s essential to understand its main types. These include; alternative hypotheses and null hypotheses. In the following section, we explore both types of hypotheses with examples.
Alternative Hypothesis (H1)
This kind of hypothesis suggests a relationship or effect between the variables. It is the main focus of the study. The researcher wants to either prove or disprove it. Many research divides this hypothesis into two subsections:
- Directional
This type of H1 predicts a specific outcome. Many researchers use this hypothesis to explore the relationship between variables rather than the groups.
- Non-directional
You can take a guess from the name. This type of H1 does not provide a specific prediction for the research outcome.
Here are some examples for your better understanding of how to write a hypothesis.
- Consuming caffeine improves cognitive performance. (This hypothesis predicts that there is a positive relationship between caffeine consumption and cognitive performance.)
- Aerobic exercise leads to reduced blood pressure. (This hypothesis suggests that engaging in aerobic exercise results in lower blood pressure readings.)
- Exposure to nature reduces stress levels among employees. (Here, the hypothesis proposes that employees exposed to natural environments will experience decreased stress levels.)
- Listening to classical music while studying increases memory retention. (This hypothesis speculates that studying with classical music playing in the background boosts students’ ability to retain information.)
- Early literacy intervention improves reading skills in children. (This hypothesis claims that providing early literacy assistance to children results in enhanced reading abilities.)
- Time management in nursing students. ( Students who use a nursing research paper writing service have more time to focus on their studies and can achieve better grades in other subjects. )
Null Hypothesis (H0)
A null hypothesis assumes no relationship or effect between the variables. If the alternative hypothesis is proven to be false, the null hypothesis is considered to be true. Usually a null hypothesis shows no direct correlation between the defined variables.
Here are some of the examples
- The consumption of herbal tea has no effect on sleep quality. (This hypothesis assumes that herbal tea consumption does not impact the quality of sleep.)
- The number of hours spent playing video games is unrelated to academic performance. (Here, the null hypothesis suggests that no relationship exists between video gameplay duration and academic achievement.)
- Implementing flexible work schedules has no influence on employee job satisfaction. (This hypothesis contends that providing flexible schedules does not affect how satisfied employees are with their jobs.)
- Writing ability of a 7th grader is not affected by reading editorial example. ( There is no relationship between reading an editorial example and improving a 7th grader’s writing abilities.)
- The type of lighting in a room does not affect people’s mood. (In this null hypothesis, there is no connection between the kind of lighting in a room and the mood of those present.)
- The use of social media during break time does not impact productivity at work. (This hypothesis proposes that social media usage during breaks has no effect on work productivity.)
As you learn how to write a hypothesis, remember that aiming for clarity, testability, and relevance to your research question is vital. By mastering this skill, you’re well on your way to conducting impactful scientific research. Good luck!
Importance of a Hypothesis in Research
A well-structured hypothesis is a vital part of any research project for several reasons:
- It provides clear direction for the study by setting its focus and purpose.
- It outlines expectations of the research, making it easier to measure results.
- It helps identify any potential limitations in the study, allowing researchers to refine their approach.
In conclusion, a hypothesis plays a fundamental role in the research process. By understanding its concept and constructing a well-thought-out hypothesis, researchers lay the groundwork for a successful, scientifically sound investigation.
How to Write a Hypothesis?
Here are five steps that you can follow to write an effective hypothesis.
Step 1: Identify Your Research Question
The first step in learning how to compose a hypothesis is to clearly define your research question. This question is the central focus of your study and will help you determine the direction of your hypothesis.
Step 2: Determine the Variables
When exploring how to write a hypothesis, it’s crucial to identify the variables involved in your study. You’ll need at least two variables:
- Independent variable : The factor you manipulate or change in your experiment.
- Dependent variable : The outcome or result you observe or measure, which is influenced by the independent variable.
Step 3: Build the Hypothetical Relationship
In understanding how to compose a hypothesis, constructing the relationship between the variables is key. Based on your research question and variables, predict the expected outcome or connection. This prediction should be specific, testable, and, if possible, expressed in the “If…then” format.
Step 4: Write the Null Hypothesis
When mastering how to write a hypothesis, it’s important to create a null hypothesis as well. The null hypothesis assumes no relationship or effect between the variables, acting as a counterpoint to your primary hypothesis.
Step 5: Review Your Hypothesis
Finally, when learning how to compose a hypothesis, it’s essential to review your hypothesis for clarity, testability, and relevance to your research question. Make any necessary adjustments to ensure it provides a solid basis for your study.
In conclusion, understanding how to write a hypothesis is crucial for conducting successful scientific research. By focusing on your research question and carefully building relationships between variables, you will lay a strong foundation for advancing research and knowledge in your field.
Hypothesis vs. Prediction: What’s the Difference?
Understanding the differences between a hypothesis and a prediction is crucial in scientific research. Often, these terms are used interchangeably, but they have distinct meanings and functions. This segment aims to clarify these differences and explain how to compose a hypothesis correctly, helping you improve the quality of your research projects.
Hypothesis: The Foundation of Your Research
A hypothesis is an educated guess about the relationship between two or more variables. It provides the basis for your research question and is a starting point for an experiment or observational study.
The critical elements for a hypothesis include:
- Specificity: A clear and concise statement that describes the relationship between variables.
- Testability: The ability to test the hypothesis through experimentation or observation.
To learn how to write a hypothesis, it’s essential to identify your research question first and then predict the relationship between the variables.
Prediction: The Expected Outcome
A prediction is a statement about a specific outcome you expect to see in your experiment or observational study. It’s derived from the hypothesis and provides a measurable way to test the relationship between variables.
Here’s an example of how to write a hypothesis and a related prediction:
- Hypothesis: Consuming a high-sugar diet leads to weight gain.
- Prediction: People who consume a high-sugar diet for six weeks will gain more weight than those who maintain a low-sugar diet during the same period.
Key Differences Between a Hypothesis and a Prediction
While a hypothesis and prediction are both essential components of scientific research, there are some key differences to keep in mind:
- A hypothesis is an educated guess that suggests a relationship between variables, while a prediction is a specific and measurable outcome based on that hypothesis.
- A hypothesis can give rise to multiple experiment or observational study predictions.
To conclude, understanding the differences between a hypothesis and a prediction, and learning how to write a hypothesis, are essential steps to form a robust foundation for your research. By creating clear, testable hypotheses along with specific, measurable predictions, you lay the groundwork for scientifically sound investigations.
Here’s a wrap-up for this guide on how to write a hypothesis. We’re confident this article was helpful for many of you. We understand that many students struggle with writing their school research . However, we hope to continue assisting you through our blog tutorial on writing different aspects of academic assignments.
For further information, you can check out our reverent blog or contact our professionals to avail amazing writing services. Paper perk experts tailor assignments to reflect your unique voice and perspectives. Our professionals make sure to stick around till your satisfaction. So what are you waiting for? Pick your required service and order away!
Order Original Papers & Essays
Your First Custom Paper Sample is on Us!

Timely Deliveries

No Plagiarism & AI

100% Refund
Try Our Free Paper Writing Service
Related blogs.

Connections with Writers and support
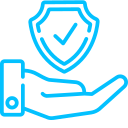
Privacy and Confidentiality Guarantee
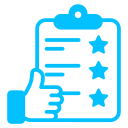
Average Quality Score
How Do You Formulate (Important) Hypotheses?
- Open Access
- First Online: 03 December 2022
Cite this chapter
You have full access to this open access chapter
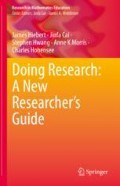
- James Hiebert 6 ,
- Jinfa Cai 7 ,
- Stephen Hwang 7 ,
- Anne K Morris 6 &
- Charles Hohensee 6
Part of the book series: Research in Mathematics Education ((RME))
11k Accesses
Building on the ideas in Chap. 1, we describe formulating, testing, and revising hypotheses as a continuing cycle of clarifying what you want to study, making predictions about what you might find together with developing your reasons for these predictions, imagining tests of these predictions, revising your predictions and rationales, and so on. Many resources feed this process, including reading what others have found about similar phenomena, talking with colleagues, conducting pilot studies, and writing drafts as you revise your thinking. Although you might think you cannot predict what you will find, it is always possible—with enough reading and conversations and pilot studies—to make some good guesses. And, once you guess what you will find and write out the reasons for these guesses you are on your way to scientific inquiry. As you refine your hypotheses, you can assess their research importance by asking how connected they are to problems your research community really wants to solve.
You have full access to this open access chapter, Download chapter PDF
Part I. Getting Started
We want to begin by addressing a question you might have had as you read the title of this chapter. You are likely to hear, or read in other sources, that the research process begins by asking research questions . For reasons we gave in Chap. 1 , and more we will describe in this and later chapters, we emphasize formulating, testing, and revising hypotheses. However, it is important to know that asking and answering research questions involve many of the same activities, so we are not describing a completely different process.
We acknowledge that many researchers do not actually begin by formulating hypotheses. In other words, researchers rarely get a researchable idea by writing out a well-formulated hypothesis. Instead, their initial ideas for what they study come from a variety of sources. Then, after they have the idea for a study, they do lots of background reading and thinking and talking before they are ready to formulate a hypothesis. So, for readers who are at the very beginning and do not yet have an idea for a study, let’s back up. Where do research ideas come from?
There are no formulas or algorithms that spawn a researchable idea. But as you begin the process, you can ask yourself some questions. Your answers to these questions can help you move forward.
What are you curious about? What are you passionate about? What have you wondered about as an educator? These are questions that look inward, questions about yourself.
What do you think are the most pressing educational problems? Which problems are you in the best position to address? What change(s) do you think would help all students learn more productively? These are questions that look outward, questions about phenomena you have observed.
What are the main areas of research in the field? What are the big questions that are being asked? These are questions about the general landscape of the field.
What have you read about in the research literature that caught your attention? What have you read that prompted you to think about extending the profession’s knowledge about this? What have you read that made you ask, “I wonder why this is true?” These are questions about how you can build on what is known in the field.
What are some research questions or testable hypotheses that have been identified by other researchers for future research? This, too, is a question about how you can build on what is known in the field. Taking up such questions or hypotheses can help by providing some existing scaffolding that others have constructed.
What research is being done by your immediate colleagues or your advisor that is of interest to you? These are questions about topics for which you will likely receive local support.
Exercise 2.1
Brainstorm some answers for each set of questions. Record them. Then step back and look at the places of intersection. Did you have similar answers across several questions? Write out, as clearly as you can, the topic that captures your primary interest, at least at this point. We will give you a chance to update your responses as you study this book.
Part II. Paths from a General Interest to an Informed Hypothesis
There are many different paths you might take from conceiving an idea for a study, maybe even a vague idea, to formulating a prediction that leads to an informed hypothesis that can be tested. We will explore some of the paths we recommend.
We will assume you have completed Exercise 2.1 in Part I and have some written answers to the six questions that preceded it as well as a statement that describes your topic of interest. This very first statement could take several different forms: a description of a problem you want to study, a question you want to address, or a hypothesis you want to test. We recommend that you begin with one of these three forms, the one that makes most sense to you. There is an advantage to using all three and flexibly choosing the one that is most meaningful at the time and for a particular study. You can then move from one to the other as you think more about your research study and you develop your initial idea. To get a sense of how the process might unfold, consider the following alternative paths.
