
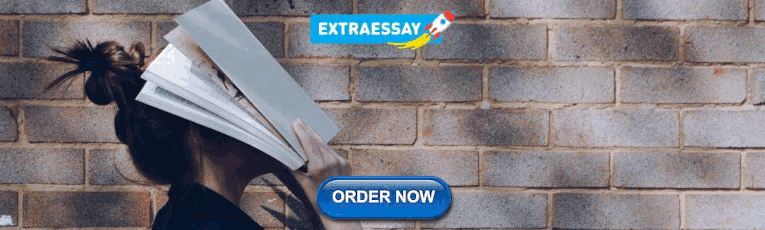
Case Study Methods and Examples
By Janet Salmons, PhD Manager, Sage Research Methods Community
What is Case Study Methodology ?
Case studies in research are both unique and uniquely confusing. The term case study is confusing because the same term is used multiple ways. The term can refer to the methodology, that is, a system of frameworks used to design a study, or the methods used to conduct it. Or, case study can refer to a type of academic writing that typically delves into a problem, process, or situation.
Case study methodology can entail the study of one or more "cases," that could be described as instances, examples, or settings where the problem or phenomenon can be examined. The researcher is tasked with defining the parameters of the case, that is, what is included and excluded. This process is called bounding the case , or setting boundaries.
Case study can be combined with other methodologies, such as ethnography, grounded theory, or phenomenology. In such studies the research on the case uses another framework to further define the study and refine the approach.
Case study is also described as a method, given particular approaches used to collect and analyze data. Case study research is conducted by almost every social science discipline: business, education, sociology, psychology. Case study research, with its reliance on multiple sources, is also a natural choice for researchers interested in trans-, inter-, or cross-disciplinary studies.
The Encyclopedia of case study research provides an overview:
The purpose of case study research is twofold: (1) to provide descriptive information and (2) to suggest theoretical relevance. Rich description enables an in-depth or sharpened understanding of the case.
It is unique given one characteristic: case studies draw from more than one data source. Case studies are inherently multimodal or mixed methods because this they use either more than one form of data within a research paradigm, or more than one form of data from different paradigms.
A case study inquiry could include multiple types of data:
multiple forms of quantitative data sources, such as Big Data + a survey
multiple forms of qualitative data sources, such as interviews + observations + documents
multiple forms of quantitative and qualitative data sources, such as surveys + interviews
Case study methodology can be used to achieve different research purposes.
Robert Yin , methodologist most associated with case study research, differentiates between descriptive , exploratory and explanatory case studies:
Descriptive : A case study whose purpose is to describe a phenomenon. Explanatory : A case study whose purpose is to explain how or why some condition came to be, or why some sequence of events occurred or did not occur. Exploratory: A case study whose purpose is to identify the research questions or procedures to be used in a subsequent study.
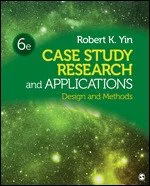
Robert Yin’s book is a comprehensive guide for case study researchers!
You can read the preface and Chapter 1 of Yin's book here . See the open-access articles below for some published examples of qualitative, quantitative, and mixed methods case study research.
Mills, A. J., Durepos, G., & Wiebe, E. (2010). Encyclopedia of case study research (Vols. 1-0). Thousand Oaks, CA: SAGE Publications, Inc. doi: 10.4135/9781412957397
Yin, R. K. (2018). Case study research and applications (6th ed.). Thousand Oaks: SAGE Publications.
Open-Access Articles Using Case Study Methodology
As you can see from this collection, case study methods are used in qualitative, quantitative and mixed methods research.
Ang, C.-S., Lee, K.-F., & Dipolog-Ubanan, G. F. (2019). Determinants of First-Year Student Identity and Satisfaction in Higher Education: A Quantitative Case Study. SAGE Open. https://doi.org/10.1177/2158244019846689
Abstract. First-year undergraduates’ expectations and experience of university and student engagement variables were investigated to determine how these perceptions influence their student identity and overall course satisfaction. Data collected from 554 first-year undergraduates at a large private university were analyzed. Participants were given the adapted version of the Melbourne Centre for the Study of Higher Education Survey to self-report their learning experience and engagement in the university community. The results showed that, in general, the students’ reasons of pursuing tertiary education were to open the door to career opportunities and skill development. Moreover, students’ views on their learning and university engagement were at the moderate level. In relation to student identity and overall student satisfaction, it is encouraging to state that their perceptions of studentship and course satisfaction were rather positive. After controlling for demographics, student engagement appeared to explain more variance in student identity, whereas students’ expectations and experience explained greater variance in students’ overall course satisfaction. Implications for practice, limitations, and recommendation of this study are addressed.
Baker, A. J. (2017). Algorithms to Assess Music Cities: Case Study—Melbourne as a Music Capital. SAGE Open. https://doi.org/10.1177/2158244017691801
Abstract. The global Mastering of a Music City report in 2015 notes that the concept of music cities has penetrated the global political vernacular because it delivers “significant economic, employment, cultural and social benefits.” This article highlights that no empirical study has combined all these values and offers a relevant and comprehensive definition of a music city. Drawing on industry research,1 the article assesses how mathematical flowcharts, such as Algorithm A (Economics), Algorithm B (Four T’s creative index), and Algorithm C (Heritage), have contributed to the definition of a music city. Taking Melbourne as a case study, it illustrates how Algorithms A and B are used as disputed evidence about whether the city is touted as Australia’s music capital. The article connects the three algorithms to an academic framework from musicology, urban studies, cultural economics, and sociology, and proposes a benchmark Algorithm D (Music Cities definition), which offers a more holistic assessment of music activity in any urban context. The article concludes by arguing that Algorithm D offers a much-needed definition of what comprises a music city because it builds on the popular political economy focus and includes the social importance of space and cultural practices.
Brown, K., & Mondon, A. (2020). Populism, the media, and the mainstreaming of the far right: The Guardian’s coverage of populism as a case study. Politics. https://doi.org/10.1177/0263395720955036
Abstract. Populism seems to define our current political age. The term is splashed across the headlines, brandished in political speeches and commentaries, and applied extensively in numerous academic publications and conferences. This pervasive usage, or populist hype, has serious implications for our understanding of the meaning of populism itself and for our interpretation of the phenomena to which it is applied. In particular, we argue that its common conflation with far-right politics, as well as its breadth of application to other phenomena, has contributed to the mainstreaming of the far right in three main ways: (1) agenda-setting power and deflection, (2) euphemisation and trivialisation, and (3) amplification. Through a mixed-methods approach to discourse analysis, this article uses The Guardian newspaper as a case study to explore the development of the populist hype and the detrimental effects of the logics that it has pushed in public discourse.
Droy, L. T., Goodwin, J., & O’Connor, H. (2020). Methodological Uncertainty and Multi-Strategy Analysis: Case Study of the Long-Term Effects of Government Sponsored Youth Training on Occupational Mobility. Bulletin of Sociological Methodology/Bulletin de Méthodologie Sociologique, 147–148(1–2), 200–230. https://doi.org/10.1177/0759106320939893
Abstract. Sociological practitioners often face considerable methodological uncertainty when undertaking a quantitative analysis. This methodological uncertainty encompasses both data construction (e.g. defining variables) and analysis (e.g. selecting and specifying a modelling procedure). Methodological uncertainty can lead to results that are fragile and arbitrary. Yet, many practitioners may be unaware of the potential scale of methodological uncertainty in quantitative analysis, and the recent emergence of techniques for addressing it. Recent proposals for ‘multi-strategy’ approaches seek to identify and manage methodological uncertainty in quantitative analysis. We present a case-study of a multi-strategy analysis, applied to the problem of estimating the long-term impact of 1980s UK government-sponsored youth training. We use this case study to further highlight the problem of cumulative methodological fragilities in applied quantitative sociology and to discuss and help develop multi-strategy analysis as a tool to address them.
Ebneyamini, S., & Sadeghi Moghadam, M. R. (2018). Toward Developing a Framework for Conducting Case Study Research . International Journal of Qualitative Methods . https://doi.org/10.1177/1609406918817954
Abstract. This article reviews the use of case study research for both practical and theoretical issues especially in management field with the emphasis on management of technology and innovation. Many researchers commented on the methodological issues of the case study research from their point of view thus, presenting a comprehensive framework was missing. We try representing a general framework with methodological and analytical perspective to design, develop, and conduct case study research. To test the coverage of our framework, we have analyzed articles in three major journals related to the management of technology and innovation to approve our framework. This study represents a general structure to guide, design, and fulfill a case study research with levels and steps necessary for researchers to use in their research.
Lai, D., & Roccu, R. (2019). Case study research and critical IR: the case for the extended case methodology. International Relations , 33 (1), 67-87. https://doi.org/10.1177/0047117818818243
Abstract. Discussions on case study methodology in International Relations (IR) have historically been dominated by positivist and neopositivist approaches. However, these are problematic for critical IR research, pointing to the need for a non-positivist case study methodology. To address this issue, this article introduces and adapts the extended case methodology as a critical, reflexivist approach to case study research, whereby the case is constructed through a dynamic interaction with theory, rather than selected, and knowledge is produced through extensions rather than generalisation. Insofar as it seeks to study the world in complex and non-linear terms, take context and positionality seriously, and generate explicitly political and emancipatory knowledge, the extended case methodology is consistent with the ontological and epistemological commitments of several critical IR approaches. Its potential is illustrated in the final part of the article with reference to researching the socioeconomic dimension of transitional justice in Bosnia and Herzegovina.
Lynch, R., Young, J. C., Boakye-Achampong, S., Jowaisas, C., Sam, J., & Norlander, B. (2020). Benefits of crowdsourcing for libraries: A case study from Africa . IFLA Journal. https://doi.org/10.1177/0340035220944940
Abstract. Many libraries in the Global South do not collect comprehensive data about themselves, which creates challenges in terms of local and international visibility. Crowdsourcing is an effective tool that engages the public to collect missing data, and it has proven to be particularly valuable in countries where governments collect little public data. Whereas crowdsourcing is often used within fields that have high levels of development funding, such as health, the authors believe that this approach would have many benefits for the library field as well. They present qualitative and quantitative evidence from 23 African countries involved in a crowdsourcing project to map libraries. The authors find benefits in terms of increased connections between stakeholders, capacity-building, and increased local visibility. These findings demonstrate the potential of crowdsourced approaches for tasks such as mapping to benefit libraries and similarly positioned institutions in the Global South in multifaceted ways.
Mason, W., Morris, K., Webb, C., Daniels, B., Featherstone, B., Bywaters, P., Mirza, N., Hooper, J., Brady, G., Bunting, L., & Scourfield, J. (2020). Toward Full Integration of Quantitative and Qualitative Methods in Case Study Research: Insights From Investigating Child Welfare Inequalities. Journal of Mixed Methods Research, 14 (2), 164-183. https://doi.org/10.1177/1558689819857972
Abstract. Delineation of the full integration of quantitative and qualitative methods throughout all stages of multisite mixed methods case study projects remains a gap in the methodological literature. This article offers advances to the field of mixed methods by detailing the application and integration of mixed methods throughout all stages of one such project; a study of child welfare inequalities. By offering a critical discussion of site selection and the management of confirmatory, expansionary and discordant data, this article contributes to the limited body of mixed methods exemplars specific to this field. We propose that our mixed methods approach provided distinctive insights into a complex social problem, offering expanded understandings of the relationship between poverty, child abuse, and neglect.
Rashid, Y., Rashid, A., Warraich, M. A., Sabir, S. S., & Waseem, A. (2019). Case Study Method: A Step-by-Step Guide for Business Researchers . International Journal of Qualitative Methods . https://doi.org/10.1177/1609406919862424
Abstract. Qualitative case study methodology enables researchers to conduct an in-depth exploration of intricate phenomena within some specific context. By keeping in mind research students, this article presents a systematic step-by-step guide to conduct a case study in the business discipline. Research students belonging to said discipline face issues in terms of clarity, selection, and operationalization of qualitative case study while doing their final dissertation. These issues often lead to confusion, wastage of valuable time, and wrong decisions that affect the overall outcome of the research. This article presents a checklist comprised of four phases, that is, foundation phase, prefield phase, field phase, and reporting phase. The objective of this article is to provide novice researchers with practical application of this checklist by linking all its four phases with the authors’ experiences and learning from recently conducted in-depth multiple case studies in the organizations of New Zealand. Rather than discussing case study in general, a targeted step-by-step plan with real-time research examples to conduct a case study is given.
VanWynsberghe, R., & Khan, S. (2007). Redefining Case Study. International Journal of Qualitative Methods, 80–94. https://doi.org/10.1177/160940690700600208
Abstract. In this paper the authors propose a more precise and encompassing definition of case study than is usually found. They support their definition by clarifying that case study is neither a method nor a methodology nor a research design as suggested by others. They use a case study prototype of their own design to propose common properties of case study and demonstrate how these properties support their definition. Next, they present several living myths about case study and refute them in relation to their definition. Finally, they discuss the interplay between the terms case study and unit of analysis to further delineate their definition of case study. The target audiences for this paper include case study researchers, research design and methods instructors, and graduate students interested in case study research.
More Sage Research Methods Community Posts about Case Study Research
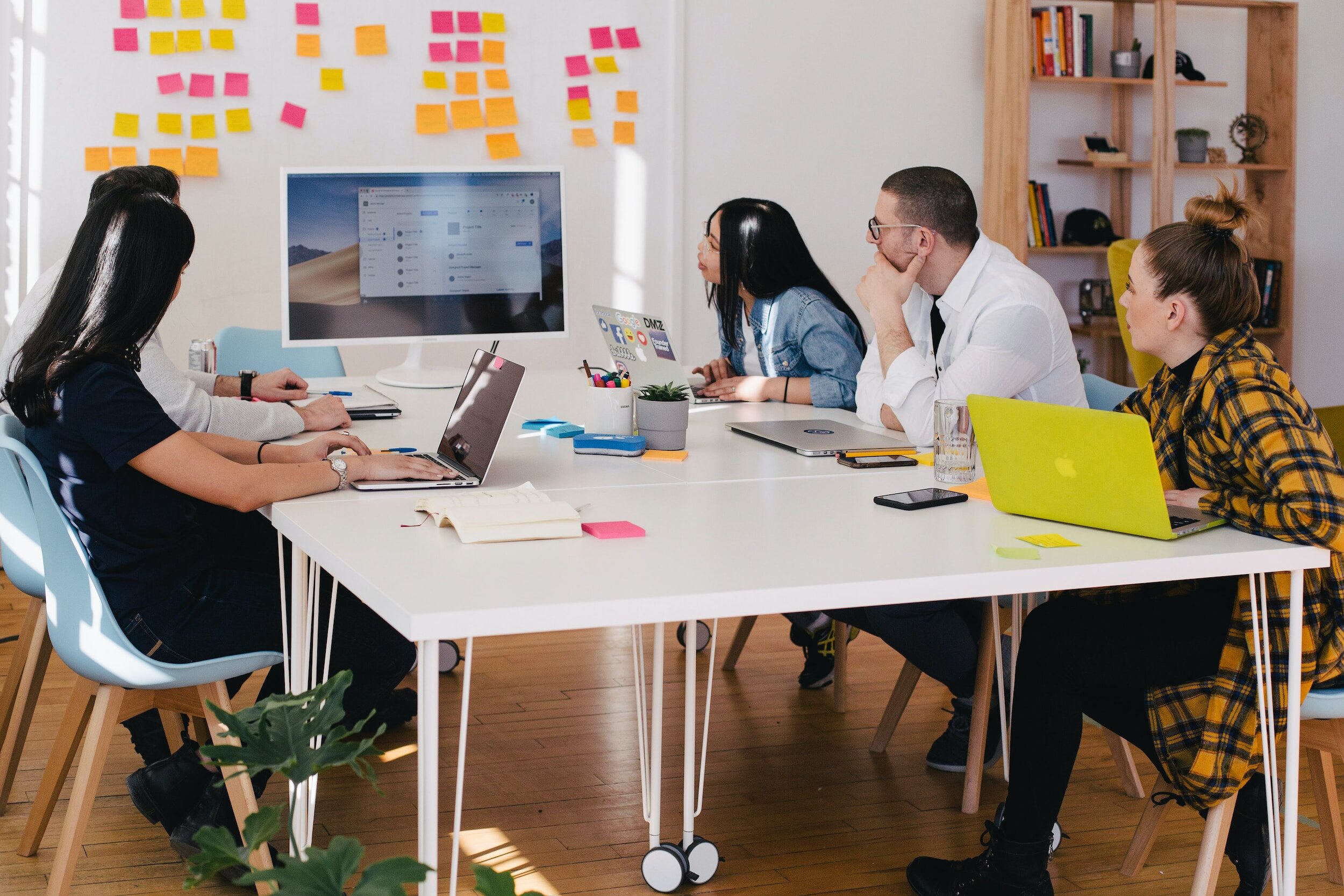
Use research cases as the basis for individual or team activities that build skills.
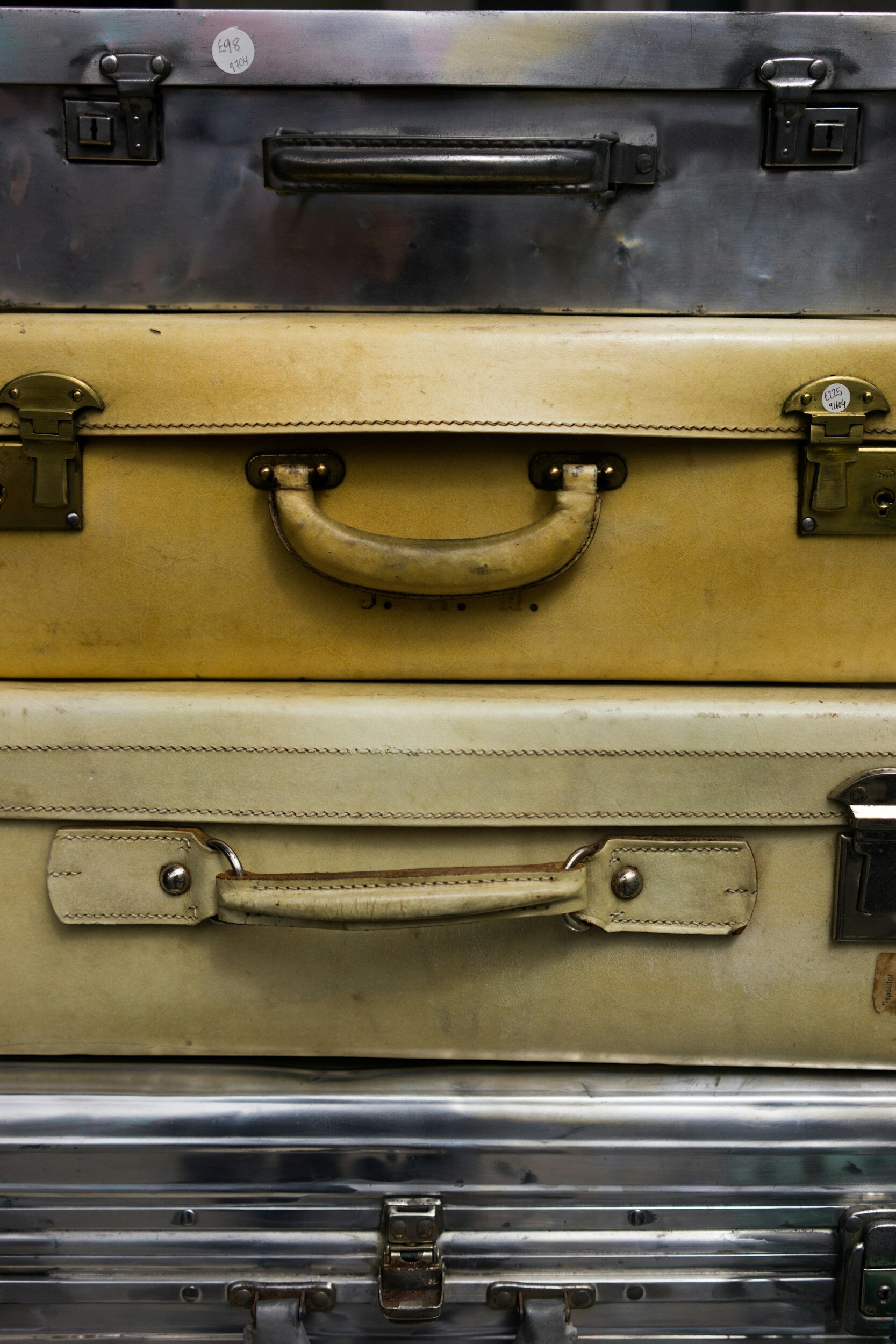
Find an 10-step process for using research cases to teach methods with learning activities for individual students, teams, or small groups. (Or use the approach yourself!)
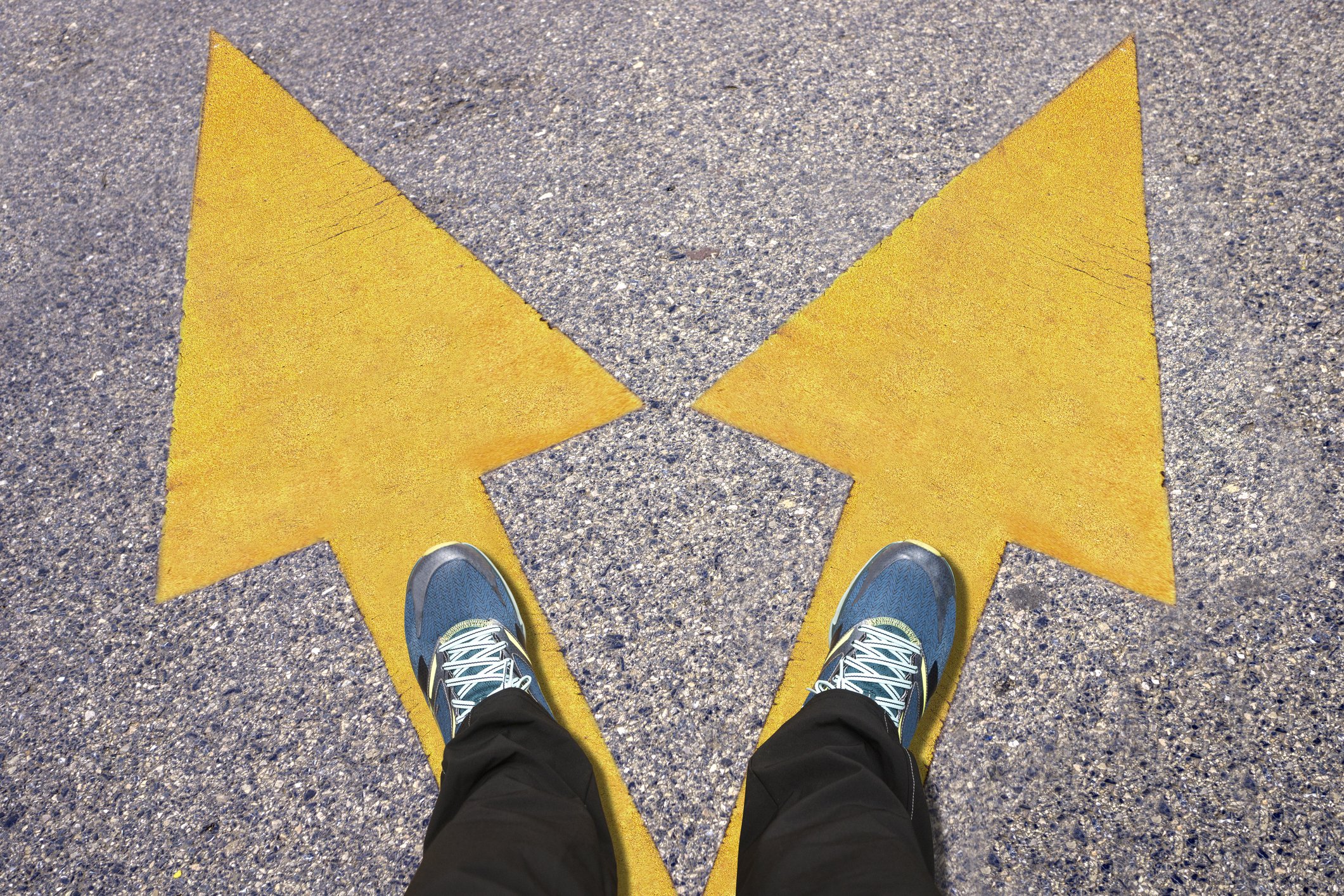
How do you decide which methodology fits your study? In this dialogue Linda Bloomberg and Janet Boberg explain the importance of a strategic approach to qualitative research design that stresses alignment with the purpose of the study.
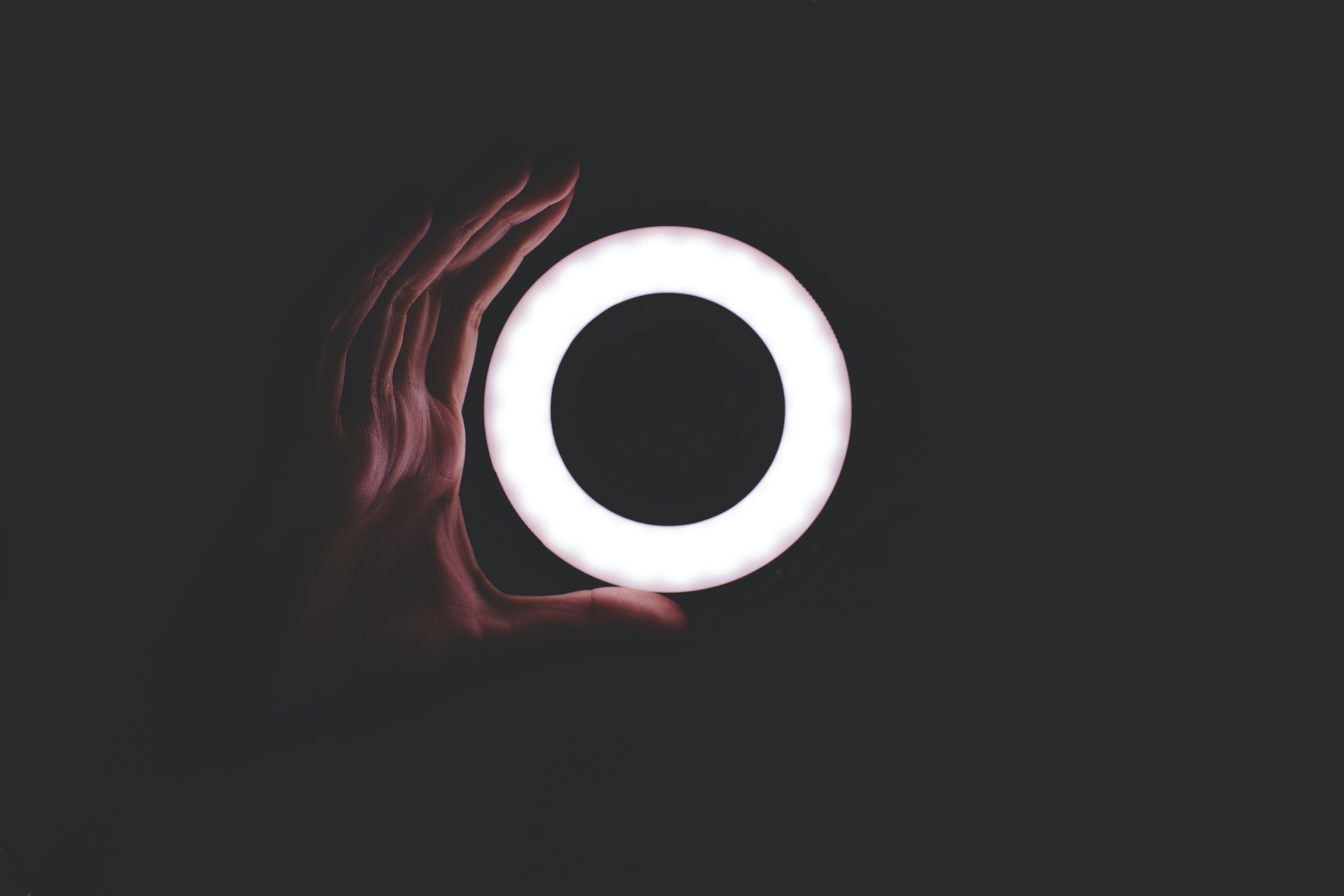
Case study methods are used by researchers in many disciplines. Here are some open-access articles about multimodal qualitative or mixed methods designs that include both qualitative and quantitative elements.
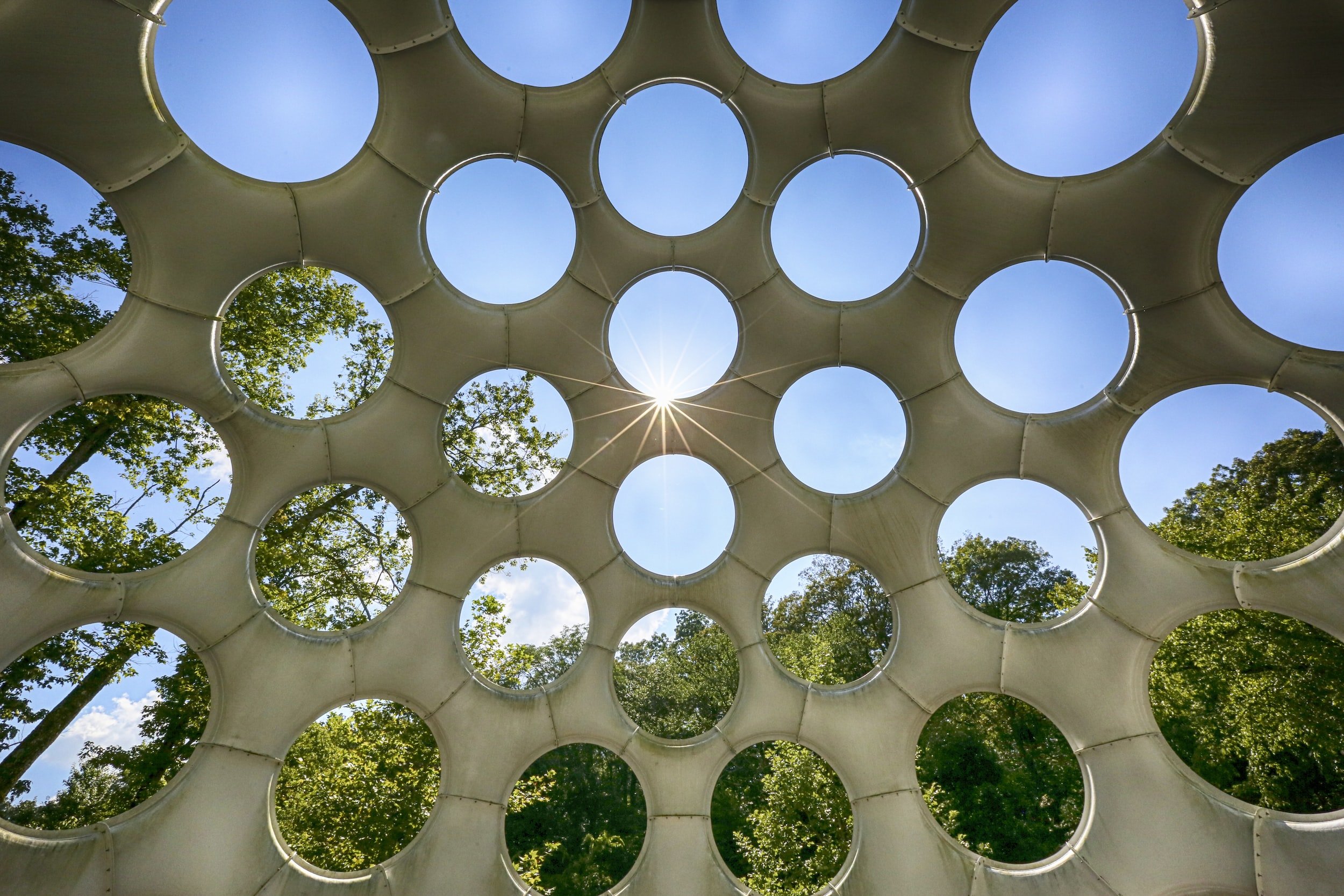
Case study methodology is both unique, and uniquely confusing. It is unique given one characteristic: case studies draw from more than one data source.
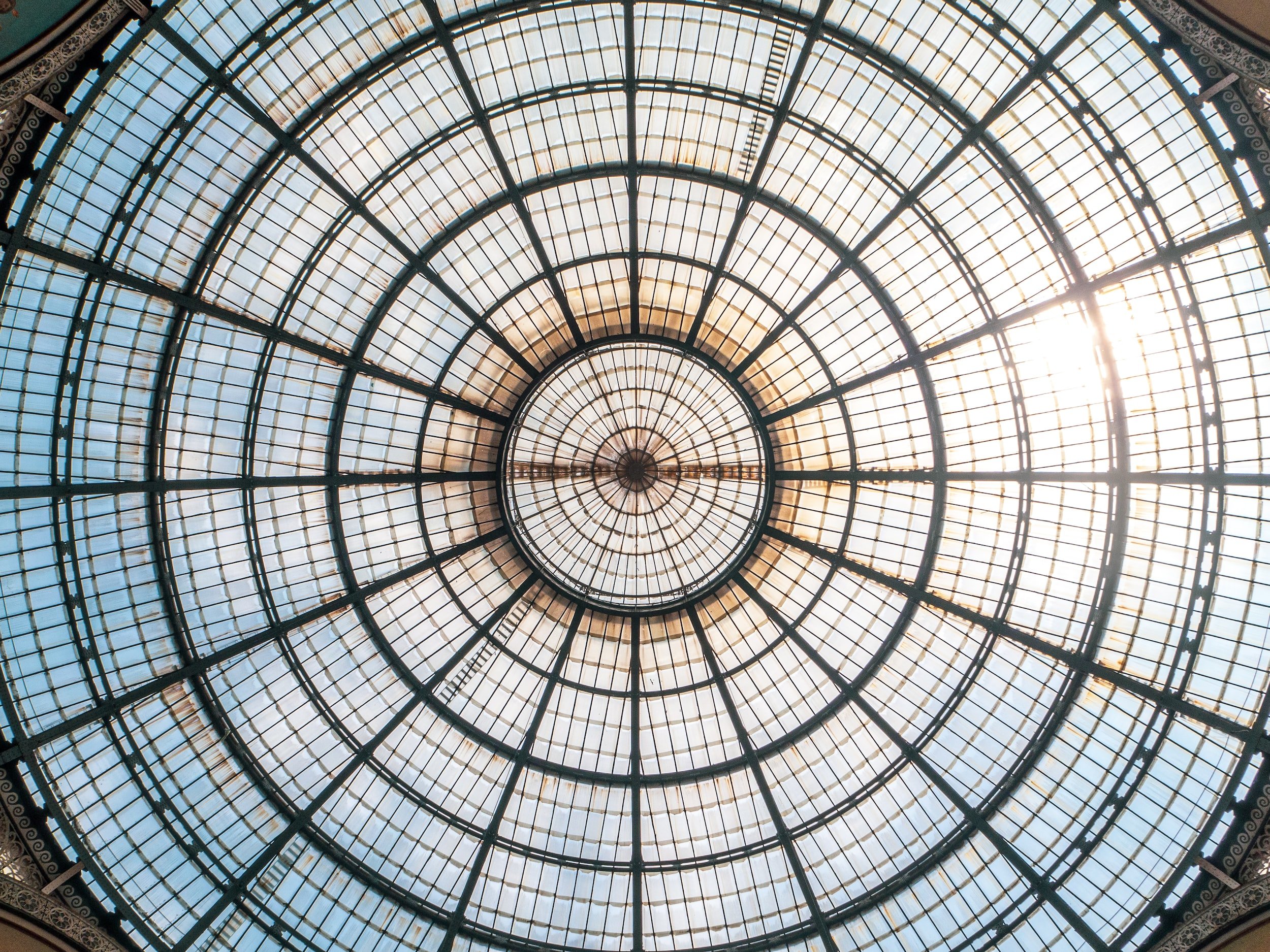
What is case study methodology? It is unique given one characteristic: case studies draw from more than one data source. In this post find definitions and a collection of multidisciplinary examples.
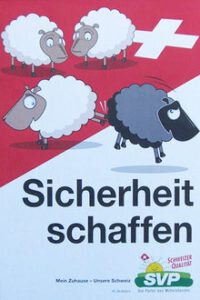
Find discussion of case studies and published examples.
Istanbul as a regional computational social science hub
Experiments and quantitative research.
- Privacy Policy

