- Data Science
- Data Analysis
- Data Visualization
- Machine Learning
- Deep Learning
- Computer Vision
- Artificial Intelligence
- AI ML DS Interview Series
- AI ML DS Projects series
- Data Engineering
- Web Scrapping
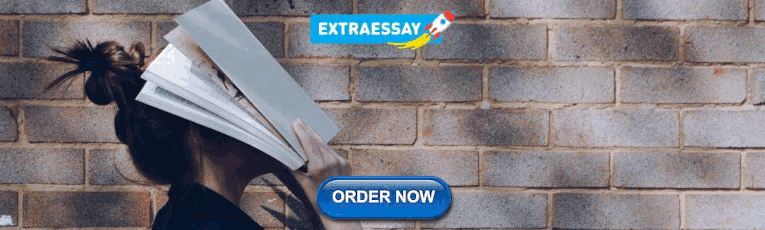
Problem Solving in Artificial Intelligence
- Game Playing in Artificial Intelligence
- Types of Reasoning in Artificial Intelligence
- Artificial Intelligence - Terminology
- Artificial Intelligence(AI) Replacing Human Jobs
- Constraint Satisfaction Problems (CSP) in Artificial Intelligence
- What Are The Ethical Problems in Artificial Intelligence?
- Artificial Intelligence | An Introduction
- Artificial Intelligence - Boon or Bane
- What is Artificial Intelligence?
- Artificial Intelligence in Financial Market
- Artificial Intelligence Tutorial | AI Tutorial
- Top 15 Artificial Intelligence(AI) Tools List
- What is Artificial Narrow Intelligence (ANI)?
- Artificial Intelligence Permeation and Application
- Dangers of Artificial Intelligence
- What is the Role of Planning in Artificial Intelligence?
- Artificial Intelligence (AI) Researcher Jobs in China
- Artificial Intelligence vs Cognitive Computing
- 5 Mistakes to Avoid While Learning Artificial Intelligence
The reflex agent of AI directly maps states into action. Whenever these agents fail to operate in an environment where the state of mapping is too large and not easily performed by the agent, then the stated problem dissolves and sent to a problem-solving domain which breaks the large stored problem into the smaller storage area and resolves one by one. The final integrated action will be the desired outcomes.
On the basis of the problem and their working domain, different types of problem-solving agent defined and use at an atomic level without any internal state visible with a problem-solving algorithm. The problem-solving agent performs precisely by defining problems and several solutions. So we can say that problem solving is a part of artificial intelligence that encompasses a number of techniques such as a tree, B-tree, heuristic algorithms to solve a problem.
We can also say that a problem-solving agent is a result-driven agent and always focuses on satisfying the goals.
There are basically three types of problem in artificial intelligence:
1. Ignorable: In which solution steps can be ignored.
2. Recoverable: In which solution steps can be undone.
3. Irrecoverable: Solution steps cannot be undo.
Steps problem-solving in AI: The problem of AI is directly associated with the nature of humans and their activities. So we need a number of finite steps to solve a problem which makes human easy works.
These are the following steps which require to solve a problem :
- Problem definition: Detailed specification of inputs and acceptable system solutions.
- Problem analysis: Analyse the problem thoroughly.
- Knowledge Representation: collect detailed information about the problem and define all possible techniques.
- Problem-solving: Selection of best techniques.
Components to formulate the associated problem:
- Initial State: This state requires an initial state for the problem which starts the AI agent towards a specified goal. In this state new methods also initialize problem domain solving by a specific class.
- Action: This stage of problem formulation works with function with a specific class taken from the initial state and all possible actions done in this stage.
- Transition: This stage of problem formulation integrates the actual action done by the previous action stage and collects the final stage to forward it to their next stage.
- Goal test: This stage determines that the specified goal achieved by the integrated transition model or not, whenever the goal achieves stop the action and forward into the next stage to determines the cost to achieve the goal.
- Path costing: This component of problem-solving numerical assigned what will be the cost to achieve the goal. It requires all hardware software and human working cost.
Please Login to comment...
Similar reads.
Improve your Coding Skills with Practice
What kind of Experience do you want to share?
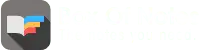
Problem Solving Agents in Artificial Intelligence
In this post, we will talk about Problem Solving agents in Artificial Intelligence, which are sort of goal-based agents. Because the straight mapping from states to actions of a basic reflex agent is too vast to retain for a complex environment, we utilize goal-based agents that may consider future actions and the desirability of outcomes.
You Will Learn
Problem Solving Agents
Problem Solving Agents decide what to do by finding a sequence of actions that leads to a desirable state or solution.
An agent may need to plan when the best course of action is not immediately visible. They may need to think through a series of moves that will lead them to their goal state. Such an agent is known as a problem solving agent , and the computation it does is known as a search .
The problem solving agent follows this four phase problem solving process:
- Goal Formulation: This is the first and most basic phase in problem solving. It arranges specific steps to establish a target/goal that demands some activity to reach it. AI agents are now used to formulate goals.
- Problem Formulation: It is one of the fundamental steps in problem-solving that determines what action should be taken to reach the goal.
- Search: After the Goal and Problem Formulation, the agent simulates sequences of actions and has to look for a sequence of actions that reaches the goal. This process is called search, and the sequence is called a solution . The agent might have to simulate multiple sequences that do not reach the goal, but eventually, it will find a solution, or it will find that no solution is possible. A search algorithm takes a problem as input and outputs a sequence of actions.
- Execution: After the search phase, the agent can now execute the actions that are recommended by the search algorithm, one at a time. This final stage is known as the execution phase.
Problems and Solution
Before we move into the problem formulation phase, we must first define a problem in terms of problem solving agents.
A formal definition of a problem consists of five components:
Initial State
Transition model.
It is the agent’s starting state or initial step towards its goal. For example, if a taxi agent needs to travel to a location(B), but the taxi is already at location(A), the problem’s initial state would be the location (A).
It is a description of the possible actions that the agent can take. Given a state s, Actions ( s ) returns the actions that can be executed in s. Each of these actions is said to be appropriate in s.
It describes what each action does. It is specified by a function Result ( s, a ) that returns the state that results from doing action an in state s.
The initial state, actions, and transition model together define the state space of a problem, a set of all states reachable from the initial state by any sequence of actions. The state space forms a graph in which the nodes are states, and the links between the nodes are actions.
It determines if the given state is a goal state. Sometimes there is an explicit list of potential goal states, and the test merely verifies whether the provided state is one of them. The goal is sometimes expressed via an abstract attribute rather than an explicitly enumerated set of conditions.
It assigns a numerical cost to each path that leads to the goal. The problem solving agents choose a cost function that matches its performance measure. Remember that the optimal solution has the lowest path cost of all the solutions .
Example Problems
The problem solving approach has been used in a wide range of work contexts. There are two kinds of problem approaches
- Standardized/ Toy Problem: Its purpose is to demonstrate or practice various problem solving techniques. It can be described concisely and precisely, making it appropriate as a benchmark for academics to compare the performance of algorithms.
- Real-world Problems: It is real-world problems that need solutions. It does not rely on descriptions, unlike a toy problem, yet we can have a basic description of the issue.
Some Standardized/Toy Problems
Vacuum world problem.
Let us take a vacuum cleaner agent and it can move left or right and its jump is to suck up the dirt from the floor.
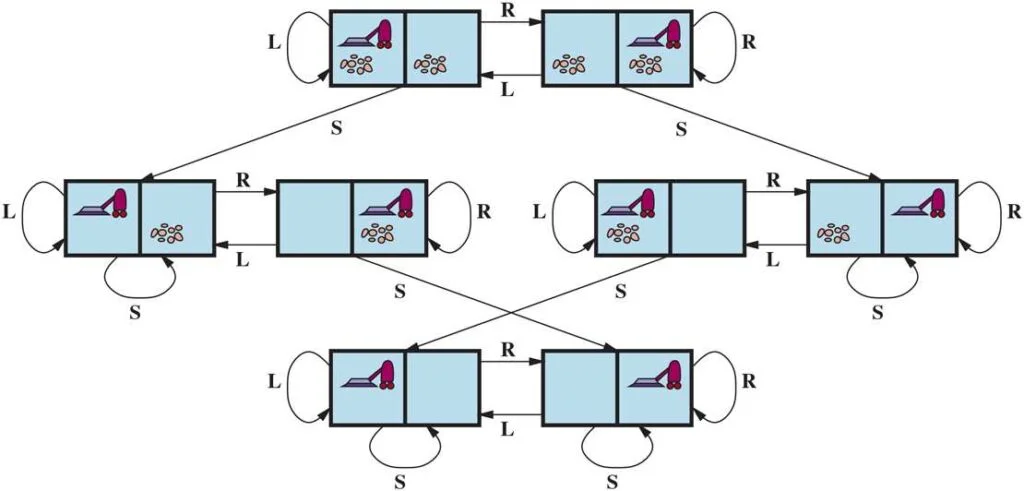
The vacuum world’s problem can be stated as follows:
States: A world state specifies which objects are housed in which cells. The objects in the vacuum world are the agent and any dirt. The agent can be in either of the two cells in the simple two-cell version, and each call can include dirt or not, therefore there are 2×2×2 = 8 states. A vacuum environment with n cells has n×2 n states in general.
Initial State: Any state can be specified as the starting point.
Actions: We defined three actions in the two-cell world: sucking, moving left, and moving right. More movement activities are required in a two-dimensional multi-cell world.
Transition Model: Suck cleans the agent’s cell of any filth; Forward moves the agent one cell forward in the direction it is facing unless it meets a wall, in which case the action has no effect. Backward moves the agent in the opposite direction, whilst TurnRight and TurnLeft rotate it by 90°.
Goal States: The states in which every cell is clean.
Action Cost: Each action costs 1.
8 Puzzle Problem
In a sliding-tile puzzle , a number of tiles (sometimes called blocks or pieces) are arranged in a grid with one or more blank spaces so that some of the tiles can slide into the blank space. One variant is the Rush Hour puzzle, in which cars and trucks slide around a 6 x 6 grid in an attempt to free a car from the traffic jam. Perhaps the best-known variant is the 8- puzzle (see Figure below ), which consists of a 3 x 3 grid with eight numbered tiles and one blank space, and the 15-puzzle on a 4 x 4 grid. The object is to reach a specified goal state, such as the one shown on the right of the figure. The standard formulation of the 8 puzzles is as follows:
STATES : A state description specifies the location of each of the tiles.
INITIAL STATE : Any state can be designated as the initial state. (Note that a parity property partitions the state space—any given goal can be reached from exactly half of the possible initial states.)
ACTIONS : While in the physical world it is a tile that slides, the simplest way of describing action is to think of the blank space moving Left , Right , Up , or Down . If the blank is at an edge or corner then not all actions will be applicable.
TRANSITION MODEL : Maps a state and action to a resulting state; for example, if we apply Left to the start state in the Figure below, the resulting state has the 5 and the blank switched.
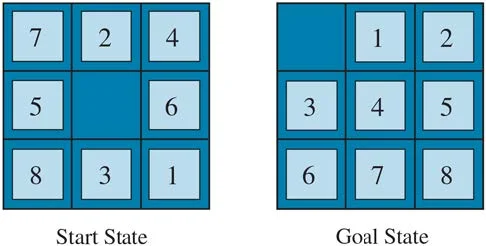
GOAL STATE : It identifies whether we have reached the correct goal state. Although any state could be the goal, we typically specify a state with the numbers in order, as in the Figure above.
ACTION COST : Each action costs 1.
You Might Like:
- Agents in Artificial Intelligence
Types of Environments in Artificial Intelligence
- Understanding PEAS in Artificial Intelligence
- River Crossing Puzzle | Farmer, Wolf, Goat and Cabbage
Share Article:
Digital image processing: all you need to know.
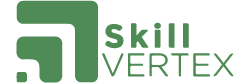
Problem-Solving Agents In Artificial Intelligence
