Thank you for visiting nature.com. You are using a browser version with limited support for CSS. To obtain the best experience, we recommend you use a more up to date browser (or turn off compatibility mode in Internet Explorer). In the meantime, to ensure continued support, we are displaying the site without styles and JavaScript.
- View all journals
- Explore content
- About the journal
- Publish with us
- Sign up for alerts
- Data Descriptor
- Open access
- Published: 30 October 2018
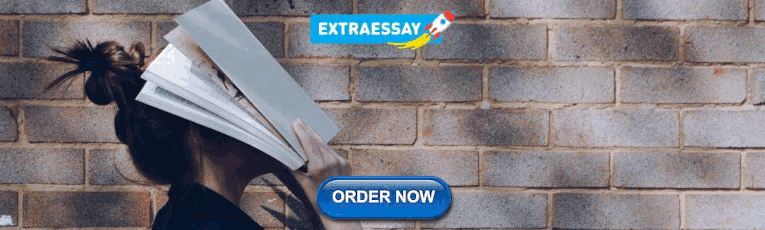
A 3D geological model of a structurally complex Alpine region as a basis for interdisciplinary research
- James M. Thornton ORCID: orcid.org/0000-0002-1447-1554 1 ,
- Gregoire Mariethoz 2 &
- Philip Brunner 1
Scientific Data volume 5 , Article number: 180238 ( 2018 ) Cite this article
18k Accesses
40 Citations
1 Altmetric
Metrics details
- Hydrogeology
A Publisher Correction to this article was published on 13 December 2019
Certain applications, such as understanding the influence of bedrock geology on hydrology in complex mountainous settings, demand 3D geological models that are detailed, high-resolution, accurate, and spatially-extensive. However, developing models with these characteristics remains challenging. Here, we present a dataset corresponding to a renowned tectonic entity in the Swiss Alps - the Nappe de Morcles - that does achieve these criteria. Locations of lithological interfaces and formation orientations were first extracted from existing sources. Then, using state-of-the-art algorithms, the interfaces were interpolated. Finally, an iterative process of evaluation and re-interpolation was undertaken. The geology was satisfactorily reproduced; modelled interfaces correspond well with the input data, and the estimated volumes seem plausible. Overall, 18 formations, including their associated secondary folds and selected faults, are represented at 10 m resolution. Numerous environmental investigations in the study area could benefit from the dataset; indeed, it is already informing integrated hydrological (snow/surface-water/groundwater) simulations. Our work demonstrates the potential that now exists to develop complex, high-quality geological models in support of contemporary Alpine research, augmenting traditional geological information in the process.
Design Type(s) | modeling and simulation objective • data integration objective |
Measurement Type(s) | geographic feature |
Technology Type(s) | digital curation |
Factor Type(s) | |
Sample Characteristic(s) | Western Alps • mountain range |
Machine-accessible metadata file describing the reported data (ISA-Tab format)
Similar content being viewed by others
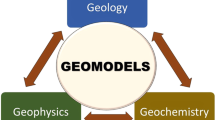
A collection of 3D geomodels of the Los Humeros and Acoculco geothermal systems (Mexico)
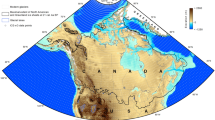
1-km resolution rebound surfaces and paleotopography of glaciated North America since the Last Glacial Maximum
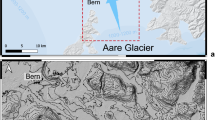
Three-dimensional gravity modelling of a Quaternary overdeepening fill in the Bern area of Switzerland discloses two stages of glacial carving
Background & summary.
Three-dimensional (3D) geological models are digital representations of subsurface formations and their associated features. Recently, the appreciation of their utility to several disciplines has grown, and software tools enabling their construction have proliferated 1 . In earth sciences and engineering, they have, inter alia , contributed to the development of improved earthquake location catalogues 2 and informed excavation and tunnelling projects 3 , 4 . They are also supporting ongoing radioactive waste storage site assessments 5 . In hydrogeology, meanwhile, they have facilitated groundwater resource estimates 6 , enabled the characterisation of karst aquifer geometries, flow pathways, and catchment areas 7 – 10 , and provided a basis for numerical modelling related to geothermal energy prospection 11 .
In tectonically and topographically complex sedimentary settings like the European Alps, 3D models must be generally detailed, high-resolution, and accurate in order to be suitable for their intended application(s). The term detailed refers to the representation of certain characteristic features which, here, would include folds, faults, and spatially-variable formation thicknesses. Developing a model with high spatial resolution, meanwhile, might involve employing a fine (e.g. cell size ≤ 10 m) Digital Terrain Model (DTM) to define the topographic surface, and/or using a sufficient density of georeferenced points to closely replicate the shapes of observed geological features. When exporting a model onto a grid or mesh, care must be exercised to ensure that the resolution is commensurate with the modelled features, especially thin layers or complex shapes. Finally, a model can be considered accurate if the estimated formations and associated features are close to their true positions (although the true positions may be impossible to establish perfectly).
Approximately 30% of the Alps are composed of carbonate rocks, the majority of which are karstified (i.e. discrete conduit networks have developed via dissolution) 12 . However, these rocks are not uniform in their chemico-mineralogical composition, and hence their degree of karstification. Moreover, they are commonly interspersed with lower permeability layers, such as marls and shales. The entire sequences have been folded, fractured, and faulted into complex geometrical arrangements by tectonic forces. Since well-karstified limestones are several orders of magnitude more permeable than marls and shales, the contrasts in hydraulic conductivity within these sequences can be considerable. Where so, the stratigraphic geometry exerts a profound influence on groundwater flow patterns 7 . For example, in a karstified limestone aquifer overlying a marly aquiclude, flow would typically be concentrated just above the interface, its direction corresponding to that of the maximum dip, i.e. flow would be broadly parallel to the strata (and so highly anisotropic). It follows that in folded settings, anticlines – assuming normal orientation – typically act as regional groundwater divides, with synclines conversely representing locations of accumulation 13 . Faults can also have a notable influence 14 ; on one hand they may act as preferential pathway permitting flow across the strata, including enabling formations that would otherwise be considered aquicludes to be bypassed, but on the other, their offsets can disconnect aquifers.
As such, considering 3D geology is crucial when conceptualising and seeking to simulate groundwater flow in these environments. 3D geological models are considerably more powerful with respect to the development, visualisation, and communication of geological understanding than traditional 2D maps and cross-sections. They also provide a direct foundation for subsequent (3D) numerical flow modelling. However, for applications in topographically complex and potentially karstified limestone terrain, geological models must meet several criteria. Firstly, subsurface features that can affect flow must be accurately characterised. Secondly, to provide a realistic overall depiction, the topographic surface must be represented at high resolution. Finally, models must be spatially extensive enough to capture any proven or hypothesised subsurface connections; such connections can function over distances of up to several kilometres, and are capable of importing or exporting water across topographical boundaries.
Despite the improving capabilities of 3D modelling software and a large body of existing geological data pertaining to the Alps, these combined requirements (for geological models to be detailed, high-resolution, accurate, and spatially-extensive) continue to represent substantial technical and computational challenges to model development. It is therefore unsurprising that, irrespective of intended application, few such geological models exist in the Alps; those that do are generally very large in scale, and therefore limited in detail 15 – 17 , although there are exceptions in this regard 18 . Furthermore, the resultant datasets themselves are rarely made available as to the broad, interdisciplinary community who could potentially benefit from them.
Focussing on hydrogeology specifically, it may be noted that flow modelling tools which are capable of incorporating 3D geological information are increasingly widespread. Consequently, research into the interactions between geology and hydrology in Alpine regions is arguably now more limited by a lack of appropriate, accessible geological models than by flow simulation code capabilities. For instance, predicting the impacts of anthropogenic climate change on mountain streamflow regimes is an important and frequently undertaken task in hydrology 19 – 23 . However, (at least partly) due to a lack of explicit 3D geological information, such studies typically employ conceptual, box-type hydrological models like HBV 24 and PREVAH 25 . These models have highly simplified structures and lack physically meaningful parameters, leaving them heavily reliant on calibration to reproduce historical observations. Indeed, they are commonly calibrated solely against stream discharge at the catchment outlet, despite multi-objective calibration and evaluation approaches – particularly those which consider spatially-distributed information – often being advocated 26 – 29 . Consequently, although it may be possible for such models to satisfactorily reproduce historical observations, they may be doing so for the “wrong reasons” 30 . Such a situation would compromise the robustness of any subsequent predictions, especially should forcing conditions exceed the range of the calibration dataset.
The representation of groundwater processes in box-type models is particularly concerning. Essentially, they contain at best only implicit information on the spatial distribution of subsurface properties. In many conceptualisations, fluxes between a soil-water reservoir and a groundwater reservoir are simply estimated as a calibrated function of the amount of water in the soil reservoir 31 . Even in spatially-distributed models, the subsurface is rarely discretised vertically, and lateral groundwater movement is generally unaccounted for. Consequently, any process understanding and predictions derived are in danger of overlooking site-specific geological influences. Even the user guide of the more physically-based and otherwise comprehensive model WaSiM recommends that coupling with an external groundwater model be undertaken wherever groundwater is expected to play an important role 32 .
Several reasons exist as to why groundwater can indeed be expected to play an important role in mountainous environments. Firstly, and most obviously, large hydraulic gradients exist. Secondly, as a result of the very tectonic processes that led to mountain formation, pronounced topography and geological complexity go hand in hand. Thirdly, it is known that this complexity in bedrock geology can strongly influence groundwater processes (and by extension overall catchment function) not only in calcareous settings, as discussed above, but elsewhere too 33 – 36 . Taken together, and alongside the fact that temperate mountain regions presently hold great hydrological importance for adjacent populations (primarily as a result of the orographic enhancement of precipitation and the storage and delayed release of water stored as snow and ice on seasonal and longer timescales) 37 , these points cause one to question whether the routinely made simplifications are appropriate.
As a result of ongoing anthropogenic climate change, mountain hydrology research is becoming increasingly pressing. Two key components of such systems, the snowpack and glaciers, are already demonstrating pronounced sensitivity 38 – 40 . Accordingly, concerns about future water resources, especially during dry summer and autumn periods, are increasing 41 , 42 . Groundwater, vegetation, and permafrost will also respond to climate change, but may do so in a more subtle fashion, involving various interactions and feedbacks with other system components 43 . Predicting the overall changes in the quantity and timing of downstream discharge thus requires more advanced hydrological models. Even prior to that, however, our fundamental knowledge of how and to what extent high-elevation aquifers are recharged, as well as how they transport and discharge water to maintain stream baseflows and spring discharges, must urgently be improved 44 , 45 . Integrated hydrological models like ParFlow-CLM 46 , HydroGeoSphere (HGS) 47 , MIKE SHE 48 , or CATHY 49 may be useful in both regards. Such models generally solve equations for 2D surface and 3D subsurface (both saturated and vadose zones) simultaneously and, crucially, can explicitly represent 3D variability in hydraulic properties defined, for instance, according to a 3D geological model. They also represent most other pertinent elements of the water cycle (including snow accumulation and melt), and simulate the interactions between them, in a coherent, spatially explicit, and transient fashion. In HGS, for instance, several options also exist with respect to karstified formations; the subsurface can either be treated as an equivalent porous media at the elemental scale, as having dual permeability or porosity, and/or as being discretely fractured. All this is possible whilst simultaneously simulating; i) surface flows (important for flood risk and sediment transport), ii) interactions between soil moisture/groundwater, vegetation characteristics, and evapotranspiration, and iii) snow processes. Theoretically, these capabilities leave integrated models uniquely placed to quantify the physical relationships between climate, geology, hydrology, vegetation, and snow in mountainous environments. However, they have found few applications in steep, geologically complex terrain to date. The limited availability of data with which they might be parameterised, calibrated, and evaluated – including 3D geological information – may be posited as an important contributory factor.
In this context, and as part of an interdisciplinary project seeking to improve predictions of Alpine water availability and vegetation species distributions ( http://wp.unil.ch/integralp ), a novel dataset characterising a section of a well-studied nappe fold in the Swiss Alps is presented. No 3D model of this complex region previously existed, and it was unclear at the outset whether developing an appropriate model was even feasible. Alongside various other datasets, the resultant dataset is currently informing catchment scale, integrated hydrological modelling efforts (to be presented in subsequent publications). Various other ongoing or future interdisciplinary environmental investigations could also benefit from the development.
Geological context
The Nappe de Morcles (western Swiss Alps) is a world-renowned example of a tectonic nappe fold, having an amplitude exceeding 10 km and a prominent inverse limb whose stratigraphy is completely reversed. It is the lowest of several such tectonic entities that strike to the SW-NE and together comprise the Helvetic Nappes ( Fig. 1 ). The nappe sits above a region of autochthonous and parautochthonous material which, in turn, overlies the crystalline Aiguilles Rouges massif. It is composed primarily of calcareous shelf sediments (limestones, marls, shales, and sandstones) of Jurrassic to Paleogene age.
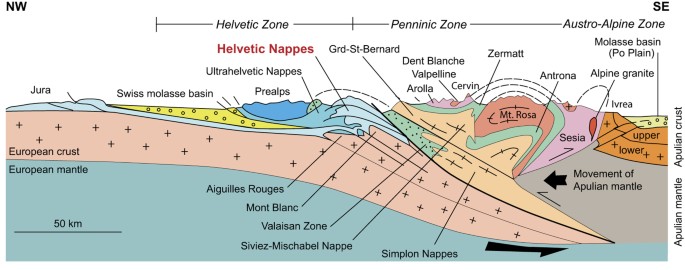
The Nappe de Morcles, which is the focus of this work, is the lowermost of the three nappes (Source: modified after Renard et al. 82 © Dunod, 2015).
The structure of the nappe has been established and refined by a series of notable works 50 – 58 . To summarise, the main fold axis plunges on average ~27° towards the NE59, and several spectacular secondary folds are superimposed upon the main structure forming a characteristic “oak leaf” pattern. Faults of potential hydrological relevance in the focus region are generally oriented E-W, although their extents are limited. No relevant thrust surfaces are present in this zone. The Nappe de Morcles remains an important focus for contemporary nappe fold formation studies 60 , 61 .
General characteristics of the focus area
At the outset of the wider project, two adjoining valleys in – the Vallon de Nant and the Vallon de la Vare – were identified as the focus for subsequent hydrological model development. The geological model domain was therefore centred on this area. These valleys lie within the north-western section of the Nappe de Morcles ( Fig. 2 ). More specifically, the Vallon de Nant has been eroded from the inverse zone of the nappe, whilst the Vallon de La Vare lies in the frontal zone. For reasons that shall be explained shortly, the focus area was extended to the southeast to include La Sarvaz spring.
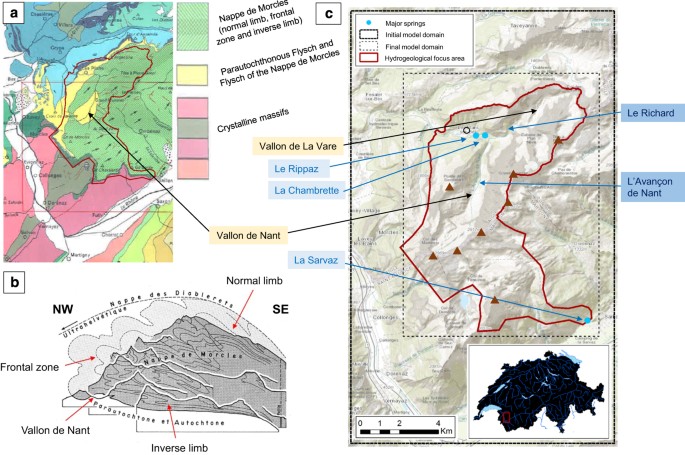
( a ) Tectonic sketch indicating the general geological situation (reproduced from Badoux 53 , non-digital version, © Source: Swiss Federal Office of Topography, 1971). ( b ) Illustrative cross-section through the Nappe de Morcles showing its pre-erosion structure, including secondary folds, and the present topographic arrangement (reproduced from Badoux et al. 53 , © Source: Swiss Federal Office of Topography, 1971), and c) The “original” and “final” geological model domains, major springs locations and the area of hydrogeological interest (the “focus area”) (original figure). In ( c ), selected peaks are marked (brown triangles); starting from the north and proceeding clockwise, these are: Tête à Pierre Grept (2,904 m), Grand Muveran (3,051 m), Petit Muveran (2,810 m), Grand Chavalard (2,899 m), Dent Favre (2,917 m), Grand Dent de Morcles (2,969 m), Petit Dent de Morcles (2, 929 m) and Point des Savolaires (2, 294 m). The village of Les Plans-sur-Bex (hollow black circle) is also marked.
The elevation range within the focus area is considerable (~2,500 m), and precipitation abundant (annual average ~1600 mm·yr -1 in the lowest reaches, increasing with elevation). Low winter temperatures result in a significant proportion of the annual precipitation falling as snow 62 , and small glaciers are able to persist at relatively low elevations in the uppermost parts. Considerable diversity is encountered with respect to vegetation, geomorphology, and hydrology. The area has remained practically untouched by anthropogenic activity; indeed, the Vallon de Nant has been a designated natural reserve since 1969. Overall, the area represents an ideal “natural laboratory” for research across the environmental sciences 63 .
The first stage of the geological model development involved establishing the spatial domains (see Fig. 3 ). Formations belonging to the other Helvetic Nappes or the Ultrahelvetic zone were excluded from the model to keep the degree of complexity manageable.
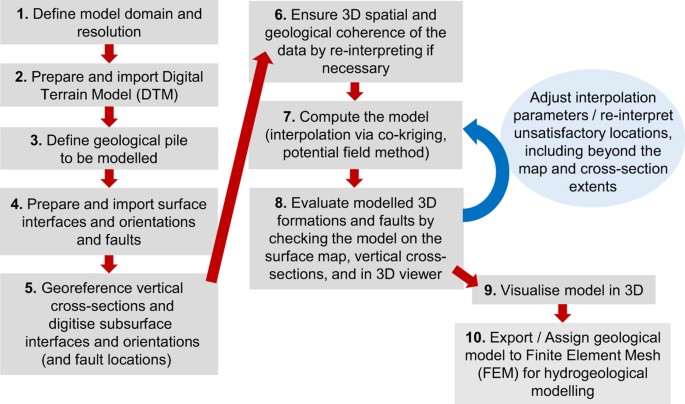
Steps 7 and 8 involved an iterative process of: i) comparing the model to the input data on both the surface and vertical sections, ii) adjusting the interpolation parameters and/or the input data density, iii) re-computing the model, and iv) re-comparing with the input data.
Stratigraphy and initial hydrogeological inferences
A sketch of the stratigraphy in the area of the Vallon de Nant, modified after Badoux 59 ( Supplementary Figure 1 ), provides a useful introduction to the regional geology. (Note that this diagram does not include all the formations that were eventually modelled; for that, consult Table 2). Having said that, the 1:25,000 scale geological maps of “Morcles” 53 and “Les Diablerets” 56 represented the primary sources of detailed geological information. The lithological descriptions provided in the accompanying explanatory notes enabled the likely hydrogeological importance of certain formations to be promptly identified. Reviewing previous studies conducted on neighbouring or nearby tectonic units where equivalent formations are encountered 12 , 64 further elucidated the probable hydrostratigraphy.
In this way, it was possible to establish, for instance, that the massive Urgonian limestone is likely to represent an important karst aquifer(s); this formation is renowned for its purity at other sites, and it hosts a major aquifer in the overlying Nappe de Diableters 64 . The Malm and Valanginian limestones are also likely aquifers 14 . The more siliceous Hauterivian limestone should still be permeable, but probably offers more resistance to flow 65 . In contrast, the Berremian marl is likely to act as a regional aquiclude, as has been reported elsewhere in the Hevetic zone 12 . However, the thinness of this unit in our study region (only ~ 30 m in the region of the Vallon de Nant, according to Supplementary Figure 1 ), and its inability to prevent a hydrological connection with La Chambrettes 65 , mean that its effectiveness as an aquiclude at our site is not guaranteed. The “top” of nappe (i.e. the “bottom” in areas with inverted stratigraphy) is comprised of a thick, clayey Oligocence flysch that is expected to have very low permeability on a regional scale. This brief overview merely seeks to highlight some potentially important formations, and should not be considered exhaustive.
Few hydrological or hydrogeological investigations have previously been conducted in the study area. The karstic source of La Chambrette, which emerges above the northern bank of L’Avançon de Nant near the village of Les Plans-sur-Bex, was, however, the subject of an early artificial tracer test 65 . This experiment demonstrated the existence of a hydrological connection between the closed basin of “La Varre” and the spring. Since these two locations are separated by a formation (the Lower Barremian, as mentioned above) that should be rather impermeable according to its lithology, it was proposed that a fault of some description must enable flow to traverse it. Today, the spring is exploited by Romande Energie SA. Another spring, Le Rippaz, is no longer karstic by the time it emerges rather diffusely from Quaternary moraines, a little downstream from La Chambrette on the opposite (southern) bank of L’Avançon. Currently, it is being developed to augment the water supply of a neighbouring commune 66 , 67 . An associated tracer test confirmed that the formations of the Lower Cretaceous near Pointe des Savolaires constitute the main karstic aquifer. This system could thus be thought of as a chain of aquifers – first karstic and then unconsolidated gravel – which together dampen the variability of spring discharge to snowmelt and rainfall inputs. Finally, as mentioned earlier, the focus area was extended several kilometres to the southeast to include La Sarvaz; another, higher discharge karstic spring that emerges near the village of Saillon, Valais. This decision was taken because, according to our initial understanding of the geological structure and likely favourable hydraulic properties – specifically of the Malm – some the precipitation falling on the southern and eastern ridges of the Vallon de Nant may ultimately drain to this location, rather than via L’Avançon de Nant. Additionally, the discharge of La Sarvaz has been monitored for several years, providing observations that could prove useful during hydrological model development.
One aspect of the regional geology that remains somewhat unclear is the extent to which the various theoretically karstifiable formations are actually karstified at this site. The only known speleological exploration in the region returned an inventory of only six small caves 68 , although this exploration was neither systematic nor exhaustive. The walls of the Urgonian limestone near Pointe des Savolaires were reported to be the only limestones pure enough to contain cavities of speleological interest 70 . However, while the presence of caves is an unambiguous indicator of karstification, the inverse is not true; an absence (or non-discovery) of explorable caves certainly does not necessarily indicate an absence of hydrologically meaningful karstification, since conduits much smaller than humanly accessible can still transport significant volumes of water extremely rapidly. Nevertheless, it should be remembered that flowing water is required for conduit development; if a theoretically karstifiable limestone has been disconnected from recharge or circulation by low permeability layers, it may remain little karstified.
Although the conclusions of this speleological prospection broadly concord with our hydrogeological expectations drawn from the lithological descriptions, they do highlight the issue of chemio-mineralogical purity, and more specifically the possibility that the degree of karstification in our study area may not be as high as elsewhere in the Helevetic zone. In any case, a benefit of our approach – of only estimating formation geometries initially – is that in contrast to the methodology followed by other authors 8 , 10 , there is no need to definitively categorise each formation as being either an aquifer or aquiclude initially; reality is certainly not this binary. Rather, it is our intention to vary and hopefully constrain parameters representing the hydraulic properties of the various formations during subsequent numerical model calibration.
Finally, aquifers are of course not confined to bedrock formations. Glacio-fluvial and other sediments (e.g. talus cones) fill the valley floors. It is expected that the recharge of these units (where permeable) is dominated by spring snowmelt, with drainage subsequently taking place over the course of the late spring, summer, and autumn via a number of springs and ephemeral “seeps”. The geological model presented herein does not include these unconsolidated formations, focussing instead on the more voluminous bedrock. That said, geoelectrical surveys will be undertaken shortly with a view to representing the geometry and heterogeneity of unconsolidated material properties in the integrated hydrological model.
Surface hydrology and its association with bedrock geology
The surface hydrology of the Vallon de Nant is characterised by the eponymous Nants; the torrents that course down its steep slopes. They are located principally to the south and east, and have a highly variable flow that responds rapidly to rainfall and snow and ice melt. The most important are the Nant des Têtes and the Torrent des Martinets, which together constitute the majority of the discharge of L’Avançon. The torrents are also responsible for a great deal of sediment transport; these deposits accumulate to form a large alluvial fan in the middle section of the valley.
The annual hydrological regime of L’Avançon de Nant may be classified as nivo-glacial 69 ; that is to say, it has a mixed snow and ice-dominated response with a discharge peak in early summer corresponding to melt of the snowpack, variable discharges from one year to another, diurnal cycles superimposed on the hydrograph due to ice melt, and groundwater contributions maintaining baseflow.
Surface water is noticeably scarcer in the upper part of the Vallon de La Vare than the Vallon de Nant, although a small stream – Le Richard – does develop and joins L’Avançon downstream of Pont de Nant. This contrast between the two valleys can probably be explained by differences in their position in the nappe. Specifically, the Vallon de Nant is underlain by low-permeability flysch, which limits groundwater exportation and permits the existence of a relatively long section of permanent stream (it is only ephemeral in the upper section in dry, cold autumn and winter periods, when the groundwater level in this upper section has fallen such that it can no longer contribute to streamflow). A clayey layer of glacial till at some intermediate depth between the surface and bedrock interface may also contribute to this behaviour, although this hypothesis remains to be tested by geophysics. In contrast, the Vallon de La Vare lies in the inverse zone, and thus the impermeable flysch is oriented approximately vertically away to the north, near L’Argentine. Hence, the bedrock beneath the valley floor is composed of the more permeable limestones of the Lower Cretaceous. One aim of the 3D model is to help visualise and better understood such influences. Additionally, having two somewhat geologically contrasting catchments adjacent to one another provides the opportunity to explore the specific influences of geology on hydrology whilst other factors, e.g. climate, remain fairly constant.
A wealth of geological information pertaining to the European Alps exists, having been developed over decades of dedicated study by committed regional experts. Presently, this information exists primarily in the form of two-dimensional (2D) maps and cross-sectional diagrams. Despite observational advances in most other fields of the geosciences, the field mapping techniques and concepts underpinning the production of such structural geology datasets have changed little in over a century 70 . As such, their quality remains similar to more contemporary outputs. Moreover, the prevalence of observable features such as stratigraphic interfaces, faults, and folds mean that such datasets are typically more accurate in mountainous regions than in settings where the bedrock is more obscured by unconsolidated deposits 18 . Indeed, the deep incision made by the Vallon de Nant into the inverse limb of the nappe can be thought of as a kind of “window” into its interior − a visible cross-section. Certainly, the availability of appropriate geological data should rarely be a limiting factor for the development of 3D models in the Alps, although the arguable under-exploitation of the existing body of information means it might have been hitherto.
As already mentioned, thanks to its reputation as a classic example of a first-order nappe and intriguing attendant complexity, the structural geology of our study region has a long history of being studied and mapped. At this juncture, it is worth briefly highlighting the effort that the production of the maps, cross-sections, and associated explanatory notes that comprise the current Geological Atlas of Switzerland in this region entailed. In the introductory remarks to his illustrated text Tectonics of the Morcles Nappe between Rhone and Lizerne , Badoux states that the work undertaken prior to the publication of the second edition maps took 8 years! 54 By this time, under the tutelage of Lugeon, he had already developed a great passion for, and expertise on, the geology of the Vaud Alps. These maps remain the highest resolution, most current geological dataset of the Swiss Confederation in this region.
As Table 1 indicates, input data for our model were derived from three primary sources: i) a Digital Terrain Model (DTM), ii) surface geological maps, and iii) vertical geological cross-sectional diagrams.
Having gained an appreciation of the study area and identified and sourced appropriate input data pertaining to it, several sequential steps were followed in order to develop the geological model ( Fig. 3 ). In summary, data extracted from existing geological maps and vertical cross-sections were compiled along with a digital terrain model (DTM) in the GeoModeller software environment 71 . GeoModeller is a commercial platform developed by the BRGM (French Geological Survey) and Intrepid Geophysics; for further information, see Calcagno et al. 72 and Guillen et al. 73 . It facilitates the estimation of continuous geological models that respect all available data indicating the locations of interfaces between different lithological formations, the spatial orientations of these formations, and any faults present in the domain. Certain geological rules are also taken into account. The following sections describe each phase in more detail.
Defining the model domain and resolution
Studying the regional geology and hydrogeology enabled the initial and final domains for the geological model development to be established ( Fig. 2 ). The initial model domain was slightly larger than the final one to ensure that all data that could potentially inform the geological model in the smaller focus area were included in the estimation. In light of the complex topography and our prior knowledge of the presence of geometrically complex and thin units, it was decided that the model development should proceed at a resolution of 10 m.
Preparing and importing the Digital Terrain Model (DTM)
The swissALTI 3D digital terrain model (DTM) is a raster dataset with a horizontal cell resolution of 2 m. It represents the land surface without vegetation or buildings. For further information, see: https://shop.swisstopo.admin.ch/en/products/height_models/alti3D .
The vertical uncertainties associated with the product, quoted in Table 1 , are more than low enough for the application at hand.
For the purpose of model development, the dataset was resampled to 10 m. This resampling served to smooth out high-frequency noise (i.e. small-scale topographic features) and reduce the computational burden. The resultant dataset was imported into GeoModeller ( Supplementary Figure 2 ). It forms the upper surface of the geological model.
Defining the geological pile to be modelled
The term geological pile refers to the sequence of lithological formations to be modelled. Since the domain extends over two separate geological map sheets, each having a slightly different formation classification scheme, some reconciliation was required to arrive at a single sequence. In the end, the sequence modelled was extremely similar to that of the Morcles map sheet. Given the potential multi-disciplinary applications of the dataset, maintaining a classification that closely resembled that of the map legends was considered preferable to grouping any formations a priori . Of course, should further simplification be required for a particular end use, the formations represented in the output dataset can be grouped later.
Preparing and importing surface formation interface data, orientation data, and faults
Two types of surficial geological data from the relevant map sheets 53 , 56 were obtained in digital format ( Fig. 4 ). These were: i) polylines formed by joined points indicating the locations of the interfaces between the formations of the geological pile which outcrop at the surface (also known as “contacts”), and ii) point features describing the orientation of these formations at certain locations on the surface (also known as “structural data”).
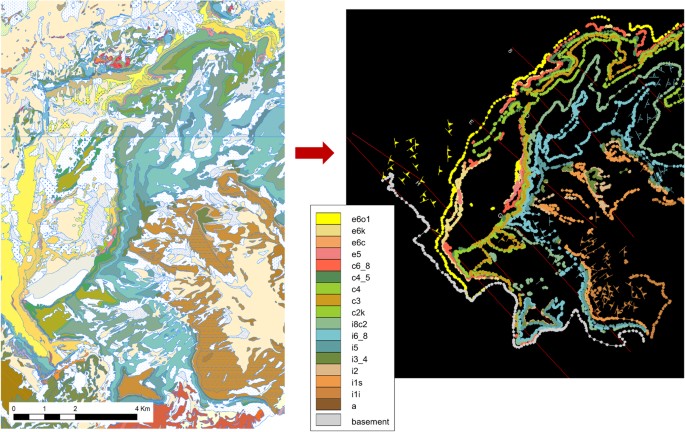
Georeferenced point features (formation interface locations and marked structural data) extracted from the surface map (left, Badoux et al. 53 , Badoux and Gabus 56 , Source: © Swiss Federal Office of Topography, 1971, 1991) and imported into the GeoModeller interface (right). The legend refers to the coding of the formations of the stratigraphic pile. Circles indicate interface points, and arrows indicate orientations. Note that since the DTM had already been imported at this juncture, these points were in fact located in 3D and not only 2D space (i.e. they could be associated with a z-value).
Where necessary, interface polylines were reattributed to match the formations defined in the geological pile. Whilst taking care to maintain their shapes, they were also simplified to reduce the number of vertices. This spatial data processing was conducted using the open source software QGIS. The processed polylines were then imported into GeoModeller. Given the complexity and number of interface shapes in the study region, this approach was more efficient than the more common practice of importing a pre-georeferenced image of a geological map into GeoModeller and then manually digitising the surface formation interfaces in that software environment. In locations where it was clear that a given boundary was continuous beneath the Quaternary cover (and hence was not actually defined) but the location of the boundary could be easily estimated, additional points were inserted. In certain other locations, bedrock interfaces completely obscured by the surficial cover could not be estimated. The consequent data gaps represent a source of uncertainty in the final model. (The model proposes continuous boundaries as a result of the interpolation).
The orientation points were attributed to formations of the geological pile by means of spatial intersection. Some surface orientation data points that fell between vertical cross-sections in the heavily folded frontal zone had to be discarded because at these locations, the polarities/younging directions (i.e. whether normal or overturned) could not be determined with confidence.
Faults that have offsets that were believed large enough to potentially disconnect aquifers, numbering 10 in total, were explicitly represented. Firstly, their surface extents were digitised and imported into GeoModeller. The trace of only one of these faults appeared on a vertical cross-section; the shape of this one was therefore be digitised in the vertical plane also. The remainder were assumed to be vertical in the absence of any other orientation information. Finally, since all faults are shown by the existing geological maps to be finite, which is to say that they do not extend across the entire domain, it was necessary to estimate an “ellipsoid of influence” for each.
Faults with more limited offsets, which are not represented, could still be responsible for preferential hydrological flow pathways; an effect that could be represented in any subsequent hydrogeological modelling by prescribing discrete fractures, or by treating the corresponding volumes as having dual permeability.
Georeferencing vertical cross-sections, digitising subsurface interfaces and orientations
The “stacked” vertical cross-sections 53 , 56 ( Fig. 5a ) provide interpretations of the subsurface structures. Including such information in the estimation of model was crucial because the non-stationarity of the domain, which arises due to the pronounced folding and faulting, precludes the straightforward extrapolation of surface observations into the subsurface.
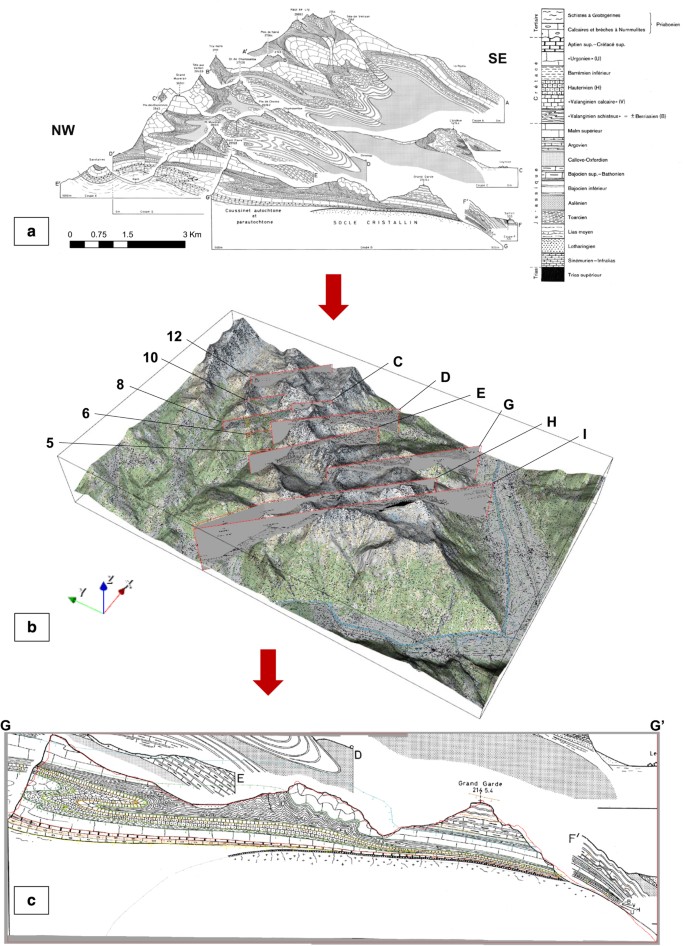
( a ) Incoming vertical cross-sections. Note that only C-I (i.e. those from the Morcles map sheet) are shown in this panel (Badoux et al. 53 , Source: © Swiss Federal Office of Topography, 1971), although Sections 2-12 (from the Diablerets map sheet; Badoux and Gabus 56 , Source: © Swiss Federal Office of Topography, 1991) were also used in the model development, ( b ) All 11 cross sections, having been georeferenced, visualised in the 3D viewer of GeoModeller, and ( c ) An example of a georeferenced cross section (GG’) visualised in the 2D viewer of GeoModeller, with subsurface interface and orientation data points digitised according to the locations and dips of the various interfaces shown (coloured lines and arrows, respectively). Once these sections were viewed, it was also ensured that the topographic surface illustrated on the diagram closely matched that derived from the DTM along the same profile (red line); a close match, as seen in this example, indicates sound georeferencing. Furthermore, since the DTM has been developed using modern technology (LiDAR and photogrammetry) and is therefore of high quality, a close match also indicates an accurate representation of the topographic surface in the original figure.
Prior to being imported into GeoModeller, the diagrams were cropped and the cross-section start and end locations georeferenced ( Fig. 5b ). For each cross-section, the correspondence between the topographic surface profiles in the GeoModeller environment (i.e. the profile of the DTM between the georeferenced cross-section start and end points) and the representation of the topography on the georeferenced diagram was assessed (see the red line in Fig. 5c ). The close matches observed gave us confidence that both the georeferencing of the cross-section diagrams and the original representation of the topography along the sections were satisfactory. Fig. 6
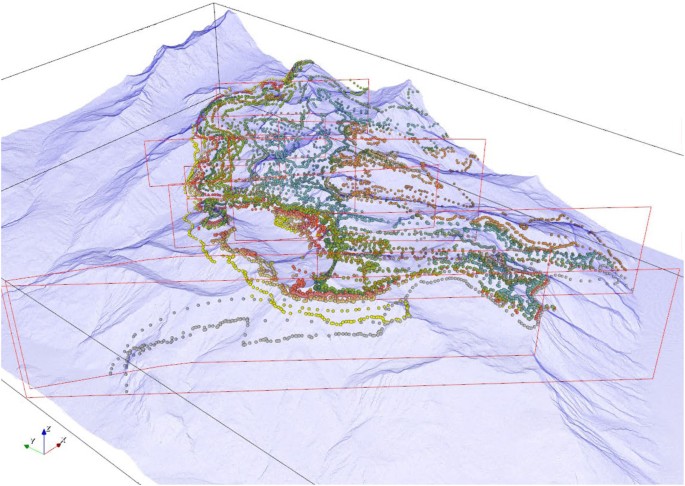
To avoid cluttering, only interface data points are shown, although orientations were also visualised and checked in the same fashion. Here, the view is towards the north-east.
The lengthy task of manually digitising the subsurface formation interfaces illustrated on the cross-sections, and their associated orientations, was then undertaken. In this process, the interface surfaces were assumed orthogonal to the section plane, i.e. the dip direction is parallel to the section, with dip angles estimated at regular intervals along each interface according to the angles formed with the horizontal plane. Due to the plunge of the axis of the main nappe structure, the subsurface dip and dip directions resulting from this assumption may not be entirely correct in all instances. However, the approach taken was the only practical way in which some subsurface orientation data could be included, which in turn was absolutely necessary to successfully model the region. This assumption is not expected to have any implications for the utility of the model for hydrological and other environmental applications.
In total, 11 cross-sections distributed throughout the domain contributed to the model.
Following the completion of Steps 4 and 5, the surface and subsurface interface and orientation points could be visualised in 3D and their consistency with one another verified and, where necessarily, improved (Step 6). Figure 6 illustrates the final arrangement of digitised formation interfaces (both surface and subsurface) that were taken into account in the computation of the model.
