Have a language expert improve your writing
Run a free plagiarism check in 10 minutes, generate accurate citations for free.
- Knowledge Base
Methodology
- Qualitative vs. Quantitative Research | Differences, Examples & Methods
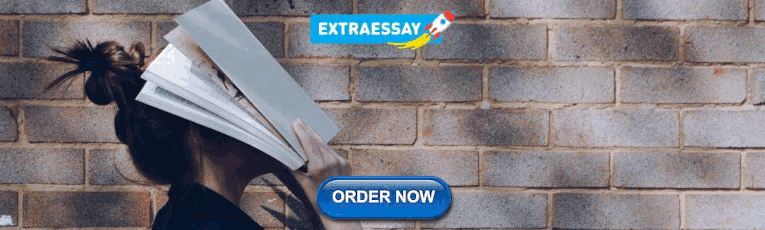
Qualitative vs. Quantitative Research | Differences, Examples & Methods
Published on April 12, 2019 by Raimo Streefkerk . Revised on June 22, 2023.
When collecting and analyzing data, quantitative research deals with numbers and statistics, while qualitative research deals with words and meanings. Both are important for gaining different kinds of knowledge.
Common quantitative methods include experiments, observations recorded as numbers, and surveys with closed-ended questions.
Quantitative research is at risk for research biases including information bias , omitted variable bias , sampling bias , or selection bias . Qualitative research Qualitative research is expressed in words . It is used to understand concepts, thoughts or experiences. This type of research enables you to gather in-depth insights on topics that are not well understood.
Common qualitative methods include interviews with open-ended questions, observations described in words, and literature reviews that explore concepts and theories.
Table of contents
The differences between quantitative and qualitative research, data collection methods, when to use qualitative vs. quantitative research, how to analyze qualitative and quantitative data, other interesting articles, frequently asked questions about qualitative and quantitative research.
Quantitative and qualitative research use different research methods to collect and analyze data, and they allow you to answer different kinds of research questions.
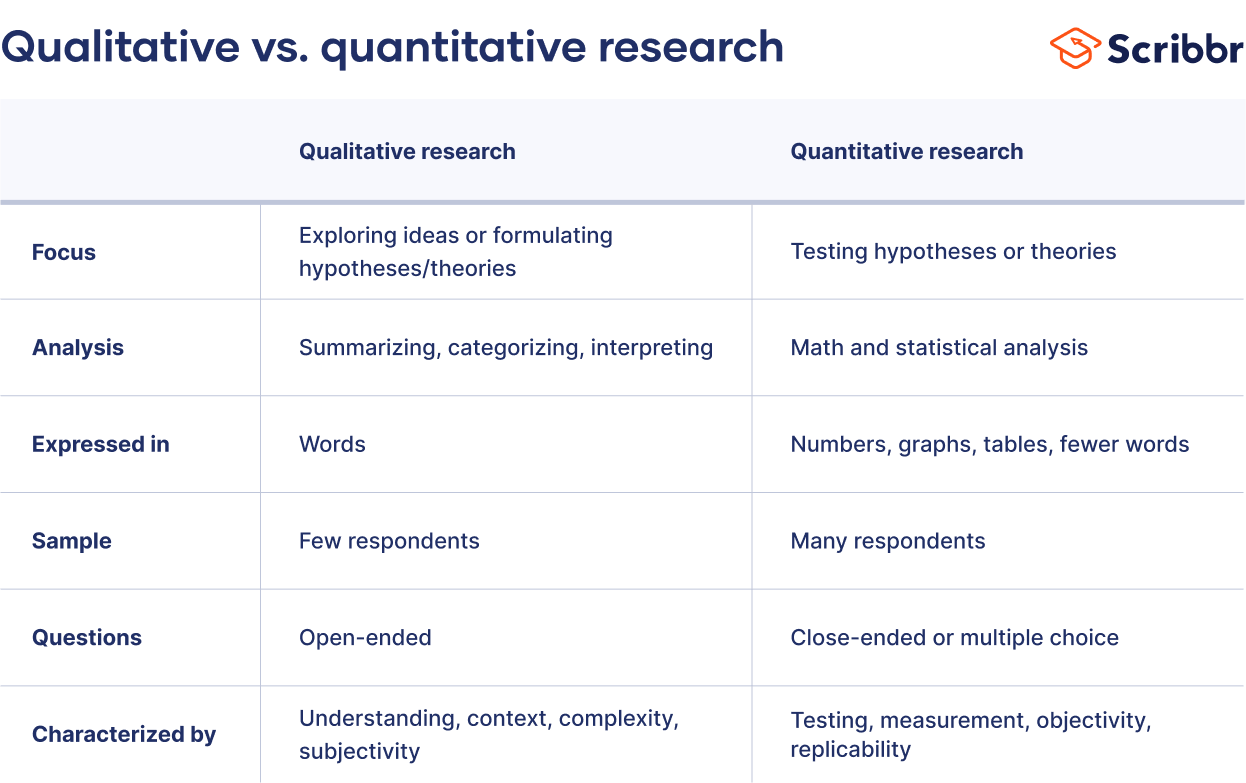
Quantitative and qualitative data can be collected using various methods. It is important to use a data collection method that will help answer your research question(s).
Many data collection methods can be either qualitative or quantitative. For example, in surveys, observational studies or case studies , your data can be represented as numbers (e.g., using rating scales or counting frequencies) or as words (e.g., with open-ended questions or descriptions of what you observe).
However, some methods are more commonly used in one type or the other.
Quantitative data collection methods
- Surveys : List of closed or multiple choice questions that is distributed to a sample (online, in person, or over the phone).
- Experiments : Situation in which different types of variables are controlled and manipulated to establish cause-and-effect relationships.
- Observations : Observing subjects in a natural environment where variables can’t be controlled.
Qualitative data collection methods
- Interviews : Asking open-ended questions verbally to respondents.
- Focus groups : Discussion among a group of people about a topic to gather opinions that can be used for further research.
- Ethnography : Participating in a community or organization for an extended period of time to closely observe culture and behavior.
- Literature review : Survey of published works by other authors.
A rule of thumb for deciding whether to use qualitative or quantitative data is:
- Use quantitative research if you want to confirm or test something (a theory or hypothesis )
- Use qualitative research if you want to understand something (concepts, thoughts, experiences)
For most research topics you can choose a qualitative, quantitative or mixed methods approach . Which type you choose depends on, among other things, whether you’re taking an inductive vs. deductive research approach ; your research question(s) ; whether you’re doing experimental , correlational , or descriptive research ; and practical considerations such as time, money, availability of data, and access to respondents.
Quantitative research approach
You survey 300 students at your university and ask them questions such as: “on a scale from 1-5, how satisfied are your with your professors?”
You can perform statistical analysis on the data and draw conclusions such as: “on average students rated their professors 4.4”.
Qualitative research approach
You conduct in-depth interviews with 15 students and ask them open-ended questions such as: “How satisfied are you with your studies?”, “What is the most positive aspect of your study program?” and “What can be done to improve the study program?”
Based on the answers you get you can ask follow-up questions to clarify things. You transcribe all interviews using transcription software and try to find commonalities and patterns.
Mixed methods approach
You conduct interviews to find out how satisfied students are with their studies. Through open-ended questions you learn things you never thought about before and gain new insights. Later, you use a survey to test these insights on a larger scale.
It’s also possible to start with a survey to find out the overall trends, followed by interviews to better understand the reasons behind the trends.
Qualitative or quantitative data by itself can’t prove or demonstrate anything, but has to be analyzed to show its meaning in relation to the research questions. The method of analysis differs for each type of data.
Analyzing quantitative data
Quantitative data is based on numbers. Simple math or more advanced statistical analysis is used to discover commonalities or patterns in the data. The results are often reported in graphs and tables.
Applications such as Excel, SPSS, or R can be used to calculate things like:
- Average scores ( means )
- The number of times a particular answer was given
- The correlation or causation between two or more variables
- The reliability and validity of the results
Analyzing qualitative data
Qualitative data is more difficult to analyze than quantitative data. It consists of text, images or videos instead of numbers.
Some common approaches to analyzing qualitative data include:
- Qualitative content analysis : Tracking the occurrence, position and meaning of words or phrases
- Thematic analysis : Closely examining the data to identify the main themes and patterns
- Discourse analysis : Studying how communication works in social contexts
If you want to know more about statistics , methodology , or research bias , make sure to check out some of our other articles with explanations and examples.
- Chi square goodness of fit test
- Degrees of freedom
- Null hypothesis
- Discourse analysis
- Control groups
- Mixed methods research
- Non-probability sampling
- Quantitative research
- Inclusion and exclusion criteria
Research bias
- Rosenthal effect
- Implicit bias
- Cognitive bias
- Selection bias
- Negativity bias
- Status quo bias
Quantitative research deals with numbers and statistics, while qualitative research deals with words and meanings.
Quantitative methods allow you to systematically measure variables and test hypotheses . Qualitative methods allow you to explore concepts and experiences in more detail.
In mixed methods research , you use both qualitative and quantitative data collection and analysis methods to answer your research question .
The research methods you use depend on the type of data you need to answer your research question .
- If you want to measure something or test a hypothesis , use quantitative methods . If you want to explore ideas, thoughts and meanings, use qualitative methods .
- If you want to analyze a large amount of readily-available data, use secondary data. If you want data specific to your purposes with control over how it is generated, collect primary data.
- If you want to establish cause-and-effect relationships between variables , use experimental methods. If you want to understand the characteristics of a research subject, use descriptive methods.
Data collection is the systematic process by which observations or measurements are gathered in research. It is used in many different contexts by academics, governments, businesses, and other organizations.
There are various approaches to qualitative data analysis , but they all share five steps in common:
- Prepare and organize your data.
- Review and explore your data.
- Develop a data coding system.
- Assign codes to the data.
- Identify recurring themes.
The specifics of each step depend on the focus of the analysis. Some common approaches include textual analysis , thematic analysis , and discourse analysis .
A research project is an academic, scientific, or professional undertaking to answer a research question . Research projects can take many forms, such as qualitative or quantitative , descriptive , longitudinal , experimental , or correlational . What kind of research approach you choose will depend on your topic.
Cite this Scribbr article
If you want to cite this source, you can copy and paste the citation or click the “Cite this Scribbr article” button to automatically add the citation to our free Citation Generator.
Streefkerk, R. (2023, June 22). Qualitative vs. Quantitative Research | Differences, Examples & Methods. Scribbr. Retrieved April 12, 2024, from https://www.scribbr.com/methodology/qualitative-quantitative-research/
Is this article helpful?
Raimo Streefkerk
Other students also liked, what is quantitative research | definition, uses & methods, what is qualitative research | methods & examples, mixed methods research | definition, guide & examples, what is your plagiarism score.

Quantitative and Qualitative Research
- I NEED TO . . .
What is Quantitative Research?
- What is Qualitative Research?
- Quantitative vs Qualitative
- Step 1: Accessing CINAHL
- Step 2: Create a Keyword Search
- Step 3: Create a Subject Heading Search
- Step 4: Repeat Steps 1-3 for Second Concept
- Step 5: Repeat Steps 1-3 for Quantitative Terms
- Step 6: Combining All Searches
- Step 7: Adding Limiters
- Step 8: Save Your Search!
- What Kind of Article is This?
- More Research Help This link opens in a new window
Quantitative methodology is the dominant research framework in the social sciences. It refers to a set of strategies, techniques and assumptions used to study psychological, social and economic processes through the exploration of numeric patterns . Quantitative research gathers a range of numeric data. Some of the numeric data is intrinsically quantitative (e.g. personal income), while in other cases the numeric structure is imposed (e.g. ‘On a scale from 1 to 10, how depressed did you feel last week?’). The collection of quantitative information allows researchers to conduct simple to extremely sophisticated statistical analyses that aggregate the data (e.g. averages, percentages), show relationships among the data (e.g. ‘Students with lower grade point averages tend to score lower on a depression scale’) or compare across aggregated data (e.g. the USA has a higher gross domestic product than Spain). Quantitative research includes methodologies such as questionnaires, structured observations or experiments and stands in contrast to qualitative research. Qualitative research involves the collection and analysis of narratives and/or open-ended observations through methodologies such as interviews, focus groups or ethnographies.
Coghlan, D., Brydon-Miller, M. (2014). The SAGE encyclopedia of action research (Vols. 1-2). London, : SAGE Publications Ltd doi: 10.4135/9781446294406
What is the purpose of quantitative research?
The purpose of quantitative research is to generate knowledge and create understanding about the social world. Quantitative research is used by social scientists, including communication researchers, to observe phenomena or occurrences affecting individuals. Social scientists are concerned with the study of people. Quantitative research is a way to learn about a particular group of people, known as a sample population. Using scientific inquiry, quantitative research relies on data that are observed or measured to examine questions about the sample population.
Allen, M. (2017). The SAGE encyclopedia of communication research methods (Vols. 1-4). Thousand Oaks, CA: SAGE Publications, Inc doi: 10.4135/9781483381411
How do I know if the study is a quantitative design? What type of quantitative study is it?
Quantitative Research Designs: Descriptive non-experimental, Quasi-experimental or Experimental?
Studies do not always explicitly state what kind of research design is being used. You will need to know how to decipher which design type is used. The following video will help you determine the quantitative design type.
- << Previous: I NEED TO . . .
- Next: What is Qualitative Research? >>
- Last Updated: Dec 8, 2023 10:05 PM
- URL: https://libguides.uta.edu/quantitative_and_qualitative_research
University of Texas Arlington Libraries 702 Planetarium Place · Arlington, TX 76019 · 817-272-3000
- Internet Privacy
- Accessibility
- Problems with a guide? Contact Us.
- Member Benefits
- Communities
- Grants and Scholarships
- Student Nurse Resources
- Member Directory
- Course Login
- Professional Development
- Institutions Hub
- ONS Course Catalog
- ONS Book Catalog
- ONS Oncology Nurse Orientation Program™
- Account Settings
- Help Center
- Print Membership Card
- Print NCPD Certificate
- Verify Cardholder or Certificate Status
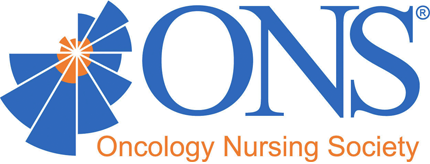
- Trouble finding what you need?
- Check our search tips.
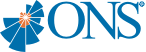
- Oncology Nursing Forum
- Number 4 / July 2014
Measurements in Quantitative Research: How to Select and Report on Research Instruments
Teresa L. Hagan
Measures exist to numerically represent degrees of attributes. Quantitative research is based on measurement and is conducted in a systematic, controlled manner. These measures enable researchers to perform statistical tests, analyze differences between groups, and determine the effectiveness of treatments. If something is not measurable, it cannot be tested.
Jump to a section
Related articles, systematic reviews, case study research methodology in nursing research, preferred reporting items for systematic reviews and meta-analyses.
Measurements in quantitative research: how to select and report on research instruments
Affiliation.
- 1 Department of Acute and Tertiary Care in the School of Nursing, University of Pittsburgh in Pennsylvania.
- PMID: 24969252
- DOI: 10.1188/14.ONF.431-433
Measures exist to numerically represent degrees of attributes. Quantitative research is based on measurement and is conducted in a systematic, controlled manner. These measures enable researchers to perform statistical tests, analyze differences between groups, and determine the effectiveness of treatments. If something is not measurable, it cannot be tested.
Keywords: measurements; quantitative research; reliability; validity.
- Clinical Nursing Research / methods*
- Clinical Nursing Research / standards
- Fatigue / nursing*
- Neoplasms / nursing*
- Oncology Nursing*
- Quality of Life*
- Reproducibility of Results

My Account  
Donate  
Research Methods
Quantitative research methods.
- Qualitative Research Methods
- Mixed Methods
- Books on Research Methods
Need Help?
- Ask us a question
- Schedule a research appointment
Quantitative research methods involve the collection of numerical data and the use of statistical analysis to draw conclusions. This method is suitable for research questions that aim to measure the relationship between variables, test hypotheses, and make predictions. Here are some tips for choosing quantitative research methods:
Identify the research question: Determine whether your research question is best answered by collecting numerical data. Quantitative research is ideal for research questions that can be quantified, such as questions that ask how much, how many, or how often.
Choose the appropriate data collection methods: Select data collection methods that allow you to collect numerical data, such as surveys, experiments, or observational studies. Surveys involve asking participants to respond to a set of standardized questions, while experiments involve manipulating variables to determine their effect on an outcome. Observational studies involve observing and recording behaviors or events in a natural setting.
When choosing a data collection method, it's important to consider the feasibility, reliability, and validity of the method. Feasibility refers to whether the method is practical and achievable within the available resources, while reliability refers to the consistency of the results over time and across different observers or settings. Validity refers to whether the method accurately measures what it's intended to measure.
The sample size: Decide on the sample size that is needed to produce statistically significant results. The sample size is the number of participants in the study. The larger the sample size, the more reliable the results are likely to be. However, a larger sample size also requires more resources and time. Therefore, it's important to determine the appropriate sample size based on the research question and available resources.
Statistical test: Choose the appropriate statistical analysis techniques based on the type of data you have collected and the research question. Common statistical analysis techniques include descriptive statistics, correlation analysis, regression analysis, and t-tests. Descriptive statistics summarize the data using measures such as mean, standard deviation, and frequency. Correlation analysis examines the relationship between two or more variables. Regression analysis examines the relationship between one dependent variable and one or more independent variables. T-tests compare the means of two groups.
When selecting a statistical analysis technique, it's important to consider the assumptions of the technique and whether they are appropriate for the data being analyzed. It's also important to consider the level of statistical significance required to draw meaningful conclusions.
Strength and Limitations
Strength and limitations of quantitative research methods:.
- The use of statistical analysis allows for the identification of patterns and relationships between variables.
- Provides a structured and standardized approach to data collection, allowing for replication of studies and comparisons across studies.
- It can produce reliable and valid results which are generalizable to larger populations.
- Allows for hypothesis testing, making it suitable for research questions that require a cause-and-effect relationship.
- It can produce numerical data, making it easy to summarize and communicate results.
Limitations:
- It may oversimplify complex phenomena by reducing them to numerical data.
- It may not capture the context and subjective experiences of individuals.
- It may not allow for the exploration of new ideas or unexpected findings.
- It may be influenced by researcher bias or the use of inappropriate statistical techniques.
- It may not account for variables that are difficult to measure or control.
- << Previous: Home
- Next: Qualitative Research Methods >>
- Seton Hall University
- 400 South Orange Avenue
- South Orange, NJ 07079
- (973) 761-9000
- Student Services
- Parents and Families
- Career Center
- Web Accessibility
- Visiting Campus
- Public Safety
- Disability Support Services
- Campus Security Report
- Report a Problem
- Login to LibApps
- Frontiers in Research Metrics and Analytics
- Research Assessment
- Research Topics
Quality and Quantity in Research Assessment: Examining the Merits of Metrics
Total Downloads
Total Views and Downloads
About this Research Topic
It is widely acknowledged that in the current academic landscape, publishing is the primary measure for assessing a researcher’s value. This is manifested by associating individuals' academic performance with different types of metrics, typically the number of publications or citations (quantity), rather
It is widely acknowledged that in the current academic landscape, publishing is the primary measure for assessing a researcher’s value. This is manifested by associating individuals' academic performance with different types of metrics, typically the number of publications or citations (quantity), rather than with the content of their works (quality). Concerns have been raised that this approach for evaluating research is causing significant ambiguity in the way science is done and how scientists' perceived performance is evaluated. For example, bibliometric indicators, such as the h-index or journal impact factor (JIF) in scientific assessments, are currently widespread. This causes multiple issues because these methods usually overlook the age or career stage of a researcher, the field size, publication, and citation cultures in different areas, any potential co-authorship, etc. Although the number of publications and citations, the h-index, the JIF, and so forth may indeed be relevant and should be considered as an indicator of visibility and popularity, they are certainly not indications of intellectual value or scientific quality by themselves.
To interrogate the conundrum between quantity and quality in research evaluation, some researchers dwell on rigorous and complementary indicators of a scientist's performance by critically analyzing a plethora of scientometric data. Others have argued that the scientific performance of an individual or group must be evaluated by peer-review processes based on their impact in their respective fields or the originality, strength, reproducibility, and relevance of their publications. Nevertheless, scientific project reviews, grant funding decisions, and university career advancement steps are often based on decisive input from non-experts who can readily use bibliometric indices. As a consequence, the newer and more robust tools or methods that consider the normalization of bibliometric indicators by the field and other influential parameters are encouraged to be shared and embraced by the research community, universities, and funding agencies. In addition, it is vital to investigate newly developed indicators or proposed quantitative methods for quality analysis and find out whether high quantity also implies high quality/significance/reputation. The role of peer review or in-depth studies in highlighting the quality based on the originality, strength, reproducibility, and relevance of the publications is additionally essential when investigating the merits of metrics in research assessment.
Keywords : conduct of research, metrics, bibliometrics, research assessment ethics, research evaluation, research quality, Responsible Research Metrics, Responsible Use of Metrics Policy
Important Note : All contributions to this Research Topic must be within the scope of the section and journal to which they are submitted, as defined in their mission statements. Frontiers reserves the right to guide an out-of-scope manuscript to a more suitable section or journal at any stage of peer review.