Home » Case Study – Methods, Examples and Guide
Case Study – Methods, Examples and Guide
Table of Contents

A case study is a research method that involves an in-depth examination and analysis of a particular phenomenon or case, such as an individual, organization, community, event, or situation.
It is a qualitative research approach that aims to provide a detailed and comprehensive understanding of the case being studied. Case studies typically involve multiple sources of data, including interviews, observations, documents, and artifacts, which are analyzed using various techniques, such as content analysis, thematic analysis, and grounded theory. The findings of a case study are often used to develop theories, inform policy or practice, or generate new research questions.
Types of Case Study
Types and Methods of Case Study are as follows:
Single-Case Study
A single-case study is an in-depth analysis of a single case. This type of case study is useful when the researcher wants to understand a specific phenomenon in detail.
For Example , A researcher might conduct a single-case study on a particular individual to understand their experiences with a particular health condition or a specific organization to explore their management practices. The researcher collects data from multiple sources, such as interviews, observations, and documents, and uses various techniques to analyze the data, such as content analysis or thematic analysis. The findings of a single-case study are often used to generate new research questions, develop theories, or inform policy or practice.
Multiple-Case Study
A multiple-case study involves the analysis of several cases that are similar in nature. This type of case study is useful when the researcher wants to identify similarities and differences between the cases.
For Example, a researcher might conduct a multiple-case study on several companies to explore the factors that contribute to their success or failure. The researcher collects data from each case, compares and contrasts the findings, and uses various techniques to analyze the data, such as comparative analysis or pattern-matching. The findings of a multiple-case study can be used to develop theories, inform policy or practice, or generate new research questions.
Exploratory Case Study
An exploratory case study is used to explore a new or understudied phenomenon. This type of case study is useful when the researcher wants to generate hypotheses or theories about the phenomenon.
For Example, a researcher might conduct an exploratory case study on a new technology to understand its potential impact on society. The researcher collects data from multiple sources, such as interviews, observations, and documents, and uses various techniques to analyze the data, such as grounded theory or content analysis. The findings of an exploratory case study can be used to generate new research questions, develop theories, or inform policy or practice.
Descriptive Case Study
A descriptive case study is used to describe a particular phenomenon in detail. This type of case study is useful when the researcher wants to provide a comprehensive account of the phenomenon.
For Example, a researcher might conduct a descriptive case study on a particular community to understand its social and economic characteristics. The researcher collects data from multiple sources, such as interviews, observations, and documents, and uses various techniques to analyze the data, such as content analysis or thematic analysis. The findings of a descriptive case study can be used to inform policy or practice or generate new research questions.
Instrumental Case Study
An instrumental case study is used to understand a particular phenomenon that is instrumental in achieving a particular goal. This type of case study is useful when the researcher wants to understand the role of the phenomenon in achieving the goal.
For Example, a researcher might conduct an instrumental case study on a particular policy to understand its impact on achieving a particular goal, such as reducing poverty. The researcher collects data from multiple sources, such as interviews, observations, and documents, and uses various techniques to analyze the data, such as content analysis or thematic analysis. The findings of an instrumental case study can be used to inform policy or practice or generate new research questions.
Case Study Data Collection Methods
Here are some common data collection methods for case studies:
Interviews involve asking questions to individuals who have knowledge or experience relevant to the case study. Interviews can be structured (where the same questions are asked to all participants) or unstructured (where the interviewer follows up on the responses with further questions). Interviews can be conducted in person, over the phone, or through video conferencing.
Observations
Observations involve watching and recording the behavior and activities of individuals or groups relevant to the case study. Observations can be participant (where the researcher actively participates in the activities) or non-participant (where the researcher observes from a distance). Observations can be recorded using notes, audio or video recordings, or photographs.
Documents can be used as a source of information for case studies. Documents can include reports, memos, emails, letters, and other written materials related to the case study. Documents can be collected from the case study participants or from public sources.
Surveys involve asking a set of questions to a sample of individuals relevant to the case study. Surveys can be administered in person, over the phone, through mail or email, or online. Surveys can be used to gather information on attitudes, opinions, or behaviors related to the case study.
Artifacts are physical objects relevant to the case study. Artifacts can include tools, equipment, products, or other objects that provide insights into the case study phenomenon.
How to conduct Case Study Research
Conducting a case study research involves several steps that need to be followed to ensure the quality and rigor of the study. Here are the steps to conduct case study research:
- Define the research questions: The first step in conducting a case study research is to define the research questions. The research questions should be specific, measurable, and relevant to the case study phenomenon under investigation.
- Select the case: The next step is to select the case or cases to be studied. The case should be relevant to the research questions and should provide rich and diverse data that can be used to answer the research questions.
- Collect data: Data can be collected using various methods, such as interviews, observations, documents, surveys, and artifacts. The data collection method should be selected based on the research questions and the nature of the case study phenomenon.
- Analyze the data: The data collected from the case study should be analyzed using various techniques, such as content analysis, thematic analysis, or grounded theory. The analysis should be guided by the research questions and should aim to provide insights and conclusions relevant to the research questions.
- Draw conclusions: The conclusions drawn from the case study should be based on the data analysis and should be relevant to the research questions. The conclusions should be supported by evidence and should be clearly stated.
- Validate the findings: The findings of the case study should be validated by reviewing the data and the analysis with participants or other experts in the field. This helps to ensure the validity and reliability of the findings.
- Write the report: The final step is to write the report of the case study research. The report should provide a clear description of the case study phenomenon, the research questions, the data collection methods, the data analysis, the findings, and the conclusions. The report should be written in a clear and concise manner and should follow the guidelines for academic writing.
Examples of Case Study
Here are some examples of case study research:
- The Hawthorne Studies : Conducted between 1924 and 1932, the Hawthorne Studies were a series of case studies conducted by Elton Mayo and his colleagues to examine the impact of work environment on employee productivity. The studies were conducted at the Hawthorne Works plant of the Western Electric Company in Chicago and included interviews, observations, and experiments.
- The Stanford Prison Experiment: Conducted in 1971, the Stanford Prison Experiment was a case study conducted by Philip Zimbardo to examine the psychological effects of power and authority. The study involved simulating a prison environment and assigning participants to the role of guards or prisoners. The study was controversial due to the ethical issues it raised.
- The Challenger Disaster: The Challenger Disaster was a case study conducted to examine the causes of the Space Shuttle Challenger explosion in 1986. The study included interviews, observations, and analysis of data to identify the technical, organizational, and cultural factors that contributed to the disaster.
- The Enron Scandal: The Enron Scandal was a case study conducted to examine the causes of the Enron Corporation’s bankruptcy in 2001. The study included interviews, analysis of financial data, and review of documents to identify the accounting practices, corporate culture, and ethical issues that led to the company’s downfall.
- The Fukushima Nuclear Disaster : The Fukushima Nuclear Disaster was a case study conducted to examine the causes of the nuclear accident that occurred at the Fukushima Daiichi Nuclear Power Plant in Japan in 2011. The study included interviews, analysis of data, and review of documents to identify the technical, organizational, and cultural factors that contributed to the disaster.
Application of Case Study
Case studies have a wide range of applications across various fields and industries. Here are some examples:
Business and Management
Case studies are widely used in business and management to examine real-life situations and develop problem-solving skills. Case studies can help students and professionals to develop a deep understanding of business concepts, theories, and best practices.
Case studies are used in healthcare to examine patient care, treatment options, and outcomes. Case studies can help healthcare professionals to develop critical thinking skills, diagnose complex medical conditions, and develop effective treatment plans.
Case studies are used in education to examine teaching and learning practices. Case studies can help educators to develop effective teaching strategies, evaluate student progress, and identify areas for improvement.
Social Sciences
Case studies are widely used in social sciences to examine human behavior, social phenomena, and cultural practices. Case studies can help researchers to develop theories, test hypotheses, and gain insights into complex social issues.
Law and Ethics
Case studies are used in law and ethics to examine legal and ethical dilemmas. Case studies can help lawyers, policymakers, and ethical professionals to develop critical thinking skills, analyze complex cases, and make informed decisions.
Purpose of Case Study
The purpose of a case study is to provide a detailed analysis of a specific phenomenon, issue, or problem in its real-life context. A case study is a qualitative research method that involves the in-depth exploration and analysis of a particular case, which can be an individual, group, organization, event, or community.
The primary purpose of a case study is to generate a comprehensive and nuanced understanding of the case, including its history, context, and dynamics. Case studies can help researchers to identify and examine the underlying factors, processes, and mechanisms that contribute to the case and its outcomes. This can help to develop a more accurate and detailed understanding of the case, which can inform future research, practice, or policy.
Case studies can also serve other purposes, including:
- Illustrating a theory or concept: Case studies can be used to illustrate and explain theoretical concepts and frameworks, providing concrete examples of how they can be applied in real-life situations.
- Developing hypotheses: Case studies can help to generate hypotheses about the causal relationships between different factors and outcomes, which can be tested through further research.
- Providing insight into complex issues: Case studies can provide insights into complex and multifaceted issues, which may be difficult to understand through other research methods.
- Informing practice or policy: Case studies can be used to inform practice or policy by identifying best practices, lessons learned, or areas for improvement.
Advantages of Case Study Research
There are several advantages of case study research, including:
- In-depth exploration: Case study research allows for a detailed exploration and analysis of a specific phenomenon, issue, or problem in its real-life context. This can provide a comprehensive understanding of the case and its dynamics, which may not be possible through other research methods.
- Rich data: Case study research can generate rich and detailed data, including qualitative data such as interviews, observations, and documents. This can provide a nuanced understanding of the case and its complexity.
- Holistic perspective: Case study research allows for a holistic perspective of the case, taking into account the various factors, processes, and mechanisms that contribute to the case and its outcomes. This can help to develop a more accurate and comprehensive understanding of the case.
- Theory development: Case study research can help to develop and refine theories and concepts by providing empirical evidence and concrete examples of how they can be applied in real-life situations.
- Practical application: Case study research can inform practice or policy by identifying best practices, lessons learned, or areas for improvement.
- Contextualization: Case study research takes into account the specific context in which the case is situated, which can help to understand how the case is influenced by the social, cultural, and historical factors of its environment.
Limitations of Case Study Research
There are several limitations of case study research, including:
- Limited generalizability : Case studies are typically focused on a single case or a small number of cases, which limits the generalizability of the findings. The unique characteristics of the case may not be applicable to other contexts or populations, which may limit the external validity of the research.
- Biased sampling: Case studies may rely on purposive or convenience sampling, which can introduce bias into the sample selection process. This may limit the representativeness of the sample and the generalizability of the findings.
- Subjectivity: Case studies rely on the interpretation of the researcher, which can introduce subjectivity into the analysis. The researcher’s own biases, assumptions, and perspectives may influence the findings, which may limit the objectivity of the research.
- Limited control: Case studies are typically conducted in naturalistic settings, which limits the control that the researcher has over the environment and the variables being studied. This may limit the ability to establish causal relationships between variables.
- Time-consuming: Case studies can be time-consuming to conduct, as they typically involve a detailed exploration and analysis of a specific case. This may limit the feasibility of conducting multiple case studies or conducting case studies in a timely manner.
- Resource-intensive: Case studies may require significant resources, including time, funding, and expertise. This may limit the ability of researchers to conduct case studies in resource-constrained settings.
About the author
Muhammad Hassan
Researcher, Academic Writer, Web developer
You may also like

Questionnaire – Definition, Types, and Examples

Observational Research – Methods and Guide

Quantitative Research – Methods, Types and...