Computing the model
In contrast to more traditional “explicit” or “surface-based” approaches, which essentially involve users generating geological surfaces or volumes based directly on the available data, GeoModeller takes an “implicit approach” to model estimation. Such an approach relies on algorithms that integrate observed data with geological interpretation. The particular theory and algorithms that are employed have been comprehensively described and exemplified elsewhere 72 , 74 , 75 , but to summarise: formation interface and orientation data are co-kriged to produce a 3D scalar field, or potential field 76 . Equipotential iso-surfaces with certain reference values represent formation interfaces, whilst the gradients of the scalar function describe their orientations. Several potential fields can be combined in the same model to, for instance, reconstruct complex erosive and/or onlap relationships between geological series (which are simply groups of individual formations). Faults can be represented by inserting discontinuities into the potential field. Once computed, the surfaces or volumes may be visualised by means of a marching cube methodology 77 .
The key benefits of taking an implicit approach are that certain conditions of geological validity (e.g. the forbiddance of overlapping interfaces) are directly enforced 78 , and that interface contact and orientation data can be considered simultaneously in the estimation of a continuous model 79 . Another positive feature is that a given model can be updated relatively quickly following the addition of new data. In our case, this allowed several “competing” models to be generated efficiently using slightly different subsets of the data, alternative interpretations of the relationship between formations in the geological pile, and varied parameter values. Drawbacks of the implicit approach include its relatively high memory usage, and the limited opportunity that exists for users to manually adjust individual modelled surfaces to match their expectations or expert knowledge.
The first stage of computing the model involved testing how the relations between the series (which are simply groups of formations) of the geological pile might best be represented. According to the legend of the Morcles map, erosive relationships exist between the Jurassic and the Cretaceous series, and also between the Cretaceous and Tertiary series. In early iterations these series were indeed treated separately with “Erode” relations, such that one or more would take precedence and cut over the others (depending on which were defined as “Erode” and which remained “Onlap”, as well as their relative positions in the pile). However, this prevented data from the upper or lower formation of one series from constraining formations in the next series, leading to some cross-cutting situations that were deemed unrealistic. Because the interfaces of all formations seem to generally follow one another, with few if any instances of formations within the modelled series having been completely eroded, it was eventually decided to treat all formations as part of a single series.
The only further inputs necessary to compute a model are the values of three (isotropic) interpolation parameters: the range, the nugget effect of geological interface data, and the nugget effect of geological orientation data. The range represents the spherical distance beyond a given location at which data points will have no influence on the model. The nugget effect parameters represent the variance between the values of observed data points that is not explained by separation distance, but could rather reflect measurement error or stochasticity (i.e. “noise”). Here, it can be thought of as the mismatch permitted between model and data.
Code availability
GeoModeller is a proprietary software owned by, and licensable from, Intrepid Geophysics (v.3.3.0 was used in this work). The approach GeoModeller takes has been thoroughly described in the peer-reviewed literature. Open source GIS tools (QGIS; https://qgis.org/en/site/ ) were applied for data preparation.
Data Records
Exporting the model.
Once an acceptable model had been produced (see Technical Validation), the model was exported in a regular voxel format at both 10 m and 50 m resolution. In this format, to reduce data volumes, the position of each voxel is given implicitly. In principle, the voxel model can be used directly for hydrogeological modelling. However, this would be rather inefficient with respect to the number of nodes and elements required. More contemporary practice would be to separately generate a finite element mesh of variable resolution, which allows appropriate representation of important features whilst minimising the total number of nodes, and then assign geological codes to each element in this mesh according to the geological model via a spatial query. Following this, one could attribute (based on knowledge of the lithology, etc.) reasonable initial estimates of the values of parameters describing physical properties throughout the domain. Such meshes could be comprised of layered prisms or, as are beginning to emerge, fully-unstructured tetrahedra. Various possibilities exist for refining or optimising the arrangement of elements according to surface or subsurface features, although this topic lies beyond the scope of this work.
3D shapes were also exported for visualisation. More specifically, the formation interface surfaces may be loaded as polygonal meshes in ParaView 80 ; an open source 3D visualisation software. In this environment, layers can be visualised or hidden at the user’s behest, and virtual interaction undertaken.
Finally, the final GeoModeller project was saved in its native format.
The model data ( Data Citation 1 ) is provided in a generic voxel format at two different resolutions: 10 m and 50 m (regular cubic cells) (Generic voxel format, Data Citation 1 ). The lithological code is listed for each cell. For ease of processing, no refinement of the vertical resolution has been made near the interface with the topographic surface in either case. The 10 m resolution voxel model is also provided in a modified voxel format that can be loaded into the freely-available visualisation software SGeMS 81 (SGeMS voxel format; also see Supplementary Figure 4 ). In addition, formation interface surface were prepared in a format that can be visualised in ParaView (Surfaces for ParaView, Data Citation 1 ). Finally, the entire GeoModeller project is provided in its native format; this can be viewed by readers with a GeoModeller licence (Native GeoModeller Project, Data Citation 1 ).
In the SGeMS format, the lithological formations are represented by the codes listed in Table 2 .
Technical Validation
The most relevant evaluation process was the visual comparison of how well lithological formation interfaces defined by the input data compared with the corresponding modelled interfaces – both on surface and on the vertical cross-sections. Accordingly, as part of the iterative computation and re-evaluation process, the adjustable parameters were systematically varied until a satisfactory balance between a smooth model and model that honoured the data was obtained. In practical terms, this was achieved by visually comparing the input data and the modelled interfaces on both surface and vertical sections. The final parameter values we arrived at are:
Range: 2,000 m
Nugget effect on geological interface data: 0.00001 (arbitrary unit of the potential field)
Nugget effect on geological orientation data: 0.1 (arbitrary unit of the potential field)
Comparisons of the input data and final modelled interfaces on the surface section and four randomly selected vertical cross-sections are shown in Fig. 7 . The close matches obtained indicate that the interpolation algorithm can reproduce the structures defined in the input data. If one then assumes that the original geological interpretation presented in the maps (i.e. the input data itself) is reasonable, one may suggest that the new 3D model is also geologically plausible. Taking slices through the domain between cross-section locations, and visualising the estimated volumes of individual formations with their corresponding input points, reinforced this assessment.
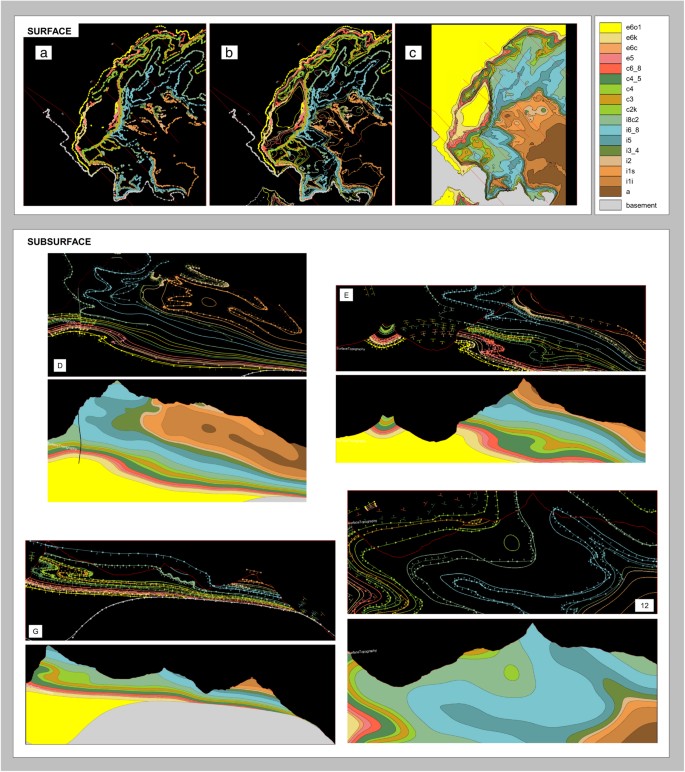
The thin lines with circles and arrows correspond to the input data extracted from the surface maps (surface section), or digitised directly from the formation interfaces illustrated on the cross-section diagrams (vertical sections). Specifically, the circles represent interface data points and arrows represent orientation data points. Input orientation data points are not shown on the surface section in the interests of clarity. The thicker, continuous lines correspond to the modelled surfaces. For the surface, the data points alone ( a ) and then data points with the interpolated interfaces underneath ( b ) are shown separately. The final filled volumes are also shown for all sections (panel c shows this for the surface section). Vertical cross-section letters follow the convention of the original inputs. In Section D, one of the faults that has been modelled is visible. Two disconnected circles (spheres in 3D) are visible; these are model artefacts, and are discussed in due course.
Although the number of input data points observations is high, given the inaccessibility of the subsurface, they are still unlikely to be sufficient to constrain a unique model 79 . For this reason, no data were explicitly withheld to provide independent evaluation data; keeping any observations aside would inevitably have had an adverse impact on the final model.
The resultant dataset ( Figure 8 ) represents 18 formations, including their associated folds and selected major faults, and covers a horizontal area of 9.6×13.4 km.
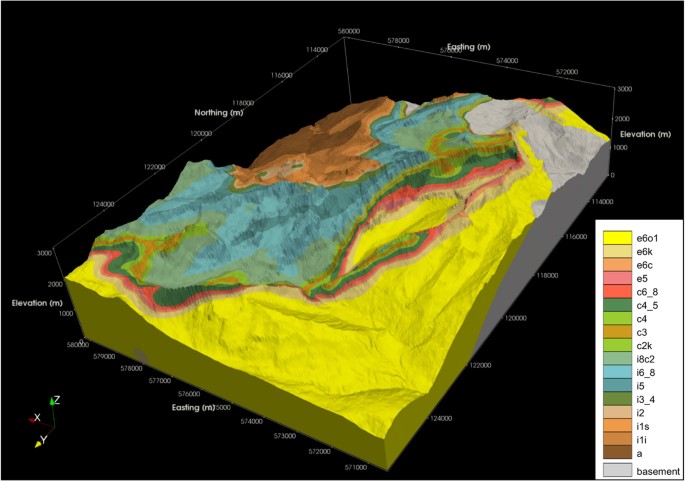
This figure was produced in ParaView. Coordinates are given in the CH1903 / LV03 system. The formation codes correspond broadly to those of the legend of the 58 Morcles sheet 53 , although the geological pile was slightly modified to reconcile it with the Diablerets sheet. Some colours were slightly adjusted to increase visual impact. In the western part of the domain, the stratigraphy is overturned, i.e. geologically older units are found above younger ones. Quaternary cover is not included in the model. Formations of the Ultrahelvetic zone are not included in the model, and thus the presence of “e6o1” (bright yellow) in the extreme north-west of the figure is not reflective of the real geology in this region (as indicated in Fig. 2a ). Similarly, the model is less well constrained in the extreme east of the domain (i.e. towards the centre of the nappe), which is away from the main area of interest.
A further visual comparison was made between a panoramic sketch of the geology of the eastern side of the Vallon de Nant (“Planche II” of Badoux 54 ) and a roughly equivalent view produced in the modelled environment, again with satisfactory results ( Supplementary Figure 3 ).
The interpolation approach employed to develop the 3D model is based on co-kriging. For this reason, the density of data points may have some effect on the final model. Whilst the relatively high-density surface points were extracted directly from the (pre-digitised) map data, and were therefore rather treated as rather “fixed” (although the density of vertices on relatively straight surface interfaces was increased so these features were not unduly de-weighted), the subsurface points had to be digitised manually from the cross-sectional diagrams. The density at which these points were inserted was at the discretion of the model developer, and was therefore somewhat arbitrary. This inevitably introduced a degree of subjectivity into the modelling process. On the other hand, it also provided a degree of flexibly to vary the extent to which data constrain the model regionally. For instance, where uncertainty in the position of an interface was considered lower (e.g. near an observable interface at the surface), the density of points could be increased. Furthermore, where the precise location of the interface was unknown in a particular region but the orientations could be inferred from adjacent layers, subsurface orientation data points could be inserted independently of interface data. Finally, as mentioned above, some additional interface points were even inserted onto the surface section where it was clear that a given boundary was continuous but was simply not marked on the map as a result of being obscured by Quaternary deposits. Accounting for these aspects, rather than simply computing the model based only on the available data, enabled us to produce a final model that both honoured the data and could be deemed geologically plausible.
The resultant model is deterministic. In regard to this, it may be noted firstly that the number of outcrops present in our highly eroded, mountainous study area mean that the geological structures are relatively easy to observe. Therefore, although the area is geologically complex, and the existing data (which formed our inputs) are naturally associated with some uncertainty (the vertical cross-sections naturally more than the surface data), this data uncertainty is arguably less pronounced than in other geological settings where bedrock outcrops are less conspicuous. The series of vertical cross-sections is also very dense. As such, it seems unlikely that attempting to refine the field data or geological interpretation, as expressed in the existing surface mapping or vertical cross-sections, would yield major improvements in the output.
Nevertheless, in future work, it would be possible to assess the uncertainty associated with the deterministic geometrical representation to be assessed by generating multiple realisations of the model. This could be achieved by perturbing the parameter values within a probabilistic framework. That said, it may be suggested that the high data density leaves little scope for model variability during the interpolation stage. Furthermore, before embarking on such a task, the relative magnitudes of various uncertainties that could arise throughout a wider modelling chain should be contemplated. For example, in our hydrogeological application, the uncertainty in the hydraulic conductivities of the various formations is likely to be much more substantial that the uncertainty in their geometrical structures. Exploring the capability of high resolution LiDAR terrain data to refine the input interface locations represents another potential avenue.
A number of challenges were encountered during the development process. Consequently, the final dataset is associated with some limitations. These limitations could motivate further improvements in 3D geological modelling algorithms in general, or the present model in particular. They are as follows:
The polarity (normal/overturned) of some surface orientation points in the frontal zone was not clear. In the horizontal plane, these points were located between vertical cross-sections. Given the extreme folding in the region, their polarities could not be easily confirmed. These points were omitted.
The subsurface orientation data derived from the vertical cross-sections were taken as if there is no plunge (i.e. as if the cross-sections are perfectly orthogonal to the main fold). In reality, the plunge angle is spatially variable, and the true dip and dip direction at any given location are dependent on the plunge angle and as well as the geometry of any secondary folds in those locations. Making the assumption of orthogonality was thus the only practical way in which subsurface orientations could be assigned, which in turn was important for successfully modelling this structurally complex region. Given its intended application(s), this assumption is not expected to have a major impact on the model.
In addition to the data prescribed, the implicit approach taken by GeoModeller inherently considers “geological concepts” when constraining the output. Whilst some of these constrains are highly beneficial (e.g. overlapping or “leaking” geological layers are not permitted), others, such as a tendency towards regularity in formation thickness throughout the domain, were more troublesome. This is because the thickness of some formations, most notably “i6_8”, does change drastically over short distances in our study area. Consequently, some interpolation artefacts such as disconnected “spheres” are present in areas of the model where the gradient in the potential field changes rapidly, particularly near the apices of sharp folds. In such a structurally complex environment, and with a large observation dataset, slightly improved results could have perhaps been obtained by assigning a greater weight to our observations and giving less freedom to the geostatistical interpolation.
When computing the model, the same range value was used for both the interface data and the orientation data. In GeoModeller, it is not possible to specify a different nugget parameter for, say, the interface data according to whether it was derived from the surface maps or the vertical cross-sections. In other words, it is only possible to make a distinction between the interface and orientation data when assigning range and nugget effect values, which are global parameters. This was unfortunate in our case because, being directly observable, the surface data points are much less uncertain than subsurface contacts, which are highly dependent on interpretation. Hence, all observations of a certain type carried an equal weight in our model.
The Quaternary cover is not represented by the model. The only available data pertaining to the unconsolidated Quaternary deposits are a series of shallow boreholes near the source of Le Rippaz, and reveal it to be discontinuous and heterogeneous. The Quaternary fill in the Vallon de Nant itself is likely to be a complex mixture of material having glacial, fluvial, and mass-movement origins. Reconstructing Quaternary cover in such environments is challenging in its own right. However, it is likely to be necessary for reliable hydrogeological flow simulations. As such, following geophysical surveys and their interpretation, plausible realisations of the heterogeneous structures could be generated using stochastic geostatistical simulation approaches such as Multiple Point Statistics 38 . An update of the present (bedrock only) model could potentially then be issued.
In conclusion, no 3D geological model the study region – which now forms the focus of a concerted interdisciplinary research effort – had been published or otherwise made available at the outset of our work. Whilst the development of a model with the requisite characteristics for hydrogeological and other applications was both technically challenging and time consuming, this data gap has now been addressed. The modelled formation interfaces correspond well with the location of these interfaces according to the input data, both at the surface and on available vertical cross-sections. Assuming the geological interpretation presented in the original maps and cross-sections is reasonable, the close matches obtained provide confidence that modelled representation of the geology also acceptable; a view that was upheld by additional comparisons with interpretive sketches.
In the sense that no new primary data has been collected, this work could be considered a data augmentation exercise. Our model thus demonstrates the considerable potential that exists to add value to existing geological data. We argue that it also amounts to more than the sum of its inputs; via the combination of the various input datasets and the process of geostatistical interpolation, it provides insight over the entire domain, ultimately forming an unprecedented geometrical description of the geological formations of the western and northern section of the Nappe de Morcles.
In contrast to certain previous publications which describe the development of 3D geological models only superficially, here, a detailed, step-by-step process has been presented. This should assist future researchers and practitioners in developing complex 3D geological models in future. Again in contrast to most previous instances, the data generated here are made freely-available, and care has been taken to ensure that they can be visualised in open source software. A range of applications across the earth and environmental sciences are likely to benefit if such work is conducted more consistently.
In one ongoing application, the geological model is being employed alongside various other datasets to parameterise physically-based, numerical simulations that integrate snow cover dynamics, surface-water flow, groundwater flow, and evapotranspiration. These simulations intend to elucidate the overall response of mountain hydrological systems to ongoing climatic change.
Finally, having been developed with the stringent, specific requirements of hydrological applications in mind, the model should also be suitable for a range of other applications, including rockfall hazard modelling, sediment provenance identification, hydro-chemical data interpretation, and pedogenic studies.
Usage Notes
Instructions to visualise the model data.
To visualise the surfaces in ParaView (as in Fig. 3 ):
Download the software from https://www.paraview.org/ , and install it.
Under “File”, select “Load State”
Navigate to “Geological_Model_State_File.pvsm”
Under “Load State Options”, select “Search file names under specified directory”, and ensure the directory path is correct.
Individual layers can be made transparent/non transparent by clicking on the “eye” symbol on the left hand side.
To visualise the voxel data in SGeMS:
Download the software from http://sgems.sourceforge.net/ , and install it.
Select “Load Object”, navigate to the file “10 m.SGEMS” and click “Open”.
In the “Select object type” dialogue box, choose “cartesian grid”, and click “Next”.
Provide a name for the grid, and enter the values shown in the following screenshot ( Fig. 9 ), then click “Finish”.
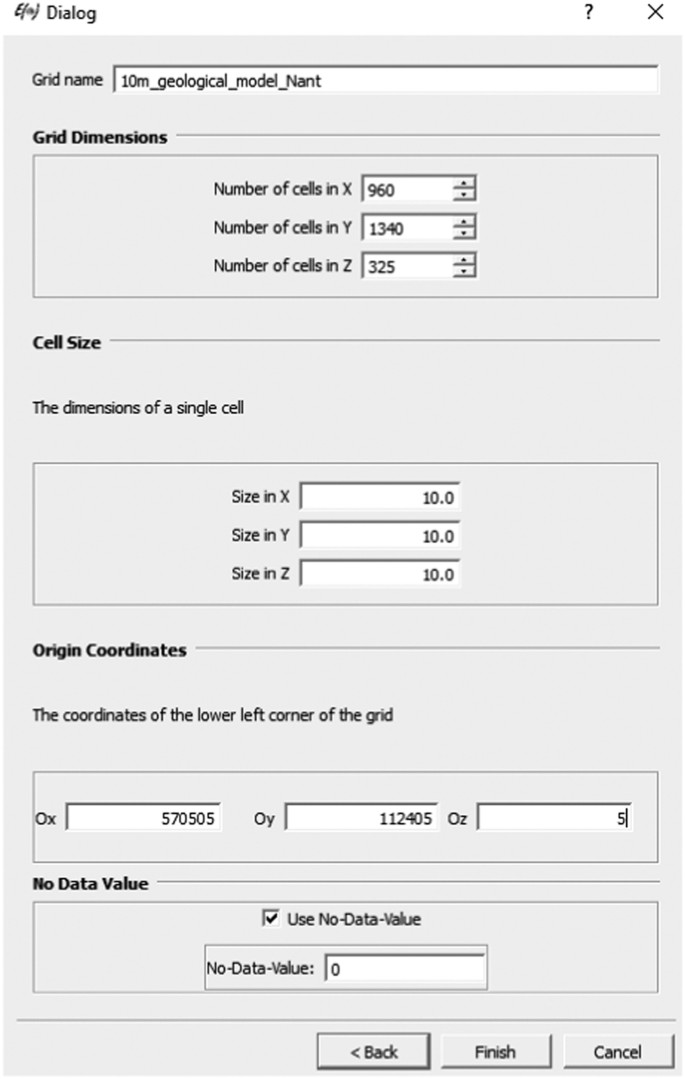
Parameters that should be entered in order to visualise the voxel data in SGeMS.
Once the data are loaded (which may take a few minutes), it may be viewed by checking the two tick boxes under the object tab.
The colour map can be changed if desired using the options under the “Preferences” tab. Also under this tab, by checking “Use Volume Explorer” and “Hide Volume”, a number of slices in the X, Y and Z planes can be visualised using the sliders.
Despite having specified the No-Data-Value on import, it does not seem possible make the grey area transparent. Nor does it seem possible to match the colour scheme in SGeMS to that used in this paper. An illustration of the model visualised in SGeMS is given above ( Supplementary Figure 4 ).
To work with the data in GeoModeller (licence required):
Launch GeoModeller
Open the project by navigating to the “.xml” file provided.
Additional information
How to cite this article : Thornton, J.M. et al . A 3D geological model of a structurally complex Alpine region as a basis for interdisciplinary research. Sci. Data . 5:180238 doi: 10.1038/sdata.2018.238 (2018).
Publisher’s note : Springer Nature remains neutral with regard to jurisdictional claims in published maps and institutional affiliations.
Jones, R. R. et al. Integration of regional to outcrop digital data: 3D visualisation of multi-scale geological models. Comput. Geosci. 35 , 4–18 (2009).
ADS Google Scholar
Béthoux, N. et al. Earthquake relocation using a 3D a-priori geological velocity model from the western Alps to Corsica: Implication for seismic hazard. Tectonophysics 670 , 82–100 (2016).
Vanneschi, C., Salvini, R., Massa, G., Riccucci, S. & Borsani, A. Geological 3D modeling for excavation activity in an underground marble quarry in the Apuan Alps (Italy). Comput. Geosci. 69 , 41–54 (2014).
Bistacchi, A. et al. 3D fold and fault reconstruction with an uncertainty model: An example from an Alpine tunnel case study. Comput. Geosci. 34 , 351–372 (2008).
Mont Terri Project. 3D geological model https://www.mont-terri.ch/en/geology%20/3D-geological-model.html (2017).
Hassen, I. et al. 3D geological modeling of the Kasserine Aquifer System, Central Tunisia: New insights into aquifer-geometry and interconnections for a better assessment of groundwater resources. J. Hydrol. 539 , 223–236 (2016).
Jeannin, P. Y. et al. KARSYS: A pragmatic approach to karst hydrogeological system conceptualisation. Assessment of groundwater reserves and resources in Switzerland. Environ. Earth Sci 69 , 999–1013 (2013).
Google Scholar
Ballesteros, D. et al. KARSYS hydrogeological 3D modeling of alpine karst aquifers developed in geologically complex areas: Picos de Europa National Park (Spain). Environ. Earth Sci 74 , 7699–7714 (2015).
CAS Google Scholar
Malard, A., Jeannin, P.-Y., Vouillamoz, J. & Weber, E. An integrated approach for catchment delineation and conduit-network modeling in karst aquifers: application to a site in the Swiss tabular Jura. Hydrogeol. J. 23 , 1341–1357 (2015).
Turk, J. et al. Hydrogeological characterization of groundwater storage and drainage in an alpine karst aquifer (the Kanin massif, Julian Alps). Hydrol. Process. 29 , 1986–1998 (2015).
Guglielmetti, L., Comina, C., Abdelfettah, Y., Schill, E. & Mandrone, G. Integration of 3D geological modeling and gravity surveys for geothermal prospection in an Alpine region. Tectonophysics 608 , 1025–1036 (2013).
Goldscheider, N. Fold structure and underground drainage pattern in the alpine karst system Hochifen-Gottesacker. Eclogae Geol. Helv. 98 , 1–17 (2005).
Goldscheider, N. & Bartolomé, A. In Methods in karst hydrogeology ( eds. Goldscheider, N. & Drew, D. ) Ch 2. (CRC Press, 2007).
Herold, T., Jordan, P. & Zwahlen, F. The influence of tectonic structures on karst flow patterns in karstified limestones and aquitards in the Jura Mountains, Switzerland. Eclogae Geol. Helv. 93 , 349–362 (2000).
Vouillamoz, N., Sue, C., Champagnac, J. D. & Calcagno, P. 3D cartographic modeling of the Alpine arc. Tectonophysics 579 , 131–143 (2012).
Sue, C. et al. Relationships between inherited crustal structures and seismicity in the western Alps inferred from 3D structural modeling. Bull. la Soc. Geol. Fr 181 , 583–590 (2010).
Schreiber, D., Lardeaux, J. M., Martelet, G., Courrioux, G. & Guillen, A. 3-D modelling of Alpine Mohos in Southwestern Alps. Geophys. J. Int 180 , 961–975 (2010).
Zanchi, A., Francesca, S., Stefano, Z., Simone, S. & Graziano, G. 3D reconstruction of complex geological bodies: Examples from the Alps. Comput. Geosci. 35 , 49–69 (2009).
Horton, P., Schaefli, B., Mezghani, A., Hingray, B. & Musy, A. Assessment of climate-change impacts on alpine discharge regimes with climate model uncertainty. Hydrol. Process. 20 , 2091–2109 (2006).
Schaefli, B., Hingray, B. & Musy, A. Climate change and hydropower production in the Swiss Alps: Quantification of potential impacts and related modelling uncertainties. Hydrol. Earth Syst. Sci. 11 , 1191–1205 (2007).
Addor, N. et al. Robust changes and sources of uncertainty in the projected hydrological regimes of Swiss catchments. Water Resour. Res. 50 , 7541–7562 (2014).
Wagner, T. et al. Impacts of climate change on stream flow and hydro power generation in the Alpine region. Environ. Earth Sci 76 , 4 (2017).
Jenicek, M., Seibert, J. & Staudinger, M. Modeling of future changes in seasonal snowpack and impacts on summer low flows in alpine catchments. Water Resour. Res. 54 , 538–556 (2018).
Lindström, G., Johansson, B., Persson, M., Gardelin, M. & Bergström, S. Development and test of the distributed HBV-96 hydrological model. J. Hydrol. 201 , 272–288 (1997).
Viviroli, D., Zappa, M., Gurtz, J. & Weingartner, R. An introduction to the hydrological modelling system PREVAH and its pre- and post-processing-tools. Environ. Model. Softw 24 , 1209–1222 (2009).
Grayson, R. & Blöschl, G. Spatial patterns in catchment hydrology: observations and modelling . (CUP, 2001).
Efstratiadis, A. & Koutsoyiannis, D. One decade of multi-objective calibration approaches in hydrological modelling: a review Multi-objective calibration approaches in hydrological modelling. Hydrol. Sci. J. – J. des Sci. Hydrol 55 , 58–78 (2010).
Parajka, J., Merz, R. & Blöschl, G. Uncertainty and multiple objective calibration in regional water balance modelling: Case study in 320 Austrian catchments. Hydrol. Process. 21 , 435–446 (2007).
Koch, J., Mendiguren, G., Mariethoz, G. & Stisen, S. Spatial sensitivity analysis of simulated land surface patterns in a catchment model using a set of innovative spatial performance metrics. J. Hydrometeorol. 18 , 1121–1142 (2017).
Kirchner, J. W. Getting the right answers for the right reasons: Linking measurements, analyses, and models to advance the science of hydrology. Water Resour. Res. 42 , W03S04, https://doi.org/10.1029/2005WR004362 (2006).
Hrachowitz, M. & Clark, M. P. HESS Opinions: The complementary merits of competing modelling philosophies in hydrology. Hydrol. Earth Syst. Sci. 21 , 3953–3973 (2017).
Schulla, J. Model Description WaSiM (Water balance Simulation Model) (2017).
Gleeson, T. & Manning, A. H. Regional groundwater flow in mountainous terrain: Three-dimensional simulations of topographic and hydrogeologic controls. Water Resour. Res. 44 , W10403, https://doi.org/10.1029/2008WR006848 (2008).
Kosugi, K. et al. Localized bedrock aquifer distribution explains discharge from a headwater catchment. Water Resour. Res. 47 , W07530, https://doi.org/10.1029/2010WR009884 (2011).
Manning, A. H. & Caine, J. S. Groundwater noble gas, age, and temperature signatures in an Alpine watershed: Valuable tools in conceptual model development. Water Resour. Res. 43 , W04404, https://doi.org/10.1029/2006WR005349 (2007).
Welch, L. A. & Allen, D. M. Hydraulic conductivity characteristics in mountains and implications for conceptualizing bedrock groundwater flow. Hydrogeol. J. 22 , 1003–1026 (2014).
Viviroli, D. & Weingartner, R. The hydrological significance of mountains: from regional to global scale. Hydrol. Earth Syst. Sci. 8 , 1017–1030 (2004).
Beniston, M. et al. The European mountain cryosphere: a review of its current state, trends, and future challenges. Cryosph 12 , 759–794 (2018).
Klein, G., Vitasse, Y., Rixen, C., Marty, C. & Rebetez, M. Shorter snow cover duration since 1970 in the Swiss Alps due to earlier snowmelt more than to later snow onset. Clim. Change 139 , 637–649 (2016).
Vincent, C. et al. Common climatic signal from glaciers in the European Alps over the last 50 years. Geophys. Res. Lett. 44 , 1376–1383 (2017).
Viviroli, D. et al. Climate change and mountain water resources: Overview and recommendations for research, management and policy. Hydrol. Earth Syst. Sci. 15 , 471–504 (2011).
Beniston, M. Impacts of climatic change on water and associated economic activities in the Swiss Alps. J. Hydrol. 412–413 , 291–296 (2012).
Tague, C., Grant, G., Farrell, M., Choate, J. & Jefferson, A. Deep groundwater mediates streamflow response to climate warming in the Oregon Cascades. Clim. Change 86 , 189–210 (2008).
Singleton, M. J. & Moran, J. E. Dissolved noble gas and isotopic tracers reveal vulnerability of groundwater in a small, high-elevation catchment to predicted climate changes. Water Resour. Res. 46 , W00F06, https://doi.org/10.1029/2009WR008718 (2010).
Green T. R., In Integrated Groundwater Management ( eds. Jakeman A. J., Barreteau O., Hunt R. J., Rinaudo J.-D. & Ross A. ) Ch. 5 (Springer International Publishing, 2016).
Maxwell, R. M. & Miller, N. L. Development of a coupled land surface and groundwater model. J. Hydrometeorol. 6 , 233–247 (2005).
Aquanty Inc. HydroGeoSphere Manual - Theory (2015).
Graham, D. & Butts, M. B. In Watershed Models ( eds. Singh, V. P. & Frevert, D. ) Ch. 10 (CRC Press, 2005).
Camporese, M., Paniconi, C., Putti, M. & Orlandini, S. Surface-subsurface flow modeling with path-based runoff routing, boundary condition-based coupling, and assimilation of multisource observation data. Water Resour. Res. 46 , W02512, https://doi.org/10.1029/2008WR007536 (2010).
Argand, E. Sur l’arc des Alpes occidentales. Eclogae Geol. Helv. 45 , 145–191 (1916).
Lugeon, M. & Argand., E. Notice explicative, Feuille 485 Saxon – Morcles, Atlas géologique de la Suisse (1:25,000) (1937).
Lugeon, M. Notice explicative, Feuille 19 - Les Diablerets, Atlas géologique de la Suisse (1:25,000) (1940).
Badoux, H. Carte et notice explicative, Feuille 1305 - Dt. de Morcles, Atlas géologique de la Suisse (1:25,000) (1971).
Badoux, H. Tectonique de la nappe de Morcles entre Rhône et Lizerne, Matériaux pour La carte Géologique de la Suisse . Kümmerly & Frey, (1972).
Ramsay, J. G. Tectonics of the Helvetic Nappes. Geol. Soc. London, Spec. Publ 9 , 293–309 (1981).
Badoux, H. & Gabus, J. H. Carte et notice explicative, Feuille 1285 - Les Diablerets, Atlas géologique de la Suisse (1:25,000) (1991).
Escher, A., Masson, H. & Steck, A. Nappe geometry in the Western Swiss Alps. J. Struct. Geol. 15 , 501–509 (1993).
Pfiffner, O. A. The structure of the Helvetic nappes and its relation to the mechanical stratigraphy. J. Struct. Geol. 15 , 511–521 (1993).
Badoux, H . Aperçu géologique du Vallon de Nant, Revue “La Thomasia” Jardin alpin du Pont de Nant 1891-1991, 2ème partie: Géologie simplifiée de la région de Morcles - Les Diablerets - Vallon de Nant http://clubjurassien.ch/archivePDF/1342426319.pdf (1991).
Bauville, A., Epard, J.-L. & Schmalholz, S. M. A simple thermo-mechanical shear model applied to the Morcles fold nappe (Western Alps). Tectonophysics 583 , 76–87 (2013).
von Tscharner, M., Schmalholz, S. M. & Epard, J.-L. 3-D numerical models of viscous flow applied to fold nappes and the Rawil depression in the Helvetic nappe system (western Switzerland). J. Struct. Geol. 86 , 32–46 (2016).
Dutoit, A. & Dutoit, Annelise. La végétation de l’étage subalpin du vallon de Nant. Sect. Prot. la Nat. des sites Conserv. la faune du Cant. Vaud. (1983).
Von Däniken, I., Guisan, A. & Lane, S. RechAlp.vd: Une nouvelle plateforme UNIL de support pour la recherche transdisciplinaire dans les Alpes vaudoises. Bull. la Société vaudoise des Sci 94 , 175–178 (2014).
Gremaud, V., Goldscheider, N., Savoy, L., Favre, G. & Masson, H. Geological structure, recharge processes and underground drainage of a glacierised karst aquifer system, Tsanfleuron-Sanetsch, Swiss Alps. Hydrogeol. J. 17 , 1833–1848 (2009).
ADS CAS Google Scholar
Lugeon, M. & Gagnebin, E. L’origine des sources de la Chambrette aux Plans sur Bex (Alpes Vaudoises). Bull. la Société vaudoise des Sci 56 .
Municipalite d’Ollon. PREAVIS MUNICIPAL n° 2014 / 08: Acquisition de la parcelle n° 4434 au lieu-dit ‘La Rippaz’ sur le territoire de la Commune de Bex (2014).
Municipalite d’Ollon. PREAVIS MUNICIPAL n° 2015/09: Recaptage des sources de La Rippaz (2015).
Perrin, J. Prospection dans le Vallon de Nant (Bex). Le Trou (Bulletin du Groupe Spéléo Lausanne) 56 , 40–45 (1993).
Aschwanden, H. & Weingartner, R. Die Abflussregimes der Schweiz. Publ. Gewässerkd. Geogr. Inst. der Univ. Bern 65 (1985).
Coe, A. L., Argles, T. W., Rothery, D. A. & Spicer, R. A. Geological Field Techniques , (Wiley-Blackwell, 2010).
Intrepid Geophysics. 3D GeoModeller Reference http://www.intrepid-geophysics.com/ig/uploads/manuals/documentation_pdf_geomodeller/pdf_en/GeoModeller_Reference.pdf (2013).
Calcagno, P., Chilès, J. P., Courrioux, G. & Guillen, A. Geological modelling from field data and geological knowledge. Part I. Modelling method coupling 3D potential-field interpolation and geological rules. Phys. Earth Planet. Inter 171 , 147–157 (2008).
Guillen, A., Calcagno, P., Courrioux, G., Joly, A. & Ledru, P. Geological modelling from field data and geological knowledge. Part II. Modelling validation using gravity and magnetic data inversion. Phys. Earth Planet. Inter 171 , 158–169 (2008).
McInerney, P. & Guillen, A. Building 3D geological models directly from the data? A new approach applied to Broken Hill, Australia. Digit. Mapp. Tech. 05 , 119–130 (2005).
Chilès, J. P., Aug, C., Guillen, A. & Lees, T. Modelling the geometry of geological units and its uncertainty in 3D from structural data: The potential-field method. Orebody Model. Strateg. Mine Plan. - Spectr 14 , 22–24 (2004).
Lajaunie, C., Courrioux, G. & Manuel, L. Foliation fields and 3D cartography in geology: Principles of a method based on potential interpolation. Math. Geol. 29 , 571–584 (1997).
Lorensen, W. E. & Cline, H. E. Marching cubes: A high resolution 3D surface construction algorithm. in Proceedings of the 14th annual conference on Computer graphics and interactive techniques - SIGGRAPH ’87 21 , 163–169 ACM Press, (1987).
Caumon, G., Collon-Drouaillet, P., Le Carlier De Veslud, C., Viseur, S. & Sausse, J. Surface-based 3D modeling of geological structures. Math. Geosci. 41 , 927–945 (2009).
CAS MATH Google Scholar
Maxelon, M., Renard, P., Courrioux, G., Brändli, M. & Mancktelow, N. A workflow to facilitate three-dimensional geometrical modelling of complex poly-deformed geological units. Comput. Geosci. 35 , 644–658 (2009).
Ayachit, U. The ParaView Guide: A Parallel Visualization Application . Kitware, Inc.: USA, (2015).
Remy, N., Boucher, A. & Wu, J. Applied geostatistics with SGeMS: A user’s guide . Cambridge University Press, (2009).
Renard, M., Lagabrielle, Y., Martin, E. & Rafélis, M. Éléments de géologie . (Dunod, 2015).
Data Citations
Thornton, J. M., Mariethoz, G., & Brunner, P. figshare https://doi.org/10.6084/m9.figshare.c.4130759 (2018)
Download references
Acknowledgements
The work was conducted as part of the IntegrAlp project, funded by the Swiss National Science Foundation (SNF project CR23I2_162754). Discussions with J.-L. Epard and A. Parriaux are gratefully acknowledged.
Author information
Authors and affiliations.
Centre for Hydrogeology and Geothermics, University of Neuchâtel, Rue Emile-Argand 11, Neuchâtel, 2000, Switzerland
James M. Thornton & Philip Brunner
Institute of Earth Surface Dynamics, University of Lausanne, Lausanne, 1015, Switzerland
Gregoire Mariethoz
You can also search for this author in PubMed Google Scholar
Contributions
J.M.T. conducted the majority of the work, including sourcing and preparing the input datasets, generating and evaluating the model, preparing the figures, and writing the manuscript. G.M. advised on data processing and participated in the redaction of the manuscript. P.B. contributed the initial idea that a 3D geological model could benefit the planned interdisciplinary research and participated in the redaction of the manuscript.
Corresponding author
Correspondence to James M. Thornton .
Ethics declarations
Competing interests.
The authors declare no competing interests.
ISA-Tab metadata
Supplementary information, supplementary figures (docx 3089 kb), rights and permissions.
Open Access This article is licensed under a Creative Commons Attribution 4.0 International License, which permits use, sharing, adaptation, distribution and reproduction in any medium or format, as long as you give appropriate credit to the original author(s) and the source, provide a link to the Creative Commons license, and indicate if changes were made. The images or other third party material in this article are included in the article’s Creative Commons license, unless indicated otherwise in a credit line to the material. If material is not included in the article’s Creative Commons license and your intended use is not permitted by statutory regulation or exceeds the permitted use, you will need to obtain permission directly from the copyright holder. To view a copy of this license, visit http://creativecommons.org/licenses/by/4.0/ The Creative Commons Public Domain Dedication waiver http://creativecommons.org/publicdomain/zero/1.0/ applies to the metadata files made available in this article.
Reprints and permissions
About this article
Cite this article.
Thornton, J., Mariethoz, G. & Brunner, P. A 3D geological model of a structurally complex Alpine region as a basis for interdisciplinary research. Sci Data 5 , 180238 (2018). https://doi.org/10.1038/sdata.2018.238
Download citation
Received : 21 February 2018
Accepted : 10 September 2018
Published : 30 October 2018
DOI : https://doi.org/10.1038/sdata.2018.238
Share this article
Anyone you share the following link with will be able to read this content:
Sorry, a shareable link is not currently available for this article.
Provided by the Springer Nature SharedIt content-sharing initiative
This article is cited by
A hybrid ensemble-based automated deep learning approach to generate 3d geo-models and uncertainty analysis.
- Abbas Abbaszadeh Shahri
- Shan Chunling
- Stefan Larsson
Engineering with Computers (2023)
- Philippe Calcagno
- Eugenio Trumpy
- Domenico Liotta
Scientific Data (2022)
A 3D geomodel of the deep aquifers in the Orléans area of the southern Paris Basin (France)
- Perrine Mas
- Virginie Hamm
3D geo-cellular modeling for Oligocene reservoirs: a marginal field in offshore Vietnam
- Hung Vo Thanh
- Kang-Kun Lee
Journal of Petroleum Exploration and Production Technology (2022)
Combined Geophysical–Geological Investigation for 3D Geological Modeling: Case of the Jeffara Reservoir Systems, Medenine Basin, SE Tunisia
- Hayet Chihi
- Simge Ayfer
Natural Resources Research (2022)
Quick links
- Explore articles by subject
- Guide to authors
- Editorial policies
Sign up for the Nature Briefing newsletter — what matters in science, free to your inbox daily.