Topic Editors
Topic coordinators, recent articles, submission deadlines.
Submission closed.
Participating Journals
Total views.
- Demographics
No records found
total views article views downloads topic views
Top countries
Top referring sites, about frontiers research topics.
With their unique mixes of varied contributions from Original Research to Review Articles, Research Topics unify the most influential researchers, the latest key findings and historical advances in a hot research area! Find out more on how to host your own Frontiers Research Topic or contribute to one as an author.
- Help and Support
- Research Guides
Measuring Research Quality and Impact - Research Guide
- Citation Metrics
- Alternative Metrics
- Researcher Impact
- Journal Quality and Impact
- Book Quality and Impact
- University Impact
Measuring Research Quality and Impact
The activity of measuring and describing the quality and impact of academic research is increasingly important in Australia and around the world. Applications for grant funding or career advancement may require an indication of both the quantity of your research output and of the quality of your research.
Research impact measurement may be calculated using researcher specific metrics such as the h-index, or by quantitative methods such as citation counts or journal impact factors. This type of measurement is also referred to as bibliometrics.
This guide provides information on a range of bibliometrics including citation metrics, alternative metrics, researcher impact, journal quality and impact, book quality and impact, and university rankings.
Key Terms and Definitions
Altmetrics - Altmetrics (alternative metrics) are qualitative data that are complementary to traditional, citation-based metrics (bibliometrics), including citations in public policy documents, discussions on research blogs, mainstream media coverage, bookmarks on reference managers, and mentions on social media.
Author identifiers - Author identifiers are unique identifiers that distinguish individual authors from other researchers and unambiguously associate an author with their work.
Bibliometrics - Bibliometrics is the quantitative analysis of traditional academic literature, such as books, book chapters, conference papers or journal articles, to determine quality and impact.
Cited reference search - A cited reference search allows you to use appropriate library resources and citation indexes to search for works that cite a particular publication.
Citation index - A citation index is an index of citations between publications, allowing the user to easily establish which later documents cite which earlier documents.
Citation report - A citation report is a compilation of the bibliographic details for all of the publications a researcher has authored, along with the number of times those publications have been cited and any relevant author metrics.
Citation - A reference to or quotation from a publication or author, especially in a scholarly work.
CiteScore - CiteScore is a measure of an academic journal's impact and quality that analyses the number of citations received by a journal in one year to publications published in the three previous years, divided by the number of publications indexed in Scopus published in those same three years.
CNCI - The Category Normalized Citation Impact (CNCI) of a document is calculated by dividing an actual citation count by an expected citation rate for documents with the same document type, year of publication, and subject area, therefore creating an unbiased indicator of impact irrespective of age, subject focus, or document type.
FWCI - The field-weighted citation impact (FWCI) is an author metric which compares the total citations actually received by a researcher's publications to the average number of citations received by all other similar publications from the same research field.
h-index - The h-index is an author metric that attempts to measure both the productivity and citation impact of the publications of an author. A researcher with an index of h has published h papers, each of which has been cited in other papers at least h times.
Impact metrics - Impact metrics are quantitative analyses of the impact of research output, using a comprehensive set of measurement tools. Impact metrics include traditional citation metrics, altmetrics, measures of researcher impact, measures of publication quality and impact, and institutional benchmarking and ranking.
Journal rankings - Journal rankings are used to evaluate an academic journal's impact and quality. Journal rankings measure the place of a journal within its research field, the relative difficulty of being published in that journal, and the prestige associated with it.
Measures of esteem - Measures of esteem are additional factors which may provide evidence of research quality, including awards and prizes, membership of professional or academic organisations, research fellowships, patents or other commercial output, international collaborations, and successfully completed research grants or projects.
SJR - SCImago Journal Rank (SJR) is a measure of an academic journal's impact and quality that analyses both the number of citations received by a journal and the prestige of the journals in which these citations occur.
SNIP - Source Normalized Impact per Paper (SNIP) is a measure of an academic journal's impact and quality that analyses contextual citation impact by weighting citations based on the total number of citations in a subject field.
Frontiers in Research Metrics and Analytics (DOAJ) - publishes rigorously peer-reviewed research on the development, applications, and evaluation of scholarly metrics, including bibliometric, scientometric, informetric, and altmetric studies.
Metrics Toolkit (RMIT) - a resource for researchers and evaluators that provides guidance for demonstrating and evaluating claims of research impact.
Research Metrics Quick Reference (Elsevier) - a consolidated quick reference to some key research impact metrics.
Using Bibliometrics: A Guide to Evaluating Research Performance with Citation Data 2015 (Thomson Reuters) - A guide to evaluating research performance for researchers, universities and libraries.
- Meaningful Metrics : A 21st Century Librarian's Guide to Bibliometrics, Altmetrics, and Research Impact by Robin Chin Roemer & Rachel Borchardt ISBN: 9780838987568 Publication Date: 2015
- The Research Impact Handbook by Mark S. Reed Call Number: 300.72 REE 2018 ISBN: 9780993548246 Publication Date: 2018 2nd edition
Research Support
Researchers at Murdoch University are supported by the Library and the Research and Innovation Office .
Ask our Librarians for advice on measuring research quality and impact.
- Next: Citation Metrics >>
- Last Updated: Feb 28, 2024 3:25 PM
- URL: https://libguides.murdoch.edu.au/measure_research
Advertisement
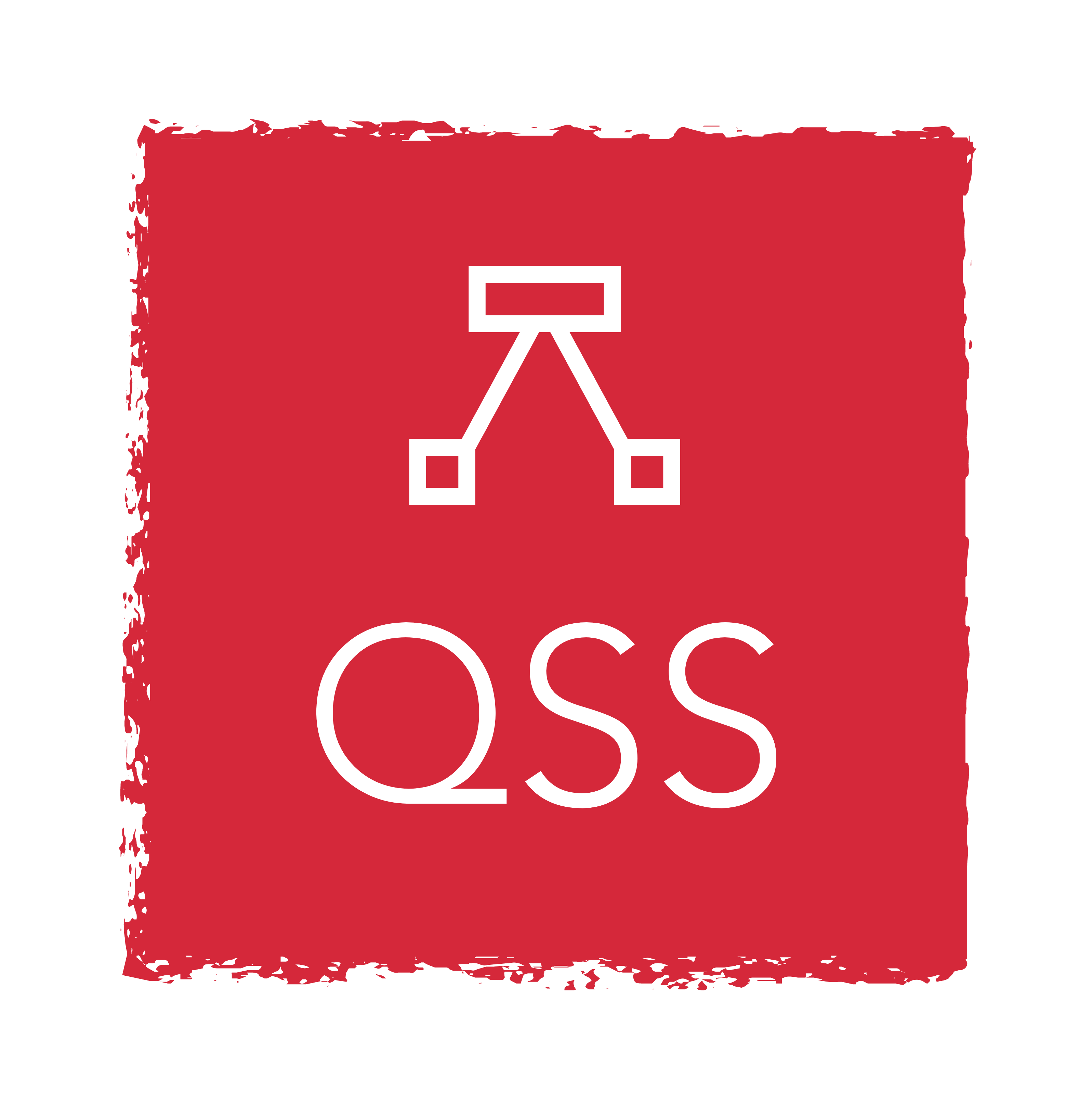
- Next Article
PEER REVIEW
1. introduction, 2. background, 5. discussion, 6. conclusion, acknowledgments, author contributions, competing interests, funding information, data availability, indicators of research quality, quantity, openness, and responsibility in institutional review, promotion, and tenure policies across seven countries.

Handling Editor: Ludo Waltman
- Funder(s): H2020 Science with and for Society
- Award Id(s): 824612
- Cite Icon Cite
- Open the PDF for in another window
- Permissions
- Article contents
- Figures & tables
- Supplementary Data
- Peer Review
- Search Site
Nancy Pontika , Thomas Klebel , Antonia Correia , Hannah Metzler , Petr Knoth , Tony Ross-Hellauer; Indicators of research quality, quantity, openness, and responsibility in institutional review, promotion, and tenure policies across seven countries. Quantitative Science Studies 2022; 3 (4): 888–911. doi: https://doi.org/10.1162/qss_a_00224
Download citation file:
- Ris (Zotero)
- Reference Manager
The need to reform research assessment processes related to career advancement at research institutions has become increasingly recognized in recent years, especially to better foster open and responsible research practices. Current assessment criteria are believed to focus too heavily on inappropriate criteria related to productivity and quantity as opposed to quality, collaborative open research practices, and the socioeconomic impact of research. Evidence of the extent of these issues is urgently needed to inform actions for reform, however. We analyze current practices as revealed by documentation on institutional review, promotion, and tenure (RPT) processes in seven countries (Austria, Brazil, Germany, India, Portugal, the United Kingdom and the United States). Through systematic coding and analysis of 143 RPT policy documents from 107 institutions for the prevalence of 17 criteria (including those related to qualitative or quantitative assessment of research, service to the institution or profession, and open and responsible research practices), we compare assessment practices across a range of international institutions to significantly broaden this evidence base. Although the prevalence of indicators varies considerably between countries, overall we find that currently open and responsible research practices are minimally rewarded and problematic practices of quantification continue to dominate.
https://publons.com/publon/10.1162/qss_a_00224
The need to reform research assessment processes related to career advancement at research institutions has become increasingly recognized in recent years, especially to better foster open and responsible research practices 1 . In particular, it is claimed that current practices focus too much on quantitative measures over qualitative measures ( Colavizza, Hrynaszkiewicz et al., 2020 ; Malsch & Tessier, 2015 ), with misuse of quantitative research metrics, including the Journal Impact Factor, among the most pressing issues for equitable research assessment generally, which aims to foster open and responsible research in particular. Therefore, recent years have seen a focus on attempts to understand how principles and practices of openness and responsibility are currently valued in the reward and incentive structures of research-performing organizations, especially by direct examination of organizations’ review, promotion, and tenure 2 (RPT) policies. Such studies have heretofore focused on specific contexts, however. Work led by Erin McKiernan and Juan Pablo Alperin examined policies in place across a range of types of institutions in the United States and Canada ( Alperin, Muñoz Nieves et al., 2019 ; Alperin, Schimanski et al., 2020 ; McKiernan, Bourne et al., 2016 ; Niles, Schimanski et al., 2020 ). Rice, Raffoul et al. (2020) studied criteria used across a range of countries, but only within biomedical sciences faculties. Hence, further work is needed to describe types of criteria in place across a range of institutional types internationally.
This paper aims to fill this gap. Our primary research question can be formulated as “What quantitative and qualitative criteria for review, promotion, and tenure are in use across research institutions in a purposive sample of seven countries internationally?” Sub-questions include “How prevalent are criteria related to open and responsible research in these contexts?,” “How prevalent are potentially problematic practices (e.g., use of publication quantity or journal impact factors)?,” and “What trends can be observed across this sample?”
To answer these questions, we investigate the prevalence of qualitative and quantitative indicators in RPT policies across seven countries: Austria, Brazil, Germany, India, Portugal, the United Kingdom and the United States. This involved manually collecting 143 RPT policy documents from 107 institutions. These documents were then systematically coded for the inclusion of language related to 17 elements 3 (including those related to qualitative or quantitative assessment of research, service to the institution or profession, and open and responsible research practices) using a predefined data-charting form. Directly comparing the indicators and criteria in place at such a range of international institutions hence aims to broaden the evidence base of the range of practices currently in place 4 .
2.1. Research Assessment and Researcher Motivations
Institutional policies regarding RPT typically focus on three broad areas: research, teaching, and service (both to the profession and the institution). The relative importance of each varies across institutions and has also changed over time ( Gardner & Veliz, 2014 ; Youn & Price, 2009 ). In the European context, a recent survey of researchers investigated indicators widely used at EU institutions for review, promotion, and tenure. The most common factors used in research assessment were (according to survey respondents): number of publications (68%), patents and securing funds (35%), teaching activities (34%), collaboration with other researchers (32%), collaboration with industry (26%), participation in scientific conferences (31%), supervision of young researchers (25%), awards (23%), and contribution to institutional visibility (17%) ( European Commission, Directorate General for Research and Innovation, 2017 ).
When it comes to the assessment of research contributions, reflecting the common idiom “publish or perish,” publication in peer-reviewed venues remains central. Primary publication types vary across disciplines. Although journal articles dominate in Science, Technology, Engineering, and Mathematics (STEM) subjects, monographs or edited collections have greater importance in the Humanities and Social Sciences ( Adler, Ewing, & Taylor, 2009 ; Alperin et al., 2020 ). Within Computer Science, meanwhile, publication in conference proceedings is the most important factor ( McGill & Settle, 2011 ). However, irrespective of which type of publication is favored, institutions tend to position productivity (often quantified via metrics) as a defining feature in RPT policies ( Gardner & Veliz, 2014 ). The ways in which this emphasis on productivity and quantification influences academics’ focus and shapes behaviors, often in detrimental ways, is worth expanding upon to understand how current trends in RPT policies may be limiting the uptake of open and responsible research.
Institutional committees tasked with determining whether research contributions are sufficient for promotion, review, or tenure face something of a dilemma. Although the quantity of publications is comparatively easy to assess, measuring their quality is a more difficult challenge. Ideally, committees would be able to read each of the contributions themselves to make their own firsthand judgments on the matter. However, the mass of material created, as well as increased research specialization drastically reducing the number of experts that possess the required expertise for such quality judgments, mean that usually proxy indicators for quality are sought. Here, two factors are particularly popular: publication venue and citation counts.
In perceptions of the prestige of academic journals, the Journal Impact Factor has assumed a particularly pernicious role. Created by Eugene Garfield of the Institute for Scientific Information, the Journal Impact Factor calculates an average of citations per article within the last 2 years to provide a metric of the relative use of academic literature at the journal level. Originally created to assist library decisions regarding journal subscriptions, the Journal Impact Factor soon came to be used as a proxy for relative journal importance by research assessors and researchers themselves ( Adler et al., 2009 ; Walker, Sykes et al., 2010 ). Various criticisms have been levelled at the Journal Impact Factor, most prominently that relatively few outlier publications with many citations skew distributions such that most publications in that journal fall far below the mean. Additional criticisms include that differences in citation practices between (and even within) fields make the Journal Impact Factor a poor tool for comparison, that it is susceptible to gaming by questionable editorial practices, and suffers a lack of transparency and reproducibility ( Fleck, 2013 ). Nonetheless, its use as a proxy for research quality in research assessment became commonplace ( Gardner & Veliz, 2014 ; McKiernan, Schimanski et al., 2019 ). McKiernan et al. (2019) studied RPT documents and found that 40% of North American research-intensive institutions mentioned the Journal Impact Factor or closely related terms. Accordingly, researchers commonly list a journal’s impact factor as a key factor they take into account when deciding where to publish ( Niles et al., 2020 ).
Citation counts at the article level are also often used as a proxy for research quality within RPT processes ( Adler et al., 2009 ; Brown, 2014 ). Indeed, Alperin et al. (2019) found that such indicators were mentioned by the vast majority of institutions. However, citations have been widely criticized for being too narrow a measure of research quality ( Curry, 2018 ; Hicks, Wouters et al., 2015 ; Wilsdon, Allen et al., 2015 ). The application of particularistic standards is especially perilous for early-career researchers who have yet to build their profile. By using citation metrics to evaluate research contributions, initial positive feedback leads to the self-reinforcement loop known as the Matthew Effect ( Wang, 2014 ). Moreover, indicators such as the h -index are highly reactive ( Fleck, 2013 ) and therefore risk reifying monopolization of resources (prestige, recognition, money) in the hands of a select elite. The h -index was designed as a measurement tool to showcase the consistency of the cited researchers but creates a disadvantage for early-career researchers and neglects the diversity of citation rates across scientific disciplines and subdisciplines ( Costas & Bordons, 2007 ).
2.2. Research Assessment and Open and Reproducible Research
Multiple initiatives in the last decade have sought to raise the alarm on the overuse of quantitative indicators and highlight the need to consider a broader range of practices (beyond publications). For instance, the San Francisco Declaration on Research Assessment (DORA) specifically criticized use of the Journal Impact Factor in research assessment 5 . The 10 principles of the Leiden Manifesto for Research Metrics ( Hicks et al., 2015 ) sought to reorient the use of metrics by critiquing their “misplaced concreteness and false precision,” arguing that quantitative should be used as a support for “qualitative, expert assessment,” with strict commitments to transparency.
Such critiques of overquantification have developed alongside movements to foster open and reproducible research. These two trends meet where advocates of Open Science or Responsible Research & Innovation (RRI) identify concern among researchers that uptake of open and responsible research practices will negatively impact their career progress ( Adler et al., 2009 ; Migheli & Ramello, 2014 ; Peekhaus & Proferes, 2015 ; Rodriguez, 2014 ; Wilsdon et al., 2015 ).
As a result, recent research has investigated if and how criteria relating to open and responsible research practices are rewarded in RPT policies. In particular, the influential “Promotion, Review, and Tenure” project headed by Erin McKiernan and Juan Pablo Alperin has examined these issues in depth by studying a corpus of RPT policy documents from 129 universities in the United States and Canada. This project found that aspects related to open and reproducible research were rare or undervalued. Alperin et al. (2019) found, for example, that only 6% of RPT policies mentioned “Open Access,” often in a negative way. Public engagement, although mentioned in a large number of policies, was nonetheless undervalued by associating it with service, rather than research work. Meanwhile, 40% of policies from research-intensive institutions mentioned the Journal Impact Factor in some way, with the overwhelming majority of those (87%) supporting its use in at least one RPT document and none heavily criticizing it ( McKiernan et al., 2019 ).
A similar study by Rice et al. (2020) studied the presence of “traditional” (e.g., publication quantity) and “nontraditional” (e.g., data-sharing) criteria used for promotion and tenure in biomedical sciences faculties. In that context, the authors found that mentions of practices associated with open research were very rare (data-sharing in just 1%, with Open Access publishing, registering research, and adherence to reporting guidelines mentioned in none). Most prevalent were traditional criteria including peer reviewed publications (95%), grant funding (67%), national or international reputation (48%), authorship order (37%), Journal Impact Factor (28%), and citations (26%).
Although general trends, including prevalence of (sometimes problematic) quantitative measures and lack of recognition for open and responsible research practices, can be observed across these two groups of work, nonetheless there are important nuances we should take into account. In the biomedical context, Rice et al. (2020) saw “notable differences” in the availability of guideline documents across continents and “subtle differences in the use of specific criteria” across countries. In the United States/Canada context, meanwhile, differences across types of institutions were observed—with, for instance, “research-intensive” institutions being more likely to encourage use of the Journal Impact Factor ( McKiernan et al., 2019 ). These differences, across institutional types and national boundaries, require further investigation.
This current study complements and extends this work. Such work is crucially important, especially as reform of rewards and recognition processes is now a policy priority, particularly in Europe. Vanguard institutions such as Utrecht University in the Netherlands are already implementing such reforms ( Woolston, 2021 ). The Paris Call on Research Assessment, announced at the Paris Open Science European Conference (organized by the French Presidency of the Council of the European Union) in February 2022, calls for evaluating the “full range of research outputs in all their diversity and evaluating them on their intrinsic merits and impact” ( Paris Call on Research Assessment, 2022 ). The Paris Call also sought the formation of a “coalition of the willing” to build consensus and momentum across institutions. The European Commission is currently building such a coalition ( Research and Innovation, 2022 ). This paper further contributes to the evidence base to inform such reform.
