- Data Management Plans: A Comprehensive Guide for Researchers
This guide outlines the main areas that researchers should consider when preparing a Data Management Plan (DMP). It includes information on the data specification, institutional repositories, long-term preservation services, IT infrastructure requirements and more.

Data Management Plans (DMPs) are documents prepared by researchers as they plan a project and write a grant proposal. These plans provide an outline of the types of data that will be collected, the data format, including data and metadata standards, and how the data will be handled throughout the project lifecycle. Many research funders require a data management plan with a grant proposal, so it is important for researchers to understand the main areas to consider when preparing one. This guide outlines the main areas that researchers should consider when preparing a data management plan.
It includes information on the data specification, including size, file format , number of files, data dictionary, and codebook. It also covers the use of institutional data repositories hosted in university libraries as an open access platform for the dissemination and archiving of university research data. Additionally, it provides information on long-term preservation of digital data files using services such as migration (limited format types), secure backups, bit-level checksums, and maintaining a persistent DOI for data sets. When a project includes data about people and organizations, this affects the design of the necessary IT infrastructure.
It is expected that the full dataset will be accessible after the study and all related publications are completed, and will remain accessible for at least 10 years after the data become publicly available. This section includes a description of the format of your data and how it will be created, collected, maintained and delivered. In addition, information from individuals or entities that own the intellectual property rights to the data should be included in this section. SPARC (the Academic Resources and Academic Publications Coalition) has compiled an excellent resource with information on the data management and data exchange requirements of all federal funding agencies.
ICPSR allows several mediated forms of exchange, where individuals interested in obtaining a less de-identified individual level would sign data use agreements before receiving the data, or would need to use special software to access it directly from ICPSR instead of downloading it, for security reasons. Some demographics may not be shareable on an individual level and would therefore only be provided in aggregate form. Finally, all applicants submitting funding proposals to the MRC must include a Data Management Plan (DMP) as an integral part of the application. Through DRUM policies, unidentified data will be accompanied by appropriate documentation, metadata and code to facilitate reuse and provide the potential for interoperability with similar datasets. The main benefit of DRUM is that everything shared through this repository is public; however, a fully open system is not optimal if any of the data can be identified.
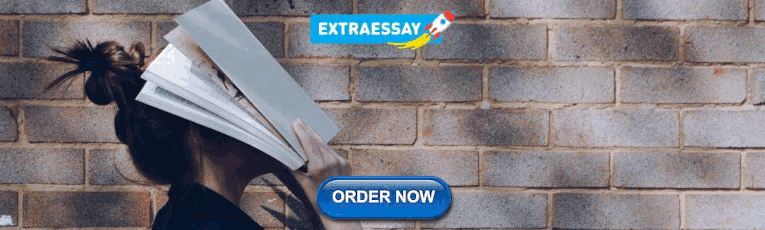
Related Posts

- Types of Management Tools: A Comprehensive Guide
Business management tools are essential for organizations to stay competitive in the market and improve their performance. This article provides an overview of the different types of management tools, their features, and how to choose the right one for your busines

- Do You Need Math to Become a Data Scientist?
Do you need math skills to become a successful Data Scientist? Learn more about what it takes to become a Data Scientist and how BloomTech can help.

- Organizing IoT Device Data: A Comprehensive Guide
Organizing IoT device data requires careful planning and consideration in order to ensure that all components are working together seamlessly. Learn more about how businesses can successfully design and implement an IoT project.

- The Significance of Data Management in Mathematics
Data management plays an essential role in today's digital economy. Learn why it's important and how it can help you get a successful job.
More articles

- Data Management Services: A Comprehensive Guide

- What are data standards and interoperability?

- 6 Major Areas for Best Practices in Data Management

- Unlocking the Secrets of Big Data Analytics: A Comprehensive Guide
- What is Data Services and How Does it Work?
- Data Management: A Comprehensive Guide
- The Benefits of Using Management Tools for Businesses
- What is data processing and its types?
- The Benefits of Data Management for Businesses
- How is big data used in biology?
- Why is tools management important?
- The Benefits of Data Management in Biology
- What is managing the data?
- What is Data Management Software and How Does it Work?
- What are data management skills?
- Why is data management and analysis important?
- The Essential Role of Data Management in Business
- What is Enterprise Data Management and How to Implement It?
- Data Management Tools: A Comprehensive Guide
- Who created data management?
- Data Management: What It Is and Examples
- The Ultimate Guide to Data Management
- Data Management: What You Need to Know
- Data Management: What is it and How to Use it?
- Data Collection Methods: An Expert's Guide
- The Benefits of Enterprise Data Management for Every Enterprise
- The Difference Between Enterprise Data Management and Data Management
- What is a value in data management?
- Data Management: Functions, Storage, Security and More
- Types of Data Management Functions Explained
- The 10 Principles of Data Management for a Successful Organization
- Four Essential Best Practices for Big Data Governance
Data Management in Research: A Comprehensive Guide
- The Benefits of Understanding Project Data and Processes
- Data Management Services: What You Need to Know
- The Benefits of Research Data Management
- Which of the following is api management tool?
- A Comprehensive Guide to Data Management Plans
- The Benefits of Data Management in Research
- What are the data management principles?
- What is the Role of Data Management in Organizations?
- Data Management in Quantitative Research: An Expert's Guide
- What are spreadsheet data tools?
- What are the 5 basic steps in data analysis?
- 8 Core Principles of Data Management
- How does data governance add value?
- What is Enterprise Data Management and How Can It Help Your Organization?
- The Advantages of Enterprise Data Management
- Data Management: A Comprehensive Guide for Researchers
- A Comprehensive Guide to Database Management Systems
- Data Management Tools: An Expert's Guide
- Data Management: Unlocking the Power of Data Analysis
What is the Purpose of a Data Management Plan?
- Types of Data Management Tools: A Comprehensive Guide
- What is Data Management and How to Manage it Effectively?
- Data Management: Unlocking the Potential of Data
- What are the 3 dimensions of data governance defined in the active data governance framework?
- Data Management Standards: A Comprehensive Guide
- What are the 5 importance of data processing?
- Data Management: A Comprehensive Guide to Activities Involved
- Six Major Areas for Best Practices in Data Management
- What software create and manage data base?
- Data Management: An Overview of the 5 Key Functions
- The Ultimate Guide to Enterprise Data Management
- What should a data management plan include?
- 4 Types of Data Management Explained
- 10 Steps to Master Data Management
- 9 Best Practices of Master Data Management
- Who will approve data management plan?
- The Benefits of Data Management: Unlocking the Power of Your Data
- What is Enterprise Data Management Framework?
- The Benefits of Good Data Management Explained
- The Benefits of Data Management Tools
- Data Management: Unlocking the Potential of Your Data
- Best Practices for Managing Big Data Analytics Programs
- Is sql a database management system?
- Data Processing: Exploring the 3 Main Methods
- Data Management Softwares: A Comprehensive Guide
- What tools are used in data analysis?
- What are the best practices in big data adoption?
- What is a data management tool?
- The Essential Role of Data Management in the Digital Economy
- What Are the Different Types of Management Tools?
- What is a data standard?
- The Benefits of Data Management: Unlocking the Value of Data
New Articles

