A study on factors limiting online shopping behaviour of consumers
Rajagiri Management Journal
ISSN : 0972-9968
Article publication date: 4 March 2021
Issue publication date: 12 April 2021
This study aims to investigate consumer behaviour towards online shopping, which further examines various factors limiting consumers for online shopping behaviour. The purpose of the research was to find out the problems that consumers face during their shopping through online stores.
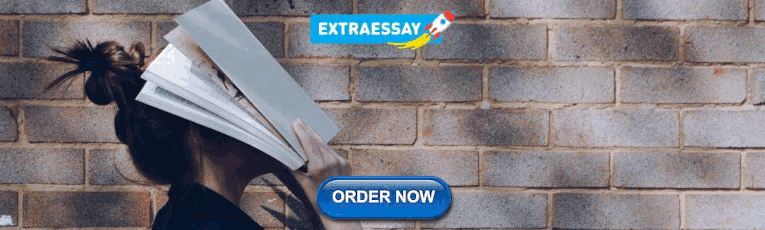
Design/methodology/approach
A quantitative research method was adopted for this research in which a survey was conducted among the users of online shopping sites.
As per the results total six factors came out from the study that restrains consumers to buy from online sites – fear of bank transaction and faith, traditional shopping more convenient than online shopping, reputation and services provided, experience, insecurity and insufficient product information and lack of trust.
Research limitations/implications
This study is beneficial for e-tailers involved in e-commerce activities that may be customer-to-customer or customer-to-the business. Managerial implications are suggested for improving marketing strategies for generating consumer trust in online shopping.
Originality/value
In contrast to previous research, this study aims to focus on identifying those factors that restrict consumers from online shopping.
- Online shopping
Daroch, B. , Nagrath, G. and Gupta, A. (2021), "A study on factors limiting online shopping behaviour of consumers", Rajagiri Management Journal , Vol. 15 No. 1, pp. 39-52. https://doi.org/10.1108/RAMJ-07-2020-0038
Emerald Publishing Limited
Copyright © 2020, Bindia Daroch, Gitika Nagrath and Ashutosh Gupta.
Published in Rajagiri Management Journal . Published by Emerald Publishing Limited. This article is published under the Creative Commons Attribution (CC BY 4.0) licence. Anyone may reproduce, distribute, translate and create derivative works of this article (for both commercial and non-commercial purposes), subject to full attribution to the original publication and authors. The full terms of this licence may be seen at http://creativecommons.org/licences/by/4.0/legalcode
Introduction
Today, people are living in the digital environment. Earlier, internet was used as the source for information sharing, but now life is somewhat impossible without it. Everything is linked with the World Wide Web, whether it is business, social interaction or shopping. Moreover, the changed lifestyle of individuals has changed their way of doing things from traditional to the digital way in which shopping is also being shifted to online shopping.
Online shopping is the process of purchasing goods directly from a seller without any intermediary, or it can be referred to as the activity of buying and selling goods over the internet. Online shopping deals provide the customer with a variety of products and services, wherein customers can compare them with deals of other intermediaries also and choose one of the best deals for them ( Sivanesan, 2017 ).
As per Statista-The Statistics Portal, the digital population worldwide as of April 2020 is almost 4.57 billion people who are active internet users, and 3.81 billion are social media users. In terms of internet usage, China, India and the USA are ahead of all other countries ( Clement, 2020 ).
The number of consumers buying online and the amount of time people spend online has risen ( Monsuwe et al. , 2004 ). It has become more popular among customers to buy online, as it is handier and time-saving ( Huseynov and Yildirim, 2016 ; Mittal, 2013 ). Convenience, fun and quickness are the prominent factors that have increased the consumer’s interest in online shopping ( Lennon et al. , 2008 ). Moreover, busy lifestyles and long working hours also make online shopping a convenient and time-saving solution over traditional shopping. Consumers have the comfort of shopping from home, reduced traveling time and cost and easy payment ( Akroush and Al-Debei, 2015 ). Furthermore, price comparisons can be easily done while shopping through online mode ( Aziz and Wahid, 2018 ; Martin et al. , 2015 ). According to another study, the main influencing factors for online shopping are availability, low prices, promotions, comparisons, customer service, user friendly, time and variety to choose from ( Jadhav and Khanna, 2016 ). Moreover, website design and features also encourage shoppers to shop on a particular website that excite them to make the purchase.
Online retailers have started giving plenty of offers that have increased the online traffic to much extent. Regularly online giants like Amazon, Flipkart, AliExpress, etc. are advertising huge discounts and offers that are luring a large number of customers to shop from their websites. Companies like Nykaa, MakeMyTrip, Snapdeal, Jabong, etc. are offering attractive promotional deals that are enticing the customers.
Despite so many advantages, some customers may feel online shopping risky and not trustworthy. The research proposed that there is a strong relationship between trust and loyalty, and most often, customers trust brands far more than a retailer selling that brand ( Bilgihan, 2016 ; Chaturvedi et al. , 2016 ). In the case of online shopping, there is no face-to-face interaction between seller and buyer, which makes it non-socialize, and the buyer is sometimes unable to develop the trust ( George et al. , 2015 ). Trust in the e-commerce retailer is crucial to convert potential customer to actual customer. However, the internet provides unlimited products and services, but along with those unlimited services, there is perceived risk in digital shopping such as mobile application shopping, catalogue or mail order ( Tsiakis, 2012 ; Forsythe et al. , 2006 ; Aziz and Wahid, 2018 ).
Literature review
A marketer has to look for different approaches to sell their products and in the current scenario, e-commerce has become the popular way of selling the goods. Whether it is durable or non-durable, everything is available from A to Z on websites. Some websites are specifically designed for specific product categories only, and some are selling everything.
The prominent factors like detailed information, comfort and relaxed shopping, less time consumption and easy price comparison influence consumers towards online shopping ( Agift et al. , 2014 ). Furthermore, factors like variety, quick service and discounted prices, feedback from previous customers make customers prefer online shopping over traditional shopping ( Jayasubramanian et al. , 2015 ). It is more preferred by youth, as during festival and holiday season online retailers give ample offers and discounts, which increases the online traffic to a great extent ( Karthikeyan, 2016 ). Moreover, services like free shipping, cash on delivery, exchange and returns are also luring customers towards online purchases.
More and more people are preferring online shopping over traditional shopping because of their ease and comfort. A customer may have both positive and negative experiences while using an online medium for their purchase. Some of the past studies have shown that although there are so many benefits still some customers do not prefer online as their basic medium of shopping.
While making online purchase, customers cannot see, touch, feel, smell or try the products that they want to purchase ( Katawetawaraks and Wang, 2011 ; Al-Debei et al. , 2015 ), due to which product is difficult to examine, and it becomes hard for customers to make purchase decision. In addition, some products are required to be tried like apparels and shoes, but in case of online shopping, it is not possible to examine and feel the goods and assess its quality before making a purchase due to which customers are hesitant to buy ( Katawetawaraks and Wang, 2011 ; Comegys et al. , 2009 ). Alam and Elaasi (2016) in their study found product quality is the main factor, which worries consumer to make online purchase. Moreover, some customers have reported fake products and imitated items in their delivered orders ( Jun and Jaafar, 2011 ). A low quality of merchandise never generates consumer trust on online vendor. A consumer’s lack of trust on the online vendor is the most common reason to avoid e-commerce transactions ( Lee and Turban, 2001 ). Fear of online theft and non-reliability is another reason to escape from online shopping ( Karthikeyan, 2016 ). Likewise, there is a risk of incorrect information on the website, which may lead to a wrong purchase, or in some cases, the information is incomplete for the customer to make a purchase decision ( Liu and Guo, 2008 ). Moreover, in some cases, the return and exchange policies are also not clear on the website. According to Wei et al. (2010) , the reliability and credibility of e-retailer have direct impact on consumer decision with regards to online shopping.
Limbu et al. (2011) revealed that when it comes to online retailers, some websites provide very little information about their companies and sellers, due to which consumers feel insecure to purchase from these sites. According to other research, consumers are hesitant, due to scams and feel anxious to share their personal information with online vendors ( Miyazaki and Fernandez, 2001 ; Limbu et al. , 2011 ). Online buyers expect websites to provide secure payment and maintain privacy. Consumers avoid online purchases because of the various risks involved with it and do not find internet shopping secured ( Cheung and Lee, 2003 ; George et al. , 2015 ; Banerjee et al. , 2010 ). Consumers perceive the internet as an unsecured channel to share their personal information like emails, phone and mailing address, debit card or credit card numbers, etc. because of the possibility of misuse of that information by other vendors or any other person ( Lim and Yazdanifard, 2014 ; Kumar, 2016 ; Alam and Yasin, 2010 ; Nazir et al. , 2012 ). Some sites make it vital and important to share personal details of shoppers before shopping, due to which people abandon their shopping carts (Yazdanifard and Godwin, 2011). About 75% of online shoppers leave their shopping carts before they make their final decision to purchase or sometimes just before making the payments ( Cho et al. , 2006 ; Gong et al. , 2013 ).
Moreover, some of the customers who have used online shopping confronted with issues like damaged products and fake deliveries, delivery problems or products not received ( Karthikeyan, 2016 ; Kuriachan, 2014 ). Sometimes consumers face problems while making the return or exchange the product that they have purchased from online vendors ( Liang and Lai, 2002 ), as some sites gave an option of picking from where it was delivered, but some online retailers do not give such services to consumer and consumer him/herself has to courier the product for return or exchange, which becomes inopportune. Furthermore, shoppers had also faced issues with unnecessary delays ( Muthumani et al. , 2017 ). Sometimes, slow websites, improper navigations or fear of viruses may drop the customer’s willingness to purchase from online stores ( Katawetawaraks and Wang, 2011 ). As per an empirical study done by Liang and Lai (2002) , design of the e-store or website navigation has an impact on the purchase decision of the consumer. An online shopping experience that a consumer may have and consumer skills that consumers may use while purchasing such as website knowledge, product knowledge or functioning of online shopping influences consumer behaviour ( Laudon and Traver, 2009 ).
From the various findings and viewpoints of the previous researchers, the present study identifies the complications online shoppers face during online transactions, as shown in Figure 1 . Consumers do not have faith, and there is lack of confidence on online retailers due to incomplete information on website related to product and service, which they wish to purchase. Buyers are hesitant due to fear of online theft of their personal and financial information, which makes them feel there will be insecure transaction and uncertain errors may occur while making online payment. Some shoppers are reluctant due to the little internet knowledge. Furthermore, as per the study done by Nikhashem et al. (2011), consumers unwilling to use internet for their shopping prefer traditional mode of shopping, as it gives roaming experience and involves outgoing activity.
Several studies have been conducted earlier that identify the factors influencing consumer towards online shopping but few have concluded the factors that restricts the consumers from online shopping. The current study is concerned with the factors that may lead to hesitation by the customer to purchase from e-retailers. This knowledge will be useful for online retailers to develop customer driven strategies and to add more value product and services and further will change their ways of promoting and advertising the goods and enhance services for customers.
Research methodology
This study aimed to find out the problems that are generally faced by a customer during online purchase and the relevant factors due to which customers do not prefer online shopping. Descriptive research design has been used for the study. Descriptive research studies are those that are concerned with describing the characteristics of a particular individual or group. This study targets the population drawn from customers who have purchased from online stores. Most of the respondents participated were post graduate students and and educators. The total population size was indefinite and the sample size used for the study was 158. A total of 170 questionnaires were distributed among various online users, out of which 12 questionnaires were received with incomplete responses and were excluded from the analysis. The respondents were selected based on the convenient sampling technique. The primary data were collected from Surveys with the help of self-administered questionnaires. The close-ended questionnaire was used for data collection so as to reduce the non-response rate and errors. The questionnaire consists of two different sections, in which the first section consists of the introductory questions that gives the details of socio-economic profile of the consumers as well as their behaviour towards usage of internet, time spent on the Web, shopping sites preferred while making the purchase, and the second section consist of the questions related to the research question. To investigate the factors restraining consumer purchase, five-point Likert scale with response ranges from “Strongly agree” to “Strongly disagree”, with following equivalencies, “strongly disagree” = 1, “disagree” = 2, “neutral” = 3, “agree” = 4 and “strongly agree” = 5 was used in the questionnaire with total of 28 items. After collecting the data, it was manually recorded on the Excel sheet. For analysis socio-economic profile descriptive statistics was used and factors analysis was performed on SPSS for factor reduction.
Data analysis and interpretation
The primary data collected from the questionnaires was completely quantified and analysed by using Statistical Package for Social Science (SPSS) version 20. This statistical program enables accuracy and makes it relatively easy to interpret data. A descriptive and inferential analysis was performed. Table 1 represents the results of socio-economic status of the respondents along with some introductory questions related to usage of internet, shopping sites used by the respondents, amount of money spent by the respondents and products mostly purchased through online shopping sites.
According to the results, most (68.4%) of the respondents were belonging to the age between 21 and 30 years followed by respondents who were below the age of 20 years (16.4%) and the elderly people above 50 were very few (2.6%) only. Most of the respondents who participated in the study were females (65.8)% who shop online as compared to males (34.2%). The respondents who participated in the study were students (71.5%), and some of them were private as well as government employees. As per the results, most (50.5%) of the people having income below INR15,000 per month who spend on e-commerce websites. The results also showed that most of the respondents (30.9%) spent less than 5 h per week on internet, but up to (30.3%) spend 6–10 h per week on internet either on online shopping or social media. Majority (97.5%) of them have shopped through online websites and had both positive and negative experiences, whereas 38% of the people shopped 2–5 times and 36.7% shopped more than ten times. Very few people (12%), shopped only once. Most of the respondents spent between INR1,000–INR5,000 for online shopping, and few have spent more than INR5,000 also.