We assembled and qualitatively analyzed RPT policy documents from academic institutions in seven countries (Austria, Brazil, Germany, India, Portugal, the United Kingdom, and the United States).
3.1. Sampling
In selecting countries, we used purposive sampling (candidate countries whose primary language was covered by the research team (i.e., English, German, or Portuguese). Although automated translations can go a long way in basic understanding, the task at hand required knowledge of the policy landscape of the studied countries, as well as the ability for precise reading of source materials. We first identified four target countries, based on our European focus and our team’s familiarity with the language (English, German, Portuguese) and policy landscapes of specific countries. In addition, the United States was included as a representative of a leading research country and to allow comparisons with previous research ( Alperin et al., 2019 ). Furthermore, we included India and Brazil as examples of large “low- and middle-income countries” based on gross national income per capita as published by the World Bank 6 . They play a growing role in research, and broaden our scope to include Asia and South America. We acknowledge that our sample of countries cannot be considered random or representative of the situation globally. However, given the current lack of knowledge of RPT criteria in place across national contexts, we nonetheless believe that our sample adds richly to current knowledge.
To include representative numbers of institutions of perceived high and low prestige, we used the Times Higher Education World University Rankings (WUR) 2020 to select institutions. Institutions from each selected country were sorted based on their relative WUR performances in the categories “Research” and “Citations.” We then divided each category into three equally sized subcategories, defining them as “High-,” “Medium-,” and “Low-” performing institutions. Next, we calculated the median of each subcategory and selected the institutions that were closest to the median as representatives of this category. We included both the “Research” and “Citations” fields from the WUR, as both of these are research-related indicators. Duplicate entries of the same institution appearing in both categories were replaced by the next available institution in the “Citations” category. Our sampling procedure resulted in a sample of 107 institutions across seven countries ( Table 1 ).
Number of institutions per country—the total number of institutions sampled relative to the number of institutions listed per country in WUR
Although selection of institutions based on university rankings is an often-used strategy (e.g., Rice et al. (2020) use the Leiden Ranking in a similar approach), it is not without flaws. First, university rankings have been criticized for their reliance on biased and unreliable reputational survey data ( Waltman, Calero-Medina et al., 2012 ) and issues of gaming and selective reporting ( Gadd, 2021 ). Second, rankings such as the WUR only include the most prominent institutions and leave out many institutions based on partly arbitrary criteria (e.g., how many yearly publications they need to be included). The reported groups of “high,” “medium,” and “low”-ranked universities are only relative to the set of institutions included in the ranking, and not academia as a whole. We see our use of the WUR as a pragmatic approach to reproducibly sampling universities. This does not negate their deficiencies for guiding prospective students to choose institutions or informing policy decisions.
3.2. Data Collection
Policy documents were collected using a shared search protocol. First, we used Google to search for the institution name along with various constellations of keywords. Table 2 shows the set of keywords identified and used for the policies identification in the three languages: English, German, and Portuguese.
Search key terms in English, German, and Portuguese that were used to retrieve related RPT policies
We did not collect advertisements for job descriptions even though these could include some insightful requirements applicable to the RPT policies.
We included RPT policies only and not other policies such as Ethics, Diversity, and OA, where similar concepts could appear.
Policies could apply to any post-PhD researcher career stage. The collected policies evaluated various research-related positions. For example, in the United Kingdom, some institutions have separate policies for associate professors, full professors, and readers. In the United States, there are separate policies for tenured and nontenured staff. In Austria, there are policies for habilitation (qualification for teaching, needed for promotion to professor) and qualification agreements for tenure track (associate professors), but no promotion to full professor exists. In India, we could often not find specific policies, but rather the evaluation forms that researchers use to apply for promotion. In these cases, we therefore analyze the evaluation forms instead.
Some institutions have separate policies for all researcher categories, (i.e., separate policies for lecturers, assistant professors, associate professors, professors, and so on), but others have a uniform policy covering all positions. Hence, the number of institutions is smaller than the total number of policies collected ( Table 1 ). Where more than one policy was identified for an institution, we assessed the indicators separately for each policy and counted an indicator as “fulfilled” when it appeared in at least one policy.
We were sometimes unable to obtain policy documents for target institutions. Specifically, where access was restricted to members of the institution only, data collectors emailed the institution’s human resources department to ask for a copy of the policy. If no response was received within 10 days, data collectors recorded this information and sampled the next institution from the list for that country and strata until a sufficient number of policies was obtained. Table 3 shows the institutions that did not have a public policy per country.
Total number of institutions we checked with no policy
An initial round of document collection occurred during the period November 2019 to March 2020. The sample was then further extended between March and April 2021. As some institutions had several distinct policies relating to different career stages, 143 total RPT policy documents were collected for analysis from the 107 institutions.
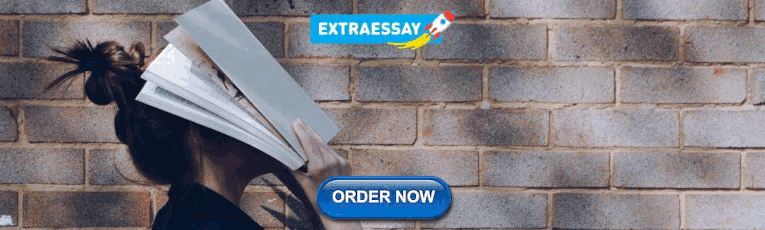
3.3. Data Charting
Data were extracted from the policy documents using a standardized data-charting form. The form was devised in multiple rounds of iteration. Key indicators for inclusion were identified from various sources, including the MoRRI indicators ( MoRRI, 2018 ) and a group of studies performed in the North American context by Alperin et al. (2019) , as well as from the surveyed literature. We collected and examined 17 different indicators ( Table 4 ), including “traditional” assessment indicators relating to quantification and quality of publications, and a set of “alternative” indicators relating to open and responsible research, and related issues such as gender equality and Citizen Science. In addition, information was gathered on the date policies came into effect, the academic positions (e.g., tenure track, professor, lecturer, senior lecturer) or types of processes (e.g., promotion, review, tenure) they governed.
Overview of data-charting form main elements (“Are the following mentioned as being taken into account in the promotion/evaluation procedures as stated in the policy?”)
Five coders were involved, all with competence in English, three in German, and one in Portuguese. Policies were assigned based on language competences. They coded the presence (1) or absence (0) of each indicator in each policy, and copied the sentence mentioning the indicator and the ones before and after. Each document was coded by one individual. To assess intercoder reliability, an independent coder (TRH) performed a reviewer audit of a random sample of 10% of the total number of institutions. Comparing this second round of review to the first responses revealed a high intercoder reliability of 96.78%.
Before carrying out the analysis, several steps were taken to ensure data integrity and consistency. Data were originally collected via spreadsheets and subsequently collated using R to avoid copy-paste errors. We checked that every indicator was present for each policy; that in cases where an indicator had been found (coded as 1) a text excerpt was present; and that in cases where no indicator had been found (coded as 0) also no text excerpt was present. The inconsistencies found were checked and resolved by TK.
To facilitate the review of our results and the reuse of the data, we translated all non-English excerpts to English in a two-step procedure. First, we used DeepL 9 to obtain an initial translation of the excerpt. A native speaker then checked the translation, revising to ensure that meaning, context, and use of special terms mirrored the original. The validated translation was then recorded alongside the original text for subsequent analysis.
3.4. Data Analysis
All data analysis was conducted using R ( R Core Team, 2021 ), with the aid of many packages from the tidyverse ( Wickham, Averick et al., 2019 ), including ggplot2 for visualizations ( Wickham, 2016 ). Computational reproducibility of the analysis is ensured through the use of the drake package ( Landau, 2018 ). The analyses presented in this paper are all exploratory and have not been preregistered. To enable the comparison of the indicators’ presence against each other, we rely on Multiple Correspondence Analysis (MCA) ( Greenacre & Nenadic, 2018 ; Nenadic & Greenacre, 2007 ). Correspondence analysis and its extension MCA are similar to principal component analysis (PCA) in mapping the relationships between variables to a high-dimensional Euclidean space. The goal of the method is then “to redefine the dimensions of the space so that the principal dimensions capture the most variance possible, allowing for lower-dimensional descriptions of the data” ( Blasius & Greenacre, 2006 , p. 5). The obtained dimensions can therefore be inspected for their alignment to specific variables, enabling conclusions about the main trends found in the data. MCA thus offers a visual representation of contingency tables and is well suited for the categorical data collected in this study. Furthermore, MCA allows us to investigate the relationship between indicators and countries jointly. Several considerations apply when analyzing data via MCA.
First, we apply MCA in a strictly exploratory fashion. Inspecting its visual output facilitates interpretation of the relationship between indicators and how they relate to countries, but we do not conduct any testing of hypotheses. Second, the graphical solution offered by MCA maximizes deviations from the average, allowing for statements of the prevalence of indicators relative to one another. For statements about absolute frequencies of indicators across countries we rely on MCA’s numerical output (see Supporting information ), as well as cell frequencies found in the corresponding contingency tables. All supporting data and required code are available via Zenodo ( Pontika, Klebel et al., 2022b ).
Assessing the prevalence of traditional and alternative (especially open/responsible research-related) criteria across policies of 107 institutions, we find substantial differences in their prevalence ( Figure 1 ). While 72% of institutions mention “service to the profession,” no institution mentions data sharing or Open Access publishing. Overall, traditional indicators, related to the profession or to scientific publications, are much more common than indicators related to open and responsible research.

Overall prevalence of indicators across all institutions/countries. In cases where an institution had more than one policy, we aggregated the policies. An institution was counted as mentioning a given indicator if at least one of the policies mentioned it.
In terms of more traditional indicators, by far the most common indicator mentioned in the policies was service to the profession, which includes activities such as organizing conferences or mentoring PhDs (72%). Extending the concept of professional service, almost half of the policies also mention peer review & editorial activities (47%). A second important aspect among the sampled policies is that of scientific publications, with frequent mentions of the number of publications, or publication quality. Although a call to rate quality over quantity is not uncommon in the policies, problematic practices were still worryingly prevalent. For example, journal metrics such as the Journal Impact Factor were mentioned in at least a quarter of the policies, while sheer productivity, as measured by quantity of publications, was present in around a fifth of cases.
Indicators relating to open and responsible research were very rare. We discovered no mentions of data sharing or Open Access publishing. Creation of software was quite well represented (13% of cases), due to its prevalence in policies in Brazil, where it is mentioned at 75% of institutions ( Figure 3 ; see Section 4.2 ). Mentions of RRI elements were more encouraging, as the RRI-related aspects of interactions with industry (37%), engagement with the public (35%), and engagement with policy makers (22%) were relatively well represented. However, issues relating to gender were mentioned only in 6–9% of cases.
4.1. Relationship Between Indicators
Institutions rely on a distinct combination of indicators and criteria to assess researchers. To investigate how these indicators are related (i.e., which aspects are commonly mentioned in tandem), we rely on MCA. This method relates criteria against each other and allows us to investigate deviations from the average, as well as which indicators commonly appear together. To further substantiate these findings, we provide bivariate correlations between all indicators in the Supporting information ( Figure S2 ). Note that these analyses are exploratory and based on a data set of moderate size.
The first apparent aspect from analyzing the variables jointly is that the studied indicators tend to be cumulative ( Figure 2 ). In broad terms, there is a basic divide between institutions that mention many criteria and those that mention few to none (see also Table 5 on how this relates to countries). Investigating relationships further, the first dimension (horizontal axis) draws heavily from engagement beyond academia: with industry, the public, and policy makers. Institutions commonly mention them together, with bivariate correlations of about .5 between the three indicators (see Figure S2 ). The same institutions also mention contributions to review & editorial activities, service to the profession, and publication quality more often than the average institution. On the other end of the spectrum (righthand side) are institutions that mention engagement beyond academia and service to the profession less frequently than the average.

Relationship between indicators for review, promotion, and tenure. The figure is a graphical representation of the relationships between indicators when considering their multivariate relationships. The figure’s origin (0, 0) represents the sample average. “++” means that an indicator is present, “—” that it is not present. Engagement is abbreviated with “E.” The horizontal axis (Dimension 1) accounts for 66.7% of variation in the data. This dimension mainly contrasts institutions that mention instances of engagement (with the public, industry, or policy makers) and citizen science, as well as service to the profession, with institutions that do neither. The vertical axis (Dimension 2) accounts for 8.4% of variation in the data. This dimension mainly contrasts institutions that value publication quality and rely on citations with institutions that value patents and journal metrics, as well as software, on the other end of the spectrum (bottom). Citizen science is found near the bottom of the axis but does not contribute strongly to this dimension. The indicators “Data sharing” and “Open Access publishing” are not included in the model, as both were not found in any of the policies. Furthermore, variables relating to gender were not included, because they relate to the composition of review panels rather than research assessment criteria per se.
Number and percentage of indicators discovered per country
Although the first distinction (between institutions mentioning many indicators and engagement beyond academia in particular) is strongest, the second dimension (vertical) provides additional insight on the interrelatedness of the indicators. The divergence along this dimension revolves around institutions that mention publication quality and reliance on citations on one side, with institutions mentioning software, patents, and journal metrics, as well as citizen science, on the other side. It is noteworthy that publication quality and citations (often seen as a proxy indicator for publication quality), are mentioned jointly at an above average rate. Mentions of publication quality, on the other hand, are unrelated to mentions of journal metrics (such as the Journal Impact Factor, r = −0.01, 95% basic bootstrap CI [−0.21, 0.17]), which are considered a much more problematic indicator of research quality. Citations and journal metrics are represented on opposite sides of the vertical spectrum, despite the criteria being weakly correlated ( r = .22, [0.02, 0.44]). This is driven by the fact that journal metrics are moderately related to patents ( r = .32, [0.14, 0.52]), but unrelated to publication quality. It should be noted that the concepts of “journal metrics” and “publication quality” might overlap in how they are applied in practice. While we coded text phrases such as “High quality scholarly outputs with significant authorship contributions” as pertaining to publication quality, this might in practice be assessed via journal metrics (e.g., Journal Impact Factor or ranking quartiles).
4.2. Country Comparison
When comparing countries, we find differences in terms of the overall prevalence of indicators, but also their relative importance. The absolute number of indicators per country varies because we sampled more institutions for larger countries than for smaller ones. Importantly, however, the relative number of indicators also varies considerably ( Table 5 ). Although just under a third of the analyzed indicators were identified in policies in Austria, Brazil, Germany, Portugal and the United Kingdom, the figures were 18% for the United States and 16% for India. The lower numbers in the United States and India may reflect the nature of the documents examined in those cases. We only examined institution-wide policies and in the United States it may be the case that detailed criteria are more often contained at departmental or faculty-level policies; in India (as stated) assessment forms were also analyzed, as few institutions had official policy documents (see Table 3 ).
Austria: A very high share of sampled institutions mention the number of publications (67%), and half also mention journal metrics. Service to the profession, while the most common concept across countries, is mentioned in only 50% of institutions in Austria. A major distinction between institutions from German-speaking countries (i.e., Austria and Germany) and all other countries is that the former frequently mention concepts of gender, with four out of six Austrian universities mentioning gender equality, while this is not found in any other country.
Brazil: All Brazilian institutions mention service to the profession, and three out of four mention patents, review & editorial activities, and software, while mentions of software are uncommon in other countries. Similar to India and Austria, journal metrics are mentioned quite frequently (42%). Sampled policies from Brazil are similar to policies from the United Kingdom in frequently mentioning service and engagement beyond academia but diametrically opposed in also frequently mentioning patents and software, both of which are very rare in the United Kingdom. Finally, both country profiles are relatively far from the sample average, indicating configurations that are less common among other countries.
Germany: Policies from German universities are very similar to their Austrian counterparts, which suggests similarities based on shared cultural and academic traditions and influences. For example, policies from both Austria and Germany commonly mention gender equity. However, the concepts of patenting and review & editorial activities appear considerably more frequently in German policies than in Austria, with the fewest mentions of service to the profession across the sample also found in Germany.
India: Contrary to all other countries, we find no evidence of policies referring to review & editorial activities as a criterion for promotion, and very few cases that refer to the number of publications that a given researcher has produced. On the other hand, mentions of journal metrics were very common in the policies sampled from Indian institutions (67%, n = 8), while less common among the other countries.
Portugal: All sampled universities mention engagement with the public, which is a strong exception in the sample. Furthermore, many institutional policies mention service to the profession, engagement with industry, patents, as well as the number of publications. Indicators that are less common across the sample, such as citizen science, software, and citations, as well as engagement with policy makers, are not found at all in Portugal.
United Kingdom: All sampled universities mention service to the profession, and four-fifths mention publication quality. Equally, institutions from the UK mention all three dimensions of engagement beyond academia (industry, public, policy makers) considerably more frequently than the average of the sample. Finally, policies mention patents and the number of publications considerably less frequently than institutions from other countries.
United States: In line with the overall finding of a low propensity of indicators across universities from the United States ( Table 5 ), all indicators were found at a slightly lower rate than in other countries. Given that the sample deliberately included more institutions from the United States, institutional policies from the United States are quite close to the average across the whole sample ( Figure 4 ). The biggest deviations from the sample average are found with engagement with industry, which is mentioned least frequently in the United States compared with all other countries.

Prevalence of indicators per country. Percentages are rounded to full integers. The number of cases (universities) per country is presented in Table 5 . Low cell frequencies and empty cells prohibit the use of common chi-square metrics for contingency tables.

Relationship between indicators with superimposed countries. The relationships between criteria displayed in this figure are the same as in Figure 3 . To allow for an investigation of which criteria are more common in a given country than in the rest of the sample, we project country profiles into this space. These “supplementary variables” do not have an influence on the layout of the indicators. The countries’ positions are to be interpreted as projections onto the respective axes by examining their distance to indicators that are central to the respective dimension (see Section 3.1 and Figure 3 for the interpretation of the axes).
Overall, we find a low uptake of alternative evaluation criteria covering open and responsible research. However, there is substantial variation between countries ( Figure 5 ). Summarizing eight core criteria (“Citizen science,” “Data,” “Engagement with industry,” “Engagement with policy makers,” “Engagement with the public,” “Gender equality,” “Open Access,” “Software”), we find the highest uptake of alternative criteria in Brazil, Portugal, and the United Kingdom, with 1.9, 1.8, and 1.8 alternative criteria per university on average. Uptake is lower in Austria and Germany, and particularly low in the United States (0.7 criteria on average) and India (0.3).

Uptake of alternative indicators. Here we display how frequently alternative indicators are found in the policies. We consider the following eight indicators: “Citizen science,” “Data,” “Engagement with industry,” “Engagement with policy makers,” “Engagement with the public,” “Gender equality,” “Open Access,” “Software”. Dots represent the mean across all universities of a given country, with bootstrapped confidence intervals (95%).
4.3. Comparison with Citation Ranking
Previous research has found no evidence of an association between universities’ ranking positions and the prevalence of traditional or alternative criteria when controlling for geographic region ( Rice et al., 2020 ). Here we conduct a similar analysis, examining differences in the citation ranking and its relationship to the set of criteria, while controlling for country. Removing the influence of countries is meaningful in this context, because an institution’s location and its citation ranking are clearly linked ( Figure S4 ).
After controlling for country, we find only small differences in the prevalence of indicators with respect to an institution’s ranking ( Figure 6 ). Institutions with a low as well as with a medium citation ranking are very close to the sample average on both dimensions. Both are characterized by slightly above-average mentions of the dimension of engagement beyond academia, as well as the dimension of service. Highly ranked institutions are characterized by slightly lower than average mentions of service, review & editorial activities, and engagement, but slightly higher mentions of publication quality, citations, and journal metrics (see also Figure S5 ).

Relationship between indicators with superimposed citation ranking groups. The relationships between criteria displayed in this figure are the same as in Figure 3 . To allow for an investigation of which criteria are more common in a given ranking group than in the rest of the sample, we project the respective profiles into this space. These “supplementary variables” do not have an influence on the layout of the indicators. Ranking categories are calculated within-country to control for the influence of country on an institution’s citation ranking. The ranking positions are to be interpreted as projections onto the respective axes, by examining their distance to indicators which are central to the respective dimension (see Section 3.1 and Figure 3 for the interpretation of the axes).
4.4. “Numbers Help”? Journal Metrics, Publication Quantities, and Publication Quality
We next look further into the ways in which two problematic practices (use of journal-level metrics and numbers of publications as indicators of quality and productivity respectively) are expressed in policies, as well as how policies discuss publication quality.
More than a quarter of the policies we examined mention the Journal Impact Factor or some other measure of journal/venue prestige as an assumed proxy for the quality of research published there. This was highest in India (67%) and Austria (50%). In the latter, unambiguous use of the Journal Impact Factor was found: “The evaluation is based on the journal rankings according to the impact factors from the unchanged ranking lists of the Institute of Scientific Information (ISI)” (Medical University of Vienna, AT_4a). Brazil (42%) also relied heavily on journal-level metrics, specifically the “QUALIS-CAPES classification,” the Brazilian official system of journal classification ( Pinto, Matias, & Moreiro González, 2016 ). Use of such metrics was least visible in the United Kingdom, where the 14% of policies that mention them also tend to be more circumspect in their language (e.g., “Excellence might be evidenced […] (in part) by proxies such as journal impact factors” (Teesside University, GB_6)).