Which cookies do you want to accept?
Thank you for visiting nature.com. You are using a browser version with limited support for CSS. To obtain the best experience, we recommend you use a more up to date browser (or turn off compatibility mode in Internet Explorer). In the meantime, to ensure continued support, we are displaying the site without styles and JavaScript.
- View all journals
- Explore content
- About the journal
- Publish with us
- Sign up for alerts
- CAREER FEATURE
- 13 March 2018
Data management made simple
- Quirin Schiermeier
You can also search for this author in PubMed Google Scholar
When Marjorie Etique learnt that she had to create a data-management plan for her next research project, she was not sure exactly what to do.
Access options
Access Nature and 54 other Nature Portfolio journals
Get Nature+, our best-value online-access subscription
24,99 € / 30 days
cancel any time
Subscribe to this journal
Receive 51 print issues and online access
185,98 € per year
only 3,65 € per issue
Rent or buy this article
Prices vary by article type
Prices may be subject to local taxes which are calculated during checkout
Nature 555 , 403-405 (2018)
doi: https://doi.org/10.1038/d41586-018-03071-1
See Editorial: Everyone needs a data-management plan
Related Articles
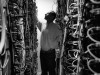
The FAIR Guiding Principles for scientific data management and stewardship
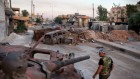
How I fled bombed Aleppo to continue my career in science
Career Feature 08 MAY 24
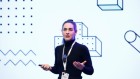
Illuminating ‘the ugly side of science’: fresh incentives for reporting negative results
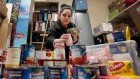
Hunger on campus: why US PhD students are fighting over food
Career Feature 03 MAY 24
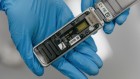
Powerful ‘nanopore’ DNA sequencing method tackles proteins too
Technology Feature 08 MAY 24
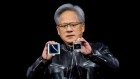
Who’s making chips for AI? Chinese manufacturers lag behind US tech giants
News 03 MAY 24
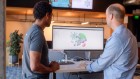
85 million cells — and counting — at your fingertips
Technology Feature 29 APR 24
Research Positions in China Spallation Neutron Source
We are seeking 23 researchers with a proven track record of conducting advanced research and demonstrating outstanding academic achievements.
Dongguan, Guangdong, China
Spallation Neutron Source Science Center
Postdoctoral Associate- Electrophysiology
Houston, Texas (US)
Baylor College of Medicine (BCM)
Postdoctoral Scholar - Clinical Pharmacy & Translational Science
Memphis, Tennessee
The University of Tennessee Health Science Center (UTHSC)
Postdoctoral Scholar - Pathology
Faculty Positions in School of Engineering, Westlake University
The School of Engineering (SOE) at Westlake University is seeking to fill multiple tenured or tenure-track faculty positions in all ranks.
Hangzhou, Zhejiang, China
Westlake University
Sign up for the Nature Briefing newsletter — what matters in science, free to your inbox daily.
Quick links
- Explore articles by subject
- Guide to authors
- Editorial policies
Ask Yale Library
My Library Accounts
Find, Request, and Use
Help and Research Support
Visit and Study
Explore Collections
Research Data Management: Plan for Data
- Plan for Data
- Organize & Document Data
- Store & Secure Data
- Validate Data
- Share & Re-use Data
- Data Use Agreements
- Research Data Policies
What is a Data Management Plan?
Data management plans (DMPs) are documents that outline how data will be collected , stored , secured , analyzed , disseminated , and preserved over the lifecycle of a research project. They are typically created in the early stages of a project, and they are typically short documents that may evolve over time. Increasingly, they are required by funders and institutions alike, and they are a recommended best practice in research data management.
Tab through this guide to consider each stage of the research data management process, and each correlated section of a data management plan.
Tools for Data Management Planning
DMPTool is a collaborative effort between several universities to streamline the data management planning process.
The DMPTool supports the majority of federal and many non-profit and private funding agencies that require data management plans as part of a grant proposal application. ( View the list of supported organizations and corresponding templates.) If the funder you're applying to isn't listed or you just want to create one as good practice, there is an option for a generic plan.
Key features:
Data management plan templates from most major funders
Guided creation of a data management plan with click-throughs and helpful questions and examples
Access to public plans , to review ahead of creating your own
Ability to share plans with collaborators as well as copy and reuse existing plans
How to get started:
Log in with your yale.edu email to be directed to a NetID sign-in, and review the quick start guide .
Research Data Lifecycle
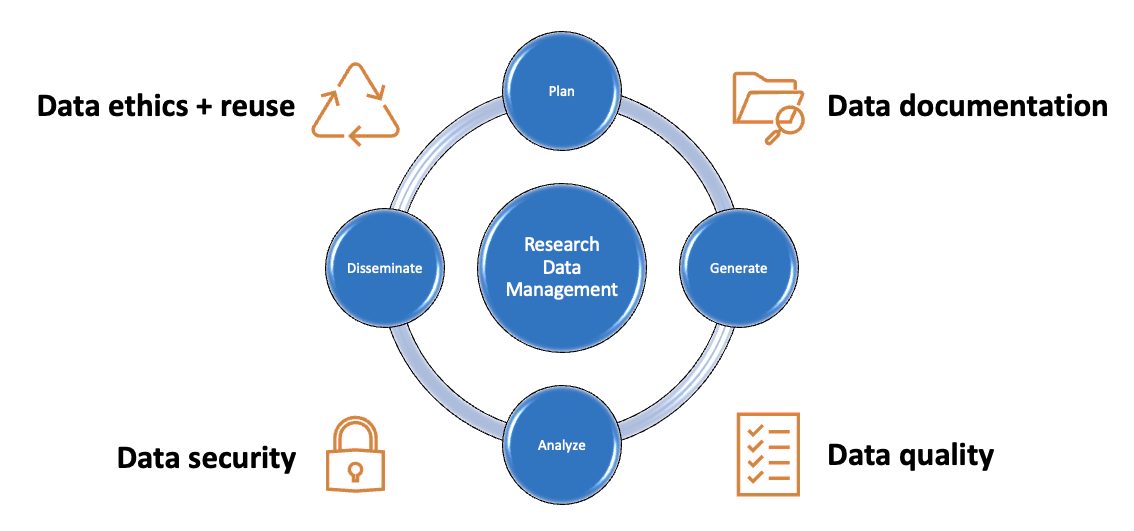
Additional Resources for Data Management Planning
- << Previous: Overview
- Next: Organize & Document Data >>
- Last Updated: Sep 27, 2023 1:15 PM
- URL: https://guides.library.yale.edu/datamanagement

Site Navigation
P.O. BOX 208240 New Haven, CT 06250-8240 (203) 432-1775
Yale's Libraries
Bass Library
Beinecke Rare Book and Manuscript Library
Classics Library
Cushing/Whitney Medical Library
Divinity Library
East Asia Library
Gilmore Music Library
Haas Family Arts Library
Lewis Walpole Library
Lillian Goldman Law Library
Marx Science and Social Science Library
Sterling Memorial Library
Yale Center for British Art
SUBSCRIBE TO OUR NEWSLETTER
@YALELIBRARY

Yale Library Instagram
Accessibility Diversity, Equity, and Inclusion Giving Privacy and Data Use Contact Our Web Team
© 2022 Yale University Library • All Rights Reserved