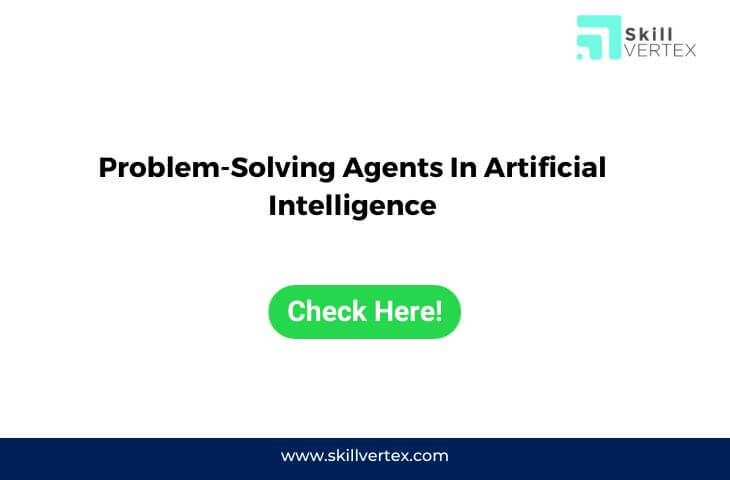
In artificial intelligence, a problem-solving agent refers to a type of intelligent agent designed to address and solve complex problems or tasks in its environment. These agents are a fundamental concept in AI and are used in various applications, from game-playing algorithms to robotics and decision-making systems. Here are some key characteristics and components of a problem-solving agent:
- Perception : Problem-solving agents typically have the ability to perceive or sense their environment. They can gather information about the current state of the world, often through sensors, cameras, or other data sources.
- Knowledge Base : These agents often possess some form of knowledge or representation of the problem domain. This knowledge can be encoded in various ways, such as rules, facts, or models, depending on the specific problem.
- Reasoning : Problem-solving agents employ reasoning mechanisms to make decisions and select actions based on their perception and knowledge. This involves processing information, making inferences, and selecting the best course of action.
- Planning : For many complex problems, problem-solving agents engage in planning. They consider different sequences of actions to achieve their goals and decide on the most suitable action plan.
- Actuation : After determining the best course of action, problem-solving agents take actions to interact with their environment. This can involve physical actions in the case of robotics or making decisions in more abstract problem-solving domains.
- Feedback : Problem-solving agents often receive feedback from their environment, which they use to adjust their actions and refine their problem-solving strategies. This feedback loop helps them adapt to changing conditions and improve their performance.
- Learning : Some problem-solving agents incorporate machine learning techniques to improve their performance over time. They can learn from experience, adapt their strategies, and become more efficient at solving similar problems in the future.
Problem-solving agents can vary greatly in complexity, from simple algorithms that solve straightforward puzzles to highly sophisticated AI systems that tackle complex, real-world problems. The design and implementation of problem-solving agents depend on the specific problem domain and the goals of the AI application.
Hello, I’m Hridhya Manoj. I’m passionate about technology and its ever-evolving landscape. With a deep love for writing and a curious mind, I enjoy translating complex concepts into understandable, engaging content. Let’s explore the world of tech together
Which Of The Following Is A Privilege In SQL Standard
Implicit Return Type Int In C
Leave a Comment Cancel reply
Save my name, email, and website in this browser for the next time I comment.
Reach Out to Us for Any Query
SkillVertex is an edtech organization that aims to provide upskilling and training to students as well as working professionals by delivering a diverse range of programs in accordance with their needs and future aspirations.
© 2024 Skill Vertex
Problem-Solving AI Agents
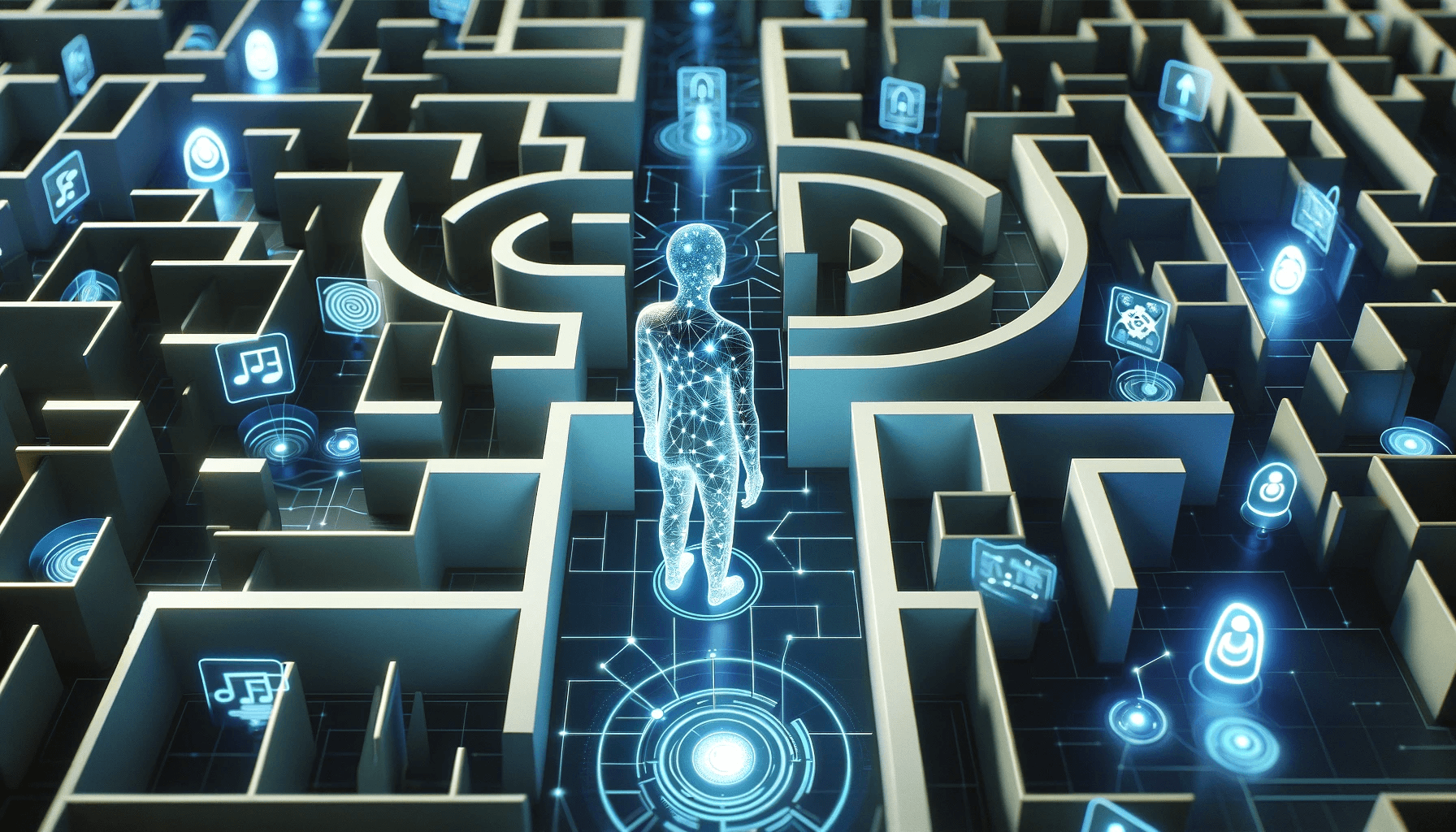
I started studying, again. October marked my new beginning at University of Pisa, as a student of the AI curriculum for the Computer Science Master's degree . I decided to begin from the Artificial Intelligence Fundamentals course which goal is to give us the fundamentals of the AI discipline. Starting from the definition of a rational agent, we will dive in into the concepts of reasoning, planning and solving problems, searching for solutions to them.
One of the first issues that an AI practicioner has to face is to understand and define as clearly as possible the environment of its problem. There are some framework that helps to standardize that definition, but you can easily imagine what are the main aspects to take attention to. One of those is the knowledge of the environment upon which the problem relies. For example, would be great to predict the presence of a disease from a simple blood analysis, and that's the case for some of them. But there are certainly some disease for which we didn't found yet a systematic correlation between values and prediction. In those cases, technologies like AI comes in help. That was an example in which there is a lack of knowledge, but you can also be faced with a real lack of visibility : let's think of a maze for example, or maybe a robot that wants to clean your house. It does not know the house "structure" before making an exploration phase. So, as you can imagine, there are a lot of possibilities here, both from the environment point of view, both from the instruments that you have to choose while addressing the problem.
The concepts in that course start to look at those problems relaxing the real-world scenarios, investigating abstract and simplified environment first. So, let's imagine ourselves in a fully observable environment. That means that in every moment, we are able to have a complete point of view upon the whole problem, aware of all the information that we need to solve it . At the same time, though, we also know that we are not able to immediately tell with certainty what's the correct action to take to reach our goal. With that environment in front of us, the correct instrument to use are the so called problem-solving agent , which strategy is to plan ahead and consider a path that bring them from a starting point toward the goal state. That process is known (by all of us) as search .
Let's give us an example problem now: we are a little funny cell of a grid. We know that this grid has a maze, and we also know that we want to find our friend, that is somewhere inside the grid. Lastly, thanks to our drone, we also have a clear view from the top of the grid that gives us the whole knowledge of the current situation of our environment.
First thing to do, we have to formalize the problem as a search problem:
- What are the states on which the environment can be in? Defining the state space , we can say that it is composed by the set of the grid's cell coordinates.
- What's the initial state ? It's where we are right know, the coordinates of our position in the grid.
- What is the goal ? Is there only one of it? We have to find our friend, so our goal will be the coordinates of our friend in the grid.
- What are the actions that we can take? Well, generally speaking we can go ahead toward the top, right, bottom or left cell. In that case, we should be careful to not step on the walls of our maze, though.
- What is the effect of our action on the environment? Also called the transition model , it serves us to explain what is the state resulting from a certain action taken from a certain state. We know this, we just need to update our coordinates based on the direction of the movement.
- What is the cost of our actions? For example, let's imagine a navigator agent. We need to know how much kilometers is long every available roads towards our goal to minimize the costs for our passenger (actually, maybe would be worth to focus on the time, but it's just an example).
Okay, we should have everything now to solve our problem. As we already said we need to find the correct sequence of actions that brings the agent from the initial state to the goal state . The agent will find it building a search tree where:
- the root of the tree is the initial state;
- on every state, the agent will consider all the legal actions that can be taken from that state (we formalized this information before) and will take them, expanding the current node to create different branches of the tree;
- all the current leaves node, form the so-called frontier , i.e. the list of nodes that can be expanded in the subsequent search step. Which node will be chosen by the agent from the frontier is what characterizes the chosen searching algorithm. This is one of the most important choice to make when designing the agent;
- when a node is chosen from the frontier for the expansion, the agent will check if that node encodes the goal state . If so, the search is ended and the problem's solved!
Let's bring that theory to a practical example. Supposing we have a 3x4 grid, with a starting and a goal position well defined.
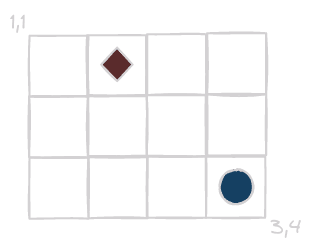
So, as you can see the initial state is (3,4) while our goal is (1,2) . At the beginning of the searching process, our frontier will be made out of only the initial state.
- Choose a node from the frontier. How? Let's just randomize this choice right know, we will later see different strategies that we can adopt in that step. Anyway, at the beginning we will have only one choice: the initial state.
- Is this a goal state? Nope, so we have to take the actions phase.
- What are the legal actions that we can take from the current state? In this particular case, we are in (3,4) so we can only go up and toward the left.
- Taking all the available actions, we will expand the tree and put those resulting nodes (obtained by adding 1 to the right coordinate, as described by our transition model) in our frontier;
- New iteration!
When a goal state is found, we just need to backtrack using the parent property to build all the way up the solution path.
I have made up an image that could help us to grasp a bit more around the search loop described above, let's see and discuss it.
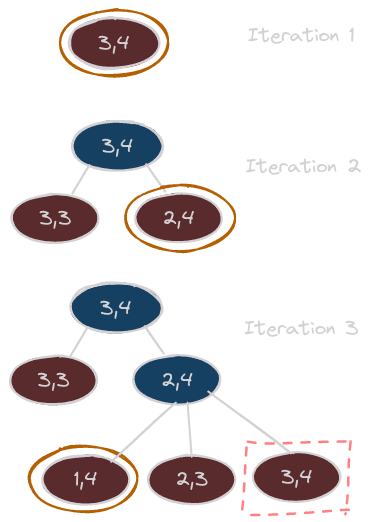
I feel like we need a legend here, so, at each iteration:
- the red nodes are the ones contained in the frontier;
- the blue nodes are the ones that have been already chosen and expanded;
- the orange outlined nodes are the one chosen from the frontier for the expansion;
You can also notice that there is a node outlined by a dashed red boxy rectangle . Looking at it, we can see that it seems to be a duplicate. Actually, it's not so right to use that duplicate word: we said that those nodes contains more information outside of the simple state that they encodes, like the cost of the path, the parent and so on. So, even if the encoded state is the same as the root, the nodes are pretty different! Anyway, it was important to highlight this possible situation, because could be part of the design process to implement some kind of strategy to avoid redundant paths like this one. Actually, there are some cases where this is a necessary choice to avoid infinite loop . My advice would be to always implement a strategy to avoid redundant path. The simplest way to do that would be to keep track of the visited state and avoid expansion that bring us again on those already checked. There are some cases, though, were this decision can be a little bit too greedy! That's because can happen that a path that we see as redundant maybe bring us to the same state with a better performance measure (i.e., with a lower cost). So, would be wise to keep track of the visited node, but also to check against them taking into account the cost spent to arrive there.
Great, so know we know the whole theory that we need, and we also understood that there are actually two main aspects in that search framework just presented:
- the strategy used to choice the node to expand from the frontier ;
- the strategy used to check for redundant paths during the search process;
These two things characterize the different search algorithms , that we can divide into two main categories:
- Uninformed search : the family of algorithms that does not exploit knowledge about what is known about the goal during the search process;