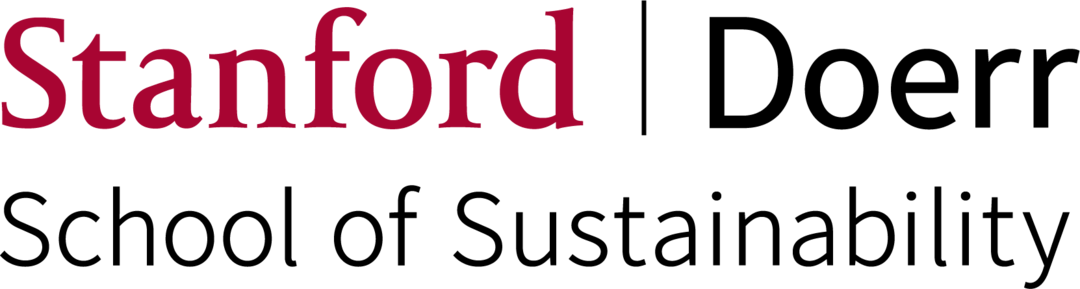
Modeling Geological CO2 Sequestration: Translations Across Spatial Scales and Advancements in Nonlinear Newton Solver
Sally M. Benson, primary advisor Hamdi Tchelepi, primary advisor Peter K. Kitanidis, advisor
Characterization of formation heterogeneity is the first step for constructing geological models that are used in simulating subsurface flow migration, such as CO 2 geological storage and oil/gas recovery. Technologies such as seismic survey, well logging, and core analysis reveal the formation heterogeneity at different scales. Due to the computational limitations on model construction and flow simulation, the gridblock size of a geological model is usually much larger than a core. The common industry practice is to assign core-scale properties directly to a geological model gridblock. The sub-grid- and sub-core-scale heterogeneities are neglected. The objective of this work is two-fold. First, we demonstrate that these small-scale heterogeneities can influence large-scale CO 2 migration during geological storage. Significant modeling error may occur if these heterogeneities are not accounted for properly. Second, we improve the nonlinear convergence performance of numerical simulation, which is a crucial tool for translating small-scale physics in large-scale modeling and for uncertainty quantifications during the scale-up. A new Newton solver is developed, which converges quickly by avoiding the oscillations and overshoots that are often encountered in traditional solvers.
Related Topics
More publications, klinkenberg effect on predicting and measuring helium permeability in gas shales, pore-scale bubble population dynamics of co2-foam at reservoir pressure, extreme capillary heterogeneities and in situ fluid compartmentalization due to clusters of deformation bands in sandstones.