Qualitative Research Methods

Explanatory Research – Types, Methods, Guide

Survey Research – Types, Methods, Examples
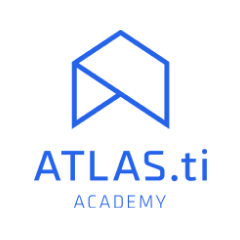
The Ultimate Guide to Qualitative Research - Part 1: The Basics
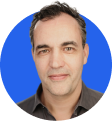
- Introduction and overview
- What is qualitative research?
- What is qualitative data?
- Examples of qualitative data
- Qualitative vs. quantitative research
- Mixed methods
- Qualitative research preparation
- Theoretical perspective
- Theoretical framework
- Literature reviews
Research question
- Conceptual framework
- Conceptual vs. theoretical framework
Data collection
- Qualitative research methods
- Focus groups
- Observational research
What is a case study?
Applications for case study research, what is a good case study, process of case study design, benefits and limitations of case studies.
- Ethnographical research
- Ethical considerations
- Confidentiality and privacy
- Power dynamics
- Reflexivity
Case studies
Case studies are essential to qualitative research , offering a lens through which researchers can investigate complex phenomena within their real-life contexts. This chapter explores the concept, purpose, applications, examples, and types of case studies and provides guidance on how to conduct case study research effectively.
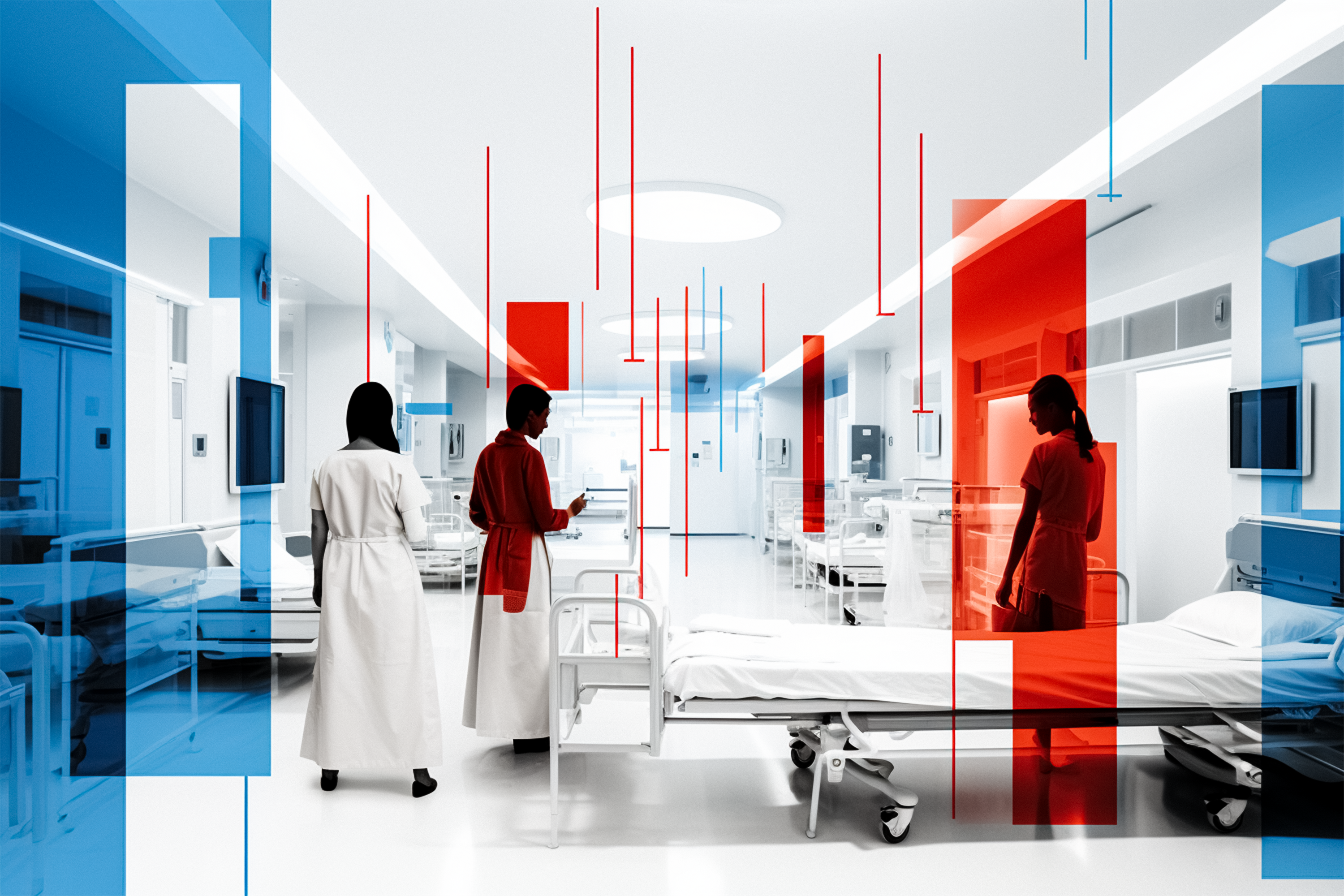
Whereas quantitative methods look at phenomena at scale, case study research looks at a concept or phenomenon in considerable detail. While analyzing a single case can help understand one perspective regarding the object of research inquiry, analyzing multiple cases can help obtain a more holistic sense of the topic or issue. Let's provide a basic definition of a case study, then explore its characteristics and role in the qualitative research process.
Definition of a case study
A case study in qualitative research is a strategy of inquiry that involves an in-depth investigation of a phenomenon within its real-world context. It provides researchers with the opportunity to acquire an in-depth understanding of intricate details that might not be as apparent or accessible through other methods of research. The specific case or cases being studied can be a single person, group, or organization – demarcating what constitutes a relevant case worth studying depends on the researcher and their research question .
Among qualitative research methods , a case study relies on multiple sources of evidence, such as documents, artifacts, interviews , or observations , to present a complete and nuanced understanding of the phenomenon under investigation. The objective is to illuminate the readers' understanding of the phenomenon beyond its abstract statistical or theoretical explanations.
Characteristics of case studies
Case studies typically possess a number of distinct characteristics that set them apart from other research methods. These characteristics include a focus on holistic description and explanation, flexibility in the design and data collection methods, reliance on multiple sources of evidence, and emphasis on the context in which the phenomenon occurs.
Furthermore, case studies can often involve a longitudinal examination of the case, meaning they study the case over a period of time. These characteristics allow case studies to yield comprehensive, in-depth, and richly contextualized insights about the phenomenon of interest.
The role of case studies in research
Case studies hold a unique position in the broader landscape of research methods aimed at theory development. They are instrumental when the primary research interest is to gain an intensive, detailed understanding of a phenomenon in its real-life context.
In addition, case studies can serve different purposes within research - they can be used for exploratory, descriptive, or explanatory purposes, depending on the research question and objectives. This flexibility and depth make case studies a valuable tool in the toolkit of qualitative researchers.
Remember, a well-conducted case study can offer a rich, insightful contribution to both academic and practical knowledge through theory development or theory verification, thus enhancing our understanding of complex phenomena in their real-world contexts.
What is the purpose of a case study?
Case study research aims for a more comprehensive understanding of phenomena, requiring various research methods to gather information for qualitative analysis . Ultimately, a case study can allow the researcher to gain insight into a particular object of inquiry and develop a theoretical framework relevant to the research inquiry.
Why use case studies in qualitative research?
Using case studies as a research strategy depends mainly on the nature of the research question and the researcher's access to the data.
Conducting case study research provides a level of detail and contextual richness that other research methods might not offer. They are beneficial when there's a need to understand complex social phenomena within their natural contexts.
The explanatory, exploratory, and descriptive roles of case studies
Case studies can take on various roles depending on the research objectives. They can be exploratory when the research aims to discover new phenomena or define new research questions; they are descriptive when the objective is to depict a phenomenon within its context in a detailed manner; and they can be explanatory if the goal is to understand specific relationships within the studied context. Thus, the versatility of case studies allows researchers to approach their topic from different angles, offering multiple ways to uncover and interpret the data .
The impact of case studies on knowledge development
Case studies play a significant role in knowledge development across various disciplines. Analysis of cases provides an avenue for researchers to explore phenomena within their context based on the collected data.
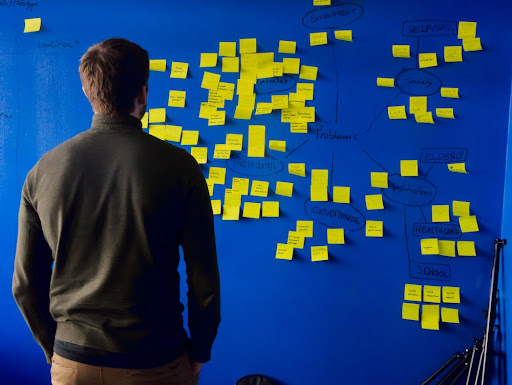
This can result in the production of rich, practical insights that can be instrumental in both theory-building and practice. Case studies allow researchers to delve into the intricacies and complexities of real-life situations, uncovering insights that might otherwise remain hidden.
Types of case studies
In qualitative research , a case study is not a one-size-fits-all approach. Depending on the nature of the research question and the specific objectives of the study, researchers might choose to use different types of case studies. These types differ in their focus, methodology, and the level of detail they provide about the phenomenon under investigation.
Understanding these types is crucial for selecting the most appropriate approach for your research project and effectively achieving your research goals. Let's briefly look at the main types of case studies.
Exploratory case studies
Exploratory case studies are typically conducted to develop a theory or framework around an understudied phenomenon. They can also serve as a precursor to a larger-scale research project. Exploratory case studies are useful when a researcher wants to identify the key issues or questions which can spur more extensive study or be used to develop propositions for further research. These case studies are characterized by flexibility, allowing researchers to explore various aspects of a phenomenon as they emerge, which can also form the foundation for subsequent studies.
Descriptive case studies
Descriptive case studies aim to provide a complete and accurate representation of a phenomenon or event within its context. These case studies are often based on an established theoretical framework, which guides how data is collected and analyzed. The researcher is concerned with describing the phenomenon in detail, as it occurs naturally, without trying to influence or manipulate it.
Explanatory case studies
Explanatory case studies are focused on explanation - they seek to clarify how or why certain phenomena occur. Often used in complex, real-life situations, they can be particularly valuable in clarifying causal relationships among concepts and understanding the interplay between different factors within a specific context.
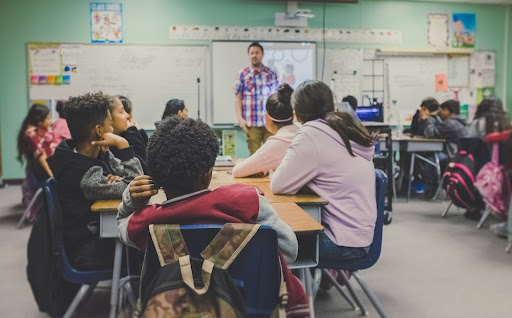
Intrinsic, instrumental, and collective case studies
These three categories of case studies focus on the nature and purpose of the study. An intrinsic case study is conducted when a researcher has an inherent interest in the case itself. Instrumental case studies are employed when the case is used to provide insight into a particular issue or phenomenon. A collective case study, on the other hand, involves studying multiple cases simultaneously to investigate some general phenomena.
Each type of case study serves a different purpose and has its own strengths and challenges. The selection of the type should be guided by the research question and objectives, as well as the context and constraints of the research.
The flexibility, depth, and contextual richness offered by case studies make this approach an excellent research method for various fields of study. They enable researchers to investigate real-world phenomena within their specific contexts, capturing nuances that other research methods might miss. Across numerous fields, case studies provide valuable insights into complex issues.
Critical information systems research
Case studies provide a detailed understanding of the role and impact of information systems in different contexts. They offer a platform to explore how information systems are designed, implemented, and used and how they interact with various social, economic, and political factors. Case studies in this field often focus on examining the intricate relationship between technology, organizational processes, and user behavior, helping to uncover insights that can inform better system design and implementation.
Health research
Health research is another field where case studies are highly valuable. They offer a way to explore patient experiences, healthcare delivery processes, and the impact of various interventions in a real-world context.
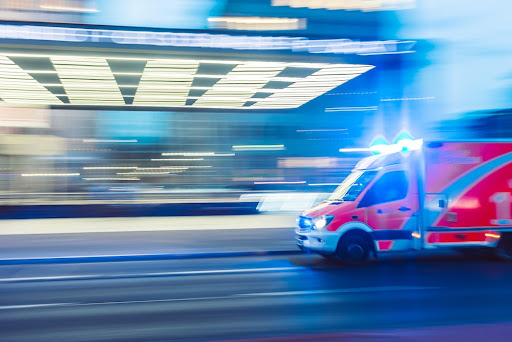
Case studies can provide a deep understanding of a patient's journey, giving insights into the intricacies of disease progression, treatment effects, and the psychosocial aspects of health and illness.
Asthma research studies
Specifically within medical research, studies on asthma often employ case studies to explore the individual and environmental factors that influence asthma development, management, and outcomes. A case study can provide rich, detailed data about individual patients' experiences, from the triggers and symptoms they experience to the effectiveness of various management strategies. This can be crucial for developing patient-centered asthma care approaches.
Other fields
Apart from the fields mentioned, case studies are also extensively used in business and management research, education research, and political sciences, among many others. They provide an opportunity to delve into the intricacies of real-world situations, allowing for a comprehensive understanding of various phenomena.
Case studies, with their depth and contextual focus, offer unique insights across these varied fields. They allow researchers to illuminate the complexities of real-life situations, contributing to both theory and practice.
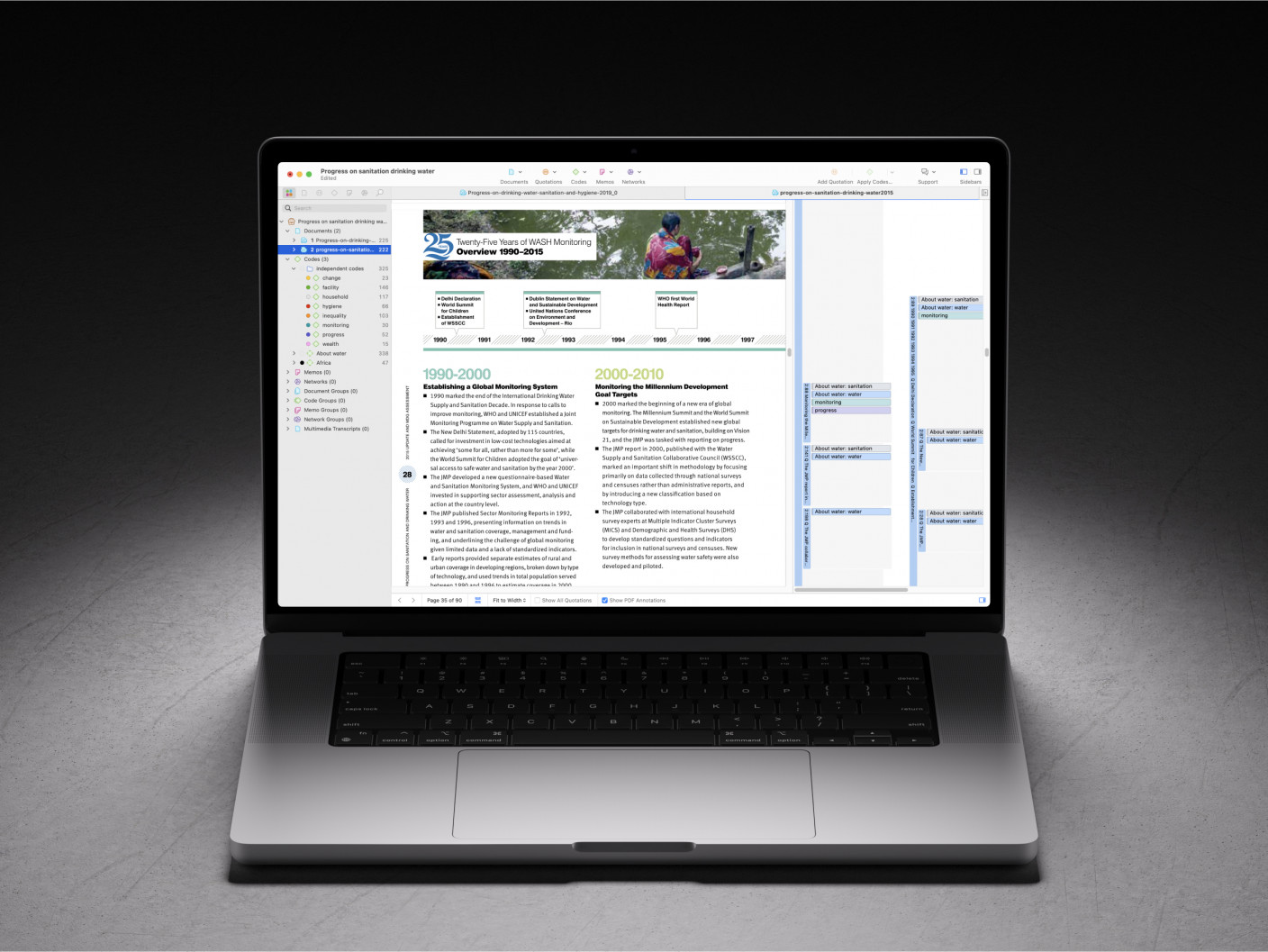
Whatever field you're in, ATLAS.ti puts your data to work for you
Download a free trial of ATLAS.ti to turn your data into insights.
Understanding the key elements of case study design is crucial for conducting rigorous and impactful case study research. A well-structured design guides the researcher through the process, ensuring that the study is methodologically sound and its findings are reliable and valid. The main elements of case study design include the research question , propositions, units of analysis, and the logic linking the data to the propositions.
The research question is the foundation of any research study. A good research question guides the direction of the study and informs the selection of the case, the methods of collecting data, and the analysis techniques. A well-formulated research question in case study research is typically clear, focused, and complex enough to merit further detailed examination of the relevant case(s).
Propositions
Propositions, though not necessary in every case study, provide a direction by stating what we might expect to find in the data collected. They guide how data is collected and analyzed by helping researchers focus on specific aspects of the case. They are particularly important in explanatory case studies, which seek to understand the relationships among concepts within the studied phenomenon.
Units of analysis
The unit of analysis refers to the case, or the main entity or entities that are being analyzed in the study. In case study research, the unit of analysis can be an individual, a group, an organization, a decision, an event, or even a time period. It's crucial to clearly define the unit of analysis, as it shapes the qualitative data analysis process by allowing the researcher to analyze a particular case and synthesize analysis across multiple case studies to draw conclusions.
Argumentation
This refers to the inferential model that allows researchers to draw conclusions from the data. The researcher needs to ensure that there is a clear link between the data, the propositions (if any), and the conclusions drawn. This argumentation is what enables the researcher to make valid and credible inferences about the phenomenon under study.
Understanding and carefully considering these elements in the design phase of a case study can significantly enhance the quality of the research. It can help ensure that the study is methodologically sound and its findings contribute meaningful insights about the case.
Ready to jumpstart your research with ATLAS.ti?
Conceptualize your research project with our intuitive data analysis interface. Download a free trial today.
Conducting a case study involves several steps, from defining the research question and selecting the case to collecting and analyzing data . This section outlines these key stages, providing a practical guide on how to conduct case study research.
Defining the research question
The first step in case study research is defining a clear, focused research question. This question should guide the entire research process, from case selection to analysis. It's crucial to ensure that the research question is suitable for a case study approach. Typically, such questions are exploratory or descriptive in nature and focus on understanding a phenomenon within its real-life context.
Selecting and defining the case
The selection of the case should be based on the research question and the objectives of the study. It involves choosing a unique example or a set of examples that provide rich, in-depth data about the phenomenon under investigation. After selecting the case, it's crucial to define it clearly, setting the boundaries of the case, including the time period and the specific context.
Previous research can help guide the case study design. When considering a case study, an example of a case could be taken from previous case study research and used to define cases in a new research inquiry. Considering recently published examples can help understand how to select and define cases effectively.
Developing a detailed case study protocol
A case study protocol outlines the procedures and general rules to be followed during the case study. This includes the data collection methods to be used, the sources of data, and the procedures for analysis. Having a detailed case study protocol ensures consistency and reliability in the study.
The protocol should also consider how to work with the people involved in the research context to grant the research team access to collecting data. As mentioned in previous sections of this guide, establishing rapport is an essential component of qualitative research as it shapes the overall potential for collecting and analyzing data.
Collecting data
Gathering data in case study research often involves multiple sources of evidence, including documents, archival records, interviews, observations, and physical artifacts. This allows for a comprehensive understanding of the case. The process for gathering data should be systematic and carefully documented to ensure the reliability and validity of the study.
Analyzing and interpreting data
The next step is analyzing the data. This involves organizing the data , categorizing it into themes or patterns , and interpreting these patterns to answer the research question. The analysis might also involve comparing the findings with prior research or theoretical propositions.
Writing the case study report
The final step is writing the case study report . This should provide a detailed description of the case, the data, the analysis process, and the findings. The report should be clear, organized, and carefully written to ensure that the reader can understand the case and the conclusions drawn from it.
Each of these steps is crucial in ensuring that the case study research is rigorous, reliable, and provides valuable insights about the case.
The type, depth, and quality of data in your study can significantly influence the validity and utility of the study. In case study research, data is usually collected from multiple sources to provide a comprehensive and nuanced understanding of the case. This section will outline the various methods of collecting data used in case study research and discuss considerations for ensuring the quality of the data.
Interviews are a common method of gathering data in case study research. They can provide rich, in-depth data about the perspectives, experiences, and interpretations of the individuals involved in the case. Interviews can be structured , semi-structured , or unstructured , depending on the research question and the degree of flexibility needed.
Observations
Observations involve the researcher observing the case in its natural setting, providing first-hand information about the case and its context. Observations can provide data that might not be revealed in interviews or documents, such as non-verbal cues or contextual information.
Documents and artifacts
Documents and archival records provide a valuable source of data in case study research. They can include reports, letters, memos, meeting minutes, email correspondence, and various public and private documents related to the case.
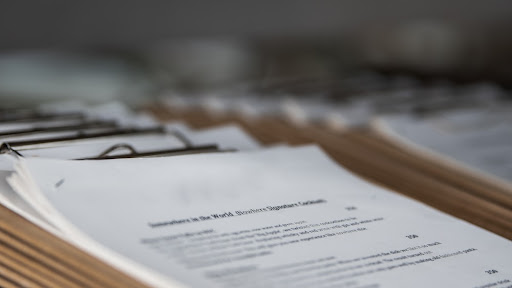
These records can provide historical context, corroborate evidence from other sources, and offer insights into the case that might not be apparent from interviews or observations.
Physical artifacts refer to any physical evidence related to the case, such as tools, products, or physical environments. These artifacts can provide tangible insights into the case, complementing the data gathered from other sources.
Ensuring the quality of data collection
Determining the quality of data in case study research requires careful planning and execution. It's crucial to ensure that the data is reliable, accurate, and relevant to the research question. This involves selecting appropriate methods of collecting data, properly training interviewers or observers, and systematically recording and storing the data. It also includes considering ethical issues related to collecting and handling data, such as obtaining informed consent and ensuring the privacy and confidentiality of the participants.
Data analysis
Analyzing case study research involves making sense of the rich, detailed data to answer the research question. This process can be challenging due to the volume and complexity of case study data. However, a systematic and rigorous approach to analysis can ensure that the findings are credible and meaningful. This section outlines the main steps and considerations in analyzing data in case study research.
Organizing the data
The first step in the analysis is organizing the data. This involves sorting the data into manageable sections, often according to the data source or the theme. This step can also involve transcribing interviews, digitizing physical artifacts, or organizing observational data.
Categorizing and coding the data
Once the data is organized, the next step is to categorize or code the data. This involves identifying common themes, patterns, or concepts in the data and assigning codes to relevant data segments. Coding can be done manually or with the help of software tools, and in either case, qualitative analysis software can greatly facilitate the entire coding process. Coding helps to reduce the data to a set of themes or categories that can be more easily analyzed.
Identifying patterns and themes
After coding the data, the researcher looks for patterns or themes in the coded data. This involves comparing and contrasting the codes and looking for relationships or patterns among them. The identified patterns and themes should help answer the research question.
Interpreting the data
Once patterns and themes have been identified, the next step is to interpret these findings. This involves explaining what the patterns or themes mean in the context of the research question and the case. This interpretation should be grounded in the data, but it can also involve drawing on theoretical concepts or prior research.
Verification of the data
The last step in the analysis is verification. This involves checking the accuracy and consistency of the analysis process and confirming that the findings are supported by the data. This can involve re-checking the original data, checking the consistency of codes, or seeking feedback from research participants or peers.
Like any research method , case study research has its strengths and limitations. Researchers must be aware of these, as they can influence the design, conduct, and interpretation of the study.
Understanding the strengths and limitations of case study research can also guide researchers in deciding whether this approach is suitable for their research question . This section outlines some of the key strengths and limitations of case study research.
Benefits include the following:
- Rich, detailed data: One of the main strengths of case study research is that it can generate rich, detailed data about the case. This can provide a deep understanding of the case and its context, which can be valuable in exploring complex phenomena.
- Flexibility: Case study research is flexible in terms of design , data collection , and analysis . A sufficient degree of flexibility allows the researcher to adapt the study according to the case and the emerging findings.
- Real-world context: Case study research involves studying the case in its real-world context, which can provide valuable insights into the interplay between the case and its context.
- Multiple sources of evidence: Case study research often involves collecting data from multiple sources , which can enhance the robustness and validity of the findings.
On the other hand, researchers should consider the following limitations:
- Generalizability: A common criticism of case study research is that its findings might not be generalizable to other cases due to the specificity and uniqueness of each case.
- Time and resource intensive: Case study research can be time and resource intensive due to the depth of the investigation and the amount of collected data.
- Complexity of analysis: The rich, detailed data generated in case study research can make analyzing the data challenging.
- Subjectivity: Given the nature of case study research, there may be a higher degree of subjectivity in interpreting the data , so researchers need to reflect on this and transparently convey to audiences how the research was conducted.
Being aware of these strengths and limitations can help researchers design and conduct case study research effectively and interpret and report the findings appropriately.
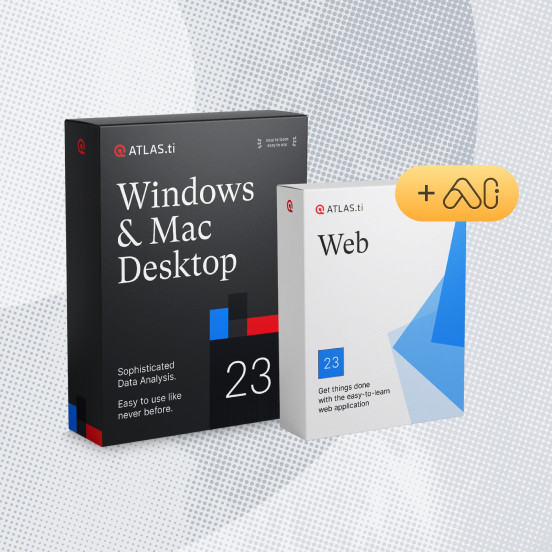
Ready to analyze your data with ATLAS.ti?
See how our intuitive software can draw key insights from your data with a free trial today.
Have a language expert improve your writing
Run a free plagiarism check in 10 minutes, automatically generate references for free.
- Knowledge Base
- Methodology
- Case Study | Definition, Examples & Methods
Case Study | Definition, Examples & Methods
Published on 5 May 2022 by Shona McCombes . Revised on 30 January 2023.
A case study is a detailed study of a specific subject, such as a person, group, place, event, organisation, or phenomenon. Case studies are commonly used in social, educational, clinical, and business research.
A case study research design usually involves qualitative methods , but quantitative methods are sometimes also used. Case studies are good for describing , comparing, evaluating, and understanding different aspects of a research problem .
Table of contents
When to do a case study, step 1: select a case, step 2: build a theoretical framework, step 3: collect your data, step 4: describe and analyse the case.
A case study is an appropriate research design when you want to gain concrete, contextual, in-depth knowledge about a specific real-world subject. It allows you to explore the key characteristics, meanings, and implications of the case.
Case studies are often a good choice in a thesis or dissertation . They keep your project focused and manageable when you don’t have the time or resources to do large-scale research.
You might use just one complex case study where you explore a single subject in depth, or conduct multiple case studies to compare and illuminate different aspects of your research problem.
Prevent plagiarism, run a free check.
Once you have developed your problem statement and research questions , you should be ready to choose the specific case that you want to focus on. A good case study should have the potential to:
- Provide new or unexpected insights into the subject
- Challenge or complicate existing assumptions and theories
- Propose practical courses of action to resolve a problem
- Open up new directions for future research
Unlike quantitative or experimental research, a strong case study does not require a random or representative sample. In fact, case studies often deliberately focus on unusual, neglected, or outlying cases which may shed new light on the research problem.
If you find yourself aiming to simultaneously investigate and solve an issue, consider conducting action research . As its name suggests, action research conducts research and takes action at the same time, and is highly iterative and flexible.
However, you can also choose a more common or representative case to exemplify a particular category, experience, or phenomenon.
While case studies focus more on concrete details than general theories, they should usually have some connection with theory in the field. This way the case study is not just an isolated description, but is integrated into existing knowledge about the topic. It might aim to:
- Exemplify a theory by showing how it explains the case under investigation
- Expand on a theory by uncovering new concepts and ideas that need to be incorporated
- Challenge a theory by exploring an outlier case that doesn’t fit with established assumptions
To ensure that your analysis of the case has a solid academic grounding, you should conduct a literature review of sources related to the topic and develop a theoretical framework . This means identifying key concepts and theories to guide your analysis and interpretation.
There are many different research methods you can use to collect data on your subject. Case studies tend to focus on qualitative data using methods such as interviews, observations, and analysis of primary and secondary sources (e.g., newspaper articles, photographs, official records). Sometimes a case study will also collect quantitative data .
The aim is to gain as thorough an understanding as possible of the case and its context.
In writing up the case study, you need to bring together all the relevant aspects to give as complete a picture as possible of the subject.
How you report your findings depends on the type of research you are doing. Some case studies are structured like a standard scientific paper or thesis, with separate sections or chapters for the methods , results , and discussion .
Others are written in a more narrative style, aiming to explore the case from various angles and analyse its meanings and implications (for example, by using textual analysis or discourse analysis ).
In all cases, though, make sure to give contextual details about the case, connect it back to the literature and theory, and discuss how it fits into wider patterns or debates.
Cite this Scribbr article
If you want to cite this source, you can copy and paste the citation or click the ‘Cite this Scribbr article’ button to automatically add the citation to our free Reference Generator.
McCombes, S. (2023, January 30). Case Study | Definition, Examples & Methods. Scribbr. Retrieved 29 April 2024, from https://www.scribbr.co.uk/research-methods/case-studies/
Is this article helpful?
Shona McCombes
Other students also liked, correlational research | guide, design & examples, a quick guide to experimental design | 5 steps & examples, descriptive research design | definition, methods & examples.
- Open access
- Published: 27 June 2011
The case study approach
- Sarah Crowe 1 ,
- Kathrin Cresswell 2 ,
- Ann Robertson 2 ,
- Guro Huby 3 ,
- Anthony Avery 1 &
- Aziz Sheikh 2
BMC Medical Research Methodology volume 11 , Article number: 100 ( 2011 ) Cite this article
774k Accesses
1037 Citations
37 Altmetric
Metrics details
The case study approach allows in-depth, multi-faceted explorations of complex issues in their real-life settings. The value of the case study approach is well recognised in the fields of business, law and policy, but somewhat less so in health services research. Based on our experiences of conducting several health-related case studies, we reflect on the different types of case study design, the specific research questions this approach can help answer, the data sources that tend to be used, and the particular advantages and disadvantages of employing this methodological approach. The paper concludes with key pointers to aid those designing and appraising proposals for conducting case study research, and a checklist to help readers assess the quality of case study reports.
Peer Review reports
Introduction
The case study approach is particularly useful to employ when there is a need to obtain an in-depth appreciation of an issue, event or phenomenon of interest, in its natural real-life context. Our aim in writing this piece is to provide insights into when to consider employing this approach and an overview of key methodological considerations in relation to the design, planning, analysis, interpretation and reporting of case studies.
The illustrative 'grand round', 'case report' and 'case series' have a long tradition in clinical practice and research. Presenting detailed critiques, typically of one or more patients, aims to provide insights into aspects of the clinical case and, in doing so, illustrate broader lessons that may be learnt. In research, the conceptually-related case study approach can be used, for example, to describe in detail a patient's episode of care, explore professional attitudes to and experiences of a new policy initiative or service development or more generally to 'investigate contemporary phenomena within its real-life context' [ 1 ]. Based on our experiences of conducting a range of case studies, we reflect on when to consider using this approach, discuss the key steps involved and illustrate, with examples, some of the practical challenges of attaining an in-depth understanding of a 'case' as an integrated whole. In keeping with previously published work, we acknowledge the importance of theory to underpin the design, selection, conduct and interpretation of case studies[ 2 ]. In so doing, we make passing reference to the different epistemological approaches used in case study research by key theoreticians and methodologists in this field of enquiry.
This paper is structured around the following main questions: What is a case study? What are case studies used for? How are case studies conducted? What are the potential pitfalls and how can these be avoided? We draw in particular on four of our own recently published examples of case studies (see Tables 1 , 2 , 3 and 4 ) and those of others to illustrate our discussion[ 3 – 7 ].
What is a case study?
A case study is a research approach that is used to generate an in-depth, multi-faceted understanding of a complex issue in its real-life context. It is an established research design that is used extensively in a wide variety of disciplines, particularly in the social sciences. A case study can be defined in a variety of ways (Table 5 ), the central tenet being the need to explore an event or phenomenon in depth and in its natural context. It is for this reason sometimes referred to as a "naturalistic" design; this is in contrast to an "experimental" design (such as a randomised controlled trial) in which the investigator seeks to exert control over and manipulate the variable(s) of interest.
Stake's work has been particularly influential in defining the case study approach to scientific enquiry. He has helpfully characterised three main types of case study: intrinsic , instrumental and collective [ 8 ]. An intrinsic case study is typically undertaken to learn about a unique phenomenon. The researcher should define the uniqueness of the phenomenon, which distinguishes it from all others. In contrast, the instrumental case study uses a particular case (some of which may be better than others) to gain a broader appreciation of an issue or phenomenon. The collective case study involves studying multiple cases simultaneously or sequentially in an attempt to generate a still broader appreciation of a particular issue.
These are however not necessarily mutually exclusive categories. In the first of our examples (Table 1 ), we undertook an intrinsic case study to investigate the issue of recruitment of minority ethnic people into the specific context of asthma research studies, but it developed into a instrumental case study through seeking to understand the issue of recruitment of these marginalised populations more generally, generating a number of the findings that are potentially transferable to other disease contexts[ 3 ]. In contrast, the other three examples (see Tables 2 , 3 and 4 ) employed collective case study designs to study the introduction of workforce reconfiguration in primary care, the implementation of electronic health records into hospitals, and to understand the ways in which healthcare students learn about patient safety considerations[ 4 – 6 ]. Although our study focusing on the introduction of General Practitioners with Specialist Interests (Table 2 ) was explicitly collective in design (four contrasting primary care organisations were studied), is was also instrumental in that this particular professional group was studied as an exemplar of the more general phenomenon of workforce redesign[ 4 ].
What are case studies used for?
According to Yin, case studies can be used to explain, describe or explore events or phenomena in the everyday contexts in which they occur[ 1 ]. These can, for example, help to understand and explain causal links and pathways resulting from a new policy initiative or service development (see Tables 2 and 3 , for example)[ 1 ]. In contrast to experimental designs, which seek to test a specific hypothesis through deliberately manipulating the environment (like, for example, in a randomised controlled trial giving a new drug to randomly selected individuals and then comparing outcomes with controls),[ 9 ] the case study approach lends itself well to capturing information on more explanatory ' how ', 'what' and ' why ' questions, such as ' how is the intervention being implemented and received on the ground?'. The case study approach can offer additional insights into what gaps exist in its delivery or why one implementation strategy might be chosen over another. This in turn can help develop or refine theory, as shown in our study of the teaching of patient safety in undergraduate curricula (Table 4 )[ 6 , 10 ]. Key questions to consider when selecting the most appropriate study design are whether it is desirable or indeed possible to undertake a formal experimental investigation in which individuals and/or organisations are allocated to an intervention or control arm? Or whether the wish is to obtain a more naturalistic understanding of an issue? The former is ideally studied using a controlled experimental design, whereas the latter is more appropriately studied using a case study design.
Case studies may be approached in different ways depending on the epistemological standpoint of the researcher, that is, whether they take a critical (questioning one's own and others' assumptions), interpretivist (trying to understand individual and shared social meanings) or positivist approach (orientating towards the criteria of natural sciences, such as focusing on generalisability considerations) (Table 6 ). Whilst such a schema can be conceptually helpful, it may be appropriate to draw on more than one approach in any case study, particularly in the context of conducting health services research. Doolin has, for example, noted that in the context of undertaking interpretative case studies, researchers can usefully draw on a critical, reflective perspective which seeks to take into account the wider social and political environment that has shaped the case[ 11 ].
How are case studies conducted?
Here, we focus on the main stages of research activity when planning and undertaking a case study; the crucial stages are: defining the case; selecting the case(s); collecting and analysing the data; interpreting data; and reporting the findings.
Defining the case
Carefully formulated research question(s), informed by the existing literature and a prior appreciation of the theoretical issues and setting(s), are all important in appropriately and succinctly defining the case[ 8 , 12 ]. Crucially, each case should have a pre-defined boundary which clarifies the nature and time period covered by the case study (i.e. its scope, beginning and end), the relevant social group, organisation or geographical area of interest to the investigator, the types of evidence to be collected, and the priorities for data collection and analysis (see Table 7 )[ 1 ]. A theory driven approach to defining the case may help generate knowledge that is potentially transferable to a range of clinical contexts and behaviours; using theory is also likely to result in a more informed appreciation of, for example, how and why interventions have succeeded or failed[ 13 ].
For example, in our evaluation of the introduction of electronic health records in English hospitals (Table 3 ), we defined our cases as the NHS Trusts that were receiving the new technology[ 5 ]. Our focus was on how the technology was being implemented. However, if the primary research interest had been on the social and organisational dimensions of implementation, we might have defined our case differently as a grouping of healthcare professionals (e.g. doctors and/or nurses). The precise beginning and end of the case may however prove difficult to define. Pursuing this same example, when does the process of implementation and adoption of an electronic health record system really begin or end? Such judgements will inevitably be influenced by a range of factors, including the research question, theory of interest, the scope and richness of the gathered data and the resources available to the research team.
Selecting the case(s)
The decision on how to select the case(s) to study is a very important one that merits some reflection. In an intrinsic case study, the case is selected on its own merits[ 8 ]. The case is selected not because it is representative of other cases, but because of its uniqueness, which is of genuine interest to the researchers. This was, for example, the case in our study of the recruitment of minority ethnic participants into asthma research (Table 1 ) as our earlier work had demonstrated the marginalisation of minority ethnic people with asthma, despite evidence of disproportionate asthma morbidity[ 14 , 15 ]. In another example of an intrinsic case study, Hellstrom et al.[ 16 ] studied an elderly married couple living with dementia to explore how dementia had impacted on their understanding of home, their everyday life and their relationships.
For an instrumental case study, selecting a "typical" case can work well[ 8 ]. In contrast to the intrinsic case study, the particular case which is chosen is of less importance than selecting a case that allows the researcher to investigate an issue or phenomenon. For example, in order to gain an understanding of doctors' responses to health policy initiatives, Som undertook an instrumental case study interviewing clinicians who had a range of responsibilities for clinical governance in one NHS acute hospital trust[ 17 ]. Sampling a "deviant" or "atypical" case may however prove even more informative, potentially enabling the researcher to identify causal processes, generate hypotheses and develop theory.
In collective or multiple case studies, a number of cases are carefully selected. This offers the advantage of allowing comparisons to be made across several cases and/or replication. Choosing a "typical" case may enable the findings to be generalised to theory (i.e. analytical generalisation) or to test theory by replicating the findings in a second or even a third case (i.e. replication logic)[ 1 ]. Yin suggests two or three literal replications (i.e. predicting similar results) if the theory is straightforward and five or more if the theory is more subtle. However, critics might argue that selecting 'cases' in this way is insufficiently reflexive and ill-suited to the complexities of contemporary healthcare organisations.
The selected case study site(s) should allow the research team access to the group of individuals, the organisation, the processes or whatever else constitutes the chosen unit of analysis for the study. Access is therefore a central consideration; the researcher needs to come to know the case study site(s) well and to work cooperatively with them. Selected cases need to be not only interesting but also hospitable to the inquiry [ 8 ] if they are to be informative and answer the research question(s). Case study sites may also be pre-selected for the researcher, with decisions being influenced by key stakeholders. For example, our selection of case study sites in the evaluation of the implementation and adoption of electronic health record systems (see Table 3 ) was heavily influenced by NHS Connecting for Health, the government agency that was responsible for overseeing the National Programme for Information Technology (NPfIT)[ 5 ]. This prominent stakeholder had already selected the NHS sites (through a competitive bidding process) to be early adopters of the electronic health record systems and had negotiated contracts that detailed the deployment timelines.
It is also important to consider in advance the likely burden and risks associated with participation for those who (or the site(s) which) comprise the case study. Of particular importance is the obligation for the researcher to think through the ethical implications of the study (e.g. the risk of inadvertently breaching anonymity or confidentiality) and to ensure that potential participants/participating sites are provided with sufficient information to make an informed choice about joining the study. The outcome of providing this information might be that the emotive burden associated with participation, or the organisational disruption associated with supporting the fieldwork, is considered so high that the individuals or sites decide against participation.
In our example of evaluating implementations of electronic health record systems, given the restricted number of early adopter sites available to us, we sought purposively to select a diverse range of implementation cases among those that were available[ 5 ]. We chose a mixture of teaching, non-teaching and Foundation Trust hospitals, and examples of each of the three electronic health record systems procured centrally by the NPfIT. At one recruited site, it quickly became apparent that access was problematic because of competing demands on that organisation. Recognising the importance of full access and co-operative working for generating rich data, the research team decided not to pursue work at that site and instead to focus on other recruited sites.
Collecting the data
In order to develop a thorough understanding of the case, the case study approach usually involves the collection of multiple sources of evidence, using a range of quantitative (e.g. questionnaires, audits and analysis of routinely collected healthcare data) and more commonly qualitative techniques (e.g. interviews, focus groups and observations). The use of multiple sources of data (data triangulation) has been advocated as a way of increasing the internal validity of a study (i.e. the extent to which the method is appropriate to answer the research question)[ 8 , 18 – 21 ]. An underlying assumption is that data collected in different ways should lead to similar conclusions, and approaching the same issue from different angles can help develop a holistic picture of the phenomenon (Table 2 )[ 4 ].
Brazier and colleagues used a mixed-methods case study approach to investigate the impact of a cancer care programme[ 22 ]. Here, quantitative measures were collected with questionnaires before, and five months after, the start of the intervention which did not yield any statistically significant results. Qualitative interviews with patients however helped provide an insight into potentially beneficial process-related aspects of the programme, such as greater, perceived patient involvement in care. The authors reported how this case study approach provided a number of contextual factors likely to influence the effectiveness of the intervention and which were not likely to have been obtained from quantitative methods alone.
In collective or multiple case studies, data collection needs to be flexible enough to allow a detailed description of each individual case to be developed (e.g. the nature of different cancer care programmes), before considering the emerging similarities and differences in cross-case comparisons (e.g. to explore why one programme is more effective than another). It is important that data sources from different cases are, where possible, broadly comparable for this purpose even though they may vary in nature and depth.
Analysing, interpreting and reporting case studies
Making sense and offering a coherent interpretation of the typically disparate sources of data (whether qualitative alone or together with quantitative) is far from straightforward. Repeated reviewing and sorting of the voluminous and detail-rich data are integral to the process of analysis. In collective case studies, it is helpful to analyse data relating to the individual component cases first, before making comparisons across cases. Attention needs to be paid to variations within each case and, where relevant, the relationship between different causes, effects and outcomes[ 23 ]. Data will need to be organised and coded to allow the key issues, both derived from the literature and emerging from the dataset, to be easily retrieved at a later stage. An initial coding frame can help capture these issues and can be applied systematically to the whole dataset with the aid of a qualitative data analysis software package.
The Framework approach is a practical approach, comprising of five stages (familiarisation; identifying a thematic framework; indexing; charting; mapping and interpretation) , to managing and analysing large datasets particularly if time is limited, as was the case in our study of recruitment of South Asians into asthma research (Table 1 )[ 3 , 24 ]. Theoretical frameworks may also play an important role in integrating different sources of data and examining emerging themes. For example, we drew on a socio-technical framework to help explain the connections between different elements - technology; people; and the organisational settings within which they worked - in our study of the introduction of electronic health record systems (Table 3 )[ 5 ]. Our study of patient safety in undergraduate curricula drew on an evaluation-based approach to design and analysis, which emphasised the importance of the academic, organisational and practice contexts through which students learn (Table 4 )[ 6 ].
Case study findings can have implications both for theory development and theory testing. They may establish, strengthen or weaken historical explanations of a case and, in certain circumstances, allow theoretical (as opposed to statistical) generalisation beyond the particular cases studied[ 12 ]. These theoretical lenses should not, however, constitute a strait-jacket and the cases should not be "forced to fit" the particular theoretical framework that is being employed.
When reporting findings, it is important to provide the reader with enough contextual information to understand the processes that were followed and how the conclusions were reached. In a collective case study, researchers may choose to present the findings from individual cases separately before amalgamating across cases. Care must be taken to ensure the anonymity of both case sites and individual participants (if agreed in advance) by allocating appropriate codes or withholding descriptors. In the example given in Table 3 , we decided against providing detailed information on the NHS sites and individual participants in order to avoid the risk of inadvertent disclosure of identities[ 5 , 25 ].
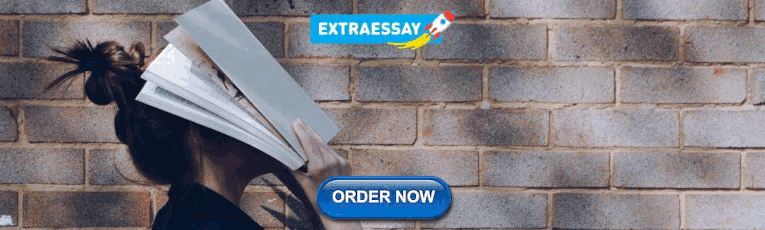
What are the potential pitfalls and how can these be avoided?
The case study approach is, as with all research, not without its limitations. When investigating the formal and informal ways undergraduate students learn about patient safety (Table 4 ), for example, we rapidly accumulated a large quantity of data. The volume of data, together with the time restrictions in place, impacted on the depth of analysis that was possible within the available resources. This highlights a more general point of the importance of avoiding the temptation to collect as much data as possible; adequate time also needs to be set aside for data analysis and interpretation of what are often highly complex datasets.
Case study research has sometimes been criticised for lacking scientific rigour and providing little basis for generalisation (i.e. producing findings that may be transferable to other settings)[ 1 ]. There are several ways to address these concerns, including: the use of theoretical sampling (i.e. drawing on a particular conceptual framework); respondent validation (i.e. participants checking emerging findings and the researcher's interpretation, and providing an opinion as to whether they feel these are accurate); and transparency throughout the research process (see Table 8 )[ 8 , 18 – 21 , 23 , 26 ]. Transparency can be achieved by describing in detail the steps involved in case selection, data collection, the reasons for the particular methods chosen, and the researcher's background and level of involvement (i.e. being explicit about how the researcher has influenced data collection and interpretation). Seeking potential, alternative explanations, and being explicit about how interpretations and conclusions were reached, help readers to judge the trustworthiness of the case study report. Stake provides a critique checklist for a case study report (Table 9 )[ 8 ].
Conclusions
The case study approach allows, amongst other things, critical events, interventions, policy developments and programme-based service reforms to be studied in detail in a real-life context. It should therefore be considered when an experimental design is either inappropriate to answer the research questions posed or impossible to undertake. Considering the frequency with which implementations of innovations are now taking place in healthcare settings and how well the case study approach lends itself to in-depth, complex health service research, we believe this approach should be more widely considered by researchers. Though inherently challenging, the research case study can, if carefully conceptualised and thoughtfully undertaken and reported, yield powerful insights into many important aspects of health and healthcare delivery.
Yin RK: Case study research, design and method. 2009, London: Sage Publications Ltd., 4
Google Scholar
Keen J, Packwood T: Qualitative research; case study evaluation. BMJ. 1995, 311: 444-446.
Article CAS PubMed PubMed Central Google Scholar
Sheikh A, Halani L, Bhopal R, Netuveli G, Partridge M, Car J, et al: Facilitating the Recruitment of Minority Ethnic People into Research: Qualitative Case Study of South Asians and Asthma. PLoS Med. 2009, 6 (10): 1-11.
Article Google Scholar
Pinnock H, Huby G, Powell A, Kielmann T, Price D, Williams S, et al: The process of planning, development and implementation of a General Practitioner with a Special Interest service in Primary Care Organisations in England and Wales: a comparative prospective case study. Report for the National Co-ordinating Centre for NHS Service Delivery and Organisation R&D (NCCSDO). 2008, [ http://www.sdo.nihr.ac.uk/files/project/99-final-report.pdf ]
Robertson A, Cresswell K, Takian A, Petrakaki D, Crowe S, Cornford T, et al: Prospective evaluation of the implementation and adoption of NHS Connecting for Health's national electronic health record in secondary care in England: interim findings. BMJ. 2010, 41: c4564-
Pearson P, Steven A, Howe A, Sheikh A, Ashcroft D, Smith P, the Patient Safety Education Study Group: Learning about patient safety: organisational context and culture in the education of healthcare professionals. J Health Serv Res Policy. 2010, 15: 4-10. 10.1258/jhsrp.2009.009052.
Article PubMed Google Scholar
van Harten WH, Casparie TF, Fisscher OA: The evaluation of the introduction of a quality management system: a process-oriented case study in a large rehabilitation hospital. Health Policy. 2002, 60 (1): 17-37. 10.1016/S0168-8510(01)00187-7.
Stake RE: The art of case study research. 1995, London: Sage Publications Ltd.
Sheikh A, Smeeth L, Ashcroft R: Randomised controlled trials in primary care: scope and application. Br J Gen Pract. 2002, 52 (482): 746-51.
PubMed PubMed Central Google Scholar
King G, Keohane R, Verba S: Designing Social Inquiry. 1996, Princeton: Princeton University Press
Doolin B: Information technology as disciplinary technology: being critical in interpretative research on information systems. Journal of Information Technology. 1998, 13: 301-311. 10.1057/jit.1998.8.
George AL, Bennett A: Case studies and theory development in the social sciences. 2005, Cambridge, MA: MIT Press
Eccles M, the Improved Clinical Effectiveness through Behavioural Research Group (ICEBeRG): Designing theoretically-informed implementation interventions. Implementation Science. 2006, 1: 1-8. 10.1186/1748-5908-1-1.
Article PubMed Central Google Scholar
Netuveli G, Hurwitz B, Levy M, Fletcher M, Barnes G, Durham SR, Sheikh A: Ethnic variations in UK asthma frequency, morbidity, and health-service use: a systematic review and meta-analysis. Lancet. 2005, 365 (9456): 312-7.
Sheikh A, Panesar SS, Lasserson T, Netuveli G: Recruitment of ethnic minorities to asthma studies. Thorax. 2004, 59 (7): 634-
CAS PubMed PubMed Central Google Scholar
Hellström I, Nolan M, Lundh U: 'We do things together': A case study of 'couplehood' in dementia. Dementia. 2005, 4: 7-22. 10.1177/1471301205049188.
Som CV: Nothing seems to have changed, nothing seems to be changing and perhaps nothing will change in the NHS: doctors' response to clinical governance. International Journal of Public Sector Management. 2005, 18: 463-477. 10.1108/09513550510608903.
Lincoln Y, Guba E: Naturalistic inquiry. 1985, Newbury Park: Sage Publications
Barbour RS: Checklists for improving rigour in qualitative research: a case of the tail wagging the dog?. BMJ. 2001, 322: 1115-1117. 10.1136/bmj.322.7294.1115.
Mays N, Pope C: Qualitative research in health care: Assessing quality in qualitative research. BMJ. 2000, 320: 50-52. 10.1136/bmj.320.7226.50.
Mason J: Qualitative researching. 2002, London: Sage
Brazier A, Cooke K, Moravan V: Using Mixed Methods for Evaluating an Integrative Approach to Cancer Care: A Case Study. Integr Cancer Ther. 2008, 7: 5-17. 10.1177/1534735407313395.
Miles MB, Huberman M: Qualitative data analysis: an expanded sourcebook. 1994, CA: Sage Publications Inc., 2
Pope C, Ziebland S, Mays N: Analysing qualitative data. Qualitative research in health care. BMJ. 2000, 320: 114-116. 10.1136/bmj.320.7227.114.
Cresswell KM, Worth A, Sheikh A: Actor-Network Theory and its role in understanding the implementation of information technology developments in healthcare. BMC Med Inform Decis Mak. 2010, 10 (1): 67-10.1186/1472-6947-10-67.
Article PubMed PubMed Central Google Scholar
Malterud K: Qualitative research: standards, challenges, and guidelines. Lancet. 2001, 358: 483-488. 10.1016/S0140-6736(01)05627-6.
Article CAS PubMed Google Scholar
Yin R: Case study research: design and methods. 1994, Thousand Oaks, CA: Sage Publishing, 2
Yin R: Enhancing the quality of case studies in health services research. Health Serv Res. 1999, 34: 1209-1224.
Green J, Thorogood N: Qualitative methods for health research. 2009, Los Angeles: Sage, 2
Howcroft D, Trauth E: Handbook of Critical Information Systems Research, Theory and Application. 2005, Cheltenham, UK: Northampton, MA, USA: Edward Elgar
Book Google Scholar
Blakie N: Approaches to Social Enquiry. 1993, Cambridge: Polity Press
Doolin B: Power and resistance in the implementation of a medical management information system. Info Systems J. 2004, 14: 343-362. 10.1111/j.1365-2575.2004.00176.x.
Bloomfield BP, Best A: Management consultants: systems development, power and the translation of problems. Sociological Review. 1992, 40: 533-560.
Shanks G, Parr A: Positivist, single case study research in information systems: A critical analysis. Proceedings of the European Conference on Information Systems. 2003, Naples
Pre-publication history
The pre-publication history for this paper can be accessed here: http://www.biomedcentral.com/1471-2288/11/100/prepub
Download references
Acknowledgements
We are grateful to the participants and colleagues who contributed to the individual case studies that we have drawn on. This work received no direct funding, but it has been informed by projects funded by Asthma UK, the NHS Service Delivery Organisation, NHS Connecting for Health Evaluation Programme, and Patient Safety Research Portfolio. We would also like to thank the expert reviewers for their insightful and constructive feedback. Our thanks are also due to Dr. Allison Worth who commented on an earlier draft of this manuscript.
Author information
Authors and affiliations.
Division of Primary Care, The University of Nottingham, Nottingham, UK
Sarah Crowe & Anthony Avery
Centre for Population Health Sciences, The University of Edinburgh, Edinburgh, UK
Kathrin Cresswell, Ann Robertson & Aziz Sheikh
School of Health in Social Science, The University of Edinburgh, Edinburgh, UK
You can also search for this author in PubMed Google Scholar
Corresponding author
Correspondence to Sarah Crowe .
Additional information
Competing interests.
The authors declare that they have no competing interests.
Authors' contributions
AS conceived this article. SC, KC and AR wrote this paper with GH, AA and AS all commenting on various drafts. SC and AS are guarantors.
Rights and permissions
This article is published under license to BioMed Central Ltd. This is an Open Access article distributed under the terms of the Creative Commons Attribution License ( http://creativecommons.org/licenses/by/2.0 ), which permits unrestricted use, distribution, and reproduction in any medium, provided the original work is properly cited.
Reprints and permissions
About this article
Cite this article.
Crowe, S., Cresswell, K., Robertson, A. et al. The case study approach. BMC Med Res Methodol 11 , 100 (2011). https://doi.org/10.1186/1471-2288-11-100
Download citation
Received : 29 November 2010
Accepted : 27 June 2011
Published : 27 June 2011
DOI : https://doi.org/10.1186/1471-2288-11-100
Share this article
Anyone you share the following link with will be able to read this content:
Sorry, a shareable link is not currently available for this article.
Provided by the Springer Nature SharedIt content-sharing initiative
- Case Study Approach
- Electronic Health Record System
- Case Study Design
- Case Study Site
- Case Study Report
BMC Medical Research Methodology
ISSN: 1471-2288
- General enquiries: [email protected]