Beginning with a Prediction If You Have One
Sometimes, when you notice an educational problem or have a question about an educational situation or phenomenon, you quickly have an idea that might help solve the problem or answer the question. Here are three examples.
You are a teacher, and you noticed a problem with the way the textbook presented two related concepts in two consecutive lessons. Almost as soon as you noticed the problem, it occurred to you that the two lessons could be taught more effectively in the reverse order. You predicted better outcomes if the order was reversed, and you even had a preliminary rationale for why this would be true.
You are a graduate student and you read that students often misunderstand a particular aspect of graphing linear functions. You predicted that, by listening to small groups of students working together, you could hear new details that would help you understand this misconception.
You are a curriculum supervisor and you observed sixth-grade classrooms where students were learning about decimal fractions. After talking with several experienced teachers, you predicted that beginning with percentages might be a good way to introduce students to decimal fractions.
We begin with the path of making predictions because we see the other two paths as leading into this one at some point in the process (see Fig. 2.1 ). Starting with this path does not mean you did not sense a problem you wanted to solve or a question you wanted to answer.
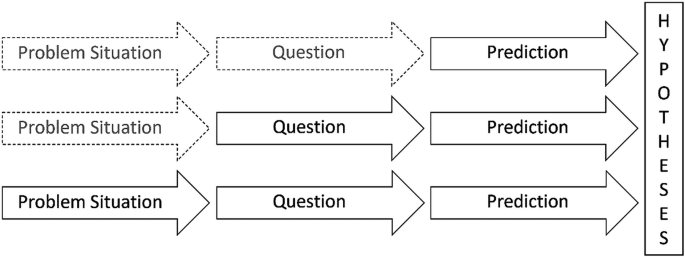
Three Pathways to Formulating Informed Hypotheses
Notice that your predictions can come from a variety of sources—your own experience, reading, and talking with colleagues. Most likely, as you write out your predictions you also think about the educational problem for which your prediction is a potential solution. Writing a clear description of the problem will be useful as you proceed. Notice also that it is easy to change each of your predictions into a question. When you formulate a prediction, you are actually answering a question, even though the question might be implicit. Making that implicit question explicit can generate a first draft of the research question that accompanies your prediction. For example, suppose you are the curriculum supervisor who predicts that teaching percentages first would be a good way to introduce decimal fractions. In an obvious shift in form, you could ask, “In what ways would teaching percentages benefit students’ initial learning of decimal fractions?”
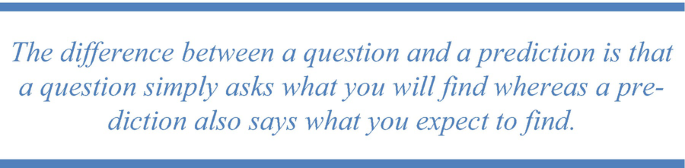
There are advantages to starting with the prediction form if you can make an educated guess about what you will find. Making a prediction forces you to think now about several things you will need to think about at some point anyway. It is better to think about them earlier rather than later. If you state your prediction clearly and explicitly, you can begin to ask yourself three questions about your prediction: Why do I expect to observe what I am predicting? Why did I make that prediction? (These two questions essentially ask what your rationale is for your prediction.) And, how can I test to see if it’s right? This is where the benefits of making predictions begin.
Asking yourself why you predicted what you did, and then asking yourself why you answered the first “why” question as you did, can be a powerful chain of thought that lays the groundwork for an increasingly accurate prediction and an increasingly well-reasoned rationale. For example, suppose you are the curriculum supervisor above who predicted that beginning by teaching percentages would be a good way to introduce students to decimal fractions. Why did you make this prediction? Maybe because students are familiar with percentages in everyday life so they could use what they know to anchor their thinking about hundredths. Why would that be helpful? Because if students could connect hundredths in percentage form with hundredths in decimal fraction form, they could bring their meaning of percentages into decimal fractions. But how would that help? If students understood that a decimal fraction like 0.35 meant 35 of 100, then they could use their understanding of hundredths to explore the meaning of tenths, thousandths, and so on. Why would that be useful? By continuing to ask yourself why you gave the previous answer, you can begin building your rationale and, as you build your rationale, you will find yourself revisiting your prediction, often making it more precise and explicit. If you were the curriculum supervisor and continued the reasoning in the previous sentences, you might elaborate your prediction by specifying the way in which percentages should be taught in order to have a positive effect on particular aspects of students’ understanding of decimal fractions.
Developing a Rationale for Your Predictions
Keeping your initial predictions in mind, you can read what others already know about the phenomenon. Your reading can now become targeted with a clear purpose.
By reading and talking with colleagues, you can develop more complete reasons for your predictions. It is likely that you will also decide to revise your predictions based on what you learn from your reading. As you develop sound reasons for your predictions, you are creating your rationales, and your predictions together with your rationales become your hypotheses. The more you learn about what is already known about your research topic, the more refined will be your predictions and the clearer and more complete your rationales. We will use the term more informed hypotheses to describe this evolution of your hypotheses.
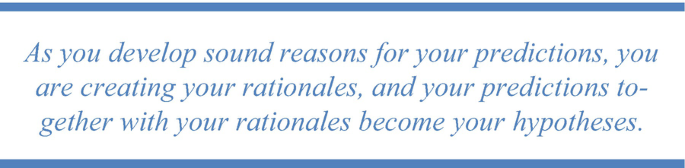
Developing more informed hypotheses is a good thing because it means: (1) you understand the reasons for your predictions; (2) you will be able to imagine how you can test your hypotheses; (3) you can more easily convince your colleagues that they are important hypotheses—they are hypotheses worth testing; and (4) at the end of your study, you will be able to more easily interpret the results of your test and to revise your hypotheses to demonstrate what you have learned by conducting the study.
Imagining Testing Your Hypotheses
Because we have tied together predictions and rationales to constitute hypotheses, testing hypotheses means testing predictions and rationales. Testing predictions means comparing empirical observations, or findings, with the predictions. Testing rationales means using these comparisons to evaluate the adequacy or soundness of the rationales.
Imagining how you might test your hypotheses does not mean working out the details for exactly how you would test them. Rather, it means thinking ahead about how you could do this. Recall the descriptor of scientific inquiry: “experience carefully planned in advance” (Fisher, 1935). Asking whether predictions are testable and whether rationales can be evaluated is simply planning in advance.
You might read that testing hypotheses means simply assessing whether predictions are correct or incorrect. In our view, it is more useful to think of testing as a means of gathering enough information to compare your findings with your predictions, revise your rationales, and propose more accurate predictions. So, asking yourself whether hypotheses can be tested means asking whether information could be collected to assess the accuracy of your predictions and whether the information will show you how to revise your rationales to sharpen your predictions.
Cycles of Building Rationales and Planning to Test Your Predictions
Scientific reasoning is a dialogue between the possible and the actual, an interplay between hypotheses and the logical expectations they give rise to: there is a restless to-and-fro motion of thought, the formulation and rectification of hypotheses (Medawar, 1982 , p.72).
As you ask yourself about how you could test your predictions, you will inevitably revise your rationales and sharpen your predictions. Your hypotheses will become more informed, more targeted, and more explicit. They will make clearer to you and others what, exactly, you plan to study.
When will you know that your hypotheses are clear and precise enough? Because of the way we define hypotheses, this question asks about both rationales and predictions. If a rationale you are building lets you make a number of quite different predictions that are equally plausible rather than a single, primary prediction, then your hypothesis needs further refinement by building a more complete and precise rationale. Also, if you cannot briefly describe to your colleagues a believable way to test your prediction, then you need to phrase it more clearly and precisely.
Each time you strengthen your rationales, you might need to adjust your predictions. And, each time you clarify your predictions, you might need to adjust your rationales. The cycle of going back and forth to keep your predictions and rationales tightly aligned has many payoffs down the road. Every decision you make from this point on will be in the interests of providing a transparent and convincing test of your hypotheses and explaining how the results of your test dictate specific revisions to your hypotheses. As you make these decisions (described in the succeeding chapters), you will probably return to clarify your hypotheses even further. But, you will be in a much better position, at each point, if you begin with well-informed hypotheses.
Beginning by Asking Questions to Clarify Your Interests
Instead of starting with predictions, a second path you might take devotes more time at the beginning to asking questions as you zero in on what you want to study. Some researchers suggest you start this way (e.g., Gournelos et al., 2019 ). Specifically, with this second path, the first statement you write to express your research interest would be a question. For example, you might ask, “Why do ninth-grade students change the way they think about linear equations after studying quadratic equations?” or “How do first graders solve simple arithmetic problems before they have been taught to add and subtract?”
The first phrasing of your question might be quite general or vague. As you think about your question and what you really want to know, you are likely to ask follow-up questions. These questions will almost always be more specific than your first question. The questions will also express more clearly what you want to know. So, the question “How do first graders solve simple arithmetic problems before they have been taught to add and subtract” might evolve into “Before first graders have been taught to solve arithmetic problems, what strategies do they use to solve arithmetic problems with sums and products below 20?” As you read and learn about what others already know about your questions, you will continually revise your questions toward clearer and more explicit and more precise versions that zero in on what you really want to know. The question above might become, “Before they are taught to solve arithmetic problems, what strategies do beginning first graders use to solve arithmetic problems with sums and products below 20 if they are read story problems and given physical counters to help them keep track of the quantities?”
Imagining Answers to Your Questions
If you monitor your own thinking as you ask questions, you are likely to begin forming some guesses about answers, even to the early versions of the questions. What do students learn about quadratic functions that influences changes in their proportional reasoning when dealing with linear functions? It could be that if you analyze the moments during instruction on quadratic equations that are extensions of the proportional reasoning involved in solving linear equations, there are times when students receive further experience reasoning proportionally. You might predict that these are the experiences that have a “backward transfer” effect (Hohensee, 2014 ).
These initial guesses about answers to your questions are your first predictions. The first predicted answers are likely to be hunches or fuzzy, vague guesses. This simply means you do not know very much yet about the question you are asking. Your first predictions, no matter how unfocused or tentative, represent the most you know at the time about the question you are asking. They help you gauge where you are in your thinking.
Shifting to the Hypothesis Formulation and Testing Path
Research questions can play an important role in the research process. They provide a succinct way of capturing your research interests and communicating them to others. When colleagues want to know about your work, they will often ask “What are your research questions?” It is good to have a ready answer.
However, research questions have limitations. They do not capture the three images of scientific inquiry presented in Chap. 1 . Due, in part, to this less expansive depiction of the process, research questions do not take you very far. They do not provide a guide that leads you through the phases of conducting a study.
Consequently, when you can imagine an answer to your research question, we recommend that you move onto the hypothesis formulation and testing path. Imagining an answer to your question means you can make plausible predictions. You can now begin clarifying the reasons for your predictions and transform your early predictions into hypotheses (predictions along with rationales). We recommend you do this as soon as you have guesses about the answers to your questions because formulating, testing, and revising hypotheses offers a tool that puts you squarely on the path of scientific inquiry. It is a tool that can guide you through the entire process of conducting a research study.
This does not mean you are finished asking questions. Predictions are often created as answers to questions. So, we encourage you to continue asking questions to clarify what you want to know. But your target shifts from only asking questions to also proposing predictions for the answers and developing reasons the answers will be accurate predictions. It is by predicting answers, and explaining why you made those predictions, that you become engaged in scientific inquiry.