As per the results, the most visited online shopping sites was amazon.com (71.5%), followed by flipkart.com (53.2%). Few respondents have also visited other e-commerce sites like eBay, makemytrip.com and myntra.com. Most (46.2%) of the time people purchase apparels followed by electronics and daily need items from the ecommerce platform. Some of the respondents have purchased books as well as cosmetics, and some were preferring online sites for travel tickets, movie tickets, hotel bookings and payments also.
Factor analysis
To explore the factors that restrict consumers from using e-commerce websites factor analysis was done, as shown in Table 3 . A total of 28 items were used to find out the factors that may restrain consumers to buy from online shopping sites, and the results were six factors. The Kaiser–Meyer–Olkin (KMO) measure, as shown in Table 2 , in this study was 0.862 (>0.60), which states that values are adequate, and factor analysis can be proceeded. The Bartlett’s test of sphericity is related to the significance of the study and the significant value is 0.000 (<0.05) as shown in Table 2 .
The analysis produced six factors with eigenvalue more than 1, and factor loadings that exceeded 0.30. Moreover, reliability test of the scale was performed through Cronbach’s α test. The range of Cronbach’s α test came out to be between 0.747 and 0.825, as shown in Table 3 , which means ( α > 0.7) the high level of internal consistency of the items used in survey ( Table 4 ).
Factor 1 – The results revealed that the “fear of bank transaction and faith” was the most significant factor, with 29.431% of the total variance and higher eigenvalue, i.e. 8.241. The six statements loaded on Factor 1 highly correlate with each other. The analysis shows that some people do not prefer online shopping because they are scared to pay online through credit or debit cards, and they do not have faith over online vendors.
Factor 2 – “Traditional shopping is convenient than online shopping” has emerged as a second factor which explicates 9.958% of total variance. It has five statements and clearly specifies that most of the people prefer traditional shopping than online shopping because online shopping is complex and time-consuming.
Factor 3 – Third crucial factor emerged in the factor analysis was “reputation and service provided”. It was found that 7.013% of variations described for the factor. Five statements have been found on this factor, all of which were interlinked. It clearly depicts that people only buy from reputed online stores after comparing prices and who provide guarantee or warrantee on goods.
Factor 4 – “Experience” was another vital factor, with 4.640% of the total variance. It has three statements that clearly specifies that people do not go for online shopping due to lack of knowledge and their past experience was not good and some online stores do not provide EMI facilities.
Factor 5 – Fifth important factor arisen in the factor analysis was “Insecurity and Insufficient Product Information” with 4.251% of the total variance, and it has laden five statements, which were closely intertwined. This factor explored that online shopping is not secure as traditional shopping. The information of products provided on online stores is not sufficient to make the buying decision.
Factor 6 – “Lack of trust” occurred as the last factor of the study, which clarifies 3.920% of the total variance. It has four statements that clearly state that some people hesitate to give their personal information, as they believe online shopping is risky than traditional shopping. Without touching the product, people hesitate to shop from online stores.
The study aimed to determine the problems faced by consumers during online purchase. The result showed that most of the respondents have both positive and negative experience while shopping online. There were many problems or issues that consumer’s face while using e-commerce platform. Total six factors came out from the study that limits consumers to buy from online sites like fear of bank transaction and no faith, traditional shopping more convenient than online shopping, reputation and services provided, experience, insecurity and insufficient product information and lack of trust.
The research might be useful for the e-tailers to plan out future strategies so as to serve customer as per their needs and generate customer loyalty. As per the investigation done by Casalo et al. (2008) , there is strong relationship between reputation and satisfaction, which further is linked to customer loyalty. If the online retailer has built his brand name, or image of the company, the customer is more likely to prefer that retailer as compared to new entrant. The online retailer that seeks less information from customers are more preferred as compared to those require complete personal information ( Lawler, 2003 ).
Online retailers can adopt various strategies to persuade those who hesitate to shop online such that retailer need to find those negative aspects to solve the problems of customers so that non-online shopper or irregular online consumer may become regular customer. An online vendor has to pay attention to product quality, variety, design and brands they are offering. Firstly, the retailer must enhance product quality so as to generate consumer trust. For this, they can provide complete seller information and history of the seller, which will preferably enhance consumer trust towards that seller.
Furthermore, they can adopt marketing strategies such as user-friendly and secure website, which can enhance customers’ shopping experience and easy product search and proper navigation system on website. Moreover, complete product and service information such as feature and usage information, description and dimensions of items can help consumer decide which product to purchase. The experience can be enhanced by adding more pictures, product videos and three-dimensional (3D), images which will further help consumer in the decision-making process. Moreover, user-friendly payment systems like cash on deliveries, return and exchange facilities as per customer needs, fast and speedy deliveries, etc. ( Chaturvedi et al. , 2016 ; Muthumani et al. , 2017 ) will also enhance the probability of purchase from e-commerce platform. Customers are concerned about not sharing their financial details on any website ( Roman, 2007 ; Limbu et al. , 2011 ). Online retailers can ensure payment security by offering numerous payment options such as cash on delivery, delivery after inspection, Google Pay or Paytm or other payment gateways, etc. so as to increase consumer trust towards website, and customer will not hesitate for financial transaction during shopping. Customers can trust any website depending upon its privacy policy, so retailers can provide customers with transparent security policy, privacy policy and secure transaction server so that customers will not feel anxious while making online payments ( Pan and Zinkhan, 2006 ). Moreover, customers not only purchase basic goods from the online stores but also heed augmented level of goods. Therefore, if vendors can provide quick and necessary support, answer all their queries within 24-hour service availability, customers may find it convenient to buy from those websites ( Martin et al. , 2015 ). Sellers must ensure to provide products and services that are suitable for internet. Retailers can consider risk lessening strategies such as easy return and exchange policies to influence consumers ( Bianchi and Andrews, 2012 ). Furthermore, sellers can offer after-sales services as given by traditional shoppers to attract more customers and generate unique shopping experience.
Although nowadays, most of the vendors do give plenty of offers in form of discounts, gifts and cashbacks, but most of them are as per the needs of e-retailers and not customers. Beside this, trust needs to be generated in the customer’s mind, which can be done by modifying privacy and security policies. By adopting such practices, the marketer can generate customers’ interest towards online shopping.
Conceptual framework of the study
Socioeconomic status of respondents
KMO and Bartlett’s test
Cronbach’s α
Agift , A. , Rekha , V. and Nisha , C. ( 2014 ), “ Consumers attitude towards online shopping ”, Research Journal of Family, Community and Consumer Sciences , Vol. 2 No. 8 , pp. 4 - 7 , available at: www.isca.in/FAMILY_SCI/Archive/v2/i8/2.ISCA-RJFCCS-2014-017.php
Akroush , M.N. and Al-Debei , M.M. ( 2015 ), “ An integrated model of factors affecting consumer attitudes towards online shopping ”, Business Process Management Journal , Vol. 21 No. 6 , pp. 1353 - 1376 , doi: 10.1108/BPMJ-02-2015-0022 .
Alam , M.Z. and Elaasi , S. ( 2016 ), “ A study on consumer perception towards e-shopping in KSA ”, International Journal of Business and Management , Vol. 11 No. 7 , p. 202 .
Alam , S. and Yasin , N.M. ( 2010 ), “ What factors influence online brand trust: evidence from online tickets buyers in Malaysia ”, Journal of Theoretical and Applied Electronic Commerce Research , Vol. 5 No. 3 , pp. 78 - 89 , doi: 10.4067/S0718-18762010000300008 .
Al-Debei , M.M. , Akroush , M.N. and Ashouri , M.I. ( 2015 ), “ Consumer attitudes towards online shopping: the effects of trust, perceived benefits, and perceived web quality ”, Internet Research , Vol. 25 No. 5 , pp. 707 - 733 , doi: 10.1108/IntR-05-2014-0146 .
Aziz , N.N.A. and Wahid , N.A. ( 2018 ), “ Factors influencing online purchase intention among university students ”, International Journal of Academic Research in Business and Social Sciences , Vol. 8 No. 7 , pp. 702 - 717 , doi: 10.6007/IJARBSS/v8-i7/4413 .
Banerjee , N. , Dutta , A. and Dasgupta , T. ( 2010 ), “ A study on customers’ attitude towards online shopping-An Indian perspective ”, Indian Journal of Marketing , Vol. 40 No. 11 , pp. 36 - 42 .
Bianchi , C. and Andrews , L. ( 2012 ), “ Risk, trust, and consumer online purchasing behaviour: a Chilean perspective ”, International Marketing Review , Vol. 29 No. 3 , pp. 253 - 275 , doi: 10.1108/02651331211229750 .
Bilgihan , A. ( 2016 ), “ Gen Y customer loyalty in online shopping: an integrated model of trust, user experience and branding ”, Computers in Human Behavior , Vol. 61 , pp. 103 - 113 , doi: 10.1016/j.chb.2016.03.014 .
Casalo , L. , Flavián , C. and Guinalíu , M. ( 2008 ), “ The role of perceived usability, reputation, satisfaction and consumer familiarity on the website loyalty formation process ”, Computers in Human Behavior , Vol. 24 No. 2 , pp. 325 - 345 , doi: 10.1016/j.chb.2007.01.017 .
Chaturvedi , D. , Gupta , D. and Singh Hada , D. ( 2016 ), “ Perceived risk, trust and information seeking behavior as antecedents of online apparel buying behavior in India: an exploratory study in context of Rajasthan ”, International Review of Management and Marketing , Vol. 6 No. 4 , pp. 935 - 943 , doi: 10.2139/ssrn.3204971 .
Cheung , C.M. and Lee , M.K. ( 2003 ), “ An integrative model of consumer trust in internet shopping ”, ECIS 2003 Proceedings , p. 48 .
Cho , C.H. , Kang , J. and Cheon , H.J. ( 2006 ), “ Online shopping hesitation ”, Cyberpsychology and Behavior , Vol. 9 No. 3 , pp. 261 - 274 , doi: 10.1089/cpb.2006.9.261 .
Clement , J. ( 2020 ), “ Worldwide digital population as of April 2020 ”, available at: www.statista.com/statistics/617136/digital-population-worldwide/ ( accessed 18 June 2020 ).
Comegys , C. , Hannula , M. and Váisánen , J. ( 2009 ), “ Effects of consumer trust and risk on online purchase decision-making: a comparison of Finnish and United States students ”, International Journal of Management , Vol. 26 No. 2 , available at: www.questia.com/library/journal/1P3-1874986651/effects-of-consumer-trust-and-risk-on-online-purchase
Forsythe , S. , Liu , C. , Shannon , D. and Gardner , L.C. ( 2006 ), “ Development of a scale to measure the perceived benefits and risks of online shopping ”, Journal of Interactive Marketing , Vol. 20 No. 2 , pp. 55 - 75 , doi: 10.1002/dir.20061 .
George , O.J. , Ogunkoya , O.A. , Lasisi , J.O. and Elumah , L.O. ( 2015 ), “ Risk and trust in online shopping: experience from Nigeria ”, International Journal of African and Asian Studies , Vol. 11 , pp. 71 - 78 , available at: https://iiste.org/Journals/index.php/JAAS/article/view/23937
Gong , W. , Stump , R.L. and Maddox , L.M. ( 2013 ), “ Factors influencing consumers’ online shopping in China ”, Journal of Asia Business Studies , Vol. 7 No. 3 , pp. 214 - 230 , doi: 10.1108/JABS-02-2013-0006 .
Huseynov , F. and Yildirim , S.O. ( 2016 ), “ Internet users’ attitudes toward business-to-consumer online shopping: a survey ”, Information Development , Vol. 32 No. 3 , pp. 452 - 465 , doi: 10.1177/0266666914554812 .
Jadhav , V. and Khanna , M. ( 2016 ), “ Factors influencing online buying behavior of college students: a qualitative analysis ”, The Qualitative Report , Vol. 21 No. 1 , pp. 1 - 15 , available at: https://nsuworks.nova.edu/tqr/vol21/iss1/1
Jayasubramanian , P. , Sivasakthi , D. and Ananthi , P.K. ( 2015 ), “ A study on customer satisfaction towards online shopping ”, International Journal of Applied Research , Vol. 1 No. 8 , pp. 489 - 495 , available at: www.academia.edu/download/54009715/1-7-136.pdf
Jun , G. and Jaafar , N.I. ( 2011 ), “ A study on consumers’ attitude towards online shopping in China ”, International Journal of Business and Social Science , Vol. 2 No. 22 , pp. 122 - 132 .
Karthikeyan ( 2016 ), “ Problems faced by online customers ”, International Journal of Current Research and Modern Education (IJCRME) , Vol. 1 No. 1 , pp. 166 - 169 , available at: http://ijcrme.rdmodernresearch.com/wp-content/uploads/2015/06/23.pdf
Katawetawaraks , C. and Wang , C.L. ( 2011 ), “ Online shopper behavior: influences of online shopping decision ”, Asian Journal of Business Research , Vol. 1 No. 2 , pp. 66 - 74 , available at: https://ssrn.com/abstract=2345198
Kumar , M. ( 2016 ), “ Consumer behavior and satisfaction in e-commerce: a comparative study based on online shopping of some electronic gadgets ”, International Journal of Research in Commerce and Management , Vol. 7 No. 7 , pp. 62 - 67 , available at: https://ijrcm.org.in/article_info.php?article_id=6785
Kuriachan , J.K. ( 2014 ), “ Online shopping problems and solutions ”, New Media and Mass Communication , Vol. 23 No. 1 , pp. 1 - 4 , available at: www.academia.edu/download/34229456/Online_shopping_problems_and_solutions
Laudon , K.C. and Traver , C.G. ( 2009 ), E-Commerce Business. Technology. Society , 5th ed ., Prentice Hall .