Main navigation
- Sa da Fonseca, J. (2024). Probabilistic assessment of pore pressure prediction with Bayesian Geophysical Basin Modeling [PhD, Stanford University]. https://searchworks.stanford.edu/view/in00000069359
- Wang, Y. (2023). A beautiful marriage between POMDPs and subsurface applications : decision making for subsurface systems [PhD Thesis, Stanford University]. https://searchworks.stanford.edu/view/in00000019458
- Kanfar, R. (2023). Stochastic geomodelling and analysis of karst morphology [PhD Thesis, Stanford University]. https://searchworks.stanford.edu/view/in00000033193
- Kashefi, K. (2023). Deep learning algorithms for computational mechanics on irregular geometries [PhD Thesis, Stanford University]. https://searchworks.stanford.edu/view/14732781
- Hall, T. (2023). Efficient greenfield mineral exploration [PhD Thesis, Stanford University]. https://searchworks.stanford.edu/view/in00000019459
- Wang, L. (2023). Integrating data and models for sustainable decision-making in hydrology [PhD Thesis, Stanford University]. https://searchworks.stanford.edu/view/14641244
- Miltenberger, A. (2022). A measure-theoretic approach to Bayesian hypothesis testing and inversion with geophysical data [PhD Thesis, Stanford University]. https://searchworks.stanford.edu/view/14233991
- Yang, L. (2021). Quantifying and visualizing uncertainty of 3D geological structures with implicit methods [PhD Thesis, Stanford University]. https://searchworks.stanford.edu/view/14641244
- Petrov, S. (2021). Seismic image segmentation with deep learning [MS Thesis, Stanford University]. https://searchworks.stanford.edu/view/qf836dh0076
- Athens, N. (2021). Stochastic inversion of gravity data in fault-controlled geothermal systems [PhD Thesis, Stanford University]. https://searchworks.stanford.edu/view/13826071
- Pollack, A. (2020). Quantifying Geological Uncertainty and Optimizing Technoeconomic Decisions for Geothermal Reservoirs [PhD Thesis, Stanford University]. https://searchworks.stanford.edu/view/13680060
- Pradhan, A. (2020). Statistical learning and inference of subsurface properties under complex geological uncertainty with seismic data [PhD Thesis, Stanford University]. https://searchworks.stanford.edu/view/13753880
- Nesvold, E. (2019). Building informative priors for the subsurface with generative adversarial networks and graphs [PhD Thesis, Stanford University]. https://searchworks.stanford.edu/view/13423377
- Muradov, R. (2019). Inference of Sub-Resolution Stacking Patterns from Seismic Data in Spatially Coupled Models [MS Thesis, Stanford University]. https://searchworks.stanford.edu/view/km001pf4033
- Al Ibrahim, M. (2019). Petroleum System Modeling of Heterogeneous Organic-Rich Mudrocks [PhD Thesis, Stanford University]. https://searchworks.stanford.edu/view/13250212
- Park, J. (2019). Uncertainty quantification and sensitivity analysis of geoscientific predictions with data-driven approaches [PhD Thesis, Stanford University]. https://searchworks.stanford.edu/view/13250154
- Mendes, J. (2018). Morphdynamic Analysis and Statistical Synthesis of Geomorphic Data [PhD Thesis, Stanford University]. https://searchworks.stanford.edu/view/12746435
- Dutta, G. (2018). Value of Information Analysis for Time-Lapse Seismic Data in Reservoir Development [PhD Thesis, Stanford University]. https://searchworks.stanford.edu/view/12742067
- Li, L. (2017). A Bayesian Approach to Causal and Evidential Analysis for Uncertainty Quantification throughout the Reservoir Forecasting Process [PhD Thesis, Stanford University]. https://searchworks.stanford.edu/view/12137330
- Aydin, O. (2017). A Bayesian Framework for Quantifying Fault Network Uncertainty Using a Marked Point Process Model [PhD Thesis, Stanford University]. https://searchworks.stanford.edu/view/11950552
- Grujić, O. (2017). A Subsurface Modeling with Functional Data [PhD Thesis, Stanford University]. https://searchworks.stanford.edu/view/12212152
- Yang, G. (2017). Holistic Strategies for Prediction Uncertainty Quantification of Contaminant Transport and Reservoir Production in Field Cases [PhD Thesis, Stanford University]. https://searchworks.stanford.edu/view/12123097
- Tong, Y. (2016). Basin and Petroleum System Modeling with Uncertainty Quantification: a Case Study on the Piceance Basin, Colorado [PhD Thesis, Stanford University]. https://searchworks.stanford.edu/view/11685974
- Luebbert, L. (2016). Quantitative Analysis of Dissimilarities Between Different Methods of Seismic Inversion to Facies Realizations [MS Thesis, Stanford University]. https://searchworks.stanford.edu/view/11956907
- Shin, Y. (2016). Reservoir Modeling with Multiple Geological Scenarios for Deformation of Reservoir Structure and Evolution of Reservoir Properties [PhD Thesis, Stanford University]. https://searchworks.stanford.edu/view/11616857
- Xue, C. (2016). The Application of OPTSPACE Algorithm and Comparison with LMAFIT Algorithm in Three dimensional Seismic Data Reconstruction via Lowrank Matrix Completion [MS Thesis, Stanford University]. https://searchworks.stanford.edu/view/11700910
- Lee, J. (2015). Joint Integration of Time-Lapse Seismic, Electromagnetic, and Production Data for Reservoir Monitoring and Management [PhD Thesis, Stanford University]. https://searchworks.stanford.edu/view/11391864
- Satija, A. (2015). Reservoir Forecasting Based on Statistical Functional Analysis of Data and Prediction Variables [PhD Thesis, Stanford University]. https://searchworks.stanford.edu/view/11061336
- Wang, Y. (2015). Rule-Based Reservoir Modeling by Integration of Multiple Information Sources: Learning Time-Varying Geologic Processes [MS Thesis, Stanford University]. https://searchworks.stanford.edu/view/11085541
- Xu, S. (2014). Integration of geomorphic experiment data in surface-based modeling: from characterization to simulation [PhD Thesis, Stanford University]. https://searchworks.stanford.edu/view/10403736
- Jeong, C. (2014). Quantitative Reservoir Characterization Integrating Seismic Data and Geological Scenario Uncertainty [PhD Thesis, Stanford University]. https://searchworks.stanford.edu/view/10530921
- Suman, A. (2013). Joint inversion of production and time-lapse seismic data: application to Norne field [PhD Thesis, Stanford University]. https://searchworks.stanford.edu/view/9959027
- Bertoncello, A. (2011). Conditioning surface-based models to well and thickness data [PhD Thesis, Stanford University]. https://searchworks.stanford.edu/view/9274420
- Park, K. (2011). Modeling Uncertainty in Metric Space [PhD Thesis, Stanford University]. https://searchworks.stanford.edu/view/9100156
- Honarkhah, M. (2011). Stochastic simulation of patterns using distance-based pattern modeling [PhD Thesis, Stanford University]. https://searchworks.stanford.edu/view/9238345
- Wang, J. (2010). A Metropolis sampling method to assess uncertainty of seismic impedance inverted from seismic amplitude data [MS Thesis, Stanford University]. https://searchworks.stanford.edu/view/ch090ff5484
- Haugen, M. (2010). Exploring direct sampling and iterative spatial resampling in history matching [MS Thesis, Stanford University]. https://searchworks.stanford.edu/view/dn958js5816
- Jia, B. (2010). Linking geostatistics with basin and petroleum system modeling: Assessment of spatial uncertainties [MS Thesis, Stanford University]. https://searchworks.stanford.edu/view/mv127hj3223
- Trainor-Guitton, W. (2010). On the value of information for spatial problems in the Earth sciences [PhD Thesis, Stanford University]. https://searchworks.stanford.edu/view/8652432
- Kuralkhanov, D. (2010). Study of pattern correlation between time lapse seismic data and saturation changes [MS Thesis, Stanford University]. https://searchworks.stanford.edu/view/xj886by3185
- Leiva, A. (2009). Construction of hybrid geostatistical models combining surface based methods with object-based simulation: use of flow direction and drainage area [MS Thesis, Stanford University]. https://searchworks.stanford.edu/view/8390172
- Suman, A. (2009). Uncertainties in rock pore compressibility and effects on seismic history matching [MS Thesis, Stanford University]. https://searchworks.stanford.edu/view/8390194
- Polyakova, E. (2008). A general theory for evaluating joint data interaction when combining diverse data sources [PhD Thesis, Stanford University]. https://searchworks.stanford.edu/view/7860490
- Fadaei, S. (2008). Streamline assisted history matching of naturally fractured reservoirs using the probability perturbation method [MS Thesis, Stanford University]. https://searchworks.stanford.edu/view/7814802
3D Geological Model of a Tunnel for Improving Construction Measures: A Case Study
- Original Paper
- Published: 25 August 2023
- Volume 42 , pages 975–989, ( 2024 )
Cite this article
- Kang Wang 1 ,
- Weidong Guo 2 ,
- Shaoshuai Shi ORCID: orcid.org/0000-0001-8984-9809 2 ,
- Ruijie Zhao 2 &
- Xin Wang 2
408 Accesses
Explore all metrics
As the demand for information technology in tunnel construction continues to increase, geological three-dimensional visualization methods have become the forefront of tunnel construction research. This paper analyzes the requirements and main issues faced in three-dimensional modeling for tunnel engineering. To meet the high precision and high timeliness requirements of geological modeling in tunnel engineering, this study establishes a rapid three-dimensional reconstruction method for tunnel engineering models based on geological feature points and line data. Line data is used to construct the model boundaries, while point data is utilized for internal geological description through local Kriging interpolation. The adoption of a unified data format effectively improves the modeling speed. This modeling method integrates advanced geological prediction data, significantly enhancing the model's accuracy. Through the three-dimensional geological model, the geological conditions ahead of the tunnel face are analyzed. The information presented in the three-dimensional geological model is validated through drilling verification and found to be accurate. Subsequently, based on the information obtained from the three-dimensional geological model, the tunnel construction scheme is optimized. Additional localized radial grouting measures are supplemented on the basis of continuous advance peripheral grouting. As a result, tunnel safety construction is achieved by reducing the high geological risk length, thereby shortening the construction period. The approach presented in this paper can be widely applied in relevant tunnels and underground engineering projects.
This is a preview of subscription content, log in via an institution to check access.
Access this article
Price includes VAT (Russian Federation)
Instant access to the full article PDF.
Rent this article via DeepDyve
Institutional subscriptions
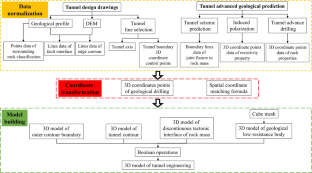
Similar content being viewed by others
The Design Geological and Geotechnical Model (DGGM) for Long and Deep Tunnels
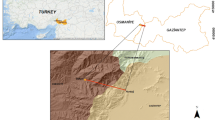
Using 3D Numerical Analysis for the Assessment of Tunnel–Landslide Relationship: Bahce–Nurdag Tunnel (South of Turkey)
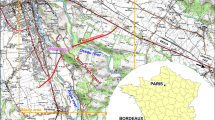
Geological model for a tunnel in Pyrenees (France): importance of the feedback loop based on re-examined investigation data
Data availability.
Enquiries about data availability should be directed to the authors.
Alimoradi A, Moradzadeh A, Naderi R, Salehi MZ, Etemadi A (2008) Prediction of geological hazardous zones in front of a tunnel face using TSP-203 and artificial neural networks. Tunn Undergr Space Technol 23(6):711–717. https://doi.org/10.1016/j.tust.2008.01.001
Article Google Scholar
An Z, Di Q, Wu F, Wang G, Wang R (2012) Geophysical exploration for a long deep tunnel to divert water from the Yangtze to the Yellow River, China. Bull Eng Geol Environ 71(1):195–200. https://doi.org/10.1007/s10064-011-0358-7
Brandel S, Schneider S, Perrin M, Guiard N, Rainaud JF, Lienhard P, Bertrand Y (2005) Automatic building of structured geological models. J Comput Inf Sci Eng 5(2):138–148. https://doi.org/10.1115/1.1884145
Cao BF, Luo XR, Zhang LK, Lei YH, Zhou JS (2019) Petrofacies prediction and 3-D geological model in tight gas sandstone reservoirs by integration of well logs and geostatistical modeling. Marine Petrol Geol 114:104202. https://doi.org/10.1016/j.marpetgeo.2019.104202
Chen G, Wu ZZ, Wang FJ, Ma YL (2011) Study on the application of a comprehensive technique for geological prediction in tunneling. Environ Earth Sci 62(8):1667–1671. https://doi.org/10.1007/s12665-010-0651-y
Article ADS Google Scholar
Chen YH, Cheng S, Li LP, Yang JY, Tu WF (2021) Applicability analysis of microseismic technology in tunnel water inrush monitoring. KSCE J Civil Eng. https://doi.org/10.1007/s12205-021-1545-0
Chu HD, Xu GL, Yasufuku N, Yu Z, Liu PL, Wang JF (2017) Risk assessment of water inrush in karst tunnels based on two-class fuzzy comprehensive evaluation method. Arab J Geosci 10(7):179. https://doi.org/10.1007/s12517-017-2957-5
Christian JT (1996) 3D geoscience modeling: Computer techniques for geological characterization. Earth Sci Rev. https://doi.org/10.1016/0012-8252(95)00067-4
Dusseault MB (2011) Geomechanical challenges in petroleum reservoir exploitation. KSCE J Civ Eng 15(4):669–678. https://doi.org/10.1016/j.gr.2019.11.014
Fan HY, Li LP, Liu HL, Shi SS, Hu J, Zhou S (2021) Advanced stability analysis of the tunnels in jointed rock mass based on TSP and DEM. KSCE J Civ Eng 25(4):1491–1503. https://doi.org/10.1007/s12205-021-0170-2
Ford JR, Mathers SJ, Royse KR, Aldiss DT, Morgan DJR (2010) Geological 3D modelling: scientific discovery and enhanced understanding of the subsurface, with examples from the UK. Zeitschrift Der Deutschen Gesellschaft Fur Geowissenschaften 161(2):205–218. https://doi.org/10.1127/1860-1804/2010/0161-0205
Gao L, Lu YT, Yu PP, Xiao F (2017) Three-dimensional visualization and quantitative prediction for mine: a case study in Xiayuandong Pb-Zn ore deposits, Pangxidong region, southern part of Qin-Hang metallogenic belt, China. Acta Petrologica Sinica 33:767–778
Google Scholar
Hassanpour J, Khoshkar AS, Farasani MG, Hashemnejad A (2022) Investigating the relationships between rock mass classification systems based on data from mechanized tunneling projects in Iran. Bull Eng Geol Environ. https://doi.org/10.1007/s10064-022-02641-y
Hoyer AS, Jorgensen F, Sandersen PBE, Viezzoli A, Moller I (2015) 3D geological modelling of a complex buried-valley network delineated from borehole and AEM data. J Appl Geophys 122:94–102. https://doi.org/10.1016/j.jappgeo.2015.09.004
Jin BX, Fang YM, Song WW (2011) 3D visualization model and key techniques for digital mine. Trans Nonferrous Met Soc China 21:S748–S752. https://doi.org/10.1016/S1003-6326(12)61674-4
Kaewkongkaew K, Phien-Wej N, Kham-Ai D (2015) Prediction of rock mass along tunnels by geostatistics. KSCE J Civ Eng 19(1):81–90. https://doi.org/10.1007/s12205-014-0505-3
Lin CJ, Wang XT, Nie LC, Sun HF, Xu ZH, Du YC, Liu L (2020) Comprehensive geophysical investigation and analysis of lining leakage for water-rich rock tunnels: a case study of Kaiyuan Tunnel, Jinan, China. Geotech Geol Eng 38:3449–3468. https://doi.org/10.1007/s10706-020-01225-5
Li SC, Liu B, Xu XJ, Nie LC, Liu ZY, Song J, Sun HF, Chen L, Fan KR (2017a) An overview of ahead geological prospecting in tunneling. Tunn Undergr Space Technol 63:69–94. https://doi.org/10.1016/j.tust.2016.12.011
Li SC, Wu J, Xu ZH, Li LP (2017b) Unascertained measure model of water and mud inrush risk evaluation in karst tunnels and its engineering application. KSCE J Civ Eng 21(4):1170–1182. https://doi.org/10.1007/s12205-016-1569-z
Liu B, Zhang FK, Li SC, Li Y, Xu S, Nie LC, Zhang CM, Zhang QS (2018) Forward modelling and imaging of ground-penetrating radar in tunnel ahead geological prospecting. Geophys Prospect 66(4):784–797. https://doi.org/10.1111/1365-2478.12613
Liu YT, Qiao J, Han TY, Li LH, Xu T (2020) A 3D image reconstruction model for long tunnel geological estimation. J Adv Transp. https://doi.org/10.1155/2020/8846955
Ma JJ, Li TB, Yang G, Dai KK, Ma CC, Tang H, Wang GW, Wang JF, Xiao B, Meng LB (2023) A real-time intelligent classification model using machine learning for tunnel surrounding rock and its application. Georisk Assess Manag Risk Eng Syst Geohazards 17(1):148–168. https://doi.org/10.1080/17499518.2023.2182891
Article CAS Google Scholar
Maleki Z, Farhadian H, Nikvar-Hassani A (2021) Geological hazards in tunnelling: the example of Gelas water conveyance tunnel, Iran. Q J Eng Geol Hydrogeol 54:1. https://doi.org/10.1144/qjegh2019-114
Mallet JL (1992) Discrete smooth interpolation in geometric modeling. Comput Aided Des 24(4):178–191. https://doi.org/10.1016/0010-4485(92)90054-E
Mei G (2014) Summary on several key techniques in 3D geological modeling. Sci World J. https://doi.org/10.1155/2014/723832
Oliver MA, Webster R (2014) A tutorial guide to geostatistics: computing and modelling variograms and kriging. CATENA 113:56–69. https://doi.org/10.1016/j.catena.2013.09.006
Peng YX, Wu L, Chen CH, Yue J (2020) Stability analysis of surrounding rock in tunnel crossing water-rich fault based on catastrophe theory. Geotech Geol Eng 38:415–423. https://doi.org/10.1007/s10706-019-01032-7
Shao YL, Zheng AL, He YB, Xiao KY (2012) 3D Geological modeling under extremely complex geological conditions. J Comput. https://doi.org/10.4304/jcp.7.3.699-705
Sun SQ, Li LP, Wang J, Liu H, Fang Z (2019a) Analysis and prediction of structural plane connectivity in tunnel based on digitalizing image. KSCE J Civ Eng 23(6):1–11. https://doi.org/10.1007/s12205-019-1000-7
Sun SQ, Li SC, Li LP, Shi SS, Wang J, Hu J, Hu C (2019b) Slope stability analysis and protection measures in bridge and tunnel engineering: a practical case study from Southwestern China. Bull Eng Geol Env 78:3305–3321. https://doi.org/10.1007/s10064-018-1362-y
Taherian A (2023) TBM tunneling hazards identification and mitigation methods through subterranean rivers: an evaluation of nowsud tunnel in the Iran. Geotech Geol Eng 41:1673–1687. https://doi.org/10.1007/s10706-022-02344-x
Tian SM, Wei W, Gong JF (2021) Development and prospect of railway tunnels in China. Tunnel Construction 41(2):308–325
Turner AK (2006) Challenges and trends for geological modelling and visualisation. Bull Eng Geol Environ 65:109–127. https://doi.org/10.1007/s10064-005-0015-0
Wu LY, Bai HB, Ma D (2021) Prediction and prevention of water inrush hazards from bed separation space. Mine Water Environ 40(3):657–670. https://doi.org/10.1007/s10230-020-00748-w
Xia Q, Xu M, Zhang H, Zhang Q, Xiao XX (2018) A dynamic modeling approach to simulate groundwater discharges into a tunnel from typical heterogenous geological media during continuing excavation. KSCE J Civ Eng 22:341–350. https://doi.org/10.1007/s12205-017-0668-9
Xue YG, Kong FM, Qiu DH, Su MX, Zhao Y, Zhang K (2021) The classifications of water and mud/rock inrush hazard: a review and update. Bull Eng Geol Environ 80(3):1907–1925. https://doi.org/10.1007/s10064-020-02012-5
Yang WM, Yang X, Fang ZD, Shi SS, Wang H, Bu L, Li LP, Zhou ZQ (2019) Model test for water inrush caused by karst caves filled with confined water in tunnels. Arab J Geosci 12(24):749. https://doi.org/10.1007/s12517-019-4907-x
Zakrevskiy KE, Popov VL (2021) The history of development of 3D geology modeling as a method for studying oil and gas reservoirs. Bull Tomsk Polytech Univ Geo Assets Eng 332(5):89–100. https://doi.org/10.18799/24131830/2021/05/3188
Zhou D, Liu ZH, Liang RY, Wu H, Wang YT (2018) A method to extract and eliminate TEM interference by metallic bodies in tunnel geological anomaly forecast. Geotech Test J 41:1. https://doi.org/10.1520/GTJ20160287
Download references
Acknowledgements
The authors thank the editor and anonymous reviewers for their valuable advices. This research was supported by National Natural Science Foundation of China (Grant Numbers 52278404 and 51979154) and Taishan Scholar Foundation of Shandong Province (Grant Number tsqn202103002) and Future scholars project of Shandong University, which are gratefully acknowledged.
This research was supported by National Natural Science Foundation of China (Grant Numbers 52278404 and 51979154) and Taishan Scholar Foundation of Shandong Province (Grant Number tsqn202103002) and Future scholars project of Shandong University.
Author information
Authors and affiliations.
School of Qilu Transportation, Shandong University, Jinan, 250061, China
Department of Geotechnical and Structural Engineering Research Center, Shandong University, Jinan, 250061, China
Weidong Guo, Shaoshuai Shi, Ruijie Zhao & Xin Wang
You can also search for this author in PubMed Google Scholar
Corresponding author
Correspondence to Shaoshuai Shi .
Ethics declarations
Conflict of interest.
The authors have no relevant financial or non-financial interests to disclose.
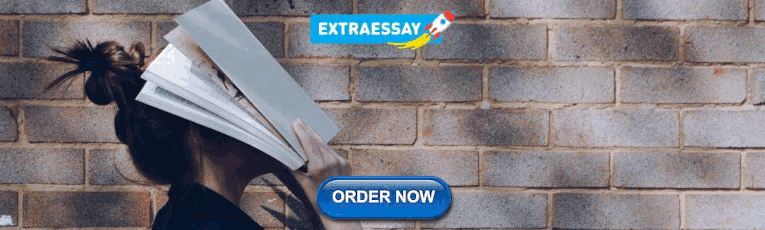
Additional information
Publisher's note.
Springer Nature remains neutral with regard to jurisdictional claims in published maps and institutional affiliations.
Rights and permissions
Springer Nature or its licensor (e.g. a society or other partner) holds exclusive rights to this article under a publishing agreement with the author(s) or other rightsholder(s); author self-archiving of the accepted manuscript version of this article is solely governed by the terms of such publishing agreement and applicable law.
Reprints and permissions
About this article
Wang, K., Guo, W., Shi, S. et al. 3D Geological Model of a Tunnel for Improving Construction Measures: A Case Study. Geotech Geol Eng 42 , 975–989 (2024). https://doi.org/10.1007/s10706-023-02599-y
Download citation
Received : 08 September 2022
Accepted : 25 July 2023
Published : 25 August 2023
Issue Date : March 2024
DOI : https://doi.org/10.1007/s10706-023-02599-y
Share this article
Anyone you share the following link with will be able to read this content:
Sorry, a shareable link is not currently available for this article.
Provided by the Springer Nature SharedIt content-sharing initiative
- Karst tunnel
- Three-dimensional model
- Geological analysis
- Tunnel construction
- Water inrush
- Find a journal
- Publish with us
- Track your research
Information
- Author Services
Initiatives
You are accessing a machine-readable page. In order to be human-readable, please install an RSS reader.
All articles published by MDPI are made immediately available worldwide under an open access license. No special permission is required to reuse all or part of the article published by MDPI, including figures and tables. For articles published under an open access Creative Common CC BY license, any part of the article may be reused without permission provided that the original article is clearly cited. For more information, please refer to https://www.mdpi.com/openaccess .
Feature papers represent the most advanced research with significant potential for high impact in the field. A Feature Paper should be a substantial original Article that involves several techniques or approaches, provides an outlook for future research directions and describes possible research applications.
Feature papers are submitted upon individual invitation or recommendation by the scientific editors and must receive positive feedback from the reviewers.
Editor’s Choice articles are based on recommendations by the scientific editors of MDPI journals from around the world. Editors select a small number of articles recently published in the journal that they believe will be particularly interesting to readers, or important in the respective research area. The aim is to provide a snapshot of some of the most exciting work published in the various research areas of the journal.
Original Submission Date Received: .
- Active Journals
- Find a Journal
- Proceedings Series
- For Authors
- For Reviewers
- For Editors
- For Librarians
- For Publishers
- For Societies
- For Conference Organizers
- Open Access Policy
- Institutional Open Access Program
- Special Issues Guidelines
- Editorial Process
- Research and Publication Ethics
- Article Processing Charges
- Testimonials
- Preprints.org
- SciProfiles
- Encyclopedia
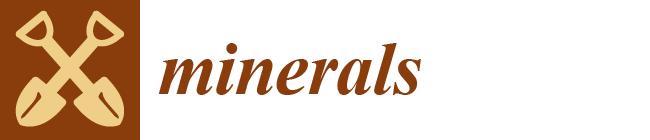
Article Menu
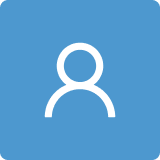
- Subscribe SciFeed
- Recommended Articles
- Google Scholar
- on Google Scholar
- Table of Contents
Find support for a specific problem in the support section of our website.
Please let us know what you think of our products and services.
Visit our dedicated information section to learn more about MDPI.
JSmol Viewer
3d mineral prospectivity mapping from 3d geological models using return–risk analysis and machine learning on imbalance data.
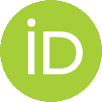
1. Introduction
2. study area and 3d geological models, 2.1. geological setting of the study area, 2.2. ore-controlling factors, 2.3. the 3d evidence layers of geological modeling, 3. methodologies, 3.1. borderline-smote, 3.2. random forest algorithm, 3.3. evaluation metrics, 3.4. return–risk analysis, 4. results and discussion, 5. conclusions, author contributions, data availability statement, acknowledgments, conflicts of interest.
- Kreuzer, O.P.; Markwitz, V.; Porwal, A.K.; McCuaig, T.C. A continent-wide study of Australia’s uranium potential: Part I: GIS-assisted manual prospectivity analysis. Ore Geol. Rev. 2010 , 38 , 334–366. [ Google Scholar ] [ CrossRef ]
- Kohanpour, F.; Lindsay, M.D.; Occhipinti, S.; Gorczyk, W. Structural controls on proterozoic nickel and gold mineral systems identified from geodynamic modelling and geophysical interpretation, east Kimberley, Western Australia. Ore Geol. Rev. 2018 , 95 , 552–568. [ Google Scholar ] [ CrossRef ]
- Rodriguez-Galiano, V.; Sanchez-Castillo, M.; Chica-Olmo, M.; Chica-Rivas, M. Machine learning predictive models for mineral prospectivity: An evaluation of neural networks, random forest, regression trees and support vector machines. Ore Geol. Rev. 2015 , 71 , 804–818. [ Google Scholar ] [ CrossRef ]
- Carranza, E.J.M. Geochemical Anomaly and Mineral Prospectivity Mapping in GIS ; Elsevier: Amsterdam, The Netherlands, 2009; Volume 11. [ Google Scholar ]
- Agterberg, F.P. Computer programs for mineral exploration. Science 1989 , 245 , 76–81. [ Google Scholar ] [ CrossRef ] [ PubMed ]
- Bérubé, C.L.; Olivo, G.R.; Chouteau, M.; Perrouty, S.; Shamsipour, P.; Enkin, R.J.; Morris, W.A.; Feltrin, L.; Thiémonge, R. Predicting rock type and detecting hydrothermal alteration using machine learning and petrophysical properties of the Canadian Malartic ore and host rocks, Pontiac Subprovince, Québec, Canada. Ore Geol. Rev. 2018 , 96 , 130–145. [ Google Scholar ] [ CrossRef ]