Research Data Management
- Finding Existing Data
- Data Citation
- Research Proposal
- RDM Requirements
- Collaboration
- Data Security
- GDPR & Privacy
- Data Management Plan
- Policies & Regulations
- Data Collection
- Data Storage
- Data Protection
- Data Provenance
- Data Processing
- Data Analysis
- High Performance Computing
- Selecting Data
- Data Documentation
- Selecting an Archive
- Data Publication
- Dataset Registration
Research Proposal / Grant Office
Grant programmes from organisations like NWO, ZonMW and ERC increasingly require you to not only think about the journey of the data in your research project, but also the method of data collection and how to protect or share data during and after the research project. It is important to bear in mind the specific laws and regulations that apply to the kind of data that is collected. If a project involves data on persons and organisations this impacts the design of the necessary IT infrastructure. A more detailed description of this will later be captured in the data management plan.
When writing your research proposal the following items are important:
- Fill in the data management paragraph (see the four questions below)
- Planning: one of the early deliverables will be a detailed data management plan
- Budget: take into account the costs (labour and material) for data storage during and data archiving after your project.
- Writing: Funders that distribute grants like to maximise the effectiveness of this investment. It is therefore highly recommended that the data will be made Findable, Accessible, Interoperable and Re-usable (FAIRdata). This does not mean that the data have to be open: laws, licenses and contracts regarding personal and sensitive data may limit the possibility to share the data publicly.
Research Data Services provides advice and help when writing a data paragraph as part of the Research proposal. The Library also regularly organises workshops to help you get started. Together with the VU Grants Office and project control we are part of the grant support team offering advice and practical aid for your grant. You will be directed to the specific unit during your support trajectory. Make sure to contact the team as early as possible.
Data Management Paragraph
In order to make data re-usable, funders require researchers to include a data section (= paragraph) in their project proposal, in which they explain whether research data will be collected or generated during the project, and how they plan to structure, archive and share their data. Depending on requirements of the funder, the paragraph can be short or more extensive.
Funders may have different requirements for the Data Management Paragraph in the project proposal. Always check what your funder asks for. For example, in 2016, the NWO formulated four questions that need to be answered in the data paragraph of the research proposal:
- Will data be collected or generated that are suitable for re-use?
- Where will the data be stored during the research?
- After the project has been completed, how will the data be stored for the long term and made available for the use by third parties? To whom will the data be accessible?
- Which facilities (ICT, (secure) archive, refrigerators or legal expertise) do you expect will be needed for the storage of data during the research and after the research? Are these available?
- << Previous: Plan & Design
- Next: RDM Costs >>
- Last Updated: Apr 24, 2024 3:47 PM
- URL: https://libguides.vu.nl/rdm
The Sheridan Libraries
- Data Management and Sharing
- Sheridan Libraries
- Write A Proposal
JHU Grant Support Services
Find funding, write a data management plan for data sharing requirements, manage and organize literature.
- Write a Data Management and Sharing Plan
- Human Participant Research Considerations
- JHU Policies for Data Management and Sharing
- Access and Collect Data
- Manage Data
- Analyze and Visualize Data
- Document Data
- Store and Preserve Data
- Overview of Data Sharing
- Prepare Your Data For Sharing
- How to Find a Data Repository
- Share Code or Software
- Conditions for Access and Reuse
JHU Research Administration Offices
The University has two divisional offices that support researchers in the grant proposal process:
School of Medicine Office of Research Administration
JHU Research Administration (JHURA) , supports research for Bloomberg School of Public Health, Carey Business School, Jhpiego, Nitze School of Advanced International Studies (SAIS), Krieger School of Arts and Sciences (KSAS), Peabody Institute, School of Education, School of Nursing, Whiting School of Engineering, and several other divisions and centers within Johns Hopkins University. JHURA holds Brown Bag Seminars regularly. Check out their current offerings and past recordings here .
WSE Office of Research and Translation
Whiting School of Engineering's Office of Research and Translation expands upon Research Administration support with the Commercial and Government Program Office (CGPO) that support faculty who are interested in pursuing large-scale grants and help researchers identify projects fall outside the scope of traditional sponsored research.
Funding Opportunities pages at JHU Research Administration and School of Medicine Office of Research Administration
- Grants.gov database of opportunities at all US Federal grant agencies
U.S. Federal funders, and many private funders, require making data associated with grants openly available for further research. Grant proposals may require data management plans (or Data Management and Sharing plans) that describe how the proposal will meet those requirements. Most funders require plans of up to two pages asking for particular topics and content.
Academic Liaisons of the Sheridan Libraries
The Academic Liaison librarians serve specific academic departments and programs by providing research support to faculty and students; instructing in the use of library resources; collecting library materials; and serving as liaisons between the libraries and their departments. You can find the librarian specific to your subject on their website .
Informationists of the Welch Medical Library
The embedded informationist service model comprises customized information services delivered where you are, at your points of research, teaching and clinical care. They conduct expert searches for various types of information (literature, funding, data, etc) to support research proposals and grants, clinical practice decisions, education instruction and article publication ( find your Informationist ). They also participate as guest instructors for formal and informal courses and act as on-site Informationists, providing input to discussions at departmental seminars, committees, case conferences and journal clubs ( register for a Welch class ). They can provide office hours for departments, collaborate on systematic review teams and offer information management instruction. Please see their main website for more information.
Reference and Citation Tools
This Sheridan Libraries’ LibGuide: Citing Resources suggests 12 citation management tools. Currently, RefWorks is free for all JHU users and librarians at the Sheridan Libraries provide support for JHU users. Zotero and Mendeley are another two popular citation management tools.
- << Previous: Plan and Design Research
- Next: Write a Data Management and Sharing Plan >>
- Last Updated: Mar 8, 2024 10:57 AM
- URL: https://guides.library.jhu.edu/dataservices
We use cookies on reading.ac.uk to improve your experience, monitor site performance and tailor content to you.
Read our cookie policy to find out how to manage your cookie settings.
This site may not work correctly on Internet Explorer. We recommend switching to a different browser for a better experience.
Writing a data management plan
Every research project that involves the collection and use of research data should have a data management plan (DMP). This is a structured document describing:
- what data will be collected or used in the course of a research project;
- how the data will be managed on a day-to-day basis;
- how relevant data will preserved for the long term and made available for re-use by others on completion of the research and publication of findings.
A DMP can enable you and your project team to work efficiently, to identify requirements and manage risks, and to apply appropriate solutions.
You may have been required to submit a DMP as part of a grant application , and this can be a useful starting point for project planning, but the DMP that is a practical instrument for your project will still need to be developed.
We provide a post-award data management guide (pdf) to help PIs and project teams address a project’s data management requirements when a project is being set up.
NERC and EC-funded researchers should be aware of post-award DMP requirements:
- NERC-funded PIs must contact the NERC Data Centre nominated on their Outline Data Management Plan within three to six months of the start date of the grant, in order to develop a full DMP for the project in discussion with them;
- Horizon 2020 funded projects (including ERC grants) that have opted in to the Open Research Data Pilot are required to submit a DMP deliverable within the first six months of the grant.
Planning tools
You should use a DMP template to structure your plan and ensure you cover all aspects of data management. When writing the DMP for use in the project, you may find a generic template more useful than the funder-specific one used for the grant application, as funders' DMP templates may not cover all aspects of data management. These template tools are recommended:
- DMPonline is an online data management planning tool. It provides funder-specific templates for use in grant applications, but you can also select the 'No funder' option when you create a new plan to generate a standard template that is suitable for any project. Plans can be saved, shared with co-applicants, commented and edited, and exported in a variety of formats.
- The Digital Curation Centre (DCC) provides a Checklist for a Data Management Plan . This is broken down into sections, with guidance to help you address all relevant requirements.
- For postgraduate students we provide a PGR Data Management Plan template, with detailed guidance and a review checklist that can be used by supervisors.
For projects with substantial and/or complex data management requirements and costs, an activity-based costing approach may be helpful in preparing the budget. A costing tool is provided by the UK Data Service for this purpose. Although the tool is primarily aimed at researchers in the social sciences, the activity-based approach can be easily applied in any discipline.
What does a data management plan involve?
A DMP will generally cover the following:
- the context of data collection : i.e. the research project, and relevant policies and contracts, e.g. funders' and institutional policies on research data, a collaboration agreement or a PhD industrial sponsorship agreement;
- data collection, storage and processing : the data to be collected, methods of collection and processing and instruments to be used, quality control procedures, solutions for storage, backup and organization of data, and relevant formats and standards;
- documentation and metadata : information that will be created and linked to the data to identify them, document the methods by which they were created, and provide you and other people with the means to understand and use the data;
- ethics and legal compliance : measures for the management of data in order to comply with any research ethics and Data Protection Act requirements, including actions to be taken to facilitate data sharing on publication of findings, e.g. securing appropriate consent, anonymising datasets;
- intellectual property rights : who owns the data that will be collected and used in the research, how ownership affects data sharing, and what permissions may need to be sought for data sharing;
- preservation and sharing : what data will be preserved over the long term, what data repositories or other services will be used to preserve and share data, and on what terms data will be made accessible;
- responsibilities and resources : how roles and responsibilities for data management will be allocated, what resources will be required, and what additional costs will be incurred, such as data storage costs, or charges for data archiving.
Tips for writing a Data Management Plan
- Always create a DMP for the project - even if data management seems simple and straightforward. You will find there is a lot more to even basic data management once you start thinking it through.
- If the research is a team project, develop the DMP as a team. The DMP should have an owner (e.g. the project PI) and be developed with the input of everyone in the team who is involved in data management.
- Use a template or checklist to structure the plan and break it down into logical parts. If you have already written a DMP as part of a grant application for the project, this can provide a basis, but you will need to add more practical detail. A standard/generic template, e.g. the DMPonline no-funder template (see above), or the University's PGR Data Management Plan , may be useful.
- Add as much detail as you need. The important thing is that the DMP is a practical resource for the project. This may involve recording considerable detail, e.g. about file organisation and naming, data processing instructions, etc. Imagine what would happen if a key member of the team left and someone else had to take over their data management responsibilities. What exactly would they need to know?
- Make data sharing central to the plan. Your DMP should identify the repository or repositories in which your data will be deposited for long-term preservation and public sharing at the end of the project, and you should factor into your plan time towards the end of the project to prepare data for archiving.
- If you will be collecting data from research participants, make sure that your data management will comply with research ethics and data protection requirements. Ensure that your recruitment and consent procedures maximise opportunities for future data sharing.
- If the research is undertaken in collaboration or partnership with other organisations, clarify issues relating to intellectual property rights and public sharing of data. Most research contracts have standard IP and Publication clauses, vesting ownership of IP in the originating party or parties, and requiring any party to give appropriate notice to the other parties of any intended publication.
- Use the DMP: don't just file it and forget it. Ensure team members are aware of and follow the plan, and review the plan on a regular basis, updating it as the project progresses. It could be a standing item on the agenda for project team meetings.
- If you need help writing your project DMP, or would like your DMP to be reviewed, contact the Research Data Manager.
Robert Darby , Research Data Manager
0118 378 6161
Manage Research Data
Categories:
- Award Management
- Regulatory Compliance
Introduction
FAIRport Guiding Principles drive the University’s scientific data management. Make sure you understand the type of data you want to share or exchange. Your proposal may require a data management plan and your award may need a data use agreement. Sharing data Stanford provides guidance and resources for research data acquisition, sharing and management. Some data is subject to particular protections which require additional terms and management considerations.
The Data Management Plan
A data management plan (DMP) may be required as part of your proposal documentation to comply with funding agency provisions on data management and to improve the visibility of their research. The DMP is a written document that describes the data you expect to acquire or generate during the course of a research project, how you will manage, describe, analyze, and store those data, and what mechanisms you will use at the end of your project to share and preserve your data.
The DMP Tool (Data Management Planning Tool) provides templates, Stanford-specific guidance, and suggested answer text for creating a data management plan for your next grant submission.
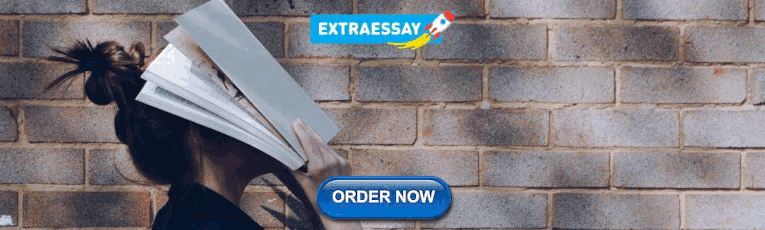
Research Data Management
Stanford University Libraries offer Data Management Services to assist Stanford's researchers with the organization, management, and curation of research data, including:
Understanding and creating data management plans
Organizing and backing up your research data
Acquiring and analyzing data
Assigning metadata to enable future discovery
Preserving your data for long-term access
In addition, the Stanford Digital Repository provides long-term preservation of your important research data in a secure, sustainable stewardship environment, combined with a persistent URL (PURL) that allows for easy data discovery, access, sharing, and reuse.
Data Use Agreements
A Data Use Agreement (“DUA”) is a contract that governs the exchange of specific data between two parties. DUA’s establish who is permitted to use and receive a unique data set, along with the allowable uses and disclosures of the data by the recipient. A DUA also assigns appropriate responsibility to the researcher and recipient for using the data. The DUA must be entered into before there is any use or disclosure of a limited data set to an outside institution or party.
Common terms of a DUA provide that the recipient will:
not use, disclose, or destroy the data set other than as permitted by the DUA, or as required by law;
use appropriate administrative, technical, and physical safeguards to prevent unauthorized uses or disclosures of the data set, including specific data transfer/access/disposition instructions;
report to the provider any uses or disclosures of the data set that are in violation of a DUA;
ensure that anyone to whom it provides the data set agree to the same requirements that apply to the recipient for receiving or accessing the data; and
not re-identify or contact the data subjects (for data related to a human subject).
Clinical Research Data
The Stanford Data Science Resources can help you access the tools, datasets, data platforms and methodologies for conducting innovative clinical and translational research.
The School of Medicine offers a limited initial consultation (underwritten by the Dean’s Office and Spectrum ) to help you identify the resources you need. These consults may lead to longer-term engagements and partnerships with one or more of the consulting groups from across the School of Medicine.
Through these consulting groups, you can access datasets, a variety of platforms and tools and research services, including expert advice on databases and management, study design and implementation, biostatistics, informatics, technology integration, and much more.
Genomic Data Sharing
Effective January 25, 2015, all NIH-supported research that generates large-scale genomic data and uses large scale human or non-human genomic data, as well as the use of these data for subsequent research:
Large-scale data include genome-wide association studies (GWAS), single nucleotide polymorphisms (SNP) arrays, and genome sequence, transcriptomic, metagenomic, epigenomic, and gene expression data, irrespective of funding level and funding mechanism (e.g., grant, contract, cooperative agreement, or intramural support).
Examples include, but are not limited to, sequence data from more than one gene or region of comparable size in the genomes of more than 1000 human research participants; sequence data from more than 100 genes in the genomes of more than 100 human research participants; comparisons of differentially methylated sites genome-wide at single-base resolution within a given sample (e.g. within the same subjects over time or across cell types). Additional examples are available in the Supplemental Information to the NIH GDS Policy. When does the policy NOT apply? Examples of NIH-funded research or research-related activities that are outside the Policy’s scope include, but are not limited to, projects that do not meet the criteria above such as: instrument calibration exercises statistical or technical methods development, or the use of genomic data for control purposes, such as for assay development.
In addition, the following types of funding generally do not fall under the GDS Policy: Institutional Training Grants, K12 Career Awards, Individual Fellowships (Fs) such as the Ruth L. Kirschstein National Research Service Award, Resource Grants and Contracts (Ss), or Facilities and coordinating centers funded to provide genotyping, sequencing, or other core services in support of GDS.
Extramural Institutional Certification is required prior to depositing human genomic data into one of the NIH-supported repositories, even if the research itself is not NIH-supported. NIHsupported repositories include, but are not limited to Database of Genotypes and Phenotypes (dbGaP), Gene Expression Omnibus (GEO), or the Sequence Read Archive (SRA).
The European Union GDPR
The European Union General Data Protection Regulation, or GDPR, is a new and substantial data privacy law that is relevant to 33 countries in the EU and European Economic Area.
GDPR applies to individuals and organizations handling personal data within the EU, transferring data into and out of the EU, and processing of EU data anywhere. It is effective as of May 25th, 2018.
Created: 03.25.2021
Updated: 05.09.2024