Numbers of publications as a criterion are present in around one in five policies, invoked in various ways. This criterion is especially common in Austria (67%), (e.g., “The list of publications of a habilitation candidate must include at least 16 scientific publications in international relevant journals with peer review procedures, which have been published in the last 12 years,” Medical University of Innsbruck, AT_3). Such quantification is sometimes used in the contexts of strict formulas that also used journal-level metrics (as at the aforementioned Medical University of Vienna (AT_4a): “The basic requirement for a habilitation is 14 points, with 1 point for a standard paper and 2 points for a top paper”). In the United States, quantity of publications is mentioned in 17% of cases, but usually emphasized as just one factor amongst others (e.g., “Quantity can be a consideration but quality must be the primary one” (University of Missouri-St Louis, USA_25)). In the striking words of one US institution, however, “ numbers help ” [emphasis ours] when reporting “the total number of peer-review articles or other creative and research outputs” (University of Nevada, Las Vegas, USA_31). In Germany, only 25% of institutions focused on publication numbers, but often emphasized “not to set a fixed minimum number, but rather an approximate guideline” (TU Dortmund, DE_12). However, in Austria and Germany we also found that as a matter of course many institutions ask for full publication lists as part of their criteria. We did not code these as explicitly supporting publication quantity as an indicator for assessment. However, in practice we might assume that the length of publication lists may be used as an unofficial factor in decisions.
As with journal metrics, UK policies only very rarely mention publication numbers as a factor (just 4%). This is in stark contrast to the number of UK policies mentioning publication quality as an important criterion (79%). Here, the influence of initiatives such as DORA and the United Kingdom’s Forum for Responsible Metrics, and the way these have translated into the UK national assessment exercise, the Research Excellence Framework (REF), is clearly visible. We found, for example, exhortations to produce “high quality” work “that is judged through peer review as being internationally excellent or better in terms of originality, significance and rigour” (University of Sheffield, GB_9a). As we discuss below, this language is highly similar to that of the REF itself, suggesting that institutions have adapted their assessment policies to REF criteria.
The need to reform reward and recognition structures for researchers to mitigate effects of overquantification and incentivize uptake of open and responsible research practices is well understood. Our results show just how far there is to go.
We found that policies for assessing researchers for review, promotion, and tenure among an international sample of 107 institutions in seven countries largely relied on traditional criteria (service to professions, review & editorial activities, publication quality, and patents). Alternative criteria related to open and responsible practices were much less prevalent. Here, considerations related to Responsible Research and Innovation, such as engagement with industry, the public, and policy makers fared better, present in between 22% and 37% of policies. Gender elements (including commitments to gender equity and gender balance of reviewers) were only present in between 6% and 9% of cases, and only found in Austria and Germany. Criteria related to Open Science were very rare—sharing of data and Open Access publishing were not found in any policy. These general findings across countries hence largely confirm previous findings, which have focused on US/Canadian institutions ( Alperin et al., 2019 ; Alperin et al., 2020 ; McKiernan et al., 2019 ; Niles et al., 2020 ) or a particular discipline ( Rice et al., 2020 ).
Regarding differences between the countries studied, we found substantial differences with some common patterns. Overall, we found very few criteria in the policies from India and the United States. India likely constitutes a special case, with institutions often seeming to lack written policies beyond those implied by the required criteria in application forms. For the case of the United States, the distinction between general RPT policies and those from specific departments and schools is crucial. The analyzed policies represented general policies, which in many cases laid out general principles but did not include more specific criteria, which were to be defined by each school or department. This is in contrast to policies from Austria or Germany, where the policies applied equally university-wide; however these policies were very specific and we did not find evidence of further policies at faculty or department level.
Criteria relating to engagement beyond academia (industry, policy makers, public) appeared together very often (correlations around r = 0.5), and most commonly in Brazil, Portugal, and the United Kingdom. The high share of UK institutions mentioning this type of outreach can be related to the influence of the REF as an organizing principle. Twenty-five per cent of the profile for an institutional score in the REF is attributed to “Impact,” defined as “an effect, change or benefit beyond academia” ( Sutton, 2020 ), and the prevalence of broader impact criteria in current institutional policies seems to reflect this importance. Similarly, the effect of the REF on definitions of quality of outputs and the diminished use of journal metrics as a proxy for quality can clearly be seen in the United Kingdom, whereas journal metrics were barely mentioned and many policies focused on the quality of publications themselves were foregrounded, sometimes literally using the REF definition of “Quality of research in terms of originality, significance and rigour,” as in the case of (University of Sheffield, GB_9a) quoted above.
There are high similarities between the United Kingdom, Portugal, and Brazil, with a strong emphasis on service, review & editorial activities, and the dimension of engagement beyond academia. However, the United Kingdom is very distinct from Portugal and Brazil in terms of patents and publication quality. While patents are very frequently mentioned in Brazil and Portugal, but almost not at all in the United Kingdom, publication quality was found very often in the United Kingdom but not at all in Brazil, and only in one out of six universities in Portugal.
Relationships between the presence or absence of specific indicators and an institution’s relative level of “prestige” (imperfectly captured here via their citation ranking) are weak. Our findings align with results by Rice et al. (2020) , who reported statistically insignificant coefficients for universities’ ranking positions on the uptake of traditional and alternative indicators, after controlling for country. Institutional policies explicitly rewarding high levels of citations or publications in journals with a high Journal Impact Factor do not seem to translate easily to an institution’s increased success in this regard. Hence, not only are such policies problematic in incentivizing gaming of metrics and potentially fostering bad practices ( Higginson & Munafò, 2016 ; Ioannidis, 2005 ), but they do not even necessarily work as desired to raise an institution’s position in such rankings.
In addition, that the prestige of institutions is less of a factor than we may have expected spotlights the extent to which local or regional norms of research assessment must be further studied. We suggest that a main takeaway from our findings is that although the overall trends (predominance of traditional and quantitative indicators and general lack of newer metrics of open and reproducible practices) are visible across the countries, substantial differences in the emphasis on specific sets of criteria exist. This has implications for reform of reward and recognition.
Current RPT policies result from a complex network of factors, including diverging evaluation cultures, differing levels of institutional autonomy, and institutional preferences ( Adler et al., 2009 ; Brown, 2014 ; Coonin & Younce, 2009 ; Gardner & Veliz, 2014 ; King, Acord, & Earl-Novell, 2010 ; McGill & Settle, 2011 ; Seipel, 2003 ; Walker et al., 2010 ). The best route forward on reforming these local assessment cultures is thus to be decided in light of historical and contextual considerations. Strict, one-size-fits-all reforms would be insufficient in bringing about desired outcomes across the complex web of differing evaluation cultures.
In addition, open and responsible research practices are still moving into the mainstream, and at different rates in differing countries, regions, and types of institutions. Factors such as levels of resources mean local contexts will have different levels of preparedness to adopt open and reproducible research practices. Because, we assert, it would be unfair for an institution to expect these practices before being able to adequately support their implementation (through training, services, and infrastructure), it is essential that reforms are built upon adequate institutional foundations for performing open and reproducible research ( Ross-Hellauer, Reichmann et al., 2022 ). In addition, particular open and responsible practices are of different relevance across disciplines, and hence reform must respect disciplinary cultures. Indeed, two recent surveys of research institutions by the European University Association highlight several barriers to change in research assessment from the institutional point-of-view ( Morais & Borrell-Damian, 2018 ; Saenen, Morais et al., 2019 ). Primary among these is the sheer complexity of the issue, which (as already stated) must account for differences in disciplines and career stage but also the various levels at which rewards and incentives can be structured, such as the level of research groups, departments, faculties, and institutions, as well as (cross-)national actors such as governments and research funders. Other factors identified by the EUA survey include lack of capacity, need to align policies with national or international agendas, resistance to reform from researchers or management, worries about increased costs, and lack of evidence on benefits ( Morais & Borrell-Damian, 2018 ; Saenen et al., 2019 ).
Current assessment of research and researchers forms a major barrier to the uptake of open and reproducible research, retaining too much focus on inappropriate indicators, productivity as determined by quantity, and individual achievements rather than collaborative open research practices, and the socioeconomic impact of research. In this paper, we have quantitatively demonstrated that across countries, inclusion of such criteria remains rare. Although outreach to stakeholders beyond academia (public, industry, policy makers) is somewhat better represented, practices related to Open Science are certainly not. Our sample is unique in addressing many countries across disciplines, and as such demonstrates that although general trends of overquantification and undervaluing of open and responsible research can be observed, important differences between countries can be seen. In seeking reform, care must be taken to respect the historical and contextual reasons for such divergences.
6.1. Limitations and Future Work
The collection of policies was conducted under time constraints and in two rounds (1 year apart). In addition, obtaining policies was difficult as they were often internal documents. Hence, there may have been some time lag in that the policies we examined were not the most recent versions.
The search protocol for the construction of the sample implied looking for the general RPT policy for the whole institution. This left out departmental policies and other policies such as Ethics, Diversity, and Open Access. In the Portuguese case, most universities have separate Open Access policies that in some cases are tied with promotion criteria, and therefore were not included in our analysis. The focus on general policies might also explain the overall low rate of policies found for the United States.
All analyses presented in this paper are of exploratory nature. Our paper strives to explore the landscape of RPT policies to gather initial evidence on the prevalence of specific concepts. Future studies that focus on particular aspects and analyze them with a highly targeted approach (with preregistered hypotheses of particular relationships) would be a meaningful extension of our work.
This work only identifies the prevalence of concepts in documents. It does not further analyze the particular contexts of use. Future qualitative work would provide this. In addition, this work does not consider how these policies were actually put into practice, and future survey or interview work would help in understanding these broader contexts. As an example, many policies mention that candidates should submit a full CV, including a complete list of publications. We did not consider these to be instances that quantify research output (indicator “Number of publications”). However, it is reasonable to assume that all requested materials will be considered to some extent, and that longer publication lists might be helpful.
A further factor to consider is, of course, the degree to which policies actually guide practice in review, promotion, and tenure evaluations. As one of our anonymous reviewers astutely notes, some studies (e.g., Langfeldt, 2001 ) indicate that reviewers are often highly selective in adhering to such criteria. If, for example, the removal of explicit reference to Journal Impact Factors is not also followed by a cultural change whereby assessors are educated about the reasons they are not a good indicator of individual performance, then such factors may continue to play a role, even unofficially. Future work may build upon existing work (e.g., Hammarfelt, 2017 ; Hammarfelt & Rushforth, 2017 ) to further explore how criteria are implemented and weighted across disciplines.
Future work could also try to collect a larger number of RPT policies from a broader variety of geographical areas that are not included in our research. A greater sample would enable enhanced understanding of local contexts. Indeed, randomized sampling of a much broader range of countries might enable stronger claims about the state of criteria in use for RPT globally.
The authors gratefully thank the following for their contribution to the paper: Helene Brinken and Anja Rainer for assistance with data collection, and Bikash Gyawali and David Pride for assistance with data investigation. In addition, we kindly thank our two anonymous reviewers and handling editor Ludo Waltman for their challenging and critical comments.
Nancy Pontika: Conceptualization, Data curation, Investigation, Methodology, Project administration, Writing—Original draft, Writing—Review & editing. Thomas Klebel: Conceptualization, Data curation, Formal analysis, Investigation, Methodology, Validation, Visualization, Writing—Original draft, Writing—Review & editing. Antonia Correia: Data curation, Investigation, Writing—Review & editing. Hannah Metzler: Conceptualization, Data curation, Investigation, Methodology, Project administration, Writing—Review & editing. Petr Knoth: Conceptualization, Funding acquisition, Methodology, Project administration, Supervision, Writing—Review & editing. Tony Ross-Hellauer: Conceptualization, Funding acquisition, Investigation, Methodology, Project administration, Supervision, Validation, Writing—Original draft, Writing—Review & editing.
The authors have no competing interests.
This work was supported by the project “ON-MERRIT,” funded by the European Commission under the Horizon 2020 program (grant number 824612).
All supporting data and required code are available in Zenodo ( Pontika et al., 2022b ).
We use this broad term in this paper to denote the confluence of two sometimes overlapping trends in research reform. Open Science is “the movement to make scientific research, data and dissemination accessible to all levels of an inquiring society” including Open Access to publications, data-sharing and increased collaboration in research ( Pontika, Knoth et al., 2015 ). Responsible Research and Innovation is an umbrella concept, dominant in Europe, which denotes research that is made responsive to society through practices such as public engagement, open access, gender equality, science education, ethics, and governance ( Owen, Macnaghten, & Stilgoe, 2012 ; von Schomberg, 2019 ).
The systems of promotion and tenure differ across these countries. While tenure and procedures to award it are central in the United States, German-speaking countries still largely rely on a “chair system”, with a stark division between full professors and nonprofessorial staff ( Brechelmacher, Park et al., 2015 ). Here, we analyze all assessment processes for progression in academic careers, including all variants of tenure.
We initially coded the additional indicator “general impact,” but discarded it for the analysis as it largely reflected an aggregate measure of other indicators on impact beyond academia (public, industry, policy-making).
Results from this study were previously made available via the ON-MERRIT project report “D6.1 Investigating Institutional Structures of Reward & Recognition in Open Science & RRI” ( Pontika, Klebel et al., 2021 ). This paper presents enhanced analysis based on a slightly modified data set (corrected to eliminate minor inconsistencies in data charting, as explained in footnote 7 , Section 3.3 ). In addition, the data underlying this study are also incorporated into the data paper ( Pontika, Gyawali et al., 2022a ).
San Francisco Declaration of Research Assessment: https://sfdora.org/ .
World Bank: https://www.worldbank.org/en/home .
For the Indian case, most institutions did not have specific policies. To retain India in the sample, we therefore coded five evaluation forms, two policy documents and five documents that included both a policy and an evaluation form.
The indicator was initially broader in scope, also covering cases mentioning a full list of publications as a mandatory part of the documents to be submitted. As we elaborate in the discussion, these requests might imply that the number of publications is being considered, but this is not evident from the policy itself. We therefore revised the indicator, referring strictly to instances where the number of publications was taken as an indicator of productivity in and of itself (e.g., “numbers help,” University of Nevada, Las Vegas, USA_31) and recoded documents accordingly.
https://www.deepl.com/translator .
Author notes
Supplementary data, email alerts, related articles, affiliations.
- Online ISSN 2641-3337
A product of The MIT Press
Mit press direct.
- About MIT Press Direct
Information
- Accessibility
- For Authors
- For Customers
- For Librarians
- Direct to Open
- Open Access
- Media Inquiries
- Rights and Permissions
- For Advertisers
- About the MIT Press
- The MIT Press Reader
- MIT Press Blog
- Seasonal Catalogs
- MIT Press Home
- Give to the MIT Press
- Direct Service Desk
- Terms of Use
- Privacy Statement
- Crossref Member
- COUNTER Member
- The MIT Press colophon is registered in the U.S. Patent and Trademark Office
This Feature Is Available To Subscribers Only
Sign In or Create an Account
Special Education Resource Project
Quality matters – research design, magnitude and effect sizes.
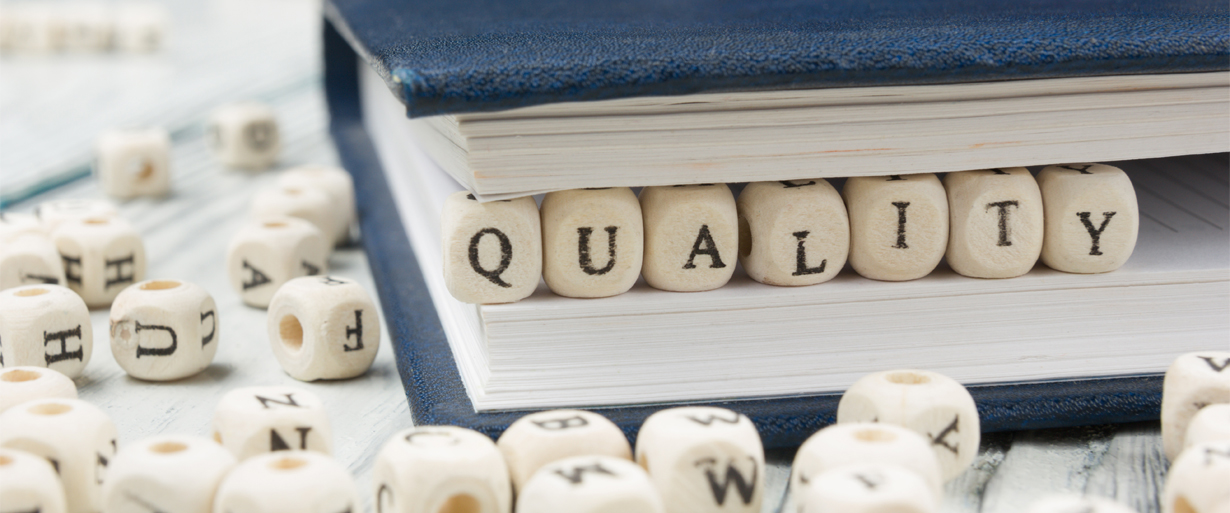
The keystone of evidence-based practices (EPBs) is research. Through research, we are able to create a solid foundation for which an EPB can stand on. However, not all research is created equal. There are studies that are the cornerstone for other researchers, studies of such high-quality that they can be cited and reference with not qualms. Then there is research that is high-quality but may not meet all of the criteria for a EBP. Then there are studies that are missing key elements of a quality study and while they do show effects, those effects may be impacted by reliability. validity or implementation problems. Then there are the studies that are not high-quality. These studies leave many of the essential elements of a high-quality out and therefore the results cannot be validated.
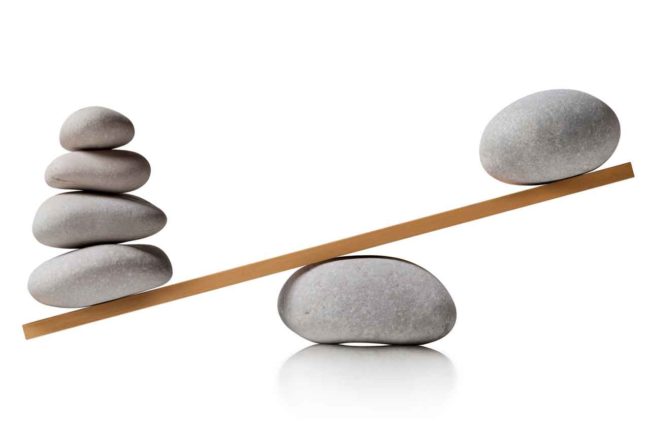
The Council for Exceptional Children has developed quality indicators to help identify studies that examine interventions and their effects on children with disabilities. These indicators look to examine if a study is methodologically sound. This is another term used to describe the quality of research.
To access The Council for Exceptional Children’s Research Quality Indicators, click here.
Here is a simplified chart of the CEC quality indicators. We will revisit these indicators later to see what is required of studies to impact the status of evidence-based practices.
CEC Quality Indicators
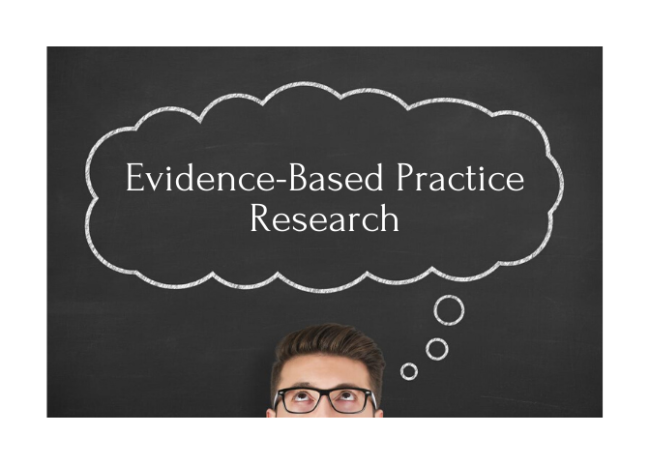
(Please note that all graphs and infographics are original.)
Experimental Designs
One of the key quality feature of EBP research is that studies employ an experimental design. An experimental design looks to compare results from two different groups to determine if an intervention was effective or not. The participants in these designs are either assigned to the treatment groups (getting the intervention) or to the control group (business as usual). Business as usual refers to students receiving their normal instruction in the classroom with no change applied. All of the participants have the same key features, for example all the participants are in the 2nd grade and have been identified as having a math disability. The participants are given the same pre-test assessments and the same post-test assessments on the same time schedule. Since one group received the treatment and one did not, the effects that the intervention has on participants can confidently be determined for the interventions effectiveness. Experimental designs are also quantitative which means the data can be measured and interpreted through different statistical manipulations.