Lawler , J.P. ( 2003 ), “ Customer loyalty and privacy on the web ”, Journal of Internet Commerce , Vol. 2 No. 1 , pp. 89 - 105 , doi: 10.1300/J179v02n01_07 .
Lee , M.K. and Turban , E. ( 2001 ), “ A trust model for consumer internet shopping ”, International Journal of Electronic Commerce , Vol. 6 No. 1 , pp. 75 - 91 , doi: 10.1080/10864415.2001.11044227 .
Lennon , S.J. , et al. ( 2008 ), “ Rural consumers’ online shopping for food and fiber products as a form of outshopping ”, Clothing and Textiles Research Journal , Vol. 27 No. 1 , pp. 3 - 30 , doi: 10.1177/0887302X07313625 .
Liang , T.P. and Lai , H.J. ( 2002 ), “ Effect of store design on consumer purchases: an empirical study of on-line bookstores ”, Information and Management , Vol. 39 No. 6 , pp. 431 - 444 , doi: 10.1016/S0378-7206(01)00129-X .
Lim , P.L. and Yazdanifard , R. ( 2014 ), “ Does gender play a role in online consumer behavior? ”, Global Journal of Management and Business Research , Vol. 14 No. 7 , pp. 48 - 56 , available at: https://journalofbusiness.org/index.php/GJMBR/article/view/1570
Limbu , Y.B. , Wolf , M. and Lunsford , D.L. ( 2011 ), “ Consumers’ perceptions of online ethics and its effects on satisfaction and loyalty ”, Journal of Research in Interactive Marketing , Vol. 5 No. 1 , pp. 71 - 89 , doi: 10.1108/17505931111121534 .
Liu , C. and Guo , Y. ( 2008 ), “ Validating the end-user computing satisfaction instrument for online shopping systems ”, Journal of Organizational and End User Computing , Vol. 20 No. 4 , pp. 74 - 96 , available at: www.igi-global.com/article/journal-organizational-end-user-computing/3849
Martin , J. , Mortimer , G. and Andrews , L. ( 2015 ), “ Re-examining online customer experience to include purchase frequency and perceived risk ”, Journal of Retailing and Consumer Services , Vol. 25 , pp. 81 - 95 , doi: 10.1016/j.jretconser.2015.03.008 .
Mittal , A. ( 2013 ), “ E-commerce: it’s impact on consumer behavior ”, Global Journal of Management and Business Studies , Vol. 3 No. 2 , pp. 131 - 138 , available at: www.ripublication.com/gjmbs_spl/gjmbsv3n2spl_09.pdf
Miyazaki , A.D. and Fernandez , A. ( 2001 ), “ Consumer perceptions of privacy and security risks for online shopping ”, Journal of Consumer Affairs , Vol. 35 No. 1 , pp. 27 - 44 , doi: 10.1111/j.1745-6606.2001.tb00101.x .
Monsuwe , T.P.Y. , Dellaert , B.G.C. and Ruyter , K.D. ( 2004 ), “ What drives consumers to shop online? A literature review ”, International Journal of Service Industry Management , Vol. 15 No. 1 , pp. 102 - 121 , doi: 10.1108/09564230410523358 .
Muthumani , A. , Lavanya , V. and Mahalakshmi , R. ( 2017 ), “ Problems faced by customers on online shopping in Virudhunagar district ”, International Journal of Science Technology and Management (IJSTM) , Vol. 6 No. 2 , pp. 152 - 159 , available at: www.ijstm.com/images/short_pdf/1486214600_S184_IJSTM.pdf .
Nazir , S. , Tayyab , A. , Sajid , A. , Ur Rashid , H. and Javed , I. ( 2012 ), “ How online shopping is affecting consumers buying behavior in Pakistan? ”, International Journal of Computer Science Issues (IJCSI) , Vol. 9 No. 3 , p. 486 .
Nikhashem , S.R. , Yasmin , F. , Haque , A. and Khatibi , A. ( 2011 ), “ Study on customer perception towards online-ticketing in Malaysia ”, In Proceedings For 2011 International Research Conference and Colloquium , Vol. 1 , No. 1 , pp. 320 - 338 .
Pan , Y. and Zinkhan , G.M. ( 2006 ), “ Exploring the impact of online privacy disclosures on consumer trust ”, Journal of Retailing , Vol. 82 No. 4 , pp. 331 - 338 , doi: 10.1016/j.jretai.2006.08.006 .
Roman , S. ( 2007 ), “ The ethics of online retailing: a scale development and validation from the consumers’ perspective ”, Journal of Business Ethics , Vol. 72 No. 2 , pp. 131 - 148 , doi: 10.1007/s10551-006-9161-y .
Sivanesan ( 2017 ), “ A study on problems faced by customers in online shopping with special reference to Kanyakumari district ”, International Journal of Research in Management and Business Studies , Vol. 4 No. 3 , pp. 22 - 25 , available at: http://ijrmbs.com/vol4issue3SPL1/sivanesan.pdf
Tsiakis , T. ( 2012 ), “ Consumers’ issues and concerns of perceived risk of information security in online framework. The marketing strategies ”, Procedia – Social and Behavioral Sciences , Vol. 62 No. 24 , pp. 1265 - 1270 , doi: 10.1016/j.sbspro.2012.09.216 .
Wei , L.H. , Osman , M.A. , Zakaria , N. and Bo , T. ( 2010 ), “ Adoption of e-commerce online shopping in Malaysia ”, In 2010 IEEE 7th International Conference on E-Business Engineering , IEEE , pp. 140 - 143 .
Yazdanifard , R. and Godwin , N.W. ( 2011 ), “ Challenges faced by customers: Highlighting E-shopping problems ”, Paper presented at international Conference on Economics, Business and Marketing Management (CEBMM 2011) , Shanghai, China , available at: http://www.researchgate.net/profile/Assc_Prof_Dr_Rashad_Yazdanifard/publication/268507745_Challenges_faced_by_customers_Highlighting_E-shopping_problems/links/546d4ade0cf26e95bc3cb0a1/Challenges-faced-by-customers-Highlighting-E-shopping-problems.pdf ( accessed 20 March 2020 ).
Further reading
Grabner-Kräuter , S. and Kaluscha , E.A. ( 2003 ), “ Empirical research in on-line trust: a review and critical assessment ”, International Journal of Human-Computer Studies , Vol. 58 No. 6 , pp. 783 - 812 .
Nurfajrinah , M.A. , Nurhadi , Z.F. and Ramdhani , M.A. ( 2017 ), “ Meaning of online shopping for indie model ”, The Social Sciences , Vol. 12 No. 4 , pp. 737 - 742 , available at: https://medwelljournals.com/abstract/?doi=sscience.2017.737.742
Corresponding author
Related articles, we’re listening — tell us what you think, something didn’t work….
Report bugs here
All feedback is valuable
Please share your general feedback
Join us on our journey
Platform update page.
Visit emeraldpublishing.com/platformupdate to discover the latest news and updates
Questions & More Information
Answers to the most commonly asked questions here
Adoption of Online Grocery Shopping: A Systematic Review of the Literature
- Conference paper
- First Online: 30 May 2024
- Cite this conference paper
- Kirti Prashar ORCID: orcid.org/0000-0001-7439-7846 9 , 10 &
- Anil Kalotra 9
Part of the book series: Communications in Computer and Information Science ((CCIS,volume 2051))
Included in the following conference series:
- International Conference on Applied Technologies
This article provides an SLR, or systematic review of the field of study known as “e-grocery adoption.’’ It suggests using grocery apps or online buying groceries to enhance marketing research. The purpose of this study is to critically revise and produce an essay about the adoption of grocery applications. 38 studies were produced by the SLR and presented concurrently to the adoption of grocery apps. Results from ahead descriptive analytics show the most important research questions around the adoption of grocery applications as well as diverse strategies that link researchers and participants in various research methods. The essay looks into the factors that led to the acceptance of grocery applications. The writers emphasized how the subject has evolved over time and noted the prospective potential future research topics. The study eventually provides references for further research on adoption of grocery applications.
This is a preview of subscription content, log in via an institution to check access.
Access this chapter
- Available as PDF
- Read on any device
- Instant download
- Own it forever
- Available as EPUB and PDF
- Compact, lightweight edition
- Dispatched in 3 to 5 business days
- Free shipping worldwide - see info
Tax calculation will be finalised at checkout
Purchases are for personal use only
Institutional subscriptions
Jain, N.K., Gajjar, H., Shah, B.J., Sadh, A.: E-fulfillment dimensions and its influence on customers in e-tailing: a critical review. Asia Pacific J. Mark. Logist. 29 (2), 347–369 (2017)
Article Google Scholar
Melacini, M., Perotti, S., Rasini, M., Tappia, E.: E-fulfilment and distribution in omni-channel retailing: a systematic literature review. Int. J. Phys. Distrib. Logist. Manag. 48 (4), 391–414 (2018)
Tranfield, D., Denyer, D., Smart, P.: Towards a methodology for developing evidenceinformed management knowledge by means of systematic review. Br. J. Manag. 14 (3), 207–222 (2003)
Kitchenham, B., Charters, S.: Guidelines for performing systematic literature reviews in software engineering (2007)
Google Scholar
Okoli, C., Schabram, K.: A guide to conducting a systematic literature review of information systems research (2010)
Singh, R., Rosengren, S.: Why do online grocery shoppers switch? an empirical investigation of drivers of switching in online grocery. J. Retail. Consum. Serv. 53 , 101962 (2020)
Mkansi, M., Nsakanda, A.L.: Leveraging the physical network of stores in e-grocery order fulfilment for sustainable competitive advantage. Res. Transp. Econ. 87 , 100786 (2019)
Cagliano, A.C., De Marco, A., Rafele, C.: E-grocery supply chain management enabled by mobile tools. Bus. Process. Manag. J. 23 (1), 47–70 (2017)
Ajzen, I.: Theory of planned behavior. Acad. Press. Inc. All 50 , 179–211 (1991)
Kureshi, S., Thomas, S.: Online grocery retailing – exploring local grocers beliefs. Int. J. Retail Distrib. Manag. 47 (2), 157–185 (2019)
Saskia, S., Mareï, N., Blanquart, C.: Innovations in e-grocery and logistics solutions for cities. Transp. Res. Procedia 12 , 825–835 (2016)
Wollenburg, J., Hübner, A., Kuhn, H., Trautrims, A.: From bricksand-mortar to bricks-and-clicks: logistics networks in omni-channel grocery retailing. Int. J. Phys. Distrib. Logist. Manag. 48 (4), 415–438 (2018)
Ulrich, M., Jahnke, H., Langrock, R., Pesch, R., Senge, R.: Distributional regression for demand forecasting in e-grocery. Eur. J. Oper. Res. 294, 831–842 (2019)
Pan, S., Giannikas, V., Han, Y., Grover-Silva, E., Qiao, B.: Using customer-related data to enhance e-grocery home delivery. Ind. Manag. Data Syst. 117 (9), 1917–1933 (2017)
Davies, A., Dolega, L., Arribas-Bel, D.: Buy online collect in-store: exploring grocery click&collect using a national case study. Int. J. Retail Distrib. Manag. 47 (3), 278–291 (2019)
Fagerstrøm, A., Eriksson, N., Sigurdsson, V.: Investigating the impact of Internet of Things services from a smartphone app on grocery shopping. J. Retail. Consum. Serv. 52, 101927 (2020)
Berg, J., Henriksson, M.: In search of the ‘good life’: Understanding online grocery shopping and everyday mobility as social practices. J. Transp. Geogr. 83 , 102633 (2020)
Fagerstrøm, A., Eriksson, N., Siguresson, V.: What’s the ‘thing’ in Internet of Things in grocery shopping? a customer approach. Procedia Comput. Sci. 121 , 384–388 (2017)
Bryła, P.: Organic food online shopping in Poland. Br. Food J. 120 (5), 1015–1027 (2018)
Osman, R., Hwang, F.: A method to study how older adults navigate in an online grocery shopping site. In: 2016 4th International Conference on User Science and Engineering (i-USEr), pp. 247–252 (2016)
Rogus, S., Guthrie, J.F., Niculescu, M., Mancino, L.: Online grocery shopping knowledge, attitudes, and behaviors among SNAP participants. J. Nutr. Educ. Behav. 52 (5), 539–545 (2020)
Martinez, O., Tagliaferro, B., Rodriguez, N., Athens, J., Abrams, C., Elbel, B.: EBT payment for online grocery orders: a mixed-methods study to understand its uptake among SNAP recipients and the barriers to and motivators for its use. J. Nutr. Educ. Behav. 50 (4), 396-402.e1 (2018)
Muhammad, N.S., Sujak, H., Rahman, S.A.: Buying groceries online: the influences of electronic service quality (eServQual) and situational factors. Procedia Econ. Finan. 37 (16), 379–385 (2016)
Crisafulli, B., Singh, J.: Service failures in e-retailing: examining the effects of response time, compensation, and service criticality. Comput. Human Behav. 77 , 413–424 (2017)
Kang, C., Moon, J., Kim, T., Choe, Y.: Why consumers go to online grocery: Comparing vegetables with grains. In: Proceedings of the Annual Hawaii International Conference on System Sciences, vol. 2016-March, pp. 3604–3613 (2016)
Davis, F.D.: Perceived usefulness, perceived ease of use, and user acceptance of information technology. MIS Q. 319–340 (1989)
Shukla, A., Sharma, S.K.: Evaluating consumers’ adoption of mobile technology for grocery shopping: an application of technology acceptance model. J. Bus. Perspect. 22 (2), 185–198 (2018)
Mukerjee, H.S., Deshmukh, G.K., Prasad, U.D.: Technology readiness and likelihood to use self-checkout services using smartphone in retail grocery stores: empirical evidences from Hyderabad, India. Bus. Perspect. Res. 7 (1), 1–15 (2019)
Chakraborty, D.: Indian shoppers’ attitude towards grocery shopping apps: a survey conducted on smartphone users. Metamorph. A J. Manag. Res. 18 (2), 83–91 (2019)
Driediger, F., Bhatiasevi, V.: Online grocery shopping in Thailand: consumer acceptance and usage behavior. J. Retail. Consum. Serv. 48 , 224–237 (2019)
Sreeram, A., Kesharwani, A., Desai, S.: Factors affecting satisfaction and loyalty in online grocery shopping: an integrated model. J. Indian Bus. Res. 9 (2), 107–132 (2017)
Kim, E., Park, M.-C., Lee, J.: Determinants of the intention to use Buy-Online, Pickup In-Store (BOPS): the moderating effects of situational factors and product type. Telemat. Inf. 34 (8), 1721–1735 (2017)