Cycles of Refining Questions and Predicting Answers
An example might provide a sense of how this process plays out. Suppose you are reading about Vygotsky’s ( 1987 ) zone of proximal development (ZPD), and you realize this concept might help you understand why your high school students had trouble learning exponential functions. Maybe they were outside this zone when you tried to teach exponential functions. In order to recognize students who would benefit from instruction, you might ask, “How can I identify students who are within the ZPD around exponential functions?” What would you predict? Maybe students in this ZPD are those who already had knowledge of related functions. You could write out some reasons for this prediction, like “students who understand linear and quadratic functions are more likely to extend their knowledge to exponential functions.” But what kind of data would you need to test this? What would count as “understanding”? Are linear and quadratic the functions you should assess? Even if they are, how could you tell whether students who scored well on tests of linear and quadratic functions were within the ZPD of exponential functions? How, in the end, would you measure what it means to be in this ZPD? So, asking a series of reasonable questions raised some red flags about the way your initial question was phrased, and you decide to revise it.
You set the stage for revising your question by defining ZPD as the zone within which students can solve an exponential function problem by making only one additional conceptual connection between what they already know and exponential functions. Your revised question is, “Based on students’ knowledge of linear and quadratic functions, which students are within the ZPD of exponential functions?” This time you know what kind of data you need: the number of conceptual connections students need to bridge from their knowledge of related functions to exponential functions. How can you collect these data? Would you need to see into the minds of the students? Or, are there ways to test the number of conceptual connections someone makes to move from one topic to another? Do methods exist for gathering these data? You decide this is not realistic, so you now have a choice: revise the question further or move your research in a different direction.
Notice that we do not use the term research question for all these early versions of questions that begin clarifying for yourself what you want to study. These early versions are too vague and general to be called research questions. In this book, we save the term research question for a question that comes near the end of the work and captures exactly what you want to study . By the time you are ready to specify a research question, you will be thinking about your study in terms of hypotheses and tests. When your hypotheses are in final form and include clear predictions about what you will find, it will be easy to state the research questions that accompany your predictions.
To reiterate one of the key points of this chapter: hypotheses carry much more information than research questions. Using our definition, hypotheses include predictions about what the answer might be to the question plus reasons for why you think so. Unlike research questions, hypotheses capture all three images of scientific inquiry presented in Chap. 1 (planning, observing and explaining, and revising one’s thinking). Your hypotheses represent the most you know, at the moment, about your research topic. The same cannot be said for research questions.
Beginning with a Research Problem
When you wrote answers to the six questions at the end of Part I of this chapter, you might have identified a research interest by stating it as a problem. This is the third path you might take to begin your research. Perhaps your description of your problem might look something like this: “When I tried to teach my middle school students by presenting them with a challenging problem without showing them how to solve similar problems, they didn’t exert much effort trying to find a solution but instead waited for me to show them how to solve the problem.” You do not have a specific question in mind, and you do not have an idea for why the problem exists, so you do not have a prediction about how to solve it. Writing a statement of this problem as clearly as possible could be the first step in your research journey.
As you think more about this problem, it will feel natural to ask questions about it. For example, why did some students show more initiative than others? What could I have done to get them started? How could I have encouraged the students to keep trying without giving away the solution? You are now on the path of asking questions—not research questions yet, but questions that are helping you focus your interest.
As you continue to think about these questions, reflect on your own experience, and read what others know about this problem, you will likely develop some guesses about the answers to the questions. They might be somewhat vague answers, and you might not have lots of confidence they are correct, but they are guesses that you can turn into predictions. Now you are on the hypothesis-formulation-and-testing path. This means you are on the path of asking yourself why you believe the predictions are correct, developing rationales for the predictions, asking what kinds of empirical observations would test your predictions, and refining your rationales and predictions as you read the literature and talk with colleagues.
A simple diagram that summarizes the three paths we have described is shown in Fig. 2.1 . Each row of arrows represents one pathway for formulating an informed hypothesis. The dotted arrows in the first two rows represent parts of the pathways that a researcher may have implicitly travelled through already (without an intent to form a prediction) but that ultimately inform the researcher’s development of a question or prediction.
Part III. One Researcher’s Experience Launching a Scientific Inquiry
Martha was in her third year of her doctoral program and beginning to identify a topic for her dissertation. Based on (a) her experience as a high school mathematics teacher and a curriculum supervisor, (b) the reading she has done to this point, and (c) her conversations with her colleagues, she has developed an interest in what kinds of professional development experiences (let’s call them learning opportunities [LOs] for teachers) are most effective. Where does she go from here?
Exercise 2.2
Before you continue reading, please write down some suggestions for Martha about where she should start.
A natural thing for Martha to do at this point is to ask herself some additional questions, questions that specify further what she wants to learn: What kinds of LOs do most teachers experience? How do these experiences change teachers’ practices and beliefs? Are some LOs more effective than others? What makes them more effective?
To focus her questions and decide what she really wants to know, she continues reading but now targets her reading toward everything she can find that suggests possible answers to these questions. She also talks with her colleagues to get more ideas about possible answers to these or related questions. Over several weeks or months, she finds herself being drawn to questions about what makes LOs effective, especially for helping teachers teach more conceptually. She zeroes in on the question, “What makes LOs for teachers effective for improving their teaching for conceptual understanding?”
This question is more focused than her first questions, but it is still too general for Martha to define a research study. How does she know it is too general? She uses two criteria. First, she notices that the predictions she makes about the answers to the question are all over the place; they are not constrained by the reasons she has assembled for her predictions. One prediction is that LOs are more effective when they help teachers learn content. Martha makes this guess because previous research suggests that effective LOs for teachers include attention to content. But this rationale allows lots of different predictions. For example, LOs are more effective when they focus on the content teachers will teach; LOs are more effective when they focus on content beyond what teachers will teach so teachers see how their instruction fits with what their students will encounter later; and LOs are more effective when they are tailored to the level of content knowledge participants have when they begin the LOs. The rationale she can provide at this point does not point to a particular prediction.
A second measure Martha uses to decide her question is too general is that the predictions she can make regarding the answers seem very difficult to test. How could she test, for example, whether LOs should focus on content beyond what teachers will teach? What does “content beyond what teachers teach” mean? How could you tell whether teachers use their new knowledge of later content to inform their teaching?
Before anticipating what Martha’s next question might be, it is important to pause and recognize how predicting the answers to her questions moved Martha into a new phase in the research process. As she makes predictions, works out the reasons for them, and imagines how she might test them, she is immersed in scientific inquiry. This intellectual work is the main engine that drives the research process. Also notice that revisions in the questions asked, the predictions made, and the rationales built represent the updated thinking (Chap. 1 ) that occurs as Martha continues to define her study.
Based on all these considerations and her continued reading, Martha revises the question again. The question now reads, “Do LOs that engage middle school mathematics teachers in studying mathematics content help teachers teach this same content with more of a conceptual emphasis?” Although she feels like the question is more specific, she realizes that the answer to the question is either “yes” or “no.” This, by itself, is a red flag. Answers of “yes” or “no” would not contribute much to understanding the relationships between these LOs for teachers and changes in their teaching. Recall from Chap. 1 that understanding how things work, explaining why things work, is the goal of scientific inquiry.
Martha continues by trying to understand why she believes the answer is “yes.” When she tries to write out reasons for predicting “yes,” she realizes that her prediction depends on a variety of factors. If teachers already have deep knowledge of the content, the LOs might not affect them as much as other teachers. If the LOs do not help teachers develop their own conceptual understanding, they are not likely to change their teaching. By trying to build the rationale for her prediction—thus formulating a hypothesis—Martha realizes that the question still is not precise and clear enough.
Martha uses what she learned when developing the rationale and rephrases the question as follows: “ Under what conditions do LOs that engage middle school mathematics teachers in studying mathematics content help teachers teach this same content with more of a conceptual emphasis?” Through several additional cycles of thinking through the rationale for her predictions and how she might test them, Martha specifies her question even further: “Under what conditions do middle school teachers who lack conceptual knowledge of linear functions benefit from LOs that engage them in conceptual learning of linear functions as assessed by changes in their teaching toward a more conceptual emphasis on linear functions?”
Each version of Martha’s question has become more specific. This has occurred as she has (a) identified a starting condition for the teachers—they lack conceptual knowledge of linear functions, (b) specified the mathematics content as linear functions, and (c) included a condition or purpose of the LO—it is aimed at conceptual learning.
Because of the way Martha’s question is now phrased, her predictions will require thinking about the conditions that could influence what teachers learn from the LOs and how this learning could affect their teaching. She might predict that if teachers engaged in LOs that extended over multiple sessions, they would develop deeper understanding which would, in turn, prompt changes in their teaching. Or she might predict that if the LOs included examples of how their conceptual learning could translate into different instructional activities for their students, teachers would be more likely to change their teaching. Reasons for these predictions would likely come from research about the effects of professional development on teachers’ practice.
As Martha thinks about testing her predictions, she realizes it will probably be easier to measure the conditions under which teachers are learning than the changes in the conceptual emphasis in their instruction. She makes a note to continue searching the literature for ways to measure the “conceptualness” of teaching.
As she refines her predictions and expresses her reasons for the predictions, she formulates a hypothesis (in this case several hypotheses) that will guide her research. As she makes predictions and develops the rationales for these predictions, she will probably continue revising her question. She might decide, for example, that she is not interested in studying the condition of different numbers of LO sessions and so decides to remove this condition from consideration by including in her question something like “. . . over five 2-hour sessions . . .”
At this point, Martha has developed a research question, articulated a number of predictions, and developed rationales for them. Her current question is: “Under what conditions do middle school teachers who lack conceptual knowledge of linear functions benefit from five 2-hour LO sessions that engage them in conceptual learning of linear functions as assessed by changes in their teaching toward a more conceptual emphasis on linear functions?” Her hypothesis is:
Prediction: Participating teachers will show changes in their teaching with a greater emphasis on conceptual understanding, with larger changes on linear function topics directly addressed in the LOs than on other topics.
Brief Description of Rationale: (1) Past research has shown correlations between teachers’ specific mathematics knowledge of a topic and the quality of their teaching of that topic. This does not mean an increase in knowledge causes higher quality teaching but it allows for that possibility. (2) Transfer is usually difficult for teachers, but the examples developed during the LO sessions will help them use what they learned to teach for conceptual understanding. This is because the examples developed during the LO sessions are much like those that will be used by the teachers. So larger changes will be found when teachers are teaching the linear function topics addressed in the LOs.
Notice it is more straightforward to imagine how Martha could test this prediction because it is more precise than previous predictions. Notice also that by asking how to test a particular prediction, Martha will be faced with a decision about whether testing this prediction will tell her something she wants to learn. If not, she can return to the research question and consider how to specify it further and, perhaps, constrain further the conditions that could affect the data.
As Martha formulates her hypotheses and goes through multiple cycles of refining her question(s), articulating her predictions, and developing her rationales, she is constantly building the theoretical framework for her study. Because the theoretical framework is the topic for Chap. 3 , we will pause here and pick up Martha’s story in the next chapter. Spoiler alert: Martha’s experience contains some surprising twists and turns.