- SUGGESTED TOPICS
- The Magazine
- Newsletters
- Managing Yourself
- Managing Teams
- Work-life Balance
- The Big Idea
- Data & Visuals
- Reading Lists
- Case Selections
- HBR Learning
- Topic Feeds
- Account Settings
- Email Preferences
What the Case Study Method Really Teaches
- Nitin Nohria

Seven meta-skills that stick even if the cases fade from memory.
It’s been 100 years since Harvard Business School began using the case study method. Beyond teaching specific subject matter, the case study method excels in instilling meta-skills in students. This article explains the importance of seven such skills: preparation, discernment, bias recognition, judgement, collaboration, curiosity, and self-confidence.
During my decade as dean of Harvard Business School, I spent hundreds of hours talking with our alumni. To enliven these conversations, I relied on a favorite question: “What was the most important thing you learned from your time in our MBA program?”
- Nitin Nohria is the George F. Baker Professor of Business Administration, Distinguished University Service Professor, and former dean of Harvard Business School.
Partner Center
Case Study Research Method in Psychology
Saul Mcleod, PhD
Editor-in-Chief for Simply Psychology
BSc (Hons) Psychology, MRes, PhD, University of Manchester
Saul Mcleod, PhD., is a qualified psychology teacher with over 18 years of experience in further and higher education. He has been published in peer-reviewed journals, including the Journal of Clinical Psychology.
Learn about our Editorial Process
Olivia Guy-Evans, MSc
Associate Editor for Simply Psychology
BSc (Hons) Psychology, MSc Psychology of Education
Olivia Guy-Evans is a writer and associate editor for Simply Psychology. She has previously worked in healthcare and educational sectors.
On This Page:
Case studies are in-depth investigations of a person, group, event, or community. Typically, data is gathered from various sources using several methods (e.g., observations & interviews).
The case study research method originated in clinical medicine (the case history, i.e., the patient’s personal history). In psychology, case studies are often confined to the study of a particular individual.
The information is mainly biographical and relates to events in the individual’s past (i.e., retrospective), as well as to significant events that are currently occurring in his or her everyday life.
The case study is not a research method, but researchers select methods of data collection and analysis that will generate material suitable for case studies.
Freud (1909a, 1909b) conducted very detailed investigations into the private lives of his patients in an attempt to both understand and help them overcome their illnesses.
This makes it clear that the case study is a method that should only be used by a psychologist, therapist, or psychiatrist, i.e., someone with a professional qualification.
There is an ethical issue of competence. Only someone qualified to diagnose and treat a person can conduct a formal case study relating to atypical (i.e., abnormal) behavior or atypical development.
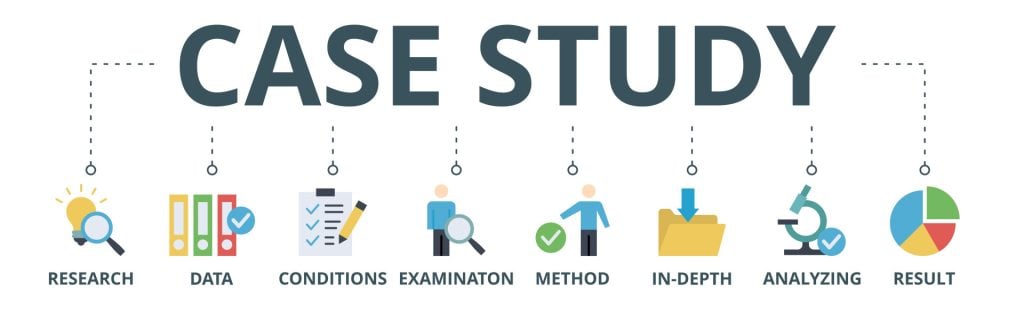
Famous Case Studies
- Anna O – One of the most famous case studies, documenting psychoanalyst Josef Breuer’s treatment of “Anna O” (real name Bertha Pappenheim) for hysteria in the late 1800s using early psychoanalytic theory.
- Little Hans – A child psychoanalysis case study published by Sigmund Freud in 1909 analyzing his five-year-old patient Herbert Graf’s house phobia as related to the Oedipus complex.
- Bruce/Brenda – Gender identity case of the boy (Bruce) whose botched circumcision led psychologist John Money to advise gender reassignment and raise him as a girl (Brenda) in the 1960s.
- Genie Wiley – Linguistics/psychological development case of the victim of extreme isolation abuse who was studied in 1970s California for effects of early language deprivation on acquiring speech later in life.
- Phineas Gage – One of the most famous neuropsychology case studies analyzes personality changes in railroad worker Phineas Gage after an 1848 brain injury involving a tamping iron piercing his skull.
Clinical Case Studies
- Studying the effectiveness of psychotherapy approaches with an individual patient
- Assessing and treating mental illnesses like depression, anxiety disorders, PTSD
- Neuropsychological cases investigating brain injuries or disorders
Child Psychology Case Studies
- Studying psychological development from birth through adolescence
- Cases of learning disabilities, autism spectrum disorders, ADHD
- Effects of trauma, abuse, deprivation on development
Types of Case Studies
- Explanatory case studies : Used to explore causation in order to find underlying principles. Helpful for doing qualitative analysis to explain presumed causal links.
- Exploratory case studies : Used to explore situations where an intervention being evaluated has no clear set of outcomes. It helps define questions and hypotheses for future research.
- Descriptive case studies : Describe an intervention or phenomenon and the real-life context in which it occurred. It is helpful for illustrating certain topics within an evaluation.
- Multiple-case studies : Used to explore differences between cases and replicate findings across cases. Helpful for comparing and contrasting specific cases.
- Intrinsic : Used to gain a better understanding of a particular case. Helpful for capturing the complexity of a single case.
- Collective : Used to explore a general phenomenon using multiple case studies. Helpful for jointly studying a group of cases in order to inquire into the phenomenon.
Where Do You Find Data for a Case Study?
There are several places to find data for a case study. The key is to gather data from multiple sources to get a complete picture of the case and corroborate facts or findings through triangulation of evidence. Most of this information is likely qualitative (i.e., verbal description rather than measurement), but the psychologist might also collect numerical data.
1. Primary sources
- Interviews – Interviewing key people related to the case to get their perspectives and insights. The interview is an extremely effective procedure for obtaining information about an individual, and it may be used to collect comments from the person’s friends, parents, employer, workmates, and others who have a good knowledge of the person, as well as to obtain facts from the person him or herself.
- Observations – Observing behaviors, interactions, processes, etc., related to the case as they unfold in real-time.
- Documents & Records – Reviewing private documents, diaries, public records, correspondence, meeting minutes, etc., relevant to the case.
2. Secondary sources
- News/Media – News coverage of events related to the case study.
- Academic articles – Journal articles, dissertations etc. that discuss the case.
- Government reports – Official data and records related to the case context.
- Books/films – Books, documentaries or films discussing the case.
3. Archival records
Searching historical archives, museum collections and databases to find relevant documents, visual/audio records related to the case history and context.
Public archives like newspapers, organizational records, photographic collections could all include potentially relevant pieces of information to shed light on attitudes, cultural perspectives, common practices and historical contexts related to psychology.
4. Organizational records
Organizational records offer the advantage of often having large datasets collected over time that can reveal or confirm psychological insights.
Of course, privacy and ethical concerns regarding confidential data must be navigated carefully.
However, with proper protocols, organizational records can provide invaluable context and empirical depth to qualitative case studies exploring the intersection of psychology and organizations.
- Organizational/industrial psychology research : Organizational records like employee surveys, turnover/retention data, policies, incident reports etc. may provide insight into topics like job satisfaction, workplace culture and dynamics, leadership issues, employee behaviors etc.
- Clinical psychology : Therapists/hospitals may grant access to anonymized medical records to study aspects like assessments, diagnoses, treatment plans etc. This could shed light on clinical practices.
- School psychology : Studies could utilize anonymized student records like test scores, grades, disciplinary issues, and counseling referrals to study child development, learning barriers, effectiveness of support programs, and more.
How do I Write a Case Study in Psychology?
Follow specified case study guidelines provided by a journal or your psychology tutor. General components of clinical case studies include: background, symptoms, assessments, diagnosis, treatment, and outcomes. Interpreting the information means the researcher decides what to include or leave out. A good case study should always clarify which information is the factual description and which is an inference or the researcher’s opinion.
1. Introduction
- Provide background on the case context and why it is of interest, presenting background information like demographics, relevant history, and presenting problem.
- Compare briefly to similar published cases if applicable. Clearly state the focus/importance of the case.
2. Case Presentation
- Describe the presenting problem in detail, including symptoms, duration,and impact on daily life.
- Include client demographics like age and gender, information about social relationships, and mental health history.
- Describe all physical, emotional, and/or sensory symptoms reported by the client.
- Use patient quotes to describe the initial complaint verbatim. Follow with full-sentence summaries of relevant history details gathered, including key components that led to a working diagnosis.
- Summarize clinical exam results, namely orthopedic/neurological tests, imaging, lab tests, etc. Note actual results rather than subjective conclusions. Provide images if clearly reproducible/anonymized.
- Clearly state the working diagnosis or clinical impression before transitioning to management.
3. Management and Outcome
- Indicate the total duration of care and number of treatments given over what timeframe. Use specific names/descriptions for any therapies/interventions applied.
- Present the results of the intervention,including any quantitative or qualitative data collected.
- For outcomes, utilize visual analog scales for pain, medication usage logs, etc., if possible. Include patient self-reports of improvement/worsening of symptoms. Note the reason for discharge/end of care.
4. Discussion
- Analyze the case, exploring contributing factors, limitations of the study, and connections to existing research.
- Analyze the effectiveness of the intervention,considering factors like participant adherence, limitations of the study, and potential alternative explanations for the results.
- Identify any questions raised in the case analysis and relate insights to established theories and current research if applicable. Avoid definitive claims about physiological explanations.
- Offer clinical implications, and suggest future research directions.
5. Additional Items
- Thank specific assistants for writing support only. No patient acknowledgments.
- References should directly support any key claims or quotes included.
- Use tables/figures/images only if substantially informative. Include permissions and legends/explanatory notes.
- Provides detailed (rich qualitative) information.
- Provides insight for further research.
- Permitting investigation of otherwise impractical (or unethical) situations.
Case studies allow a researcher to investigate a topic in far more detail than might be possible if they were trying to deal with a large number of research participants (nomothetic approach) with the aim of ‘averaging’.
Because of their in-depth, multi-sided approach, case studies often shed light on aspects of human thinking and behavior that would be unethical or impractical to study in other ways.
Research that only looks into the measurable aspects of human behavior is not likely to give us insights into the subjective dimension of experience, which is important to psychoanalytic and humanistic psychologists.
Case studies are often used in exploratory research. They can help us generate new ideas (that might be tested by other methods). They are an important way of illustrating theories and can help show how different aspects of a person’s life are related to each other.
The method is, therefore, important for psychologists who adopt a holistic point of view (i.e., humanistic psychologists ).
Limitations
- Lacking scientific rigor and providing little basis for generalization of results to the wider population.
- Researchers’ own subjective feelings may influence the case study (researcher bias).
- Difficult to replicate.
- Time-consuming and expensive.
- The volume of data, together with the time restrictions in place, impacted the depth of analysis that was possible within the available resources.
Because a case study deals with only one person/event/group, we can never be sure if the case study investigated is representative of the wider body of “similar” instances. This means the conclusions drawn from a particular case may not be transferable to other settings.
Because case studies are based on the analysis of qualitative (i.e., descriptive) data , a lot depends on the psychologist’s interpretation of the information she has acquired.
This means that there is a lot of scope for Anna O , and it could be that the subjective opinions of the psychologist intrude in the assessment of what the data means.
For example, Freud has been criticized for producing case studies in which the information was sometimes distorted to fit particular behavioral theories (e.g., Little Hans ).
This is also true of Money’s interpretation of the Bruce/Brenda case study (Diamond, 1997) when he ignored evidence that went against his theory.
Breuer, J., & Freud, S. (1895). Studies on hysteria . Standard Edition 2: London.
Curtiss, S. (1981). Genie: The case of a modern wild child .
Diamond, M., & Sigmundson, K. (1997). Sex Reassignment at Birth: Long-term Review and Clinical Implications. Archives of Pediatrics & Adolescent Medicine , 151(3), 298-304
Freud, S. (1909a). Analysis of a phobia of a five year old boy. In The Pelican Freud Library (1977), Vol 8, Case Histories 1, pages 169-306
Freud, S. (1909b). Bemerkungen über einen Fall von Zwangsneurose (Der “Rattenmann”). Jb. psychoanal. psychopathol. Forsch ., I, p. 357-421; GW, VII, p. 379-463; Notes upon a case of obsessional neurosis, SE , 10: 151-318.
Harlow J. M. (1848). Passage of an iron rod through the head. Boston Medical and Surgical Journal, 39 , 389–393.
Harlow, J. M. (1868). Recovery from the Passage of an Iron Bar through the Head . Publications of the Massachusetts Medical Society. 2 (3), 327-347.
Money, J., & Ehrhardt, A. A. (1972). Man & Woman, Boy & Girl : The Differentiation and Dimorphism of Gender Identity from Conception to Maturity. Baltimore, Maryland: Johns Hopkins University Press.
Money, J., & Tucker, P. (1975). Sexual signatures: On being a man or a woman.
Further Information
- Case Study Approach
- Case Study Method
- Enhancing the Quality of Case Studies in Health Services Research
- “We do things together” A case study of “couplehood” in dementia
- Using mixed methods for evaluating an integrative approach to cancer care: a case study