- RIT Libraries
- Data Analytics Resources
- Writing a Research Proposal
- Electronic Books
- Print Books
- Data Science: Journals
- More Journals, Websites
- Alerts, IDS Express
- Readings on Data
- Sources with Data
- Google Scholar Library Links
- Zotero-Citation Management Tool
- Writing a Literature Review
- ProQuest Research Companion
- Thesis Submission Instructions
- Associations
Writing a Rsearch Proposal
A research proposal describes what you will investigate, why it’s important, and how you will conduct your research. Your paper should include the topic, research question and hypothesis, methods, predictions, and results (if not actual, then projected).
Research Proposal Aims
The format of a research proposal varies between fields, but most proposals will contain at least these elements:
- Introduction
Literature review
- Research design
Reference list
While the sections may vary, the overall objective is always the same. A research proposal serves as a blueprint and guide for your research plan, helping you get organized and feel confident in the path forward you choose to take.
Proposal Format
The proposal will usually have a title page that includes:
- The proposed title of your project
- Your supervisor’s name
- Your institution and department
Introduction The first part of your proposal is the initial pitch for your project. Make sure it succinctly explains what you want to do and why.. Your introduction should:
- Introduce your topic
- Give necessary background and context
- Outline your problem statement and research questions To guide your introduction , include information about:
- Who could have an interest in the topic (e.g., scientists, policymakers)
- How much is already known about the topic
- What is missing from this current knowledge
- What new insights will your research contribute
- Why you believe this research is worth doing
As you get started, it’s important to demonstrate that you’re familiar with the most important research on your topic. A strong literature review shows your reader that your project has a solid foundation in existing knowledge or theory. It also shows that you’re not simply repeating what other people have done or said, but rather using existing research as a jumping-off point for your own.
In this section, share exactly how your project will contribute to ongoing conversations in the field by:
- Comparing and contrasting the main theories, methods, and debates
- Examining the strengths and weaknesses of different approaches
- Explaining how will you build on, challenge, or synthesize prior scholarship
Research design and methods
Following the literature review, restate your main objectives . This brings the focus back to your project. Next, your research design or methodology section will describe your overall approach, and the practical steps you will take to answer your research questions. Write up your projected, if not actual, results.
Contribution to knowledge
To finish your proposal on a strong note, explore the potential implications of your research for your field. Emphasize again what you aim to contribute and why it matters.
For example, your results might have implications for:
- Improving best practices
- Informing policymaking decisions
- Strengthening a theory or model
- Challenging popular or scientific beliefs
- Creating a basis for future research
Lastly, your research proposal must include correct citations for every source you have used, compiled in a reference list . To create citations quickly and easily, you can use free APA citation generators like BibGuru. Databases have a citation button you can click on to see your citation. Sometimes you have to re-format it as the citations may have mistakes.
- << Previous: ProQuest Research Companion
- Next: DIR >>
Edit this Guide
Log into Dashboard
Use of RIT resources is reserved for current RIT students, faculty and staff for academic and teaching purposes only. Please contact your librarian with any questions.