Before leaving Martha, however, we point out two aspects of the process in which she has been engaged. First, it can be useful to think about the process as identifying (1) the variables targeted in her predictions, (2) the mechanisms she believes explain the relationships among the variables, and (3) the definitions of all the terms that are special to her educational problem. By variables, we mean things that can be measured and, when measured, can take on different values. In Martha’s case, the variables are the conceptualness of teaching and the content topics addressed in the LOs. The mechanisms are cognitive processes that enable teachers to see the relevance of what they learn in PD to their own teaching and that enable the transfer of learning from one setting to another. Definitions are the precise descriptions of how the important ideas relevant to the research are conceptualized. In Martha’s case, definitions must be provided for terms like conceptual understanding, linear functions, LOs, each of the topics related to linear functions, instructional setting, and knowledge transfer.
A second aspect of the process is a practice that Martha acquired as part of her graduate program, a practice that can go unnoticed. Martha writes out, in full sentences, her thinking as she wrestles with her research question, her predictions of the answers, and the rationales for her predictions. Writing is a tool for organizing thinking and we recommend you use it throughout the scientific inquiry process. We say more about this at the end of the chapter.
Here are the questions Martha wrote as she developed a clearer sense of what question she wanted to answer and what answer she predicted. The list shows the increasing refinement that occurred as she continued to read, think, talk, and write.
Early questions: What kinds of LOs do most teachers experience? How do these experiences change teachers’ practices and beliefs? Are some LOs more effective than others? What makes them more effective?
First focused question: What makes LOs for teachers effective for improving their teaching for conceptual understanding?
Question after trying to predict the answer and imagining how to test the prediction: Do LOs that engage middle school mathematics teachers in studying mathematics content help teachers teach this same content with more of a conceptual emphasis?
Question after developing an initial rationale for her prediction: Under what conditions do LOs that engage middle school mathematics teachers in studying mathematics content help teachers teach this same content with more of a conceptual emphasis?
Question after developing a more precise prediction and richer rationale: Under what conditions do middle school teachers who lack conceptual knowledge of linear functions benefit from five 2-hour LO sessions that engage them in conceptual learning of linear functions as assessed by changes in their teaching toward a more conceptual emphasis on linear functions?
Part IV. An Illustrative Dialogue
The story of Martha described the major steps she took to refine her thinking. However, there is a lot of work that went on behind the scenes that wasn’t part of the story. For example, Martha had conversations with fellow students and professors that sharpened her thinking. What do these conversations look like? Because they are such an important part of the inquiry process, it will be helpful to “listen in” on the kinds of conversations that students might have with their advisors.
Here is a dialogue between a beginning student, Sam (S), and their advisor, Dr. Avery (A). They are meeting to discuss data Sam collected for a course project. The dialogue below is happening very early on in Sam’s conceptualization of the study, prior even to systematic reading of the literature.
Thanks for meeting with me today. As you know, I was able to collect some data for a course project a few weeks ago, but I’m having trouble analyzing the data, so I need your help. Let me try to explain the problem. As you know, I wanted to understand what middle-school teachers do to promote girls’ achievement in a mathematics class. I conducted four observations in each of three teachers’ classrooms. I also interviewed each teacher once about the four lessons I observed, and I interviewed two girls from each of the teachers’ classes. Obviously, I have a ton of data. But when I look at all these data, I don’t really know what I learned about my topic. When I was observing the teachers, I thought I might have observed some ways the teachers were promoting girls’ achievement, but then I wasn’t sure how to interpret my data. I didn’t know if the things I was observing were actually promoting girls’ achievement.
What were some of your observations?
Well, in a couple of my classroom observations, teachers called on girls to give an answer, even when the girls didn’t have their hands up. I thought that this might be a way that teachers were promoting the girls’ achievement. But then the girls didn’t say anything about that when I interviewed them and also the teachers didn’t do it in every class. So, it’s hard to know what effect, if any, this might have had on their learning or their motivation to learn. I didn’t want to ask the girls during the interview specifically about the teacher calling on them, and without the girls bringing it up themselves, I didn’t know if it had any effect.
Well, why didn’t you want to ask the girls about being called on?
Because I wanted to leave it as open as possible; I didn’t want to influence what they were going to say. I didn’t want to put words in their mouths. I wanted to know what they thought the teacher was doing that promoted their mathematical achievement and so I only asked the girls general questions, like “Do you think the teacher does things to promote girls’ mathematical achievement?” and “Can you describe specific experiences you have had that you believe do and do not promote your mathematical achievement?”
So then, how did they answer those general questions?
Well, with very general answers, such as that the teacher knows their names, offers review sessions, grades their homework fairly, gives them opportunities to earn extra credit, lets them ask questions, and always answers their questions. Nothing specific that helps me know what teaching actions specifically target girls’ mathematics achievement.
OK. Any ideas about what you might do next?
Well, I remember that when I was planning this data collection for my course, you suggested I might want to be more targeted and specific about what I was looking for. I can see now that more targeted questions would have made my data more interpretable in terms of connecting teaching actions to the mathematical achievement of girls. But I just didn’t want to influence what the girls would say.
Yes, I remember when you were planning your course project, you wanted to keep it open. You didn’t want to miss out on discovering something new and interesting. What do you think now about this issue?
Well, I still don’t want to put words in their mouths. I want to know what they think. But I see that if I ask really open questions, I have no guarantee they will talk about what I want them to talk about. I guess I still like the idea of an open study, but I see that it’s a risky approach. Leaving the questions too open meant I didn’t constrain their responses and there were too many ways they could interpret and answer the questions. And there are too many ways I could interpret their responses.
By this point in the dialogue, Sam has realized that open data (i.e., data not testing a specific prediction) is difficult to interpret. In the next part, Dr. Avery explains why collecting open data was not helping Sam achieve goals for her study that had motivated collecting open data in the first place.
Yes, I totally agree. Even for an experienced researcher, it can be difficult to make sense of this kind of open, messy data. However, if you design a study with a more specific focus, you can create questions for participants that are more targeted because you will be interested in their answers to these specific questions. Let’s reflect back on your data collection. What can you learn from it for the future?
When I think about it now, I realize that I didn’t think about the distinction between all the different constructs at play in my study, and I didn’t choose which one I was focusing on. One construct was the teaching moves that teachers think could be promoting achievement. Another is what teachers deliberately do to promote girls’ mathematics achievement, if anything. Another was the teaching moves that actually do support girls’ mathematics achievement. Another was what teachers were doing that supported girls’ mathematics achievement versus the mathematics achievement of all students. Another was students’ perception of what their teacher was doing to promote girls’ mathematics achievement. I now see that any one of these constructs could have been the focus of a study and that I didn’t really decide which of these was the focus of my course project prior to collecting data.
So, since you told me that the topic of this course project is probably what you’ll eventually want to study for your dissertation, which of these constructs are you most interested in?
I think I’m more interested in the teacher moves that teachers deliberately do to promote girls’ achievement. But I’m still worried about asking teachers directly and getting too specific about what they do because I don’t want to bias what they will say. And I chose qualitative methods and an exploratory design because I thought it would allow for a more open approach, an approach that helps me see what’s going on and that doesn’t bias or predetermine the results.
Well, it seems to me you are conflating three issues. One issue is how to conduct an unbiased study. Another issue is how specific to make your study. And the third issue is whether or not to choose an exploratory or qualitative study design. Those three issues are not the same. For example, designing a study that’s more open or more exploratory is not how researchers make studies fair and unbiased. In fact, it would be quite easy to create an open study that is biased. For example, you could ask very open questions and then interpret the responses in a way that unintentionally, and even unknowingly, aligns with what you were hoping the findings would say. Actually, you could argue that by adding more specificity and narrowing your focus, you’re creating constraints that prevent bias. The same goes for an exploratory or qualitative study; they can be biased or unbiased. So, let’s talk about what is meant by getting more specific. Within your new focus on what teachers deliberately do, there are many things that would be interesting to look at, such as teacher moves that address math anxiety, moves that allow girls to answer questions more frequently, moves that are specifically fitted to student thinking about specific mathematical content, and so on. What are one or two things that are most interesting to you? One way to answer this question is by thinking back to where your interest in this topic began.
In the preceding part of the dialogue, Dr. Avery explained how the goals Sam had for their study were not being met with open data. In the next part, Sam begins to articulate a prediction, which Sam and Dr. Avery then sharpen.
Actually, I became interested in this topic because of an experience I had in college when I was in a class of mostly girls. During whole class discussions, we were supposed to critically evaluate each other’s mathematical thinking, but we were too polite to do that. Instead, we just praised each other’s work. But it was so different in our small groups. It seemed easier to critique each other’s thinking and to push each other to better solutions in small groups. I began wondering how to get girls to be more critical of each other’s thinking in a whole class discussion in order to push everyone’s thinking.
Okay, this is great information. Why not use this idea to zoom-in on a more manageable and interpretable study? You could look specifically at how teachers support girls in critically evaluating each other’s thinking during whole class discussions. That would be a much more targeted and specific topic. Do you have predictions about what teachers could do in that situation, keeping in mind that you are looking specifically at girls’ mathematical achievement, not students in general?
Well, what I noticed was that small groups provided more social and emotional support for girls, whereas the whole class discussion did not provide that same support. The girls felt more comfortable critiquing each other’s thinking in small groups. So, I guess I predict that when the social and emotional supports that are present in small groups are extended to the whole class discussion, girls would be more willing to evaluate each other’s mathematical thinking critically during whole class discussion . I guess ultimately, I’d like to know how the whole class discussion could be used to enhance, rather than undermine, the social and emotional support that is present in the small groups.
Okay, then where would you start? Would you start with a study of what the teachers say they will do during whole class discussion and then observe if that happens during whole class discussion?
But part of my prediction also involves the small groups. So, I’d also like to include small groups in my study if possible. If I focus on whole groups, I won’t be exploring what I am interested in. My interest is broader than just the whole class discussion.
That makes sense, but there are many different things you could look at as part of your prediction, more than you can do in one study. For instance, if your prediction is that when the social and emotional supports that are present in small groups are extended to whole class discussions, girls would be more willing to evaluate each other’s mathematical thinking critically during whole class discussions , then you could ask the following questions: What are the social and emotional supports that are present in small groups?; In which small groups do they exist?; Is it groups that are made up only of girls?; Does every small group do this, and for groups that do this, when do these supports get created?; What kinds of small group activities that teachers ask them to work on are associated with these supports?; Do the same social and emotional supports that apply to small groups even apply to whole group discussion?
All your questions make me realize that my prediction about extending social and emotional supports to whole class discussions first requires me to have a better understanding of the social and emotional supports that exist in small groups. In fact, I first need to find out whether those supports commonly exist in small groups or is that just my experience working in small groups. So, I think I will first have to figure out what small groups do to support each other and then, in a later study, I could ask a teacher to implement those supports during whole class discussions and find out how you can do that. Yeah, now I’m seeing that.
The previous part of the dialogue illustrates how continuing to ask questions about one’s initial prediction is a good way to make it more and more precise (and researchable). In the next part, we see how developing a precise prediction has the added benefit of setting the researcher up for future studies.
Yes, I agree that for your first study, you should probably look at small groups. In other words, you should focus on only a part of your prediction for now, namely the part that says there are social and emotional supports in small groups that support girls in critiquing each other’s thinking . That begins to sharpen the focus of your prediction, but you’ll want to continue to refine it. For example, right now, the question that this prediction leads to is a question with a yes or no answer, but what you’ve said so far suggests to me that you are looking for more than that.
Yes, I want to know more than just whether there are supports. I’d like to know what kinds. That’s why I wanted to do a qualitative study.