- Informed Search : the family of algorithms that exploit that knowledge to improve the quality of their search. With quality, we means maximize the performance measure of the agent (e.g., minimize time when using our Google Maps application).
Uninformed Search
Breadth-first search (bfs).
The idea of this algorithm is to explore broadly the search tree, checking all the nodes at a certain depth before going down on the tree. I labeled in that graphic the order in which the nodes would be visited by this algorithm:
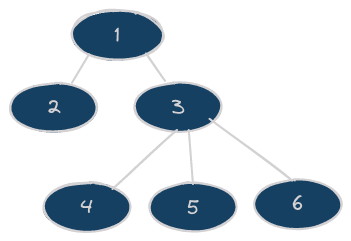
From a practical point of view, this is implemented treating the frontier as a FIFO queue (for example, using the shift array method of Javascript when retrieving, assuming the use of push when inserting). Every time we want to expand a node, we have to choose the oldest one in the frontier. Talking about the strategy of checking against already visited nodes, we just need to check for the encoded state, without taking care of comparing the cost between the old and the redundant node. This is because, as per its nature, the Breadth First search algorithm ensure us that every time we visit a node, this has been visited through the optimal way (i.e., with the best performance measure).
Depth-First search (DFS)
Differently, as its name suggests, DFS algorithm tries to go as deep as possible before trying other branching routes. Again, here is a little graphic labeled to show you the order of visited nodes:
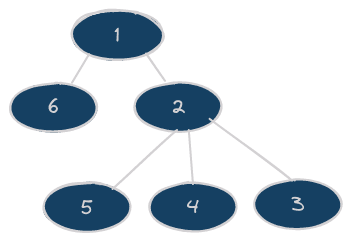
From a practical point of view, this is implemented treating the frontier as a LIFO queue (for example, using the pop array method of Javascript when retrieving, assuming the use of push when inserting). The idea is to expand always the last inserted node, the youngest one. Different is the issue with redundant paths here. First of all, it is extremely important to implement one, because this algorithm is very vulnerable to infinite loops . There is another issue, though: differently from the Breadth First search, DFS does not ensure us to find the optimal solution. For that reason, is really suggested to implement that redundant strategy not only checking against the state encoded in the node, but also using the current cost of the already visited state. Maybe that redundant path is better!
Dijkstra's algorithm
We are now moving over something that prove more reasoning. DFS and BFS have inside them a lot of mechanical-nature. They are systematic, and they face a great challenge when the state space is huge . We should start to think smarter, introducing a little bit of (artificial) intelligence. The Dijkstra's algorithm is still an uninformed algorithm, but at least it tries to exploit what it can knows about the environment: the frontier management strategy is to choose the node with the lower path cost (up to that moment). Now, let's make up another example with respect to the simple grid. That's because in the grid example every action has the same cost, so it's not so helpful to visualize the nature of this algorithm. Inspired from the AIMA example of Romanian roads, let's imagine this situation:
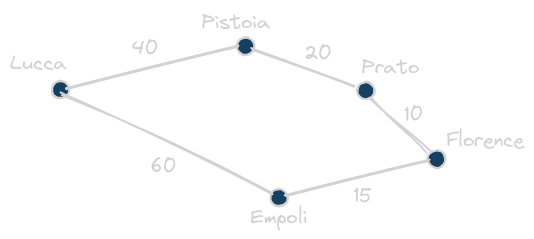
We are in Florence, and we want to reach the city of Lucca, following the instructions given by the Dijkstra's algorithm. The numbers on the arches are the costs of the roads (they are random, they don't recall the reality). That's the reasoning done by the algorithm:
- at the beginning we just have Florence in our frontier, let's expand it;
- we have two nodes in the frontier: Empoli, with a cost of 15, and Prato, with a cost of 10. The algorithm will choose the cheaper one: Prato! Expanding it, we put in the frontier Pistoia, with a cost of 30;
- we have two nodes in the frontier: Empoli, with a cost of 15, and Pistoia, with a cost of 30. Empoli is chosen and expanded. Now we have Lucca in the frontier, with a cost of 75. It is important to notice that, even though Lucca is our goal, we are not stopping here. That's because we can still find some better paths to it.
- we have two nodes in the frontier: Lucca, with a cost of 75, and Pistoia, with a cost of 30. Expanding Pistoia, we are ready for our last step!
- we have two nodes in the frontier: Lucca, with a cost of 75, and Lucca with a cost of 70. The chosen solution will then be Florence-Prato-Pistoia-Lucca one!
We have just experienced an interesting property of this algorithm. Even though we found a goal state earlier, we waited on labeling it as a solution, cause as long as we have some path with a lower cost, we could have potential solutions that are better!
Informed Search
Before presenting two of the most famous informed search algorithms, we need to define the meaning of that informed definition . The main idea is that the agent will exploit some kind of information about the goal state. This information is called heuristic . Defined as a function of the current node n , we can say that h(n) is the estimated cost from the node n toward our goal state. Now, there is a bunch of theory around those concepts, especially to prove optimality of some algorithms based on the heuristic's nature. By now, let's just assume those requirements for our heuristic function:
- the heuristic function is always greater than 0;
- the heuristic function of a goal state is equal to 0;
- the heuristic function of a node is always less or equal to the real optimal cost from that node to a goal state (i.e., the heuristic function is literally an optimistic estimation );
Now we can take a look at the two algorithms.
Greedy best-first search
The idea here is to choose from the frontier the nodes with lower heuristic value first. It seems to be reasonable: we know that the heuristic is an estimation of the real optimal cost, so, we prefer to focus on those nodes that seems to cost less toward our solution. Actually, this is not so straight-forward, and we can see why with an example. Then, we'll have pretty clear the reason of the greedy definition. Let's imagine this strange, but possible, situation:
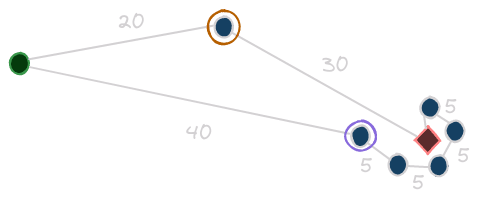
First thing first, we should define our heuristic. For sake of simplicity, let's say that h(n) is the straight-line distance between the state n and the goal state (i.e., the red diamond). Okay now, supposing that our initial state is the green filled circle, the greedy approach will rather choose the state outlined by the purple circle with respect to the orange one. From there, it will follow the chain of states so close to the goal. If you sum the path cost, though, you can see that this path is worse than the other one. It is clear indeed what is the issue with that algorithm: it relies only on the estimation of the heuristic, without taking care of the actual cost needed to follow the current route.
A* Algorithm
To approach this limitation, the A* algorithm comes in our help. Its idea is to take into account, when choosing the node to expand, not only the value of the heuristic, but also the real cost to reach the node (it combines them computing the sum of those when evaluating nodes). Intuitively, we can understand that this algorithm will try to minimize the estimated cost to reach the goal while minimizing the actual cost of the solution path. This makes the A* algorithm not only complete, but also optimal (with the requirements that we put on our heuristic before). Recalling the previous example:
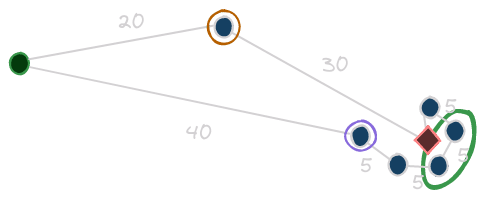
With respect to the path followed by the greedy approach, with the A* algorithm there will be a moment where the cost of the sub-optimal route will make the whole evaluation function greater with respect to the other one. This moment will be more or less around the nodes that I outlined with the light green ellipse . It is there that the A* will switch to the other path, finding the one that is actually optimal!
Introducing Searchy 🔎
If you have read my previous article, you already know that I don't like theory without practice. The goal of this article was to consolidate to myself the understanding of those concepts, as they are part of the program of my University's course. But while studying them, I thought would be cool to implement and visualize them from a concrete point of view. That is why I'm introducing to you my little side-project 🔎 Searchy .
🔎 Searchy wants to be an environment to test and visualize different problem-solving AI agents (i.e., searching algorithms) upon a simple problem as a fully-observable maze scenario. You can see a glance of it in that screenshot:
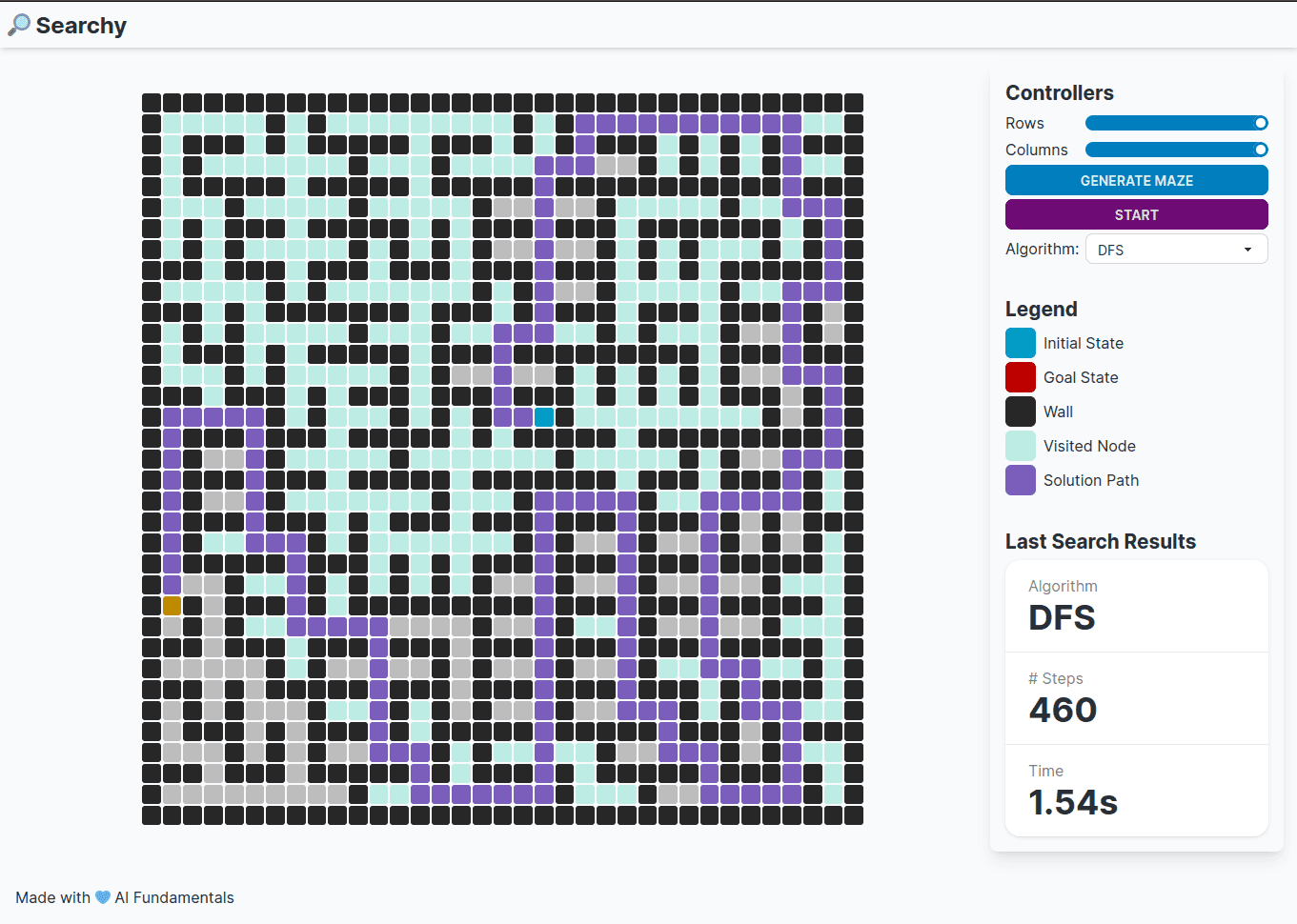
Right know, the usage is pretty simple: you have a grid, and you can customize the number of rows and columns. You can generate a randomized maze and the chosen algorithm will perform the search. Once the search is started, a couple of stats will be gathered during the process. Those are finally shown in the result section in the sidebar, together with the solution path on the grid.
My willingness is to open source this and try to continuously improve and evolve it to support less trivial scenarios, more algorithms, more insightful performance comparisons and so on. Would be good to have this application as a robust environment on which try, understand and test searching algorithms while studying those concepts.
📚 References
- Artificial Intelligence: a Modern Approach
💻 Practical References:
- Speakers & Mentors
- AI services
Learn About Problem Solving Agents in Artificial Intelligence on Tutorialspoint
Artificial intelligence is a rapidly growing field that aims to develop computer systems capable of performing tasks that typically require human intelligence. One of the key areas in AI is problem solving, where agents are designed to find solutions to complex problems.
TutorialsPoint provides an in-depth tutorial on problem solving agents in artificial intelligence, covering various techniques and algorithms used to tackle different types of problems. Whether you are a beginner or an experienced AI practitioner, this tutorial will equip you with the knowledge and skills needed to build effective problem solving agents.
In this tutorial, you will learn about different problem solving frameworks, such as the goal-based approach, the utility-based approach, and the constraint satisfaction approach. You will also explore various search algorithms, including uninformed search algorithms like depth-first search and breadth-first search, as well as informed search algorithms like A* search and greedy search.
Problem solving agents in artificial intelligence play a crucial role in many real-world applications, ranging from robotics and automation to data analysis and decision-making systems. By mastering the concepts and techniques covered in this tutorial, you will be able to design and develop intelligent agents that can effectively solve complex problems.
What are Problem Solving Agents?
In the field of artificial intelligence, problem solving agents are intelligent systems that are designed to solve complex problems. These agents are equipped with the ability to analyze a given problem, search for possible solutions, and select the best solution based on a set of defined criteria.
Problem solving agents can be thought of as entities that can interact with their environment and take actions to achieve a desired goal. These agents are typically equipped with sensors to perceive the environment, an internal representation of the problem, and actuators to take actions.