What is the Case Study Method?
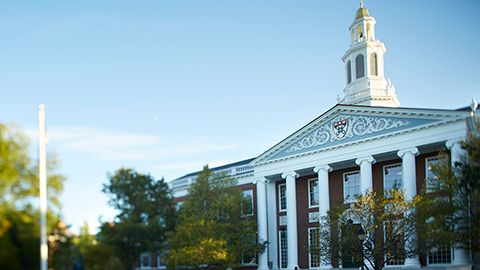
Overview Dropdown up
Overview dropdown down, celebrating 100 years of the case method at hbs.
The 2021-2022 academic year marks the 100-year anniversary of the introduction of the case method at Harvard Business School. Today, the HBS case method is employed in the HBS MBA program, in Executive Education programs, and in dozens of other business schools around the world. As Dean Srikant Datar's says, the case method has withstood the test of time.
Case Discussion Preparation Details Expand All Collapse All
In self-reflection in self-reflection dropdown down, in a small group setting in a small group setting dropdown down, in the classroom in the classroom dropdown down, beyond the classroom beyond the classroom dropdown down, how the case method creates value dropdown up, how the case method creates value dropdown down, in self-reflection, in a small group setting, in the classroom, beyond the classroom.
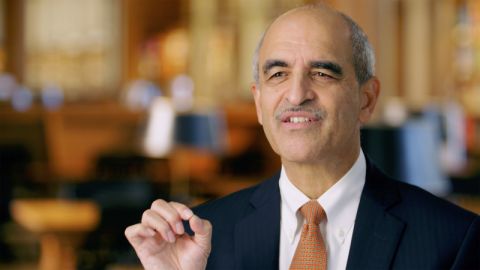
How Cases Unfold In the Classroom
How cases unfold in the classroom dropdown up, how cases unfold in the classroom dropdown down, preparation guidelines expand all collapse all, read the professor's assignment or discussion questions read the professor's assignment or discussion questions dropdown down, read the first few paragraphs and then skim the case read the first few paragraphs and then skim the case dropdown down, reread the case, underline text, and make margin notes reread the case, underline text, and make margin notes dropdown down, note the key problems on a pad of paper and go through the case again note the key problems on a pad of paper and go through the case again dropdown down, how to prepare for case discussions dropdown up, how to prepare for case discussions dropdown down, read the professor's assignment or discussion questions, read the first few paragraphs and then skim the case, reread the case, underline text, and make margin notes, note the key problems on a pad of paper and go through the case again, case study best practices expand all collapse all, prepare prepare dropdown down, discuss discuss dropdown down, participate participate dropdown down, relate relate dropdown down, apply apply dropdown down, note note dropdown down, understand understand dropdown down, case study best practices dropdown up, case study best practices dropdown down, participate, what can i expect on the first day dropdown down.
Most programs begin with registration, followed by an opening session and a dinner. If your travel plans necessitate late arrival, please be sure to notify us so that alternate registration arrangements can be made for you. Please note the following about registration:
HBS campus programs – Registration takes place in the Chao Center.
India programs – Registration takes place outside the classroom.
Other off-campus programs – Registration takes place in the designated facility.
What happens in class if nobody talks? Dropdown down
Professors are here to push everyone to learn, but not to embarrass anyone. If the class is quiet, they'll often ask a participant with experience in the industry in which the case is set to speak first. This is done well in advance so that person can come to class prepared to share. Trust the process. The more open you are, the more willing you’ll be to engage, and the more alive the classroom will become.
Does everyone take part in "role-playing"? Dropdown down
Professors often encourage participants to take opposing sides and then debate the issues, often taking the perspective of the case protagonists or key decision makers in the case.
View Frequently Asked Questions
Subscribe to Our Emails
Thank you for visiting nature.com. You are using a browser version with limited support for CSS. To obtain the best experience, we recommend you use a more up to date browser (or turn off compatibility mode in Internet Explorer). In the meantime, to ensure continued support, we are displaying the site without styles and JavaScript.
- View all journals
- My Account Login
- Explore content
- About the journal
- Publish with us
- Sign up for alerts
- Open access
- Published: 02 May 2024
Research on the method of determining the block size for an open-pit mine integrating mining parameters and shovel-truck’s operation efficiency
- Weiqiang Guo ORCID: orcid.org/0000-0003-0095-2637 1 ,
- Guangwei Liu ORCID: orcid.org/0000-0002-1678-7472 1 ,
- Jiaming Li 1 ,
- Senlin Chai ORCID: orcid.org/0000-0002-8464-9522 2 &
- Shupeng Guo 3
Scientific Reports volume 14 , Article number: 10119 ( 2024 ) Cite this article
Metrics details
- Energy science and technology
- Engineering
The production plan of an open-pit mine depends on the block model, so it's crucial to determine the appropriate method and size for partitioning it. This study proposes a new method based on a closed shell three-dimensional geological model for determining block model size in open-pit mines. Instead of using regular block models, the shell model is directly cut, and the discrete geological body is referred to as the "mining model." Mining parameters and the shovel-truck's performance are integrated into the method. Bench height determines the Z-axis size, bench slope angle determines the inclination angle, and shovel width determines the X-axis size of the block model. The operation efficiency of the shovel-truck considers the probability distribution of simultaneous operations, allowing the determination of the Y-axis size of block models for different types of shovels. The developed "Mining Model" module in the software "Life Cycle Mining System" is used for practical implementation. By comparing the results with traditional block models, the superiority of the proposed method is demonstrated. This study provides a more accurate model for optimizing the production plan of open-pit mines throughout their life cycle.
Introduction
The block model serves as the foundation for various crucial tasks in open-pit mining, including production planning, grade and geological resource reserve estimation, ultimate pit design, and ore quality control. It provides a digital representation of the spatial distribution of deposit attributes. The block model's accuracy and reliability directly impact the investment, production, operational costs, and even the longevity of the mining operation 1 , 2 , 3 , 4 . Moreover, the open-pit mine's three-dimensional deposit model is discretized into a block model, allowing for the simulation of the dynamic evolution process throughout the mining life cycle 5 , 6 .
The Selective Mining Unit (SMU) represents the smallest unit in mining, designated as an ore block if the average grade of the SMU is equal to or higher than the cutoff grade; otherwise, it is considered an unminable waste block 7 . During the production phase of mining, it is theoretically ideal for the block size to align with the SMU, ensuring that the extracted tonnage closely approximates the results of reserve estimation. Therefore, the rational selection of block size in resource reserve estimation is essentially a determination of the SMU. In the case of open-pit mining, the determination of the SMU is influenced by various factors, categorized into geological, technical, and economic factors. Technical factors include mining parameters and equipment operational capabilities specific to open-pit mining.
The choice of block size and shape holds significant importance in resource quality assessment, mine optimization, and mine planning processes, directly influencing the economic viability of mining projects. Intuitively, a smaller block size should result in a more accurate block model. However, during practical implementation, the statistical problems of geological spatial information, and computer computing power constraints pose challenges in determining the optimal block size. Nevertheless, establishing the appropriate block size remains a crucial factor for effective open-pit mine production scheduling and the optimization of mining operations 8 . Currently, the prevailing approach in open-pit mining involves the utilization of the conventional block model construction method. This method entails filling the three-dimensional geological solid model with standardized blocks of a set size. Through this process, the construction of the block model is accomplished, and the three-dimensional geological model is discretized accordingly 9 . Subsequently, the spatial estimation interpolation method is employed to assign the geological attributes to each individual block, thereby facilitating the simulation of open-pit mining operations based on the block's designated mining sequence 10 , 11 .
The determination of block size division parameters is a complex engineering decision that has been extensively studied by numerous scholars. Yarahmadi 12 developed a computer program to determine geometry and size of rock blocks in two dimensional spaces. Hartman 13 emphasized that the size of the block model can be determined by considering the mining method and the selectivity of the ore, aiming to minimize ore dilution. David 14 conducted a comprehensive study on the block size problem utilizing advanced geostatistical methods. The research highlighted the crucial significance of block size selection in determining recoverable resource reserves, evaluating resource quality, optimizing mine designs, and formulating mining plans. The findings emphasized that the choice of block size directly impacts the economic benefits of mining operations. Jara et al. 15 also conducted a study investigating the impact of block model size on open-pit mine design and production planning. They quantified the influence of block model size on mining selectivity, such as grade and dilution, and assessed its effects on the economic outcomes of mining, including income, costs, and discounted cash flow. The findings revealed that as the block size increases, the overall quality of the ore body improves, but the average grade decreases. Additionally, the study observed a decrease in discounted cash flow with larger block model sizes. Similarly, Birch 16 examined the influence of block size on the final grade-tonnage curve and observed its impact on the average grade of the ore body. Furthermore, variations in block size were found to affect the overall income and net present value of the mine. Ruiseco 17 proposed guidelines for determining the block size in relation to the horizontal direction of the ore body. The recommendation suggests that the block size should be greater than 1/4 of the average distance between exploration boreholes and not less than 1/3 of the distance between two boreholes. Furthermore, it is advised that the block height should not exceed the height of the working bench. Furthermore, several researchers have taken into account various uncertainties, including average grade, recovery rate, production capacity, and dilution degree. They have employed Multi-Criteria Decision-Making (MCDM) methods to effectively select the appropriate size of the block model. Leuangthong et al. 18 , 19 highlighted that the determination of block size relies on various factors, including mining equipment, mining method, mining direction, and the sedimentary environment of the ore body. Recognizing the interconnected nature of these factors, they proposed the utilization of the Analytic Hierarchy Process (AHP) to select the optimal block size for mine production planning. Hayati et al. 8 employed the VlseKriterijumska Optimizacija I Kompromisno Resenje (VIKOR) method to ascertain the optimal block size for the Angouran open-pit mine in Iran, resulting in the determination of a 10 m-sized block model. Subsequently, Abdollahei 20 proposed the utilization of the Fuzzy Delphi Analytic Hierarchy Process (FDAHP) and Fuzzy Multi-Objective Ratio Analysis (FMOORA) to optimize the size of the block model. These methods provide a framework for assessing and determining the most favorable block model size. Stevanovic et al. 21 adopted a range of 1/3 to 1/2 of the borehole spacing in the X and Y directions, based on the arrangement of the exploration work, as the division size range for blocks. The Z direction was constrained by the bench height, determining multiple sets of block size division schemes. Ultimately, the (AHP) was utilized to identify the optimal block size for mine production planning, considering the defined range of division sizes.
In summary, researchers have dedicated their efforts to studying the impact of block size on ore dilution and boundary grade, as well as determining the most suitable block size to minimize ore dilution and error in boundary grade selection. In theory, reducing the block size leads to improved accuracy and minimizes the effects on ore dilution and boundary grade. However, practical limitations in computer computing power prevent achieving the ideal block size. It is essential to acknowledge that no matter how small the block size becomes, it can only approximate the original three-dimensional geological body and cannot fully represent the volume and grade of the actual entity. Simultaneously, researchers commonly employ techniques such as integer programming, linear programming, mixed integer programming, and dynamic programming to formulate the mathematical model for open-pit mine production planning 22 , 23 , 24 , 25 . The mathematical models developed using the methods rely on regular cube or cuboid blocks as the foundational representation. However, during the simulation of mine production, the shape of the working bench side often deviates from the regular shape observed in practical mining operations. Meanwhile, the formulation of the open-pit mine production plan does not accurately correspond to the real output of each excavator's working area as depicted in the block model. Therefore, this research plan has introduced a novel approach by altering the foundation of the block model. It proposes a method to divide the size of the open-pit mine block model, taking into account the stripping process parameters and the efficiency of vehicle-shovel equipment.
Open-pit coal mining employs benches as the fundamental mining operational unit, extracting in a strip-sequence manner. The mining parameters and the operational capabilities of the truck-shovel system are inherently related to the block model. Thus, this study departs from the conventional approach of approximating the three-dimensional geological model using regular block models to represent the mining process, instead mapping the stripping parameters and equipment operational capabilities onto the block model. This serves as a dimensional parameter for block model segmentation. This approach can reduce constraints in subsequent studies on optimizing open-pit coal mining plans, enhance the efficiency of solving optimization models, and align more closely with on-site construction and operational realities.
Based on this research approach and considering temporary equipment failures, maintenance, and unbalanced truck-shovel combinations in actual production operations that affect the overall production system output, thereby influencing the practical operational capabilities of the truck-shovel system, the study incorporates a probabilistic analysis method as presented in reference 26 . The literature suggests that the actual number of operational trucks and shovels fluctuates around a certain mathematical expectation, and the study employs probability analysis to investigate the actual operational capabilities of the equipment. Each mining equipment's daily production is considered the volume of an individual mining block in the field, integrating mining parameters. The study proposes a method that integrates mining parameters and truck-shovel equipment operational capabilities into the discretization of a three-dimensional geological model for open-pit coal mining. This approach provides a crucial model foundation for optimizing production plans in open-pit coal mining.
Mining parameters constraint
The conventional approach involves utilizing the standard block model to populate the three-dimensional geological body. Subsequently, the dynamic deduction of the open-pit mine production plan is carried out from this foundation, as depicted in Fig. 1 . When the block model is filled to the irregular final pit boundary, if the centroid position of the block is within the boundary, the block is filled, but there will be a multi-volume calculation part. If the mass center point of the block is outside the boundary, it will not be filled, but the volume will be undercounted, as shown in Fig. 2 a. In cases where the geological body is extensive, and there exist thousands of block models, the cumulative effect of errors arising from block filling at the final pit boundary becomes significant. This, in turn, adversely impacts the accuracy of determining the quantity of ore and rock during the formulation of the production plan. The block model proposed in this study is generated by cutting directly on the closed shell 3D geological body, as shown in Fig. 3 , and the discrete geological body is defined as ‘Mining model’.
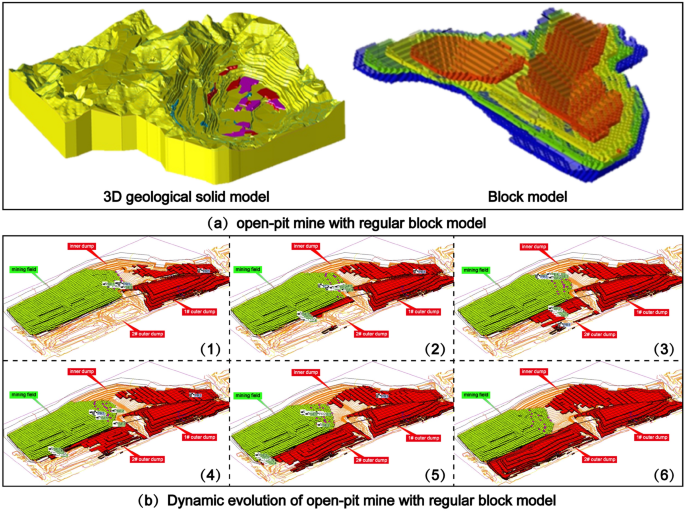
Dynamic evolution of the open-pit mine production scheduling with regular block models.
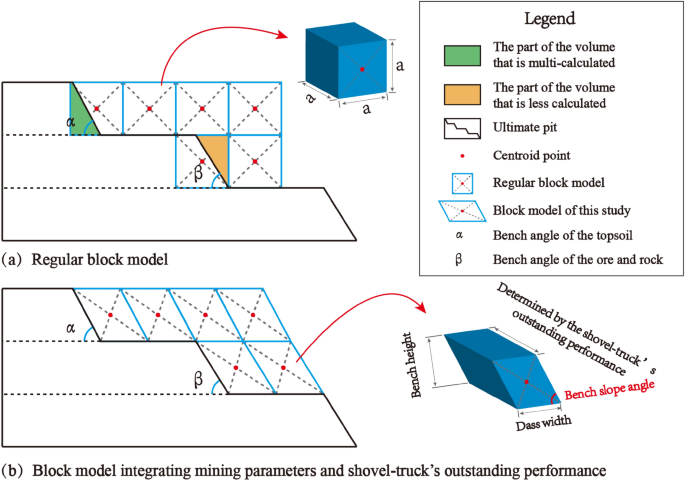
Comparison of ore deposit block models.
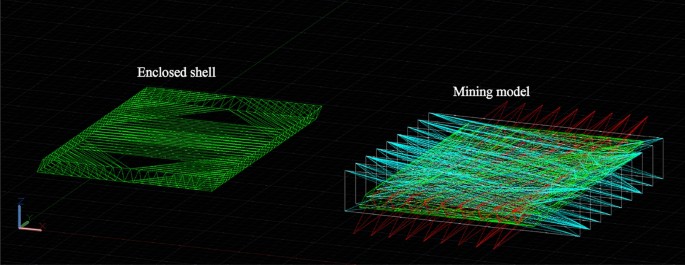
Mining model.
The mining parameters depend on the open-pit mining technology. The basic parameters include bench height h , bench slope angle α , mining belt width A , and bench width B 27 . Taking into consideration the on-site operations, the constrained block model incorporates the actual dimensions. The Z -axis size of the block corresponds to the bench height h . The Y -axis size represents the mining belt width A . The block dip angle is equivalent to the bench slope angle α . Lastly, the X -axis size is determined based on the daily operation efficiency of various excavator types. The division method proposed in this paper has no deviation from the three-dimensional geological body in accuracy and ensures the integrity of the step shape. The specific morphology is shown in Fig. 2 b.
Bench height constraint
The ore and rock in open-pit mines is generally divided into multiple benches for extraction. The division of benches should be conducive to maximizing equipment efficiency, improving ore quality, and ensuring operational safety. The bench height is one of the most important geometric parameters in open-pit mining 28 . Factors influencing the bench height include the burial conditions and properties of rock formations, operational conditions of mining equipment, drilling and blasting operations, production scale, technical specifications of mining and loading equipment, layout of transportation routes, and mining advancing speed, among others. For excavators with a bucket capacity of 3 m 3 to 4 m 3 , the bench height ranges from 10 to 15 m. Larger excavators can have bench heights of up to 20 m to 25 m, while large recasting excavators can reach bench heights of over 30 m. Wheel and chain excavators can have bench heights of 40 m to 50 m. Therefore, the selection of bench height should be determined based on the type of excavator.
Bench slope angle constraint
The bench slope angle primarily depends on the stability of the rock mass, and its value increases as the rock mass stability improves. Furthermore, the inclination of the bedding plane in the rock mass directly affects the bench slope angle. When the inclination of the bedding plane aligns or closely resembles that of the bench slope, and the bedding plane has a steep dip angle, the bench slope angle equals the inclination of the bedding plane.
Mining belt width constraint
In mining operations, the benches are typically divided into sequential strips of certain widths based on the direction of rock layers, known as mining belt. The mining belt width represents the width of excavation carried out by the excavator in one pass, and it influences various aspects such as drilling and blasting, mining and loading, track relocation, and bench width. Consequently, the mining belt width plays a crucial role in determining the progress of stripping and mining operations. In cases where the ore and rock are soft and do not require blasting, the mining area width is equivalent to the mining belt width. However, for ore and rock that necessitate blasting, the mining belt width generally refers to the width of one mining operation after a single blast, commonly known as "one blasting, two mining" or "one blasting, one mining". The determination of the mining belt width is primarily based on the technical specifications of the excavator. It is important to ensure that the mining belt width does not exceed 1.5 times the radius of the maximum excavator at the standing level, denoted as A ≤ 1.5 R wp . Additionally, the minimum width of the mining belt should meet the following requirements:
where \(e\) is the safe distance from the center line of the drilling rig to the top line of the bench slope, generally 2.5–3 m; \(h\) is bench height, m; \(\alpha\) is bench slope angle, °.
When using track transportation, due to its flexibility, small turning radius, and climbing ability, it can effectively cooperate with the mining equipment in the production to improve the mining efficiency. Simultaneously, the mining belt width can be set without considering the problem of blasting pile buried road after blasting. The mining belt width can be larger, which is only related to the technical specifications of the excavator, the shunting mode and the safe distance of the transportation channel.
Shovel-truck’s operation efficiency constraint
Equipment operation efficiency refers to the ability of equipment to achieve maximum production efficiency with minimal resource consumption, while ensuring quality during the production process 29 , 30 . In this study, the analysis and calculation will be carried out using the example of the coordinated operation between open-pit mining shovels and trucks. Furthermore, to align with practical applications, the matching operation of multiple equipment models between self-operated and outsourcing units is taken into consideration.
In a multi-link production system, the equipment within each production link is operated in a sequential and mutually constrained manner. The production system consists of M production links. The i link contains N i equipment with an average operation rate of p i . The actual number of units in the system will fluctuate around a specific mathematical expectation, causing corresponding changes in the overall system index. Probability analysis can be employed to study this distribution characteristic, facilitating the rational selection and utilization of equipment.
Probability distribution of simultaneous operation equipment quantity
The self-operated and outsourced units within the system collectively possess x devices, and the probability of simultaneous operation follows a binomial distribution:
where N is the total number of equipment, N S denotes the number of shovels, and N T represents the number of trucks; x is the number of devices operating simultaneously; p is the average operating rate of the equipment, p s is the average operating rate of the shovels, and p t is the average operating rate of the trucks.
For convenience, the following recursive formula can be utilized to calculate the probability values for different x :
From Eq. ( 3 ), the probability distribution matrix of the number of shovel-truck simultaneous operations can be obtained. Among them:
where \(P\left( {i,j} \right)\) is the probability of simultaneous with i shovels and j trucks; \(P_{s} \left( i \right)\) is the probability of i shovels operating simultaneously; \(P_{t} \left( j \right)\) is the probability of j trucks operating simultaneously.
Assuming the system consists of: shovel N s = 1 and truck N T = 5. The shovel operation rate is p s = 0.8, and the truck operation rate is p t = 0.75. The probability distribution of simultaneous shovel-truck operations can be found in Table 1 .
By conducting an analysis of Table 1 , it becomes evident that the probability matrix can be effectively partitioned into three distinct regions:
No product area. That is, the number of working shovels or the trucks is zero.
Oversaturated area. It refers to the area where the truck to shovel ratio is too large and the truck cannot give full play to its efficiency. When the quantities of trucks and shovels are not zero and the shovel quantity is fixed, the probability values gradually increase as the number of trucks grows. Once a certain quantity is reached, the probability values start to decrease. The region where the probability values decrease is defined as the oversaturated area.
The research findings indicate that Fig. 4 illustrates the correlation between the efficiency of each shovel or truck and the truck to shovel ratio. When the truck to shovel ratio K is below a critical value K 0 , the efficiency Q s of each shovel demonstrates a proportional relationship with the truck to shovel ratio K . However, once K > K 0 , the growth rate of Q s becomes significantly sluggish. The efficiency Q t of each truck primarily remains constant when K < K 0 . However, when K > K 0 , the efficiency Q t becomes inversely proportional to K , indicating that as K increases, the efficiency of each truck decreases. The region where K > K 0 is referred to as the oversaturated area, signifying an excessive number of trucks that prevent the improvement of shovel efficiency and lead to a decrease in truck efficiency. This ultimately results in wasteful utilization of equipment. In Table 1 , the division is made based on K 0 = 4 to delineate the different areas.
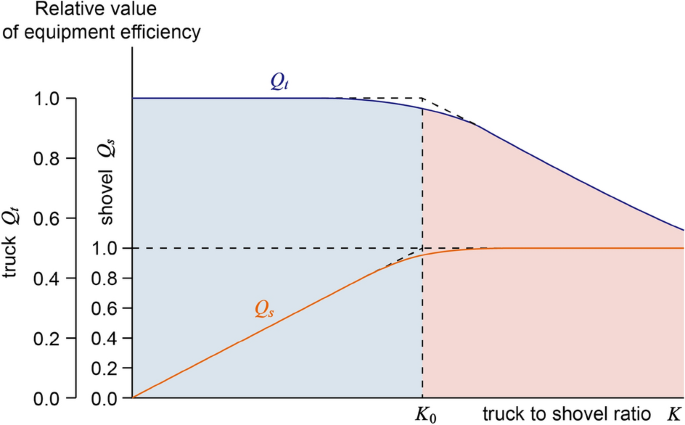
Relationship between efficiency of truck and shovel and truck to shovel ratio.
General operating area: This classification encompasses the regions that are not part of the previously mentioned no product area and oversaturated area.
System production capacity and equipment efficiency
The total system production capacity can be obtained by Eq. ( 5 ):
where Q c is the total system production capacity; P ( i , j ) is the probability of i shovels and j trucks operating simultaneously; Q ( i , j ) is the system production capacity of i shovels and j trucks operating simultaneously; N s is the number of shovels in the system; N t is the number of trucks in the system.
Considering the simultaneous operation of both self-operated and outsourced equipment, a combination of various types of shovel and truck equipment is employed to work together. As a result, the total system production capacity can be determined:
where Q m is the production capacity of each set of shovel-truck; \(N_{t}^{\prime}\) is the number of trucks calculated, when \(j \le iK_{0}\) , \(N_{t}^{\prime} = j\) , when \(j > iK_{0}\) , \(N_{t}^{\prime} = iK_{0}\) ; Q 0 is the efficiency of each truck when shovels and trucks are working continuously; K 0 is the truck to shovel ratio saturation value (round-off number).
The average efficiency of each equipment is:
where Q s is the average efficiency per shovel; Q t is the average efficiency per truck.
Suppose \(Q_{0} = 10^{6}\) t/a, \(K_{0} = 4\) ,the following results are obtained.
When \(N_{s} = 1\) , \(N_{t} = 5\) , \(Q_{c} = 2.810264 \times 10^{6}\) t/a; \(Q_{s} = 2.810264 \times 10^{6}\) t/a; \(Q_{t} = 5.62053 \times 10^{5}\) t/a.
Block model size
The volume \(V\) of a single block is determined by the daily production capacity of a shovel. The number of working days per year is calculated at 330. Once the mining technology and operational equipment model are determined, the bench height h , the bench slope angle α , and the bench width A , that is, Z -axis size \(\overset{\lower0.5em\hbox{$\smash{\scriptscriptstyle\frown}$}}{Z}\) and Y -axis size \(\overset{\lower0.5em\hbox{$\smash{\scriptscriptstyle\frown}$}}{Y}\) of the block model can be determined. With these values known, the X -axis size \(\overset{\lower0.5em\hbox{$\smash{\scriptscriptstyle\frown}$}}{X}\) can be obtained using the volume calculation Eqs. ( 10 ) and ( 11 ).
where \(\tilde{Q}\) is the shovel’s daily operation efficiency.
The specific process of the method proposed in this study is depicted in Fig. 5 .
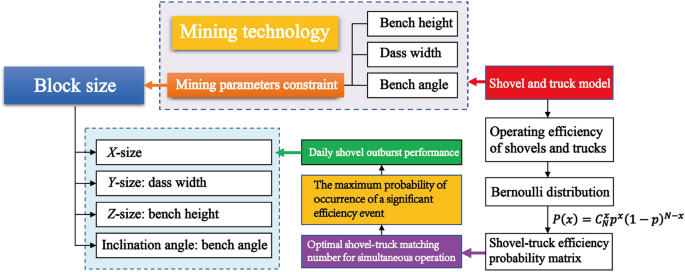
Flow chart of the block model size determination method.
This study utilizes the Baorixile open-pit mine as a case study (as shown in Fig. 6 ). The Chenbaerhuqi coal field in Hulunbuir City, Inner Mongolia Autonomous Region of China, is where the Baorixile open-pit coal mine is situated 31 , 32 . The mine's external perimeter spans a 50.72 km 2 region, measuring 5.86 km in width from north to south and 10.98 km in length from east to west. The mine was constructed in 1998. The primary coal seams targeted for mining are coal B, coal 1 2 , coal 2 1 , and coal 3 1 . The original design’s annual production capacity was 1.80 million t. In 2014, the approved production capacity of the Baorixile Open-pit Coal Mine was 35 million t/a. The semi-continuous mining technology of shovel-single bucket truck-semi-fixed crushing station is adopted.
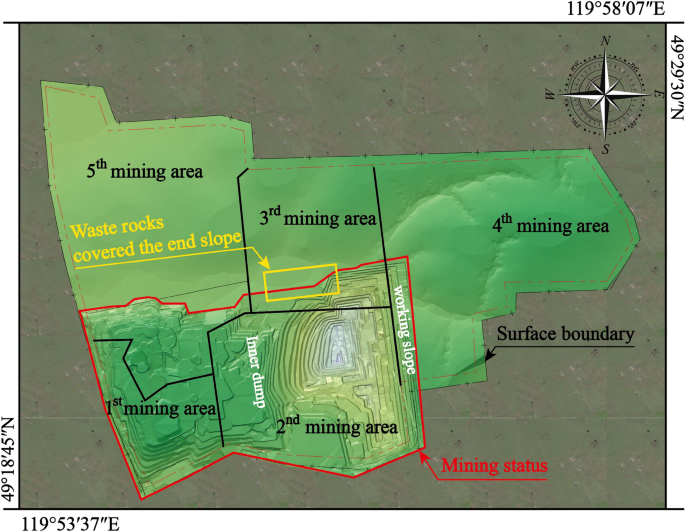
The Baorixile open-pit mine.
The self-operated and outsourcing units’ shovels and trucks of the open-pit mine simultaneously performs on-site coal mining and rock stripping operations 33 . The models of self-operated trucks and shovel are as follows:
Coal mining equipment models
The single bucket excavators of WK-35 (bucket capacity 35 m 3 ), WK-10B (bucket capacity 10 m 3 ) and WK-12 (bucket capacity 12 m 3 ) are selected for the coal mining equipment, and the dump trucks of MT4400AC-220T (load 220t) and TR100 (load 91t) are selected for the coal transportation equipment.
Rock stripping equipment models
The single bucket excavators of WK-35 (bucket capacity 35 m 3 ), WK-10B (bucket capacity 10 m 3 ) and WK-12 (bucket capacity 12 m 3 ) are selected for rock stripping equipment, and the dump trucks of MT4400AC-220T (load 220t) and TR100 (load 91t) are selected for rock transportation equipment.
The outsourcing equipment is mainly responsible for rock stripping, and their models are 4 m 3 hydraulic shovel and 60t dump truck.
Table 2 presents the models, quantities, and operational efficiencies of all shovels and trucks utilized in the open-pit mine.
Block model
Mining parameter.
The following mining parameters are obtained from the preliminary design report of the mine 33 .
Bench height
The bench height is determined by considering the properties of the stripping soil and rock, process characteristics, equipment specifications, mining requirements, and a comprehensive assessment aimed at enhancing equipment operating conditions and improving production efficiency 34 . Bench height stands as a significant mining parameter in open-pit mines. To determine the appropriate bench height for stripping and coal mining operations, several factors are considered, including the maximum excavation height of hydraulic excavators, production stripping ratio, and other relevant considerations.
Based on a careful evaluation of the physical and mechanical properties of the stripping material and its burial conditions, as well as considering the specifications of the mining equipment, the determined stripping step height for the mine is 15 m (that is \(\overset{\lower0.5em\hbox{$\smash{\scriptscriptstyle\frown}$}}{Z}_{r}\) = 15 m). The benches are horizontally divided to ensure effective and efficient mining operations.
The coal seam of this mine is a nearly horizontal and the dip angle is 1–3°. Hence, the standard coal bench height is set at 15 m, denoted as \(\overset{\lower0.5em\hbox{$\smash{\scriptscriptstyle\frown}$}}{Z}_{c}\) = 15 m.
Bench slope angle
The topsoil bench slope angle is 65°, that is α t = 65°; the coal and rock bench slope angle are 70°, that is α c = 70°, α r = 70°.
Mining belt width
Based on process characteristics, it is observed that a wider mining belt width leads to a reduced number of pit lines in the annual operation, resulting in higher system efficiency. However, an increase in the mining belt width will result in a slower working slope angle, leading to an increase in the amount of stripping work required. Considering the specifications of the working equipment, mining and loading conditions, and other relevant factors, the mining belt width for each stripping equipment is determined as follows:
The mining belt width of shovel WK-35 is 25 m, that is \(\overset{\lower0.5em\hbox{$\smash{\scriptscriptstyle\frown}$}}{Y}_{WK - 35} = 25\) m;
The bench width of shovel WK-10B is 20 m, that is \(\overset{\lower0.5em\hbox{$\smash{\scriptscriptstyle\frown}$}}{Y}_{WK - 10B} = 20\) m;
The bench width of shovel WK-12 is 20 m, that is \(\overset{\lower0.5em\hbox{$\smash{\scriptscriptstyle\frown}$}}{Y}_{WK - 12} = 20\) m;
The mining belt width of 4 m 3 hydraulic backhoe is 10 m, that is \(\overset{\lower0.5em\hbox{$\smash{\scriptscriptstyle\frown}$}}{Y}_{{4m^{3} }} = 10\) m.
Shovel-truck’s operation efficiency
Table 2 illustrates the operational efficiency of various shovel types in both self-operated and outsourcing units as follows: P WK-35 = 0.96, P WK-10B = 0.95, P WK-12 = 0.92, P 4m3 = 0.93. Operating efficiency of different types of trucks: P MT4400AC-220 T = 0.93, P TR100 = 0.94, P 60t = 0.80.
Based on Eq. ( 3 ), the probability distribution of the number of shovels and trucks operating simultaneously can be calculated, as demonstrated in Table 3 .
Due to the extensive volume of data, only a portion of the data is presented in Table 3 . Based on the data calculation presented in Table 3 , it can be concluded that the maximum probability of simultaneous operation for all self-operated and outsourced equipment is P = 2.8483E−04. Then the combination of utilizing 6 WK-35, 4 WK-10B, 2 WK-12, 28 MT4400AC-220T, 32 TR100, 106 4 m 3 hydraulic shovels, and 365 60t dump trucks exhibits the highest probability, as depicted in Table 4 . Simultaneously, Table 4 showcases the specific combinations of trucks and shovels employed in the actual production operations of the open-pit mine.
According to the specific saturation value K 0 of each combination of trucks and shovels in Table 4 , as well as the working efficiency of each type of truck when both the shovel and the truck are continuously operated, the production capacity of each combination can be calculated using Eq. ( 7 ) as follows:
WK-35 + MT4400AC-220T:
\(Q_{WK - 35} = \sum\limits_{i = 1}^{{N_{s} }} {\sum\limits_{j = 1}^{{iK_{0} }} {P\left( {i,j} \right) \cdot j \cdot Q_{MT400AC - 200T} } } = 1954.16 \cdot {1}0^{{4}} \;{\text{m}}^{{3}} {\text{/a}}\)
WK-10B + TR100T:
\(Q_{WK - 10B} = \sum\limits_{i = 1}^{{N_{s} }} {\sum\limits_{j = 1}^{{iK_{0} }} {P\left( {i,j} \right) \cdot j \cdot Q_{TR100T} } } = 993.21 \cdot {1}0^{{4}} \;{\text{m}}^{{3}} {\text{/a}}\)
WK-12 + TR100T:
\(Q_{WK - 12} = \sum\limits_{i = 1}^{{N_{s} }} {\sum\limits_{j = 1}^{{iK_{0} }} {P\left( {i,j} \right) \cdot j \cdot Q_{TR100T} } } + \sum\limits_{i = 1}^{{N_{s} }} {\sum\limits_{{i = iK_{0} + 1}}^{{N_{t} }} {P\left( {i,j} \right) \cdot iK_{0} \cdot Q_{TR100T} } } = 608.29 \cdot {1}0^{{4}} \;{\text{m}}^{{3}} {\text{/a}}\)
4 m 3 hydraulic backhoe + 60t dump truck:
\(Q_{{4m^{3} }} = \sum\limits_{i = 1}^{{N_{s} }} {\sum\limits_{j = 1}^{{iK_{0} }} {P\left( {i,j} \right) \cdot j \cdot Q_{60t} } } + \sum\limits_{i = 1}^{{N_{s} }} {\sum\limits_{{i = iK_{0} + 1}}^{{N_{t} }} {P\left( {i,j} \right) \cdot iK_{0} \cdot Q_{60t} } } = 10313.50 \cdot {1}0^{{4}} \;{\text{m}}^{{3}} {\text{/a}}\)
The daily operation efficiency of different types of shovels can be calculated using Eq. ( 11 ).
\(\tilde{Q}_{WK - 35} = \frac{1954.16}{{6 \times 330}} \times 10000 = 9869.50\;{\text{m}}^{{3}} {\text{/d;}}\)
\(\tilde{Q}_{WK - 10B} = \frac{993.21}{{4 \times 330}} \times 10000 = 7524.32\;{\text{m}}^{{3}} {\text{/d;}}\)
\(\tilde{Q}_{WK - 12} = \frac{608.29}{{2 \times 330}} \times 10000 = 9216.52\;{\text{m}}^{{3}} {\text{/d;}}\)
\(\tilde{Q}_{{4m^{3} }} = \frac{10313.50}{{106 \times 330}} \times 10000 = 2948.40\;{\text{m}}^{{3}} {\text{/d}}{.}\)
Based on the mining parameters outlined in section " Mining parameter " and the daily operation efficiency of each shovel model calculated in section " Shovel-truck’s operation efficiency ", the X -axis size of the block model for each shovel's working area are calculated using Eq. ( 11 ) as follows:
One of the working areas of the shovel WK-35 is at the level of 565 m to 580 m. The X -axis size of the block model for this specific bench is: \(\overset{\lower0.5em\hbox{$\smash{\scriptscriptstyle\frown}$}}{X}_{WK - 35} = \frac{{\tilde{Q}_{WK - 35} }}{{\overset{\lower0.5em\hbox{$\smash{\scriptscriptstyle\frown}$}}{Y}_{WK - 35} \cdot \overset{\lower0.5em\hbox{$\smash{\scriptscriptstyle\frown}$}}{Z} }} = \frac{9869.50}{{25 \times 15}} = 26\) m;
One of the working areas of the shovel WK-10B is at the level of 505 m to 520 m. The X -axis size of the block model for this specific bench is: \(\overset{\lower0.5em\hbox{$\smash{\scriptscriptstyle\frown}$}}{X}_{WK - 10B} = \frac{{\tilde{Q}_{WK - 10B} }}{{\overset{\lower0.5em\hbox{$\smash{\scriptscriptstyle\frown}$}}{Y}_{WK - 10B} \cdot \overset{\lower0.5em\hbox{$\smash{\scriptscriptstyle\frown}$}}{Z} }} = \frac{7524.32}{{20 \times 15}} = 25\) m;
One of the working areas of the shovel WK-12 is at the level of 490 m to 505 m. The X -axis size of the block model for this specific bench is: \(\overset{\lower0.5em\hbox{$\smash{\scriptscriptstyle\frown}$}}{X}_{WK - 12} = \frac{{\tilde{Q}_{WK - 12} }}{{\overset{\lower0.5em\hbox{$\smash{\scriptscriptstyle\frown}$}}{Y}_{WK - 12} \cdot \overset{\lower0.5em\hbox{$\smash{\scriptscriptstyle\frown}$}}{Z} }} = \frac{9216.52}{{20 \times 15}} = 30\) m;
One of the working areas of the 4 m 3 hydraulic backhoes is at the level of 590 m to 610 m. The X -axis size of the block model for this specific bench is: \(\overset{\lower0.5em\hbox{$\smash{\scriptscriptstyle\frown}$}}{X}_{{4m^{3} }} = \frac{{\tilde{Q}_{{4m^{3} }} }}{{\overset{\lower0.5em\hbox{$\smash{\scriptscriptstyle\frown}$}}{Y}_{{4m^{3} }} \cdot \overset{\lower0.5em\hbox{$\smash{\scriptscriptstyle\frown}$}}{Z} }} = \frac{2948.40}{{10 \times 15}} = 20\) m;
The "Mining model" function module of the self-developed "Life Cycle Mining Software System" was developed using the C++ programming language. The module successfully realizes the construction of the block model for this study, as depicted in Fig. 7 .
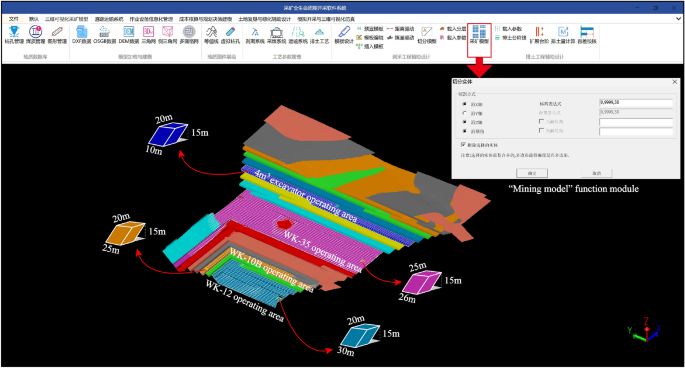
The self-developed software system and the generation of the ‘Mining model’.
Accuracy comparative analysis
The closed shell model is a geometric representation defined by its surface or boundary, encompassing only the outer surface or boundary without considering the specific shape of the interior set. The solid model provides a higher level of detail as it encompasses the internal structure and geometry. In comparison to the block model, the solid model offers enhanced computational accuracy; however, it comes with a trade-off of significantly slower efficiency. If the block were to be divided based on mining process parameters and equipment efficiency using the solid model as a foundation, the limited computing power of the computer would hinder the completion of this operation. In the case of layered deposits, there is no disparity in volume accuracy between the closed shell model and the solid model. Additionally, the computer exhibits fast operation speed when performing segmentation on the shell model. Consequently, this study opts for the closed shell model to construct the “Mining model”. To assess the superiority of the proposed method in terms of volume accuracy, a comparative analysis was conducted using the four-year mining and stripping engineering models of Baorixile open-pit mine. The selected time periods for analysis encompassed the years 2021 to 2022, 2022 to 2023, 2023 to 2024, and 2023 to 2024. This study employed these specific models to facilitate a comprehensive evaluation. Figure 8 illustrates the closed shell model depicting the mining and stripping engineering operations over a span of four years. The annual stripping engineering model is constructed separately using block configurations of 3 * 3 * 3, 6 * 6 * 6, 12 * 12 * 12, as shown in Fig. 9 .
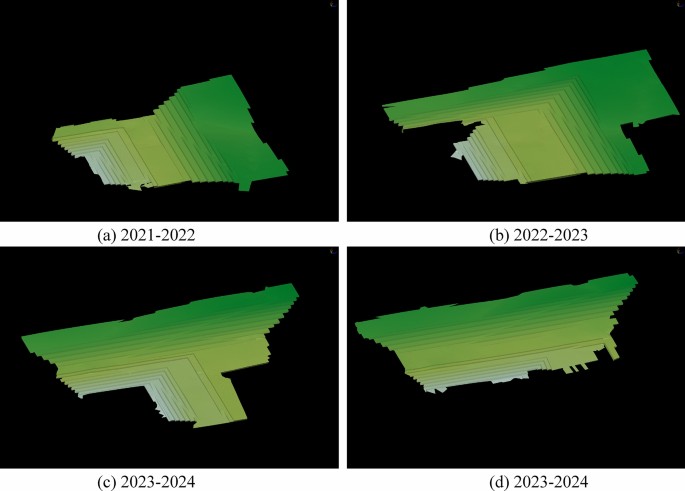
Closed shell model of 2021–2025 mining and stripping project in Baorixile open-pit mine.
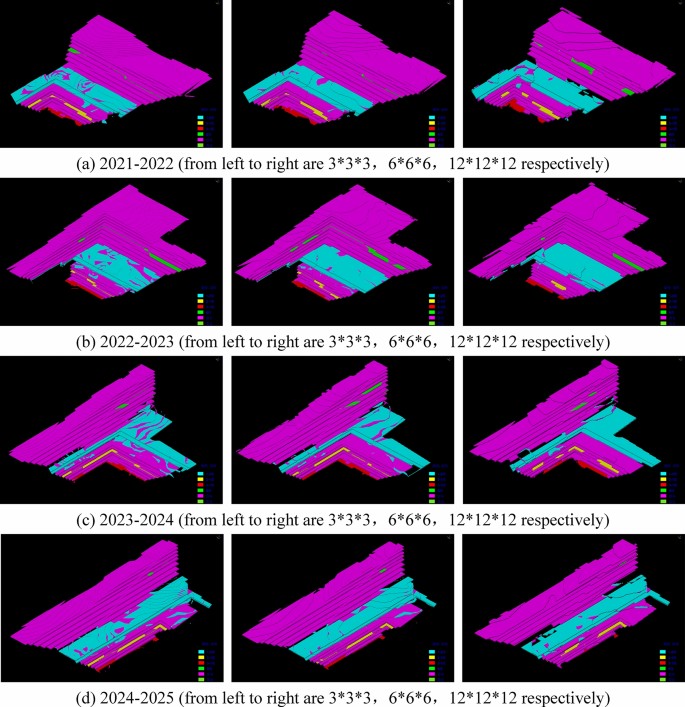
Block models of mining engineering with different specifications in 2021–2025.
Table 5 illustrates the annual quantities of Coal B, Coal 1 2 , Coal 2 1 , and Coal 3 1 , as well as the total coal quantity, total volume, and error between the closed shell model and the block models, within the closed shell model and the corresponding 3 * 3 * 3, 6 * 6 * 6, 12 * 12 * 12 three different specifications of the block model of the mining and stripping project for the four years production plans.
To provide a clearer representation of the engineering quantity errors between the three different specifications of the block model and the closed shell model at each stage, two comparison diagrams (refer to Figs. 10 and 11 ) are presented. These diagrams aim to enhance the understanding and visual depiction of the variations in engineering quantity. After conducting a comprehensive comparison, it is evident that the 12 * 12 * 12 block model generally exhibits the highest error, while the 3 * 3 * 3 block model demonstrates the lowest error in general. Nevertheless, it is not necessarily that as the regular block model size decreases, the accuracy tends to improve. For instance, the 6 * 6 * 6 block model for the 2024–2025 period in Fig. 10 a and for the 2021–2022 and 2022–2023 periods in Fig. 10 c, exhibit higher accuracy compared to the 3 * 3 * 3 block model. The 12 * 12 * 12 block model exhibit higher accuracy compared to the 3 * 3 * 3 block model for the 2023–2024 period in Fig. 10 e. In the design of the production plan for the next few years, the open-pit mine will not always carry out the experiment of optimal block size selection. Therefore, the presence of interference factors resulting from human subjective consciousness continues to exert a significant influence on the accuracy. Upon error superposition, the block specification with the highest error in the 2021–2022 period, as depicted in Fig. 11 e, is 12 × 12 × 12, with a maximum error reaching 7.4252%. Similarly, in Fig. 11 f, the block specification with the highest error is 6 × 6 × 6, with a maximum error of 2.4798%. This highlights the challenge in selecting the optimal block size when utilizing a regular block model for constructing a three-dimensional geological body. Simultaneously, the findings also demonstrate that the block division method proposed in this study yields the highest level of accuracy in terms of volume precision. The method introduced in this study effectively mitigates volume errors associated with utilizing a regular block model to populate the three-dimensional geological model for production planning design. Moreover, it ensures the regularity of mining benches, aligning more closely with the practical construction conditions in open-pit mines.
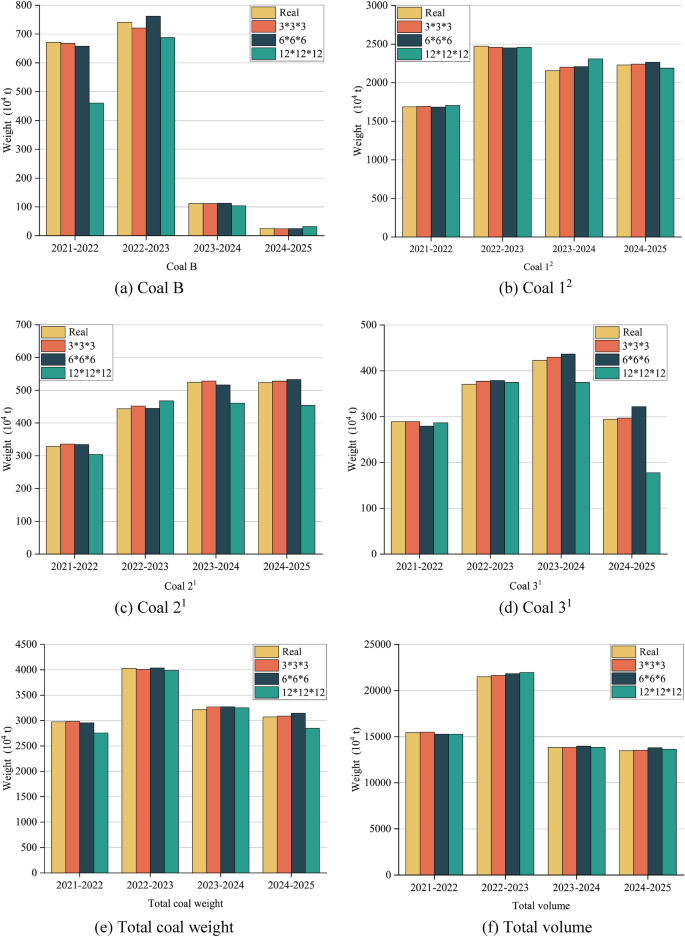
The comparison of coal quantity of each layer, total coal quantity and total volume between the closed shell model and the corresponding block model for 4 years.
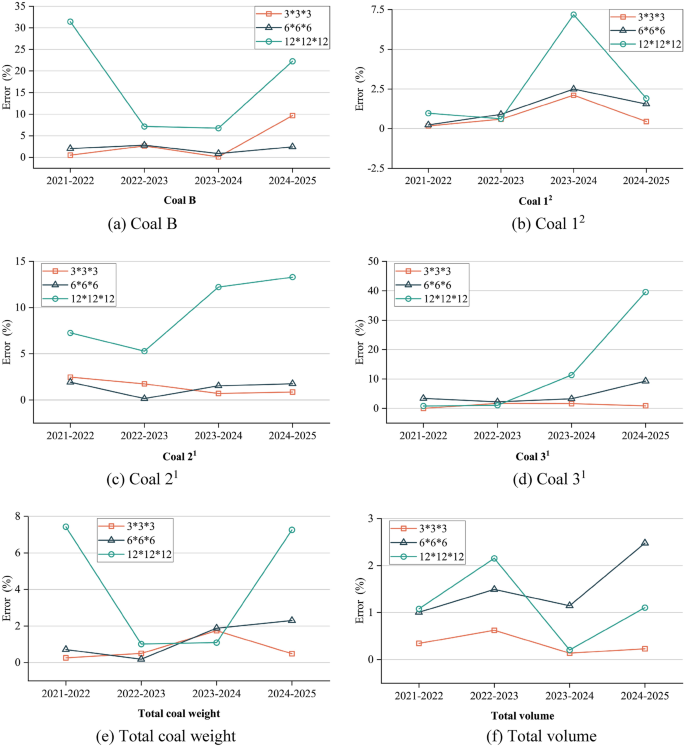
The accuracy error of 4 years of different specifications of mining and stripping engineering block model.
Discussions
In this study, the aim was to enhance the accuracy of the foundational model for production planning by expanding the application of the open-pit mine three-dimensional geological model. To achieve this, a departure from the conventional approach of employing a regular block model to populate the solid model was pursued. To achieve this, a departure from the conventional approach of employing a regular block model to populate the solid model was pursued. The closed shell model is directly discretized into a block model while incorporating the constraints imposed by mining parameters and shovel-truck’s operation efficiency. This study serves as a foundation for our future research on attribute assignment of the mining model, block model migration order, and migration path prediction. Our overarching objective is to develop a comprehensive optimization method for production planning throughout the life cycle of open-pit mines, and to create a prototype system for decision-making in open-pit mine life cycle mining planning. It is noteworthy that in this study, the daily equipment operation efficiency is utilized to determine the X-axis size of the block. However, it is also possible to partition the blocks based on weekly or monthly equipment operation efficiency, enabling the creation of long-term production plans. Based on the diverse equipment types and their respective layout positions, the block size of the equipment's operational area can be determined. This approach aligns more closely with the actual operational conditions. Simultaneously, it significantly reduces the number of blocks, minimizes the computer's processing time, and enhances overall work efficiency. However, it is important to note that this study focuses specifically on the shovel-truck intermittent mining technology, and there remains a dearth of research and development regarding other mining technologies. Subsequent research endeavors will aim to build upon and expand the findings of this study, with the goal of further improvement and in-depth exploration.
Conclusions
The following conclusions and comments can be made from the present study:
Based on the three-dimensional geological model of the closed shell type, the block model is generated considering mining parameters as constraints. Specifically, the bench height is employed as the Z -axis size of the block model, the mining belt width of is adopted as the Y -axis size of the block model, and the bench slope angle serves as the inclination angle of the block model.
Considering the random fluctuation of the number of equipment, compared with the method of calculating equipment efficiency and output by average index, the results of equipment operation efficiency obtained by probability analysis method are more in line with the actual situation. In this study, the daily equipment outburst performance of each type of excavator is calculated based on the maximum probability of simultaneous operation of self-operated and outsourced units. This calculation aids in determining the X -axis size of the block model in the operational area of different types of excavators.
In this paper, using Baorixile open-pit mine as a case study, a ‘Mining model’ function module is developed within the self-developed system. A closed shell block model of the mining and stripping project at the open-pit mine for a four-year period from 2021 to 2025 was created. The regular block models of 3 * 3 * 3, 6 * 6 * 6, and 12 * 12 * 12 were selected and compared to the block model used in this study. This highlights the challenge in selecting the optimal block size when utilizing a regular block model for constructing a three-dimensional geological body. Simultaneously, the findings also demonstrate that the block division method proposed in this study yields the highest level of accuracy in terms of volume precision. The method introduced in this study effectively mitigates volume errors associated with utilizing a regular block model to populate the three-dimensional geological model for production planning design. Moreover, it ensures the regularity of mining benches, aligning more closely with the practical construction conditions in open-pit mines.
Data availability
The data used and analysed during the current study available from the corresponding author on reasonable request.
Osanloo, M., Gholamnejad, J. & Karimi, B. Long-term open pit mine production planning: A review of models and algorithms. Int. J. Min. Reclam. Environ. 22 (1), 3–35 (2008).
Article Google Scholar
Li, J. Analysis of Medium and Long-Term Open-Pit Planning Under Massive Blocks (Xi’an University of Architecture and Technology, 2022).
Google Scholar
Cullenbine, C., Wood, R. K. & Newman, A. A sliding time window heuristic for open pit mine block sequencing. Optim. Lett. 5 , 365–377 (2011).
Article MathSciNet Google Scholar
Mousavi, A., Kozan, E. & Liu, S. Q. Open-pit block sequencing optimization: A mathematical model and solution technique. Eng. Optim. 48 (11), 1932–1950 (2016).
Fathollahzadeh, K. et al. A mathematical model for open pit mine production scheduling with Grade Engineering ® and stockpiling. Int. J. Min. Sci. Technol. 31 (4), 717–728 (2021).
Both, C. & Dimitrakopoulos, R. Integrating geometallurgical ball mill throughput predictions into short-term stochastic production scheduling in mining complexes. Int. J. Min. Sci. Technol. 33 (2), 185–199 (2023).
Sari, Y. A. & Kumral, M. Dig-limits optimization through mixed-integer linear programming in open-pit mines. J. Oper. Res. Soc. 69 (2), 171–182 (2018).
Article ADS Google Scholar
Hayati, M., Rajabzadeh, R. & Darabi, M. Determination of optimal block size in Angouran mine using VIKOR method. J. Mater. Environ. Sci. 6 (11), 3236–3244 (2015).
CAS Google Scholar
Sirelda, B. & Resmi, K. The ore deposit 3D modelling, new effective solution in the optimization of geological and mining works. Earth Sci. 6 (3), 35–43 (2017).
Nancel-Penard, P., Morales, N. & Cornillier, F. A recursive time aggregation-disaggregation heuristic for the multidimensional and multiperiod precedence-constrained knapsack problem: An application to the open-pit mine block sequencing problem. Eur. J. Oper. Res. 303 (3), 1088–1099 (2022).
Paithankar, A. et al. Simultaneous stochastic optimization of production sequence and dynamic cut-off grades in an open pit mining operation. Resour. Policy 66 , 101634 (2020).
Yarahmadi, R. et al. Development of 2D computer program to determine geometry of rock mass blocks. Int. J. Min. Sci. Technol. 24 (2), 191–194 (2014).
Hartman, H. L. SME Mining Engineering Handbook (Society for Mining, Metallurgy, and Exploration, 1992).
David, M. Geostatistical Ore Reserve Estimation (Elsevier, 2012).
Jara, R. M., Couble, A., Emery, X., Magri, E. J. & Ortiz, J. M. Block size selection and its impact on open-pit design and mine planning. J. South. Afr. Inst. Min. Metall. 106 (3), 205–211 (2006).
Birch, C. Optimisation of mining block size for narrow tabular gold deposits. In Proceedings of the 27th International Symposium on Mine Planning and Equipment Selection-MPES 2018. Springer International Publishing , 121–141 (2019).
Ruiseco, J. R., Williams, J. & Kumral, M. Optimizing ore–waste dig-limits as part of operational mine planning through genetic algorithms. Nat. Resour. Res. 25 , 473–485 (2016).
Leuangthong, O. et al. Optimal selection of selective mining unit size for geostatistical modeling. In International Mining Innovation Conference (MININ) (2004).
Leuangthong, O., Neufeld, C. & Deutsch, C. V. Optimal Selection of Selective Mining Unit (SMU) Size 1–16 (University of Alberta, 2003).
Abdollahei Sharif, J., Jafarpour, A. & Yousefi, S. A hybrid fuzzy MCDM approach to determine an optimal block size in open-pit mine modeling: A case study. J. Min. Environ. 11 (2), 611–627 (2020).
Stevanovic, D. R. et al. Application of MCDA in the determination of optimal block size for open-pit modelling and mine planning. Podzemni radovi 38 , 67–85 (2021).
Samavati, M. et al. A new methodology for the open-pit mine production scheduling problem. Omega 81 , 169–182 (2018).
Paithankar, A. & Chatterjee, S. Open pit mine production schedule optimization using a hybrid of maximum-flow and genetic algorithms. Appl. Soft Comput. 81 , 105507 (2019).
Moreno, E. et al. Linear models for stockpiling in open-pit mine production scheduling problems. Eur. J. Oper. Res. 260 (1), 212–221 (2017).
Rimélé, M. A., Dimitrakopoulos, R. & Gamache, M. A stochastic optimization method with in-pit waste and tailings disposal for open pit life-of-mine production planning. Resour. Policy 57 , 112–121 (2018).
Zhang, Y. Mining and transportation equipment operation probability analysis. J. China Univ. Min. Technol. 1 , 16–21 (1984).
Luo, Z. Surface Mining (Volume 1) (China University of Mining and Technology Press, 1986).
Soltanmohammadi, H. et al. Selection of practical bench height in open pit mining using a multi-criteria decision making solution. J. Geol. Min. Res. 2 (3), 48–59 (2010).
Zhang, Y. et al. Determination of truck-shovel configuration of open-pit mine: A simulation method based on mathematical model. Sustainability 14 (19), 12338 (2022).
Yang, R. Surface Mining (Volume 2) (China University of Mining and Technology Press, 1990).
Liu, G. et al. Research on production capacity planning method of open-pit coal mine. Sci. Rep. 13 (1), 8676 (2023).
Article ADS CAS PubMed PubMed Central Google Scholar
Liu, G. et al. Dynamic optimization of open-pit coal mine production scheduling based on ARIMA and fuzzy structured element. Front. Earth Sci. 10 , 2043 (2023).
Su, W. Open-Pit Coal Mine 35 Million t/a Preliminary Design 124–134 (Shenhua Baorixile Energy Co., Ltd, Inner Mongolia Coal Mine Design & Research Institute Limited Liability Company, 2022).
Tyulenev, M. et al. The model of direct dumping technology implementation for open pit coal mining by high benches. Acta Montanistica Slovaca 23 (4), 368–377 (2018).
Download references
Acknowledgements
This research was supported by the National Natural Science Foundation of China (51974144), the Youth Fund of the National Natural Science Foundation of China (52204158), 'Jie Bang Gua Shuai' (Take the Lead) of the Key Scientific and Technological Project for Liaoning Province (2021JH1/10400011), Discipline Innovation Team of Liaoning Technical University (LNTU20TD-07), and School level scientific research project of Yancheng Institute of Technology (xjr2020039).
Author information
Authors and affiliations.
School of Mining, Liaoning Technical University, Fuxin, 123000, China
Weiqiang Guo, Guangwei Liu & Jiaming Li
School of Economics & Management, Yancheng Institute of Technology, Yancheng, 224051, China
Senlin Chai
State Grid Energy Hami Coal and Electricity Co., Ltd., Dananhu No. 2 Mine, Hami, 839000, China
Shupeng Guo
You can also search for this author in PubMed Google Scholar
Contributions
W.G. wrote the main manuscript text. G.L wrote the original model. All authors reviewed the manuscript.
Corresponding author
Correspondence to Guangwei Liu .
Ethics declarations
Competing interests.
The authors declare no competing interests.
Additional information
Publisher's note.
Springer Nature remains neutral with regard to jurisdictional claims in published maps and institutional affiliations.
Rights and permissions
Open Access This article is licensed under a Creative Commons Attribution 4.0 International License, which permits use, sharing, adaptation, distribution and reproduction in any medium or format, as long as you give appropriate credit to the original author(s) and the source, provide a link to the Creative Commons licence, and indicate if changes were made. The images or other third party material in this article are included in the article's Creative Commons licence, unless indicated otherwise in a credit line to the material. If material is not included in the article's Creative Commons licence and your intended use is not permitted by statutory regulation or exceeds the permitted use, you will need to obtain permission directly from the copyright holder. To view a copy of this licence, visit http://creativecommons.org/licenses/by/4.0/ .
Reprints and permissions
About this article
Cite this article.
Guo, W., Liu, G., Li, J. et al. Research on the method of determining the block size for an open-pit mine integrating mining parameters and shovel-truck’s operation efficiency. Sci Rep 14 , 10119 (2024). https://doi.org/10.1038/s41598-024-52815-9
Download citation
Received : 13 July 2023
Accepted : 24 January 2024
Published : 02 May 2024
DOI : https://doi.org/10.1038/s41598-024-52815-9
Share this article
Anyone you share the following link with will be able to read this content:
Sorry, a shareable link is not currently available for this article.
Provided by the Springer Nature SharedIt content-sharing initiative
By submitting a comment you agree to abide by our Terms and Community Guidelines . If you find something abusive or that does not comply with our terms or guidelines please flag it as inappropriate.
Quick links
- Explore articles by subject
- Guide to authors
- Editorial policies
Sign up for the Nature Briefing newsletter — what matters in science, free to your inbox daily.