Okay, this aligns more with my thinking about research as being prediction driven. It’s about collecting data that would help you revise your existing predictions into better ones. What I mean is that you would focus on collecting data that would allow you to refine your prediction, make it more nuanced, and go beyond what is already known. Does that make sense, and if so, what would that look like for your prediction?
Oh yes, I like that. I guess that would mean that, based on the data I collect for this next study, I could develop a more refined prediction that, for example, more specifically identifies and differentiates between different kinds of social and emotional supports that are present in small groups, or maybe that identifies the kinds of small groups that they occur in, or that predicts when and how frequently or infrequently they occur, or about the features of the small group tasks in which they occur, etc. I now realize that, although I chose qualitative research to make my study be more open, really the reason qualitative research fits my purposes is because it will allow me to explore fine-grained aspects of social and emotional supports that may exist for girls in small groups.
Yes, exactly! And then, based on the data you collect, you can include in your revised prediction those new fine-grained aspects. Furthermore, you will have a story to tell about your study in your written report, namely the story about your evolving prediction. In other words, your written report can largely tell how you filled out and refined your prediction as you learned more from carrying out the study. And even though you might not use them right away, you are also going to be able to develop new predictions that you would not have even thought of about social and emotional supports in small groups and your aim of extending them to whole-class discussions, had you not done this study. That will set you up to follow up on those new predictions in future studies. For example, you might have more refined ideas after you collect the data about the goals for critiquing student thinking in small groups versus the goals for critiquing student thinking during whole class discussion. You might even begin to think that some of the social and emotional supports you observe are not even replicable or even applicable to or appropriate for whole-class discussions, because the supports play different roles in different contexts. So, to summarize what I’m saying, what you look at in this study, even though it will be very focused, sets you up for a research program that will allow you to more fully investigate your broader interest in this topic, where each new study builds on your prior body of work. That’s why it is so important to be explicit about the best place to start this research, so that you can build on it.
I see what you are saying. We started this conversation talking about my course project data. What I think I should have done was figure out explicitly what I needed to learn with that study with the intention of then taking what I learned and using it as the basis for the next study. I didn’t do that, and so I didn’t collect data that pushed forward my thinking in ways that would guide my next study. It would be as if I was starting over with my next study.
Sam and Dr. Avery have just explored how specifying a prediction reveals additional complexities that could become fodder for developing a systematic research program. Next, we watch Sam beginning to recognize the level of specificity required for a prediction to be testable.
One thing that would have really helped would have been if you had had a specific prediction going into your data collection for your course project.
Well, I didn’t really have much of an explicit prediction in mind when I designed my methods.
Think back, you must have had some kind of prediction, even if it was implicit.
Well, yes, I guess I was predicting that teachers would enact moves that supported girls’ mathematical achievement. And I observed classrooms to identify those teacher moves, I interviewed teachers to ask them about the moves I observed, and I interviewed students to see if they mentioned those moves as promoting their mathematical achievement. The goal of my course project was to identify teacher moves that support girls’ mathematical achievement. And my specific research question was: What teacher moves support girls’ mathematical achievement?
So, really you were asking the teacher and students to show and tell you what those moves are and the effects of those moves, as a result putting the onus on your participants to provide the answers to your research question for you. I have an idea, let’s try a thought experiment. You come up with data collection methods for testing the prediction that there are social and emotional supports in small groups that support girls in critiquing each other’s thinking that still puts the onus on the participants. And then I’ll see if I can think of data collection methods that would not put the onus on the participants.
Hmm, well. .. I guess I could simply interview girls who participated in small groups and ask them “are there social and emotional supports that you use in small groups that support your group in critiquing each other’s thinking and if so, what are they?” In that case, I would be putting the onus on them to be aware of the social dynamics of small groups and to have thought about these constructs as much as I have. Okay now can you continue the thought experiment? What might the data collection methods look like if I didn’t put the onus on the participants?
First, I would pick a setting in which it was only girls at this point to reduce the number of variables. Then, personally I would want to observe a lot of groups of girls interacting in groups around tasks. I would be looking for instances when the conversation about students’ ideas was shut down and instances when the conversation about students’ ideas involved critiquing of ideas and building on each other’s thinking. I would also look at what happened just before and during those instances, such as: did the student continue to talk after their thinking was critiqued, did other students do anything to encourage the student to build on their own thinking (i.e., constructive criticism) or how did they support or shut down continued participation. In fact, now that I think about it, “critiquing each other’s thinking” can be defined in a number of different ways. I could mean just commenting on someone’s thinking, judging correctness and incorrectness, constructive criticism that moves the thinking forward, etc. If you put the onus on the participants to answer your research question, you are stuck with their definition, and they won’t have thought about this very much, if at all.
I think that what you are also saying is that my definitions would affect my data collection. If I think that critiquing each other’s thinking means that the group moves their thinking forward toward more valid and complete mathematical solutions, then I’m going to focus on different moves than if I define it another way, such as just making a comment on each other’s thinking and making each other feel comfortable enough to keep participating. In fact, am I going to look at individual instances of critiquing or look at entire sequences in which the critiquing leads to a goal? This seems like a unit of analysis question, and I would need to develop a more nuanced prediction that would make explicit what that unit of analysis is.
I agree, your definition of “critiquing each other’s thinking” could entirely change what you are predicting. One prediction could be based on defining critiquing as a one-shot event in which someone makes one comment on another person’s thinking. In this case the prediction would be that there are social and emotional supports in small groups that support girls in making an evaluative comment on another student’s thinking. Another prediction could be based on defining critiquing as a back-and-forth process in which the thinking gets built on and refined. In that case, the prediction would be something like that there are social and emotional supports in small groups that support girls in critiquing each other’s thinking in ways that do not shut down the conversation but that lead to sustained conversations that move each other toward more valid and complete solutions.
Well, I think I am more interested in the second prediction because it is more compatible with my long-term interests, which are that I’m interested in extending small group supports to whole class discussions. The second prediction is more appropriate for eventually looking at girls in whole class discussion. During whole class discussion, the teacher tries to get a sustained conversation going that moves the students’ thinking forward. So, if I learn about small group supports that lead to sustained conversations that move each other toward more valid and complete solutions , those supports might transfer to whole class discussions.
In the previous part of the dialogue, Dr. Avery and Sam showed how narrowing down a prediction to one that is testable requires making numerous important decisions, including how to define the constructs referred to in the prediction. In the final part of the dialogue, Dr. Avery and Sam begin to outline the reading Sam will have to do to develop a rationale for the specific prediction.
Do you see how your prediction and definitions are getting more and more specific? You now need to read extensively to further refine your prediction.
Well, I should probably read about micro dynamics of small group interactions, anything about interactions in small groups, and what is already known about small group interactions that support sustained conversations that move students’ thinking toward more valid and complete solutions. I guess I could also look at research on whole-class discussion methods that support sustained conversations that move the class to more mathematically valid and complete solutions, because it might give me ideas for what to look for in the small groups. I might also need to focus on research about how learners develop understandings about a particular subject matter so that I know what “more valid and complete solutions” look like. I also need to read about social and emotional supports but focus on how they support students cognitively, rather than in other ways.
Sounds good, let’s get together after you have processed some of this literature and we can talk about refining your prediction based on what you read and also the methods that will best suit testing that prediction.
Great! Thanks for meeting with me. I feel like I have a much better set of tools that push my own thinking forward and allow me to target something specific that will lead to more interpretable data.
Part V. Is It Always Possible to Formulate Hypotheses?
In Chap. 1 , we noted you are likely to read that research does not require formulating hypotheses. Some sources describe doing research without making predictions and developing rationales for these predictions. Some researchers say you cannot always make predictions—you do not know enough about the situation. In fact, some argue for the value of not making predictions (e.g., Glaser & Holton, 2004 ; Merton, 1968 ; Nemirovsky, 2011 ). These are important points of view, so we will devote this section to discussing them.
Can You Always Predict What You Will Find?
One reason some researchers say you do not need to make predictions is that it can be difficult to imagine what you will find. This argument comes up most often for descriptive studies. Suppose you want to describe the nature of a situation you do not know much about. Can you still make a prediction about what you will find? We believe that, although you do not know exactly what you will find, you probably have a hunch or, at a minimum, a very fuzzy idea. It would be unusual to ask a question about a situation you want to know about without at least a fuzzy inkling of what you might find. The original question just would not occur to you. We acknowledge you might have only a vague idea of what you will find and you might not have much confidence in your prediction. However, we expect if you monitor your own thinking you will discover you have developed a suspicion along the way, regardless how vague the suspicion might be. Through the cyclic process we discussed above, that suspicion or hunch gradually evolves and turns into a prediction.
The Benefits of Making Predictions Even When They Are Wrong: An Example from the 1970s
One of us was a graduate student at the University of Wisconsin in the late 1970s, assigned as a research assistant to a project that was investigating young children’s thinking about simple arithmetic. A new curriculum was being written, and the developers wanted to know how to introduce the earliest concepts and skills to kindergarten and first-grade children. The directors of the project did not know what to expect because, at the time, there was little research on five- and six-year-olds’ pre-instruction strategies for adding and subtracting.
After consulting what literature was available, talking with teachers, analyzing the nature of different types of addition and subtraction problems, and debating with each other, the research team formulated some hypotheses about children’s performance. Following the usual assumptions at the time and recognizing the new curriculum would introduce the concepts, the researchers predicted that, before instruction, most children would not be able to solve the problems. Based on the rationale that some young children did not yet recognize the simple form for written problems (e.g., 5 + 3 = ___), the researchers predicted that the best chance for success would be to read problems as stories (e.g., Jesse had 5 apples and then found 3 more. How many does she have now?). They reasoned that, even though children would have difficulty on all the problems, some story problems would be easier because the semantic structure is easier to follow. For example, they predicted the above story about adding 3 apples to 5 would be easier than a problem like, “Jesse had some apples in the refrigerator. She put in 2 more and now has 6. How many were in the refrigerator at the beginning?” Based on the rationale that children would need to count to solve the problems and that it can be difficult to keep track of the numbers, they predicted children would be more successful if they were given counters. Finally, accepting the common reasoning that larger numbers are more difficult than smaller numbers, they predicted children would be more successful if all the numbers in a problem were below 10.
Although these predictions were not very precise and the rationales were not strongly convincing, these hypotheses prompted the researchers to design the study to test their predictions. This meant they would collect data by presenting a variety of problems under a variety of conditions. Because the goal was to describe children’s thinking, problems were presented to students in individual interviews. Problems with different semantic structures were included, counters were available for some problems but not others, and some problems had sums to 9 whereas others had sums to 20 or more.
The punchline of this story is that gathering data under these conditions, prompted by the predictions, made all the difference in what the researchers learned. Contrary to predictions, children could solve addition and subtraction problems before instruction. Counters were important because almost all the solution strategies were based on counting which meant that memory was an issue because many strategies require counting in two ways simultaneously. For example, subtracting 4 from 7 was usually solved by counting down from 7 while counting up from 1 to 4 to keep track of counting down. Because children acted out the stories with their counters, the semantic structure of the story was also important. Stories that were easier to read and write were also easier to solve.