One of the key challenges in designing problem solving agents is to define a suitable representation of the problem and its associated constraints. This representation allows the agent to reason about the problem and generate potential solutions. The agent can then evaluate these solutions and select the one that is most likely to achieve the desired goal.
Problem solving agents can be encountered in various domains, such as robotics, computer vision, natural language processing, and even in game playing. These agents can be implemented using different techniques, including search algorithms, constraint satisfaction algorithms, and machine learning algorithms.
In conclusion, problem solving agents are intelligent systems that are designed to solve complex problems by analyzing the environment, searching for solutions, and selecting the best solution based on a set of defined criteria. These agents can be encountered in various domains and can be implemented using different techniques.
Types of Problem Solving Agents
In the field of artificial intelligence, problem solving agents are designed to tackle a wide range of issues and tasks. These agents are built to analyze problems, explore potential solutions, and make decisions based on their findings. Here, we will explore some of the common types of problem solving agents.
Simple Reflex Agents
A simple reflex agent is a basic type of problem solving agent that relies on a set of predefined rules or conditions to make decisions. These rules are typically in the form of “if-then” statements, where the agent takes certain actions based on the current state of the problem. Simple reflex agents are often used in situations where the problem can be easily mapped to a small set of conditions.
Model-Based Reflex Agents
A model-based reflex agent goes beyond simple reflex agents by maintaining an internal model of the problem and its environment. This model allows the agent to have a better understanding of its current state and make more informed decisions. Model-based reflex agents use the current state and the model to determine the appropriate action to take. These agents are often used in more complex problem-solving scenarios.
Goal-Based Agents
A goal-based agent is designed to achieve a specific goal or set of goals. These agents analyze the current state of the problem and then determine a sequence of actions that will lead to the desired outcome. Goal-based agents often use search algorithms to explore the possible paths and make decisions based on their analysis. These agents are commonly used in planning and optimization problems.
Utility-Based Agents
Utility-based agents make decisions based on a utility function or a measure of the desirability of different outcomes. These agents assign a value or utility to each possible action and choose the action that maximizes the overall utility. Utility-based agents are commonly used in decision-making problems where there are multiple possible outcomes with varying levels of desirability.
These are just a few examples of the types of problem solving agents that can be found in the field of artificial intelligence. Each type of agent has its own strengths and weaknesses and is suited to different problem-solving scenarios. By understanding the different types of agents, developers and researchers can choose the most appropriate agent for their specific problem and improve the efficiency and effectiveness of their problem-solving solutions.
Components of Problem Solving Agents
A problem-solving agent is a key concept in the field of artificial intelligence (AI). It is an agent that can analyze a given problem and take appropriate actions to solve it. The agents are designed using a set of components that work together to achieve their goals.
One of the main components of a problem-solving agent is the solving component. This component is responsible for applying different algorithms and techniques to find the best solution to a given problem. The solving component can use various approaches such as search algorithms, constraint satisfaction, optimization techniques, and machine learning.
TutorialsPoint is a popular online platform that offers a wealth of resources on various topics, including artificial intelligence and problem-solving agents. These tutorials provide step-by-step instructions and examples to help learners understand and implement different problem-solving techniques.
Another important component of a problem-solving agent is the knowledge component. This component stores the agent’s knowledge about the problem domain, including facts, rules, and constraints. The knowledge component is crucial for guiding the agent’s problem-solving process and making informed decisions.
The problem component is responsible for representing and defining the problem that the agent needs to solve. It includes information such as the initial state, goal state, and possible actions that the agent can take. The problem component provides the necessary context for the agent to analyze and solve the problem effectively.
Finally, the agents component is responsible for coordinating the activities of different components and controlling the overall behavior of the problem-solving agent. It receives inputs from the environment, communicates with the other components, and takes actions based on the current state of the problem. The agents component plays a crucial role in ensuring the problem-solving agent operates efficiently and effectively.
In conclusion, problem-solving agents in artificial intelligence are designed using various components such as solving, knowledge, problem, and agents. These components work together to analyze a problem, apply appropriate techniques, and find the best solution. TutorialsPoint is a valuable resource for learning about different problem-solving techniques and implementing them in practice.
Search Strategies for Problem Solving Agents
Intelligence is a complex and fascinating field that encompasses a wide range of topics and technologies. One area of focus in artificial intelligence is problem solving. Problem solving agents are designed to find solutions to specific problems by searching through a large space of possible solutions.
TutorialsPoint provides valuable resources and tutorials on problem solving agents in artificial intelligence. These tutorials cover various search strategies that can be employed by problem solving agents to efficiently find optimal solutions.
Search strategies play a crucial role in the efficiency and effectiveness of problem solving agents. Some common search strategies include:
These are just a few examples of search strategies that problem solving agents can utilize. The choice of search strategy depends on the specific problem at hand and the available resources.
TutorialsPoint’s comprehensive tutorials on problem solving agents in artificial intelligence provide in-depth explanations, examples, and implementation guides for each search strategy. By leveraging these tutorials, developers and researchers can enhance their understanding of problem solving agents and apply them to real-world scenarios.
Uninformed Search Algorithms
Uninformed search algorithms are a category of algorithms used by problem-solving agents in artificial intelligence. These algorithms do not have any information about the problem domain and make decisions solely based on the current state and possible actions.
Breadth-First Search
Breadth-first search is one of the basic uninformed search algorithms. It explores all the neighbor nodes at the present depth before moving on to nodes at the next depth level. It guarantees the shortest path to the goal state if there is one, but it can be inefficient for large search spaces.
Depth-First Search
Depth-first search is another uninformed search algorithm. It explores a path all the way to the deepest level before backtracking. It is often implemented using a stack data structure. Depth-first search is not guaranteed to find the shortest path to the goal state, but it can be more memory-efficient than breadth-first search.
Uninformed search algorithms are widely used in problem-solving scenarios where there is no additional information available about the problem domain. They are important tools in the field of artificial intelligence and are taught in many tutorials and courses, including the ones available on TutorialsPoint.
Breadth First Search (BFS)
Breadth First Search (BFS) is a fundamental algorithm used in artificial intelligence for problem solving. It is a graph traversal algorithm that explores all vertices of a graph in a breadthward motion, starting from a given source vertex.
BFS is commonly used to solve problems in AI, such as finding the shortest path between two nodes, determining if a graph is connected, or generating all possible solutions to a problem.
In BFS, the algorithm continuously explores the vertices adjacent to the current vertex before moving on to the next level of vertices. This approach ensures that all vertices at each level are visited before proceeding to the next level. The algorithm uses a queue to keep track of the vertices that need to be visited.
The main steps of the BFS algorithm are as follows:
- Choose a source vertex and mark it as visited.
- Enqueue the source vertex.
- While the queue is not empty, dequeue a vertex and visit it.
- Enqueue all the adjacent vertices of the visited vertex that have not been visited before.
- Mark the dequeued vertex as visited.
By following these steps, BFS explores the graph level by level, guaranteeing that the shortest path from the source vertex to any other vertex is found.
BFS has a time complexity of O(V + E), where V is the number of vertices and E is the number of edges in the graph. It is considered an efficient algorithm for solving problems in artificial intelligence.
Depth First Search (DFS)
Depth First Search (DFS) is a popular graph traversal algorithm commonly used in artificial intelligence and problem solving agents. It is also commonly used in tutorialspoint for teaching the concepts of graph traversal algorithms.
DFS starts at a given node of a graph and explores as far as possible along each branch before backtracking. It uses a stack data structure to keep track of the nodes to be visited. The algorithm visit nodes in a depthward motion, meaning that it explores the deepest paths in the graph first.
The DFS algorithm can be implemented as follows:
- Start at the given node and mark it as visited.
- If the node has unvisited neighbors, choose one and push it onto the stack.
- If there are no unvisited neighbors, backtrack by popping the stack.
- If the stack is empty, the algorithm terminates.
- Repeat steps 2-4 until all nodes are visited.
DFS is often used to solve problems that can be represented as graphs, such as finding solutions in a maze or searching for a path between two points. It can also be used to perform topological sorting and cycle detection in directed graphs.
DFS has a few advantages over other graph traversal algorithms. It requires less memory than breadth-first search (BFS) because it only needs to store the visited nodes in a stack. It can also be easily implemented recursively.
However, DFS may not find the shortest path between two nodes in a graph, as it explores deep paths first. It can also get stuck in infinite loops if the graph contains cycles.
Overall, DFS is a powerful algorithm that can be used to solve a wide range of problems in artificial intelligence and problem solving agents. By understanding how it works, developers can effectively apply it to various scenarios in their projects.