- Bonham-Carter, G.F. Geographic Information Systems for Geoscientists: Modelling with GIS ; Pergamon: Toronto, ON, Canada, 1994; p. 398. [ Google Scholar ]
- Yousefi, M.; Nykänen, V. Data-driven logistic-based weighting of geochemical and geological evidence layers in mineral prospectivity mapping. J. Geochem. Explor. 2016 , 164 , 94–106. [ Google Scholar ] [ CrossRef ]
- Carranza, E.J.M. Natural Resources Research Publications on Geochemical Anomaly and Mineral Potential Mapping, and Introduction to the Special Issue of Papers in These Fields. Nat. Resour. Res. 2017 , 26 , 379–410. [ Google Scholar ] [ CrossRef ]
- Yousefi, M.; Carranza, E.J.M. Fuzzification of continuous-value spatial evidence for mineral prospectivity mapping. Comput. Geosci. 2015 , 74 , 97–109. [ Google Scholar ] [ CrossRef ]
- Porwal, A.; Carranza, E.J.M. Introduction to the Special Issue: GIS-based mineral potential modelling and geological data analyses for mineral exploration. Ore Geol. Rev. 2015 , 71 , 477–483. [ Google Scholar ] [ CrossRef ]
- Zuo, R.G.; Cheng, Q.M.; Agterberg, F.P. Application of a hybrid method combining multilevel fuzzy comprehensive evaluation with asymmetric fuzzy relation analysis to mapping prospectivity. Ore Geol. Rev. 2009 , 35 , 101–108. [ Google Scholar ] [ CrossRef ]
- Houlding, S.W. Introduction. In 3D Geoscience Modeling: Computer Techniques for Geological Characterization ; Springer: Berlin/Heidelberg, Germany, 1994; pp. 1–4. ISBN 978-3-642-79012-6. [ Google Scholar ]
- Kaufmann, O.; Martin, T. 3D geological modelling from boreholes, cross-sections and geological maps, application over former natural gas storages in coal mines. Comput. Geosci. 2008 , 34 , 278–290. [ Google Scholar ] [ CrossRef ]
- Wang, G.; Zhang, S.; Yan, C.; Song, Y.; Sun, Y.; Li, D.; Xu, F. Mineral potential targeting and resource assessment based on 3D geological modeling in Luanchuan region, China. Comput. Geosci. 2011 , 37 , 1976–1988. [ Google Scholar ] [ CrossRef ]
- Wang, G.; Ma, Z.; Li, R.; Song, Y.; Qu, J.; Zhang, S.; Yan, C.; Han, J. Integration of multi-source and multi-scale datasets for 3D structural modeling for subsurface exploration targeting, Luanchuan Mo-polymetallic district, China. J. Appl. Geophys. 2017 , 139 , 269–290. [ Google Scholar ] [ CrossRef ]
- Chen, J.P.; Lu, P.; Wu, W.; Zhao, J.; Hu, Q. A 3-D Prediction Method for Blind Orebody Based on 3-D Visualization Model and Its Application. Earth Sci. Front. 2007 , 14 , 54–61. [ Google Scholar ] [ CrossRef ]
- Zuo, R.G.; Carranza, E.J.M. Support vector machine: A tool for mapping mineral prospectivity. Comput. Geosci. 2011 , 37 , 1967–1975. [ Google Scholar ] [ CrossRef ]
- Wang, G.C.; Carr, T.R.; Ju, Y.W.; Li, C.F. Identifying organic-rich Marcellus Shale lithofacies by support vector machine classifier in the Appalachian basin. Comput. Geosci. 2014 , 64 , 52–60. [ Google Scholar ] [ CrossRef ]
- Hariharan, S.; Tirodkar, S.; Porwal, A.; Bhattacharya, A.; Joly, A. Random Forest-Based Prospectivity Modelling of Greenfield Terrains Using Sparse Deposit Data: An Example from the Tanami Region, Western Australia. Nat. Resour. Res. 2017 , 26 , 489–507. [ Google Scholar ] [ CrossRef ]
- Carranza, E.J.M.; Laborte, A.G. Random forest predictive modeling of mineral prospectivity with small number of prospects and data with missing values in Abra (Philippines). Comput. Geosci. 2015 , 74 , 60–70. [ Google Scholar ] [ CrossRef ]
- Zhang, Z.Q.; Zhang, J.J.; Wang, G.W.; Carranza, E.J.M.; Pang, Z.; Wang, H. From 2D to 3D Modeling of Mineral Prospectivity Using Multi-source Geoscience Datasets, Wulong Gold District, China. Nat. Resour. Res. 2020 , 29 , 345–364. [ Google Scholar ] [ CrossRef ]
- Li, H.; Li, X.H.; Yuan, F.; Jowitt, S.M.; Zhang, M.M.; Zhou, J.; Zhou, T.F.; Li, X.L.; Ge, C.; Wu, B.C. Convolutional neural network and transfer learning based mineral prospectivity modeling for geochemical exploration of Au mineralization within the Guandian–Zhangbaling area, Anhui Province, China. Appl. Geochem. 2020 , 122 , 104747. [ Google Scholar ] [ CrossRef ]
- Li, S.; Chen, J.P.; Liu, C.; Wang, Y. Mineral Prospectivity Prediction via Convolutional Neural Networks Based on Geological Big Data. J. Earth Sci. 2021 , 32 , 327–347. [ Google Scholar ] [ CrossRef ]
- Yang, N.; Zhang, Z.K.; Yang, J.H.; Hong, Z.L.; Shi, J. A Convolutional Neural Network of GoogLeNet Applied in Mineral Prospectivity Prediction Based on Multi-Source Geoinformation. Nat. Resour. Res. 2021 , 30 , 3905–3923. [ Google Scholar ] [ CrossRef ]
- Xiong, Y.; Zuo, R.; Carranza, E.J.M. Mapping mineral prospectivity through big data analytics and a deep learning algorithm. Ore Geol. Rev. 2018 , 102 , 811–817. [ Google Scholar ] [ CrossRef ]
- Xiong, Y.H.; Zuo, R.G. Recognizing multivariate geochemical anomalies for mineral exploration by combining deep learning and one-class support vector machine. Comput. Geosci. 2020 , 140 , 104484. [ Google Scholar ] [ CrossRef ]
- Singh, R.K.; Ray, D.; Sarkar, B.C. Recurrent neural network approach to mineral deposit modelling. In Proceedings of the 2018 4th International Conference on Recent Advances in Information Technology (RAIT), Dhanbad, India, 15–17 March 2018; IEEE: Piscataway, NJ, USA, 2018. [ Google Scholar ]
- Wang, Z.; Zuo, R. Mineral prospectivity mapping using a joint singularity-based weighting method and long short-term memory network. Comput. Geosci. 2022 , 158 , 104974. [ Google Scholar ] [ CrossRef ]
- Xu, Y.Y.; Li, Z.X.; Xie, Z.; Cai, H.H.; Niu, P.F.; Liu, H. Mineral prospectivity mapping by deep learning method in Yawan-Daqiao area, Gansu. Ore Geol. Rev. 2021 , 138 , 104316. [ Google Scholar ] [ CrossRef ]
- Zhang, C.J.; Zuo, R.G. Recognition of multivariate geochemical anomalies associated with mineralization using an improved generative adversarial network. Ore Geol. Rev. 2021 , 136 , 104264. [ Google Scholar ] [ CrossRef ]
- Cheng, Q.M. Ideas and Methods for Mineral Resources Integrated Prediction in Covered Areas. Earth Sci. 2012 , 37 , 1109–1125. [ Google Scholar ]
- Xiong, Y.H.; Zuo, R.G. A positive and unlabeled learning algorithm for mineral prospectivity mapping. Comput. Geosci. 2021 , 147 , 104667. [ Google Scholar ] [ CrossRef ]
- Granek, J.; Haber, E. Data mining for real mining: A robust algorithm for prospectivity mapping with uncertainties. In Proceedings of the 2015 SIAM International Conference on Data Mining. Society for Industrial and Applied Mathematics, Vancouver, BC, Canada, 30 April–2 May 2015; pp. 145–153. [ Google Scholar ]
- Xiong, Y.H.; Zuo, R.G. Effects of misclassification costs on mapping mineral prospectivity. Ore Geol. Rev. 2017 , 82 , 1–9. [ Google Scholar ] [ CrossRef ]
- Zhao, P.; Chi, S.; Li, D.; Cao, X. Theory and Methods for Mineral Exploration , 6th ed.; China University of Geosciences Press: Wuhan, China, 2013. [ Google Scholar ]
- Pazzani, M.; Merz, C.; Murphy, P.; Ali, K.; Hume, T.; Brunk, C. Reducing misclassification costs. In Proceedings of the 17th International Conference on Machine Learning, San Francisco, CA, USA, 29 June–2 July 2000; Morgan Kaufmann Publishers Inc.: New Brunswick, NJ, USA, 2000. [ Google Scholar ]
- Chen, Y.; Wu, W. Mapping mineral prospectivity by using one-class support vector machine to identify multivariate geological anomalies from digital geological survey data. Aust. J. Earth Sci. 2017 , 64 , 639–651. [ Google Scholar ] [ CrossRef ]
- Goncalves, M.A.; Mateus, A.; Pinto, F.; Vieira, R. Using multifractal modelling, singularity mapping, and geochemical indexes for targeting buried mineralization: Application to the W-Sn Panasqueira ore-system, Portugal. J. Geochem. Explor. 2018 , 189 , 42–53. [ Google Scholar ] [ CrossRef ]
- Xiong, Y.H.; Zuo, R.G. Recognition of geochemical anomalies using a deep autoencoder network. Comput. Geosci. 2016 , 86 , 75–82. [ Google Scholar ] [ CrossRef ]
- Zhang, S.; Carranza, E.J.M.; Wei, H.T.; Xiao, K.Y.; Yang, F.; Xiang, J.; Zhang, S.H.; Xu, Y. Data-driven Mineral Prospectivity Mapping by Joint Application of Unsupervised Convolutional Auto-Encoder Network and Supervised Convolutional Neural Network. Nat. Resour. Res. 2021 , 30 , 1011–1031. [ Google Scholar ] [ CrossRef ]
- Zhang, Z.Q.; Wang, G.W.; Liu, C.; Cheng, L.Z.; Sha, D.M. Bagging-based positive-unlabeled learning algorithm with Bayesian hyperparameter optimization for three-dimensional mineral potential mapping. Comput. Geosci. 2021 , 154 , 104817. [ Google Scholar ] [ CrossRef ]
- Breslow, N.E.; Cain, K.C. Logistic regression for twostage case-control data. Biometrika 1988 , 75 , 11–20. [ Google Scholar ] [ CrossRef ]
- Schill, W.; Jockel, K.H.; Drescher, K.; Timm, J. Logistic analysis in case–control studies under validation sampling. Biometrika 1993 , 80 , 339–352. [ Google Scholar ] [ CrossRef ]
- King, G.; Zeng, L. Logistic regression in rare events data. Political Anal. 2001 , 9 , 137–163. [ Google Scholar ] [ CrossRef ]
- Li, T.F.; Xia, Q.L.; Zhao, M.Y.; Gui, Z.; Leng, S. Prospectivity Mapping for Tungsten Polymetallic Mineral Resources, Nanling Metallogenic Belt, South China: Use of Random Forest Algorithm from a Perspective of Data Imbalance. Nat. Resour. Res. 2020 , 29 , 203–227. [ Google Scholar ] [ CrossRef ]
- Nykanen, V.; Lahti, I.; Niiranen, T.; Korhonen, K. Receiver operating characteristics (ROC) as validation tool for prospectivity models—A magmatic Ni-Cu case study from the Central Lapland Greenstone Belt, Northern Finland. Ore Geol. Rev. 2015 , 71 , 853–860. [ Google Scholar ] [ CrossRef ]
- Kreuzer, O.P.; Etheridge, M.A.; Guj, P.; McMahon, M.E.; Holden, D.J. Linking mineral deposit models to quantitative risk analysis and decision-making in exploration. Econ. Geol. 2008 , 103 , 829–850. [ Google Scholar ] [ CrossRef ]
- Burkin, J.N.; Lindsay, M.D.; Occhipinti, S.A.; Holden, E.J. Incorporating conceptual and interpretation uncertainty to mineral prospectivity modelling. Geosci. Front. 2019 , 10 , 1383–1396. [ Google Scholar ] [ CrossRef ]
- Wang, Z.Y.; Yin, Z.; Caers, J.; Zuo, R.G. A Monte Carlo-based framework for risk-return analysis in mineral prospectivity mapping. Geosci. Front. 2020 , 11 , 2297–2308. [ Google Scholar ] [ CrossRef ]
- Zuo, R.G.; Kreuzer, O.P.; Wang, J.; Xiong, Y.H.; Zhang, Z.J.; Wang, Z.Y. Uncertainties in GIS-Based Mineral Prospectivity Mapping: Key Types, Potential Impacts and Possible Solutions. Nat. Resour. Res. 2021 , 30 , 3059–3079. [ Google Scholar ] [ CrossRef ]
- Chen, M.; Mao, J.; Uttley, P.J.; Norman, T.; Wu, L.; Zheng, J.; Qin, Y. Structure analysis and structural metallogenesis of Jinfeng (Lannigou) gold deposit in Guizhou province. Miner. Depos. 2007 , 26 , 380–396. [ Google Scholar ]
- Chen, M. The Genetic Model of Jinfeng (Lannigou) Gold Deposit Based on the Coupling of Metallotectonics and Oreforming Fluid. Ph.D. Thesis, Chinese Academy of Geological Sciences, Beijing, China, 2007. [ Google Scholar ]
- Su, H.; Wang, G. Tectonic ore-controlling and oreforming research of Lannigou gold deposit in SW Guizhou: Constraint from experiment simulations and scanning electron microscope. Glob. Geol. 2013 , 32 , 403–411. [ Google Scholar ]
- Wyborn, L.A.I.; Heinrich, C.A.; Jaques, A.L. Australian Proterozoic Mineral Systems—Essential Ingredients and Mappable Criteria. In 1994 Ausimm Annual Conference: Australian Mining Looks North—The Challenges and Choices: Technical Program Proceedings ; Australasian Institute of Mining and Metallurgy: Carlton, Australia, 1994; pp. 109–115. [ Google Scholar ]
- Zhai, Y.S. Research on Metallogenic System. Geol. Surv. Res. 2003 , 26 , 129–135. [ Google Scholar ]
- Hagemann, S.G.; Lisitsin, V.A.; Huston, D.L. Mineral system analysis: Quo vadis. Ore Geol. Rev. 2016 , 76 , 504–522. [ Google Scholar ] [ CrossRef ]
- Chawla, N.V.; Bowyer, K.W.; Hall, L.O.; Kegelmeyer, W.P. SMOTE: Synthetic minority over-sampling technique. J. Artif. Intell. Res. 2002 , 16 , 321–357. [ Google Scholar ] [ CrossRef ]
- Breiman, L. Random forests. Mach. Learn. 2001 , 45 , 5–32. [ Google Scholar ] [ CrossRef ]
- Breiman, L.; Friedman, J.H.; Olshen, R.A.; Stone, C.J. Classification and Regression Trees, the Wadsworth Statistics and Probability Series ; Wadsworth International Group: Belmont, CA, USA, 1984. [ Google Scholar ]
- Rodriguez-Galiano, V.F.; Chica-Olmo, M.; Chica-Rivas, M. Predictive modelling of gold potential with the integration of multisource information based on random forest: A case study on the Rodalquilar area, Southern Spain. Int. J. Geogr. Inf. Sci. 2014 , 28 , 1336–1354. [ Google Scholar ] [ CrossRef ]
- Carranza, E.J.M.; Laborte, A.G. Data-driven predictive mapping of gold prospectivity, Baguio district, Philippines: Application of Random Forests algorithm. Ore Geol. Rev. 2015 , 71 , 777–787. [ Google Scholar ] [ CrossRef ]
- Carranza, E.J.M.; Laborte, A.G. Data-Driven Predictive Modeling of Mineral Prospectivity Using Random Forests: A Case Study in Catanduanes Island (Philippines). Nat. Resour. Res. 2016 , 25 , 35–50. [ Google Scholar ] [ CrossRef ]
- Yen, S.J.; Lee, Y.S. Cluster-based under-sampling approaches for imbalanced data distributions. Expert Syst. Appl. 2009 , 36 , 5718–5727. [ Google Scholar ] [ CrossRef ]
- Gao, Y.; Zhang, Z.; Xiong, Y.; Zuo, R. Mapping mineral prospectivity for Cu polymetallic mineralization in southwest Fujian Province, China. Ore Geol. Rev. 2016 , 75 , 16–28. [ Google Scholar ] [ CrossRef ]
- Bharti, J.P.; Mishra, P.; Moorthy, U.; Sathishkumar, V.E.; Cho, Y.; Samui, P. Slope Stability Analysis Using Rf, Gbm, Cart, Bt and Xgboost. Geotech. Geol. Eng. 2021 , 39 , 3741–3752. [ Google Scholar ] [ CrossRef ]
- Yousefi, M.; Carranza, E.J.M. Prediction–area (P–A) plot and C–A fractal analysis to classify and evaluate evidential maps for mineral prospectivity modeling. Comput. Geosci. 2015 , 79 , 69–81. [ Google Scholar ] [ CrossRef ]
Click here to enlarge figure
Ore Controlling Factors | Description of Factors | 3D Models |
---|---|---|
Fault | The fault structures provide favorable pathways for the migration of ore-forming fluids. | Regional fault model and fault distance model. |
Strata | Ore-bearing strata exhibit distinct trends. | Stratigraphic model. |
Hydrothermal solution | Shallow, low-temperature hydrothermal deposits show coexistence and development of Au with As, Sb, and Hg. | Regional geochemical model. |
Gathering and protecting | Mineralization occurs in favorable combinations of lithology and intersectional faults. | Geological complexity model and resistivity geophysical model. |
Data | Number | Description |
---|---|---|
Geological map | 1 | 1:10,000-scale geological map; strike/dip measurements of surface structural elements. |
Drill holes | 635 | Depth varies from 75 m to 1266 m, with an average depth of 403 m, containing 11,339 stratigraphic data and nearly 300,000 geochemical data. |
Geological profiles | 40 | 1:1000-scale geological profiles at an average depth of 400 m. |
2D RT inversion maps | 16 | 1:5000-scale average line length of 3875 m, data effective depth of 4000 m, point spacing of 60 m. |
Surface geochemical sampling | 430 | Each point was tested for Au, As, and Hg taste data, and other chemical elements were tested using XRF. |
Stratum | Faults | Values |
---|---|---|
Bianyang and Xuman Formations | F3, F3N, F7, F70 | 0.75 |
Niluo Formation | F5, F7N, F14, F80 | 0.5 |
Luolou, Wujiaping, Dachang, and Maokou Formations | F1, F2, F4…… | 0.25 |
The statements, opinions and data contained in all publications are solely those of the individual author(s) and contributor(s) and not of MDPI and/or the editor(s). MDPI and/or the editor(s) disclaim responsibility for any injury to people or property resulting from any ideas, methods, instructions or products referred to in the content. |
Share and Cite
Peng, Q.; Wang, Z.; Wang, G.; Zhang, W.; Chen, Z.; Liu, X. 3D Mineral Prospectivity Mapping from 3D Geological Models Using Return–Risk Analysis and Machine Learning on Imbalance Data. Minerals 2023 , 13 , 1384. https://doi.org/10.3390/min13111384
Peng Q, Wang Z, Wang G, Zhang W, Chen Z, Liu X. 3D Mineral Prospectivity Mapping from 3D Geological Models Using Return–Risk Analysis and Machine Learning on Imbalance Data. Minerals . 2023; 13(11):1384. https://doi.org/10.3390/min13111384
Peng, Qingming, Zhongzheng Wang, Gongwen Wang, Wengao Zhang, Zhengle Chen, and Xiaoning Liu. 2023. "3D Mineral Prospectivity Mapping from 3D Geological Models Using Return–Risk Analysis and Machine Learning on Imbalance Data" Minerals 13, no. 11: 1384. https://doi.org/10.3390/min13111384
Article Metrics
Article access statistics, further information, mdpi initiatives, follow mdpi.
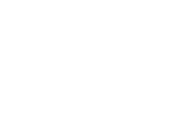
Subscribe to receive issue release notifications and newsletters from MDPI journals
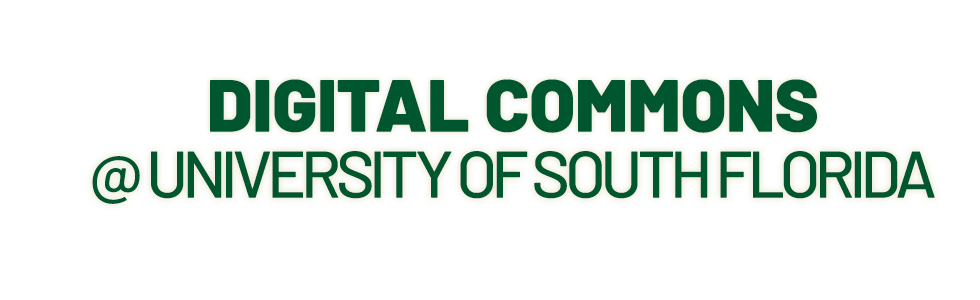
- USF Research
- USF Libraries
Digital Commons @ USF > College of Arts and Sciences > School of Geosciences > Geology > Theses and Dissertations
Geology Theses and Dissertations
Theses/dissertations from 2023 2023.
Understanding Controls on Spring Hydrographs in an Eogenetic Karst Aquifer in North-Central Florida , Ryan Almeqhem
Lithium and Lithium Isotope Behavior During High Temperature Solid/Fluid Exchanges: Examples from Iceland and the Izu-Bonin Forearc , Alex Maruszczak
Theses/Dissertations from 2022 2022
Functional morphology and taphonomy of Ordovician–Silurian diploporan echinoderms , Stephen Ashley Hill
Determining Depth of Closure Based on Time-series Beach Profiles and Empirical Formulas: A Case Study along the Florida Coast , Elizabeth Royer
Desertification Risk Analysis for Wildlife in the Desert Renewable Energy Conversation Plan Area of California , Serdar Sagdic
A Technique-Based Approach to Structure-from-Motion: Applications to Human-Coastal Environments , Robert Van Alphen
Theses/Dissertations from 2021 2021
Assessing Morphological Response and Vulnerability of Barrier Islands to Extreme Storms in Northwest Florida , Jacob Adam
Three-Dimensional Intrusion Geometries in the Monogenetic San Rafael (Utah) Sub-volcanic Field Revealed by Nonlinear Inversion of Magnetic Anomaly Data , Troy A. Berkey
Impacts of Experiential Learning on the Affective Domain: Gaining Insight into How to Broaden Participation in the Geosciences , Meghan Lindsey Cook
Resolution of Lava Tubes with Ground Penetrating Radar , Sanaz Esmaeili
Seismic Attenuation, Time Delays and Raypath Bending of Teleseisms Beneath Uturuncu Volcano, Bolivia , Alexandra K. Farrell
Geological and Geochemical Analysis of Phosphorus Bearing Minerals and Natural Glasses on Earth and in Meteorites: Implications of the Origin of Life , Tian Feng
Expansion-Contraction: Spatial and Temporal Variability in Connectivity in a Stream-Wetland Flow Network , Savannah Fransbergen
Revisiting Darwin’s Little Pond as a Method to Liberate Phosphorus from Apatite Under Prebiotic Hadean Earth Conditions , Jennifer Lago
Field and Remote Sensing Analysis of the 2015 Pyroclastic Density Currents at Colima (Mexico) and Calbuco (Chile) Volcanoes: Implications for Hazard Assessment and Crisis Management , Elodie Macorps
Investigating the Hydrology of the Western Greenland Ice Sheet: Spatiotemporal Variability and Implications on Ice-Dynamics , Jessica Z. Mejia
Shorebird Response to Human-Induced Changes at Three Pinellas County Beaches , Rebecca J. Ruthberg-Campagna
The Importance of Lithologic Variability and Stratigraphic Architecture in the Development of Eogenetic Karst Systems , Nicholas J. Soto-Kerans
Factors Controlling Longshore Variations of Beach Changes Induced by Tropical Storm Eta along Pinellas County Beaches, West-central Florida , Francesca Luisa Toledo Cossu
Factors Controlling Circulation at the Terminus of a Shallow Seagrass-Dominated Estuary, Fort DeSoto Bay, West-Central Florida , Charles Gray Vickery
Slow Slip Events and the Earthquake Cycle , Nicholas K. Voss
Investigation of Hurricane Irma Deposits, Big Pine Key, Florida , Youzhu Wang
Factors Controlling Longshore Variations of Beach Changes Induced by Hurricane Hermine along Pinellas County Beaches, West-central Florida , Wenhan Zhai
Theses/Dissertations from 2020 2020
Porosity and Permeability Extremes in an Eogenetic Carbonate Platform: Mechanisms for Formation and Implications for Fluid Flow , Charles I. Breithaupt
Using Geophysical and Geodetic Data to Improve Natural and Human-induced Hazard Assessments , Fanghui Deng
Using Geospatial Data to Predict the Locations of Groundwater Discharge to Salmon-Bearing Streams, Alaska , Mary Gerlach
Biogeochemical Cycling of Nutrients and Carbon in Subtropical Wetlands , Lauren N. Griffiths
Using Tidal Analysis to Examine Lake-Aquifer Connectivity on a Modern Carbonate Platform , Ronald A. Knoll
Shallow Water Seafloor Geodesy: GPS on An Anchored Spar Buoy , Surui Xie
The Effects of Surface-Water Flow on the Quality of Groundwater and Surface-Water Systems , Quanghee Yi
Theses/Dissertations from 2019 2019
Stable Isotope Geochemistry of Shelled Marine Invertebrates: Wide-Ranging Applications , Nasser M. Al-Qattan
Tracking Sediment Bypassing, Geomorphological Analysis, and Regional Sediment Management at Tidal Inlets , Tanya M. Beck
Habitat Suitability Index Model of the Florida Sandhill Crane ( Grus canadensis pratensis ) in West-Central Florida , Courtney E. Buck
Past hydroclimate and vegetation variation in Romania inferred from isotopic geochemistry and pollen of cave bat guano , Daniel Martin Cleary
Past Climate and Sea-level Reconstruction Using Cave Deposits from Mallorca, Spain , Oana-Alexandra Dumitru
Modeling and Assessing Lava Flow Hazards , Elisabeth Gallant
Full-waveform Inversion of Common-Offset Ground Penetrating Radar (GPR) data , Sajad Jazayeri
Fluid-mobile Trace Element Variability of Serpentinites and Entrained Crustal Rocks across the Mariana Forearc System , Raymond M. Johnston
Fewer Wetlands More Connectivity: Implications of Land use-Land cover Change in an Agricultural Landscape , Stephanie Lawlor
Assessing Paleo-Coastal Caves Evolution and Sea Level Changes in Mallorca: Results from Radiometric Dating of Speleothems , Giuseppe Lucia
On the Ablation of Meteors and the Implications on Organic Delivery to Earth , Christopher Alan Mehta
The Peculiar Nature of Florida’s Sandhill Wetlands, Ponds & Lakes— Their Ecohydrology, Relationship with the Regional Aquifer & Importance within the Landscape. , ReNae Starr Nowicki
Examining the Spatial and Temporal Variations in CO 2 Partial Pressure in the Deep Vadose Zone Above Jinapsan Cave, Guam , Jamar Regis
Framing Geologic Numeracy for the Purpose of Geoscience Education: The Geoscience Quantitative Preparation Survey , Victor J. Ricchezza
Timing and Rates of Events in the Generic Volcanic Earthquake Swarm Model , Tianyu Rong
Documenting Evolution: Comparing and Contrasting Late Mesozoic and Late Cenozoic Molluscan Patterns , Joshua Slattery
Volcanic Electrification: A Multiparametric Case Study of Sakurajima Volcano, Japan , Cassandra M. Smith
Modeled Affinity Constants for Phosphorus Adsorption and Desorption due to Saltwater Intrusion , Yasemin Taşcı
Sinkhole Lake Infiltration of Contaminants of Emerging Concern from Onsite Water Treatment Systems , Ethan A. Upton
Boron Variation and the Subducted Sediment Component in the Volcanics from the Bifurcated Portion of the Lesser Antilles Island Arc (LAIA) , Ami Margret Ngo Ward
Rethinking Map Literacy and an Analysis of Quantitative Map Literacy , Ming Xie
Theses/Dissertations from 2018 2018
Flow and Transport in Low-Gradient Rivers and Estuaries , Hunter Lee Clasen
Geochemical Modeling of Primary MORB Magmas: Implications for Parental Melting Regimes in Melt Lenses Along-Axis of the Hess Deep Rift , Stephanie Michelle Drumm
Theory and Application of Geophysical Geodesy for Studying Earth Surface Deformation , Makan A. Karegar
The Utility of the Conductivity Mass-Balance Method for Base Flow Separation in Rivers and Streams , Darline Alegria Lott
Evaluation of Academic and Social Engagement in a Technology-Based Collaborative Approach to Inclusive Geoscience Field Learning , Anita Marie Stone Marshall
Ice and Guano Deposits in El Malpais Lava Tubes: Potential Paleoclimate Archives for the Southwest United States , Dylan S. Parmenter
Quantifying the Effect of Topographic Slope on Lava Flow Thickness: A First Step to Improve Lava Flow Volume Estimation Methods , Steven R. Rizo
An evaluation of marsh shoreline erosion and sediment deposition in the Grand Bay National Estuarine Research Reserve, Mississippi, USA , Joseph Terrano
Assessing Bald Cypress (Taxodium distichum) Tree Dynamic Change in USF Forest Preserve Area Using Mixture-Tuned Matched Filtering and Multitemporal Satellite Imagery , Yujia Wang
Morphodynamics of Shell Key and Mullet Key Barrier Islands: Their Origin and Development , Zachary J. Westfall
Comparison of Beach Changes Induced by Two Hurricanes along the Coast of West-Central Florida , Ruoshu Zhao
Theses/Dissertations from 2017 2017
Imaging Wetland Hydrogeophysics: Applications of Critical Zone Hydrogeophysics to Better Understand Hydrogeologic Conditions in Coastal and Inland Wetlands and Waters , Christine Marie Downs
Morphodynamics and Sediment Pathways of the John's Pass-Blind Pass Dual-Inlet System: Pinellas County, Florida , Mark H. Horwitz
3D Cave and Ice Block Morphology from Integrated Geophysical Methods: A Case Study at Scărişoara Ice Cave, Romania , Jackson Durain Hubbard
A Geochemical and Petrological Analysis of the San Rafael Volcanic Field, Utah , Danielle Koebli
Characterizing the Evolution of Slab Inputs in the Earliest Stages of Subduction: Preliminary Evidence from the Fluid-Mobile Element (B, Cs, As, Li) Systematics of Izu-Bonin Boninitic Glasses Recovered During IODP Expedition 352 , Keir Aavon Sanatan
Theses/Dissertations from 2016 2016
Lava Flow Hazard Assessment for the Idaho National Laboratory, Idaho Falls, and Pocatello, Idaho, U.S.A. , Elisabeth Gallant
The Geophysical Kitchen Sink Approach to Improving our Understanding of Volcano-Tectonic Interactions , Ophelia Ann George
A Molluscan Record of Monsoonal Precipitation along the Western Shoreline of the Late Maastrichtian Western Interior Seaway , Scott Allen Ishler
Lilliput Effect Dynamics across the Cretaceous-Paleogene Mass Extinction: Approaches, Prevalence, and Mechanisms , Matthew Brett Jarrett
Improved 2D and 3D resistivity surveys using buried electrodes and optimized arrays: The multi-electrode resistivity implant technique (MERIT) , Henok Gidey Kiflu
Alumni Narratives on Computational Geology (Spring 1997 – Fall 2013) , Victor J. Ricchezza