To make a very long story very short, other researchers were, at about the same time, reporting similar results about children’s pre-instruction arithmetic capabilities. A clear pattern emerged regarding the relative difficulty of different problem types (semantic structures) and the strategies children used to solve each type. As the data were replicated, the researchers recognized that kindergarten and first-grade teachers could make good use of this information when they introduced simple arithmetic. This is how Cognitively Guided Instruction (CGI) was born (Carpenter et al., 1989 ; Fennema et al., 1996 ).
To reiterate, the point of this example is that the study conducted to describe children’s thinking would have looked quite different if the researchers had made no predictions. They would have had no reason to choose the particular problems and present them under different conditions. The fact that some of the predictions were completely wrong is not the point. The predictions created the conditions under which the predictions were tested which, in turn, created learning opportunities for the researchers that would not have existed without the predictions. The lesson is that even research that aims to simply describe a phenomenon can benefit from hypotheses. As signaled in Chap. 1 , this also serves as another example of “failing productively.”
Suggestions for What to Do When You Do Not Have Predictions
There likely are exceptions to our claim about being able to make a prediction about what you will find. For example, there could be rare cases where researchers truly have no idea what they will find and can come up with no predictions and even no hunches. And, no research has been reported on related phenomena that would offer some guidance. If you find yourself in this position, we suggest one of three approaches: revise your question, conduct a pilot study, or choose another question.
Because there are many advantages to making predictions explicit and then writing out the reasons for these predictions, one approach is to adjust your question just enough to allow you to make a prediction. Perhaps you can build on descriptions that other researchers have provided for related situations and consider how you can extend this work. Building on previous descriptions will enable you to make predictions about the situation you want to describe.
A second approach is to conduct a small pilot study or, better, a series of small pilot studies to develop some preliminary ideas of what you might find. If you can identify a small sample of participants who are similar to those in your study, you can try out at least some of your research plans to help make and refine your predictions. As we detail later, you can also use pilot studies to check whether key aspects of your methods (e.g., tasks, interview questions, data collection methods) work as you expect.
A third approach is to return to your list of interests and choose one that has been studied previously. Sometimes this is the wisest choice. It is very difficult for beginning researchers to conduct research in brand-new areas where no hunches or predictions are possible. In addition, the contributions of this research can be limited. Recall the earlier story about one of us “failing productively” by completing a dissertation in a somewhat new area. If, after an exhaustive search, you find that no one has investigated the phenomenon in which you are interested or even related phenomena, it can be best to move in a different direction. You will read recommendations in other sources to find a “gap” in the research and develop a study to “fill the gap.” This can be helpful advice if the gap is very small. However, if the gap is large, too large to predict what you might find, the study will present severe challenges. It will be more productive to extend work that has already been done than to launch into an entirely new area.
Should You Always Try to Predict What You Will Find?
In short, our answer to the question in the heading is “yes.” But this calls for further explanation.
Suppose you want to observe a second-grade classroom in order to investigate how students talk about adding and subtracting whole numbers. You might think, “I don’t want to bias my thinking; I want to be completely open to what I see in the classroom.” Sam shared a similar point of view at the beginning of the dialogue: “I wanted to leave it as open as possible; I didn’t want to influence what they were going to say.” Some researchers say that beginning your research study by making predictions is inappropriate precisely because it will bias your observations and results. The argument is that by bringing a set of preconceptions, you will confirm what you expected to find and be blind to other observations and outcomes. The following quote illustrates this view: “The first step in gaining theoretical sensitivity is to enter the research setting with as few predetermined ideas as possible—especially logically deducted, a priori hypotheses. In this posture, the analyst is able to remain sensitive to the data by being able to record events and detect happenings without first having them filtered through and squared with pre-existing hypotheses and biases” (Glaser, 1978, pp. 2–3).
We take a different point of view. In fact, we believe there are several compelling reasons for making your predictions explicit.
Making Your Predictions Explicit Increases Your Chances of Productive Observations
Because your predictions are an extension of what is already known, they prepare you to identify more nuanced relationships that can advance our understanding of a phenomenon. For example, rather than simply noticing, in a general sense, that students talking about addition and subtraction leads them to better understandings, you might, based on your prediction, make the specific observation that talking about addition and subtraction in a particular way helps students to think more deeply about a particular concept related to addition and subtraction. Going into a study without predictions can bring less sensitivity rather than more to the study of a phenomenon. Drawing on knowledge about related phenomena by reading the literature and conducting pilot studies allows you to be much more sensitive and your observations to be more productive.
Making Your Predictions Explicit Allows You to Guard Against Biases
Some genres and methods of educational research are, in fact, rooted in philosophical traditions (e.g., Husserl, 1929/ 1973 ) that explicitly call for researchers to temporarily “bracket” or set aside existing theory as well as their prior knowledge and experience to better enter into the experience of the participants in the research. However, this does not mean ignoring one’s own knowledge and experience or turning a blind eye to what has been learned by others. Much more than the simplistic image of emptying one’s mind of preconceptions and implicit biases (arguably an impossible feat to begin with), the goal is to be as reflective as possible about one’s prior knowledge and conceptions and as transparent as possible about how they may guide observations and shape interpretations (Levitt et al., 2018 ).
We believe it is better to be honest about the predictions you are almost sure to have because then you can deliberately plan to minimize the chances they will influence what you find and how you interpret your results. For starters, it is important to recognize that acknowledging you have some guesses about what you will find does not make them more influential. Because you are likely to have them anyway, we recommend being explicit about what they are. It is easier to deal with biases that are explicit than those that lurk in the background and are not acknowledged.
What do we mean by “deal with biases”? Some journals require you to include a statement about your “positionality” with respect to the participants in your study and the observations you are making to gather data. Formulating clear hypotheses is, in our view, a direct response to this request. The reasons for your predictions are your explicit statements about your positionality. Often there are methodological strategies you can use to protect the study from undue influences of bias. In other words, making your vague predictions explicit can help you design your study so you minimize the bias of your findings.
Making Your Predictions Explicit Can Help You See What You Did Not Predict
Making your predictions explicit does not need to blind you to what is different than expected. It does not need to force you to see only what you want to see. Instead, it can actually increase your sensitivity to noticing features of the situation that are surprising, features you did not predict. Results can stand out when you did not expect to see them.
In contrast, not bringing your biases to consciousness might subtly shift your attention away from these unexpected results in ways that you are not aware of. This path can lead to claiming no biases and no unexpected findings without being conscious of them. You cannot observe everything, and some things inevitably will be overlooked. If you have predicted what you will see, you can design your study so that the unexpected results become more salient rather than less.
Returning to the example of observing a second-grade classroom, we note that the field already knows a great deal about how students talk about addition and subtraction. Being cognizant of what others have observed allows you to enter the classroom with some clear predictions about what will happen. The rationales for these predictions are based on all the related knowledge you have before stepping into the classroom, and the predictions and rationales help you to better deal with what you see. This is partly because you are likely to be surprised by the things you did not anticipate. There is almost always something that will surprise you because your predictions will almost always be incomplete or too general. This sensitivity to the unanticipated—the sense of surprise that sparks your curiosity—is an indication of your openness to the phenomenon you are studying.
Making Your Predictions Explicit Allows You to Plan in Advance
Recall from Chap. 1 the descriptor of scientific inquiry: “Experience carefully planned in advance.” If you make no predictions about what might happen, it is very difficult, if not impossible, to plan your study in advance. Again, you cannot observe everything, so you must make decisions about what you will observe. What kind of data will you plan to collect? Why would you collect these data instead of others? If you have no idea what to expect, on what basis will you make these consequential decisions? Even if your predictions are vague and your rationales for the predictions are a bit shaky, at least they provide a direction for your plan. They allow you to explain why you are planning this study and collecting these data. They allow you to “carefully plan in advance.”
Making Your Predictions Explicit Allows You to Put Your Rationales in Harm’s Way
Rationales are developed to justify the predictions. Rationales represent your best reasoning about the research problem you are studying. How can you tell whether your reasoning is sound? You can try it out with colleagues. However, the best way to test it is to put it in “harm’s way” (Cobb, Confrey, diSessa, Lehrer, & Schauble, 2003 p. 10). And the best approach to putting your reasoning in harm’s way is to test the predictions it generates. Regardless if you are conducting a qualitative or quantitative study, rationales can be improved only if they generate testable predictions. This is possible only if predictions are explicit and precise. As we described earlier, rationales are evaluated for their soundness and refined in light of the specific differences between predictions and empirical observations.
Making Your Predictions Explicit Forces You to Organize and Extend Your (and the Field’s) Thinking
By writing out your predictions (even hunches or fuzzy guesses) and by reflecting on why you have these predictions and making these reasons explicit for yourself, you are advancing your thinking about the questions you really want to answer. This means you are making progress toward formulating your research questions and your final hypotheses. Making more progress in your own thinking before you conduct your study increases the chances your study will be of higher quality and will be exactly the study you intended. Making predictions, developing rationales, and imagining tests are tools you can use to push your thinking forward before you even collect data.
Suppose you wonder how preservice teachers in your university’s teacher preparation program will solve particular kinds of math problems. You are interested in this question because you have noticed several PSTs solve them in unexpected ways. As you ask the question you want to answer, you make predictions about what you expect to see. When you reflect on why you made these predictions, you realize that some PSTs might use particular solution strategies because they were taught to use some of them in an earlier course, and they might believe you expect them to solve the problems in these ways. By being explicit about why you are making particular predictions, you realize that you might be answering a different question than you intend (“How much do PSTs remember from previous courses?” or even “To what extent do PSTs believe different instructors have similar expectations?”). Now you can either change your question or change the design of your study (i.e., the sample of students you will use) or both. You are advancing your thinking by being explicit about your predictions and why you are making them.
The Costs of Not Making Predictions
Avoiding making predictions, for whatever reason, comes with significant costs. It prevents you from learning very much about your research topic. It would require not reading related research, not talking with your colleagues, and not conducting pilot studies because, if you do, you are likely to find a prediction creeping into your thinking. Not doing these things would forego the benefits of advancing your thinking before you collect data. It would amount to conducting the study with as little forethought as possible.
Part VI. How Do You Formulate Important Hypotheses?
We provided a partial answer in Chap. 1 to the question of a hypothesis’ importance when we encouraged considering the ultimate goal to which a study’s findings might contribute. You might want to reread Part III of Chap. 1 where we offered our opinions about the purposes of doing research. We also recommend reading the March 2019 editorial in the Journal for Research in Mathematics Education (Cai et al., 2019b ) in which we address what constitutes important educational research.
As we argued in Chap. 1 and in the March 2019 editorial, a worthy ultimate goal for educational research is to improve the learning opportunities for all students. However, arguments can be made for other ultimate goals as well. To gauge the importance of your hypotheses, think about how clearly you can connect them to a goal the educational community considers important. In addition, given the descriptors of scientific inquiry proposed in Chap. 1 , think about how testing your hypotheses will help you (and the community) understand what you are studying. Will you have a better explanation for the phenomenon after your study than before?
Although we address the question of importance again, and in more detail, in Chap. 5 , it is useful to know here that you can determine the significance or importance of your hypotheses when you formulate them. The importance need not depend on the data you collect or the results you report. The importance can come from the fact that, based on the results of your study, you will be able to offer revised hypotheses that help the field better understand an important issue. In large part, it is these revised hypotheses rather than the data that determine a study’s importance.
A critical caveat to this discussion is that few hypotheses are self-evidently important. They are important only if you make the case for their importance. Even if you follow closely the guidelines we suggest for formulating an important hypothesis, you must develop an argument that convinces others. This argument will be presented in the research paper you write.