Iterative Deepening Depth First Search (IDDFS)
The Iterative Deepening Depth First Search (IDDFS) is a technique used in artificial intelligence to solve problems efficiently. It is a combination of depth-first search (DFS) and breadth-first search (BFS) algorithms.
The IDDFS algorithm starts with a depth limit of 0 and gradually increases the depth limit until the goal is found or all possibilities have been explored. It works by performing a depth-first search up to the current depth limit, and if the goal is not found, it resets the visited nodes and increases the depth limit by 1.
This approach allows the IDDFS algorithm to explore the search space more efficiently compared to standard depth-first search. It avoids the disadvantages of BFS, such as high memory usage, by only keeping track of the current path.
The IDDFS algorithm is particularly useful in situations where the search space is large and the goal is likely to be found at a relatively small depth. It guarantees that the algorithm will find the solution if it exists within the search space.
Overall, the IDDFS algorithm is a powerful tool in problem-solving agents in artificial intelligence. It combines the advantages of depth-first search and breadth-first search, making it an efficient and effective approach for solving complex problems.
Uniform Cost Search (UCS)
Uniform Cost Search (UCS) is a problem-solving algorithm used in the field of artificial intelligence. It is a variant of the general graph search algorithm, which aims to find the cheapest path from a starting node to a goal node in a weighted graph.
In UCS, each action in the problem domain has a cost associated with it. The algorithm expands the nodes in a graph in a cost-effective manner, always choosing the node with the lowest cost so far. This ensures that the optimal solution with the minimum total cost is found.
The UCS algorithm maintains a priority queue of nodes, where the priority is based on the accumulated cost to reach each node. Initially, the start node is inserted into the priority queue with a cost of zero. The algorithm then iteratively selects and expands the node with the lowest cost, updating the priority queue accordingly.
During the expansion process, the algorithm checks if the goal node has been reached. If not, it generates the successor nodes of the expanded node and adds them to the priority queue. The cost of each successor node is calculated by adding the cost of the action that led to that node to the total cost so far. This process continues until the goal node is reached.
Uniform Cost Search is considered to be complete and optimal, meaning it will always find a solution if one exists, and it will find the optimal solution with the minimum cost. However, it can be computationally expensive, especially in large graphs or graphs with high branching factors.
In summary, Uniform Cost Search is a powerful problem-solving algorithm used in artificial intelligence to find the cheapest path from a starting node to a goal node in a weighted graph. By prioritizing nodes based on their accumulated cost, UCS ensures that the optimal solution with the minimum total cost is found.
Informed Search Algorithms
In the field of Artificial Intelligence, informed search algorithms are a group of problem-solving agents that use knowledge or information about the problem domain to guide the search process. Unlike uninformed search algorithms, which do not have any additional information about the problem, informed search algorithms make use of heuristics or other measures of desirability to guide the search towards the goal state more efficiently.
One of the most well-known informed search algorithms is the A* algorithm. The A* algorithm uses a combination of the cost to reach a certain state and an estimate of the cost from that state to the goal state, known as the heuristic function. By selecting the states with the lowest total cost, the A* algorithm can efficiently navigate through the search space and find the optimal solution.
Another popular informed search algorithm is the greedy best-first search. Greedy best-first search evaluates each state based solely on the heuristic estimate of the cost to reach the goal state. It always chooses the state that appears to be the closest to the goal, without considering the overall cost. Although this algorithm is efficient for some problems, it can also get stuck in local optima and fail to find the optimal solution.
In addition to A* and greedy best-first search, there are various other informed search algorithms, such as the iterative deepening A* (IDA*) algorithm and the simulated annealing algorithm. Each algorithm has its strengths and weaknesses and is suited for different types of problems.
In conclusion, informed search algorithms play an important role in problem-solving in the field of Artificial Intelligence. They use additional knowledge or information about the problem domain to guide the search process and find optimal solutions more efficiently. By considering both the cost to reach a certain state and an estimate of the cost to the goal state, these algorithms can effectively navigate through the search space and find the best possible solution.
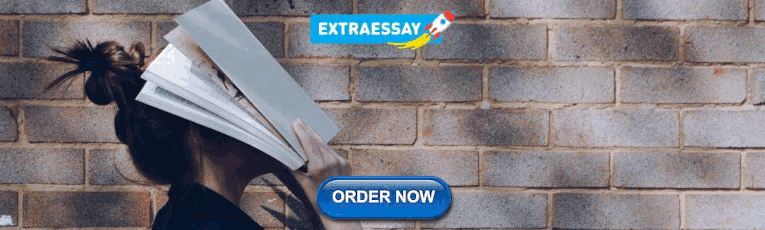
Heuristic Functions
In the field of artificial intelligence, heuristic functions play a crucial role in problem solving. A heuristic function is a function that estimates the cost or utility of reaching a goal state from a given state in a problem. It provides a way for agents to make informed decisions about their actions while navigating through a problem-solving process.
Heuristic functions are designed to guide the search process towards the most promising directions in order to find a solution efficiently. They provide a measure of how close a particular state is to the goal state, without having complete information about the problem domain. This allows the agent to prioritize its actions and choose the most promising ones at each step.
When designing a heuristic function, it is important to consider the specific problem at hand and the available knowledge about it. The function should be able to assess the cost or utility of reaching the goal state based on the available information and the characteristics of the problem. It should also be computationally efficient to ensure that it can be used in real-time problem-solving scenarios.
The effectiveness of a heuristic function depends on its ability to accurately estimate the cost or utility of reaching the goal state. A good heuristic function should provide a tight lower bound on the actual cost, meaning that it should never overestimate the distance to the goal. This allows the agent to efficiently explore the solution space and reach the goal state optimally.
In conclusion, heuristic functions are essential tools in artificial intelligence for problem solving. They enable agents to make informed decisions and guide the search process towards the most promising directions. By accurately estimating the cost or utility of reaching the goal state, heuristic functions help agents find optimal solutions efficiently.
A* Algorithm
The A* algorithm is a commonly used artificial intelligence technique for solving problems. It is particularly useful in problem-solving agents that aim to find the optimal path or solution. With the A* algorithm, an agent can navigate through a problem space, evaluating and selecting the most promising paths based on heuristic estimates and actual costs.
A* combines the advantages of both uniform cost search and best-first search algorithms. It uses a heuristic function to estimate the cost from the current state to the goal state, allowing the agent to prioritize its search and explore the most promising paths first. The heuristic function provides an estimation of the remaining cost, often referred to as the “H-cost”.
The algorithm maintains a priority queue, also known as an open list, that keeps track of the states or nodes to be expanded. At each step, the agent selects the state with the lowest combined cost, known as the “F-cost”, which is calculated as the sum of the actual cost from the starting state to the current state (known as the “G-cost”) and the heuristic cost.
The A* algorithm guarantees to find the optimal path if certain conditions are met. The heuristic function must be admissible, meaning that it never overestimates the actual cost. Additionally, the function should be consistent or monotonic, which ensures that the heuristic estimate is always less than or equal to the cost of reaching the goal state from the current state.
In conclusion, the A* algorithm is a powerful tool used in artificial intelligence for solving problems. It combines the benefits of both uniform cost search and best-first search algorithms, making it an efficient and effective choice for problem-solving agents. By prioritizing the most promising paths, as estimated by the heuristic function, the A* algorithm can find the optimal solution in a timely manner.
Greedy Best-first Search
The Greedy Best-first Search is a type of problem solving algorithm that is used in artificial intelligence. It is an informed search algorithm that uses heuristics to guide its search through a problem space.
The goal of the Greedy Best-first Search is to find a solution to a problem by always choosing the most promising path at each step. It does this by evaluating the estimated cost of each possible next step based on a heuristic function. The heuristic function provides an estimate of how close the current state is to the goal state.
This algorithm is called “greedy” because it always chooses the path that appears to be the best at the current moment, without considering the future consequences. It does not take into account the possibility that a different path might lead to a better solution in the long run.
Despite its simplicity, the Greedy Best-first Search can be quite effective in certain types of problems. However, it has some limitations. Since it only considers the local information at each step, it may overlook better paths that require more steps to reach the goal. In addition, it may get stuck in loops or infinite cycles if there are no better paths to follow.
Algorithm Steps:
- Initialize the search with the initial state.
- Choose the most promising state based on the heuristic function.
- Move to the chosen state.
- If the chosen state is the goal state, stop the search.
In conclusion, the Greedy Best-first Search is a simple yet effective problem solving algorithm in artificial intelligence. It can be a useful tool when solving certain types of problems, but it also has its limitations. It is important to understand the strengths and weaknesses of different search algorithms when applying them to real-world problems.
Hill Climbing
The Hill Climbing algorithm is a popular search algorithm used in artificial intelligence to solve problem-solving tasks. It is commonly used in optimization problems where the goal is to find the best possible solution among a set of alternatives.
In hill climbing, the agent starts with an initial solution and iteratively makes small improvements to the solution by locally exploring the neighboring states. The agent selects the next state to move to based on an evaluation function, which assigns a value to each state indicating its desirability. The agent moves to the state with the highest value and repeats the process until no better state can be found.