Modeling the Construction and Evolution of Distributed Volcanic Fields on Earth and Mars , Jacob Armstrong Richardson
Morphodynamics of Egmont Key at the Mouth of Tampa Bay: West-Central Florida , Zachary James Tyler
Optimizing Methods for Extraction of Organic Compounds from Molluscan Shells , Kaydee Jo West
A New Volcanic Event Recurrence Rate Model and Code For Estimating Uncertainty in Recurrence Rate and Volume Flux Through Time With Selected Examples , James Adams Wilson
Applications of Satellite Geodesy in Environmental and Climate Change , Qian Yang
Storm Induced Beach Profile Changes along the Coast of Treasure Island, West-Central Florida, U.S.A. , Zhaoxu Zhu
Theses/Dissertations from 2015 2015
Reconstructing Paleoenvironments of the Plio-Pleistocene Tamiami Formation of Florida with Benthic Foraminifera , Heather Bender
Communication of Lava Flow Hazards at the San Francisco Volcanic Field, Flagstaff, Arizona , Catherine St. John Carter
Multiple Scales of Beach Morphodynamic Processes: Measurements and Modelling , Jun Cheng
A Bat-Guano-Derived δ 15 N and δ 13 C Record of Paleoenvironmental Change: Zidită Cave, Romania , Daniel Martin Cleary
The Speleogenesis of Vallgornera Cave (Mallorca, Spain): a Mineralogical and Morphological Study , Jacqueline Amelia Diehl
Phosphorus Sorption Dynamics in Shallow Groundwater, Coastal Everglades, Florida, USA , Hilary Flower
Investigation of the Mechanisms for Mobilization of Arsenic in Two ASR Systems in Southwest Central Florida , Gregg William Jones
Schreibersite: Synthesis, Characterization and Corrosion and Possible Implications for Origin of Life , Nikita Latesha La Cruz
Is Nubia Plate Rigid? A Geodetic Study of the Relative Motion of Different Cratonic Areas within Africa. , Mary Wambui Njoroge
Modeling Intrusive Geometries of a Shallow Crustal Intrusion: New Evidence From Mount Ellsworth, Utah , Nathan Nushart
Morphodynamics of Mullet Key, West-Central Florida , Emeli Sandoval
Ground-Coupled Air Waves: A Seismological Case Study of the Explosion Quakes of the 2007 Eruption of Pavlof Volcano, Alaska , Cassandra Marie Smith
Geophysical Evidence for Mid-crustal Magma Reservoirs in the Lassen Volcanic Region, California , Samantha Catherine Tavarez
Glaciological Applications of Terrestrial Radar Interferometry , Denis Voytenko
Theses/Dissertations from 2014 2014
Characterization of the Depositional Environment of Phreatic Overgrowths on Speleothems in the Littoral Caves of Mallorca (Spain): a Physical, Geochemical, and Stable Isotopic Study , Liana Marie Boop
Evolution and Equilibration of Artificial Morphologic Perturbations in the Form of Nearshore Berm Nourishments Along the Florida Gulf Coast , Katherine Emily Brutsché
Groundwater Controls on Physical and Chemical Processes in Streamside Wetlands and Headwater Streams in the Kenai Peninsula, Alaska , Michael Kroh Callahan
Interpolating Beach Profile Data Using Linear and Non-linear Functions , Lance Calloway Croft
Probabilistic Modeling of Lava Flows: A Hazard Assessment for the San Francisco Volcanic Field, Arizona , Aleeza Harburger
Response of Benthic Foraminifera to Ocean Acidification and Impact on Florida's Carbonate Sediment Production , Paul O. Knorr
Advanced Search
- Email Notifications and RSS
- All Collections
- USF Faculty Publications
- Open Access Journals
- Conferences and Events
- Theses and Dissertations
- Textbooks Collection
Useful Links
- Geology Home
- Rights Information
- SelectedWorks
- Submit Research
Home | About | Help | My Account | Accessibility Statement | Language and Diversity Statements
Privacy Copyright
Science – Society – Technology
- Open access
- Published: 09 August 2017
Development of an updated geothermal reservoir conceptual model for NW Sabalan geothermal field, Iran
- Mirmahdi Seyedrahimi-Niaraq 1 , 2 ,
- Faramarz Doulati Ardejani 1 , 2 ,
- Younes Noorollahi 3 &
- Soheil Porkhial 4
Geothermal Energy volume 5 , Article number: 14 ( 2017 ) Cite this article
5867 Accesses
14 Citations
7 Altmetric
Metrics details
In this paper, a conceptual model has been developed for NW Sabalan geothermal field using exploration indicators. These indicators include the data and results of subsurface and surface investigations comprising geology, geophysics , hydro-geochemistry, hydrology and temperature and pressure distribution. The subsurface information was obtained from 10 deep exploration wells as well as from the results of previous studies in this field. All available data together with the stratigraphy and 1:20,000 geological map covering the study area were combined to produce a two-dimensional geological cross section. Also, a subsurface three-dimensional geological model was developed using available drilling logs. NW Sabalan geothermal field is one of the 18 detected potential areas in Iran that is located in Northwest of the country. This field includes a deep geothermal reservoir with a temperature range of 230–242 °C. The reservoir is covered by a cap rock with an approximate thickness of 500 m. There are four major geological units identified in the study area including Quaternary alluvium, fan and terrace deposits; Pleistocene post-caldera trachyandesitic Flows; Pleistocene syn-caldera trachydacitic to trachyandesitic domes and Pliocene pre-caldera trachyandesitic lavas, tuffs and pyroclastic. A hydraulic conductivity zone has been assessed by magnetotelluric surveys at deeper zones, suggesting that the main outflow direction is towards west and north of the area. The fluid chemistry is consistent with high chloride, neutral pH and immature liquids that partially equilibrated with host rock and they are classified as mixed water. The results of exploration and geological studies during a 10-year period have been integrated together to build a new overall conceptual model of the field.
A conceptual model provides a basis for a numerical model. The data available on geothermal systems are unified through the development of an appropriate conceptual model of the geothermal system of interest. They play a key role in all phases of geothermal exploration and development. Conceptual models are mainly based on surface and subsurface geological information, remote sensing data, results of geophysical surveys, the chemical and isotopic datasets of fluids in surface manifestations and reservoir fluid samples collected from wells, information on temperature and pressure conditions based on analysis of the available well logging data as well as other reservoir engineering information. A realistic conceptual model allows an explicit description of the main properties of a geothermal system such as hydraulic characteristics, groundwater flow, solute and heat transfer. A realistic and accurate conceptual model is probably not possible without a numerical model to prove its accuracy.
Northwest Sabalan (NW) geothermal field is one of the eighteen fields of identified potential and the fourth main high temperature areas in Iran that is situated in the northwest of Iran in Ardabil Province. Its distance from Tehran is 859 km (Fig. 1 a) (Noorollahi et al. 2009 ; Porkhial et al. 2015 ; Kosari and Sattari 2015 ). This field has practically been exploring and developing recently. The area has been under geological investigation since 1978 (Fotouhi 1995 ).
a Geographical situation of study area, b geological map of west and northwest of Mt. Sabalan (based on KML 1999a , detailed faults map is based on EDC 2010 )—geothermal wells and faults are shown in bold and bold italic , respectively. The letters A , B , C , D and E on geological map represent exploration well pads
The plan of electricity generation from NW Sabalan geothermal field has been presented since 1994. Afterwards emphasis has been put on this field as a top priority area. From 1998 to 2005, the detailed geo-based study was directed by the joint cooperation of renewable energy organization of Iran (SUNA) and Sinclair Knight Merz Ltd (SKM) of New Zealand. The NW Sabalan geothermal field was finally distinguished as an important potential for power generation purpose (Noorollahi et al. 2009 ).
Accordingly, during the time period of 2002–2004, three deep exploration and delineation wells (NWS-1, NWS-3 and NWS-4) have been drilled. Besides, a shallow injection well named NWS-2 (Fig. 1 b, PADs A, B and C) was drilled, in order to evaluate the subsurface geology and provide data for assessment and modelling the reservoir (Najafi and Ghobadian 2011 ; Porkhial et al. 2015 ). The location of these wells was determined based on the results obtained from a Magnetotelluric (MT) survey conducted in 1998 (SKM 2005a ).
The wells NWS-1 and NWS-4 were the first deep wells drilled in Sabalan volcanic area (Porkhial et al. 2015 ; Kosari and Sattari 2015 ). A maximum temperature of 242 °C was measured in well NWS-1 at a minimum depth of 832 m. Subsequently, from 2008 to 2011, SUNA performed new geophysical exploration studies. Based on the new results, the wells NWS-5 ( T max = 240 °C, D min = 1635 m) and NWS-11 ( T max = 174.5 °C, D min = 2701 m) on Pad A and C, respectively, NWS-6 ( T max = 238 °C, D min = 1144 m), NWS-7 ( T max = 237 °C, D min = 1341 m) and NWS-10 (maximum temperature 242 °C, D min = 1890 m) on Pad D and NWS-8 and NWS-9 (maximum temperature 230.5 °C at a minimum depth of 2301 m), respectively, on Pad E were drilled (Fig. 1 b).
A few studies have been carried out in the past to present a conceptual model for the NW Sabalan geothermal field. The conceptual/geological models presented by KML ( 1999b ), Noorollahi and Itoi ( 2011a , b ), Porkhial et al. ( 2010a , b ), Khojamli ( 2012 ) and Sabzemeidani ( 2016 ) are all noteworthy.
KML ( 1999a ) built a hydrogeological geothermal model for the Mt. Sabalan volcanic complex based on multi-disciplinary combination of geological, geochemical and geophysical data. The heat source, exploration wells, meteoric waters, deeply circulated meteoric waters, magmatic volatiles and condensates are illustrated. Based on this model, one may explain that a large residual magma mass is shown to underlie the Sabalan caldera complex with intrusive apophyses developing upwards from the magma mass to shallow depths controlled by caldera ring-faults. KML ( 1999a ) further explained that the thermal features might all have a common origin within the Sabalan caldera.
Noorollahi and Itoi ( 2011a , b ) have presented a conceptual model from the available three deep exploration wells data as a basis for their reservoir numerical model. The simulation predicted a power plant equivalent to be designed with a capacity of 50 MWe and an age of more than 30 years. Now construction of the first pilot plant is running with a capacity of 5 MWe, the produced fluid temperature of 86 °C and maximum flow rate of 58 l/s (average flow rate: 46 l/s) in the NW Sabalan Site. This plant is located between Pad A and Pad D with geographical coordinates x = 739,108, y = 4,238,580 and elevation of 2635 masl (Fig. 1 b).
Literature review indicates that despite several researches related to the development of conceptual models for NW Sabalan geothermal field, a comprehensive conceptual model based on the combination of geological, geophysical, hydrogeochemical, hydrological and deep well data has not been yet presented to provide a basis for developing a numerical model for the study geothermal reservoir. The weakness of these works (KML 1999a , b ; Porkhial et al. 2010a , b ; Noorollahi and Itoi 2011a , b ; Khojamli 2012 ; Sabzemeidani 2016 ) is that they only employed the information obtained from three exploration wells for the development of the conceptual model. Besides, they ignored some of important exploration indicators to be considered. Therefore, these models cannot fully explain the processes of Sabalan geothermal system, such as conduction and convection zones. In addition, they presented an incomplete explanation about the fluid flow cycle. Moreover, they did not define an exact boundary for the geothermal reservoir due to the lack of adequate data. In the present work, attention has been focused on the development of a conceptual model which takes all geothermal system variables into account and provides a visual representation of the geothermal system. The structure of the conceptual model presented here was completed by the use of geological and thermal data obtained from the new deep exploration wells. Conduction and convection zones and the top of the reservoir were added to this model by the incorporation of the geophysical results together with subsurface temperature distribution. To derive the conceptual model, Datamine Studio3 Version 3.21.7164 and ArcGIS Desktop software were used as tools.
NW Sabalan geothermal field is located in the northwest part of Mt. Sabalan. A geological map at a scale of 1:20,000 of Sabalan region is shown in Fig. 1 b. Mt. Sabalan is a large stratovolcano comprising a wide-ranging central structure which is constructed on a likely tectonic horst of underlying intrusive and effusive volcanic rocks. According to KML ( 1999a ), the output magma had a great role in forming a collapsed caldera about 12 km in diameter and a depression of about 400 m.
The Mt. Sabalan region sits on the south Caspian plate, which underthrusts the Eurasian Plate to the north and it is in turn underthrust by the Iranian plate, which causes compression in a NW direction. Besides, a dextral rotational motion resulted by the movement of Arabian plate beneath the Iranian plate makes the geological structures in the region more complicated. As a result of this tectonic structure, the Cenozoic geological history and stratigraphy of the region under consideration are complicated and the geological units in this region might have different structural characteristics (Mckenzie 1972 ; Amidi 1978 ; TBCE 1979 ; ENEL 1983 ; Nejad 1987 ; Manouchehri 1989 ; Emami 1994 ; Noorollahi et al. 2008 ).
The lava flows are typically trachyandesite and trachydacite with alternating explosive phases (KML 1999b ). There are four major geological units identified in the NW Sabalan area that include (1) quaternary alluvium, fan and terrace deposits; (2) pleistocene post-caldera trachyandesitic flows; (3) pleistocene syn-caldera trachydacitic to trachyandesitic domes and (4) pliocene pre-caldera trachyandesitic lavas, tuffs and pyroclastic (SKM 2005a , b ).
Two major geological structures have been identified in the study area that comprise a set of linear faults and several ring-faults (Fig. 1 b) (KML 1999a ; SKM 2005a , b ; Noorollahi et al. 2008 ; Ghaedrahmati et al. 2013 ). The results of interpretation of Centre National d’Etudes Spatiales (SPOT) satellite images and aerial photographs showed a WNW trending structural zone passing through the Moeil Valley. The faults strike mostly towards the NW and NE (KML 1999a ).
Stratigraphy
By increasing data due to adding new exploration wells and constructing Pads D and E, the realisation of the deep volcanic succession in the NW Sabalan geothermal field became easier. These data have been used in this paper to understand the stratigraphy in details. It is noted that the deepest geothermal wells in NW Sabalan are NWS-1 and NWS-3 with approximate depths of 3177 and 3197, respectively.
A three-dimensional geological model (Fig. 2 ) was generated using both the available wellbore and surface geological data. Datamine Studio3 Version 3.21.764 software was used as a tool. Figure 3 shows a two-dimensional cross section including surface and subsurface geological data. The geological map (Fig. 1 b), wellbore data and the three-dimensional model (Fig. 2 ) were employed to build this section.
Subsurface three-dimensional geological model (by Datamine software). Dip diorite Porphyri, Diz dizu formation, Epa Epa formation, Hfs hornfels, Mon monzonite, Nga Ngab formation, Pla plat formation, Val valhazir formation). a All geological units, b indicator geological units, c transparent model
NW-SE geological cross section of the NW Sabalan site with the exploration wells and stratigraphy
Monzonite basement rock was revealed at the depth of 1021 m of NWS-1 well in southeast. This rock was further approved at the depth of 2140 m of NWS-6. However, it appeared as the monzonite dykes at the depth zone of 1978–2700 m of NWS-9 well. The outcrop of monzonite extends as a bound, 5–10 km wide, for 100 km with a general NW strike. Mineralogical study of this rock showed minerals comprising plagioclase, orthoclase and slight amounts of quartz, biotite and minor hornblendes. Also, the wells NWS-4 and NWS-5 reached to Diorite at an approximate depth of 1840 m. This rock type appeared as old and young diorite intrusive in NWS-1.
Epa Formation
The thickness of Epa formation varies from a few hundred metres to 2200 m in the study area and it includes trachyandesite, trachybasalt, andesite, altered andesite and lahar. Name of this unit has been taken from the local geological map at a scale of 1:100,000 (Emami 1994 ). This formation has a separate weathering horizon at its upper surface. This unit identifies an unconformity with the overlying Valhazir formation (Fig. 3 ) (SKM 2005c ).
Valhazir formation
This formation has been introduced by Bogie et al. in ( 2000 ). Its thickness varies between 300 and 2000 m with a variable alteration zone (from 420 to 970 m). This formation includes Pliocence pre-caldera trachy-andesitic lava flows, tuffs and pyroclastic breccia as the oldest volcanic rocks identified in the study area. According to Bogie et al. ( 2000 ) these volcanic units are more fractured and affected by faulting than the younger units.
The subsurface studies identify that pyroclastics is dominant in the upper parts of the unit with lavas in lower portions (SKM 2005a , b ).
The Valhazir formation is equivalent with Qpad of the geological map of Meshginshahr (Emami 1994 ). Samples of Valhazir formation taken through the exploration well is totally altered to clay, silica and pyrite with slight amounts of chlorite and Fe-oxides. This means that the initial lithology of Valhazir is not clear, hence a term of altered volcanic is used for this formation (Eshaghpour 2005 ).
Coarser-grained pyroclastics comprise lapilli tuff and tuff breccia. However, their fine cutting size cannot be easily recognised. Lithic clasts are dominant and include a variety of andesites with either biotite or hornblende as the main mafic minerals. The andesite at the base of the formation includes euhedral phenocrysts of plagioclase and hornblende in a dark and very fine-grained matrix (SKM 2005a , b ).
Plat and Ngab formation
These units have been named by Emami ( 1994 ) and were identified in NWS-3 well, only. The main indication for the presence of these units in NWS-3 is the existence of olivine phenocrysts in the altered rocks with trachybasalts. Trachybasalts have been first reported in the stratigraphic sequence in the Ngab unit, which is indicated in the cross section of geological map presented by Emami ( 1994 ). The Ngab unit is underlined by the Plat unit (Fig. 3 ). Plat formation includes Pliocene Lahar, Crystalitic Tuffs and Andesitic Breccia with a thickness of 114 m (SKM 2005c ).
Dizu formation
The Dizu formation has been identified to be the upper stratigraphic sequence at NW Sabalan geothermal field (Bogie et al. 2000 ). It is equivalent to the Q t1 and Q t2 units (Amini 1988 ).
This formation comprises terrace deposits displaying a mixture of poorly sorted sand, granules, pebbles, cobbles and boulders with some sand intercalations. The terrace deposits show a blend of fluviatile processes, mainly during flash floods and by mass flow from the surrounding slopes (SKM 2005a ; Mousavi and Darvishzadeh 2010 ).
The rounded clasts of andesite, trachyandesite and subordinate trachydacite are dominant in this formation. The andesite includes phenocrysts of plagioclase and hornblende in a grey matrix. The trachyandesite also comprises plagioclase and hornblende phenocrysts, but further includes K-feldspar phenocrysts showing a brown matrix. The trachydacite consists of coarse phenocrysts of sanidine along with plagioclase and hornblende and displays a light grey vesicular matrix. The coarser grains are very poorly sorted and clasts range from granule to boulder size, with a sand matrix. They are uncemented and show no indication of hydrothermal alteration. However, the rare clasts of chalcedonic quartz are the erosional products of the hydrothermally altered sequences of the Valhazir formation (SKM 2005a , b ).
Geophysical surveys including Direct Current (DC) resistivity, Transient Electromagnetic (TEM) and MagnetoTelluric (MT) were conducted in the Mt. Sabalan region by the Renewable Energy Organization of Iran (SUNA) in 1998. The surveys were carried out at 212 resistivity stations over an area of about 860 km 2 . 110 of these stations were located in NW of Mt. Sabalan. These surveys resulted in five anomalies that the largest one is located at Moeil Valley (Fig. 1 b) (KML 1999a , b ; Bromley et al. 2000 ; SKM 2005a ; Noorollahi et al. 2008 ; Ghaedrahmati et al. 2013 ).
The resistivity of geological layers at shallow depths was provided by DC resistivity data, and the magnetotelluric soundings with a spacing of 50 m were employed to provide resistivity data for deeper layers. In order to provide resistivity data for those layers at a depth zone of 100–300 m and for the treatment of “static-shifts effects”, the TEM surveys have been conducted. The natural-source MT method with a frequency range of 8 kHz–0.02 Hz was employed to estimate the exploratory drilling depth. The MT method identified an area in the Moeil valley (NW Mt. Sabalan) with a low resistivity anomaly which is accessible at a depth range of 300–3000 m (Bromley et al. 2000 ; Noorollahi et al. 2008 ). Three deep exploratory wells (namely NWS-1, NWS-3 and NWS-4) were consequently drilled between 2002 and 2004 (at Pads A, B and C, Fig. 1 b). Their locations were chosen based on a 4 Ωm low resistivity anomaly zone. However, this drilling program was not successful to identify the exact location of the upflow zone (SKM 2005a ).
Subsequent interpretation of good quality MT data by SKM ( 2003 ) and Talebi et al. ( 2005 ) revealed a new location for outflow zone near the well NWS-3. The anomalous zone with low resistivity extended to southeast of NWS-1 (Pad D, E, Fig. 1 b) that suggests a location for likely upflow zone. They recommended further investigation of the upflow zone location applying low-frequency magnetotelluric data and further drilling operation in this location.
A new MT study incorporating 78 deep soundings with a frequency range of 385–0.0005 Hz was conducted by EDC (EDC 2008 ) in 2007, to determine the location of likely reservoir and possible drilling targets for the development of a geothermal power plant. The results showed a shallow resistivity anomaly as the likely centre of geothermal system on the east of Pad E and west of the young trachyandesite domes of post-caldera volcanic formation. Due to inadequate coverage of MT stations, especially, at the east of the study area, another MT survey was designed and conducted in 2009 for more identification of the geothermal system (EDC 2010 ). Based on the joint interpretation of new MT sections and two-dimensional geological models (EDC 2010 ), a final analysis was performed between years 2007 and 2009 to confirm the presence of a hotter zone in the east side of Pad D.
Ghaedrahmati et al. ( 2013 ) developed a three-dimensional invention code using a long-period MT data to obtain a realistic resistivity model. The resistivity slices of the different horizons from their model are shown in Fig. 4 . A low resistivity zone [zone (1), on the resistivity slice map related to the depths below 300 m] has extended from northwest to the centre of the area. This zone is almost parallel to the general structural trend and faults of the study area. This zone has been identified as an alteration zone due to the upward thermal flows along the faults and permeable structures. These structures have been delineated from wells NWS-1 and NWS-4 and further validated by the presence of thermal springs (Fig. 1 b).
The resistivity slices at different depths (modified from Ghaedrahmati et al. 2013 ), the black dots on maps show MT stations and the labels 1 , 2 , 3 , 4 , 5 and 6 display conductive (anomaly) zones related to geothermal resource obtained from 3-D modelling results. New location of the hotter zone (as an ellipse ) and its previously identified location by EDC ( 2010 ) (as a circle ) are also illustrated on the 4600 m depth slice map
The resistivity cross section given by EDC ( 2010 ) with a direction almost similar to that of geological section (Fig. 1 b) was considerably modified and is shown in Fig. 5 . The indicators of alteration zone identified in wells of Pad D and Pad E are also illustrated in this figure. Joint interpretation of Figs. 4 and 5 reveals that the geothermal resource is associated with the hydraulic conductivity zones (3) and (4) at an approximate depth of 4600 m. This deep conductive zone could be related to fluid flow within the zones consisting of high fracture density.
Resistivity cross section modified from EDC ( 2010 ) incorporating wells data and alteration indicators. Sm smectite, Il ilite, Ser sericite, Ep epidote, Me metamorphic
Also, two additional hydraulic conductivity zones can be highlighted in southern and south-eastern area in the 1000 m depth resistivity slice map [zones (5) and (6) in Fig. 4 , first row, right figure]. The results of 3-D modelling approximated a depth more than 1500 m for these two conductive zones (see Fig. 4 , second row, left figure). These zones are probably altered due to interaction of thermal fluids and country rocks along the main fractured zones and faults (EDC 2008 , 2010 ; Ghaedrahmati et al. 2013 ).
Hydraulic conductivity zone is one of the main indicators of hydrothermal activity in Mt. Sabalan area with resistivity values less than 20 Ωm which have been identified at elevation zones varying from 1200–2800 masl. The presence of hydraulic conductivity zones at deeper horizons suggests that the main outflow directions in Mt. Sabalan area are towards the west and north. These conductive zones have about 600–1000 m thickness below Pad D (Fig. 5 ).
In addition, the data obtained from well NWS-7 show that smectite and illite–smectite units are coincident with the hydraulic conductivity zones and also epidote unit is coincident with the increase in resistivity values (>30 Ωm). From the well NWS-8 data, it is obvious that smectite, chlorite and illite units have resistivity values less than 30 Ωm, whereas illite and smectite units are coincident with the resistivity contour lines above 30 Ωm (modified from Ghaedrahmati et al. 2013 ).