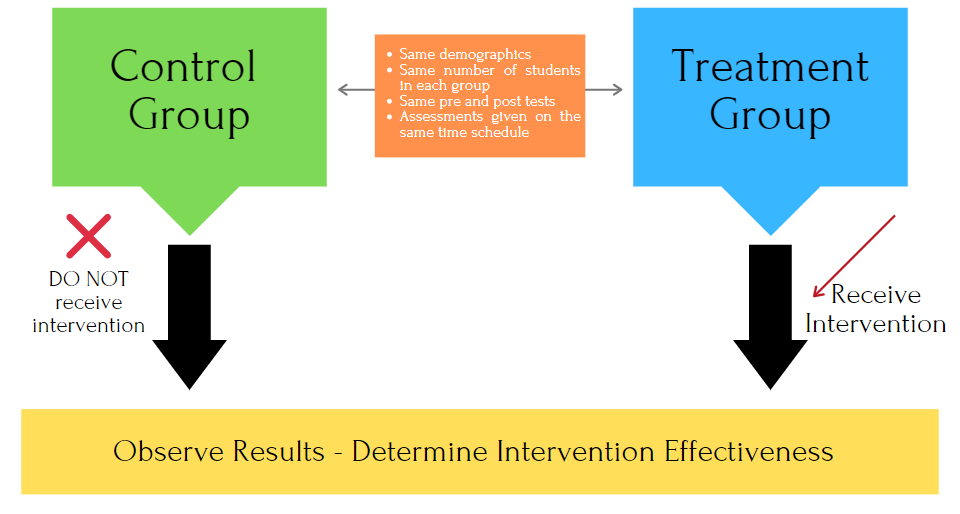
Quasi-Experimental Designs
There are studies that are quasi-experimental. These studies do not have randomization of their groups. They still have the same participants as pre and post assessments but their is no “business as usual” group. These studies look to see which of two interventions were more impactful on student outcomes. For example, a group of 3rd graders may be receiving two different reading interventions. One looks at phonics instruction only and the other at fluency only. The two groups receive the same intervention over the same number of weeks and at the end of intervention treatment, post tests are given. Based off the results, researchers can determine which intervention was more impactful.
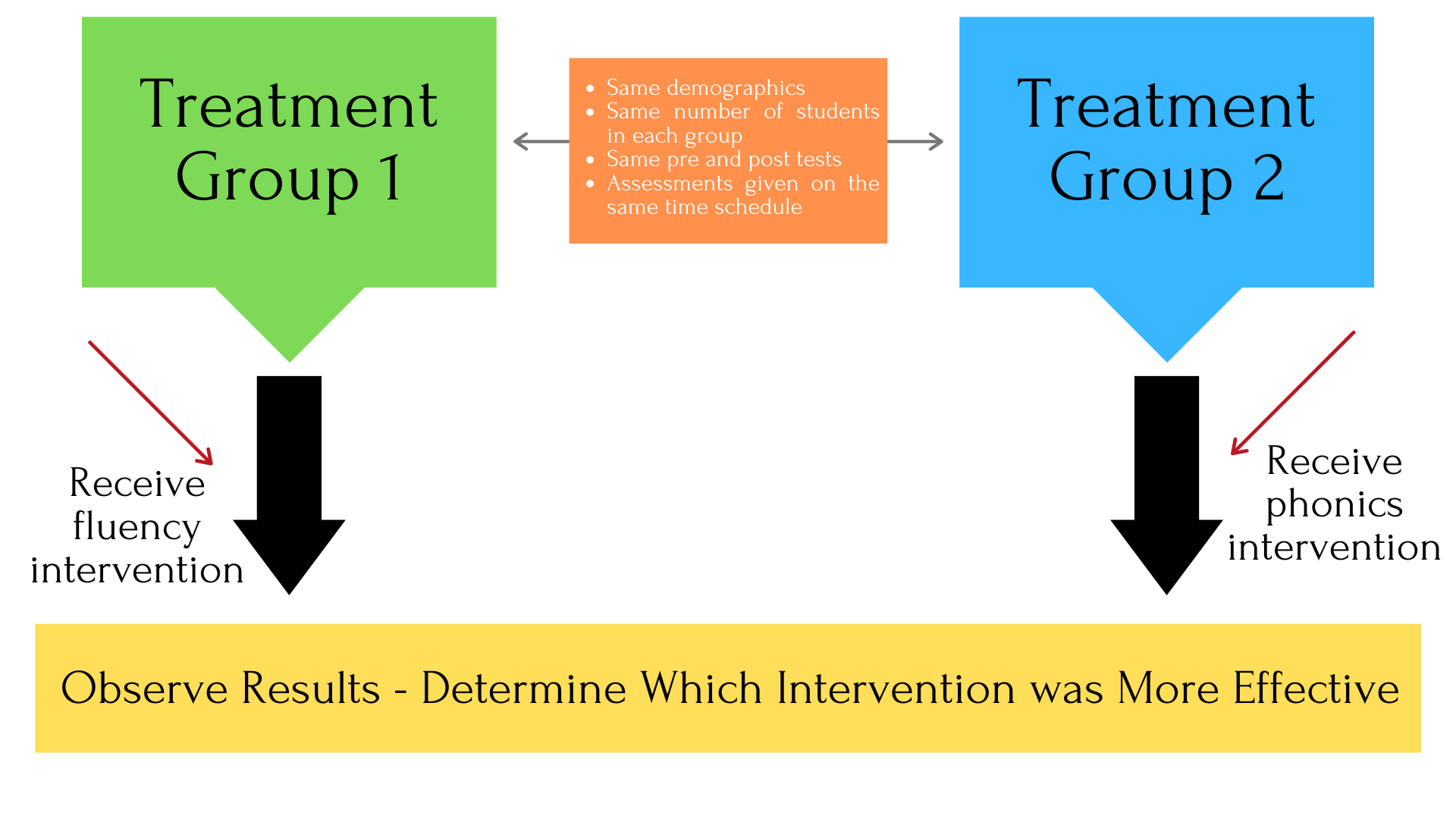
Single-Subject Designs
Single-subject designs are just as the name suggests…a single subject. This design type looks to examine the effects of an intervention on a single subject but the intervention could be looking at its effects on different behaviors or skills. You could have the same intervention (single-subject) across participants which looks at the interventions effects across participants of the same age, level or skill set. You could also have a single-subject designs that looks at alternating treatments and their effects on a participant. All of these different single subject designs demonstrate a interventions effects. Note that single-case design data looks most like data that teachers collect in their own classrooms.
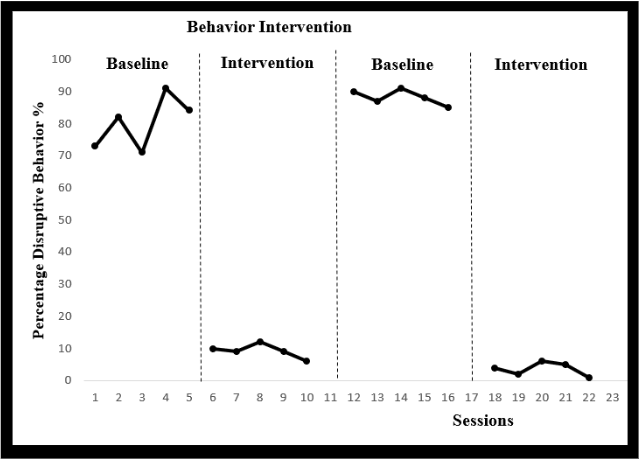
Qualitative Designs
Qualitative designs are different from quantitative in that the data is not measurable. Qualitative studies involve things like interviews, surveys and anecdotal information. These studies look at things like teachers’ perceptions of the learning environment, survey information about why a teacher left the classroom or descriptive information like effective teachers have these qualities. While the information from qualitative studies can be used for determining an evidence-based practice, they cannot be the only type of research in the body of evidence. This is because in these studies, there is no measurable data to compare and intervention and it’s effects on students or teachers.
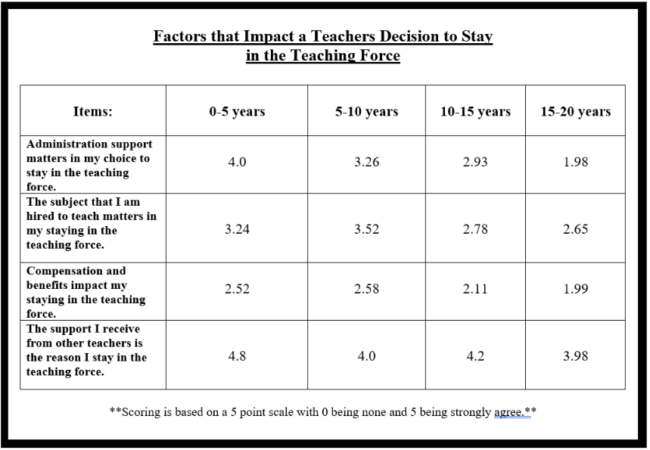
What is magnitude and what are effect sizes? Magnitude refers to the amount of studies that show a strong, positive cause-and-effect relationship between an intervention and improved academic or behavioral outcomes. Basically the more studies you have that meet the quality indicators, the larger the magnitude of the effects of the intervention. A intervention with 3 quality studies versus an intervention with 13 studies means that the intervention with 13 studies has a greater magnitude for effectiveness.
Magnitude is also applied to effect sizes. Effect sizes report statistical information about how impactful an intervention was on student performance. For example an effect size of .15 demonstrates that there was some effectiveness to the intervention but not enough to be statistically significant. However an effect size of 1.5 shows a greater amount of effectiveness of the intervention on student outcomes. Rule of thumb for effect sizes is the closer you get to 1.0, the more statistically significant the effects. Oh and remember too that the closer to zero or negative numbers show a negative outcome for the intervention on student outcomes. The larger the effect size, the greater the magnitude of its impact.
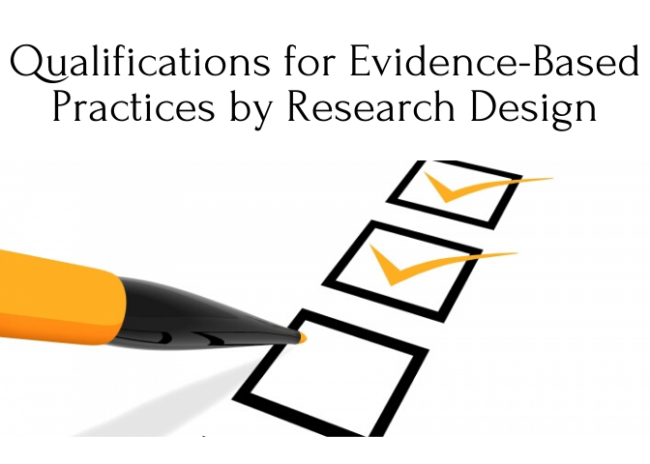
So what do these research designs have to do with a practice being evidence-based? Based on the rigor and quality of each of the design types, there is a minimum numbers of quality studies needed to identify a practice or intervention as evidence-based.
Here is the general guidelines that are used in special education:
General Guidelines for Evidence -Based Practices in Special Education
Now that we have looked at design types, magnitude and effect sizes, let’s look at some resources for selecting Evidence-Based Practices.
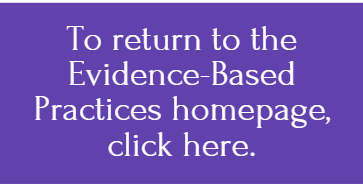
References:
Council for Exceptional Children (CEC). ( 2014 ). Council for Exceptional Children standards for evidence-based practices in special education . Retrieved from http://www.cec.sped.org/~/media/Files/Standards/Evidence%20based%20Practices%20and%20Practice/CECs%20EBP%20Standards.pdf
Gersten, R., Fuchs, L. S., Compton, D., Coyne, M., Greenwood, C., & Innocenti, M. S. (2005). Quality indicators for group experimental and quasi-experimental research in special education. Exceptional Children, 71 , 149–164.
Horner, R. H., Carr, E. G., Halle, J., McGee, G., Odom, S., & Wolery, M. (2005). The use of single-subject research to identify evidence-based practice in special education. Exceptional Children, 71 , 165–179.
Kratochwill, T. R., Hitchcock, J. H., Horner, R. H., Levin, J. R., Odom, S. L., Rindskopf, D. M., & Shadish, W. R. (2013). Single-case intervention research design standards. Remedial and Special Education, 34 , 26–38.
Torres, C., Farley, C. A., & Cook, B. G. (2012). A special educator’s guide to successfully implementing evidence-based practices. TEACHING Exceptional Children, 45(1) , 64–73.

An official website of the United States government
The .gov means it's official. Federal government websites often end in .gov or .mil. Before sharing sensitive information, make sure you're on a federal government site.
The site is secure. The https:// ensures that you are connecting to the official website and that any information you provide is encrypted and transmitted securely.
- Publications
- Account settings
- Browse Titles
NCBI Bookshelf. A service of the National Library of Medicine, National Institutes of Health.
Committee on Assessing the Value of Research in Advancing National Goals; Division of Behavioral and Social Sciences and Education; National Research Council; Celeste RF, Griswold A, Straf ML, editors. Furthering America's Research Enterprise. Washington (DC): National Academies Press (US); 2014 Oct 28.
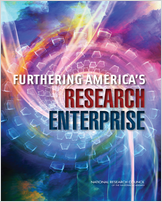
Furthering America's Research Enterprise.
- Hardcopy Version at National Academies Press
5 Measuring Research Impacts and Quality
Key points in this chapter.
- Metrics are used by various nations, by various types of organizations (e.g., public research universities, private industry), and for various purposes (e.g., to measure research impacts retrospectively, to assess current technology diffusion activities, to examine the return on investment in medical research). However, no metric can be used effectively in isolation.
- Industry tends to rely heavily on metrics and expert judgment to assess research performance. Because the goals of the private sector are different from those of the public sector, however, metrics used by industry may not be appropriate for assessing public-sector research activities.
- Universities often use metrics to make the case for annual budgets without infrastructure to analyze research outcomes over time. Alternative measures focus on presenting the income earned from and expenditures devoted to technology transfer activities, tracking invention disclosures, reporting on equity investments, and tracking reimbursement of legal fees.
- Many problems can be avoided if evaluation is built into the design of a research funding program from the outset.
Chapter 4 details the challenges of using existing metrics and existing data, even data from large-scale programs such as Science and Technology for America's Reinvestment: Measuring the Effect of Research on Innovation, Competitiveness and Science (STAR METRICS), to measure research impacts and quality. Despite these challenges, a number of attempts have been made to make these measurements. In preparing this report, the committee drew on a number of relevant studies in the literature. Among the most useful was a recent study ( Guthrie et al., 2013 ) by the RAND Corporation, Measuring Research: A Guide to Research Evaluation Frameworks and Tools , which is summarized in Appendix C and cited frequently in Chapter 4 . We also relied on previous National Research Council (NRC) reports, including a report on innovation in information technology (IT) informally known as the “tire tracks” report ( National Research Council, 2012a ) and a summary of a recent workshop ( National Research Council, 2011b ) on measuring the impacts of federal investments in research. In this chapter, we review some of the relevant studies; we also examine the use of metrics by selected governmental, industry, and nonprofit organizations, pointing out the purposes for which these metrics are useful, as well as those for which they are not.
- USE OF METRICS BY OTHER NATIONS
Many nations other than the United States, such as Australia, Canada, and the United Kingdom, and have struggled with the challenge of measuring research returns, and the committee drew substantially on the literature on those efforts. As noted in Chapter 3 , the benefits of scientific research require extensive time to percolate and may not come to fruition for decades or even centuries. Canada's National Research Council states:
No theory exists that can reliably predict which research activities are most likely to lead to scientific advances or to societal benefit ( Council of Canadian Academies, 2012 , p. 162).
This conclusion is particularly accurate because science is constantly changing in unpredictable directions. For example, progress in the IT field may depend on economics and other social science research on keyword auctions, cloud pricing, social media, and other areas. Economics and other social sciences are becoming even more critical fields of research with the increasing importance of understanding human and organizational behavior, which is needed to enable the adoption of new technologies. As a result, the social sciences are valuable contributors to interdisciplinary research and education.
Metrics have been developed that span multiple disciplines and countries. Nonetheless, the development of universal evaluation systems has proven challenging, in particular because of variations in policies, research funding approaches, and missions ( National Research Council, 2006 ). The United Kingdom's Council for Industry and Higher Education describes three other factors that complicate the accurate assessment of publicly funded research impacts: (1) the influence of complementary investments (e.g., industry funding); (2) the time lag involved in converting knowledge to outcomes; and (3) the skewed nature of research outcomes, such that 50-80 percent of the value created from research will result from 10-20 percent of the most successful projects ( Hughes and Martin, 2012 ). This last constraint might be addressed by analyzing the funding portfolios of each funding agency by research and development (R&D) phase/type and by assessing the behavior of individual researchers in addition to using outcome-based assessments ( Hughes and Martin, 2012 ).
The Australian Group of Eight ( Rymer, 2011 ) notes additional barriers to assessing research impacts: research can have both positive and negative effects (e.g., the creation of chlorofluorocarbons reduced stratospheric ozone); the adoption of research findings depends on sociocultural factors; transformative innovations often depend on previous research; it is extremely difficult to assess the individual and collective impacts of multiple researchers who are tackling the same problem; and finally, it is difficult to assess the transferability of research findings to other, unintended problems. Equally difficult to measure is the ability of research to create an evidence-based context for policy decisions, which is important but poses a formidable challenge ( Rymer, 2011 ; National Research Council, 2012e ).
Even when effective indicators and metrics are developed, their use to determine which research projects should be funded inevitably inspires positive and negative behavioral changes among researchers and research institutions ( OECD, 2010 ), an issue noted also in Chapter 4 . In Australia, Norway, and the United Kingdom, incorporating the number of publications into the grant review process led to a significant increase in publication output ( Butler, 2003 ; Moed et al., 1985 ; OECD, 2010 ). This might be viewed as a positive effect except that in some cases, this increase in output was followed by a decline in publication quality as researchers traded quality for volume. (The quality of a research publication often is assessed by the quality of the journal in which it is published, which may depend on how widely cited the journal is. This can be problematic because the top research in some fields is presented at conferences, not published in journals, and not every study published in high-impact journals is exemplary of high-quality or high-impact research.) This negative effect was more pronounced in Australia than in Norway or the United Kingdom, as the latter nations rely on metrics that account for quality as well as quantity ( Butler, 2003 ).
While metrics based on both quantity and quality have generally proven useful to other nations, two issues have arisen: the potentially subjective definition of a “high-quality” journal, and the difficulty of determining whether widely cited journals are in fact better than specialized or regional journals ( Council of Canadian Academies, 2012 ). For instance, China provides strong incentives to publish in international and widely cited journals; researchers receive 15 to 300 times larger financial bonuses for research published in Nature or Science compared with that published in other journals ( Shao and Shen, 2011 ). As described by Bruce Alberts in an editorial in Science ( Alberts, 2013 ), however, the San Francisco Declaration on Research Assessment 1 acknowledges the potential of journal impact factors to distort the evaluation of scientific research. Alberts asserts that the impact factor must not be used as “a surrogate measure of the quality of individual research articles, to assess an individual scientist's contributions, or in hiring, promotion, or funding decisions.”
Serious consequences—both positive and negative—can occur when governments use metrics with the potential to change researchers' behavior. Researchers and institutions can focus so intently on the metric that achieving a high metric value becomes the goal, rather than improving outcomes. In some cases, there is documented evidence of researchers and institutions resorting to questionable behavior to increase their scores on metrics ( Research Evaluation and Policy Project, 2005 ). A recent survey published in Nature revealed that one in three researchers at Chinese universities have falsified data to generate more publications and publish in more widely cited journals. Some Chinese researchers report hiring ghostwriters to produce false publications ( Qiu, 2010 ). Aside from ethical concerns, such practices have presented serious problems for other researchers in the field, who unknowingly have designed their own research studies on the basis of false reports in the literature. Another negative though less serious outcome occurred when the Australian Research Council incorporated rankings for 20,000 journals, developed through a peer review process, into its Excellence for Research in Australia (ERA) initiative ( Australian Research Council, 2008 ). One year after being developed, the ranking was dropped from ERA because some university research managers were encouraging faculty to publish only in the highest-ranking journals, which had negative implications for smaller journals ( Australian Government, 2011 ).
Additional concerns regarding the development and implementation of metrics have revolved around training and collaboration. The United Kingdom found that use of the Research Assessment Exercise (RAE), a peer-reviewed tool for assessing research strength at universities, significantly affected researchers' morale as certain researchers were promoted as being “research active,” while some departments were dissolved because of poor reviews ( Higher Education Funding Council of England, 1997 ; OECD, 2010 ). Researchers also have noted that the RAE discourages high-risk research because of its focus on outputs, and that it also discourages collaboration, particularly with nonacademic institutions ( Evaluation Associates, Ltd., 1999 ; McNay, 1998 ; OECD, 2010 ). Another commonly used metric, previous external research funding, has been criticized by the Council of Canadian Academies as being subjective because of the nature of previous expert judgment and funding decisions ( Council of Canadian Academies, 2012 ). Using funding as a criterion also poses the risk of allowing outside money to drive research topics (e.g., pharma funding for positive drug evaluation), as well as rewarding inefficient and costly researchers who ask for more money. Additional indicators, such as previous educational institutions attended by students and esteem-based indicators (e.g., awards, prestigious appointments) have been criticized as being subjective in countries such as Canada and Australia. Performance on these indicators may be influenced by external factors such as geographic location and personal choice rather than the institution's quality, and the quality of a researcher's work at the time of funding of a previous award may not characterize his or her current accomplishments ( Council of Canadian Academies, 2012 ; Donovan and Butler, 2007 ).