Help is Available

Email a Librarian
A librarian is available by e-mail at [email protected]
Meet with a Librarian
Call reference desk voicemail.
A librarian is available by phone at (585) 475-2563 or on Skype at llll
Or, call (585) 475-2563 to leave a voicemail with the reference desk during normal business hours .
Chat with a Librarian
Data analytics resources infoguide url.
https://infoguides.rit.edu/DA
Use the box below to email yourself a link to this guide
Examples of data management plans
These examples of data management plans (DMPs) were provided by University of Minnesota researchers. They feature different elements. One is concise and the other is detailed. One utilizes secondary data, while the other collects primary data. Both have explicit plans for how the data is handled through the life cycle of the project.
School of Public Health featuring data use agreements and secondary data analysis
All data to be used in the proposed study will be obtained from XXXXXX; only completely de-identified data will be obtained. No new data collection is planned. The pre-analysis data obtained from the XXX should be requested from the XXX directly. Below is the contact information provided with the funding opportunity announcement (PAR_XXX).
Types of data : Appendix # contains the specific variable list that will be used in the proposed study. The data specification including the size, file format, number of files, data dictionary and codebook will be documented upon receipt of the data from the XXX. Any newly created variables from the process of data management and analyses will be updated to the data specification.
Data use for others : The post-analysis data may be useful for researchers who plan to conduct a study in WTC related injuries and personal economic status and quality of life change. The Injury Exposure Index that will be created from this project will also be useful for causal analysis between WTC exposure and injuries among WTC general responders.
Data limitations for secondary use : While the data involve human subjects, only completely de-identified data will be available and used in the proposed study. Secondary data use is not expected to be limited, given the permission obtained to use the data from the XXX, through the data use agreement (Appendix #).
Data preparation for transformations, preservation and sharing : The pre-analysis data will be delivered in Stata format. The post-analysis data will also be stored in Stata format. If requested, other data formats, including comma-separated-values (CSV), Excel, SAS, R, and SPSS can be transformed.
Metadata documentation : The Data Use Log will document all data-related activities. The proposed study investigators will have access to a highly secured network drive controlled by the University of Minnesota that requires logging of any data use. For specific data management activities, Stata “log” function will record all activities and store in relevant designated folders. Standard file naming convention will be used with a format: “WTCINJ_[six letter of data indication]_mmddyy_[initial of personnel]”.
Data sharing agreement : Data sharing will require two steps of permission. 1) data use agreement from the XXXXXX for pre-analysis data use, and 2) data use agreement from the Principal Investigator, Dr. XXX XXX ([email protected] and 612-xxx-xxxx) for post-analysis data use.
Data repository/sharing/archiving : A long-term data sharing and preservation plan will be used to store and make publicly accessible the data beyond the life of the project. The data will be deposited into the Data Repository for the University of Minnesota (DRUM), http://hdl.handle.net/11299/166578. This University Libraries’ hosted institutional data repository is an open access platform for dissemination and archiving of university research data. Date files in DRUM are written to an Isilon storage system with two copies, one local to each of the two geographically separated University of Minnesota Data Centers. The local Isilon cluster stores the data in such a way that the data can survive the loss of any two disks or any one node of the cluster. Within two hours of the initial write, data replication to the 2nd Isilon cluster commences. The 2nd cluster employs the same protections as the local cluster, and both verify with a checksum procedure that data has not altered on write. In addition, DRUM provides long-term preservation of digital data files for at least 10 years using services such as migration (limited format types), secure backup, bit-level checksums, and maintains a persistent DOIs for data sets, facilitating data citations. In accordance to DRUM policies, the de-identified data will be accompanied by the appropriate documentation, metadata, and code to facilitate reuse and provide the potential for interoperability with similar data sets.
Expected timeline : Preparation for data sharing will begin with completion of planned publications and anticipated data release date will be six months prior.
Back to top
College of Education and Human Development featuring quantitative and qualitative data
Types of data to be collected and shared The following quantitative and qualitative data (for which we have participant consent to share in de-identified form) will be collected as part of the project and will be available for sharing in raw or aggregate form. Specifically, any individual level data will be de-identified before sharing. Demographic data may only be shared at an aggregated level as needed to maintain confidentiality.
Student-level data including
- Pre- and posttest data from proximal and distal writing measures
- Demographic data (age, sex, race/ethnicity, free or reduced price lunch status, home language, special education and English language learning services status)
- Pre/post knowledge and skills data (collected via secure survey tools such as Qualtrics)
- Teacher efficacy data (collected via secure survey tools such as Qualtrics)
- Fidelity data (teachers’ accuracy of implementation of Data-Based Instruction; DBI)
- Teacher logs of time spent on DBI activities
- Demographic data (age, sex, race/ethnicity, degrees earned, teaching certification, years and nature of teaching experience)
- Qualitative field notes from classroom observations and transcribed teacher responses to semi-structured follow-up interview questions.
- Coded qualitative data
- Audio and video files from teacher observations and interviews (participants will sign a release form indicating that they understand that sharing of these files may reveal their identity)
Procedures for managing and for maintaining the confidentiality of the data to be shared
The following procedures will be used to maintain data confidentiality (for managing confidentiality of qualitative data, we will follow additional guidelines ).
- When participants give consent and are enrolled in the study, each will be assigned a unique (random) study identification number. This ID number will be associated with all participant data that are collected, entered, and analyzed for the study.
- All paper data will be stored in locked file cabinets in locked lab/storage space accessible only to research staff at the performance sites. Whenever possible, paper data will only be labeled with the participant’s study ID. Any direct identifiers will be redacted from paper data as soon as it is processed for data entry.
- All electronic data will be stripped of participant names and other identifiable information such as addresses, and emails.
- During the active project period (while data are being collected, coded, and analyzed), data from students and teachers will be entered remotely from the two performance sites into the University of Minnesota’s secure BOX storage (box.umn.edu), which is a highly secure online file-sharing system. Participants’ names and any other direct identifiers will not be entered into this system; rather, study ID numbers will be associated with the data entered into BOX.
- Data will be downloaded from BOX for analysis onto password protected computers and saved only on secure University servers. A log (saved in BOX) will be maintained to track when, at which site, and by whom data are entered as well as downloaded for analysis (including what data are downloaded and for what specific purpose).
Roles and responsibilities of project or institutional staff in the management and retention of research data
Key personnel on the project (PIs XXXXX and XXXXX; Co-Investigator XXXXX) will be the data stewards while the data are “active” (i.e., during data collection, coding, analysis, and publication phases of the project), and will be responsible for documenting and managing the data throughout this time. Additional project personnel (cost analyst, project coordinators, and graduate research assistants at each site) will receive human subjects and data management training at their institutions, and will also be responsible for adhering to the data management plan described above.
Project PIs will develop study-specific protocols and will train all project staff who handle data to follow these protocols. Protocols will include guidelines for managing confidentiality of data (described above), as well as protocols for naming, organizing, and sharing files and entering and downloading data. For example, we will establish file naming conventions and hierarchies for file and folder organization, as well as conventions for versioning files. We will also develop a directory that lists all types of data and where they are stored and entered. As described above, we will create a log to track data entry and downloads for analysis. We will designate one project staff member (e.g., UMN project coordinator) to ensure that these protocols are followed and documentation is maintained. This person will work closely with Co-Investigator XXXXX, who will oversee primary data analysis activities.
At the end of the grant and publication processes, the data will be archived and shared (see Access below) and the University of Minnesota Libraries will serve as the steward of the de-identified, archived dataset from that point forward.
Expected schedule for data access
The complete dataset is expected to be accessible after the study and all related publications are completed, and will remain accessible for at least 10 years after the data are made available publicly. The PIs and Co-Investigator acknowledge that each annual report must contain information about data accessibility, and that the timeframe of data accessibility will be reviewed as part of the annual progress reviews and revised as necessary for each publication.
Format of the final dataset
The format of the final dataset to be available for public access is as follows: De-identified raw paper data (e.g., student pre/posttest data) will be scanned into pdf files. Raw data collected electronically (e.g., via survey tools, field notes) will be available in MS Excel spreadsheets or pdf files. Raw data from audio/video files will be in .wav format. Audio/video materials and field notes from observations/interviews will also be transcribed and coded onto paper forms and scanned into pdf files. The final database will be in a .csv file that can be exported into MS Excel, SAS, SPSS, or ASCII files.
Dataset documentation to be provided
The final data file to be shared will include (a) raw item-level data (where applicable to recreate analyses) with appropriate variable and value labels, (b) all computed variables created during setup and scoring, and (c) all scale scores for the demographic, behavioral, and assessment data. These data will be the de-identified and individual- or aggregate-level data used for the final and published analyses.
Dataset documentation will consist of electronic codebooks documenting the following information: (a) a description of the research questions, methodology, and sample, (b) a description of each specific data source (e.g., measures, observation protocols), and (c) a description of the raw data and derived variables, including variable lists and definitions.
To aid in final dataset documentation, throughout the project, we will maintain a log of when, where, and how data were collected, decisions related to methods, coding, and analysis, statistical analyses, software and instruments used, where data and corresponding documentation are stored, and future research ideas and plans.
Method of data access
Final peer-reviewed publications resulting from the study/grant will be accompanied by the dataset used at the time of publication, during and after the grant period. A long-term data sharing and preservation plan will be used to store and make publicly accessible the data beyond the life of the project. The data will be deposited into the Data Repository for the University of Minnesota (DRUM), http://hdl.handle.net/11299/166578 . This University Libraries’ hosted institutional data repository is an open access platform for dissemination and archiving of university research data. Date files in DRUM are written to an Isilon storage system with two copies, one local to each of the two geographically separated University of Minnesota Data Centers. The local Isilon cluster stores the data in such a way that the data can survive the loss of any two disks or any one node of the cluster. Within two hours of the initial write, data replication to the 2nd Isilon cluster commences. The 2nd cluster employs the same protections as the local cluster, and both verify with a checksum procedure that data has not altered on write. In addition, DRUM provides long-term preservation of digital data files for at least 10 years using services such as migration (limited format types), secure backup, bit-level checksums, and maintains persistent DOIs for datasets, facilitating data citations. In accordance to DRUM policies, the de-identified data will be accompanied by the appropriate documentation, metadata, and code to facilitate reuse and provide the potential for interoperability with similar datasets.
The main benefit of DRUM is whatever is shared through this repository is public; however, a completely open system is not optimal if any of the data could be identifying (e.g., certain types of demographic data). We will work with the University of MN Library System to determine if DRUM is the best option. Another option available to the University of MN, ICPSR ( https://www.icpsr.umich.edu/icpsrweb/ ), would allow us to share data at different levels. Through ICPSR, data are available to researchers at member institutions of ICPSR rather than publicly. ICPSR allows for various mediated forms of sharing, where people interested in getting less de-identified individual level would sign data use agreements before receiving the data, or would need to use special software to access it directly from ICPSR rather than downloading it, for security proposes. ICPSR is a good option for sensitive or other kinds of data that are difficult to de-identify, but is not as open as DRUM. We expect that data for this project will be de-identifiable to a level that we can use DRUM, but will consider ICPSR as an option if needed.
Data agreement
No specific data sharing agreement will be needed if we use DRUM; however, DRUM does have a general end-user access policy ( conservancy.umn.edu/pages/drum/policies/#end-user-access-policy ). If we go with a less open access system such as ICPSR, we will work with ICPSR and the Un-funded Research Agreements (UFRA) coordinator at the University of Minnesota to develop necessary data sharing agreements.
Circumstances preventing data sharing
The data for this study fall under multiple statutes for confidentiality including multiple IRB requirements for confidentiality and FERPA. If it is not possible to meet all of the requirements of these agencies, data will not be shared.
For example, at the two sites where data will be collected, both universities (University of Minnesota and University of Missouri) and school districts have specific requirements for data confidentiality that will be described in consent forms. Participants will be informed of procedures used to maintain data confidentiality and that only de-identified data will be shared publicly. Some demographic data may not be sharable at the individual level and thus would only be provided in aggregate form.
When we collect audio/video data, participants will sign a release form that provides options to have data shared with project personnel only and/or for sharing purposes. We will not share audio/video data from people who do not consent to share it, and we will not publicly share any data that could identify an individual (these parameters will be specified in our IRB-approved informed consent forms). De-identifying is also required for FERPA data. The level of de-identification needed to meet these requirements is extensive, so it may not be possible to share all raw data exactly as collected in order to protect privacy of participants and maintain confidentiality of data.
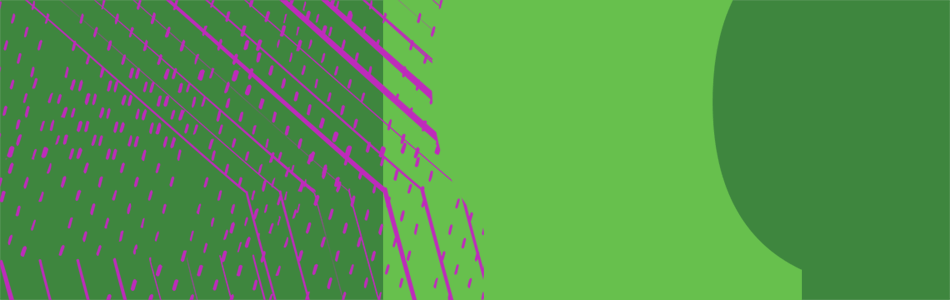
MRC data management plan template
This template should be used to develop a data management plan, part of a research proposal, for the Medical Research Council (MRC).
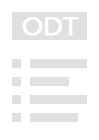
MRC data management plan template (ODT)
ODT , 26 KB
A data management plan should be submitted as part of a research proposal.
You should use this template to make sure that the right information is included.
- 1 April 2024 The data management plan has been updated in line with the revised MRC data sharing policy. It must also include considerations in relation to the UKRI principles and guidance on trusted research and innovation.
This is the website for UKRI: our seven research councils, Research England and Innovate UK. Let us know if you have feedback or would like to help improve our online products and services .