The hill climbing algorithm can be thought of as climbing a hill, where the agent starts at the bottom and tries to reach the highest point by taking steps uphill. However, hill climbing can get stuck at local optima, which are points that are higher than their immediate neighbors but lower than the global optimum. This means that the algorithm may fail to find the best solution if it gets trapped in a local maximum.
Types of Hill Climbing
There are different variations of the hill climbing algorithm that address its limitations:
Steepest Ascent Hill Climbing
The steepest ascent hill climbing algorithm is a variation of hill climbing where the agent considers all possible moves from the current state and selects the one that leads to the state with the highest value. This approach ensures that the agent moves uphill as much as possible in each iteration, but it can be computationally expensive as it needs to evaluate all possible moves.
First-Choice Hill Climbing
The first-choice hill climbing algorithm is another variation of hill climbing where the agent randomly selects one move from the current state and moves to the state with the highest value among the randomly selected moves. This approach can be more efficient than steepest ascent hill climbing as it does not need to evaluate all possible moves, but it may still get stuck in local optima.
In conclusion, hill climbing is a widely used algorithm in artificial intelligence for solving problem-solving tasks. Despite its limitations, it can be effective in finding good solutions for optimization problems. However, it is important to be aware of the possibility of getting stuck in local optima and consider using other algorithms or techniques to overcome this limitation.
Simulated Annealing
Simulated Annealing is a problem-solving algorithm used in artificial intelligence. It is a probabilistic method that is inspired by the annealing process used in metallurgy.
The goal of simulated annealing is to find a good solution to a problem, even when the search space is large and the problem is difficult to solve. It starts with an initial solution and gradually explores the search space, making small random changes to the solution. These changes may improve the solution or make it worse.
The algorithm uses a temperature parameter to control the probability of accepting a worse solution. At high temperatures, there is a high probability of accepting a worse solution, allowing the algorithm to escape local maxima. As the temperature decreases, the probability of accepting a worse solution decreases, leading to the convergence of the algorithm to a near-optimal solution.
Simulated annealing is particularly useful for solving problems where finding the optimal solution is difficult or time-consuming. It has been successfully applied to various problems such as the traveling salesman problem, the job shop scheduling problem, and the graph coloring problem.
In conclusion, simulated annealing is a powerful tool for problem-solving agents in artificial intelligence. It allows them to navigate complex search spaces and find near-optimal solutions. By using a probabilistic approach and gradually reducing the temperature, it can efficiently explore the solution space and overcome local maxima. This makes it an essential technique for solving challenging problems in artificial intelligence.
Genetic Algorithms
Genetic Algorithms (GAs) are a type of Artificial Intelligence (AI) technique used for problem-solving. They are commonly used in various fields, including optimization, machine learning, and data mining. GAs are inspired by the process of natural selection and evolution.
In a GA, a population of candidate solutions evolves over time to find the optimal solution to a given problem. Each candidate solution, also known as an individual, is represented as a set of chromosomes, which are strings of binary digits. The fitness of each individual is evaluated based on how well it solves the problem.
During the evolution process, individuals with higher fitness have a higher chance of being selected for reproduction. Genetic operators such as crossover and mutation are applied to the selected individuals to create new offspring. The new offspring then replace the less fit individuals in the population.
This process of selection, reproduction, and replacement continues over multiple generations until the population converges to a near-optimal solution. The convergence speed and quality of the solution depend on the parameters and characteristics of the GA, such as the selection method, crossover rate, mutation rate, and population size.
The use of GAs allows for the exploration of a large search space and can often find good solutions to complex problems. However, GAs do not guarantee finding the optimal solution, but rather give a good approximation.
TutorialsPoint is a great resource for learning about Artificial Intelligence, including Genetic Algorithms. They provide detailed tutorials and examples that help beginners understand the concepts and implementation of GAs. By following their tutorials, you can learn how to apply Genetic Algorithms to solve various problems in the field of AI.
Constraint Satisfaction Problems (CSPs)
In the field of artificial intelligence, problem solving agents often encounter situations where they need to fulfill a set of constraints in order to find a solution. These problems are known as Constraint Satisfaction Problems (CSPs). CSPs involve finding values for a set of variables that satisfy a given set of constraints.
A CSP can be defined as a triple (X, D, C), where:
- X is a set of variables, each with a domain of possible values.
- D is a set of domains, where each domain contains the possible values for its corresponding variable.
- C is a set of constraints, which define the relationship between the variables and their possible values.
Solving CSPs
Solving CSPs involves finding assignments of values to variables that satisfy all the given constraints. This can be done using various techniques, such as:
- Backtracking : This is a systematic search algorithm that explores the possible assignments of values to variables, backtracking when a conflict is encountered.
- Constraint propagation : This technique involves using the constraints to eliminate values from the domains of variables, reducing the search space.
- Constraint satisfaction algorithms : These algorithms use heuristics to guide the search for solutions, making it more efficient.
CSPs are used to solve a wide range of problems, such as scheduling, planning, resource allocation, and configuration. They provide a powerful framework for representing and solving problems in the field of artificial intelligence.
CSP Formulation
In the context of problem-solving agents, a CSP (Constraint Satisfaction Problem) is a formulation used to represent and solve problems in artificial intelligence. CSPs are widely used in various domains, such as scheduling, planning, and optimization.
In a CSP, the problem is represented as a set of variables, each with a domain of possible values, and a set of constraints that specify the relationships between variables. The goal is to find an assignment of values to variables that satisfies all the constraints.
Variables and Domains
Variables represent the unknowns in the problem, and each variable has a domain that defines the possible values it can take. For example, in a scheduling problem, the variables could represent tasks, and the domain of each variable could be the possible time slots for that task.
Constraints
Constraints define the relationships between variables. They specify the conditions that must be satisfied by the variable assignments. For example, in a scheduling problem, a constraint could be that two tasks cannot be scheduled at the same time.
Constraints can have different types, such as binary constraints that involve two variables, or unary constraints that involve only one variable. They can also have different degrees, such as constraints that involve more than two variables.
To solve a CSP, the agent searches for a consistent assignment of values to variables that satisfies all the constraints. This can be done using various search algorithms, such as backtracking or constraint propagation.
Let’s consider an example of a CSP formulation for a scheduling problem. We have three tasks to schedule, A, B, and C, and each task can be scheduled at two possible time slots, 1 and 2.
The variables are A, B, and C, and their domains are {1, 2}. The constraints are:
The goal is to find an assignment of values to variables that satisfies all the constraints. In this case, the valid assignments are:
- A = 1, B = 2, C = 1
- A = 2, B = 1, C = 2
These assignments satisfy all the constraints, as there are no conflicts between the tasks scheduled at the same time.
In conclusion, CSP formulation is a powerful technique used in problem-solving agents to represent and solve problems in artificial intelligence. It provides a flexible and efficient way to model and reason about complex problems.
Backtracking Algorithm
In the field of artificial intelligence, problem-solving agents are designed to find solutions to complex problems. One popular approach is the use of backtracking algorithms.
Backtracking is a systematic way of finding solutions by exploring all possible paths and discarding those that do not lead to a solution. It is often used when the problem can be represented as a search tree, where each node represents a partial solution and the edges represent possible choices.
The Backtracking Process
The backtracking algorithm starts by examining the first choice and moves forward along the path until a dead end is reached. At this point, it backtracks to the previous choice and explores the next option. This process continues until a solution is found or all possibilities have been exhausted.
During the backtracking process, the algorithm uses pruning techniques to optimize the search. Pruning involves eliminating portions of the search tree that are known to lead to dead ends, reducing the number of nodes that need to be explored.
Applications of Backtracking
The backtracking algorithm can be applied to a wide range of problem-solving tasks in artificial intelligence. Some common applications include:
- Constraint satisfaction problems: Backtracking can be used to find solutions that satisfy a set of constraints. For example, in a Sudoku puzzle, backtracking can be used to fill in the empty cells while ensuring that no number is repeated in a row, column, or block.
- Graph problems: Backtracking can be used to find paths in a graph that satisfy certain conditions. For example, in a maze, backtracking can be used to find a path from the starting point to the goal.
- Combinatorial optimization: Backtracking can be used to find the optimal solution among a set of possibilities. For example, in the traveling salesman problem, backtracking can be used to find the shortest possible route that visits all cities.
In summary, backtracking algorithms are a powerful tool for solving complex problems in artificial intelligence. They allow problem-solving agents to systematically explore all possible solutions and find the best one.
Forward Checking Algorithm
Forward Checking is an algorithm used in artificial intelligence to solve problems by systematically exploring the search space. It is particularly useful in constraint satisfaction problems where a set of constraints must be satisfied.