Numbers, Facts and Trends Shaping Your World
Read our research on:
Full Topic List
Regions & Countries
- Publications
- Our Methods
- Short Reads
- Tools & Resources
Read Our Research On:
International Surveys
Pew Research Center regularly conducts public opinion surveys in countries outside the United States as part of its ongoing exploration of attitudes, values and behaviors around the globe. To date, the Center has conducted more than 800,000 interviews in over 110 countries, mainly in conjunction with the longstanding Global Attitudes and Religion & Public Life projects but including others such as a 20-country international science study and another on digital connectivity in 11 emerging economies.
Country Specific Methodology
View detailed information such as mode of interview, sampling design, margin of error, and design effect, for each country we survey.
Cross-national studies constitute the bulk of Pew Research Center’s international survey research. Such studies pose special challenges when it comes to ensuring the comparability of data across multiple languages, cultures and contexts. To learn more about the challenges and best practices of polling in foreign countries and in multiple languages, see here.
Pew Research Center staff are responsible for the overall design and execution of each cross-national survey project, including topical focus, questionnaire development, countries to be surveyed and sample design. The Center’s staff frequently contract with a coordinating vendor to identify local, reputable research organizations, which are hired to collaborate on all aspects of sample and questionnaire design, survey administration and data processing. Both coordinating vendors and local research organizations are consulted on matters of sampling, fieldwork logistics, data quality and weighting. In addition, Pew Research Center often seeks the advice of subject matter experts and experienced survey researchers regarding the design and content of its cross-national studies.
Field periods for cross-national studies vary by country, study complexity and mode of administration, typically ranging from four to eight weeks. To the degree possible, Pew Research Center attempts to synchronize fieldwork across countries in order to minimize the chance that major events or developments might interfere with the comparability of results.
Pew Research Center’s cross-national studies are designed to be nationally representative using probability-based methods and target the non-institutional adult population (18 and older) in each country. The Center strives for samples that cover as much of the adult population as possible, given logistical, security and other constraints. Coverage limitations are noted in the detailed methods for each country.
Sample sizes for Pew Research Center’s cross-national studies are usually designed to yield at least 1,000 interviews, though larger samples may be required for more robust within-country comparisons. Reported margins of error account for design effects due to clustering, stratification and weighting.
In the case of both telephone and face-to-face surveys, weighting procedures correct for unequal selection probabilities as well as adjust to key sociodemographic distributions – such as gender, age and education – to align as closely as possible with reliable, official population statistics. To learn about the weighting approach used for U.S. surveys using the nationally representative online American Trends Panel, visit here .
Pew Research Center is a charter member of the American Association of Public Opinion Research (AAPOR) Transparency Initiative .
INTERNATIONAL SURVEYS
Other research methods, sign up for our weekly newsletter.
Fresh data delivered Saturday mornings
1615 L St. NW, Suite 800 Washington, DC 20036 USA (+1) 202-419-4300 | Main (+1) 202-857-8562 | Fax (+1) 202-419-4372 | Media Inquiries
Research Topics
- Age & Generations
- Coronavirus (COVID-19)
- Economy & Work
- Family & Relationships
- Gender & LGBTQ
- Immigration & Migration
- International Affairs
- Internet & Technology
- Methodological Research
- News Habits & Media
- Non-U.S. Governments
- Other Topics
- Politics & Policy
- Race & Ethnicity
- Email Newsletters
ABOUT PEW RESEARCH CENTER Pew Research Center is a nonpartisan fact tank that informs the public about the issues, attitudes and trends shaping the world. It conducts public opinion polling, demographic research, media content analysis and other empirical social science research. Pew Research Center does not take policy positions. It is a subsidiary of The Pew Charitable Trusts .
Copyright 2024 Pew Research Center
Terms & Conditions
Privacy Policy
Cookie Settings
Reprints, Permissions & Use Policy