Chen, H., Duan, W., Zhou, W.: The interplay between free sampling and word of mouth in the online software market. Decis. Support. Syst. 95 , 82–90 (2017)
Sigurdsson, V., Larsen, N.M., Alemu, M.H., Gallogly, J.K., Menon, R.G.V., Fagerstrøm, A.: Assisting sustainable food consumption: the effects of quality signals stemming from consumers and stores in online and physical grocery retailing. J. Bus. Res. 112 , 458–471 (2019)
González, X.: Chain heterogeneity and price-setting behavior: evidence from e-grocery retailers. Electron. Commer. Res. Appl. 26 (September), 62–72 (2017)
Faraoni, M., Rialti, R., Zollo, L., Pellicelli, A.C.: Exploring eLoyalty antecedents in B2C e-Commerce: empirical results from Italian grocery retailers. Br. Food J. 121 (2), 574–589 (2019)
Inman, J.J., Nikolova, H.: Shopper-facing retail technology: a retailer adoption decision framework incorporating shopper attitudes and privacy concerns. J. Retail. 93 (1), 7–28 (2017)
Huyghe, E., Verstraeten, J., Geuens, M., Van Kerckhove, A.: Clicks as a healthy alternative to bricks: how online grocery shopping reduces vice purchases. J. Mark. Res. 54 (1), 61–74 (2017)
Prashar, K., Kalotra, A.: Modelling the effect of E service quality on consumer satisfaction towards E grocery. Webology 18 (4) (2021). ISSN: 1735–188X
Mackenzie, A.: Personalization and probabilities: impersonal propensities in online grocery shopping. Big Data Soc. 5 (1), 1–15 (2018)
Alzubairi, A., Alrabghi, A.: Assessing the profitable conditions of online grocery using simulation store to home click-and-collect home drive-through. In: Proceedings of the 2017, pp. 1838–1842. IEEE IEEM (2017)
C. Fikar, A. Mild, and M. Waitz, “Facilitating consumer preferences and product shelf life data in the design of e-grocery deliveries,” Eur. J. Oper. Res., vol. 239, no. 3, (2019)
Bjørgen, A., Bjerkan, K.Y., Hjelkrem, O.A.: E-groceries: sustainable last mile distribution in city planning. Res. Transp. Econ. 87 , 100805 (2019)
Ajzen, I., Fishbein, M.: Attitude-behavior relations: a theoretical analysis and review of empirical research. Psychol. Bull. 84 (5), 888 (1977)
Hofstede, G.: Dimensionalizing cultures: the hofstede model in context. Online Read. Psychol. Cult. 2 (1), 1092–1096 (2011)
Venkatesh, V.: Determinants of perceived ease of use: Integrating control, intrinsic motivation, and emotion into the technology acceptance model. Inf. Syst. Res. 11 (4), 342–365 (2000)
Venkatesh, V., Morris, M.G., Davis, G.B., Davis, F.D.: User acceptance of information technology: toward a unified view. MIS Q. 425–478 (2003)
Download references
Author information
Authors and affiliations.
University School of Business, Chandigarh University, Ajitgarh, Punjab, India
Kirti Prashar & Anil Kalotra
Sharda University, Greater Noida, U.P, India
Kirti Prashar
You can also search for this author in PubMed Google Scholar
Corresponding author
Correspondence to Kirti Prashar .
Editor information
Editors and affiliations.
Eindhoven University of Technology, Eindhoven, The Netherlands
Miguel Botto-Tobar
Universidad Técnica del Norte, Ibarra, Ecuador
Marcelo Zambrano Vizuete
Universidad Rey Juan Carlos, Madrid, Spain
Sergio Montes León
Universidad Técnica Particular de Loja, Loja, Ecuador
Pablo Torres-Carrión
International University of Sarajevo, Sarajevo, Bosnia and Herzegovina
Benjamin Durakovic
Rights and permissions
Reprints and permissions
Copyright information
© 2024 The Author(s), under exclusive license to Springer Nature Switzerland AG
About this paper
Cite this paper.
Prashar, K., Kalotra, A. (2024). Adoption of Online Grocery Shopping: A Systematic Review of the Literature. In: Botto-Tobar, M., Zambrano Vizuete, M., Montes León, S., Torres-Carrión, P., Durakovic, B. (eds) International Conference on Applied Technologies. ICAT 2023. Communications in Computer and Information Science, vol 2051. Springer, Cham. https://doi.org/10.1007/978-3-031-58950-8_2
Download citation
DOI : https://doi.org/10.1007/978-3-031-58950-8_2
Published : 30 May 2024
Publisher Name : Springer, Cham
Print ISBN : 978-3-031-58949-2
Online ISBN : 978-3-031-58950-8
eBook Packages : Computer Science Computer Science (R0)
Share this paper
Anyone you share the following link with will be able to read this content:
Sorry, a shareable link is not currently available for this article.
Provided by the Springer Nature SharedIt content-sharing initiative
- Publish with us
Policies and ethics
- Find a journal
- Track your research
ORIGINAL RESEARCH article
Online consumer satisfaction during covid-19: perspective of a developing country.

- 1 Antai College of Economics & Management, Shanghai Jiao Tong University, Shanghai, China
- 2 School of Management, Zhejiang Shuren University, Hangzhou, China
- 3 Faculty of Management Sciences, Riphah International University, Faisalabad Campus, Punjab, Pakistan
- 4 Department of Professional Psychology, Bahria University, Islamabad, Pakistan
A conceptual model based on the antecedents and consequences of online consumer satisfaction has been proposed and empirically proved in this study. Data were collected during Smart Lockdown of COVID-19 from 800 respondents to observe the difference between perceived and actual, and direct and indirect e-stores. Confirmatory factor analysis was used to observe the validity of the data set. The structural equation modeling technique was used to test the hypotheses. The findings indicated that consumers feel more satisfied when they shop through direct e-store than indirect e-store, whereas their perception and actual experience are different. Implications have also been added to the study.
Introduction
Online shopping is the act of buying a product or service through any e-stores with the help of any website or app. Tarhini et al. (2021) stated that shopping through online channels is actively progressing due to the opportunity to save time and effort. Furthermore, online shopping varies from direct e-store and indirect e-store about their perception against the actual experience. Developing countries still face various conflicts and issues while promoting and utilizing e-commerce to the maximum compared with the developed countries ( Rossolov et al., 2021 ). In the developing countries, the difference between the perception and actual experience of the consumers varies when buying from indirect e-store compared to the direct e-store. On the contrary, as the world has been suffering from the COVID-19 pandemic, it has brought drastic changes globally in many sectors, business being one of them. De Vos (2020) stated that a large-scale lockdown was imposed worldwide to prevent the virus from spreading.
To survive, switching traditional shopping or trade toward digital was one factor that captured the attention across the globe on a larger scale. In April 2020, Walmart reported a 74% increase in online sales even though they faced a low customer walk-in at stores ( Nassauer, 2020 ; Redman, 2020 ). This upsurge of swift adoption of online channels has led this research to ask a few questions. First, what will be the difference between the perceived and the actual product purchased online? A recent study has documented that consumers bear actual risk after shopping through online channels ( Yang et al., 2020 ). Research reported that 30% of the products through online channels get returned and are not according to their perception ( Saleh, 2016 ). The same author also showed that the return and complaint rates are getting higher when consumers shop through an online channel.
Second, is there any difference between the perceived and the actual product purchase online from a direct e-store or an indirect e-store? Direct e-store means the online brand store, for example, Walmart, and indirect e-store means third-party stores such as Amazon, Alibaba, Jingdong (JD), and Daraz. The direct e-store strives hard to maintain a clear, potent perception in the mind of its buyer ( Grewal et al., 2009 ). Kumar and Kim (2014) stated that a brand strengthening its relationship with its consumer satisfies its needs through the actual product or services. In the literature ( Olotewo, 2017 ; Rossolov et al., 2021 ), it is stated that the shopping patterns of buyers from direct and indirect e-stores vary greatly, especially in the developing countries. In this way, when shopping through a direct e-store, consumers may easily recognize the difference in buying from a direct and indirect e-stores ( Mendez et al., 2008 ).
Third, a conceptual framework from a consumer perspective, antecedents and consequences of customer satisfaction, has been proposed and empirically proved. The literature ( Alharthey, 2020 ) discussed different risk types in online shopping. Three main types of risk, perceived uncertainty, perceived risk, and price, are addressed in this model. To the best of the knowledge of the authors, no such investigation directed specific circumstances, particularly in the developing countries. Therefore, it is necessary to look for the antecedents and consequences of customer satisfaction to promote online shopping in the developing countries. The degree of consumer satisfaction defines his/her experience and emotions about the product or service purchased through the online channel. Recent studies ( Guzel et al., 2020 ; Mamuaya and Pandowo, 2020 ) stated that the intention of the consumers to repurchase and their electronic-word-of-mouth (e-WOM) depends on their degree of satisfaction. In light of these heavy investments in online shopping, there is an exciting yet unexplored opportunity to comprehend better how the purchasing experiences of consumers through online channels influence their satisfaction level.
The study contributed to the current marketing literature in several ways. First, this study has highlighted that the perceived risk is very high when shopping through online channels, mainly the indirect e-stores. Therefore, the managers should develop strategies that reduce the perceived risk for the online consumer to shop more. Second, the study also disclosed that the perceived uncertainty in shopping through the online channel is high. While shopping online, the website design, graphics, and color scheme make the product more attractive than the actual one. Therefore, the managers must balance the visual appearance of the product on the website with the actual appearance of the product. This would increase the confidence and satisfaction of the consumer. Third, this study has also revealed that people are more satisfied while shopping from direct e-stores than indirect e-stores. Because the focal brands officially sponsor the direct e-stores, they pay more attention to their quality to retain consumers and maintain their brand reputation. Fourth, an indirect e-store works as a third party or a retailer who does not own the reputation of the product. This study exhibited the difference between the perception of the consumer being very high and the actual experience of using that product being quite different when shopping from the indirect channel. Last but not the least, this study is the first to report pre- and post-purchase consumer behavior and confirmed the perceived and the actual quality of a product bought from (i) direct e-store and (ii) indirect e-store.
Literature Review
Theoretical review.
Literature shows that when consumers get influenced to buy a particular product or service, some underlying roots are based on their behavior ( Wai et al., 2019 ). Appraisal theory significantly explains consumer behavior toward shopping and provides an opportunity to analyze the evaluation process (e.g., Roseman, 2013 ; Kähr et al., 2016 ; Moors et al., 2017 ; Ul Haq and Bonn, 2018 ). This research, aligned with the four dimensions of appraisal theory as the first stage, clearly defines the agency stage that either of the factors is responsible for customer satisfaction. The second stage explains that consumer's degree of satisfaction holds great importance and refers to novelty in the literature. The third stage of the model briefly explains the feelings and emotions of the consumers about the incident, aligning with the certainty phase. The last step explains whether the consumers have achieved their goal or are not aligned with the appetitive purpose.
Cognitive appraisal researchers stated that various emotions could be its root cause ( Scherer, 1997 ); it could be the reaction to any stimulus or unconscious response. On the contrary, four dimensions of appraisal theory are discussed in this research ( Ellsworth and Smith, 1988 ; Ma et al., 2013 ). Agency (considering themselves or objects are answerable for the result of the circumstance) ( Smith and Ellswoth, 1985 ; Durmaz et al., 2020 ); novelty (assessing the difference between the perception of an individual and his actual experience) ( Ma et al., 2013 ); certainty (analysis of the apparent probability of a specific outcome and its effect on the emotions of the buyer) ( Roseman, 1984 ), and appetitive goal (judging the degree to what extent the goal has been achieved) ( Hosany, 2012 ).
Hypotheses Development
Perceived risk and consumer satisfaction.