In the context of problem solving agents, the Forward Checking algorithm is used to efficiently eliminate values from the domains of variables during the search process. It works by propagating information about constraints from assigned variables to unassigned variables, reducing their domains and increasing the efficiency of the search.
The Forward Checking algorithm can be summarized in the following steps:
Step 1: Initialization
Initialize the domain of each variable with all possible values.
Step 2: Assign a Value
Select an unassigned variable and assign a value from its domain.
Step 3: Forward Checking
Update the domains of other unassigned variables based on the assigned value and the constraints.
In the table above, the domains of the variables are updated after assigning a value to one of the variables.
The Forward Checking algorithm continues by selecting the next unassigned variable with the smallest domain and repeating steps 2 and 3 until a solution is found or all variables have been assigned.
By efficiently propagating constraints, the Forward Checking algorithm can greatly reduce the search space and improve the efficiency of problem solving agents in artificial intelligence.
Constraint Propagation
Constraint propagation is one of the key techniques used by problem-solving agents in artificial intelligence. It is the process of using constraints to narrow down the search space and reduce the number of possible solutions.
In the context of artificial intelligence, a constraint represents a restriction or limitation on the values that certain variables can take. These constraints can be used to model real-world constraints or dependencies between variables. For example, in a scheduling problem, the constraint might state that two tasks cannot be scheduled at the same time.
Constraint propagation works by iteratively applying constraints to the problem domain and updating the values of variables based on the constraints. The goal is to eliminate values that are not consistent with the constraints, thus reducing the search space and making it easier to find a solution.
Types of Constraint Propagation
There are different types of constraint propagation techniques, including:
- Local Constraint Propagation: This technique applies constraints at a local level, focusing on individual variables or groups of variables. It updates the value of a variable based on the constraints without considering the global context of the problem.
- Global Constraint Propagation: This technique considers the global context of the problem and applies constraints across all variables simultaneously. It updates the values of variables based on the constraints and the implications of those constraints on other variables.
Constraint propagation can be a powerful technique for problem-solving agents as it allows them to prune the search space and focus on more promising solutions. It can help reduce the time and computational resources required to find a solution to a problem.
In conclusion, constraint propagation is a fundamental technique used by problem-solving agents in artificial intelligence. It leverages constraints to reduce the search space and find consistent values for variables. By applying constraints at a local or global level, agents can effectively narrow down the possibilities and improve the efficiency of their problem-solving process.
Local Search in CSPs
Local search is a common approach used in solving constraint satisfaction problems (CSPs) in artificial intelligence. CSPs involve finding solutions to a set of variables subject to a set of constraints. Local search algorithms focus on improving a current solution by making small modifications to it.
In the context of CSPs, local search algorithms explore the solution space by starting with an initial assignment of values to variables. They then iteratively improve this assignment by considering different neighborhoods of the current solution.
Local search algorithms aim to find the best possible assignment of values that satisfies all constraints in the problem. However, they do not guarantee finding the global optimal solution. Instead, they focus on finding a solution that is acceptable or meets certain criteria within a given time limit.
Tutorialspoint offers various tutorials and resources on local search algorithms in artificial intelligence. These tutorials provide in-depth explanations and implementations of different local search algorithms, such as hill climbing, simulated annealing, and genetic algorithms.
By learning and understanding local search algorithms, you can apply them to solve a wide range of problem-solving tasks in artificial intelligence. Whether it’s optimizing a complex scheduling problem or finding the best configuration for a system, local search algorithms provide practical solutions to real-world problems.
Tabu Search
Tabu Search is an artificial intelligence technique used for solving complex problem instances. It is a metaheuristic method that efficiently explores a problem space by keeping track of previously visited states, known as the tabu list, to avoid revisiting them. This allows the search algorithm to overcome local optima and find better solutions.
The main idea behind Tabu Search is to use memory to guide the search process. It maintains a short-term memory of recent moves and a long-term memory of the best solutions found so far. This allows the algorithm to make informed decisions and avoid getting stuck in suboptimal regions of the problem space.
During the search, Tabu Search uses different strategies to explore the problem space, such as generating and evaluating neighboring solutions, choosing the best move, and updating the tabu list. The tabu list contains forbidden moves that are temporarily avoided to prevent the search algorithm from going backward or cycling through the same solutions.
Tabu Search is particularly effective in solving optimization problems, combinatorial problems, and scheduling problems. It has been successfully applied in various domains, including operations research, computer science, engineering, and economics.
In conclusion, Tabu Search is a powerful technique used by problem-solving agents in artificial intelligence to tackle complex problem instances. It leverages memory to guide the search process and avoid revisiting previously explored states. By doing so, it is able to overcome local optima and find better solutions.+
Min-Conflict Algorithm
The Min-Conflict algorithm is a popular approach in the field of problem-solving intelligence. It is commonly used to solve constraint satisfaction problems.
This algorithm comes in handy when we need to find a solution that satisfies a set of constraints. It is especially useful when we encounter a problem where we have partial information or conflicting information.
The Min-Conflict algorithm works by iteratively adjusting the current solution to the problem until a feasible solution is found. It starts with an initial solution and then repeatedly selects a variable with a conflict and changes its value to minimize the number of conflicts. This process continues until either a solution with no conflicts is found or a predefined number of iterations is reached.
One of the advantages of the Min-Conflict algorithm is its ability to quickly find solutions to complex problems. It can handle large domains and a high number of constraints efficiently, making it a favored technique in artificial intelligence.
Implementing the Min-Conflict Algorithm
To implement the Min-Conflict algorithm, we need to follow these steps:
- Initialize the problem with an initial solution.
- While a solution with no conflicts is not found and the maximum number of iterations is not reached, repeat the following steps:
- Select a variable with conflicts.
- Choose a value for that variable that minimizes the number of conflicts.
- Update the assignments based on the new value.
- If a solution is found within the iterations limit, return it. Otherwise, return that no solution exists.
The Min-Conflict algorithm is a powerful approach for solving constraint satisfaction problems. Its iterative nature and ability to handle conflicts efficiently make it a preferred technique in artificial intelligence. By following a few simple steps, we can implement this algorithm to solve a wide range of complex problems.
Questions and answers
What are problem-solving agents in artificial intelligence.
Problem-solving agents in artificial intelligence are programs that are designed to find solutions to specific problems by searching through a set of possible actions and states.
How do problem-solving agents work?
Problem-solving agents work by starting with an initial state and applying a series of actions to reach a goal state. They use different search algorithms, such as depth-first search or breadth-first search, to explore the problem space and find the most optimal solution.
What are some examples of problem-solving agents?
Examples of problem-solving agents include route-planning systems, chess-playing programs, and automated theorem provers. These agents are designed to solve specific problems by searching for the best possible solution.
What are the advantages of problem-solving agents in artificial intelligence?
Problem-solving agents in artificial intelligence have several advantages. They can solve complex problems that would be difficult for humans to solve manually. They can also work quickly and efficiently, and they can explore a large number of possible solutions to find the best one.
What are some limitations of problem-solving agents in artificial intelligence?
Although problem-solving agents are powerful tools in artificial intelligence, they have some limitations. They require a well-defined problem and goal state, and they may not always find the optimal solution. Additionally, the search space can be very large, which can make finding a solution time-consuming or even infeasible.
Related posts:
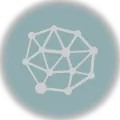
About the author
AI and Handyman: The Future is Here
Embrace ai-powered cdps: the future of customer engagement, elon musk’s vision ai, creating a powerful gpt telegram chatbot.
Towards Problem Solving Agents that Communicate and Learn
Anjali Narayan-Chen , Colin Graber , Mayukh Das , Md Rakibul Islam , Soham Dan , Sriraam Natarajan , Janardhan Rao Doppa , Julia Hockenmaier , Martha Palmer , Dan Roth
Export citation
- Preformatted
Markdown (Informal)
[Towards Problem Solving Agents that Communicate and Learn](https://aclanthology.org/W17-2812) (Narayan-Chen et al., RoboNLP 2017)
- Towards Problem Solving Agents that Communicate and Learn (Narayan-Chen et al., RoboNLP 2017)
- Anjali Narayan-Chen, Colin Graber, Mayukh Das, Md Rakibul Islam, Soham Dan, Sriraam Natarajan, Janardhan Rao Doppa, Julia Hockenmaier, Martha Palmer, and Dan Roth. 2017. Towards Problem Solving Agents that Communicate and Learn . In Proceedings of the First Workshop on Language Grounding for Robotics , pages 95–103, Vancouver, Canada. Association for Computational Linguistics.
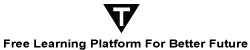
Artificial Intelligence
Control System
- Interview Q
Intelligent Agent
Problem-solving, adversarial search, knowledge represent, uncertain knowledge r., subsets of ai, artificial intelligence mcq, related tutorials.
- Send your Feedback to [email protected]
Help Others, Please Share