Introduction to implementation research
- Introduction
- The audience for this toolkit
- Relevance of IR for improved access and delivery of interventions
- The purpose of this Toolkit
- Research teams
- Self-assessment and reflection activities
Understanding implementation research
- The need for IR
- Outcomes of IR
- Characteristics of IR
- How IR works
- Community engagement in IR
- Ethical challenges in IR
Developing an Implementation Research Proposal
- The team and the research challenge
- Structure of an IR proposal
- Components of an IR proposal
- Research Design
- Project plan
- Impact and measuring project results
- Supplements
- Funding an IR project
- Common problems with applications
Research methods and data management
- Study design for IR projects
- Selecting research methods
- Mixed methods
- Research tools and techniques
- Data collection
- Data management
- Data analysis
IR-Planning and Conducting IR
- Project planning
- Project monitoring plan
- Developing a logic model
- Developing monitoring questions
- Data use and reporting
- Project execution
- Ethical issues
- Good practices in planning and conducting IR
IR-related communications and advocacy
- Productive Dialogue
- Knowledge Translation
- Research Evidence: Barriers and Facilitators to Uptake
- Policy Advocacy and Strategic Communications
- Data Presentation and Visualization
- Developing a Communication Strategy
- Steps in Developing a Communication Strategy
- Communication materials and Platforms
Integrating implementation research into health systems
- Start up, mapping and convening
- Productive dialogue
- Ownership, trust, responsibilities and roles
- Setting priorities, defining problems and research questions
- Capacity strengthening
- Uptake of findings
- Documentation
- Using the WHO Health Systems Framework in IR
- Principles of sustainability
Developing implementation research projects with an intersectional gender lens
- Integrating an intersectional gender lens in IR
- Proposal development with an intersectional gender lens
- Execution of an IR project with an intersectional gender lens
- Good practices in IR projects with an intersectional gender perspective

The purpose of this module is to describe the fundamentals of implementation research (IR) methodologies including study design, data collection methods, data analysis, presentation and interpretation of IR findings with the objective of enhancing their uptake and use by target audiences.

After reviewing of this module, you should be able to:
- Describe the designs commonly used in IR projects.
- Identify the strengths of quantitative, qualitative and mixed methods approaches in IR data collection.
- Select the appropriate data collection approaches and tools for your IR project(s).
- Describe the sampling processes used for both quantitative and qualitative research tools.
- Highlight relevant ethical issues in data collection.
- Describe appropriate data analysis processes for both quantitative and qualitative data and for mixed methods approaches.
- Describe various formats of data presentation.

TDR Implementation research toolkit (Second edition)
- Acknowledgements
- Self-assessment tool
- © Photo credit
- Download PDF version
- Download offline site

Research Data Management & Security
Nyu policies and guidance, electronic data and system risk classification policy, working with research data, file storage services, retention of and access to research data, guidelines for technology in faculty research, research data.
The following resources provide information and guidance on properly categorizing and storing research data. Keep in mind that NYU’s Policies and guidance applies to all data at the University, including student data, administrative data, and other institutional data. If you have questions as to how your data fits into these categories, or how it should be managed, please reach out to Victoria at [email protected] . The University encourages that every research project, regardless of sponsor, have a data management plan.
Data Use Agreement (DUA) Guidance
Sponsored Projects International and AFAR
Dmptool.org, 12 days of data management and sharing tips & resources, data management (guide), safe computing, research security.
Research security is critical to protecting public investment in research, preventing the misuse of research data and advanced technology generated at universities, and protecting the safety and security of NYU’s employees and students while traveling abroad. Because NYU receives more than $50 million in annual federal research funding, the U.S. government requires that we implement a Research Security Program. The Senior Director of Research Data Management and Security, reporting to the Associate Vice Provost for Research, serves as NYU’s point of contact during this implementation phase. This website provides resources to NYU’s research community and formalizes the ongoing development of NYU’s Research Security program as required by National Security Presidential Memorandum 33 (NSPM-33).
Securing Research Data
Traveling with devices, software, and equipment, export compliance, conflict of interest, conflict of commitment, global travel, cybersecurity (tbd), book travel through concur, intellectual property & tech transfer, public access and open access.
Several federal agencies have public access initiatives or requirements. Check the links below for agency specific information:
- https://sharing.nih.gov/
- https://sharing.nih.gov/faqs#/data-management-and-sharing-policy.htm?anchor=56972
- https://new.nsf.gov/public-access
NYU Commitment to Open Access to and Sharing of Research Data and Publications
New York University is committed to disseminating the results of its research and scholarship as widely as possible. In addition to the public benefit of such dissemination, this commitment serves faculty interests by promoting collaboration across the globe, greater reach and impact for articles, simplifying authors’ retention of distribution rights, and aiding preservation, and acknowledges of the ever-increasing number of agencies and foundations which require the outputs of the research they sponsor be shared openly. Therefore, where required, and if not required, to the extent practicable, NYU encourages its research community to:
Make available, as of the date of publication and without any embargo period, an electronic copy of any final, peer-reviewed manuscript upon acceptance for publication at no charge in an appropriate format (such as PDF). The manuscript shall be made available to the public in one of NYU’s open-access repositories (e.g., Faculty Digital Archive, Ultraviolet., etc.) or another open repository of choice or as required by the project sponsor, and should include all graphics and supplemental material associated with the manuscript.
Additionally it is the expectation of NYU, and in some cases the requirement of external sponsors, that the underlying data supporting the manuscript shall be immediately accessible and open upon article publication. NYU encourages its research community to adhere to the FAIR principles to improve the findability, accessibility, interoperability, and reuse of digital assets. Data shall be made available to the public in one of NYU's open-access repositories (e.g. Faculty Digital Archive, Ultraviolet, etc.) or another data repository of choice or as required by the project sponsor.
Finally, acknowledging that lack of publication does not necessarily mean that research findings are null or negative; NYU encourages its research community to similarly share research data not used to support a publication.
In all cases and in accordance with NYU’s commitment to the integrity and reproducibility of its research outputs, research data shared by the NYU community should be of sufficient quality to validate and replicate research findings.
The National Institutes of Health have adopted the following definition of research data as “the recorded factual material commonly accepted in the scientific community as necessary to validate and replicate research findings, regardless of whether the data are used to support scholarly publications.” Where in doubt, NYU encourages its research community to use a similar standard for determining what data should be shared.
Communications
- https://wikis.nyu.edu/display/RES/Phase+1+-+DMSP+templates
- https://thefdp.org/demonstrations-resources/nih-data-management-sharing-pilot/
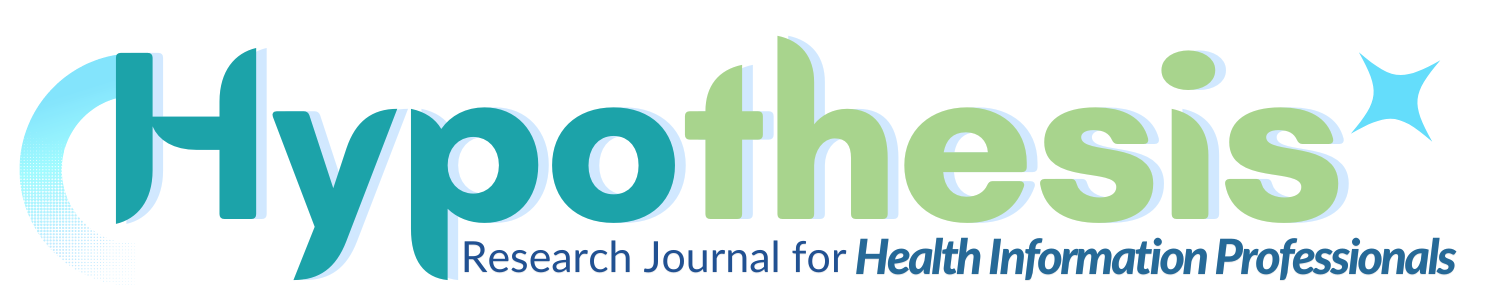
Preparing for the New NIH Data Management and Sharing Policy
Outreach & engagement.
- Sara Samuel University of Michigan https://orcid.org/0000-0001-6808-2514
The Taubman Health Sciences Library (THSL) at the University of Michigan established the Research Data Core Team (Data Team) to raise awareness and educate people about the new Data Management & Sharing Policy released by the National Institutes of Health (NIH). This was accomplished with an initial outreach phase, followed by an engagement phase. Outreach was launched by updating a LibGuide with information about the new policy, creating a slide for digital screens, and producing a short video. For the engagement phase, a presentation was created to review the policy’s main points, emphasizing that a Data Management and Sharing (DMS) Plan needed to be included in grant applications. Throughout the Fall 2022 and Winter 2023 semesters, the Data Team facilitated eight presentations, two workshops, and two office hours. Increased LibGuide views peaked at 1,873 in January. The Data Team anticipates continuing outreach and engagement after seeing how the NIH responds to the first DMS Plans.
Author Biography
Sara samuel, university of michigan.
As an informationist at the University of Michigan Taubman Health Sciences Library, my goal is to connect students, staff, faculty, researchers, and clinicians with the information they need to be successful. I am a liaison to the Hematology and Oncology Department and the Radiation Oncology Department, as well as the Rogel Cancer Center and Taubman Institute. My work includes helping people navigate data management plan requirements and data sharing, develop an online researcher presence, and understand research impact indicators. Prior to my current position, I was a research analyst for a think tank that researched the future of manufacturing in the U.S., and was also an engineering librarian for 7 years.
Samuel S. Research Guides: Research Data Management (Health Sciences): NIH Data Management & Sharing Plan [Internet]. [cited 2023 Jun 8]. Available from: https://guides.lib.umich.edu/datamanagement/nihpolicy
Quick Overview: The New NIH Data Management and Sharing Policy [Internet]. 2022 [cited 2023 Jun 8]. Available from: https://lib.mivideo.it.umich.edu/media/t/1_q4i3f8mw
How to Cite
- Endnote/Zotero/Mendeley (RIS)
- Peer Reviewed Articles
Copyright (c) 2023 Sara Samuel