Perceived risk is the perception of shoppers having unpleasant results for buying any product or service ( Gozukara et al., 2014 ). Consumers who buy a specific product or service strongly impact their degree of risk perception toward buying ( Jain, 2021 ). Buyers who tend to indulge in buying through online channels face perceived risk characterized by their perception compared to the actual uncertainty involved in it ( Kim et al., 2008 ). Literature ( Ashoer and Said, 2016 ; Ishfaq et al., 2020 ) showed that as the risk of buying is getting higher, it influences the degree of consumers about information about their buying, either purchasing from the direct or indirect e-shop. Johnson et al. (2008) stated that consumer judgment that appears due to their experience strongly impacts their satisfaction level. Jin et al. (2016) said that as the ratio of risk perception of their consumer decreases, it enhances customer satisfaction. Thus, from the above arguments, it is hypothesized as follows:
H 1 : Perceived risk has a significant negative impact on consumer satisfaction—direct vs. indirect e-store; perceived vs. actual experience .
Perceived Uncertainty and Consumer Satisfaction
Uncertainty is defined as a time that occurs in the future that comprises the predictable situation due to the asymmetry nature of data ( Salancik and Pfeffer, 1978 ). Consumers may not expect the outcome of any type of exchange conducted as far as the retailer and product-oriented elements are concerned ( Pavlou et al., 2007 ). Therefore, uncertainty initiates that retailers may not be completely predictable; on the contrary, consumers tend to analyze and understand their actions about decision making ( Tzeng et al., 2021 ). Thus, the degree of uncertainty involved in buying through online channels influences that degree of customer satisfaction. In addition, when the performance of any particular product or service matches the degree of expectations, he gets satisfied and, hence, repeats his decision of buying ( Taylor and Baker, 1994 ). But if the product quality fails to meet the requirements, it negatively affects the degree of satisfaction ( Cai and Chi, 2018 ).
H 2 : Perceived uncertainty has a significant negative impact on consumer satisfaction—direct vs. indirect e-store; perceived vs. actual experience .
Price Value and Consumer Satisfaction
Oliver and DeSarbo (1988) suggested that the price value is the proportion of the result of the buyer to the input of the retailer. It is defined as an exchange of products/services based on their quality against a price that is to be paid ( Dodds et al., 1991 ). Consumers look for a higher value in return; consumers are willing to pay a higher price ( Pandey et al., 2020 ). Yet, it leads to higher dissatisfaction when they receive a lower degree of profitable products. Besides, the buyers associate such type of product/service they use as less favorable or not according to their needs and desires. Hence, the buyers regret their decision-making degree for choosing that particular product ( Zeelenberg and Pieters, 2007 ). Aslam et al. (2018) indicated that a product/service price influences the satisfaction of a buyer. Afzal et al. (2013) recommended that the price is among those factors that hold great significance for the degree of satisfaction of the consumer. If the price value of any product/service differs from consumer to consumer, consumers tend to switch brands. Hence, it is hypothesized that:
H3 : Price value has a significant positive impact on consumer satisfaction—direct vs. indirect e-store; perceived vs. actual experience .
Consumer Satisfaction With Consumer Delight, Consumer Regret, and Outrage
Satisfaction is defined as how a consumer is pleased with a particular brand or view about a product/service that matches requirements. It is an essential factor that triggers when the product or service performance exceeds the expectation and perception of the customers ( Woodside et al., 1989 ). The decision of the buyer significantly affects their satisfaction toward the product or service ( Park et al., 2010 ). If buyers are satisfied with the product/service they purchased online, this degree of satisfaction significantly affects their repurchase intention and WOM ( Butt et al., 2017 ). Tandon (2021) stated that a consumer satisfied with the product/service would get delighted. Consumer satisfaction, when exceeding the expectations, leads to consumer delight ( Mikulić et al., 2021 ). Mattila and Ro (2008) recommended that the buyer gets disappointed by anger, regret, and outrage. It also defines that negative emotions have a significant effect on the purchasing intention of the consumers. Oliver (1989) stated that unsatisfied buyers or products that do not fulfill the needs of the customers can create negative emotions. Sometimes, their feelings get stronger and result in sadness and outrage. Bechwati and Xia (2003) recommended that the satisfaction of the consumers influences their behavior to repurchase; outraged consumers due to dissatisfaction sometimes want to hurt the company. Besides deciding to purchase, consumers mostly regret their choices compared to other existing choices ( Rizal et al., 2018 ). Hechler and Kessler (2018) investigated that consumers who are outraged in nature actively want to hurt or harm the company or brand from which they got dissatisfied or hurt. Thus, it is proposed that:
H 4 : Consumer satisfaction has a significant negative impact on (a) consumer delight, (b) consumer regret, (c) consumer outrage—direct vs. indirect e-store; perceived vs. actual experience .
Consumer Delight and E-WOM
Oliver et al. (1997) recommended that a degree of delight in a buyer is termed as a positive emotion. Consumers purchase a product/service that raises their degree of expectation and gets them delighted ( Crotts and Magnini, 2011 ). Delighted buyers are involved in sharing their experiences with their friends and family and spreading positive WOM to others ( Parasuraman et al., 2020 ). Happy buyers generally share their opinions while posting positive feedback through social media platforms globally ( Zhang, 2017 ). A positive WOM of the buyer acts as a fundamental factor in spreading awareness about the product/service and strongly impacts other buyers regarding buying it ( Rahmadini and Halim, 2018 ). Thus, it is proposed that:
H5 : Consumer delight has a significant positive impact on E-WOM—direct vs. indirect e-store; perceived vs. actual experience .
Consumer Delight and Repurchase Intention
Delighted consumers tend toward brand loyalty; thus, they increase their buying intention of the service or product ( Ludwig et al., 2017 ; Ahmad et al., 2021 ). Customers can understand the objective of loyalty in purchasing a similar product or a new one from the same company. Delighted consumers tend to indulge in a higher degree of an emotional state that leads them to higher purchase intentions; it eliminates the switching of brands ( Parasuraman et al., 2020 ). Kim et al. (2015) stated that consumers delighted with a product or service of a brand become loyal to it, and the possibility of switching brands gets very low. Research ( Loureiro and Kastenholz, 2011 ; Tandon et al., 2020 ) shows that delighted consumers are more eager to purchase the same product again. Hence, it is proposed that:
H6 : Consumer delight has a significant positive impact on his repurchase intention—direct Vs. indirect e-store; Perceived Vs. actual experience
Consumer Regret and E-WOM
Regret is considered a negative emotion in reaction to an earlier experience or action ( Tsiros and Mittal, 2000 ; Kumar et al., 2020 ). Regret is when individuals frequently feel pity, disgrace, shame, or humiliation after acting in a particular manner and afterward try to amend their possible actions or decisions ( Westbrook and Oliver, 1991 ; Tsiros and Mittal, 2000 ). Regret is that specific negative emotion the buyers feel while making a bad decision that hurts them; their confidence level is badly affected. They blame themselves for choosing or creating a terrible decision ( Lee and Cotte, 2009 ). Li et al. (2010) suggested that buyers quickly start regretting and find their way to express their negative emotions. When they feel betrayed, they tend to spread negative WOM (NWOM) as a response to their anxiety or anger. Jalonen and Jussila (2016) suggested that buyers who get dissatisfied with their selections get involved in negative e-WOM about that particular brand/company. Earlier research says that buyers suffering from failure to buy any product/services tend to participate actively and play a role in spreading NWOM due to the degree of regret after making bad choices. Whelan and Dawar (2014) suggested that consumers sense that business has treated them unreasonably, and many consumers complain about their experience, resulting in e-WOM that may reduce consumer repurchase intention. Thus, it can be stated that:
H7 : Consumer regret has a significant negative impact on e-WOM—direct vs. indirect e-store; perceived vs. actual experience .
Consumer Regret and Repurchase Intention
Regret has a substantial influence on the intentions of the consumers to not entirely be measured by their degree of happiness ( Thibaut and Kelley, 2017 ). Results may not be evaluated by matching the structured degree of expectation but are also linked to alternatives reachable in the market. Therefore, such sort of evaluation and assessments will probably influence repurchase intention. For example, suppose the skipped reserve overtakes the picked alternative. In that case, the customer might change the replacement for the future purchase, regardless of whether the individual is profoundly happy with the picked option ( Liao et al., 2017 ). According to the researchers, there is a negative relationship between regret and consumer repurchase intention ( Liao et al., 2017 ; Durmaz et al., 2020 ). Furthermore, Unal and Aydin (2016) stated that perceived risk negatively impacts regret, influencing the repurchase intention of the consumers. Thus, it can be stated that:
H8 : Customer's regret has a significantly negative influence on his repurchase intention—direct vs. indirect e-store; perceived vs. actual experience .
Consumer Outrage and E-WOM
The disappointment of the consumers is a negative response to a product or a service ( Anderson and Sullivan, 1993 ). Outrage is the negative emotion a consumer experience when he purchases something totally against his requirements ( Lindenmeier et al., 2012 ). Besides, when the perception of the buyer is infringed, such behaviors occur. According to Torres et al. (2019) , enraged consumers get involved in communicating their outrage through e-WOM. Outraged consumers actively hurt the firm or brand from which they got hurt ( Hechler and Kessler, 2018 ). Consumers give e-WOM online reviews to decrease the negative emotions from the experiences of the consumer and re-establish a calm mental state to equilibrium ( Filieri et al., 2021 ). Thus, such consumers tend to give negative comments about the brand or product, which failed to match their expectations. NWOM has been characterized as negative reviews shared among people or a type of interpersonal communication among buyers concerning their experiences with a particular brand or service provider ( Balaji et al., 2016 ). Hence, it is hypothesized that:
H9 : Consumer outrage has a significant negative impact on e-WOM—direct vs. indirect e-store; perceived vs. actual experience .
Consumer Outrage and Repurchase Intentions
Repurchase intentions are characterized as the expressed trust of a buyer that they will or will not purchase a specific product and service again in the future ( Malhotra et al., 2006 ). Establishing relations with buyers should result in the repurchase. Negative disconfirmation ensues dissatisfaction or a higher level of outrage ( Escobar-Sierra et al., 2021 ). When a service/product fails and is not correctly addressed, the negative appraisal is overstated. Hence, “it may be more difficult to recover from feelings of victimization than to recover from irritation or annoyance” typically associated with dissatisfaction ( Schneider and Bowen, 1999 , p. 36). Therefore, consumers get outraged from buying such a product that fails to match their perception. When the experience of a consumer prompts a negative disconfirmation, the purchaser will also have a higher urging level through outrage. Therefore, consumers will probably have negative intentions to repurchase and do not want to indgule in making the same decision repeatedly ( Wang and Mattila, 2011 ; Tarofder et al., 2016 ). Therefore, it is proposed that:
H10 : Consumer outrage has a significant negative impact on repurchase intention—direct vs. indirect e-store; perceived vs. actual experience .
Methodology
This research explores the difference between the perception of the consumers and the actual online shopping experience through direct and indirect e-stores. It was an experimental design in which online shopping was studied in the developing countries. Data were collected from those individuals who shop from online channels; direct e-store and indirect e-store. Taking care of COVID-19 standard operating procedures, only 50 respondents were gathered two times, every time in a university auditorium after obtaining the permission from the administration. The total capacity of the auditorium was 500, as the lockdown restrictions were lifted after the first wave of the coronavirus.
Data Collection Tool
A questionnaire was used for the survey. The questionnaire was adapted in English to guarantee that the respondents quickly understood the questions used. A cross-sectional study technique was used for this research. A cross-sectional study helps in gathering the data immediately and collects data from a large sample size. The total number of distributed questionnaires was 1,250, out of which 800 were received in the usable form: 197 incomplete, 226 incorrect, and dubious responses, and 27 were eliminated. Thus, a 64% response rate was reported. Research showed that a 1:10 ratio is accepted ( Hair et al., 1998 ) as far as the data collection is concerned; for that instance, this study data fell in the acceptable range.
Indirect E-Store
Consumers who prefer to shop through online channels were gathered in an auditorium of an institute. Only those consumers were eligible for this experiment, who themselves buy through e-stores. A few products were brought from an indirect e-store, and later on, those products were shown to the respondents from the website of that indirect e-store. After showing products, we asked the respondents to fill the survey as per their perception of the product. Then we asked them to fill out another questionnaire to ascertain the difference between the perception and actual experience when purchasing from an indirect e-store. Once all the respondents completed the survey, we have shown them the actual products they have selected by seeing the website of the indirect e-store.
Direct E-Store
The second experiment was carried out on those consumers who shop from direct e-stores. For that purpose, a few popular reviewed clothing articles were purchased from the e-store. As in the case of an indirect e-store, respondents were also shown these articles from the websites of these direct e-stores. We then asked the respondents to fill the survey to confirm their perception of the products. Once all the respondents completed the survey, we showed them the actual product and asked them to fill out another questionnaire according to their actual purchasing experience from the direct e-store. The primary purpose of this experiment was to compare buying from direct e-store and indirect e-store.
Construct Instruments
The total number of items was 34, which were added in the earlier section of the questionnaire. These items were evaluated with the help of using a five-point Likert scale that falls from strongly disagree (1) to strongly agree (5). The items used in the study were empirically validated. Table 2 carries the details of the items of the questionnaire. The price value was evaluated using three items used by Venkatesh et al. (2012) . The perceived uncertainty was one of the independent variables that carry four items derived from Pavlou et al. (2007) . Perceived risk was the third independent variable used, held three items; thus, its scale was derived from Shim et al. (2001) . Wang (2011) validated consumer satisfaction carrying three items; consumer delight was measured by a 3-item scale proposed by Finn (2012) ; consumer regret was measured by the scale proposed by Wu and Wang (2017) . It carries a three-item scale. Consumer outrage was measured by Liu et al. (2015 ); it has six items. Repurchase intention was measured through a scale adapted from Zeithaml et al. (1996) , which carries four items. e-WOM was validated by the scale adapted from Goyette et al. (2010) ; it has five items.