- Geochemistry
The surface geochemistry of Mt. Sabalan area was first time interpreted by Stefansson ( 1989 ) and further reported by Fotouhi ( 1995 ). Subsequently, the geochemistry of hot springs has been studied by Khosrawi ( 1996 ). Similar investigations have been carried out by Stelbitskaya and Radmehr ( 2010 ) and Kosari ( 2011 ).
The natural thermal features at NW Sabalan have been discovered as hot springs, most of which are located on the outskirts of the Moeil valley with a temperature range of between 25 and 85 °C. This area is characterised with the most thermal features in Mt. Sabalan (Fig. 1 b).
The spring waters are acid SO 4 , acid Cl–SO 4 and natural Cl–SO 4 types (Table 1 ). Hydrothermal alteration can be seen at the land surface. The main hydrothermal alteration is associated with the Valhazir formation (Rahmani 2007 ).
Springs chemistry
All thermal features in NW Sabalan arise mainly from the gravels of the Dizu formation (Fig. 1 b). There is no spring reported in downstream areas at lower elevations. Their water is characterised with a simple dilution trend indicating mixing with varying amounts of shallow groundwater (Table 1 ). They show a strong seasonal cyclic variation in flow rate; however, very slight seasonal deviation in temperature or chemistry can be seen. Despite the elevated Cl concentration, isotopic data categorised the waters to lie on the local meteoric water line. Mg concentrations exhibit a weak inverse correlation with Cl. This may suggest that geothermal fluids containing elevated Cl concentrations are diluting with the shallow groundwater aquifer consisting of high Mg concentrations.
Assuming the fact that all of the springs are not boiling, quartz geothermometer without stream loss (Fournier 1977 ; Fournier and Potter 1982 ) may be acceptable and a temperature range of 80–156 °C was calculated using this geothermometer. The Na–K geothermometer resulted in temperatures greater than 250 °C. However, elevated Ca and Mg concentrations reject application of this geothermometer. The Na–K–Ca geothermometer calculated a temperature range of 75.5–213 °C. Accordingly, recent temperature estimation applying various geothermometers by the authors is given in Table 2 . As well shown, the reservoir temperature at NW Sabalan varies between 88 and 244 °C.
According to the chemistry of the springs water and the different temperature ranges estimated by various geothermometers and also comparison them with the measured temperature of the reservoir (242 °C, in well NWS-10), it seems that the agreement between the calculated and measured reservoir temperature is somewhat close.
Reservoir chemistry
Although not given, several samples were collected from well NWS-1 in May and June 2004 and from well NWS-4 in September 2004. The samples were collected by SUNA. The samples were both untreated and acidified water. Chemical analyses of pH, Cl, HCO 3 , SO 4 , Ca, B and CO 2 were carried out in the site laboratory of SUNA and for Li, Na, K, Ca, Mg and SiO 2 in laboratory of GNS Wairakei (New Zealand). Stable isotope analysis (δ 18 O and δ 2 H) was conducted in the laboratory of GNS Wellington (Stelbitskaya and Radmehr 2010 ).
In the second stage of development and reservoir assessment, the discharged fluids from four wells, including NWS-6D, NWS-7D, NWS-9D and NWS-10D were analysed. The water samples have been classified as high chloride, neutral pH and mature liquids showing partial equilibrium with host rock. The analytical data of liquid samples comprise Ca, Na, K, Mg, Li, Fe, Mg, Mn, B, Cl, F, SiO 2 , SO 4 , CO 2 , H 2 S, HCO 3 and NH 3 concentrations in the liquid phase of Webre and Weir box samples. The steam samples were analysed for all gas components containing CO 2 , H 2 S, He, H 2 , Ar, O 2 , N 2 , CH 4 and NH 3 (Kosari and Sattari 2015 ).
Liquid chemistry
Based on SKM reports on production wells NWS-1 (SKM 2005a ) and NWS-4 (SKM 2005b ) and further interpretation by Rahmani ( 2007 ), the discharging fluid chemistry of wells NWS-1 and NWS-4 was classified as an alkaline-Ph, medium salinity and sodium chloride water. The chemistry of discharging fluid was almost identical for most of the chemical concentrations except Ca, which slightly varies from well NWS-4 (25–30 ppm) to well NWS-1 (15–30 ppm). In well NWS-1, Ca concentration decreased compared with Cl over the first week of discharging. Kosari ( 2011 ) suggested that the initial water discharged from the wells may have originated from deeper and different inflow zones with relatively different compositions apparently with higher Ca concentration.
The calculated calcite saturation of deep liquid phase in the aquifer water through wells NWS-1, NWS-4, NWS-6, NWS-7, NWS-9 and NWS-10 (Fig. 6 a, b) revealed that the deep liquid is generally super-saturated at lower temperatures than reference temperatures and under- saturated at temperatures more than reference temperatures (see reference temperatures in Table 3 ) (SKM 2004a ; Kosari and Sattari 2015 ).
Calcite saturation states of the NW Sabalan reservoir fluid ( a SKM 2004a , b Kosari 2015 )
Figure 7 shows Na–K–Mg ternary diagram (Giggenbach 1991 ) related to the springs of NW Sabalan. It is well indicated that most of the samples are plotted in the partial equilibration and mixed waters domains. Applying two geothermometers including K–Mg and Na–K (Giggenbach 1988 ) on this geo-indicator reveals that the deep fluid temperatures range is from 235 to 320 °C and between 285 and 335 °C, respectively. Table 3 shows the reservoir temperatures calculated by several geothermometers and compared with the reference temperatures (Strelbitskaya and Radmehr 2010 ; Kosari and Sattari 2015 ). This table further indicates that there is a close agreement between temperatures calculated by quartz geothermometers and the reference temperatures.
Na–K–Mg ternary diagram and geo-indicator representing equilibrium state of discharging waters from NW Sabalan wells (Kosari and Sattari 2015 )
The chemistry of discharging stream from the wells indicated that about 98% of the total gas content is CO 2 , while H 2 S, N 2 , Ar, H 2 and CH 4 form the remaining steam components (Kosari 2011 ). The temperature calculated by gas geothermometers, particularly H 2 S geothermometer changes from 269.1 to 277.1 °C (with an average of 274.2 °C), is fairly well correlated with that of Na–K geothermometer (279 °C). However, CO 2 geothermometer estimated temperatures ranging from 287.3 to 308.9 °C (having an average of 301.8 °C) which is higher than those calculated applying all the other gas and solute thermometers (Table 3 ). The elevated CO 2 concentration in deep liquid is the main reason for this overestimation (Kosari and Sattari 2015 ).
In NW Sabalan area, the magmatic intrusions are permeable due to the abundance of fractures, typically in the upper parts. Field investigations have shown that the volcanics of the Oligo-Quaternary succession made up mainly of lava at shallow depths are also permeable. Pyroclastic deposits and altered zones have medium-to-low permeability (Khosrawi 1996 ). The permeability of these rock units eases the movement of recharging water to the subsurface. However, the hydrogeology of the NW Sabalan is mostly controlled by faults.
The average annual atmospheric temperature is about 8 °C while in higher elevations, it decreases to 0 °C. The average temperature is very low from December to February and it reaches to 0 °C or even below (−15 °C in January). The maximum value of average monthly temperatures is obtained in May and April. The average monthly precipitation has been recorded in synoptic stations between 1996 and 2015. The precipitation occurs mainly in the form of snow in the winter. Based on available data, the maximum average monthly precipitation is 72.79 mm in May and its minimum is 12.14 mm in August (Fig. 8 ).
Average monthly precipitation from 1996 to 2015
Subsurface distribution of temperature and pressure
The reservoir thermodynamic data including temperature and pressure were measured from the exploration boreholes.
In a geothermal system, there is a need to specify the temperature distribution in assessing reservoir characteristic. To achieve the goal, it is necessary to measure downhole temperature under an equilibrium condition and to be described under the stable state of reservoir. To study the reservoir temperature changes vertically and horizontally, the contour plots and vertical cross sections have to be provided, accurately. These plots are useful to show how the interaction of hot and cold fluids occurs, so they are very important in developing a proper framework for the hydrogeological model of the system (Abdollahzadeh Bina 2009 ; Porkhial et al. 2015 ).
Figure 9 shows the stable temperature profiles for ten wells including NWS-1, NWS-3, NWS-4, NWS-5, NWS-6, NWS-7, NWS-8, NWS-9, NWS-10 and NWS-11. According to a measured water level equal to 2413 masl at well NWS-6, a representative ‘Boiling-Point-for-Depth’ (BPD) curve is also shown. Since, all of the temperature profiles are below the BPD curve, one may say that the reservoir in the study area contains water only. At an altitude of about 1800 masl, behind the casing of well NWS-1, the condition is near the BPD curve. It means that there is probably a two-phase fluid condition. For wells NWS-6, NWS-7 and NWS-10, the temperatures below the altitude of +1500 masl are near the BPD curve. The temperature reverse mode is slightly observed between +700 and −200 masl. Below the altitude of −200 masl at well NWS-1, +600 masl at well NWS-3 and +300 masl at well NWS-11, the temperature increases with depth. The highest temperature can be estimated at about 240–243 °C in well NWS-1 and in the altitude of about +1800 masl and also at well NWS-10 at an altitude of about 850 masl. There is a deep upflow zone between the wells NWS-1, NWS-6, NWS-7 and NWS-10, vertically and in southern direction.
Stable well temperatures for the NW Sabalan wells
By the use of measured temperatures in all exploration wells, temperature contours were plotted in a cross section along the section AB of Fig. 1 b. The result is shown in Fig. 10 a. As illustrated in this figure, the temperature increases from north to south. This means that there is possibly a high temperature upflow zone in the southern part of the area under investigation which is located below the Pad D. At a depth zone of about 1300–1750 masl, between wells NWS-6, NWS-7 and NWS-10 and NWS-1 located between Pad D and Pad A, the highest temperature of the reservoir (>240 °C) can be estimated. Also, a hot outflow from there originates and flows to SE-NW direction towards the well NWS-3. Besides, the cap rock with a thickness of about 500 m can be well recognised in the range of about 2300–1800 masl. The main flow below the drilled area covers at least a 2200 m thick convection zone approximately below the altitude 1700 masl.
Distribution of subsurface pressure and temperature for cross section AB of Fig. 1 b ( a temperature, b pressure)
Several faults, namely NW3, NW5, NE5, NNW2 and NW4 build the upflow zone for the reservoir system. Figure 10 b shows the cross section of estimated pressure using available data obtained from 10 deep wells. This cross section was built using the pressure profiles of the wells which are equivalent to the pressure formed at the major feed zones, only.
Conceptual model
Figure 11 shows the final conceptual model for the cross section AB of Fig. 1 b. This model has been developed based on the geological, geophysical, geochemical, temperature data described in the previous sections. The results of geophysical survey revealed that the geothermal reserve is associated with the hydraulic conductivity zones below the Pads E and D, 1000 m below sea level. In addition, the hydraulic conductivity zones were identified below the Pad D and E by resistivity values of less than 20 Ωm at elevation zones varying from 1200 to 2800 masl. The results of the temperature profiles near the reservoir show that the top of the reservoir is at about 1800 masl, below which there are little temperature changes in the convecting reservoir. The model includes a deep geothermal reservoir with a temperature range of 230–243 °C. Faults NW3 and NE5 possibly control the conductivity of the reservoir. Moreover, they have important role in the creation of upflow zone below the wells NWS-6, 7, 10 (Pad D) where the heat and pressure differences cause a single-phase flow travel through these faults and the other fractures by convection phenomenon. It is noteworthy to say that there is a good correlation between the presence of hot springs and faults in the study area. It seems that the upward flow takes place through faults NNW2 and NE5 and NE1 and eventually discharges to surface as hot springs. According to the results of stable temperature profiles (Fig. 9 ) and distribution of subsurface temperature (Fig. 10 a), it is remarkable to express the idea that the infiltration of meteoric water creates a shallow cold zone and this mixes with the upflow zone moving upwards to the shallower zones in subsurface. By penetrating meteoric waters to deeper zones, the geothermal system is fed and the fluid flow circle is eventually completed.
Overall conceptual model of NW Sabalan geothermal field
Conclusions
Identifying physical and chemical processes involved in a particular problem and creating an accurate conceptual model are the most critical issues in the development of a successful numerical model. An appropriate conceptual model can be used to describe the crucial features of geological situations and define the principal processes in a geothermal system. In this study, a conceptual model has been presented based on the available geological, exploration and drilling data for NW Sabalan geothermal field. The model includes a deep geothermal reservoir with a temperature range of 230–243 °C. This model can provide useful information regarding temperature, pressure, outflow zone and fluid flow circulation within the system that can be successfully used to improve the previous numerical models and provide a basis for sustainable development of geothermal field for imminent targets. However, timely updates of this conceptual model based on new surface and subsurface exploration data are critical for successful development planning, well siting and resource assessment of NW Sabalan geothermal field. Faults NW3 and NE5 possibly control the conductivity of the reservoir rocks. Furthermore, they have important role in the creation of upflow zone below the wells NWS-6, 7, 10 on pad D.
Abdollahzadeh Bina F. Geothermal resource assessment of the NW-Sabalan Field, Iran, through well testing. Geothermal training programme. United National University (UNU). 2009;6:15–44.
Amidi M. 1:250,000 geological quadrangle map of Iran: Ahar. Ministry of mines and metals, geological survey of Iran, Tehran. 1978.
Amini A. Geological report of Meshginshahr area. Ministry of mines and metals, geological survey of Iran, Tehran. 1988.
Arnorsson S, Gunnlaugsson E, Svavarsson H. The chemistry of geothermal waters in Iceland III. Chemical geothermometry in geothermal investigations. Geochim Cosmochim Acta. 1983;47:567–77.
Article Google Scholar
Arnórsson S. The use of mixing models and chemical geothermometers for estimating underground temperatures in geothermal systems. J Volcanol Geotherm Res. 1985;23:209–335.
Bogie I, Cartwright AJ, Khosrawi Kh, Talebi B, Sahabi F. The Meshkin Shahr geothermal prospect, Iran. In: Proceedings world geothermal congress 2000, Japan. 2000;997–1002.
Bromley C, Khosrawi K, Talebi B. Geophysical exploration of Sabalan geothermal prospects in Iran. World Geothermal Congress 2000, Japan. 2000.
EDC (Energy Development Corporation). 2007MT survey of NW Sabalan geothermal project, NW Iran. Report submitted to SUNA. 2008.
EDC (Energy Development Corporation). 2009MT survey of NW Sabalan geothermal project, NW Iran. Report submitted to SUNA. 2010.
Emami M. 1:100,000 geological map of Iran, Sheet 5566. Ministry of mines and metals, geological survey of Iran, Tehran. 1994.
ENEL (Ente Nazionale per l’Energia Elettrica). Geothermal power development studies in Iran. General report on Sabalan Zone. Ente Nazionale per l’Energia Elettrica (Italy) report to the Ministry of Energy, Islamic Republic of Iran, Tehran. 1983.
Eshaghpour M. Borehole geology and alteration mineralogy of well NWS-4, Mt. Sabalan geothermal field, NW-Iran. In: Proceedings world geothermal congress 2005, Turkey. 2005;24–29.
Fotouhi M. Geothermal development in Sabalan, Iran. In: Proceedings world geothermal congress 1995, Italy. 1995;191–196.
Fournier RO. Chemical geothermometers and mixing model for geothermal systems. Geothermics. 1977;5:41–50.
Fournier RO. A revised Na/K geothermometer. Geotherm Resour Counc Trans. 1979;3:221–4.
Google Scholar
Fournier RO, Potter RWII. Magnesium correction to Na–K–Ca geothermometer. Geochim Cosmochim Acta. 1979;43:1543–50.
Fournier RO, Potter RWII. A revised and expanded silica (quartz) geothermometer. Geotherm Res Counc Bull. 1982;11–10:3–12.
Fournier RO. Water geothermometers applied to geothermal energy, in applications of geochemistry in geothermal reservoir development (F. D’Amore, ed.). UNITAR/UNDP Centre on Small Energy Resources (Ed.), Rome. 1991;37–69.
Ghaedrahmati R, Moradzadeh A, Fathianpour N, Lee SK, Porkhial S. 3-D inversion of MT data from the Sabalan geothermal field, Ardabil, Iran. J Appl Geophys. 2013;93:12–24.
Giggenbach WF. Geothermal solute equilibria. Derivation of. Na–K–Mg–Ca geoindicators. Geochimica et Cosmochimica. Acta 52. 1988;2749–2765.
Giggenbach WF. Chemical techniques in geothermal exploration, application of geochemistry in geothermal reservoir development (D’Amore, F., Ed.). UNITAR/UNDP Centre on Small Energy Resources, Rome. 1991;119–144.
Khojamli A. A conceptual model for Meshkinshahr geothermal resources using modelling of Magnetotelluric data, hydrogeochemical, geological and drilling data. M.Sc. thesis. Shahrood University of Technology. Shahrood, Iran. 2012.
Khosrawi Kh. Geochemistry of geothermal springs in the Sabalan Area, Azarbydjan-Iran. Geothermal training programme. United National University (UNU). 1996;7:135–159.
KML (Kingston Morrison). Sabalan geothermal project, Stage 1—surface exploration, final exploration report, Kingston Morrison Limited Co., report 2505-RPT-GE-003 for the Renewable Energy Organization of Iran, Tehran. 1999a.
KML (Kingston Morrison). Sabalan geothermal project stage 2—preparation for drilling, Kingston Morrison Limited Co. Report submitted to the Renewable Energy Organization of Iran, Tehran. 1999b.
Kosari Torbehbar A, Sattari SM. Geochemistry and isotope study of discharged geothermal fluids, NW Sabalan geothermal field, NW Iran. In: Proceedings world geothermal congress 2015, Melbourne. 2015;19–25.
Kosari Torbehbar A. Interpretation of geochemical well test data for well NWS-6D, NW-Sabalan, Iran: an implication for scaling potential and recommended inhibition methods. Geothermal training programme. United National University (UNU). 2011;18:357–390.
Manouchehri M. 1:250,000 Geological quadrangle map of Iran. Tabriz-Poldasht No. B1 & B2. Ministry of mines and metals, geological survey of Iran, Tehran. 1989.
McKenzie DS. Active tectonics of the Mediterranean region. Geophys J R Astr Soc. 1972;30:109–85.
Mousavi Z, Darvishzadeh A. Volcanology and geothermal research by using hydrothermal alteration processes of well NWS-4 at Sabalan volcano geothermal project, Iran. In: Proceedings world geothermal congress 2010, Indonesia. 2010;25–29.
Najafi Gh, Ghobadian B. Geothermal resources in Iran: the sustainable future. Renew Sustain Energy Rev. 2011;15:3946–51.
Nieva D, Nieva R. Developments in geothermal energy in Mexico. XII. A cationic composition geothermometer for prospection of geothermal resources’. Heat Recover Syst. 1987;7(3):243–58.
Nejad JE. 1:250,000 scale geological quadrangle map of Iran. NO D2 Ardabil. Ministry of mines and metals, geological survey of Iran, Tehran. 1987.
Noorollahi Y, Itoi R. Numerical simulation of Northwest Sabalan geothermal reservoir, Iran. In: World renewable energy congress. Sweden: Linköping University Electronic Press; 2011a, pp. 1257–1264.
Noorollahi Y, Itoi R. Production capacity estimation by reservoir numerical simulation of northwest (NW) Sabalan geothermal field, Iran. Energy. 2011;36:4552–69.
Noorollahi Y, Itoi R, Fujii H, Tanaka T. GIS integration model for geothermal exploration and well siting. Geothermics. 2008;37:107–31.
Noorollahi Y, Yousefi H, Itoi R, Ehara S. Geothermal energy resources and development in Iran. Renew Sustain Energy Rev. 2009;13:1127–32.
Porkhial S, Ghomshei MM, Yousefi P. Geothermal energy in Iran. In: Proceeding world geothermal congress 2010, Indonesia. 2010a;25–29.
Porkhial S, Rigor DM Jr, Lauro FB, Domingo BL. Magnetotelluric survey of NW Sabalan geothermal project, Iran. In: Proceeding world geothermal congress 2010, Indonesia. 2010b;25–29.
Porkhial S, Abdollahzadeh Bina F, Radmehr B, Johari Sefid P. Interpretation of the injection and heat up tests at Sabalan geothermal field, Iran. In: Proceedings world geothermal congress 2015, Melbourne. 2015;19–25.
Rahmani MR. Assessment of calcite scaling potential in the geothermal wells of the NW-Sabalan geothermal prospect, NW-Iran. Geothermal training programme. United National University (UNU). 2007;19:447–460.
Sabzemeidani R. Application of hydrogeological studies in considering the geothermal reservoirs in Meshkinshahr area. M.Sc. thesis. School of Mining, College of Engineering. University of Tehran. 2016.
SKM (Sinclair Knight Merz). Northwest Sabalan geothermal project, MT survey reanalysis. Report submitted to SUNA (Renewable Energy Organization of Iran). 2003.
SKM (Sinclair Knight Merz). Geological report for well NWS-4. Report for SUNA (Renewable Energy Organization of Iran), 2004a.
SKM (Sinclair Knight Merz). Geochemical evaluation of well NWS-1. Sinclair Knight Merz. Report for SUNA (Renewable Energy Organization of Iran). 2005a.
SKM (Sinclair Knight Merz). Well NWS-4 discharge evaluation report. Sinclair Knight Merz. Report for SUNA (Renewable Energy Organization of Iran). 2005b.
SKM (Sinclair Knight Merz). Well NWS-3 discharge evaluation report. Sinclair Knight Merz. Report for SUNA (Renewable Energy Organization of Iran). 2005c.
Stefansson V. Geothermal energy of Sabalan. U.N. mission report. 1989.
Strelbitskaya S, Radmehr B. Geochemical characteristics of reservoir fluid from NW-Sabalan geothermal field, Iran. In: Proceedings world geothermal congress 2010, Indonesia. 2010;25–29.
Talebi B, Khosrawi Kh, Ussher G. Review of resistivity surveys from the NW Sabalan geothermal field, Iran. In: Proceedings world geothermal congress 2005, Turkey. 2005;24–29.
Tassi F, Aguilera F, Vaselli O, Medina E. A geochemical survey of geothermal resources in the Tarapacá and Antofagasta regions (northern Chile). 7th International Symposium on Andean Geodynamics (ISAG 2008, Nice). Extended Abstracts. 2008;530–533.
TBCE (Tehran Berkeley Consulting Engineers). Geothermal power development studies, Sabalan zone. Tehran Berkeley Consulting Engineers. Report to the Ministry of Energy. Islamic Republic of Iran. Tehran. 1979.
Download references
Authors’ contributions
MS carried out the modelling and developing the results. MS also drafted the manuscript. The second and third authors, FD and YN, supervised the research and guided the interpretation of results. FD and YN also considerably edited and improved the drafts. SP advised the research and gave technical support during the data acquisition process. All authors read and approved the final manuscript.
Acknowledgements
The authors thank the School of Mining, College of Engineering, University of Tehran for supporting this research. The technical support given by Renewable Energy Organization of Iran (SUNA) during the data acquisition process is acknowledged.
Competing interests
The authors declare that they have no competing interests.
Availability of data and materials
Not applicable.
Consent for publication
Ethics approval and consent to participate, publisher’s note.
Springer Nature remains neutral with regard to jurisdictional claims in published maps and institutional affiliations.
Author information
Authors and affiliations.
School of Mining, College of Engineering, University of Tehran, Tehran, Iran
Mirmahdi Seyedrahimi-Niaraq & Faramarz Doulati Ardejani
Mine Environment and Hydrogeology Research Laboratory, University of Tehran, Tehran, Iran
Department of Renewable Energies Engineering, Faculty of New Sciences & Technologies, University of Tehran, Tehran, Iran
Younes Noorollahi
Renewable Energy Organization of Iran (SUNA), Tehran, Iran
Soheil Porkhial
You can also search for this author in PubMed Google Scholar
Corresponding author
Correspondence to Faramarz Doulati Ardejani .
Rights and permissions
Open Access This article is distributed under the terms of the Creative Commons Attribution 4.0 International License ( http://creativecommons.org/licenses/by/4.0/ ), which permits unrestricted use, distribution, and reproduction in any medium, provided you give appropriate credit to the original author(s) and the source, provide a link to the Creative Commons license, and indicate if changes were made.
Reprints and permissions
About this article
Cite this article.
Seyedrahimi-Niaraq, M., Doulati Ardejani, F., Noorollahi, Y. et al. Development of an updated geothermal reservoir conceptual model for NW Sabalan geothermal field, Iran. Geotherm Energy 5 , 14 (2017). https://doi.org/10.1186/s40517-017-0073-0
Download citation
Received : 24 May 2017
Accepted : 03 August 2017
Published : 09 August 2017
DOI : https://doi.org/10.1186/s40517-017-0073-0
Share this article
Anyone you share the following link with will be able to read this content:
Sorry, a shareable link is not currently available for this article.
Provided by the Springer Nature SharedIt content-sharing initiative
- NW Sabalan geothermal field
- Subsurface temperature
- Geothermal conceptual model
- Mechanical Engineering
- Flow Simulation
Practical Aspects of Upscaling Geocellular Geological Models for Reservoir Fluid Flow Simulations: A Case Study in Integrating Geology, Geophysics, and Petroleum Engineering Multiscale Data from the Hunton Group
- Energies 13(7):1604