This work is licensed under a Creative Commons Attribution-NonCommercial-NoDerivatives 4.0 International License .
All works in Hypothesis are licensed under a Creative Commons Attribution 4.0 International license . Authors own copyright of their articles appearing in Hypothesis . Readers may copy articles without permission of the copyright owner(s), as long as the author(s) and the Medical Library Association are acknowledged in the copy, and the copy is used for educational, not-for-profit purposes. For any other use of articles, please contact the copyright owner(s).
Similar Articles
- Gregg A. Steven, Tony Nguyen, Rachel C. Lerner, Phill Jo, Rising Stars Research Projects 2016-2017: Action Research to Improve MLA’s Communities , Hypothesis: Research Journal for Health Information Professionals: Vol. 29 No. 2 (2017)
- Marianne D Burke, Sharing research data to comply with a journal policy: Experience of a first-time depositor , Hypothesis: Research Journal for Health Information Professionals: Vol. 32 No. 1 (2020)
- Rachel Stark, Mickel Paris, Ed Rogan, Mary-Kate Finnegan Dopkins, Cassie Etter, Art Show Paints a Collaborative Picture: Increasing Engagement in a Pharmacy and Health Sciences Library , Hypothesis: Research Journal for Health Information Professionals: Vol. 29 No. 2 (2017)
- Kristine M Alpi, Jonathan D Eldredge, Heather N Holmes, Katherine G Akers, Kimberly R Powell, Margaret A. Hoogland, Research Roadmap: Understanding the Research Process (Part 1) , Hypothesis: Research Journal for Health Information Professionals: Vol. 30 No. 1 (2018)
- Kimberly R. Powell, “Librarian’s Role in Reproducibility of Research” 2017 Symposium: Research Section Stipend Winner Reflection , Hypothesis: Research Journal for Health Information Professionals: Vol. 29 No. 2 (2017)
- Liz Kellermeyer, Matthew Strand, Factors affecting clinical referrals to the medical library , Hypothesis: Research Journal for Health Information Professionals: Vol. 32 No. 1 (2020)
- Jessica Kirschner, Jenn Monnin, Christine Andresen, Gaining Ground: OER at 3 Health Sciences Institutions , Hypothesis: Research Journal for Health Information Professionals: Vol. 35 No. 2 (2023)
- Caitlin Bakker, An Introduction to Statistics for Librarians (Part Two): , Hypothesis: Research Journal for Health Information Professionals: Vol. 35 No. 1 (2023)
- Margaret A. Hoogland, A year in review , Hypothesis: Research Journal for Health Information Professionals: Vol. 31 No. 1 (2019)
- Jolene M Miller, Challenges of the two-year manuscript: Engaging in reflective practice with a manuscript about reflective practice , Hypothesis: Research Journal for Health Information Professionals: Vol. 32 No. 1 (2020)
1 2 3 4 > >>
You may also start an advanced similarity search for this article.
Make a Submission
Developed by, information.
- For Readers
- For Authors
- For Librarians
- Español (España)
Hypothesis ISSN: 1093-5665 eISSN: 2688-1268
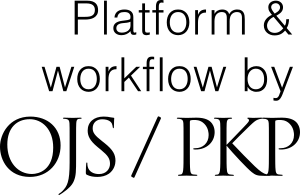
College of Education and Human Ecology

« All Events
Introduction to the 2023 NIH Data Management and Sharing Policy (Virtual Event)
May 9 @ 2:00 pm - 3:30 pm.
Do you have an upcoming NIH proposal due? Join us to learn about the newly implemented NIH Data Management and Sharing (DMS) policy, including the requirement to submit a DMS plan with all new applications and renewals. Compliance with the submitted and accepted plan will be assessed, and non-compliance can impact future funding.
This informational session will provide an introduction to the policy, an overview of tools and resources provided by Ohio State to help you, and preliminary feedback from select Institutes and Centers about submitted plans.
Topics to be covered include:
- How the DMPTool can help you address the required elements of a DMS plan
- Tips for identifying a suitable repository
- Guidance on how to budget for data-related costs
This workshop is ideal for researchers who currently receive NIH funding or may apply in the future, as well as staff who support NIH-funded researchers. This session is scheduled for 90 minutes to allow ample time for attendee questions.
Can’t make the workshop? Check out the NIH DMS Policy Guide for the latest updates, including the slides from this session.
For questions, or additional information, please contact us at [email protected] .
- Google Calendar
- Outlook 365
- Outlook Live
Office of Research, Innovation and Collaboration (ORIC) 153 Arps Hall 1945 N. High St. Columbus, OH 43210
Meet Our Staff
OFFICE HOURS
8 a.m. to 5 p.m. Monday–Friday
Phone: (614) 247-2412 Email: [email protected]