Demographics of the Respondents
A total of 800 questionnaires were filled, and the respondents expressed their perception and actual experience from direct e-store and indirect e-store. Respondents belonged to different age groups from 18 to 50 years and above. There were 49% women and 51% men who took part in filling this survey. The income level of the respondents was grouped in different categories from “above 10,000 to above 50,000. The majority (56%) of the respondents were single, and 44% were married (Details can be viewed in Figure 1 ; Table 1 ). Data for both direct and indirect e-store was collected equally; 50% each to compare each category better.
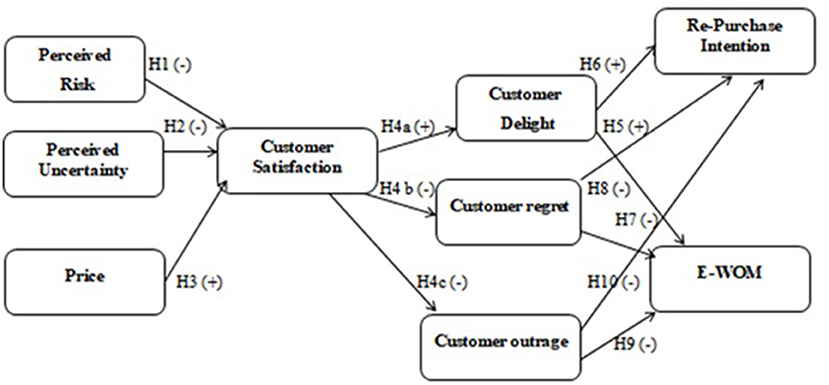
Figure 1 . Proposed conceptual framework.
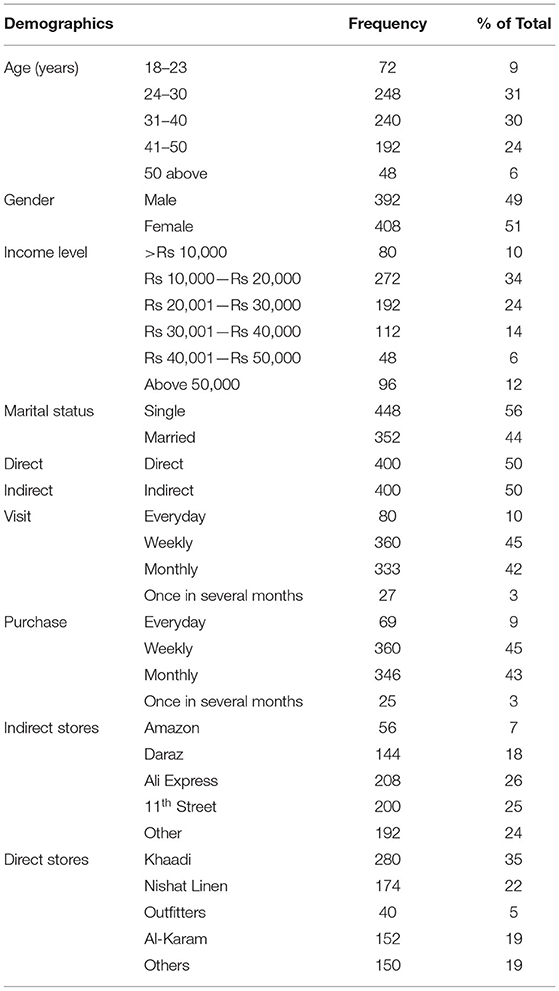
Table 1 . Demographics of the respondents.
Reliability and Validity
Reliability evaluates with the help of composite reliability (CR). All CR values fall into the range of 0.7–0.9, which is acceptable ( Hair et al., 2011 ). Convergent and discriminant validity has been observed through confirmatory factor analysis as recommended by some researchers ( Fornell and Larcker, 1981 ; Hair et al., 2010 ).
Convergent Validity
Convergent validity is evaluated with the help of two standards mentioned in the literature earlier, factor loading and average variance extracted (AVE), both the values should be >0.5 ( Yap and Khong, 2006 ). The values are mentioned in Table 2 .
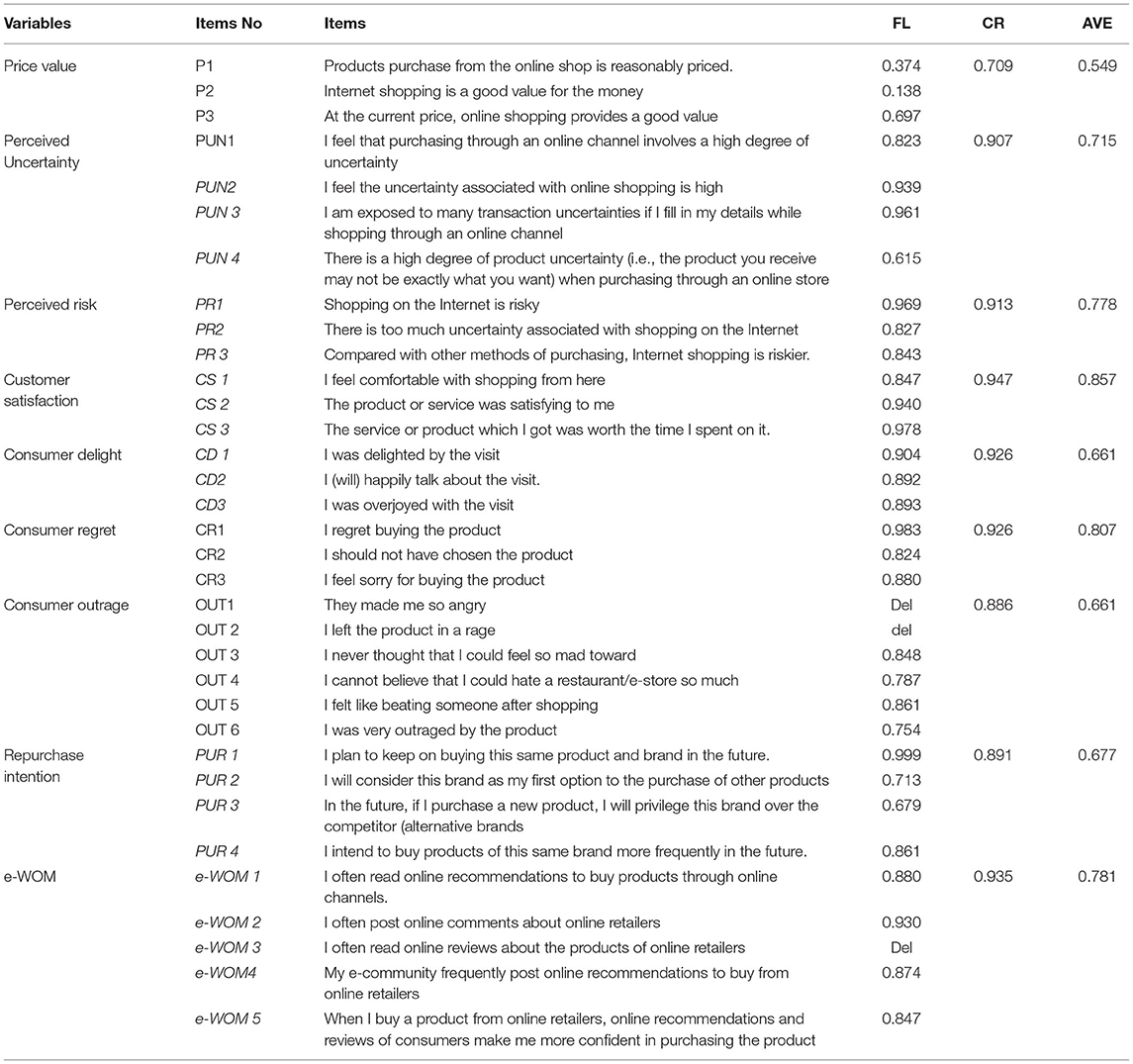
Table 2 . Reliability and convergent validity.
Discriminant Validity
Discriminant validity is evaluated based on two conditions that are required to evaluate it. First, the correlation between the conceptual model variables should be <0.85 ( Kline, 2005 ). Second, the AVE square value must be less than the value of the conceptual model ( Fornell and Larcker, 1981 ). Table 3 depicts the discriminant validity of the construct of the study.
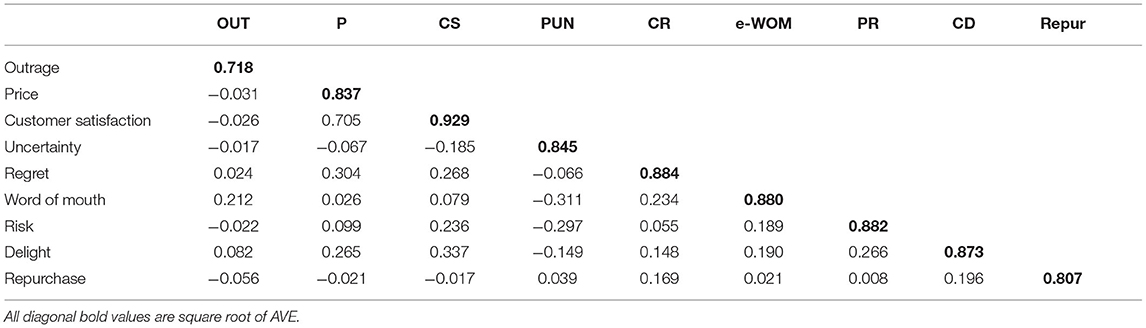
Table 3 . Discriminant validity.
Multi-Group Invariance Tests
Multi-group confirmatory factor analysis was conducted as the pre-requisites for the measurement model. The multi-group analysis was used to investigate a variety of invariance tests. Different invariance tests were performed to guarantee the items working precisely in the same manner in all the groups. In this research, the following are the model fit indexes, that is, CMIN/dF =2.992 CFI = 0.915, TLI = 0.906, and RMSEA = 0.071. Byrne (2010) and Teo et al. (2009) stated that CFI gives more accurate results, especially when comparing variables in different groups.
Hypotheses Testing
Scanning electron microscope technique was used to run and test the proposed hypotheses for the conceptual model. First, all the hypotheses proposed were checked, from which eight were initially accepted. Later, the multi-group test was utilized to test the proposed hypotheses and compare the shopping experience from direct e-store with indirect e-store and consumer perception with actual experience. Table 4 explains this in detail.
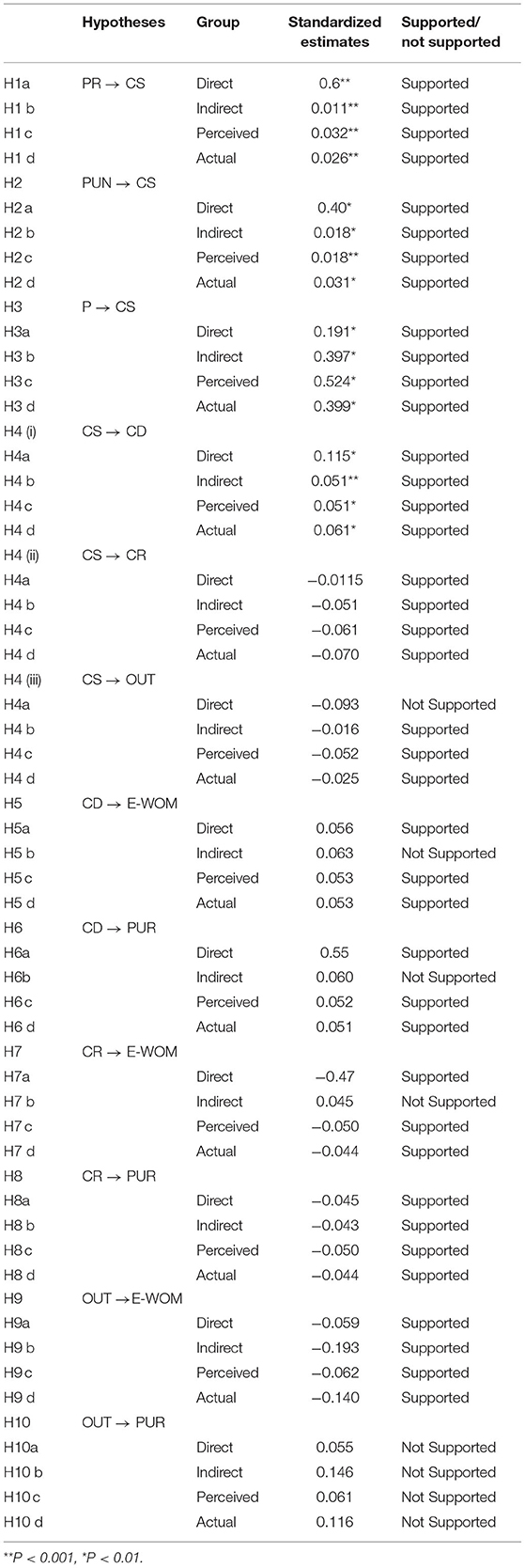
Table 4 . Hypotheses results.