- University of Oklahoma| Mewbourne College of Earth and Energy

- Indian Institute of Technology (ISM) Dhanbad

- University of Oklahoma

Abstract and Figures

Discover the world's research
- 25+ million members
- 160+ million publication pages
- 2.3+ billion citations
- INT J GREENH GAS CON
- Shakhawat Hossain
- Gary J. Hampson

- Sanskar Sharma
- Aditya Kukreti
- Arnim Rawat

- Rouzbeh Moghanloo
- Nicholas W Hayman
- Zhenxue Jiang
- Guanghui Wu

- Yintao Zhang
- Yicheng Liu

- Mashhad Fahes
- Mohamed Suliman
- J PETROL SCI ENG

- J BRAZ SOC MECH SCI
- Guilherme Daniel Avansi

- Mun-Hong Hui
- Mohammad Karimi-Fard

- Louis J. Durlofsky

- Recruit researchers
- Join for free
- Login Email Tip: Most researchers use their institutional email address as their ResearchGate login Password Forgot password? Keep me logged in Log in or Continue with Google Welcome back! Please log in. Email · Hint Tip: Most researchers use their institutional email address as their ResearchGate login Password Forgot password? Keep me logged in Log in or Continue with Google No account? Sign up

An official website of the United States government
Here's how you know
Official websites use .gov A .gov website belongs to an official government organization in the United States.
Secure .gov websites use HTTPS A lock ( ) or https:// means you’ve safely connected to the .gov website. Share sensitive information only on official, secure websites.
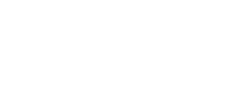
- Digg
Latest Earthquakes | Chat Share Social Media
USGS Announces Elevation and Hydrography Data Acquisition Partnership Awards, as part of President Biden’s Investing in America agenda
The U.S. Geological Survey has selected 21 projects in 18 states to receive funding for the collection of elevation and hydrography data through the new 3D National Topography Model (3DNTM) Data Collaboration Announcement (DCA) as part of President Biden’s Investing in America agenda.
These new partnerships are made possible through $6 million in funding from the Inflation Reduction Act, with additional federal funding provided by USDA through the Natural Resources Conservation Service and Farm Production and Conservation Business Center and by the USGS.
Current and accurate 3D elevation and hydrography data aid critical decision-making that protects people, property and the environment, including flood-risk management , agriculture , infrastructure projects, and natural hazards risk management. The benefit to the American public of these USGS programs has been estimated at approximately $8.6 billion annually.
Responding to the ever-greater need for high-quality, three-dimensional elevation data, the USGS created the 3D Elevation Program (3DEP) with the goal of providing the first-ever publicly accessible baseline of consistent, high-resolution topographic elevation data for the Nation. Using those data, the USGS’s 3D Hydrography Program (3DHP) is also working to completely refresh information and models on the Nation’s hydrography that can improve discovery and sharing of water-related data.
The 21 new partnerships announced today are expected to add more than 72,000 square miles of lidar data to 3DEP data holdings and more than 97,000 square miles of terrestrial hydrography data to 3DHP data holdings.
"We are thrilled with the number of proposals submitted through the new 3DNTM DCA process, confirming interest in the future of 3DEP and 3DHP,” said Darcee Killpack, USGS Acting Associate Director for Core Science Systems. “It is inspiring to see the variety of landscapes and user applications the data will support. We are also grateful for the inclusion of Inflation Reduction Act funds to accelerate our goal of acquiring nationwide coverage of 3D data .”
The 3DNTM DCA is an open process for finding and selecting partnership opportunities. Federal agencies, state and local governments, Tribes, academic institutions, and the private sector are eligible to submit project proposals. The DCA presents an annual opportunity to leverage the expertise and capacity of private-sector mapping firms that acquire high-quality, three-dimensional elevation data of the United States.
More information about 3DNTM, 3DEP and 3DHP, including selected DCA projects and updates on current and future partnership opportunities, is available at www.usgs.gov/3d-national-topography-model
Get Our News
These items are in the RSS feed format (Really Simple Syndication) based on categories such as topics, locations, and more. You can install and RSS reader browser extension, software, or use a third-party service to receive immediate news updates depending on the feed that you have added. If you click the feed links below, they may look strange because they are simply XML code. An RSS reader can easily read this code and push out a notification to you when something new is posted to our site.
Information Systems News
Maps and Mapping News
Technical Announcement News
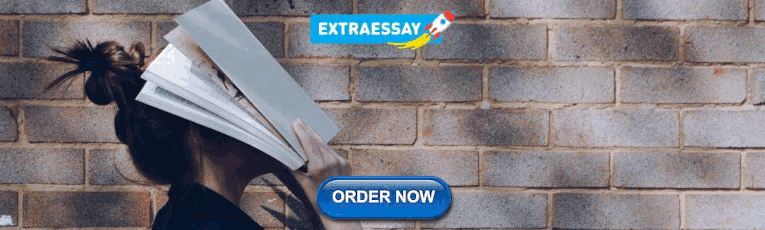
IMAGES
VIDEO
COMMENTS
In order to obtain an adequate geological model of any kind, proper inte- gration of geophysical data, borehole logs and geological expert knowledge is important. ... This thesis addresses these ...
Figure 1 illustrates the steps in a typical geological modeling process. Raw data collected from various. sources can be considered as two types - spatial data and pr operties data. The spatial ...
Compared with the traditional information display form, the 3D geological model can more flexibly and intuitively express the geological structure, stratigraphic lithology, hydrogeological conditions and other geological environmental conditions closely related to the development and utilization of underground space, and this model is widely ...
In either case, there are several key considerations to successfully approach an integrated geological and geophysical modelling exercise. Interpret your data - assess the geophysical signatures and their relationship with geology and rock properties. Develop a starting model and establish a geological framework for modelling.
This thesis describes the geostatistics associated with creating a geological model of the Abacherli reservoir within the Mahala oil field of the Los Angeles Basin of Southern California using a variogram-based two-point geostatistical approach. The geology of this study area
The term model is used by scientists and engineers to describe things as varied as scaled physical replicas, drawings, governing equations and computer simulations. C25's working definition of the term model as used in engineering geology is simply: A model is an approximation of reality created for the purpose of solving a problem.
This paper summarizes the general concept of geological modeling; compares the characteristics of borehole-based modeling, cross-section based modeling and multi-source interactive modeling; analyses key techniques in 3-D geological modeling; and highlights the main difficulties and directions of future studies.
In Solid Modeling, a boundary representation (b-rep) defines solids by their bounding surfaces, providing an efficient volume description.Building on this representation, we present the notion of a Sealed Geological Model.In such a model, the geological surfaces define a partition of the domain of interest into regions; analytic functions can be defined in these regions to describe the spatial ...
Certain applications, such as understanding the influence of bedrock geology on hydrology in complex mountainous settings, demand 3D geological models that are detailed, high-resolution, accurate ...
The common industry practice is to assign core-scale properties directly to a geological model gridblock. The sub-grid- and sub-core-scale heterogeneities are neglected. The objective of this work is two-fold. First, we demonstrate that these small-scale heterogeneities can influence large-scale CO 2 migration during geological storage ...
This paper proposes a geostatistical approach for geological modelling and for validating an interpreted geological model, by identifying the areas of an ore deposit with a high probability of being misinterpreted, based on quantitative coregionalised covariates correlated with the geological categories. This proposal is presented through a case study of an iron ore deposit at a stage where ...
A Bayesian Framework for Quantifying Fault Network Uncertainty Using a Marked Point Process Model [PhD Thesis, Stanford University]. https://searchworks.stanford.edu/view ... Quantitative Reservoir Characterization Integrating Seismic Data and Geological Scenario Uncertainty [PhD Thesis, Stanford University]. https://searchworks.stanford.edu ...
The development of the geological model for any site therefore requires specific consideration of the regional and local geological and geomorphological history and current ground surface conditions. This concept is examined from the scale of plate tectonics to the smallest geological features and micro climate. Simple models of various ...
geochemical information). This thesis aims at producing an integrated 3D geological model in order to identify new interesting prospective areas around the Mullikkoräme deposit. All the available geophysical, geological, geochemical data has been integrated into a unique comprehensive 3D gOcad© prospective geomodel. A draft 3D skeleton model is
The 3D geological model usually reflects the geometric characteristics, spatial contact relationships, and pattern of change in the attributes of the geological body. To guarantee the accuracy and practical application value of the geological model, a large amount of geological data is needed to provide constraints for the construction of the ...
Three-dimensional Mineral Prospectivity Mapping (3DMPM) is an innovative approach to mineral exploration that combines multiple geological data sources to create a three-dimensional (3D) model of a mineral deposit. It provides an accurate representation of the subsurface that can be used to identify areas with mineral potential. These 3D geological models are the typical data source for 3D ...
A Systematic Approach to Hydrogeological Conceptual Model Testing, Combining Remote Sensing and Geophysical Data. Trine Enemark, Corresponding Author. Trine Enemark ... The geology consists of sand and clay sediments of Mesozoic-Cenozoic origin ranging in thickness of less than 25 m to over 100 m and overlaying the basement geology of dolostone
To conceive and build a groundwater model (e.g., for water resources), one must go through a geological. model; both models are complex in nature and require e x perts in each domain with very ...
The geometry of the geological units represented in the numerical groundwater model (Taylor, 2022; Taylor & Peach, 2024, this Special Issue) has been based on a 3D geological model developed with 10 geological units, in which some deposits with similar properties (e.g., alluvial deposits of differing ages) have been grouped together. The ...
Theses/Dissertations from 2022. PDF. Functional morphology and taphonomy of Ordovician-Silurian diploporan echinoderms, Stephen Ashley Hill. PDF. Determining Depth of Closure Based on Time-series Beach Profiles and Empirical Formulas: A Case Study along the Florida Coast, Elizabeth Royer. PDF.
This thesis is aimed at the current high-water-phase development of Shengtuo Oilfield reservoir and the problems existing in geological research. ... The 3D geological model of the undulating ...
In this paper, a conceptual model has been developed for NW Sabalan geothermal field using exploration indicators. These indicators include the data and results of subsurface and surface investigations comprising geology, geophysics , hydro-geochemistry, hydrology and temperature and pressure distribution. The subsurface information was obtained from 10 deep exploration wells as well as from ...
The original geologic model using a 20 x 30 x 1 ft geologic grid re sulted in 13,292,228 cells which exceeded the capabilities of our computer resources. We therefore upscaled the initial grid to ...
The U.S. Geological Survey has selected 21 projects in 18 states to receive funding for the collection of elevation and hydrography data through the new 3D National Topography Model (3DNTM) Data Collaboration Announcement (DCA) as part of President Biden's Investing in America agenda.