- IMPACT ASSESSMENTS IN THE UNITED KINGDOM
A review of UK research impact studies by the chair of the Economic and Social Research Council (ESRC) Evaluation Committee notes that so-called “knowledge mobilization”—defined as “getting the best evidence to the appropriate decision makers in both an accessible format and in a timely fashion so as to influence decision making”—can help overcome major impediments that would otherwise limit the economic and social impacts of high-quality research ( Buchanan, 2013 , p. 172). Beginning in the 1990s, a growing body of evidence in the United Kingdom ( Griffith et al., 2001 ; Griliches, 1992 ; Guellec and van Pottelsberghe de la Potterie, 2004 ) suggested that the economic returns of research were limited by researchers' weak attention to knowledge transfer. A 2006 report ( Warry Report, 2006 ) strongly urges research councils to take the lead on the knowledge transfer agenda, to influence the knowledge transfer behavior of universities and research institutes, to better engage user organizations, and to consider metrics that would better demonstrate the economic and social impacts of scientific research. These metrics, it is argued, should assess research excellence as well as the relevance of research findings to user needs, the propensity for economic benefits, and the quality of the relationship between research findings and their likely users ( Warry Report, 2006 , p. 19).
A report commissioned by Universities UK (2007) and a related paper ( Adams, 2009 ) suggest that the research process might be aptly evaluated by being considered in terms of “inputs–activity–outputs–outcomes.” Moreover, indicators for one field of science may not be strong tools for assessing other fields. For example, bibliometric tools were found to be strong indicators of performance in some areas of the social sciences, such as psychology and economics, but not in more applied or policy-related areas. Publication counts were found to be similarly problematic, as they give an idea of a researcher's output volume but do not reflect research quality or the potential for social or economic impact ( Adams, 2009 ). In recognition of these findings, the Research Excellence Framework (REF) assessment 2 in the United Kingdom will consider citation data in science, technology, engineering, and mathematics fields but not in the social sciences.
The ESRC issued a report ( Economic and Social Research Council, 2009 ) identifying several drivers of research impact, including networks of researchers, the involvement of users throughout the research process, and the supportiveness of the current policy environment. A subsequent ESRC report suggests a more comprehensive picture of the interactions between researchers and policy makers might aid efforts to track the policy impacts of research ( Economic and Social Research Council, 2012 ).
On the basis of this literature, the ongoing effort to develop an REF assessment promotes greater knowledge mobilization by compelling academic researchers to engage with the public and demonstrate more clearly the economic and social implications of their work. Current efforts are aimed at ensuring that the quality of research is not compromised by the emphasis on impact and open-access data. It remains to be seen whether and how this latest introduction of new incentives and measurement schemes in a highly centralized national research funding system will create perverse incentives for UK researchers, leading gifted scientists to devote more time to lobbying policy makers or industry managers, or whether it will enhance the impacts of the country's publicly funded research. Nonetheless, these new evaluation measures likely have some potential to distort researchers' behavior and reduce rather than increase positive research impacts.
- USE OF METRICS TO EVALUATE THE ECONOMIC RETURNS OF MEDICAL RESEARCH
Some major studies have sought to measure the economic returns on investments in medical research. In the United States, the Lasker Foundation supported a study leading to the 2000 report Exceptional Returns: The Economic Value of America's Investment in Medical Research ( Passell, 2000 ). In this report and a subsequent volume ( Murphy and Topel, 2003 ), a number of economists describe the “exceptional” returns on the $45 billion (in 2000 dollars) annual investment in medical research from public and private sources and attempt to estimate the economic impact of diagnostic and treatment procedures for particular diseases.
The economic value of medical research was assessed by monetizing the value of improved health and increased life span (i.e., by adapting data from work-related studies performed in the 1970s-1990s), then isolating the direct and indirect impacts of medical research from gains unrelated to R&D (i.e., by accounting for the total economic value of improved survival due to technologies and therapies). The report offers the widely criticized calculation that increases in life expectancy during the 1970s and 1980s were worth a total of $57 trillion to Americans, a figure six times larger than the entire output of tangible good and services in 1999 (the year prior to the report's publication). The gains associated with the prevention and treatment of cardiovascular disease alone totaled $31 trillion. The report suggests that medical research that reduces cancer deaths by just one-fifth is worth approximately $10 trillion to Americans—double the national debt in 2000. The report states that all of these gains were made possible by federal spending that amounted to a mere $0.19 per person per day. Critics of the report note that it simply attributes outcomes in their entirety to investments in medical research without considering, for example, how the returns on medical research in lung cancer might compare with the equally poorly measured returns on education in smoking cessation.
Researchers in Australia ( Access Economics, 2003 , 2008 ) sought to replicate this U.S. study. The first such study, which used the same value for a year of life as that used in the U.S. study, led to some anomalies. By using disability-adjusted life years (DALYs), a measure that accounts for extended years of life adjusted for the effects of disability, this study suggests that the value of mental health research was negative because of the decline in DALYs for mental health. A second Australian study used a different methodology, comparing past research investments with projected future health benefits and basing the value of life on a meta-analysis of studies.
In the United Kingdom, the Academy of Medical Sciences, the Medical Research Council, and the Wellcome Trust commissioned research to assess the economic impact of UK medical research. According to the report:
The overall aim of the work was to compare the macroeconomic benefits accruing from UK medical research with the cost of that research—ultimately to give a quantitative assessment of the benefit of medical research to the UK. It was also expected that the research would critically appraise both the selected approach and previous attempts to estimate the economic returns from research. In this way, the goal was not to obtain a definitive answer about the returns on the investment in UK medical research, but to generate a piece of work that would help to move this young field forward and inform methodologies for future assessments ( Health Economics Research Group et al., 2008 , p. 3).
The study focused on cardiovascular disease and mental health. It used a “bottom-up” approach based on evidence on the “effects and costs of specific research-derived interventions, rather than [on] macro-level, temporal changes in mortality or morbidity” (p. 5).
These and other studies, including work by the Canadian Academy of Health Sciences ( Canadian Academy of Health Sciences, 2009 ) and for the World Health Organization ( Buxton et al., 2004 ), raise many issues concerning the valuation of research aimed at improving health:
- Measuring the economic returns on research investments —Approaches include using a benefit/cost ratio (ratio of the value of health benefits to the costs of research), a return on investment (ratio of the amount by which health benefits exceed research costs to research costs), or an internal rate of return (IRR, the rate of return for which net present value is zero or alternatively, the discount rate at which the net present value of research costs equals the net present value of health benefits over time). The UK study used IRR.
- Valuing health benefits —Examples include using a monetary value for a year of life or a quality-adjusted year of life, direct cost savings for health services, indirect cost savings when improved health leads to productivity increases, or increases in gross domestic product or other economic gains. These efforts, however, are widely criticized.
- Measuring the costs of research —Questions that arise include how costs of research are determined; how infrastructure is accounted for; whether measures of public and private research costs are comparable; and how the effect of research failures, which, as noted earlier, may advance knowledge, can be accounted for.
- Time lag —The appropriate time lag between research and health benefits must be determined.
- Global benefits —Issues include identifying the global health benefits from U.S. research and the health benefits that accrue to the United States from research in other countries, and determining how such international transfers of research knowledge should be accounted for.
- Attribution —It is difficult to disentangle how much of health improvement can be attributed to health care, as opposed to improved hygiene, diet, and other behaviors; to what extent behavior changes to improve health can be attributed to behavioral and social science research; and how the contributions of behavioral and social science research to improved health can be distinguished from those of medical research on therapeutics.
- Intangibles —The extent to which research in a health care system increases the system's capacity to use research findings is difficult to understand ( Belkhodja et al., 2007 ).
- USE OF METRICS IN ECONOMIC IMPACT ASSESSMENTS OF FEDERAL PROGRAMS
Given that, as noted earlier, the results of basic research are largely public goods, the federal government funds a large portion of this research in the United States. There are also reasons why government funding may be needed for some technologies that have both public and private characteristics. These reasons include long gestation periods; the inability to capture the full economic value of an R&D investment; broad scopes of potential market applications; coordination difficulties among the various private-sector entities that must conduct the R&D and eventually integrate the resulting component into the final technology system; and the inability (often due to small firm size) to price an innovation at a level sufficient to rationalize the investment, assuming the generally large technical and market risks associated with R&D investments ( Tassey, 2014 ).
As previously discussed, the typical industrial technology is a complex system combining multiple hardware and software technologies. Many of these component technologies are derived from multiple areas of science and developed by a range of public and private entities. The complex genealogy of many innovations of great economic value reflects the fact that private firms may lack sufficient incentives (e.g., assurance of a return on their investment) to support the development of technological knowledge of a quasi-public good nature, including standards and research infrastructure, or “infratechnologies” (see Chapter 2 ). Without adequate and timely investment in these technology elements, industry's investment in proprietary technologies or other innovations will be both inadequate and inefficient.
Federal R&D policy has implicitly embraced investment to overcome these market failures for agencies whose R&D targets social objectives such as defense (U.S. Department of Defense [DoD]), health (National Institutes of Health [NIH]), and energy (U.S. Department of Energy [DoE]). Thus, DoD funds technology platform research through the Defense Advanced Research Projects Agency, and DoE funds similar research through the Advanced Research Projects Agency-Energy. DoE also funds considerable research in measurement infratechnology and standards.
The National Institute of Standards and Technology (NIST), part of the U.S. Commerce Department, also focuses on infratechnology research. NIST undertook economic impact studies in the 1990s to demonstrate the value of its research. Over the past 20 years, it has conducted 40 such retrospective studies across a wide range of technologies that it supports. To undertake such studies, NIST had to choose a set of metrics from among three basic alternatives (see Figure 5-1 ):
Influences of processes, outputs, and outcomes on research impact. NOTE: Role rationalization and impact assessment are part of a recursive process. Both must be modeled correctly and their interactive nature recognized, as depicted in this figure. The conceptual (more...)
- measures to guide public R&D policies such as allocation of resources, including those that influence investment decisions by firms and businesses (a process measure);
- measures to guide private industry investments in R&D, such as net present value, return on investment, and benefit-cost ratio (an output measure); or
- measures with which to evaluate the research and innovation systems, such as productivity growth, employment growth, and other economic and societal impacts (an outcome measure).
Because the focus of impact assessment was at the program level and evaluation budgets were limited, NIST chose the middle ground—the set used in corporate finance. Under the circumstances (no government-wide guidance and limited resources), this approach yielded the most useful quantitative impact data. NIST's impact reports also provide considerable qualitative analysis, which is essential for interpreting the quantitative results and placing them in context.
USE OF METRICS TO EVALUATE DEPARTMENT OF ENERGY (DOE) FOSSIL FUEL R&D PROGRAMS
At the committee's third meeting, Robert Fri of Resources for the Future discussed a retrospective study that looked at DoE-sponsored research from that agency's inception through 2000 ( National Research Council, 2001 ), as well as two prospective evaluations of DoE applied R&D ( National Research Council, 2005b , 2007b ). The 2001 NRC study in particular used an evaluative framework that emphasized three types of benefits from DoE-sponsored R&D in energy efficiency and fossil fuels: (1) the economic benefits associated with technological advances attributed to the R&D; (2) the “option value” of the technological advances facilitated by the R&D that have not yet been introduced; and (3) the value of the scientific and technological knowledge, not all of which has yet been embodied in innovations, resulting from the R&D. Like most such retrospective studies, the 2001 study faced challenges in attributing these three types of benefits to specific DoE R&D programs, since substantial investments in many of the technologies were made by industry. An important source of the estimated economic benefits of DoE R&D programs was the programs' contributions to accelerating the introduction of the innovations studied.
The attempt in the 2001 report to highlight the “options value” of technological advances points to another type of benefit that is difficult to capture in retrospective evaluations of R&D investments but is important nonetheless: in a world of great uncertainty about economic, climatic, and technological developments, there is value in having a broad array of technological options through which to respond to changes in the broader environment. 3 The DoE programs examined in the 2001 study produced a number of innovations that were not introduced commercially simply because their characteristics and performance did not make them competitive with existing or other new technologies. But there is a definite value associated with the availability of these technological alternatives or options in the face of an uncertain future (see Box 5-1 for a discussion of shale oil extraction technologies, many of which benefited from DoE and other federal R&D but have been applied only in the past decade).
Shale Oil Recovery. The potential importance of shale oil and gas has been known for more than a century, but only in the past decade have oil companies been able to access this vast resource. The booming industry that exists today was built on technologies (more...)
Deriving a quantitative estimate of the “option value” of such innovations, however, is very difficult precisely because of the uncertainty about conditions under which they might be of use (this is an important difference between the value of financial and technological options). As with other retrospective evaluations, however, it was impossible to incorporate any quasi-experimental elements into the assessment scheme for these DoE programs. No effort was made to examine the question of what would have happened had these programs not existed (e.g., whether similar investments in R&D would have come from other sources). There was also no attempt to compare firms that exploited the results of DoE R&D with some type of “control” population, for obvious reasons. These limitations are hardly critical or fatal, but they illustrate the challenges of developing designs for R&D program evaluation that approximate the “gold standard” of randomized assignment of members of a population (of firms, individuals, institutions, etc.) to treatment and control groups.
The 2001 report also includes a detailed set of case studies of DoE R&D programs in the areas of energy efficiency and fossil fuels. Overall, the report states that in the area of energy efficiency, DoE R&D investments of roughly $7 billion during the 22-year life of this program yielded economic benefits amounting to approximately $30 billion in 1999 dollars. DoE fossil energy programs during 1978-1986 invested $6 billion in R&D (this period included some costly synthetic fuels projects) and yielded economic benefits of $3.4 billion (all amounts in 1999 dollars). DoE fossil energy programs during 1986-2000, by contrast, accounted for an investment of $4.5 billion and yielded economic benefits estimated at $7.4 billion (again in 1999 dollars). Many other significant conclusions in the 2001 report concern qualitative “lessons” for program design based on observations of successful and less successful programs.
An important source of estimated economic benefit for the DoE R&D programs examined in the 2001 NRC and related studies was the fact that the innovations that benefited from these federal R&D investments were in use, and their economic payoffs could be estimated relatively directly. Nevertheless, the efforts in these studies to at least highlight (if not quantify) the “options” and “knowledge” benefits of federal R&D investments are highly relevant to the present study.
The 2001 NRC and related studies further conclude that there is a need for broadly based incentives for the private sector to invest in basic research across a wide range of disciplines to increase the odds of success. The government plays an important role in sponsoring high-risk, mission-driven basic research; funding risky demonstration projects; partnering with industry-driven technology programs; and encouraging industry's adoption of new technology.
- USE OF METRICS BY PRIVATE INDUSTRY: IBM'S PERSPECTIVE
In his presentation to the committee, John E. Kelly, III, director of research for IBM, noted that metrics are essential tools for ensuring continued growth and competitiveness. To stay on the leading edge of technology, IBM designs metrics and processes around four primary missions: (1) seeing and creating the future, (2) supporting business units with innovative technologies for their product and service roadmaps, (3) exploring the science underlying IT, and (4) creating and nurturing a research environment of risk taking and innovation. In general, most research funding decisions made by IBM rely more heavily on judgment than on quantitative metrics, although the relative importance of qualitative versus quantitative data shifts during the transition from long-term research to near-term development.
Although industry's goals for research differ from those of the government, strategic planning is a valuable way to identify gaps in existing platforms and infratechnologies, as discussed earlier in this chapter. IBM relies largely on a process called the Global Technology Outlook (GTO) to fulfill its mission of seeing and creating the future. Every year, it initiates a corporate-wide effort to look years into the future and shift its strategy based on the technology changes it foresees. As a result, IBM has been inspired to create new businesses, acquire companies, and divest itself of others. The GTO process has proven invaluable in efforts involving long lead times, such as architecture, where a correct or incorrect decision can have profound effects on a company. Metrics are used in the GTO process in an attempt to quantify how many future technology disruptions and business trends will be identified and how many will be missed. These predictive metrics emphasize qualitative information, relying heavily on the judgment of experienced managers and scientists.
To fulfill its mission of supporting business units with innovative technologies for their product and service roadmaps, IBM continually focuses on creating new technologies—innovative hardware or software features—to integrate into its product lines over 2- and 5-year horizons. The metrics used here include near-term and relatively easy-to-quantify outcomes such as product competitiveness and market share. IBM also measures the intellectual property being generated through counts of patents and other means.
According to Kelly, IBM believes a deep understanding of science is essential to making sustained progress; through this understanding, IBM fulfills its mission of exploring the science underlying IT. The company supports large research efforts in hardware, software, and service sciences (i.e., people and technologies organized in a certain way to produce a desired impact). Some of these efforts are enhanced by partnerships with universities, government, and industrial laboratories around the world. Metrics used to assess progress toward fulfillment of this mission include those pertaining to key publications, recruiting and retention of top scientists, and impact on various scientific disciplines.
Finally, IBM supports its mission of creating and nurturing a research environment of risk taking and innovation by focusing on inputs. It makes a concerted effort to hire the best people and provide them with a large degree of freedom to pursue innovative ideas. According to Kelly, one cannot manage research the way one manages development or manufacturing. When it comes to research, micromanagement is counterproductive to growth, innovation, and competitiveness.
- USE OF METRICS BY PRIVATE NONPROFITS: BATTELLE'S PERSPECTIVE
In his presentation to the committee, Jeff Wadsworth, president and chief executive officer of Battelle Memorial Institute, noted that metrics are critically important for guiding research investments and monitoring the success of R&D. Battelle uses metrics throughout the R&D process—from tracking the long-term success rate of its project selection process, to improving the productivity (and therefore capital efficiency) of its R&D activity, to tracking the financial contributions of its innovation system with lagging metrics such as percentage of sales from new products.
However, Wadsworth noted that while certain private-sector management approaches—such as DoD's use of the business process improvement approach known as Lean Six Sigma or the national laboratories' use of private management and operations contractors—may lend value to government research activities, many public-sector research activities require different measures from those used by the private sector since the latter are defined almost exclusively by economics ( Cooper, 1986 ). Wadsworth suggested that economic analysis combined with analyses of future impacts can be used to measure the impact of public-sector research. For example, Battelle's 2011 and 2013 studies suggested that the economic returns of the Human Genome Project have approached a trillion dollars ( Batelle, 2013 ). That analysis, however, has not been universally accepted, in part because it examined only economic activity and not the impact on human health, and it attributed the economic returns to the government's investment when other factors, including private investments in genomics, have contributed ( Brice, 2013 ; Wadman, 2013a ).
- USE OF METRICS TO EVALUATE THE REGIONAL ECONOMIC IMPACTS OF RESEARCH UNIVERSITIES
Many public research and education institutions have conducted studies of their impact on local, regional, and state economies. Some institutions, such as Massachusetts Institute of Technology (MIT), have commissioned economic impact reports to illustrate these returns. Like many retrospective evaluations, however, such reports contain useful data but are rarely able to address the counterfactual issues that loom large: For example, what would have happened in the absence of a specific set of policies or channels for economic interaction between university researchers and the regional economy?
A 2009 study, Entrepreneurial Impact: The Role of MIT ( Ewing Marion Kauffman Foundation, 2009 ), analyzes the economic impacts of companies started by MIT alumni. The analysis is based on a 2003 survey of all living MIT alumni and revenue and employment figures updated to 2006. The study concludes that if all of the companies (excluding Hewlett-Packard and Intel) were combined, they would employ 3.3 million people and generate annual revenues of $2 trillion, representing the 17th-largest economy in the world ( Ewing Marion Kauffman Foundation, 2009 ). In addition, the study offers the following conclusions:
- An estimated 6,900 MIT alumni companies with worldwide sales of approximately $164 billion are located in Massachusetts alone and represent 26 percent of the sales of all Massachusetts companies.
- 4,100 MIT alumni-founded firms are based in California, and generate an estimated $134 billion in worldwide sales.
- States currently benefiting most from jobs created by MIT alumni companies are Massachusetts (estimated at just under 1 million jobs worldwide from Massachusetts-based companies); California (estimated at 526,000 jobs), New York (estimated at 231,000 jobs), Texas (estimated at 184,000), and Virginia (estimated at 136,000).
This study provides an accounting of the economic effects of firms founded by alumni of one research university, MIT. It does not isolate or highlight the mechanisms through which the economic benefits were realized, so one cannot conclude that some of the benefits would not have occurred otherwise. Moreover, research universities contribute to the production of knowledge for the development of new technologies and firms in many ways other than through alumni.
- USE OF METRICS TO MONITOR TECHNOLOGY TRANSFER FROM UNIVERSITIES
Universities use various metrics to track the diffusion of technology resulting from the research they conduct (see Appendix B ). Most of the metrics widely used for this purpose (e.g., inputs such as collaborations, intermediate outputs such as innovation creation and knowledge acceleration, and final impacts such as qualitative outcomes or economic development) have been criticized as ignoring some of the more important formal and informal channels of knowledge flow to and from universities ( Walsh et al., 2003a , b ). Examples of these channels include the flow of trained degree holders, faculty publications, sabbaticals in university laboratories for industry scientists, faculty and student participation in conferences, and faculty consulting. It should be noted as well that at least some metrics proposed or implemented for faculty evaluation at some universities, such as patenting, could have effects similar to the use of publication counts in China and other economies: if faculty perceive an incentive to obtain more patents, they are likely to file for more patents; however, the quality of these patents could well be low, and the legal fees paid by academic institutions to protect the rights to a larger flow of patent applications could increase.