Study identifies method to reduce extended-spectrum antibiotic use for UTI
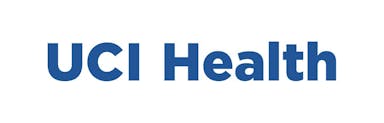
In total, the study included 127,403 noncritically ill adult patients admitted to the hospital with UTI.
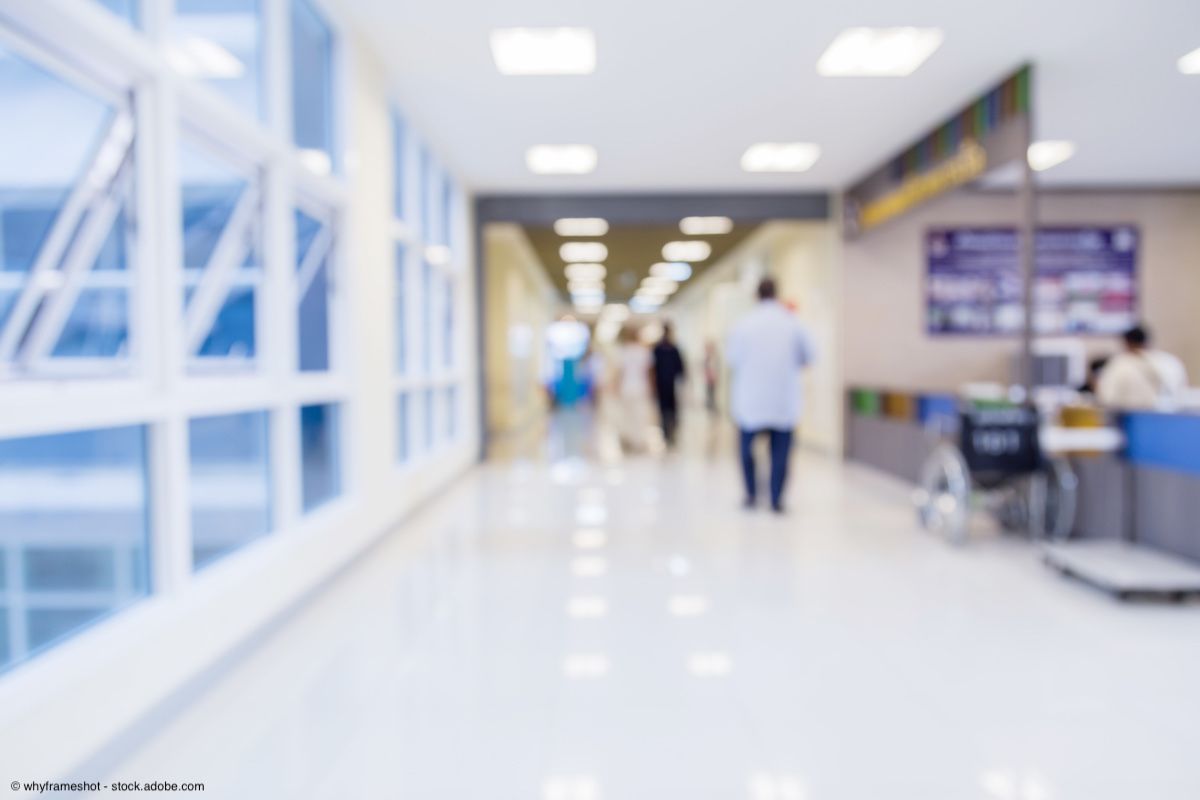
The method involves the use of a computerized provider order entry (CPOE) that provides real-time recommendations to prescribing physicians on the best antibiotic match for each patient. With the program, patients’ risk for an antibiotic-resistant infection is determined using information on patient characteristics from electronic medical records as well as hospital and location-specific data. For the study, physicians treating patients with a low estimated risk (less than 10%) of MDRO UTI were prompted to give standard-spectrum antibiotics.
Concurrently, the INSPIRE Pneumonia Trial was published in JAMA demonstrating the same approach in patients admitted with pneumonia. 2
“The right information at the right time can improve physician antibiotic selection. Many different bacteria can cause pneumonia or UTI, and picking the best matched antibiotic can be a challenge. Results from these trials show that giving physicians an alert informing them of their patient’s actual risk for antibiotic resistance can help them choose the best antibiotic and reduce extended-spectrum antibiotic use,” said Shruti Gohil, MD, MPH, in a news release on the findings. 3 Gohil is the lead author for both INSPIRE trials, as well as an assistant professor in the Division of Infectious Diseases at the University of California, Irvine School of Medicine.
Overall, 59 US-based hospitals participated in the INSPIRE UTI trial, of which 29 were cluster randomized to the CPOE stewardship bundle (education, feedback, and real-time and risk-based CPOE prompts) and 30 were cluster randomized to continued routine antibiotic stewardship strategies in accordance with national standards.
Data showed that the CPOE alerts led to 17.4% significantly lower rate of empiric extended-spectrum days of therapy (rate ratio, 0.83; 95% CI, 0.77-0.89; P < .001) in the CPOE bundle group compared with the routine stewardship group. Specifically, the empiric extended-spectrum days of therapy per 100 days in the routine stewardship group was 431.1 during the baseline period and 446.0 during the intervention period. For the CPOE bundle group, the empiric extended-spectrum days of therapy was 392.2 during the baseline period and 326.0 during the intervention period.
Regarding safety, 4.0% of patients in the routine group and 3.7% of patients in the CPOE bundle group were transferred to the ICU. Further, 10.2% and 10.0% of patients in the routine and CPOE bundle groups, respectively, required antibiotic escalation.
There were no significant differences noted between the intervention and routine groups regarding mean days to ICU transfer (7.0 vs 6.6 days, respectively) or hospital length of stay (6.5 vs 6.3 days, respectively).
In total, the study included 127,403 noncritically ill adult patients admitted to the hospital with UTI, of whom 71,991 participated in the 18-month baseline period and 55,412 participated in the 15-month intervention period. Among all patients, 30.5% were male. The mean age of participants was 69 (SD, 17.9) years.
The primary outcome measure for the study was the extended-spectrum days of therapy in the first 3 days of hospitalization (empiric period). Safety outcome measures included days to antibiotic escalation, days to ICU transfer, and hospital length of stay in days.
Co-author Sujan Reddy, MD, Medical Officer in the Epidemiology, Research and Innovations Branch of CDC’s Division of Healthcare Quality Promotion, concluded in the news release, 3 “Pneumonia and urinary tract infections are two of the most common infections requiring hospitalization and a major reason for overuse of broad-spectrum antibiotics. The INSPIRE trials have found a highly effective way to help physicians follow treatment recommendations to optimize antibiotic selection for each patient. These trials show the value of harnessing electronic health data to improve best practice.”
1. Gohil SK, Septimus E, Kleinman K, et al. Stewardship Prompts to Improve Antibiotic Selection for Urinary Tract Infection: The INSPIRE Randomized Clinical Trial. JAMA . 2024. doi:10.1001/jama.2024.6259
2. Gohil SK, Septimus E, Kleinman K, et al. Stewardship Prompts to Improve Antibiotic Selection for Pneumonia: The INSPIRE Randomized Clinical Trial. JAMA . 2024. doi:10.1001/jama.2024.6248
3. Two clinical trials identify a better way to target appropriate antibiotics for patients hospitalized with pneumonia or urinary tract infection. News release. April 19, 2024. Accessed May 1, 2024. https://www.businesswire.com/news/home/20240419656742/en/Two-Clinical-Trials-Identify-a-Better-Way-to-Target-Appropriate-Antibiotics-for-Patients-Hospitalized-With-Pneumonia-or-Urinary-Tract-Infection
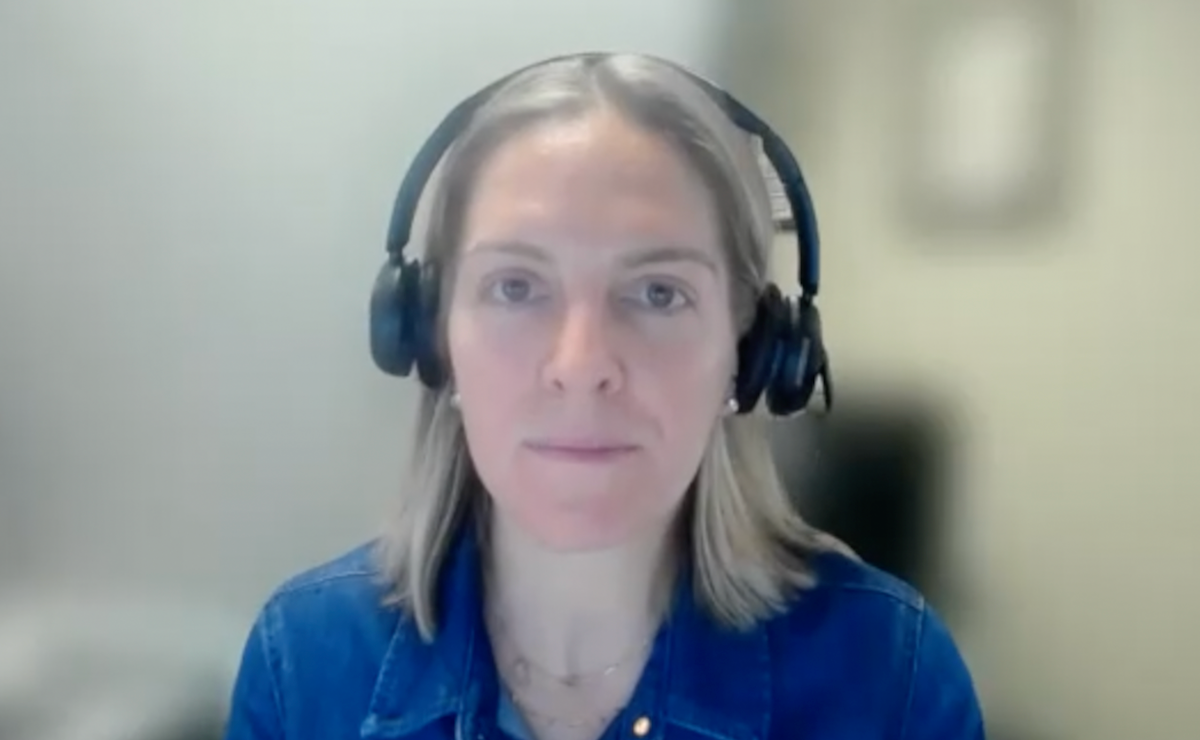
FDA approves pivmecillinam tablets for uncomplicated UTIs
The approval is based on 3 clinical trials evaluating the efficacy of different pivmecillinam dosing regimens in comparison with either placebo, another oral antibacterial drug, or ibuprofen.
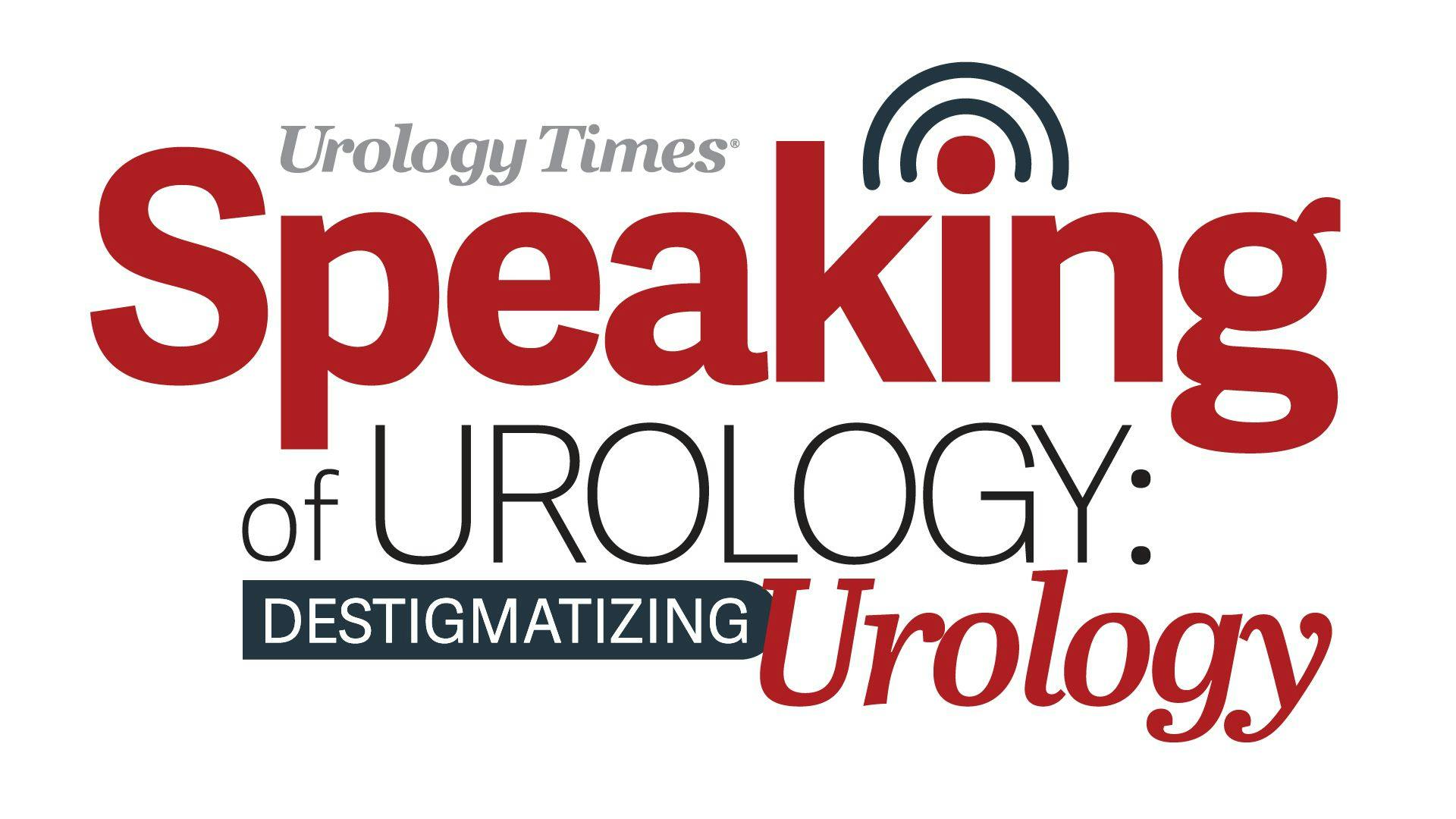
Destigmatizing Urology: Dr. Winter discusses STDs/STIs
“We need to just approach STIs as the medical conditions they are because until we do, and until we take those stigmas away, people will truly have resistance to discussing it, hesitance to discussing it, and resistance to diagnosis and intervention,” says Ashley Winter, MD.
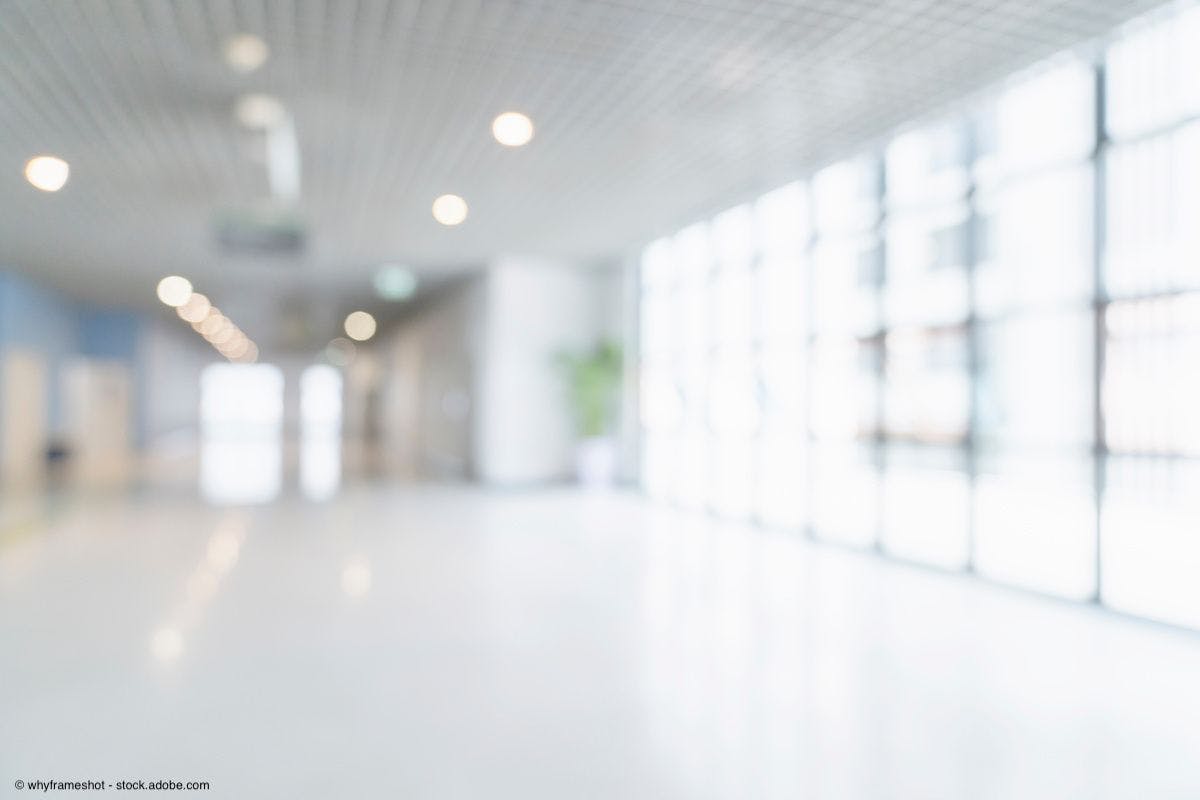
The dilemma of using disposable scopes
"I am still struggling with disposing the scopes after each use, both from the environmental aspect and the fact that the scope technically can be used more than once," writes Gopal H. Badlani, MD.
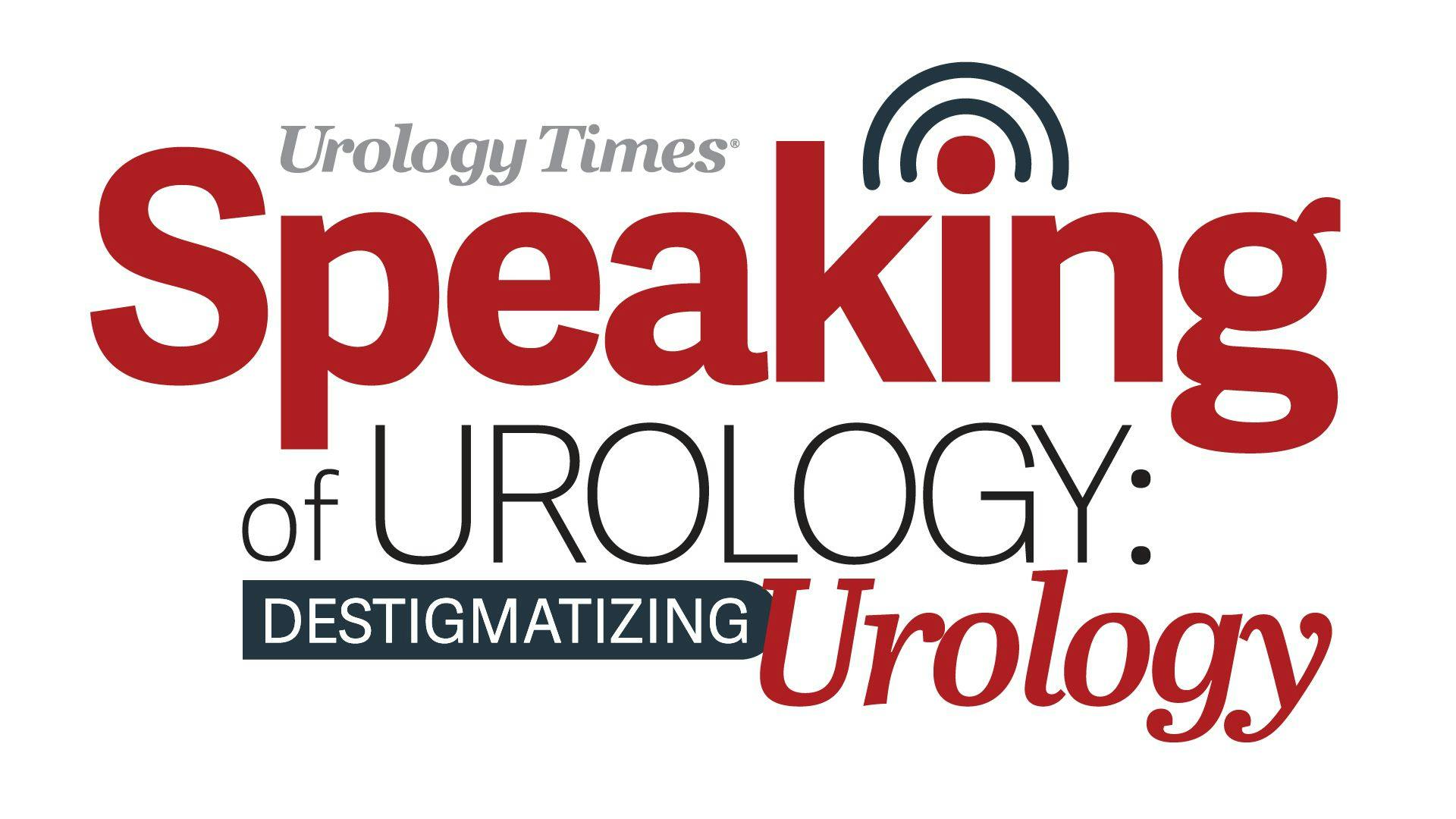
Destigmatizing Urology: Dr. Clemens discusses interstitial cystitis
“I think people can feel like they're all alone, or they have something that's uncommon, and it may not be,” says J. Quentin Clemens, MD, FACS, MSCI.
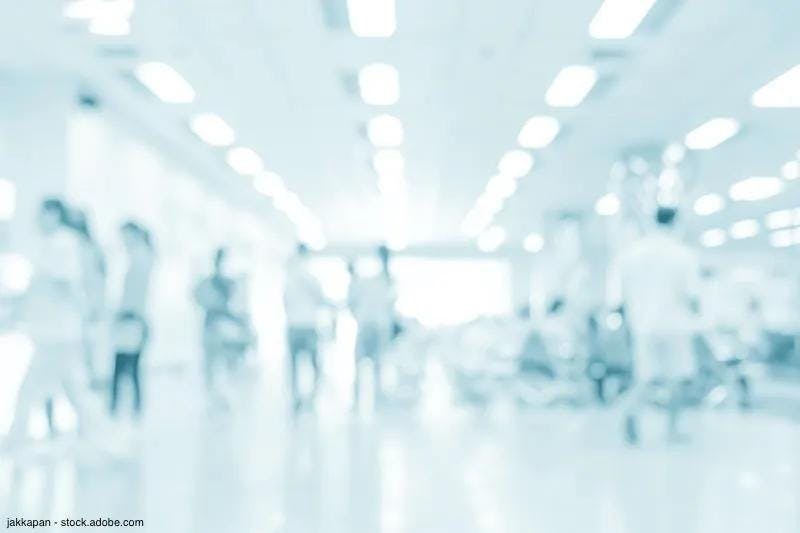
AUGS Clinical Consensus Statement on Postoperative Urinary Retention
Postoperative urinary retention is a common outcome after surgical procedures for pelvic organ prolapse and stress urinary incontinence.
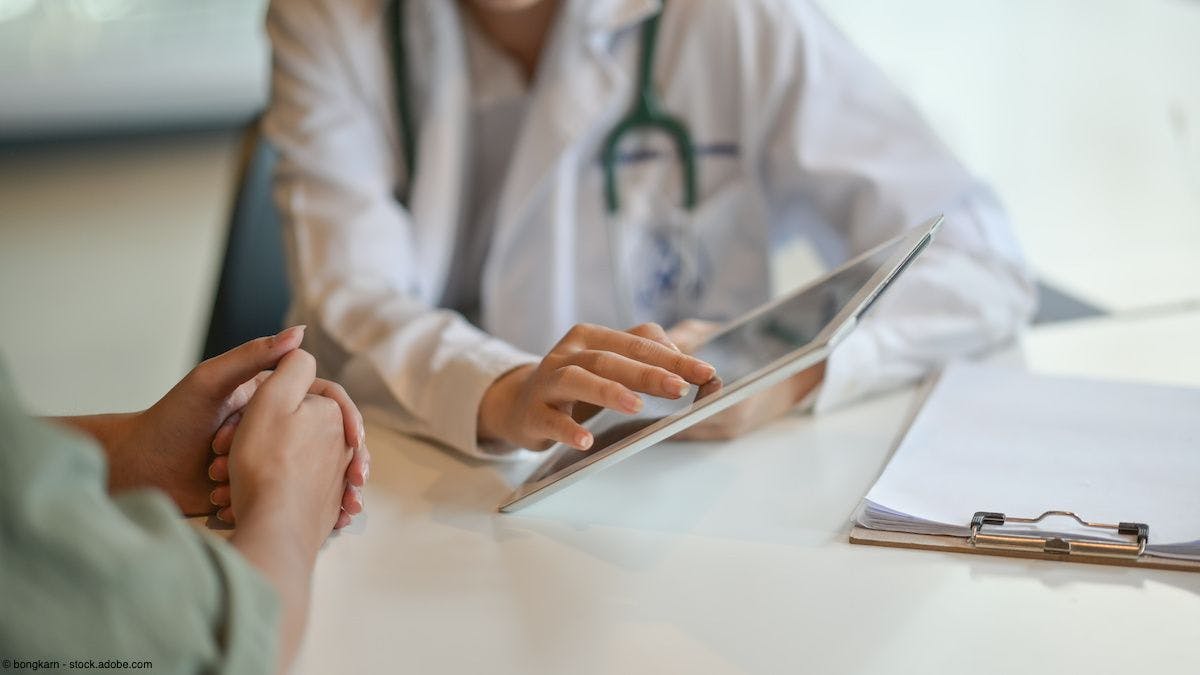
Trial to investigate efficacy of aloe vera in patients with interstitial cystitis
"We have over 30 years of safety data, and no adverse events in over 30 years," says Heather Florio.
2 Commerce Drive Cranbury, NJ 08512
609-716-7777