An official website of the United States government
Here's how you know
Official websites use .gov A .gov website belongs to an official government organization in the United States.
Secure .gov websites use HTTPS. A lock ( Lock Locked padlock ) or https:// means you've safely connected to the .gov website. Share sensitive information only on official, secure websites.
Important information for proposers
All proposals must be submitted in accordance with the requirements specified in this funding opportunity and in the NSF Proposal & Award Policies & Procedures Guide (PAPPG) that is in effect for the relevant due date to which the proposal is being submitted. It is the responsibility of the proposer to ensure that the proposal meets these requirements. Submitting a proposal prior to a specified deadline does not negate this requirement.
Leveraging Cyberinfrastructure for Research Data Management (RDM)
Dear Colleagues:
With this Dear Colleague Letter (DCL), the U.S. National Science Foundation (NSF), led by the Directorate for Computer and Information Science and Engineering (CISE) Office of Advanced Cyberinfrastructure (OAC), invites Early-Concept Grants for Exploratory Research (EAGER) and conference/workshop proposals that aim to leverage cyberinfrastructure to advance research data management (RDM) and public access to research data in alignment with the goals of OAC's Cyberinfrastructure for Public Access and Open Science (CI PAOS) program [ https://new.nsf.gov/funding/opportunities/cyberinfrastructure-public-access-open-science-ci ] and the NSF Public Access Initiative (PAI) [ https://new.nsf.gov/public-access ]. The NSF PAI aims to make the results of NSF-supported research publicly available to the greatest extent possible. The CI PAOS program aims to catalyze new and transformative socio-technical partnerships supporting research data infrastructure ecosystems across domains through early-stage collaborative activities between cyberinfrastructure researchers, scientists, research computing experts, data management experts, research labs, university libraries, and other communities of practice.
Advancements in data management across all research disciplines are needed to achieve national public access goals, including to "make publications and their supporting data resulting from federally funded research publicly accessible without embargo on their free and public release" 1 and implementing guidance to digital repositories that hold data from federally funded projects. This DCL responds to these needs while building upon previous NSF Dear Colleague Letters: Reproducibility and Replicability in Science ( NSF 23-018 ), Open Science for Research Data ( NSF 20-068 ), and Effective Practices for Data ( NSF 19-069 ).
Accordingly, proposals are invited from across communities for Early-concept Grants for Exploratory Research (EAGER) projects and for workshops to address competency building, capability building, and community building supporting RDM and public access. There is also a growing demand for international FAIR research collaboration, coordination, and RDM capacity. NSF particularly welcomes proposals involving new and innovative partnerships (such as between cyberinfrastructure (CI) researchers and providers, computer scientist (CS) and information technology (IT) researchers, domain scientists, and/or university libraries) that address RDM topics such as, but not limited to, the following examples:
- Addressing data formats, metadata standards, and harmonization across domains while leveraging existing cyberinfrastructure to advance research data lifecycle management;
- Addressing data curation, provenance, record keeping, organization of data, storage of data, linking, reporting of research data and results, sharing and access, institutional policies, and data management standard operating procedures for projects;
- Promoting machine-readability, reproducibility, and utility for AI computing, resource, and tools to deploy modular RDM training for PIs, trainees, students at any scale;
- Improving domain-specific data quality guidance, formats, RDM best practices, scientific connections, restrictive/sensitive RDM, transfer of large datasets, and case studies;
- Leveraging existing data repositories or hardware/software computational resources for improving research data management, linking datasets within repositories, and combining data to gain new insights for sharing information with research communities;
- Enabling community-scale integration of, and access to, data sets from funded research and/or those generated by industry to enable new research and discovery broadly for the CISE and other research communities;
- Investigating interdisciplinary and international collaborations, costs, and continuity of RDM infrastructure for publications and data across projects, centers, and facilities for NSF-funded research;
- Coordinating, facilitating, and/or evaluating U.S. and international FAIROS integrated research infrastructure efforts.
Proposals must follow the guidance contained in the NSF Proposal and Award Policies and Procedures Guide (PAPPG) for EAGER (see PAPPG Chapter II.F.3) and Conference/Workshop (see PAPPG Chapter II.F.8) proposals. Proposals should clearly describe the intellectual merit, broader impact, and budget for the proposed effort.
- Early-Concept Grants for Exploratory Research (EAGER) proposals are encouraged for high-risk/high-reward innovative concepts and pilot and other research proposals that contribute to community readiness and advancements in RDM for public access and open science. EAGERs can be supported up to $300,000 for up to a two-year duration.
- Conference/Workshop proposals are encouraged for community workshops and other events that bring together communities of practice to explore and advance scientific community readiness in response to this DCL (i.e., such as HPC experts, information technology and computer scientists, library and information science, research labs, research libraries). Each Conference/Workshop proposal must not exceed $100,000 in total budget for a one-or two-year duration.
HOW TO RESPOND TO THIS DEAR COLLEAGUE LETTER
- Proposers are required to email a Concept Outline, following the guidance in PAPPG Chapter I.D.1, to the cognizant Program Director listed in this DCL. The email subject header should start with "NSF DCL: Leveraging Cyberinfrastructure for Research Data Management (RDM)".
- The PD may invite submission of an EAGER or Conference proposal based on an assessment of the alignment of the project description with the goals of the DCL and CI PAOS program [ https://new.nsf.gov/funding/opportunities/cyberinfrastructure-public-access-open-science-ci ].
When submitting your proposal to the CI PAOS Program Description, the project title should be of the form "CI PAOS: Title", after the PAPPG required prefix "EAGER" or "Conference". Please also include "Public Access" as a keyword in the at the beginning of the overview section of the project summary, and ensure inclusion of the following information in the project description:
- A dedicated section describing the Appropriateness of Proposed Work for EAGER or Conference funding,
- A dedicated section describing the relevance of the proposed work to CI PAOS goals (not to exceed one page).
After submitting the proposal, the NSF-assigned proposal number should be emailed to the program director identified below with the subject header starting with "NSF DCL: Leveraging Cyberinfrastructure for Research Data Management (RDM) - Proposal Number".
To be considered for priority consideration for FY 2024 funding, invited proposals must be submitted by 5:00 P.M. submitting organization's local time on July 3, 2024. There is not a deadline for concept outlines.
Concept outlines, as well as questions and inquiries about project ideas, should be sent to Plato Smith, Program Director, CISE/OAC, telephone: (703) 292-4278, email: [email protected] with a copy to [email protected] .
Dilma Da Silva Acting Assistant Director, Directorate for Computer and Information Science and Engineering (CISE)
Alexandra R. Isern Assistant Director, Directorate for Geosciences (GEO)
Sylvia M. Butterfield Acting Assistant Director, Directorate for Social, Behavioral and Economic Sciences (SBE)
C. Denise Caldwell Acting Assistant Director, Directorate for Mathematical and Physical Sciences (MPS)
James L. Moore III Assistant Director, Directorate for STEM Education (EDU)
Susan S. Margulies Assistant Director, Directorate for Engineering (ENG)
Susan Marqusee Assistant Director, Directorate for Biological Sciences (BIO)
Alicia J. Knoedler Office Head, Office of Integrative Activities (OIA)
Kendra Sharp Office Head, Office of International Science and Engineering (OISE)
1 2022 OSTP Memorandum (2022 Nelson Memo). https://www.whitehouse.gov/wp-content/uploads/2022/08/08-2022-OSTP-Public-access-Memo.pdf .
Organization(s)
- Division of Research on Learning in Formal and Informal Settings (EDU/DRL)
- Directorate for STEM Education (EDU)
- Division of Biological Infrastructure (BIO/DBI)
- Directorate for Biological Sciences (BIO)
- Directorate for Engineering (ENG)
- Division of Earth Sciences (GEO/EAR)
- Division of Atmospheric and Geospace Sciences (GEO/AGS)
- Directorate for Geosciences (GEO)
- Office of Advanced Cyberinfrastructure (CISE/OAC)
- Directorate for Computer and Information Science and Engineering (CISE)
- Division of Social and Economic Sciences (SBE/SES)
- Directorate for Social, Behavioral and Economic Sciences (SBE)
- Division of Physics (MPS/PHY)
- Directorate for Mathematical and Physical Sciences (MPS)
- Office of International Science and Engineering (OD/OISE)
- Office of Integrative Activities (OD/OIA)
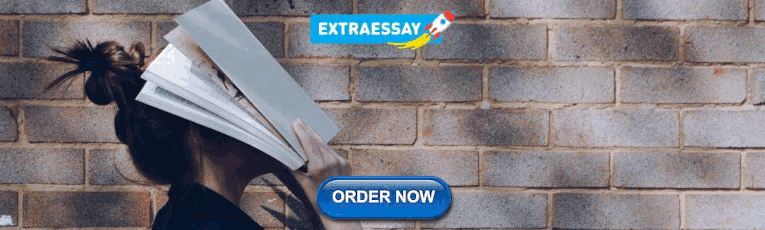
IMAGES
VIDEO
COMMENTS
These plans provide an outline of the types of data that will be collected, the data format, including data and metadata standards, and how the data will be handled throughout the project lifecycle. Many research funders require a data management plan with a grant proposal, so it is important for researchers to understand the main areas to ...
Research proposal examples. Writing a research proposal can be quite challenging, but a good starting point could be to look at some examples. We've included a few for you below. Example research proposal #1: "A Conceptual Framework for Scheduling Constraint Management".
For this reason, your plan should describe data management and sharing during your research and, importantly, after your research is complete. Many funding agencies and sponsors require a data management plan with each proposal, but any researcher or team will benefit from developing a data management plan at the beginning of a project.
A Data Management Cautionary Tale. Why Manage Data? Research today not only has to be rigorous, innovative, and insightful - it also has to be organized! As improved technology creates more capacity to create and store data, it increases the challenge of making data FAIR: Findable, Accessible, Interoperable, and Reusable (The FAIR Guiding ...
A data-management plan explains how researchers will handle their data during and after a project, and encompasses creating, sharing and preserving research data of any type, including text ...
Research papers and data products are key outcomes of the science enterprise. Governmental, nongovernmental, and private foundation sponsors of research are increasingly recognizing the value of research data. As a result, most funders now require that sufficiently detailed data management plans be submitted as part of a research proposal.
This guide gathers overviews and resources for data management and sharing following the research workflow for data, from preparing data management and sharing plans for grant proposals, conducting research, to sharing research data. ... Here is an overview of typical components of a proposal data management plan for U.S. funders. The elements ...
Tab through this guide to consider each stage of the research data management process, and each correlated section of a data management plan. ... The DMPTool supports the majority of federal and many non-profit and private funding agencies that require data management plans as part of a grant proposal application.
When writing your research proposal the following items are important: Fill in the data management paragraph (see the four questions below) Planning: one of the early deliverables will be a detailed data management plan. Budget: take into account the costs (labour and material) for data storage during and data archiving after your project.
Grant proposals may require data management plans (or Data Management and Sharing plans) that describe how the proposal will meet those requirements. Most funders require plans of up to two pages asking for particular topics and content. For detailed guidance, go to the section: Write a Data Management and Sharing Plan.
Writing a data management plan. Every research project that involves the collection and use of research data should have a data management plan (DMP). This is a structured document describing: what data will be collected or used in the course of a research project; how the data will be managed on a day-to-day basis; how relevant data will ...
Sharing data Stanford provides guidance and resources for research data acquisition, sharing and management. Some data is subject to particular protections which require additional terms and management considerations. The Data Management Plan. A data management plan (DMP) may be required as part of your proposal documentation to comply with ...
Show that you understand the current state of research on your topic. Approach: Make a case for your methodology. Demonstrate that you have carefully considered the data, tools, and procedures necessary to conduct your research: Achievability: Confirm that your project is feasible within the timeline of your program or funding deadline
The two-page data management plan is a required part of a proposal to the U.S. National Science Foundation. It describes how a proposal will follow NSF policy on managing, disseminating and sharing research results. This page provides an overview of requirements for the data management plan. See the Proposal and Award Policies and Procedures ...
al. 2014) and standards (e.g. FAIR - Wilkinson et al. 2016) highlight that proper data management is a key factor in enabling effective data sharing which is itself a key factor in establishing research transparency and reproducibility. Complementing calls for improved data management and more widespread data sharing
Examples of data management plans. These examples of data management plans (DMPs) were provided by University of Minnesota researchers. They feature different elements. One is concise and the other is detailed. One utilizes secondary data, while the other collects primary data. Both have explicit plans for how the data is handled through the ...
Proposals must include a document of no more than two pages uploaded under "Data Management Plan" in the supplementary documentation section of Research.gov. This supplementary document should describe how the proposal will conform to NSF policy on the dissemination and sharing of research results.
The Requirement: Include a Data Management Plan in Proposals. An appropriate data management plan is required as a supplementary document (maximum of two pages) for all full research proposals submitted. This plan is to be included in the Supplementary Documents section of the proposal and is not part of the 15-page limit for the Project ...
A data management plan should be submitted as part of a research proposal. You should use this template to make sure that the right information is included. Updates. 1 April 2024 The data management plan has been updated in line with the revised MRC data sharing policy.
If you are using research data in your research, you must include a data management plan in your research proposal and your research data must be stored in a secure environment, in a tamper-free form, and with sufficient detail (as metadata). You will be asked to upload your research data management plan in the PeopleSoft Ethics Application and ...
Research Data Management (RDM) process, distinguishes between different kinds of data, ... The DMP must be included in the research proposal for the project and in the application for Ethics approval through the relevant Faculty Ethics Committee. In externally funded research, the DMP must satisfy the requirements of national and/or ...
Plans for data management and sharing of the products of research. Proposals must include a document of no more than two pages uploaded under "Data Management Plan" in the supplementary documentation section of FastLane. This supplementary document should describe how the proposal will conform to NSF policy on the dissemination and sharing ...
Research methods and data management. The purpose of this module is to describe the fundamentals of implementation research (IR) methodologies including study design, data collection methods, data analysis, presentation and interpretation of IR findings with the objective of enhancing their uptake and use by target audiences.
Research Data. The following resources provide information and guidance on properly categorizing and storing research data. Keep in mind that NYU's Policies and guidance applies to all data at the University, including student data, administrative data, and other institutional data. If you have questions as to how your data fits into these categories, or how it should be managed, please ...
The Taubman Health Sciences Library (THSL) at the University of Michigan established the Research Data Core Team (Data Team) to raise awareness and educate people about the new Data Management & Sharing Policy released by the National Institutes of Health (NIH). This was accomplished with an initial outreach phase, followed by an engagement phase.Outreach was launched by updating a LibGuide ...
Do you have an upcoming NIH proposal due? Join us to learn about the newly implemented NIH Data Management and Sharing (DMS) policy, including the requirement to submit a DMS plan with all new applications and renewals. Compliance with the submitted and accepted plan will be assessed, and non-compliance can impact future funding. This informational
Leveraging existing data repositories or hardware/software computational resources for improving research data management, linking datasets within repositories, and combining data to gain new insights for sharing information with research communities; ... proposals are encouraged for high-risk/high-reward innovative concepts and pilot and other ...