Consider Martha’s hypothesis presented earlier. When we left Martha, she predicted that “Participating teachers will show changes in their teaching with a greater emphasis on conceptual understanding with larger changes on linear function topics directly addressed in the LOs than on other topics.” For researchers and educators not intimately familiar with this area of research, it is not apparent why someone should spend a year or more conducting a dissertation to test this prediction. Her rationale, summarized earlier, begins to describe why this could be an important hypothesis. But it is by writing a clear argument that explains her rationale to readers that she will convince them of its importance.
How Martha fills in her rationale so she can create a clear written argument for its importance is taken up in Chap. 3 . As we indicated, Martha’s work in this regard led her to make some interesting decisions, in part due to her own assessment of what was important.
Part VII. Beginning to Write the Research Paper for Your Study
It is common to think that researchers conduct a study and then, after the data are collected and analyzed, begin writing the paper about the study. We recommend an alternative, especially for beginning researchers. We believe it is better to write drafts of the paper at the same time you are planning and conducting your study. The paper will gradually evolve as you work through successive phases of the scientific inquiry process. Consequently, we will call this paper your evolving research paper .

You will use your evolving research paper to communicate your study, but you can also use writing as a tool for thinking and organizing your thinking while planning and conducting the study. Used as a tool for thinking, you can write drafts of your ideas to check on the clarity of your thinking, and then you can step back and reflect on how to clarify it further. Be sure to avoid jargon and general terms that are not well defined. Ask yourself whether someone not in your field, maybe a sibling, a parent, or a friend, would be able to understand what you mean. You are likely to write multiple drafts with lots of scribbling, crossing out, and revising.
Used as a tool for communicating, writing the best version of what you know before moving to the next phase will help you record your decisions and the reasons for them before you forget important details. This best-version-for-now paper also provides the basis for your thinking about the next phase of your scientific inquiry.
At this point in the process, you will be writing your (research) questions, the answers you predict, and the rationales for your predictions. The predictions you make should be direct answers to your research questions and should flow logically from (or be directly supported by) the rationales you present. In addition, you will have a written statement of the study’s purpose or, said another way, an argument for the importance of the hypotheses you will be testing. It is in the early sections of your paper that you will convince your audience about the importance of your hypotheses.
In our experience, presenting research questions is a more common form of stating the goal of a research study than presenting well-formulated hypotheses. Authors sometimes present a hypothesis, often as a simple prediction of what they might find. The hypothesis is then forgotten and not used to guide the analysis or interpretations of the findings. In other words, authors seldom use hypotheses to do the kind of work we describe. This means that many research articles you read will not treat hypotheses as we suggest. We believe these are missed opportunities to present research in a more compelling and informative way. We intend to provide enough guidance in the remaining chapters for you to feel comfortable organizing your evolving research paper around formulating, testing, and revising hypotheses.
While we were editing one of the leading research journals in mathematics education ( JRME ), we conducted a study of reviewers’ critiques of papers submitted to the journal. Two of the five most common concerns were: (1) the research questions were unclear, and (2) the answers to the questions did not make a substantial contribution to the field. These are likely to be major concerns for the reviewers of all research journals. We hope the knowledge and skills you have acquired working through this chapter will allow you to write the opening to your evolving research paper in a way that addresses these concerns. Much of the chapter should help make your research questions clear, and the prior section on formulating “important hypotheses” will help you convey the contribution of your study.
Exercise 2.3
Look back at your answers to the sets of questions before part II of this chapter.
Think about how you would argue for the importance of your current interest.
Write your interest in the form of (1) a research problem, (2) a research question, and (3) a prediction with the beginnings of a rationale. You will update these as you read the remaining chapters.
Part VIII. The Heart of Scientific Inquiry
In this chapter, we have described the process of formulating hypotheses. This process is at the heart of scientific inquiry. It is where doing research begins. Conducting research always involves formulating, testing, and revising hypotheses. This is true regardless of your research questions and whether you are using qualitative, quantitative, or mixed methods. Without engaging in this process in a deliberate, intense, relentless way, your study will reveal less than it could. By engaging in this process, you are maximizing what you, and others, can learn from conducting your study.
In the next chapter, we build on the ideas we have developed in the first two chapters to describe the purpose and nature of theoretical frameworks . The term theoretical framework, along with closely related terms like conceptual framework, can be somewhat mysterious for beginning researchers and can seem like a requirement for writing a paper rather than an aid for conducting research. We will show how theoretical frameworks grow from formulating hypotheses—from developing rationales for the predicted answers to your research questions. We will propose some practical suggestions for building theoretical frameworks and show how useful they can be. In addition, we will continue Martha’s story from the point at which we paused earlier—developing her theoretical framework.
Cai, J., Morris, A., Hohensee, C., Hwang, S., Robison, V., Cirillo, M., Kramer, S. L., & Hiebert, J. (2019b). Posing significant research questions. Journal for Research in Mathematics Education, 50 (2), 114–120. https://doi.org/10.5951/jresematheduc.50.2.0114
Article Google Scholar
Carpenter, T. P., Fennema, E., Peterson, P. L., Chiang, C. P., & Loef, M. (1989). Using knowledge of children’s mathematics thinking in classroom teaching: An experimental study. American Educational Research Journal, 26 (4), 385–531.
Fennema, E., Carpenter, T. P., Franke, M. L., Levi, L., Jacobs, V. R., & Empson, S. B. (1996). A longitudinal study of learning to use children’s thinking in mathematics instruction. Journal for Research in Mathematics Education, 27 (4), 403–434.
Glaser, B. G., & Holton, J. (2004). Remodeling grounded theory. Forum: Qualitative Social Research, 5(2). https://www.qualitative-research.net/index.php/fqs/article/view/607/1316
Gournelos, T., Hammonds, J. R., & Wilson, M. A. (2019). Doing academic research: A practical guide to research methods and analysis . Routledge.
Book Google Scholar
Hohensee, C. (2014). Backward transfer: An investigation of the influence of quadratic functions instruction on students’ prior ways of reasoning about linear functions. Mathematical Thinking and Learning, 16 (2), 135–174.
Husserl, E. (1973). Cartesian meditations: An introduction to phenomenology (D. Cairns, Trans.). Martinus Nijhoff. (Original work published 1929).
Google Scholar
Levitt, H. M., Bamberg, M., Creswell, J. W., Frost, D. M., Josselson, R., & Suárez-Orozco, C. (2018). Journal article reporting standards for qualitative primary, qualitative meta-analytic, and mixed methods research in psychology: The APA Publications and Communications Board Task Force report. American Psychologist, 73 (1), 26–46.
Medawar, P. (1982). Pluto’s republic [no typo]. Oxford University Press.
Merton, R. K. (1968). Social theory and social structure (Enlarged edition). Free Press.
Nemirovsky, R. (2011). Episodic feelings and transfer of learning. Journal of the Learning Sciences, 20 (2), 308–337. https://doi.org/10.1080/10508406.2011.528316
Vygotsky, L. (1987). The development of scientific concepts in childhood: The design of a working hypothesis. In A. Kozulin (Ed.), Thought and language (pp. 146–209). The MIT Press.
Download references
Author information
Authors and affiliations.
School of Education, University of Delaware, Newark, DE, USA
James Hiebert, Anne K Morris & Charles Hohensee
Department of Mathematical Sciences, University of Delaware, Newark, DE, USA
Jinfa Cai & Stephen Hwang
You can also search for this author in PubMed Google Scholar
Rights and permissions
Open Access This chapter is licensed under the terms of the Creative Commons Attribution 4.0 International License ( http://creativecommons.org/licenses/by/4.0/ ), which permits use, sharing, adaptation, distribution and reproduction in any medium or format, as long as you give appropriate credit to the original author(s) and the source, provide a link to the Creative Commons license and indicate if changes were made.
The images or other third party material in this chapter are included in the chapter's Creative Commons license, unless indicated otherwise in a credit line to the material. If material is not included in the chapter's Creative Commons license and your intended use is not permitted by statutory regulation or exceeds the permitted use, you will need to obtain permission directly from the copyright holder.
Reprints and permissions
Copyright information
© 2023 The Author(s)
About this chapter
Hiebert, J., Cai, J., Hwang, S., Morris, A.K., Hohensee, C. (2023). How Do You Formulate (Important) Hypotheses?. In: Doing Research: A New Researcher’s Guide. Research in Mathematics Education. Springer, Cham. https://doi.org/10.1007/978-3-031-19078-0_2
Download citation
DOI : https://doi.org/10.1007/978-3-031-19078-0_2
Published : 03 December 2022
Publisher Name : Springer, Cham
Print ISBN : 978-3-031-19077-3
Online ISBN : 978-3-031-19078-0
eBook Packages : Education Education (R0)
Share this chapter
Anyone you share the following link with will be able to read this content:
Sorry, a shareable link is not currently available for this article.
Provided by the Springer Nature SharedIt content-sharing initiative
- Publish with us
Policies and ethics
- Find a journal
- Track your research

MCQs on Hypothesis Testing
This section of Data Science questions and answers include MCQs on Hypothesis Testing. Hypothesis testing is a fundamental statistical concept used to make inferences about a population based on a sample of data. The Hypothesis Testing MCQs address various aspects of hypothesis testing, including definitions, types of errors, significance levels, p-values, effect size, critical regions, and specific tests like t-tests and ANOVA.
1. What is the null hypothesis in a statistical hypothesis test?
a) The hypothesis that there is a significant difference
b) The hypothesis that there is no significant difference
c) The hypothesis that only one variable is involved
d) The hypothesis that the data is perfectly normal
Answer: b) The hypothesis that there is no significant difference
Answer Explanation: The null hypothesis assumes no effect or no difference until evidence suggests otherwise.
2. In hypothesis testing, what is the p-value?
a) The probability of making a Type I error
b) The probability of making a Type II error
c) The probability of observing the data if the null hypothesis is true
d) The probability of rejecting the null hypothesis when it is true
Answer: c) The probability of observing the data if the null hypothesis is true
Answer Explanation: A lower p-value suggests stronger evidence against the null hypothesis.
3. What is a Type I error in the context of hypothesis testing?
a) Incorrectly rejecting a true null hypothesis
b) Incorrectly accepting a false null hypothesis
c) Correctly rejecting a true null hypothesis
d) Correctly accepting a false null hypothesis
Answer: a) Incorrectly rejecting a true null hypothesis
Answer Explanation: Type I error occurs when we falsely conclude that there is an effect when there isn’t.
4. What does the term ‘statistical power’ refer to?
c) The probability of correctly rejecting a false null hypothesis
d) The probability of correctly accepting a true null hypothesis
Answer: c) The probability of correctly rejecting a false null hypothesis
Answer Explanation: Statistical power is the ability to detect a true effect when it exists.
5. Which statistical test is appropriate for comparing means of two independent groups?
b) Chi-square test
d) Pearson correlation
Answer: a) t-test
Answer Explanation: The t-test is used for comparing means of two groups.
6. What is the purpose of the p-value in hypothesis testing?
a) To determine the effect size
b) To quantify the strength of evidence against the null hypothesis
c) To establish causation
d) To calculate the confidence interval
Answer: b) To quantify the strength of evidence against the null hypothesis
Answer Explanation: A lower p-value indicates stronger evidence against the null hypothesis.