Learn Latest Tutorials

Transact-SQL

Reinforcement Learning

R Programming

React Native

Python Design Patterns

Python Pillow

Python Turtle

Preparation

Verbal Ability

Interview Questions

Company Questions
Trending Technologies

Cloud Computing

Data Science

Machine Learning

B.Tech / MCA

Data Structures

Operating System

Computer Network

Compiler Design

Computer Organization

Discrete Mathematics

Ethical Hacking

Computer Graphics

Software Engineering

Web Technology

Cyber Security

C Programming

Data Mining

Data Warehouse

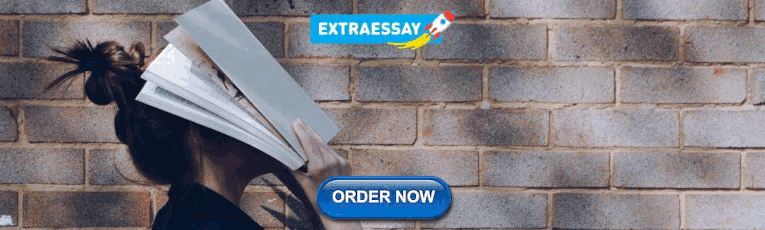
IMAGES
VIDEO
COMMENTS
The problem-solving agent performs precisely by defining problems and several solutions. So we can say that problem solving is a part of artificial intelligence that encompasses a number of techniques such as a tree, B-tree, heuristic algorithms to solve a problem. We can also say that a problem-solving agent is a result-driven agent and always ...
The problem solving agent follows this four phase problem solving process: Goal Formulation: This is the first and most basic phase in problem solving. It arranges specific steps to establish a target/goal that demands some activity to reach it. AI agents are now used to formulate goals. Problem Formulation: It is one of the fundamental steps ...
The problem solving agent chooses a cost function that reflects its own performance measure. The solution to the problem is an action sequence that leads from initial state to goal state and the ...
Problem-solving agents can vary greatly in complexity, from simple algorithms that solve straightforward puzzles to highly sophisticated AI systems that tackle complex, real-world problems. The design and implementation of problem-solving agents depend on the specific problem domain and the goals of the AI application.
Problem-Solving Agents goal formulation objectives that need to be achieved problem formulation actions and states to consider problem types single-/multiple state, contingency, exploration example problems toy problems real-world problems search strategies solution execution of the action sequence
Problem-solving agents are a type of artificial intelligence that helps automate problem-solving. They can be used to solve problems in natural language, algebra, calculus, statistics, and machine learning. There are three types of problem-solving agents: propositional, predicate, and automata. Propositional problem-solving agents can ...
Problem formulation ♦ Example problems ♦ Basic search algorithms Chapter 3 2 Problem-solving agents Restricted form of general agent: function Simple-Problem-Solving-Agent (percept) returns an action static: seq, an action sequence, initially empty state, some description of the current world state goal, a goal, initially null problem, a ...
Definition and Importance of Problem Solving Agents A problem solving agent is a type of artificial intelligence agent that is designed to identify and solve problems. These agents are programmed to analyze information, develop potential solutions, and select the best course of action to solve a given problem.
Starting from the definition of a rational agent, we will dive in into the concepts of reasoning, planning and solving problems, searching for solutions to them. One of the first issues that an AI practicioner has to face is to understand and define as clearly as possible the environment of its problem.
Chapter 3. Outline. Chapter3 1. Problem-solving agents. function Simple-Problem-Solving-Agent(percept) returns an action static: seq, an action sequence, initially empty state, some description of the current world state goal, a goal, initially null problem, a problem formulation. state←Update-State(state,percept)
How to define the problem to make it easier for finding solutions, and some basics on search algorithms in general. ... There are two kinds of goal-based agents: problem-solving agents and ...
Problem Solving and Search Problem Solving • Agent knows world dynamics • World state is finite, small enough to enumerate • World is deterministic • Utility for a sequence of states is a sum over path The utility for sequences of states is a sum over the path of the utilities of the individual states.
Problem-solving agents Restricted form of general agent: function Simple-Problem-Solving-Agent(percept) returns an action static: seq, an action sequence, initially empty state, some description of the current world state goal, a goal, initially null problem, a problem formulation state Update-State(state,percept) if seq is empty then
Problem Solving Agents and Uninformed Search An intelligent agents act to increase their performance measure. Some do this by adopting a goal. Four general steps in problem solving: Goal formulation - deciding on what the goal states are - based on current situation and agent's performance measure - What are the successful world states
A problem-solving agent is a key concept in the field of artificial intelligence (AI). It is an agent that can analyze a given problem and take appropriate actions to solve it. The agents are designed using a set of components that work together to achieve their goals.
We define an agent architecture for this scenario and present a series of experiments in the Blocks World domain that illustrate how our architecture supports language learning and problem solving in this domain. ... %0 Conference Proceedings %T Towards Problem Solving Agents that Communicate and Learn %A Narayan-Chen, Anjali %A Graber ...
After formulating a goal and a problem to solve, the agent calls a search procedure to solve it. It then uses the solution to guide its actions, doing whatever the solution recommends as 1 Notice that each of these "states" actually corresponds to a large set of world states, because a real world state specifies every aspect of reality.
Problem Solving Agents The remainder of the article will focus on a particular type of goal-based agent called a problem-solving agent . These agents use what is regarded as an atomic ...
Problem-solving agents are the goal-based agents and use atomic representation. In this topic, we will learn various problem-solving search algorithms. Search Algorithm Terminologies: Search: Searchingis a step by step procedure to solve a search-problem in a given search space. A search problem can have three main factors:
Key components of problem formulation include the initial state, possible actions, transition model describing how actions change the state, a goal test, and path cost function. Two examples of well-defined problems are given: the 8-puzzle problem and the 8-queens problem. Read more. Education. 1 of 10. Download now.
The problem-solving agent selects a cost function, which reflects its performance measure. Remember, an optimal solution has the lowest path cost among all the solutions.
Creative Problem Solving (CPS) is a sub-area within Artificial Intelligence (AI) that focuses on methods for solving off-nominal, or anomalous problems in autonomous systems. Despite many ...
Artificial intelligence (AI) problem-solving often involves investigating potential solutions to problems through reasoning techniques, making use of polynomial and differential equations, and carrying them out and use modelling frameworks. A same issue has a number of solutions, that are all accomplished using an unique algorithm.