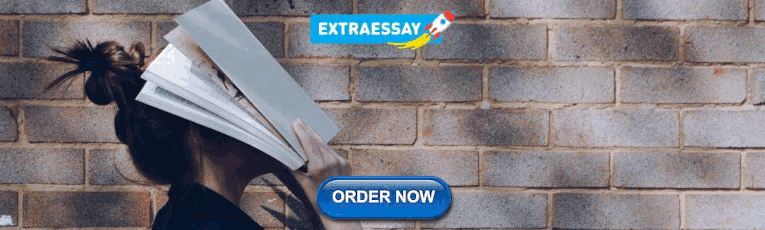
Discussion and Implications
This research offers a remarkable number of facts for practitioners. This study can benefit marketing strategists by reducing the perceived risk, decreasing the intensity of perceived uncertainty, stabilizing the price, enhancing consumer satisfaction, promoting delighting consumers, accepting the negative behavior of the consumers, consumer retention, and establishing a positive e-WOM.
Reducing Risks
Certain factors play a role in antecedents of consumer satisfaction; they are particularly those that resist consumers to shop from any online channel, neither direct e-store nor indirect e-store. Perceived risk, perceived uncertainty, and the price are some of those antecedents that play a significant role in affecting the degree of satisfaction of the consumers, resulting in either to retain a consumer or to outrage a consumer. This study aligns with the existing literature. Tandon et al. (2016) ; Bonnin (2020) and Pandey et al. (2020) showed that consumers seek to shop from an e-store without bearing any risk. Consumers feel more confident about an e-store when the perceived risk is less than shopping from traditional ones as consumers want to feel optimistic about their decision. Second, e-vendors should ensure that the quality of a product is up to the mark and according to the consumer needs. Therefore, vendors should offer complete details about the product/service and its risks to the consumers. Moreover, this study suggests that e-stores must align the visuals of a product with its actual appearance. This would help them to increase customer satisfaction and confidence in the e-store.
Focus on Consumer Satisfaction
Consumer satisfaction is the deal-breaker factor in the online sector. Literature ( Shamsudin et al., 2018 ; Hassan et al., 2019 ) showed that organizations prioritize their consumers by fulfilling their requirements and required assistance. As a result, consumers are more confident and become satisfied consumers in the long run. This study adds to the literature that the degree of satisfaction of the consumers plays an essential role in shopping from an e-store. Consumers feel more confident in shopping from a direct e-store than an indirect e-store as the difference in the perception of consumers and the actual experience varies. Therefore, online vendors should focus on satisfying their consumers as it plays a remarkable role in retaining consumers.
Value Consumer Emotions
Online, retaining, and satisfying consumers are the most vital factor that directly affects the organization. This research aligns with the existing literature ( Jalonen and Jussila, 2016 ; Hechler and Kessler, 2018 ; Coetzee and Coetzee, 2019 ); when the retailer successfully fulfills its requirements, the consumer gets delighted repeating his choice to repurchase. On the other hand, if the online retailer fails to serve the consumer, the consumer regrets and, in extreme cases, becomes outraged about his decision. The negative emotions of the consumers threaten the company from many perspectives, as the company loses its consumer and its reputation in the market is affected. Therefore, first, market practitioners should avoid ignoring the requirements of consumers. Second, online vendors should pay special attention to the feedback of the consumers and assure them that they are valued.
Consumer Retention
The ultimate goal is to retain its consumers, but e-vendors should make proper strategies to satisfy their consumers as far as the online sector is concerned. The earlier studies of Zhang et al. (2015) and Ariffin et al. (2016) contributed to the literature that consumer satisfaction is a significant aspect in retaining a consumer. This research has also suggested that the satisfaction of the consumers plays a vital role in retaining them. Moreover, online shoppers provide the fastest spread of the right WOM about the product/ service. Second, consumers should feel valued and committed to vendors.
Pre- and Post-buying Behavior
This study contributed to a conceptual model that deals with consumer pre- and post-purchase behavior from the direct and indirect e-stores. With the help of experimental design, this study has reported its finding, highlighted how a satisfied customer is delightful and shares e-WOM, and showed repurchase intention. However, if the customer is not satisfied with the flip of a coin, he may feel regretted or outraged and cannot share e-WOM or have a repurchase intention.
Conclusions
This research concludes that online shopping has boomed during this COVID-19 pandemic period, as the lockdown prolonged in both the developed and the developing countries. The study further supports the difference between shopping from a direct e-store and an indirect e-store. The perception of the consumers shopping from direct e-store is more confident, and their degree of satisfaction is much higher, as the actual experience of the consumers aligns with their perceptions. Instead, consumers feel dissatisfied or outraged to choose an indirect e-store for shopping. Indirect e-store makes false promises and guarantees to its buyers, and eventually, when the consumers experience the product, it is against their perception.
This research fills the literature gap about the antecedents that lead to online shopping growth in the developing countries. This study aligns with Hechler and Kessler's (2018) earlier research, which stated that dissatisfied consumers threaten the reputation of the organization. Furthermore, Klaus and Maklan (2013) , Lemon and Verhoef (2016) suggested that handling the experience and satisfaction of the buyers plays a significant role in surviving among its competitors. Grange et al. (2019) recommended that e-commerce develops and attracts consumers by fulfilling their needs and requirements quickly. This study aligned with the existing literature by adding factors influencing the shopping preferences of the consumers from an e-store.
Limitations and Future Research
Despite its significant findings, this research has some limitations and scope for future research. First, this research only examined a few risks involved in online shopping. Future research studies should analyze other risks, for example, quality risk and privacy risk. Second, this study focused on shopping through direct e-stores and indirect e-stores. Future research can implement a conceptual model of a specific brand. Third, this study can be implemented in other sectors, for example, tourism, and hospitality. Fourth, it may be fascinating to look at other fundamentals, such as age, gender, education, relation with the retailer, or the degree of involvement with online shopping to differentiate other factors.
The proposed framework can be utilized in other developing countries, as every country faces different problems according to its growth and development. The model can be examined among specific direct e-stores to compare new customers and loyal customers. Future studies can explore indirect relationships along with adding mediators and moderators in the proposed model.
Data Availability Statement
The original contributions presented in the study are included in the article, further inquiries can be directed to the corresponding author.
Ethics Statement
The studies involving human participants were reviewed and approved by This study involving human participants was reviewed and approved by the Ethics Committee of the Department of Management Sciences, Riphah International University, Faisalabad Campus, Faisalabad, Pakistan. The participants provided their written informed consent to participate in this study. The patients/participants provided their written informed consent to participate in this study.
Author Contributions
AS contributed to the conceptualization and writing the first draft of the research. JU contributed to visualizing and supervising the research. All authors who contributed to the manuscript read and approved the submitted version.
Conflict of Interest
The authors declare that the research was conducted in the absence of any commercial or financial relationships that could be construed as a potential conflict of interest.
Publisher's Note
All claims expressed in this article are solely those of the authors and do not necessarily represent those of their affiliated organizations, or those of the publisher, the editors and the reviewers. Any product that may be evaluated in this article, or claim that may be made by its manufacturer, is not guaranteed or endorsed by the publisher.
Afzal, S., Chandio, A. K., Shaikh, S., Bhand, M., and Ghumro, B. A. (2013). Factors behind brand switching in cellular networks. Int. J. Asian Soc. Science 3, 299–307.
Google Scholar
Ahmad, W., Kim, W. G., Choi, H. M., and Ul Haq, J. (2021). Modeling behavioral intention to use travel reservation apps: a cross-cultural examination between US and China. J. Retail. Consum. Serv. 63:102689. doi: 10.1016/j.jretconser.2021.102689
CrossRef Full Text | Google Scholar
Alharthey, B. (2020). The role of online trust in forming online shopping intentions. Int. J. Online Market. 10, 32–57. doi: 10.4018/IJOM.2020010103
Anderson, E. W., and Sullivan, M. W. (1993). The antecedents and consequences of customer satisfaction for firms. Market. Sci. 12, 125–143. doi: 10.1287/mksc.12.2.125
Ariffin, S., Yusof, J. M., Putit, L., and Shah, M. I. A. (2016). Factors influencing perceived quality and repurchase intention towards green products. Proc. Econ. Finan. 37, 391–396. doi: 10.1016/S2212-5671(16)30142-3
Ashoer, M., and Said, S. (2016). “The impact of perceived risk on consumer purchase intention in Indonesia; a social commerce study,” in Proceeding of the International Conference on Accounting, Management, Economics and Social Sciences . 1–13.
Aslam, W., Arif, I., Farhat, K., and Khursheed, M. (2018). The role of customer trust, service quality and value dimensions in determining satisfaction and loyalty: an Empirical study of mobile telecommunication industry in Pakistan. Market-TrŽište 30, 177–194. doi: 10.22598/mt/2018.30.2.177
Balaji, M. S., Khong, K. W., and Chong, A. Y. L. (2016). Determinants of negative word-of-mouth communication using social networking sites. Inform. Manage. 53, 528–540. doi: 10.1016/j.im.2015.12.002
Bechwati, N. N., and Xia, L. (2003). Do computers sweat? the impact of perceived effort of online decision aids on consumers' satisfaction with the decision process. J. Consum. Psychol. 13, 139–148. doi: 10.1207/S15327663JCP13-1andamp;2_12
Bonnin, G. (2020). The roles of perceived risk, attractiveness of the online store and familiarity with AR in the influence of AR on patronage intention. J. Retail. Consum. Serv. 52:101938. doi: 10.1016/j.jretconser.2019.101938
Butt, M. M., Rose, S., Wilkins, S., and Haq, J. U. (2017). MNCs and religious influences in global markets: drivers of consumer-based halal brand equity. Int. Market. Rev . 12:277. doi: 10.1108/IMR-12-2015-0277
Byrne, B. M. (2010). Structural Equation Modeling With AMOS: Basic Concepts, Applications, and Programming , 2nd Edn. New York, NY: Routledge.
Cai, R., and Chi, C. G. Q. (2018). The impacts of complaint efforts on customer satisfaction and loyalty. Serv. Industr. J. 38, 1095–1115. doi: 10.1080/02642069.2018.1429415
Coetzee, A., and Coetzee, J. (2019). Service quality and attitudinal loyalty: the mediating effect of delight on retail banking relationships. Glob. Bus. Econ. Rev. 21, 120–138. doi: 10.1504/GBER.2019.096856
Crotts, J. C., and Magnini, V. P. (2011). The customer delight construct: is surprise essential? Ann. Tourism Res. 38, 719–722. doi: 10.1016/j.annals.2010.03.004
De Vos, J. (2020). The effect of COVID-19 and subsequent social distancing on travel behavior. Transport. Res. Interdisciplin. Perspect. 5:100121. doi: 10.1016/j.trip.2020.100121
PubMed Abstract | CrossRef Full Text | Google Scholar
Dodds, W. B., Monroe, K. B., and Grewal, D. (1991). Effects of price, brand, and store information on buyers' product evaluations. J. Market. Res. 28, 307–319. doi: 10.1177/002224379102800305
Durmaz, Y., Demira,g, B., and Çavuşoglu, S. (2020). Influence of regret and regret reversing effort on dissatisfaction and repurchase intention after purchasing fashion products. Preprints. doi: 10.20944/preprints202003.0280.v1
Ellsworth, P. C., and Smith, C. A. (1988). Shades of joy: patterns of appraisal differentiating pleasant emotions. Cogn. Emot. 2, 301–331. doi: 10.1080/02699938808412702
Escobar-Sierra, M., García-Cardona, A., and Vera Acevedo, L. D. (2021). How moral outrage affects consumer's perceived values of socially irresponsible companies. Cogent Bus. Manage. 8:1888668. doi: 10.1080/23311975.2021.1888668
Filieri, R., Galati, F., and Raguseo, E. (2021). The impact of service attributes and category on eWOM helpfulness: an investigation of extremely negative and positive ratings using latent semantic analytics and regression analysis. Comput. Human Behav. 114:106527. doi: 10.1016/j.chb.2020.106527
Finn, A. (2012). Customer delight: distinct construct or zone of nonlinear response to customer satisfaction? J. Serv. Res. 15, 99–110. doi: 10.1177/1094670511425698
Fornell, C., and Larcker, D. F. (1981). Evaluating structural equation models with unobservable variables and measurement error. J. Market. Res. 18, 39–50. doi: 10.1177/002224378101800104
Goyette, I., Ricard, L., Bergeron, J., and Marticotte, F. (2010). e-WOM Scale: word-of-mouth measurement scale for e-services context. Can. J. Admin. Sci. 27, 5–23. doi: 10.1002/cjas.129
Gozukara, E., Ozyer, Y., and Kocoglu, I. (2014). The moderating effects of perceived use and perceived risk in online shopping. J. Glob. Strateg. Manage. 16, 67–81. doi: 10.20460/JGSM.2014815643
Grange, C., Benbasat, I., and Burton-Jones, A. (2019). With a little help from my friends: Cultivating serendipity in online shopping environments. Inf. Manage . 56, 225–235.
Grewal, D., Levy, M., and Kumar, V. (2009). Customer experience management in retailing: An organizing framework. J. Retail. 85, 1–14. doi: 10.1016/j.jretai.2009.01.001
Guzel, M., Sezen, B., and Alniacik, U. (2020). Drivers and consequences of customer participation into value co-creation: a field experiment. J. Product Brand Manage. doi: 10.1108/JPBM-04-2020-2847
Hair, J. F., Anderson, R. E., Tatham, R. L., and Black, W. C. (1998). Multivariate Data Analysis . 5th ed., Hoboken, NJ: Prentice-Hall.
Hair, J. F., Celsi, M., Ortinau, D. J., and Bush, R. P. (2010). Essentials of Marketing Research , Vol. 2. New York, NY: McGraw-Hill/Irwin.