Remaining Useful Life Prediction Method for Multi-Component System Considering Maintenance: Subsea Christmas Tree System as A Case Study
- Published: 02 May 2024
- Volume 38 , pages 198–209, ( 2024 )
Cite this article
- Qi-bing Wu 1 ,
- Bao-ping Cai 2 ,
- Hong-yan Fan 2 ,
- Guan-nan Wang 1 ,
- Weifeng Ge 3 ,
- Xiao-yan Shao 2 &
- Yong-hong Liu 2
Maintenance is an important technical measure to maintain and restore the performance status of equipment and ensure the safety of the production process in industrial production, and is an indispensable part of prediction and health management. However, most of the existing remaining useful life (RUL) prediction methods assume that there is no maintenance or only perfect maintenance during the whole life cycle; thus, the predicted RUL value of the system is obviously lower than its actual operating value. The complex environment of the system further increases the difficulty of maintenance, and its maintenance nodes and maintenance degree are limited by the construction period and working conditions, which increases the difficulty of RUL prediction. An RUL prediction method for a multi-omponent system based on the Wiener process considering maintenance is proposed. The performance degradation model of components is established by a dynamic Bayesian network as the initial model, which solves the uncertainty of insufficient data problems. Based on the experience of experts, the degree of degradation is divided according to Poisson process simulation random failure, and different maintenance strategies are used to estimate a variety of condition maintenance factors. An example of a subsea tree system is given to verify the effectiveness of the proposed method.
This is a preview of subscription content, log in via an institution to check access.
Access this article
Price excludes VAT (USA) Tax calculation will be finalised during checkout.
Instant access to the full article PDF.
Rent this article via DeepDyve
Institutional subscriptions
Cai, B.P., Fan, H.Y., Shao, X.Y., Liu, Y.H., Liu, G.J., Liu, Z.K. and Ji, R.J., 2021. Remaining useful life re-prediction methodology based on Wiener process: Subsea Christmas tree system as a case study, Computers & Industrial Engineering , 151, 106983.
Article Google Scholar
Cai, B.P., Shao, X.Y., Liu, Y.H., Kong, X.D., Wang, H.F., Xu, H.Q. and Ge, W.F., 2020. Remaining useful life estimation of structure systems under the influence of multiple causes: Subsea pipelines as a case study, IEEE Transactions on Industrial Electronics , 67(7), 5737–5747.
Cai, B.P., Sheng, C.Y., Gao, C.T., Liu, Y.H., Shi, M.W., Liu, Z.K., Feng, Q. and Liu, G.J., 2023. Artificial intelligence enhanced reliability assessment methodology with small samples, IEEE Transactions on Neural Networks and Learning Systems , 34(9), 6578–6590.
Cai, B.P., Wang, Z.D., Zhu, H.M., Liu, Y.H., Hao, K.K., Yang, Z.Q., Ren, Y., Feng, Q. and Liu, Z.K., 2022. Artificial intelligence enhanced two-stage hybrid fault prognosis methodology of PMSM, IEEE Transactions on Industrial Informatics , 18(10), 7262–7273.
Chen, Y.X., Wang, Z.Z. and Cai, Z.Y., 2020. Optimal maintenance decision based on remaining useful lifetime prediction for the equipment subject to imperfect maintenance, IEEE Access , 8, 6704–6716.
Gou, B., Xu, Y. and Feng, X., 2020. State-of-health estimation and remaining-useful-life prediction for lithium-ion battery using a hybrid data-driven method, IEEE Transactions on Vehicular Technology , 69(10), 10854–10867.
Guida, M. and Pulcini, G., 2009. Reliability analysis of mechanical systems with bounded and bathtub shaped intensity function, IEEE Transactions on Reliability , 58(3), 432–443.
Gupta, N. and Kumar, A., 2023. Fractional Poisson processes of order k and beyond, Journal of Theoretical Probability , 36, 2165–2191.
Article MathSciNet Google Scholar
Han, X., Wang, Z.L., Xie, M., He, Y.H., Li, Y. and Wang, W.Z., 2021. Remaining useful life prediction and predictive maintenance strategies for multi-state manufacturing systems considering functional dependence, Reliability Engineering & System Safety , 210, 107560.
Hanachi, H., Yu, W.N., Kim, I.Y., Liu, J. and Mechefske, C.K., 2018. Hybrid data-driven physics-based model fusion framework for tool wear prediction, The International Journal of Advanced Manufacturing Technology , 101(9–12), 2861–2872.
Google Scholar
Hesabi, H., Nourelfath, M. and Hajji, A., 2022. A deep learning predictive model for selective maintenance optimization, Reliability Engineering & System Safety , 219, 108191.
Hu, C.H., Pei, H., Si, X.S., Du, D.B., Pang, Z.N. and Wang, X., 2020. A prognostic model based on DBN and diffusion process for degrading bearing, IEEE Transactions on Industrial Electronics , 67(10), 8767–8777.
Hu, Y., Miao, X.W., Si, Y., Pan, E.S. and Zio, E., 2022. Prognostics and health management: A review from the perspectives of design, development and decision, Reliability Engineering & System Safety , 217, 108063.
Kumar, A., Chinnam, R.B. and Tseng, F., 2019. An HMM and polynomial regression based approach for remaining useful life and health state estimation of cutting tools, Computers & Industrial Engineering , 128, 1008–1014.
Lee, J., Wu, F.J., Zhao, W.Y., Ghaffari, M., Liao, L.X. and Siegel, D., 2014. Prognostics and health management design for rotary machinery systems—Reviews, methodology and applications, Mechanical Systems and Signal Processing , 42(1–2), 314–334.
Lei, Y.G., Li, N.P., Guo, L., Li, N.B., Yan, T. and Lin, J., 2018. Machinery health prognostics: A systematic review from data acquisition to RUL prediction, Mechanical Systems and Signal Processing , 104, 799–834.
Li, H., Deng, Z.M., Golilarz, N.A. and Guedes Soares, C., 2021. Reliability analysis of the main drive system of a CNC machine tool including early failures, Reliability Engineering & System Safety , 215, 107846.
Li, N.P., Lei, Y.G., Yan, T., Li, N.B. and Han, T.Y., 2019. A Wiener-process-model-based method for remaining useful life prediction considering unit-to-unit variability, IEEE Transactions on Industrial Electronics , 66(3), 2092–2101.
Li, T.F., Zhao, Z.B., Sun, C., Cheng, L., Chen, X.F., Yan, R.Q. and Gao, R.X., 2022. WaveletKernelNet: An interpretable deep neural network for industrial intelligent diagnosis, IEEE Transactions on Systems, Man, and Cybernetics : Systems , 52(4), 2302–2312.
Li, W.H., Zhang, S.H. and Rakheja, S., 2016. Feature denoising and nearest-farthest distance preserving projection for machine fault diagnosis, IEEE Transactions on Industrial Informatics , 12(1), 393–404.
Li, X., Zhang, W., Ma, H., Luo, Z. and Li, X., 2020. Data alignments in machinery remaining useful life prediction using deep adversarial neural networks, Knowledge-Based Systems , 197, 105843.
Liao, L.X. and Kottig, F., 2014. Review of hybrid prognostics approaches for remaining useful life prediction of engineered systems, and an application to battery life prediction, IEEE Transactions on Reliability , 63(1), 191–207.
Liu, Q.N., Ma, L., Wang, N.C., Chen, A.K. and Jiang, Q.H., 2022. A condition-based maintenance model considering multiple maintenance effects on the dependent failure processes, Reliability Engineering & System Safety , 220, 108267.
Liu, X.L., Cai, B.P., Yuan, X.B., Shao, X.Y., Liu, Y.L., Khan, J.A., Fan, H.Y., Liu, Y.H., Liu, Z.K. and Liu, G.J., 2023. A hybrid multistage methodology for remaining useful life prediction of control system: Subsea Christmas tree as a case study, Expert Systems with Applications , 215, 119335.
Mosayebi Omshi, E. and Grall, A., 2021. Replacement and imperfect repair of deteriorating system: Study of a CBM policy and impact of repair efficiency, Reliability Engineering & System Safety , 215, 107905.
Peng, W.W., Ye, Z.S. and Chen, N., 2019. Joint online RUL prediction for multivariate deteriorating systems, IEEE Transactions on Industrial Informatics , 15(5), 2870–2878.
Shahraki, A.F., Yadav, O.P. and Vogiatzis, C., 2020. Selective maintenance optimization for multi-state systems considering stochastically dependent components and stochastic imperfect maintenance actions, Reliability Engineering & System Safety , 196, 106738.
Tamssaouet, F., Nguyen, K.T.P. and Medjaher, K., 2021. System-level prognostics under mission profile effects using inoperability input-output model, IEEE Transactions on Systems, Man, and Cybernetics : Systems , 51(8), 4659–4669.
Wang, X.L., Zhou, H., Parlikad, A.K. and Xie, M., 2020. Imperfect preventive maintenance policies with unpunctual execution, IEEE Transactions on Reliability , 69(4), 1480–1492.
Wu, J., Su, Y.H., Cheng, Y.W., Shao, X.Y., Deng, C. and Liu, C., 2018. Multi-sensor information fusion for remaining useful life prediction of machining tools by adaptive network based fuzzy inference system, Applied Soft Computing , 68, 13–23.
Zhai, Q.Q. and Ye, Z.S., 2017. RUL prediction of deteriorating products using an adaptive wiener process model, IEEE Transactions on Industrial Informatics , 13(6), 2911–2921.
Zhu, X.Y., Chen, Z.Q. and Borgonovo, E., 2021. Remaining-useful-lifetime and system-remaining-profit based importance measures for decisions on preventive maintenance, Reliability Engineering & System Safety , 216, 107951.
Download references
Author information
Authors and affiliations.
CNOOC Safety Technology Services Co., Ltd., Tianjin, 300456, China
Qi-bing Wu, Guan-nan Wang & Xi Rao
College of Mechanical and Electronic Engineering, China University of Petroleum, Qingdao, 266580, China
Bao-ping Cai, Hong-yan Fan, Xiao-yan Shao & Yong-hong Liu
Safety & Environmental Protection Branch, CNOOC EnerTech, Tianjin, 300456, China
You can also search for this author in PubMed Google Scholar
Corresponding author
Correspondence to Bao-ping Cai .
Ethics declarations
The authors declare no competing interests.
Additional information
Foundation item: This work was financially supported by the National Key Research and Development Program of China (Grant No. 2022YFC3004802), the National Natural Science Foundation of China (Grant Nos. 52171287 and 52325107), High Tech Ship Research Project of Ministry of Industry and Information Technology (Grant Nos. 2023GXB01-05-004-03 and GXBZH2022-293), the Science Foundation for Distinguished Young Scholars of Shandong Province (Grant No. ZR2022JQ25), the Taishan Scholars Project (Grant No. tsqn201909063), and the sub project of the major special project of CNOOC Development Technology, “Research on the Integrated Technology of Intrinsic Safety of Offshore Oil Facilities” (Phase I), “Research on Dynamic Quantitative Analysis and Control Technology of Risks in Offshore Production Equipment” (Grant No. HFKJ-2D2X-AQ-2021-03).
Rights and permissions
Reprints and permissions
About this article
Wu, Qb., Cai, Bp., Fan, Hy. et al. Remaining Useful Life Prediction Method for Multi-Component System Considering Maintenance: Subsea Christmas Tree System as A Case Study. China Ocean Eng 38 , 198–209 (2024). https://doi.org/10.1007/s13344-024-0017-y
Download citation
Received : 05 May 2023
Revised : 18 July 2023
Accepted : 10 August 2023
Published : 02 May 2024
Issue Date : April 2024
DOI : https://doi.org/10.1007/s13344-024-0017-y
Share this article
Anyone you share the following link with will be able to read this content:
Sorry, a shareable link is not currently available for this article.
Provided by the Springer Nature SharedIt content-sharing initiative
- remaining useful life
- Wiener process
- dynamic Bayesian networks
- maintenance
- subsea Christmas tree system
Advertisement
- Find a journal
- Publish with us
- Track your research
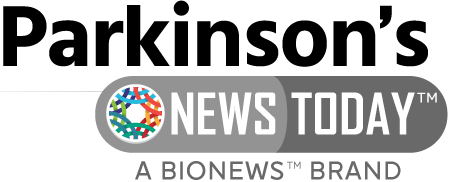
Machine learning approaches can benefit Parkinson’s research: Study
1 method found to help ID 'more potent' treatment candidates in lab

by Patricia Inácio, PhD | April 29, 2024
Share this article:
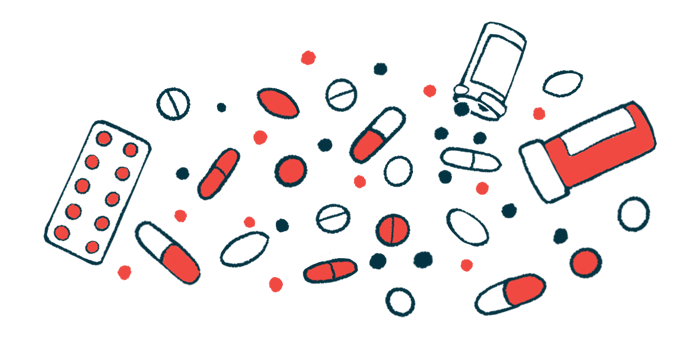
Scientists exploring the potential of machine learning approaches in drug discovery for Parkinson’s disease and other neurodegenerative disorders — focusing on misfolded proteins that are the hallmark of such conditions — found that one such method identified compounds “two orders of magnitude more potent” than ones previously reported, per a new study.
Using this method allowed the researchers, from the U.K. and the U.S., to identify compounds that can effectively block the clumping, or aggregation, of alpha-synuclein protein, an underlying cause of Parkinson’s, the study reported.
“We anticipate that using machine learning approaches of the type described here could be of considerable benefit to researchers working in the field of protein misfolding diseases [such as Parkinson’s], and indeed early-stage drug discovery research in general,” the researchers wrote.
Their study, “ Discovery of potent inhibitors of α-synuclein aggregation using structure-based iterative learning ” was published in the journal Nature Chemical Biology.
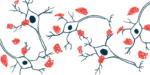
Simulations uncover mechanisms in alpha-synuclein protein buildup
Machine learning approaches ‘speeding up the whole process’ of drug discovery.
Parkinson’s disease is marked by the toxic accumulation of misfolded forms of the alpha-synuclein protein within dopamine-producing nerve cells — those responsible for releasing the neurotransmitter dopamine. Dopamine is a signaling molecule that plays a role in controlling movement; Parkinson’s results from the progressive loss of these cells.
Despite efforts to identify compounds that stop this toxic accumulation, there are, to date, no disease-modifying treatments available for Parkinson’s.
Traditional strategies to identify novel therapies — which involve screening large chemical libraries looking for potential candidates prior to any testing in humans — are time-consuming, expensive, and often unsuccessful.
In the case of Parkinson’s, the development of effective therapies has been hampered by the lack of methods to identify the right molecular targets.
“One route to search for potential treatments for Parkinson’s requires the identification of small molecules that can inhibit the aggregation of alpha-synuclein. … But this is an extremely time-consuming process — just identifying a lead candidate for further testing can take months or even years,” Michele Vendruscolo, a professor at the University of Cambridge and the study’s lead author, said in a university press release .
Now, the researchers developed a method that was able to use machine learning to quickly screen chemical libraries containing literally millions of compounds. The goal was to identify small molecules able to block the clumping of alpha-synuclein.
From a list of small molecules predicted to have a good binding to the alpha-synuclein aggregates, the researchers chose a small number of the top-ranking compounds to test experimentally as potent inhibitors of aggregation.
The results from these experimental assays were then fed to the machine learning model, which identified those with the most promising effects. This process was repeated a few times, so that highly potent compounds were identified.
“Instead of screening experimentally, we screen computationally,” Vendruscolo said.
Machine learning is having a real impact on the drug discovery process — it’s speeding up the whole process of identifying the most promising candidates. For us this means we can start work on multiple drug discovery programs — instead of just one.
“By using the knowledge we gained from the initial screening with our machine learning model, we were able to train the model to identify the specific regions on these small molecules responsible for binding, then we can re-screen and find more potent molecules,” Vendruscolo said.
Using this method, the researchers optimized the initial compounds to target pockets on the surfaces of the alpha-synuclein clumps.
In lab tests using brain tissue samples from patients with Lewy body dementia (LBD) and multiple system atrophy (MSA), two forms of atypical parkinsonism , the compounds effectively blocked aggregation of alpha-synuclein.
“Machine learning is having a real impact on the drug discovery process — it’s speeding up the whole process of identifying the most promising candidates,” Vendruscolo said. “For us this means we can start work on multiple drug discovery programs — instead of just one.”
According to Vendruscolo, “so much is possible due to the massive reduction in both time and cost – it’s an exciting time.”
About the Author

Recent Posts
- Method generates dopaminergic neurons lost in Parkinson’s disease
- Parkinson’s disease self-care tips include tracking, support: Study
- Researchers float ‘brain-first, body-first’ model as Parkinson’s origin
- Parkinson’s may change the way emotions manifest in body: Study
- My husband’s Parkinson’s diagnosis prompts ever-evolving feelings
Recommended reading
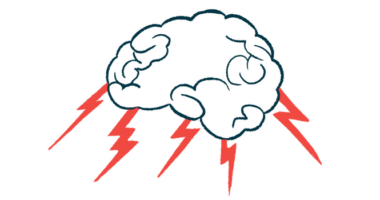
Machine learning tool may help detect Parkinson’s disease: Study
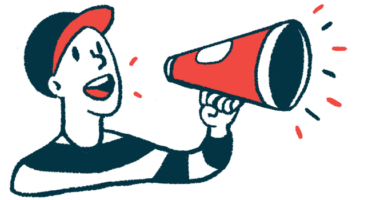
Machine learning aids distinguishing Parkinson’s, essential tremor voice
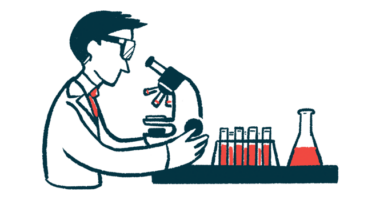
AI analysis helps identify molecular subtypes of Parkinson’s
Subscribe to our newsletter.
Get regular updates to your inbox.
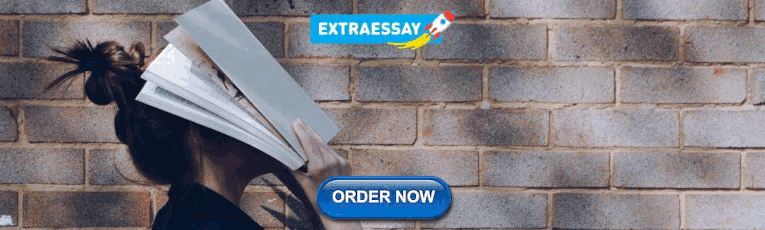
IMAGES
VIDEO
COMMENTS
By Janet Salmons, PhD Manager, Sage Research Methods Community. What is Case Study Methodology?. Case studies in research are both unique and uniquely confusing. The term case study is confusing because the same term is used multiple ways. The term can refer to the methodology, that is, a system of frameworks used to design a study, or the methods used to conduct it.
A case study is a detailed study of a specific subject, such as a person, group, place, event, organization, or phenomenon. Case studies are commonly used in social, educational, clinical, and business research. A case study research design usually involves qualitative methods, but quantitative methods are sometimes also used.
Defnition: A case study is a research method that involves an in-depth examination and analysis of a particular phenomenon or case, such as an individual, organization, community, event, or situation. It is a qualitative research approach that aims to provide a detailed and comprehensive understanding of the case being studied.
Definitions of qualitative case study research. Case study research is an investigation and analysis of a single or collective case, intended to capture the complexity of the object of study (Stake, 1995).Qualitative case study research, as described by Stake (), draws together "naturalistic, holistic, ethnographic, phenomenological, and biographic research methods" in a bricoleur design ...
A case study protocol outlines the procedures and general rules to be followed during the case study. This includes the data collection methods to be used, the sources of data, and the procedures for analysis. Having a detailed case study protocol ensures consistency and reliability in the study.
A case study is a detailed study of a specific subject, such as a person, group, place, event, organisation, or phenomenon. Case studies are commonly used in social, educational, clinical, and business research. A case study research design usually involves qualitative methods, but quantitative methods are sometimes also used.
A case study is a research approach that is used to generate an in-depth, multi-faceted understanding of a complex issue in its real-life context. It is an established research design that is used extensively in a wide variety of disciplines, particularly in the social sciences. A case study can be defined in a variety of ways (Table 5 ), the ...
Case Study Methodology: Basic Definitions and Concepts ... In a case study research, multiple methods of data collection are used, as it involves an in-depth study of a phenomenon. It must be noted, as highlighted by Yin (2009), a case study is not a method of data collection, rather is a research strategy or design to study a social unit.
The case study is a qualitative methodology that supports research on studying complex phenomena within their contexts (Baxter and Jack, 2008). The case study strategy was selected as contextual ...
Qualitative case study methodology provides tools for researchers to study complex phenomena within their contexts. When the approach is applied correctly, it becomes a valuable method for health science ... treatment of case study methods we encourage you to read Hancock and Algozzine's, Doing case study research: A practical guide for ...
What the Case Study Method Really Teaches. Summary. It's been 100 years since Harvard Business School began using the case study method. Beyond teaching specific subject matter, the case study ...
Using multiple data collection methods is a key characteristic of all case study methodology; it enhances the credibility of the findings by allowing different facets and views of the phenomenon to be explored. 23 Common methods include interviews, focus groups, observation, and document analysis. 5,37 By seeking patterns within and across data ...
Case studies are in-depth investigations of a person, group, event, or community. Typically, data is gathered from various sources using several methods (e.g., observations & interviews). The case study research method originated in clinical medicine (the case history, i.e., the patient's personal history). In psychology, case studies are ...
Overview. Simply put, the case method is a discussion of real-life situations that business executives have faced. On average, you'll attend three to four different classes a day, for a total of about six hours of class time (schedules vary). To prepare, you'll work through problems with your peers. Read More.
Qualitative analysis methods. Qualitative analysis is used to understand words, ideas, and experiences. You can use it to interpret data that was collected: From open-ended surveys and interviews, literature reviews, case studies, ethnographies, and other sources that use text rather than numbers. Using non-probability sampling methods.
Wave definitions for the current expanded case study-mixed methods Study of the COVID-19 pandemic in Alberta, Canada based on case count data. Note. The Y-axis represents case counts and the X-axis the date. Case count data from the Government of Alberta via its interactive COVID-19 data app (Government of Alberta, 2021).
Perceptual consequences of online group speech treatment for individuals with Parkinson's disease: A pilot study case series. ... Method . Seven speakers with hypokinetic dysarthria associated with PD participated in a university and community-based online group speech program for 10 weeks. Speech recordings occurred remotely 1 week before ...
This study utilizes the Baorixile open-pit mine as a case study (as shown in Fig. 6). The Chenbaerhuqi coal field in Hulunbuir City, Inner Mongolia Autonomous Region of China, is where the ...
Daily flow discharge prediction using integrated methodology based on LSTM models: Case study in Brahmani-Baitarani basin. Author links open overlay panel Abinash Sahoo a, Swayamshu Satyapragnya Parida a, ... The hybrid ConvLSTM technique, a mix of deep learning methods, is used in this study's objective model. ConvLSTM is a hybrid form of the ...
Pew Research Center's cross-national studies are designed to be nationally representative using probability-based methods and target the non-institutional adult population (18 and older) in each country. The Center strives for samples that cover as much of the adult population as possible, given logistical, security and other constraints.
The method involves the use of a computerized provider order entry (CPOE) that provides real-time recommendations to prescribing physicians on the best antibiotic match for each patient. With the program, patients' risk for an antibiotic-resistant infection is determined using information on patient characteristics from electronic medical ...
Maintenance is an important technical measure to maintain and restore the performance status of equipment and ensure the safety of the production process in industrial production, and is an indispensable part of prediction and health management. However, most of the existing remaining useful life (RUL) prediction methods assume that there is no maintenance or only perfect maintenance during ...
Scientists exploring the potential of machine learning approaches in drug discovery for Parkinson's disease and other neurodegenerative disorders — focusing on misfolded proteins that are the hallmark of such conditions — found that one such method identified compounds "two orders of magnitude more potent" than ones previously reported, per a new study.
In order to further differentiate case study method from casework, case method, and case history (case records), she stresses its unique distinctive attributes: particularistic (it focuses on a particular situation, event, program, or phenomenon); descriptive (it yields a rich, thick description of the phenomenon under study); heuristic (it ...