7. In a two-tailed hypothesis test, when do you reject the null hypothesis?
a) If the test statistic is too small
b) If the test statistic is too large
c) If the p-value is too small or too large
d) If the p-value is exactly 0.05
Answer: c) If the p-value is too small or too large
Answer Explanation: In a two-tailed test, extreme values in either direction lead to rejection.
8. What is the critical region in hypothesis testing?
a) The range of values where the null hypothesis is likely true
b) The range of values where the null hypothesis is likely false
c) The range of values that leads to rejection of the null hypothesis
d) The range of values that leads to acceptance of the null hypothesis
Answer: c) The range of values that leads to rejection of the null hypothesis
Answer Explanation: The critical region is determined based on the chosen significance level.
9. Which of the following statements about the significance level (α) is correct?
a) It is the probability of rejecting the null hypothesis when it is true
b) It is the probability of accepting the null hypothesis when it is false
c) It is chosen by the researcher and represents the maximum tolerable probability of Type I error
d) It is fixed at 0.05 for all hypothesis tests
Answer: c) It is chosen by the researcher and represents the maximum tolerable probability of Type I error
Answer Explanation: The significance level is a user-defined threshold for the probability of Type I error.
10. What does a confidence interval represent?
c) The range of values that includes the true population parameter with a certain level of confidence
d) The range of values that leads to rejection of the null hypothesis
Answer: c) The range of values that includes the true population parameter with a certain level of confidence
Answer Explanation: A confidence interval provides an estimated range of likely values for a population parameter.
Data Science MCQs on Hypothesis Testing
11. what is the difference between type i and type ii errors in hypothesis testing.
a) Type I error is rejecting a true null hypothesis, while Type II error is accepting a false null hypothesis
b) Type I error is accepting a false null hypothesis, while Type II error is rejecting a true null hypothesis
c) Both Type I and Type II errors involve incorrectly accepting the null hypothesis
d) Both Type I and Type II errors involve incorrectly rejecting the null hypothesis
Answer: a) Type I error is rejecting a true null hypothesis, while Type II error is accepting a false null hypothesis
Answer Explanation: Type I error involves a false positive, while Type II error involves a false negative.
12. In a one-sample t-test, if the p-value is 0.02, what can you conclude?
a) Reject the null hypothesis at a 0.05 significance level
b) Fail to reject the null hypothesis at a 0.05 significance level
c) The null hypothesis is true
d) The test is inconclusive
Answer: a) Reject the null hypothesis at a 0.05 significance level
Answer Explanation: A p-value of 0.02 is less than 0.05, providing evidence to reject the null hypothesis.
13. What is the purpose of the Bonferroni correction in multiple comparisons?
a) To increase the chance of Type I errors
b) To decrease the chance of Type I errors
c) To increase the chance of Type II errors
d) To decrease the chance of Type II errors
Answer: b) To decrease the chance of Type I errors
Answer Explanation: The Bonferroni correction helps control the familywise error rate in multiple comparisons.
14. What is a critical region in a hypothesis test?
c) The range of values that leads to acceptance of the null hypothesis
d) The range of values that leads to neither rejection nor acceptance of the null hypothesis
Answer: b) The range of values where the null hypothesis is likely false
Answer Explanation: The critical region is where the null hypothesis is rejected in favor of the alternative hypothesis.
15. What is the purpose of an effect size measure in hypothesis testing?
a) To determine the statistical power of the test
b) To quantify the strength of a significant result
Answer: b) To quantify the strength of a significant result
Answer Explanation: Effect size helps assess the practical significance of a significant result.
16. Fill in the blank: The ________ is the probability of correctly failing to reject a false null hypothesis.
b) Significance level
c) Type I error rate
d) Type II error rate
Answer: d) Type II error rate
Answer Explanation: Type II error is the probability of accepting a false null hypothesis.
17. In a two-sample t-test, what assumption is made about the variances of the two groups?
a) They must be equal
b) They must be different
c) Variance assumption is not relevant in a t-test
d) The larger variance must be known
Answer: a) They must be equal
Answer Explanation: The assumption of equal variances is crucial for the validity of the t-test.
18. Which statistical test is appropriate for comparing means of more than two independent groups?
a) Paired t-test
b) Wilcoxon signed-rank test
d) Mann-Whitney U test
Answer: c) ANOVA
Answer Explanation: ANOVA is used for comparing means of more than two groups.
19. What is the formula for the standard error of the mean in a one-sample t-test?
a) \( \frac{s}{\sqrt{n}} \)
b) \( \frac{s}{n} \)
c) \( \frac{\sigma}{\sqrt{n}} \)
d) \( \frac{\sigma}{n} \)
Answer: a) \( \frac{s}{\sqrt{n}} \)
Answer Explanation: The standard error of the mean in a t-test is calculated as the sample standard deviation divided by the square root of the sample size.
20. What is the purpose of the Shapiro-Wilk test in hypothesis testing?
a) To test the equality of variances
b) To test the normality of the data
c) To test the independence of observations
d) To test the homogeneity of regression slopes
Answer: b) To test the normality of the data
Answer Explanation: The Shapiro-Wilk test assesses whether a sample comes from a normally distributed population.
MCQBase Learning Series – Data Science
To practice and learn all the minute nuances of Data Science, here is the complete list of 1000+ Data Science MCQs . Multiple-choice questions on Data Science are conveniently indexed in Chapters and Topics for easy learning.
© 2023 MCQ Base. All Rights Reserved.
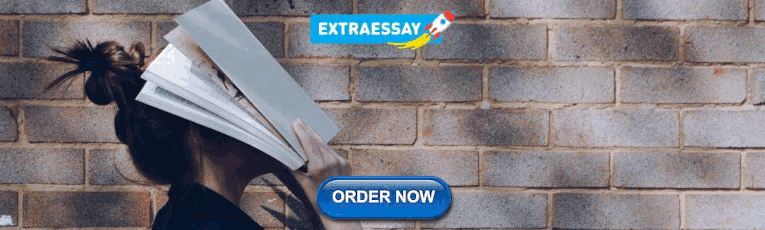
IMAGES
VIDEO
COMMENTS
Developing a hypothesis (with example) Step 1. Ask a question. Writing a hypothesis begins with a research question that you want to answer. The question should be focused, specific, and researchable within the constraints of your project. Example: Research question.
Step 5: Phrase your hypothesis in three ways. To identify the variables, you can write a simple prediction in if … then form. The first part of the sentence states the independent variable and the second part states the dependent variable. If a first-year student starts attending more lectures, then their exam scores will improve.
3 Define your variables. Once you have an idea of what your hypothesis will be, select which variables are independent and which are dependent. Remember that independent variables can only be factors that you have absolute control over, so consider the limits of your experiment before finalizing your hypothesis.
Then you will have everything you need to formulate your hypothesis. Formulate the Hypothesis; Based on your research question and preliminary research, now you can create your hypothesis. A good hypothesis should be clear, concise, and testable. It typically takes a statement form, predicting a potential outcome or relationship between variables.
Study with Quizlet and memorize flashcards containing terms like What is the first step in before forming a hypothesis?, What should you do to test a hypothesis?, Indipendent variable and more.
Forming a Hypothesis. The next step in a scientific investigation is forming a hypothesis.A hypothesis is a possible answer to a scientific question, but it isn't just any answer. A hypothesis must be based on scientific knowledge, and it must be logical. A hypothesis also must be falsifiable. In other words, it must be possible to make observations that would disprove the hypothesis if it ...
The scientific method. At the core of biology and other sciences lies a problem-solving approach called the scientific method. The scientific method has five basic steps, plus one feedback step: Make an observation. Ask a question. Form a hypothesis, or testable explanation. Make a prediction based on the hypothesis.
Table of contents. Step 1: State your null and alternate hypothesis. Step 2: Collect data. Step 3: Perform a statistical test. Step 4: Decide whether to reject or fail to reject your null hypothesis. Step 5: Present your findings. Other interesting articles. Frequently asked questions about hypothesis testing.
First, a good hypothesis must be testable and falsifiable. We must be able to test the hypothesis using the methods of science and if you'll recall Popper's falsifiability criterion, it must be possible to gather evidence that will disconfirm the hypothesis if it is indeed false. Second, a good hypothesis must be logical.
Forming a Hypothesis. When conducting scientific experiments, researchers develop hypotheses to guide experimental design. A hypothesis is a suggested explanation that is both testable and falsifiable. You must be able to test your hypothesis, and it must be possible to prove your hypothesis true or false.
Hypothesis Question 3 Detailed Solution. The correct answer is When there is no difference between the variables. Key Points. A null hypothesis, often denoted as "H0," is a fundamental concept in statistics and hypothesis testing. It represents a statement or assumption that there is no significant difference or effect.
The steps to write a research hypothesis are: 1. Stating the problem: Ensure that the hypothesis defines the research problem. 2. Writing a hypothesis as an 'if-then' statement: Include the action and the expected outcome of your study by following a 'if-then' structure. 3.
What is a hypothesis and how can you write a great one for your research? A hypothesis is a tentative statement about the relationship between two or more variables that can be tested empirically. Find out how to formulate a clear, specific, and testable hypothesis with examples and tips from Verywell Mind, a trusted source of psychology and mental health information.
Examples of Hypothesis. Here are a few examples of hypotheses in different fields: Psychology: "Increased exposure to violent video games leads to increased aggressive behavior in adolescents.". Biology: "Higher levels of carbon dioxide in the atmosphere will lead to increased plant growth.".
Step 3: Build the Hypothetical Relationship. In understanding how to compose a hypothesis, constructing the relationship between the variables is key. Based on your research question and variables, predict the expected outcome or connection.
tested by scientific experiment. Suppose you study how speed affects the braking distance of a car. Match each part of the hypothesis phase with its output. 1. Formulate the hypothesis - Braking distance grows proportionally with speed. 2. Choose a scope - We will focus on how braking distance depends on speed. 3.
Building on the ideas in Chap. 1, we describe formulating, testing, and revising hypotheses as a continuing cycle of clarifying what you want to study, making predictions about what you might find together with developing your reasons for these predictions, imagining tests of these predictions, revising your predictions and rationales, and so ...
Design an experiment. Collect/analyze data. Draw conclusion. Data. Quantitive data. Qualitative data. Data. Questions to ask. Study with Quizlet and memorize flashcards containing terms like Observation / asking questions, Form a hypothesis, Design an experiment and more.
7. In a two-tailed hypothesis test, when do you reject the null hypothesis? a) If the test statistic is too small. b) If the test statistic is too large. c) If the p-value is too small or too large. d) If the p-value is exactly 0.05. View Answer. Answer: c) If the p-value is too small or too large
replicate. Replication is important in science because. It can help validate claims of research to be true. It can help prove research claims to be false. It can help a hypothesis become a theory. Getting the same result when an experiment is repeated is called _____. replication, repeated trials, repeat experiments.
1. Identify a topic. Be the first to add your personal experience. 2. Narrow your focus. Be the first to add your personal experience. 3. Generate a hypothesis. Be the first to add your personal ...
Alice_Stein25. Preview. Study with Quizlet and memorize flashcards containing terms like _________ is the first step in the scientific method., What should you do before you form a hypothesis?, Which variable is manipulated to cause a change in a measured characteristic? and more.
6 Descriptive studies may not have a specific hypothesis but rather have a purpose statement. 7 The methods section is how the study was done and should include enough detail for reviewers to ...