Moreover, the appropriateness of commonplace metrics depends largely on whether the goal of the university's technology transfer office is to increase the university's revenue through licensing, to assist university entrepreneurs, to support small firms, to support regional development, to attract and retain entrepreneurial faculty, or any number of other goals. A disconnect often exists between the selection of metrics and the university's broader strategic goals, which can make it difficult to use the metrics to analyze performance or draw comparisons among universities. Box 5-2 elaborates on the value of university technology transfer metrics.
Value of University Technology Transfer Metrics. To assess the value of technology transfer metrics and their utility for assessing the value of research, it is important to understand the nature of many technology transfer offices and the environment (more...)
- EVALUATION OF RESEARCH FUNDING PROGRAMS
A fundamental question with which the committee grappled was how to assess which research funding programs are effective and how to choose among them to maximize returns to society (i.e., what areas of research should be funded and through what agencies). Addressing this question leads to evaluation of the effectiveness of the wide variety of programs adopted by research funding agencies in the United States to select individuals and groups for research support. The agencies employ two types of approaches—one for selecting recipients of research funding (i.e., prospective assessment) and another for evaluating the performance of those funded (i.e., retrospective evaluation).
Evaluating the effectiveness of a research funding program requires a different strategy and different forms of data gathering from those typically used by research funding agencies and program managers. As we have noted, neither the Executive Branch nor Congress has an institutional mechanism for attempting cross-field comparisons or undertaking an R&D budget portfolio analysis.
Moreover, few federal agencies dedicate resources within programs for retrospective evaluation. NIH has a separate evaluation staff that provides guidance to programs, but expects each program to implement its own evaluations. NIH programs tend to fund external research organizations to conduct both process and outcome evaluations early in the program and at about the 5-year point, respectively. Evaluations are rarely conducted beyond this point. The National Science Foundation (NSF) requires an evaluator for some of its grant programs, such as the 10-year Industry-University Cooperative Research Center Program and some of its educational grant programs. The outputs of these evaluation efforts are descriptive statistics and case studies, which are useful for describing the programs but rarely yield insights valuable for measuring impact. For other programs, NSF follows the same model as NIH and contracts with research organizations to conduct process and outcome evaluations.
Program managers at NIH generally are open to program evaluation. Accessing data is time-consuming and bureaucratic, but once access is obtained, NIH has more data in structured format, which facilitates data analysis. Obtaining access, however, may take several months. As previously noted, in addition to STAR METRICS, NIH research data systems include the Research Portfolio Online Reporting Tools, the Scientific Publication Information Retrieval and Evaluation System, and the Electronic Scientific Portfolio Assistant. NSF data often must first be “scraped” and computer programs (e.g., Python) used to create variables from unstructured text data.
Government-wide mandates such as the Government Performance and Results Act (GPRA) and the Program Assessment Rating Tool have been implemented with good intent, and their language focuses on measuring outcomes and impacts. However, implementation focuses on measuring short-term outputs because they can be measured more easily than longer-term outcomes. Without staff resources dedicated to evaluation, it is difficult to do more. (See National Academy of Sciences [1999 , 2011 ] for discussion of how GPRA has led to federal agency measurement of the performance of research.)
We distinguish between two types of comparison for program evaluation: (1) comparing different research areas, and (2) comparing proposals submitted by individuals or groups of researchers within a research area, either retrospectively or prospectively. The two approaches present very different analytic challenges.
In the committee's judgment, comparisons involving the allocation of funding among widely varied research areas and those involving the assessment of different researchers or groups within a given research field or specialty are conceptually different tasks, and treating them as related or somehow similar is a source of confusion. Programs that allocate funds among different research areas, such as NSF's Science and Technology Centers Program, are more difficult to evaluate than programs that allocate funds among researchers in a specific research area, such as economics research supported by NSF. One reason for this greater difficulty is the many alternative research funding programs with which the program under consideration should be compared. Even the attribution of outcomes may not be clear: If a new research program stimulates a research proposal that is funded by another program, to which program should the outcomes be attributed?
Further complication is introduced by efforts to measure the success of a program, even assuming that a clear set of agreed-upon outcome measures exists. Impact or outcome measures are ideal, but require data that may be impossible or very difficult to obtain at reasonable cost. Finally, it is not always clear that specific outcomes can be attributed to a research funding program when many other factors influence impact.
Guthrie and colleagues (2013) provide a synthesis of existing and previously proposed frameworks and indicators for evaluating research. They note that research evaluation aims to do one or more of the following:
- Advocate: to demonstrate the benefits of supporting research, enhance understanding of research and its processes among policymakers and the public, and make the case for policy and practice change
- Show accountability: to show that money and other resources have been used efficiently and effectively, and to hold researchers to account
- Analyse: to understand how and why research is effective and how it can be better supported, feeding into research strategy and decision making by providing a stronger evidence base
- Allocate: to determine where best to allocate funds in the future, making the best use possible of a limited funding pot (pp. ix-x).
In particular, Guthrie and colleagues (2013) reviewed 14 research evaluation frameworks, 6 of which they investigated in detail, and 10 research evaluation tools such as STAR METRICS. Most of these frameworks require data on inputs and outputs, as well as information about the scientific process.
While such evaluation approaches are valuable for many purposes, they do not address the fundamental question that faced the committee: What would have happened without the research funding program, or if the resources had been used on other programs or had been allocated in different ways within the program? Instead, these frameworks look at the allocations within programs and attempt to measure scientific productivity or even innovation, often using publications, patents, or related output measures. These are useful for performance measures (see the discussion in Chapter 6 ), but even if the outputs are assumed to be surrogates for eventual outcomes, they do not provide an evaluation without a counterfactual.
Research funding programs in which evaluation is built in from the outset are superior to those that attempt evaluation retrospectively, as the latter evaluations often are more prone to unmeasurable biases of various sorts. Few studies or approaches consider the role of formal statistical field studies or experiments with randomization used to control for biases and input differences.
The standard review mechanism for prospective evaluation of research grant and contract proposals is some form of peer review and assessment. Some have criticized peer review for discouraging the funding of high-risk research or radically new research approaches, but more recently, others have criticized it for the dilution of expertise in the NIH review process:
Historically, study sections that review applications were composed largely of highly respected leaders in the field, and there was widespread trust in the fairness of the system. Today it is less common for senior scientists to serve. Either they are not asked or, when asked, it is more difficult to persuade them to participate because of very low success rates, difficulties of choosing among highly meritorious proposals, and the perception that the quality of evaluation has declined ( Alberts et al., 2014 , p. 2).
Yet despite the need for improvements in the peer review process, and especially in light of the decreasing success rate for research proposals, there is limited experience with the widespread use by public agencies of alternative mechanisms, and little existing evidence suggests that there is generally a better mechanism. The committee cautions that peer review is not designed to assess overall program effectiveness, but rather investigator qualifications and the innovativeness of individual projects within a given research program. Thus, peer review typically is most appropriate as a means of awarding funding rather than assessing performance. There have been cases, however, in which panels of experts have assessed the outputs of research programs using peer review and other approaches ( Guthrie et al., 2013 ; National Academy of Sciences, 1999 , 2011 ). Some interesting evaluation studies also have been conducted using the methodologies reviewed by Guthrie and colleagues (2013) , but they appear to be limited both in focus and in implementation. Other evaluations, such as that by Jacob and Lefgren (2011) using a regression continuity design, appear to be internally focused (i.e., not comparative) and subject to many possible biases.
As an example, consider the NSF Science and Technology Centers Program, aimed at developing large-scale, long-term, potentially transformative research collaborations ( National Science Foundation, 2014b ). Efforts to evaluate this program have focused primarily on individual center reviews, both for the selection of centers for funding and for the assessment of ongoing effectiveness. Evaluation in this case does not attempt to compare the performance of different centers, nor does it assess the performance of centers funded versus those not funded by NSF ( Chubin et al., 2010 ). Comparing funded centers with those not funded might somehow help, but such a comparison would be limited to an examination of this one program. To our knowledge, there have been no systematic reviews of unfunded center proposals and the research output of the investigators involved in these proposals. Nor has there been any counterfactual analysis of what would have happened had there been no Science and Technology Center funding or of what benefit for science might have been gained had the dollars been spent differently (i.e., on other programs).
Similar to the report by Azoulay and colleagues (2010) mentioned in Chapter 4 , a study by Lal and colleagues (2012) evaluates the NIH Director's Pioneer Award (NDPA). The authors set out to answer the following questions: To what extent does the research supported by the NDPA (or the “Pioneer”) Program produce unusually high impacts, and to what extent are the research approaches used by the NDPA grantees (or the “Pioneers”) highly innovative?
Inevitably the answers to such questions are comparative. Lal and colleagues (2012) conclude that the performance of the first three cohorts of Pioneer Award winners was comparable or superior to that of most other groups of funded investigators—excluding Howard Hughes Medical Institute investigators, whose performance exceeded that of the Pioneers on some but not all impact indicators (e.g., on number of publications, number of citations per awardee, and journal rankings). Lal and colleagues set out to compare the effects of different funding programs using retrospective matching. Retrospective matching is inevitably inferior to prospective randomization as an evaluation design, and the analyses that use it cannot control adequately for the award mechanisms of the various programs and for the multiplicity of sources of funding that teams of investigators seek and receive. Nevertheless, this study was the best one could do after the fact, given the available information. Building evaluation into the program prospectively might have yielded quite different results.
We have discussed two types of comparison used in research program evaluation—comparing different research areas and comparing proposals submitted by individuals or groups of researchers within a research area, either retrospectively or prospectively. A third type is seen in international benchmarking, which uses review panels to assess the relative status of research fields among countries or regions ( National Academy of Sciences, 1993 , 1995, 2000 ). Although international benchmarking can be used to assess whether the United States is losing ground compared with other countries in certain research areas, it is not designed to assess the effectiveness of federal research programs in forestalling such declines. Instead, international benchmarking can only measure outcomes that may be loosely connected with the funding or management of research programs. Moreover, the selection of outcome measures in international benchmarking is even more difficult and controversial than in the other types of comparison.
All three types of comparison also face challenges of attribution of observed outputs, outcomes, or performance. The fundamental statistical tool of randomized experiments could play a role in these comparisons, but it may be feasible only for the first type—comparison of individuals or groups within a research area. Even so, very little evaluation has been conducted through randomized experimentation, and we believe there are both small and large opportunities for wider use of this method. We encourage continuing to experiment with modifications of this approach to evaluation for both prospective and retrospective assessments.
One opportunity for randomization would be to evaluate peer review. Awards could be randomized among proposals near the cut-off point for funding, and the results of both those funded and not funded could be followed up. Or randomization could be used among reviewers of proposals, because once the outliers of exceptionally good- or bad-quality proposals have been determined, variation among reviewers may exceed the variation among proposals.
Regardless of what approach to prospective evaluation of a research funding program is explored, it is preferable to build evaluation into the program from the very beginning. Doing so helps clarify goals and expectations and allows for the collection of important data that might otherwise be missed. If counterfactual models appropriate for the evaluation are defined in advance, data that allow for comparisons with those models can be identified for collection. Advance planning allows for interventions in the program that can be part of the evaluation.
The ideal design of an experiment for an evaluation may be achievable if it is built into new programs, but this approach requires the commitment of scarce funds and talent within federal research programs, including staff trained to carry out, or at least oversee, its implementation. Other requirements of a research program may compete for resources needed for evaluation. A program may be required to allocate all of its funds to awards for research, leaving none for evaluation. In some cases, programs may receive set-aside funds for evaluation, but only years after the program has begun.
Despite the difficulties, evaluation can be built into the design of a research program, as is illustrated by the Advanced Technology Program (ATP) in the Department of Commerce. That program conducted a number of evaluations, including comparisons with firms that had not applied for an ATP grant and with applicants that had applied but not been funded ( Advanced Technology Program, 2005 ; Kerwin and Campbell, 2007 ). Evaluation was built into the design of the program, with data being collected throughout the life of a project and into its postfunding period.
Finally, evaluation can be conducted retrospectively. With this approach, an outcome is observed, and assuming that reasonable evaluators agree on its importance and measurement, the question for evaluation is whether this outcome was due to the research funded by the program. To answer this question, a different form of counterfactual analysis, sometimes referred to as “causes of effects,” is necessary. The potential outcomes of alternative treatments (e.g., program structures or portfolios), or at least a framework for speculating about them, need to be specified. This approach often requires many qualifications and assumptions ( Dawid et al., 2013 ).
Regardless of what approach is used for evaluation, it is important to keep in mind the need for careful, controlled, systematic measurement of well-defined concepts:
Research that can reach causal conclusions has to involve well-defined concepts, careful measurement, and data gathered in controlled settings. Only through the accumulation of information gathered in a systematic fashion can one hope to disentangle the aspects of cause and effect that are relevant ( National Research Council, 2012e , p. 91).
Investment in scientific research propelled the U.S. economy to global leadership during the Industrial Revolution and again in the more recent Information Revolution. Today, the amount and composition of these assets are changing at an increasingly rapid pace, presenting leading economies, such as the United States, with challenges to maintaining competitive positions in a sufficient number of industries to achieve national economic growth goals, especially in employment and income. The levels, composition, and efficiency of federally funded research need to be adjusted to meet today's circumstances. Better metrics can be developed to inform policy decisions about research. This can be the charge of a government unit with the capability to systematically evaluate the research enterprise, assess its impact, and develop policy options for federally funded research. As noted, however, no federal agency or department currently is tasked with performing policy analysis for research. And as observed in Chapter 2 , while NSF's National Center for Science and Engineering Statistics produces valuable data (e.g., Science and Engineering Indicators ) that could be used in policy analysis, NSF's role differs from that of federal policy analysis agencies or statistics agencies such as the Bureau of Economic Analysis or the Economic Research Service that conduct policy analysis. Therefore, the committee's judgment is that no such institutionalized capability currently exists within the U.S. government.
The Declaration is available from http://am .ascb.org/dora/ [August 2014].
In 2007, the United Kingdom announced plans to establish the REF to gauge the quality of research in the nation's institutions of higher education. According to the REF's official website ( http://www .ref.ac.uk/faq/ [August 2014]), the 2014 version of the REF will replace the nation's former system, the RAE.
Other academic studies of the options value of R&D investments include Bloom and van Reenen (2002) and McGrath and Nerkar (2004) .
- Cite this Page Committee on Assessing the Value of Research in Advancing National Goals; Division of Behavioral and Social Sciences and Education; National Research Council; Celeste RF, Griswold A, Straf ML, editors. Furthering America's Research Enterprise. Washington (DC): National Academies Press (US); 2014 Oct 28. 5, Measuring Research Impacts and Quality.
- PDF version of this title (3.2M)
In this Page
- USE OF METRICS TO EVALUATE DEPARTMENT OF ENERGY (DOE) FOSSIL FUEL R&D PROGRAMS
Recent Activity
- Measuring Research Impacts and Quality - Furthering America's Research Enterpris... Measuring Research Impacts and Quality - Furthering America's Research Enterprise
Your browsing activity is empty.
Activity recording is turned off.
Turn recording back on
Connect with NLM
National Library of Medicine 8600 Rockville Pike Bethesda, MD 20894
Web Policies FOIA HHS Vulnerability Disclosure
Help Accessibility Careers
- Question Papers
- Scholarships
Top 20 Research Design MCQ With Answers
Below given are top 20 important Research Design MCQ with answers. These updated multiple choice questions on research design are helpful for BBA, B Com, MBA, MMS, BMS, B Sc, Engineering, PGDM, M Phil and Ph D students and researchers. These MCQs will help for UGC NET, SET, MPSC, UPSC and other competitive entrance exams.
_______research is based on the measurement of quantity or amount.
A. Qualitative
B. Descriptive
C. Quantitative
D. Numerical
______ describes the present state of affairs as it exists without having any control over variables.
A. Analytical research
B. Descriptive research
C. Applied research
D. Distinctive research
In the _______research, the researcher has to use facts or information already available .
A. Analytical
D. Distinctive
__ ___ research is concerned with qualitative phenomena.
______ is related to some abstract ideas or theory.
A. Contextual research
B. Conceptual research
C. Ideal research
D. Empirical research
______ is data-based, coming up with conclusions that are capable of being verified, by observation or by experiment.
The objective of ______ is the development of hypotheses rather than their testing .
A. Laboratory research
B. Diagnostic research
C. Exploratory research
A ________ refers to some difficulty that a researcher experiences in either a theoretical or practical situation
A. research hypothesis
B. research experience
C. research problem
D. research crisis
_______ as a testable statement of a potential relationship between two or more variables.
Research design is a _________for conducting the marketing research project.
A. strategy
B. framework
C. blueprint
D. both B & C
______ is a hypothetical statements denying what are explicitly indicated in working hypotheses.
A. Null hypotheses
B. Working hypotheses
C. Descriptive hypotheses
D. Relational hypotheses
A Blue print of Research work is known as _______
A. sampling design
B. research design
C. research hypotheses
D. research approach
Research design is a blue print, outline and a _________
A. guidance
D. strategy
The choice of research design is influenced by the ________
A. the nature of the research problem
B. the audiences for the study
C. the researchers’ personal experiences
D. all of the above
A Blue print of Research work is called ____
A. Research design
B. Research Problem
C. Research methods
D. Research tools
_______ affect the choice of research methods .
A. Whether the research is ethical or not
B. Time and money available
C. Aims of the researcher
________ is the name of the conceptual framework in which the research is carried out.
A. Research paradigm
B. Synopsis of Research
C. Research design
D. Research hypothesis
The longitudinal research approach mainly deal with _____
A. Horizontal research
B. Vertical Research
C. Short-term research
D. Long-term research
Authenticity of a research finding is its ____
A. Objectivity
B. Tangibility
C. Originality
D. Validity
Research design is a blue print, outline and a ______
A. Strategy
This is all about solved MCQ on Research Design and related concepts.
You’ll also like Business Research Methods MCQ With Answers .
Share with friends

View all MCQs in
No comments yet
Related MCQs
- ‐‐‐‐‐‐‐‐‐‐‐‐‐‐‐‐‐‐‐‐‐‐‐ research in applicable to phenomena that are measurable so that they can expressed in terms of quantity.
- Motivation Research is a type of …………… research
- After formulating the research problem the research will prepare ‐‐‐‐‐‐‐‐‐‐‐‐‐‐‐‐‐‐‐‐‐.
- Experimental research is based on ‐‐‐‐‐‐‐‐‐‐‐‐‐‐‐‐‐‐‐‐.
- Quantitative research is based on ‐‐‐‐‐‐‐‐‐‐‐‐‐‐‐‐‐‐‐‐‐‐‐.
- Who authored the book “methods in Social Research”
- “Research is an organized and systematic enquiry” Defined by
- Research is a “Scientific undertaking” opined by
- Social Science Research ……………. Problems
- Social research aims at ……………….
Measurement in social research: some misunderstandings
- Published: 15 July 2016
- Volume 51 , pages 2219–2243, ( 2017 )
Cite this article
- Alessandro Bruschi ORCID: orcid.org/0000-0002-0150-0432 1
649 Accesses
2 Citations
Explore all metrics
The concept of numerical measurement based on the manipulation of objects has impoverished and distorted the meaning of magnitude and scale. This article aims to contribute to a concept of the most fruitful measurement for the development of the social sciences, with reference to specific aspects indicating the differences from the natural one and regarding, in particular, the non-numeric.
This is a preview of subscription content, log in via an institution to check access.
Access this article
Price includes VAT (Russian Federation)
Instant access to the full article PDF.
Rent this article via DeepDyve
Institutional subscriptions
Similar content being viewed by others
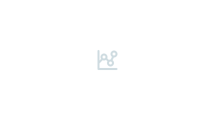
What is Qualitative in Qualitative Research
Patrik Aspers & Ugo Corte
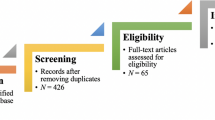
Criteria for Good Qualitative Research: A Comprehensive Review
Drishti Yadav
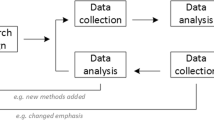
How to use and assess qualitative research methods
Loraine Busetto, Wolfgang Wick & Christoph Gumbinger
According to Stevens “The numerosity of collections of objects (number in the layman sense) constitutes the oldest and one of the most basic scales of measurement. It belongs to the class that I have called ratio scales” ( 1959 , p. 20). But, in this interpretation, ratio scales become both those that give rise to a discrete, natural, and to a continuous, real numerical measurement.
The general validity of the division of physical properties into extensive and intensive kinds, introduced by Tolman, has been addressed in the course of science. Redlich noted that these two categories are not inclusive of all the properties ( 1970 ).
The distinction recalls that of extensive and intensive classification, but the two classifications are based on different criteria: the extensive/intensive on intrinsic properties of magnitudes, the other on operations that are performed for the measurement. And in fact the distinction between extensive properties and intensive does not coincide with that between fundamental and derived of Campbell. There are properties such as temperature which, despite being considered fundamental, are intensive.
This is the Ellis classification with some differences. For Ellis there are at least four types of measurement: elementary, fundamental, associative, and derived ( 1966 , pp. 58–67). The first two are direct, distinct in fundamental and elementary, where elementary is the ordinal scale, common to all quantitative magnitudes, because, if a quantity exists, it has a relation of order; fundamental is one direct that allows additive operations. The associative and the derivatives are indirect. I deleted the elementary because it does not concern the priority, but the level of measurement.
According to the IS, fundamental are those fundamental of Campbell and the associative ones; all other are derived.
In Book V of the Metaphysics, par. 13, Aristotle says that "one thing has quantity when it is divisible into parts that belong to it, and each part is by nature something of a single particularly determined one. A quantity is a multiplicity if is countable, a magnitude if it is measurable. It is said multiplicity which is potentially divisible in parts that are not continuous; it is said magnitude which is divisible into continuous parts." ( 2014 , p. 321).
Actually Campbell ( 1957 , p. 327) does not exclude other ways of doing numerical assignments but, in his opinion, it is hard to discuss their possibility, not finding examples in physics.
As well as, with some differentiation, in the work of Pfanzagl ( 1968 ) and Narens ( 1981 ). For an analysis of the development of the RT, see Martin ( 2003 ).
However, it was observed that the Rasch model is based on a probabilistic logic, while that of the SCM is deterministic. It also leaves doubtful the relationship between the empirical and the formal structures of the IRT, which is denied by Kyngdon ( 2008 ), because he considers the first ones as formal and numerical as the second ones, produced by parameter estimates and probability. Michell ( 2008 ) believes that the logic of Rasch model differs from that of SCM, it does not reach the final result by controlling the order relations of joint propriety, but using a function between property intensity and response probability.
But later Stevens seems to have some doubt, stating: “Although the definition of measurement could, if we wished, be broadened to include the determination of any kind of relation between properties of objects or events, it seems reasonable, for the present, to restrict its meaning to those relations for which one or another propriety of the real number system might serve as a useful model” (Stevens 1959 , p. 24).
Moreover, subjects can be compared even if the same items are not administered to them. Further advantages come from the greater precision of the IRT tools in measuring specific levels of a property, for which tests with a few items may have a high level of information, unlike what happens in the classical measurement, where accuracy goes up increasing their number.
And Pap, in a more liberal way, considered magnitude a property measurable only in a numerical sense, when it is continuous or at least amenable to cardinal measurement ( 1962 , chap. 8).
There is also the problem of the theoretical validity of the criterion used (the other scale) for the control, because both scales may not be valid, or not be just the one introduced as a criterion.
For a comparison between the two measurements, see also Finkelstein ( 2003 , 2009 ).
For a history of the relations between sociology and mathematics see Capecchi ( 2010 ).
As is evidenced by the debate on Qualitative Comparative Analysis . We can refer to, among others, the contributions in Sociological Theory and Methods , in Studies in Comparative International Development , in Sociological Methodology , and the recent contribution by Lucas and Szatrowski ( 2014 ) with a vast bibliography to this regard.
So, for Torgerson numerical labels can be used to name the classes, but the fact that a librarian assigns the number 8105 to a book does not mean that he has measured the book; otherwise, the classification, and even the name of the individual cases become a form of measurement (Torgerson 1958 : pp. 9, 14). And, according to Sartori, a nominal scale is only a classification, not a scale that measures something; certainly, also the items of a classification may be numbered: but this is just a coding gimmick that has nothing to do with a quantification (Sartori 1971 , p. 53).
According to McDonald the question is not whether the scale fulfills the properties of a given level of measurement, but if the statistical required assumptions are fulfilled and the statistical hypotheses remain invariant to the change of scale ( 1999 , p. 418). See also Labovitz ( 1970 , p. 515), Velleman and Wilkinson ( 1993 ).
See Acock and Martin ( 1974 ), Grether ( 1979 ), Young ( 1981 ), Henry ( 1982 ), O'Brien ( 1985 ).
Acock, A.C., David Martin, J.: The undermeasurement controversy: should ordinal data be treated as interval? Sociol. Soc. Res. LVIII , 4 (1974)
Google Scholar
Aristotle: Metafisica. In: Viano, C.A. (ed.) UTET, Milano (2014)
Blalock Jr., H.M.: Conceptualization and Measurement in the Social Science. Sage, Beverly Hills (1982)
Bridgman, P.: William: The Logic of Modern Phisics. N. York, McMillan (1927)
Bruschi, A.: La metodologia povera. NIS, Roma (1993)
Bruschi, A.: Metodologia delle Scienze Sociali. Bruno Mondatori, Milano (1999)
Bruschi, A.: Logical models and logical method. In: Negrotti, M. (ed.) Yearbook of Artificial, vol. 2. Models in Contemporary Sciences. Peter Lang, Bern (2004)
Bureau international des poids et mesures (BIPM): International Vocabulary of Metrology—basic and general concepts and associated terms, JCGM (2012)
Campbell, N.R.: Physics, the Elements. Cambridge University Press, Cambridge (1920)
Campbell, N.R.: What is Science? Methuen & Co., London (1921)
Campbell, N.R.: Symposium: measurement and its importance for philosophy. Aristotelian Society, Suppl. vol. 17. Harrison, London (1938)
Campbell, N.R.: Foundations of Science: the Philosophy of Theory and Experiment. Dover, New York (1957)
Capecchi, V.: From lazarsfeld to artificial neural networks. In: Vittoriom, C., Buscema, M., Contucci, L., d’Amore, B. (eds.) Applications of Mathematics in Models, Artificial Neural Networks and Arts. Springer, Dordrecht (2010)
Chapter Google Scholar
Carnap, R.: Logical Foundations of Probability. Chicago University Press, Chicago (1950)
Carnap, R.: The methodological character of theoretical concepts. In: Feigl, H., Scriven, M. (eds.) Minnesota Studies in the Philosophy of Science, vol. 1. University of Minnesota Press, Minneapolis (1956)
Carnap, R.: Philosophical Foundations of Physics. Basic Books, New York (1966)
Caws, P.: Definition and measurement in physics. In: West Churchman, C., Ratoosch, P. (eds.) Measurement: Definition and Theories. Wiley, New York (1959)
Coombs, C.H.: A Theory of Data. Wiley, New York (1964)
Ellis, B.: Basic Concepts of Measurement. Cambridge University Press, Cambridge (1966)
Euclide: Elementi, In: Acerbi, F. (ed.) Bompiani, Milano (2007)
Ferguson, A., et al.: Quantitative estimates of sensory events. Adv. Sci. 2 , 33 (1940)
Finkelstein, L.: Widely, strongly and weakly defined measurement. Measurement 34 (1), 1–74 (2003)
Article Google Scholar
Finkelstein, L.: Widely-defined measurement—an analysis of challenges. Measurement 42 (9), 1270–1277 (2009)
Frigerio, A., Giordani, A., Mari, L.: Outline of a general model of measurement. Synthese 175 (2), 123–149 (2010)
George, B., Borgatta, E.F. (eds.): Social Measurement. Sage, Beverly Hills (1981)
Grether, D.M.: On the use of ordinal data in correlation analysis. Am. Soc. Rev. XLIV , 851–857 (1979)
Guttman, L.A.: The basis for scalogram analysis. In: Stouffer, S.A. (ed.) Measurement and Prediction. Princeton University Press, Princeton (1950)
Hand, D.J.: Measurement: Theory and Practice. The World through Quantification. Arnold, London (2004)
Hempel, C.G.: Fundamentals of Concept Formation in Empirical Science. The University of Chicago Press, Chicago (1952)
Henry, A.F.: Multivariate analysis and ordinal data. Am. Soc. Rev. XLVII , 299–307 (1982)
Ifrah, G.: The Universal History of Numbers. Harvill Press, London (1998)
Kaplan, A.: The Conduct of Inquiry. Chandler, San Francisco (1964)
Koyré, A.: Du mond de l’à-peu-près à l’univers de la precision. In: Koyré, A. (ed.) Études d’histoire de la pensée philsophique. Colin, Paris (1961)
Krantz, D.H., Duncan Luce, R., Suppes, P., Tversky, A.: Foundations of Measurement. Volume I Additive and Polynomial Representations [1971]. Dover Publication, New York (2007)
Kyngdon, A.: The Rasch model for the perspective of the representational theory of measurement. Theory Psychol. 18 , 119–124 (2008)
Labovitz, S.: The assignment of numbers to rank order categories. Am. Sociol. Rev. XXXV , 515–524 (1970)
Lucas, S.R., Szatrowski, A.: Qualitative comparative analysis in critical perspective. Sociol. Methodol. 44 (1), 1–79 (2014)
Luce, R.D., Tukey, J.W.: Simultaneous conjoint measurement: a new type of fundamental measurement. J. Math. Psychol. 1 , 1–27 (1964)
Luce, R.D., Krantz, D.H., Suppes, P., Tversky, A.: Foundations of Measurement. Volume III: Representation, Axiomatization, and Invariance [1990]. Dover Publication, New York (2007)
Mari, L.: Epistemology of measurement. Measurement 34 (1), 17–30 (2003)
Mari, L.: The problem of foundations of measurement. Measurement 38 (4), 259–266 (2005)
Mari, L., Zingales, G.: Uncertainty in measurement science. In: Karija, K., Finkelstein, L. (eds.) Measurement Science—A Discussion. Ohmsha, Tokio (2000)
Mari, L., Giordani, A.: Modeling measurement: error and uncertainty. In: Boumans, M., Hon, G., Petersen, A. (eds.) Error and uncertainty in scientific practice. Taylor & Francis, London (2013)
Martin, O.: Les origines philosophiques et scientifiques de la théorie representationelle de la mesure (1930–50). In: Leplège, A., Picavet, E. (eds.) Epistemology of Measurement in the Social Sciences. Social Science Information, vol. 42 (2003)
McDonald, R.P.: Test Theory: A Unified Treatment. LEA, Mahwah (1999)
McNaught, et al.: IUPAC. Compendium of Chemical Terminology. Blackwell Scientific Publications, Oxford (2014)
Merton, R.K., Sills, D.L., Stigler, S.M.: The Kelvin Dictum and Social Science: an Excursion into the History of an Idea. J. Hist. Behav. Sci. 20 , 319–331 (1984)
Michell, J.: Measurement scales and statistics: a clash of paradigms. Psychol. Bull. 100 (3), 398–407 (1986)
Michell, J.: Measurement in Psychology: A Critical History of a Methodological Concept. Cambridge University Press, Cambridge (1999)
Book Google Scholar
Michell, J.: Epistemology of measurement: the relevance of its history for quantification in the social sciences. In: Leplège, A., Picavet, E. (eds.) Epistemology of Measurement in the Social Sciences. Social Science Information, vol. 42 (2003)
Michell, J.: Conjoint measurement and the Rasch paradox: a response to Kyngdon. Theory Psychol. 18 (1), 125–131 (2008)
Narens, L.: On the scales of measurement. J. Math. Psychol. 24 , 249–275 (1981)
Nunnally, J.C.: Psychometric Theory. McGraw-Hill, New York (1978)
O’Brien, R.M.: The relationship between ordinal measure and their underlying values: why all the disagreement? Qual. Quant. XIX (3), 265–267 (1985)
Pap, A.: An Introduction to the Philosophy of Science. The Free Press of Glencoe, New York (1962)
Pfanzagl, J.: Theory of Measurement. Wiley, Oxford (1968)
Ragin, C.C.: The Comparative Method: Moving beyond Qualitative and Quantitative Strategies. University of California Press, Berkley (1987)
Rasch, G.: On specific objectivity. In: Glevgad, M. (ed.) The Danish Yearbook of Philosophy. Munksgaard, Copenhagen (1977)
Redlich, O.: Intensive and extensive properties. J. Chem. Educ. 47 (2), 154 (1970)
Rudin, W.: Real and Complex Analysis. McGraw Hill, New York (1970)
Russell, B.: The Principles of Mathematics. Cambridge University Press, Cambridge (1903)
Sartori, G.: Concept misformation in comparative politics. Am. Polit. Sci. Rev. LXIV , 1033–1053 (1970)
Sartori, G.: La politica comparata: premesse e problemi. Riv. Ital. Sci. Polit. I (1), 7–37 (1971)
Sartori, G.: Tower of babel. In: Sartori, G., Riggs, F.W., Teune, H. (eds.) Tower of Babel: On the Definition and Analysis of Concepts in the Social Sciences. Occasional Paper 8. International Studies Association (1975)
Stevens, S.S.: On the theory of scales of measurement. Science 103 , 677–680 (1946)
Stevens, S.S.: Mathematics, measurement and psychophysics. In: Stevens, S.S. (ed.) Handbook of Experimental Psychology. Wiley, New York (1951)
Stevens, S.S.: Measurement, psychophysics, and utility. In: West Churchman, C., Ratoosh, P. (eds.) Measurement: Definitions and Theories. Wiley, New York (1959)
Suppes, P., Krantz, D.H., Duncan Luce, R., Amos, T.: Foundations of Measurement. Volume II: Geometrical. Threshold, and Probabilistic Representations [1989]. Dover Publication, New York (2007)
Tal, E.: Measurement in Science. In: Edward, N.Z. (ed.) The Stanford Encyclopedia of Philosophy (2015). http://plato.stanford.edu/archives/sum2015/entries/measurement-science/
Thurstone, L.L.: The measurement of social attitudes. J. Abnorm. Soc. Psychol. XXVI (2), 249–269 (1931)
Torgerson, W.S.: Theory and Method of Scaling. Wiley, New York (1958)
Velleman, P.F., Wilkinson, L.: Nominal, Ordinal, Interval, and Ratio Typologies Are Misleading. The American Statistician 47 (1), 65–72 (1993)
Wilson, T.P.: Critique of ordinal variable. Soc. Forces 49 (3), 432–444 (1971)
Wright, G.H.V.: A Treatise on Induction and Probability. Routledge and Kegan, London (1951)
Young, F.W.: Quantitative analysis of qualitative data. Psychometrika XLVI (4), 357–388 (1981)
Download references
Author information
Authors and affiliations.
University of Florence, Via delle Pandette 21, 50127, Florence, Italy
Alessandro Bruschi
You can also search for this author in PubMed Google Scholar
Corresponding author
Correspondence to Alessandro Bruschi .
Rights and permissions
Reprints and permissions
About this article
Bruschi, A. Measurement in social research: some misunderstandings. Qual Quant 51 , 2219–2243 (2017). https://doi.org/10.1007/s11135-016-0383-5
Download citation
Published : 15 July 2016
Issue Date : September 2017
DOI : https://doi.org/10.1007/s11135-016-0383-5
Share this article
Anyone you share the following link with will be able to read this content:
Sorry, a shareable link is not currently available for this article.
Provided by the Springer Nature SharedIt content-sharing initiative
- Foundation of measurement
- Representational theory
- Qualitative measurement
- Natural and social sciences measurement
- Find a journal
- Publish with us
- Track your research
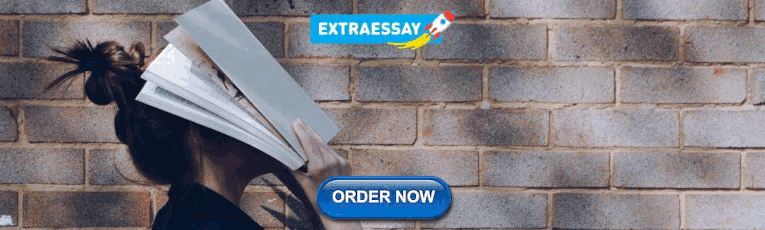
IMAGES
VIDEO
COMMENTS
The research methods you use depend on the type of data you need to answer your research question. If you want to measure something or test a hypothesis, use quantitative methods. If you want to explore ideas, thoughts and meanings, use qualitative methods. If you want to analyze a large amount of readily-available data, use secondary data.
Quantitative research is a way to learn about a particular group of people, known as a sample population. Using scientific inquiry, quantitative research relies on data that are observed or measured to examine questions about the sample population. Allen, M. (2017). The SAGE encyclopedia of communication research methods (Vols. 1-4). Thousand ...
Measurements in Quantitative Research: How to Select and Report on Research Instruments. ONF 2014, 41 (4), 431-433. DOI: 10.1188/14.ONF.431-433. Measures exist to numerically represent degrees of attributes. Quantitative research is based on measurement and is conducted in a systematic, controlled manner.
Quantitative research is based on measurement and is conducted in a systematic, controlled manner. These measures enable researchers to perform statistical tests, analyze differences between groups, and determine the effectiveness of treatments. If something is not measurable, it cannot be tested. Keywords: measurements; quantitative research ...
T eresa L. Hagan, BSN, BA, RN. Measurements in Quantitative Research: How to Select and Report on Research Instruments. ONF, 41 (4), 431-433. doi: 10.1188/14.ONF .431-433. M easures exist to ...
Mixed-methods research is a flexible approach, where the research design is determined by what we want to find out rather than by any predetermined epistemological position. In mixed-methods research, qualitative or quantitative components can predominate, or both can have equal status. 1.4. Units and variables.
Organisational analysts may use time-and-motion studies to assess how much time workers spend doing particular components of a work task. Educational researchers may measure the amount of time children spend reading portions of printed texts. In each of these instances, the time-based measures do constitute precise ratio scale measures.
Recognizing this complexity, statisticians have defined four basic groups of measures, or levels of measurement, based on the amount of information that each takes advantage of. The four are generally seen as occupying different positions, or levels, on a ladder of measurement (see Fig. 2.1 ): nominal, ordinal, interval, and ratio.
Measurement is central to empirical research whether observational or experimental. A study of a novel, well-defined research question can fall apart due to inappropriate measurement. Measurement is defined in a variety of ways (Last 2001; Thorndike 2007; Manoj and Lingyak 2014 ), yet common to all definitions is the systematic application of ...
Quantitative research methods involve the collection of numerical data and the use of statistical analysis to draw conclusions. This method is suitable for research questions that aim to measure the relationship between variables, test hypotheses, and make predictions. Here are some tips for choosing quantitative research methods:
Abstract. Evaluating individual research performance is a complex task that ideally examines productivity, scientific impact, and research quality--a task that metrics alone have been unable to achieve. In January 2011, the French Academy of Sciences published a report on current bibliometric (citation metric) methods for evaluating ...
Go to: Abstract. The current push for rigor and reproducibility is driven by a desire for confidence in research results. Here, we suggest a framework for a systematic process, based on consensus principles of measurement science, to guide researchers and reviewers in assessing, documenting, and mitigating the sources of uncertainty in a study.
1. Introduction. A science of science is flourishing in all disciplines and promises to boost discovery on all research fronts [].Commonly branded 'meta-science' or 'meta-research', this rapidly expanding literature of empirical studies, experiments, interventions and theoretical models explicitly aims to take a 'bird's eye view' of science and a decidedly cross-disciplinary ...
It is widely acknowledged that in the current academic landscape, publishing is the primary measure for assessing a researcher's value. This is manifested by associating individuals' academic performance with different types of metrics, typically the number of publications or citations (quantity), rather than with the content of their works (quality). Concerns have been raised that this ...
This chapter begins the exploration of quantitative studies in detail. Chapters 6 through 13 address the design of studies, along with how to develop measurement procedures to collect data and how subsequently to analyze the data collected. The methods introduced relate directly to the comparison-based, objectives-based, and decision-facilitation approaches to evaluation described in Chap. 2.
Applications for grant funding or career advancement may require an indication of both the quantity of your research output and of the quality of your research. Research impact measurement may be calculated using researcher specific metrics such as the h-index, or by quantitative methods such as citation counts or journal impact factors.
Abstract. The need to reform research assessment processes related to career advancement at research institutions has become increasingly recognized in recent years, especially to better foster open and responsible research practices. Current assessment criteria are believed to focus too heavily on inappropriate criteria related to productivity and quantity as opposed to quality, collaborative ...
Quality Matters - Research Design, Magnitude and Effect Sizes. The keystone of evidence-based practices (EPBs) is research. Through research, we are able to create a solid foundation for which an EPB can stand on. However, not all research is created equal. There are studies that are the cornerstone for other researchers, studies of such high-quality that they can be cited and reference with...
Chapter 4 details the challenges of using existing metrics and existing data, even data from large-scale programs such as Science and Technology for America's Reinvestment: Measuring the Effect of Research on Innovation, Competitiveness and Science (STAR METRICS), to measure research impacts and quality. Despite these challenges, a number of attempts have been made to make these measurements ...
Research Design MCQ with answers, Research design multiple choice questions for BBA, B Com, MBA, BMS, Ph D, researchers for UGC NET, UPSC ... _____research is based on the measurement of quantity or amount. A. Qualitative. B. Descriptive. C. Quantitative. D. Numerical. View Answer. ... _____ is data-based, coming up with conclusions that are ...
The definitions of <measurement>—"process of experimentally obtaining one or more quantity values that can reasonably be attributed to a quantity" (JCGM, 2012a: 2.1)—and <measurement procedure>—"detailed description of a measurement according to one or more measurement principles and to a given measurement method, based on a ...
Reducing food waste in the student population is important for promoting sustainable economic, social, and ecological development. In this paper, with the help of CiteSpace software (versions 6.1.R6 and 6.2.R4), we visually analyze the literature related to the food waste of students in the WoS core collection database. It is found that (1) scholars are paying increasing attention to the field ...
McqMate.com is an educational platform, Which is developed BY STUDENTS, FOR STUDENTS, The main objective of our platform is to assist fellow students in preparing for ...
The concept of numerical measurement based on the manipulation of objects has impoverished and distorted the meaning of magnitude and scale. This article aims to contribute to a concept of the most fruitful measurement for the development of the social sciences, with reference to specific aspects indicating the differences from the natural one and regarding, in particular, the non-numeric.