Hair, J. F., Ringle, C. M., and Sarstedt, M. (2011). PLS-SEM: indeed a silver bullet. J. Market. Theor. Pract. 19, 139–152. doi: 10.2753/MTP1069-6679190202
Hassan, S., Shamsudin, M. F., and Mustapha, I. (2019). The effect of service quality and corporate image on student satisfaction and loyalty in TVET higher learning institutes (HLIs). J. Tech. Educ. Train. 11:4.
Hechler, S., and Kessler, T. (2018). On the difference between moral outrage and empathic anger: anger about wrongful deeds or harmful consequences. J. Exp. Soc. Psychol. 76, 270–282. doi: 10.1016/j.jesp.2018.03.005
Hosany, S. (2012). Appraisal determinants of tourist emotional responses. J. Trav. Res. 51, 303–314. doi: 10.1177/0047287511410320
Ishfaq, M., Nazir, M. S., Qamar, M. A. J., and Usman, M. (2020). Cognitive bias and the Extraversion personality shaping the behavior of investors. Front. Psychol. 11:556506. doi: 10.3389/fpsyg.2020.556506
Jain, S. (2021). Examining the moderating role of perceived risk and web atmospherics in online luxury purchase intention. J. Fash. Market. Manage. Int. J. 05:89. doi: 10.1108/JFMM-05-2020-0089
Jalonen, H., and Jussila, J. (2016). “Developing a conceptual model for the relationship between social media behavior, negative consumer emotions and brand disloyalty,” in Conference on e-Business, e-Services and e-Society (Cham: Springer), 134–145. doi: 10.1007/978-3-319-45234-0_13
Jin, N., Line, N. D., and Merkebu, J. (2016). The impact of brand prestige on trust, perceived risk, satisfaction, and loyalty in upscale restaurants. J. Hospital. Market. Manage. 25, 523–546. doi: 10.1080/19368623.2015.1063469
Johnson, M. S., Sivadas, E., and Garbarino, E. (2008). Customer satisfaction, perceived risk and affective commitment: an investigation of directions of influence. J. Serv. Market. 5:120. doi: 10.1108/08876040810889120
Kähr, A., Nyffenegger, B., Krohmer, H., and Hoyer, W. D. (2016). When hostile consumers wreak havoc on your brand: the phenomenon of consumer brand sabotage. J. Mark. 80, 25–41. doi: 10.1509/jm.15.0006
Kim, D. J., Ferrin, D. L., and Rao, H. R. (2008). A trust-based consumer decision-making model in electronic commerce: the role of trust, perceived risk, and their antecedents. Decis. Support Syst. 44, 544–564. doi: 10.1016/j.dss.2007.07.001
Kim, M., Vogt, C. A., and Knutson, B. J. (2015). Relationships among customer satisfaction, delight, and loyalty in the hospitality industry. J. Hospital. Tourism Res. 39, 170–197. doi: 10.1177/1096348012471376
Klaus, P. P., and Maklan, S. (2013). Towards a better measure of customer experience. Int. J. Market Res. 55, 227–246. doi: 10.2501/IJMR-2013-021
Kline, R. B. (2005). Principles and Practice of Structural Equation Modeling , 2nd Edn. New York, NY: Guilford Press.
Kumar, A., Chaudhuri, D., Bhardwaj, D., and Mishra, P. (2020). Impulse buying and post-purchase regret: a study of shopping behaviour for the purchase of grocery products. Int. J. Manage. 11:57. doi: 10.34218/IJM.11.12.2020.057
Kumar, A., and Kim, Y. K. (2014). The store-as-a-brand strategy: the effect of store environment on customer responses. J. Retail. Consum. Serv. 21, 685–695. doi: 10.1016/j.jretconser.2014.04.008
Lee, S. H., and Cotte, J. (2009). Post-purchase Consumer Regret: Conceptualization and Development of the PPCR Scale . ACR North American Advances.
Lemon, K. N., and Verhoef, P. C. (2016). Understanding customer experience throughout the customer journey. J. Market. 80, 69–96. doi: 10.1509/jm.15.0420
Li, S., Zhou, K., Sun, Y., Rao, L. L., Zheng, R., and Liang, Z. Y. (2010). Anticipated regret, risk perception, or both: which is most likely responsible for our intention to gamble? J. Gambl. Stud. 26, 105–116. doi: 10.1007/s10899-009-9149-5
Liao, C., Lin, H. N., Luo, M. M., and Chea, S. (2017). Factors influencing online shoppers' repurchase intentions: the roles of satisfaction and regret. Inform. Manage. 54, 651–668. doi: 10.1016/j.im.2016.12.005
Lindenmeier, J., Schleer, C., and Pricl, D. (2012). Consumer outrage: emotional reactions to unethical corporate behavior. J. Bus. Res. 65, 1364–1373. doi: 10.1016/j.jbusres.2011.09.022
Liu, M. W., and Keh, H. T. (2015). Consumer delight and outrage: scale development and validation. J. Serv. Theory Pract . 25, 680–699. doi: 10.1108/JSTP-08-2014-0178
Loureiro, S. M. C., and Kastenholz, E. (2011). Corporate reputation, satisfaction, delight, and loyalty towards rural lodging units in Portugal. Int. J. Hospital. Manage. 30, 575–583. doi: 10.1016/j.ijhm.2010.10.007
Ludwig, N. L., Heidenreich, S., Kraemer, T., and Gouthier, M. (2017). Customer delight: universal remedy or a double-edged sword? J. Serv. Theory Pract . 8:197. doi: 10.1108/JSTP-08-2015-0197
Ma, J., Gao, J., Scott, N., and Ding, P. (2013). Customer delight from theme park experiences: the antecedents of delight based on cognitive appraisal theory. Ann. Tour. Res. 42, 359–381. doi: 10.1016/j.annals.2013.02.018
Malhotra, N., Hall, J., Shaw, M., and Oppenheim, P. (2006). Marketing Research: An Applied Orientation . Melbourne, VC: Pearson Education Australia.
Mamuaya, N. C., and Pandowo, A. (2020). Determinants of customer satisfaction and its implications on word of mouth in e-commerce industry: case study in Indonesia. Asia Pacific J. Manage. Educ. 3, 16–27. doi: 10.32535/apjme.v3i1.740
Mattila, A. S., and Ro, H. (2008). Discrete negative emotions and customer dissatisfaction responses in a casual restaurant setting. J. Hosp. Tour. Res. 32, 89–107. doi: 10.1177/1096348007309570
Mendez, J. L., Oubina, J., and Rubio, N. (2008). Expert quality evaluation and price of store vs. manufacturer brands: an analysis of the Spanish mass market. J. Retail. Consum. Serv. 15, 144–155. doi: 10.1016/j.jretconser.2007.11.003
Mikulić, J., Kreši,ć, D., and Šerić, M. (2021). The factor structure of medical tourist satisfaction: exploring key drivers of choice, delight, and frustration. J. Hospital. Tour. Res. 1177:1096348020987273. doi: 10.1177/1096348020987273
Moors, A., Boddez, Y., and De Houwer, J. (2017). The power of goal-directed processes in the causation of emotional and other actions. Emot. Rev. 9, 310–318. doi: 10.1177/1754073916669595
Nassauer, S. (2020). Walmart sales surge as Coronavirus drives Americans to stockpile. Wall Street J . Availale online at: https://www.wsj.com/articles/walmart-sales-surge-as-coronavirus-drivesamericans-to-stockpile-11589888464?mod=hp_lead_pos5 (accessed on May 18, 2020).
Oliver, R. L. (1989). Processing of the satisfaction response in consumption. J. Consum. Satisfact. Dissatisfact. Complain. Behav. 2, 1–26.
Oliver, R. L., and DeSarbo, W. S. (1988). Response determinants in satisfaction judgments. J. Consum. Res. 14, 495–507. doi: 10.1086/209131
Oliver, R. L., Rust, R. T., and Varki, S. (1997). Customer delight: foundations, findings, and managerial insight. J. Retail. 73:311. doi: 10.1016/S0022-4359(97)90021-X
Olotewo, J. (2017). Examining the antecedents of in-store and online purchasing behavior: a case of Nigeria. J. Market. Res. Case Stud. 15, 1–16. doi: 10.5171/2017.668316
Pandey, N., Tripathi, A., Jain, D., and Roy, S. (2020). Does price tolerance depend upon the type of product in e-retailing? role of customer satisfaction, trust, loyalty, and perceived value. J. Strateg. Market. 28, 522–541. doi: 10.1080/0965254X.2019.1569109
Parasuraman, A., Ball, J., Aksoy, L., Keiningham, T. L., and Zaki, M. (2020). More than a feeling? toward a theory of customer delight. J. Serv. Manage . 3:34. doi: 10.1108/JOSM-03-2019-0094
Park, E. O., Chung, K. H., and Shin, J. I. (2010). The relationship among internal marketing, internal customer satisfaction, organizational commitment and performance. Product. Rev. 24, 199–232. doi: 10.15843/kpapr.24.2.201006.199
CrossRef Full Text
Pavlou, P. A., Liang, H., and Xue, Y. (2007). Understanding and mitigating uncertainty in online exchange relationships: a principal-agent perspective. MIS Q. 105–136. doi: 10.2307/25148783
Rahmadini, Y., and Halim, R. E. (2018). The “Influence of social media towards emotions, brand relationship quality, and word of Mouth (WOM) on Concert's Attendees in Indonesia,” in MATEC Web of Conferences (EDP Sciences) , 05058.
Redman, R. (2020). “Online grocery sales to grow 40% in 2020,” in Supermarket News . Available online at: https://www.supermarketnews.com/onlineretail/online-grocery-sales-grow-40-2020 (accessed on May 21, 2020).
Rizal, H., Yussof, S., Amin, H., and Chen-Jung, K. (2018). EWOM towards homestays lodging: extending the information system success model. J. Hosp. Tour. Technol. doi: 10.1108/JHTT-12-2016-0084
Roseman, I. J. (1984). Cognitive determinants of emotion: a structural theory. Rev. Person. Soc. Psychol. 5, 11–36.
Roseman, I. J. (2013). Author reply: on the frontiers of appraisal theory. Emot. Rev. 5, 187–188. doi: 10.1177/1754073912469592
Rossolov, A., Rossolova, H., and Holguín-Veras, J. (2021). Online and in-store purchase behavior: shopping channel choice in a developing economy. Transportation 20, 1–37. doi: 10.1007/s11116-020-10163-3
Salancik, G. R., and Pfeffer, J. (1978). Uncertainty, secrecy, and the choice of similar others. Soc. Psychol. 23, 246–255. doi: 10.2307/3033561
Saleh, M. A. H. (2016). Website design, technological expertise, demographics, and consumer's e-purchase transactions. Int. J. Market. Stud. 8, 125–138. doi: 10.5539/ijms.v8n1p125
Scherer, K. R. (1997). The role of culture in emotion-antecedent appraisal. J. Pers. Soc. Psychol. 73:902. doi: 10.1037/0022-3514.73.5.902
Schneider, B., and Bowen, D. E. (1999). Understanding customer delight and outrage. Sloan Manage. Rev. 41, 35–45. doi: 10.1016/S0022-4359(01)00035–45
Shamsudin, M. F., Razak, A. A., and Salem, M. A. (2018). The role of customer interactions towards customer satisfaction in theme parks experience. Opcion 34, 546–558.
Shim, S., Eastlick, M. A., Lotz, S. L., and Warrington, P. (2001). An online prepurchase intentions model: the role of intention to search: best overall paper award—the Sixth Triennial AMS/ACRA Retailing Conference, 2000? J. Retail. 77, 397–416. doi: 10.1016/S0022-4359(01)00051-3
Smith, C. A., and Ellsworth, P. C. (1985). Patterns of cognitive appraisal in emotion. J. Pers. Soc. Psychol . 48:813.
Tandon, A., Aakash, A., and Aggarwal, A. G. (2020). Impact of EWOM, website quality, and product satisfaction on customer satisfaction and repurchase intention: moderating role of shipping and handling. Int. J. Syst. Assur. Eng. Manage. 54, 1–8. doi: 10.1007/s13198-020-00954-3
Tandon, U. (2021). Predictors of online shopping in India: an empirical investigation. J. Market. Anal. 9, 65–79. doi: 10.1057/s41270-020-00084-6
Tandon, U., Kiran, R., and Sah, A. N. (2016). Understanding online shopping adoption in India: unified theory of acceptance and use of technology 2 (UTAUT2) with perceived risk application. Serv. Sci. 8, 420–437. doi: 10.1287/serv.2016.0154
Tarhini, A., Alalwan, A. A., Al-Qirim, N., and Algharabat, R. (2021). “An analysis of the factors influencing the adoption of online shopping,” in Research Anthology on E-Commerce Adoption, Models, and Applications for Modern Business (Pennsylvania: IGI Global), 363–384.
Tarofder, A. K., Nikhashemi, S. R., Azam, S. F., Selvantharan, P., and Haque, A. (2016). The mediating influence of service failure explanation on customer repurchase intention through customers satisfaction. Int. J. Qual. Serv. Sci. 4:44. doi: 10.1108/IJQSS-04-2015-0044
Taylor, S. A., and Baker, T. L. (1994). An assessment of the relationship between service quality and customer satisfaction in the formation of consumers' purchase intentions. J. Retail. 70, 163–178. doi: 10.1016/0022-4359(94)90013-2
Teo, T., Lee, C. B., Chai, C. S., and Wong, S. L. (2009). Assessing the intention to use technology among preservice teachers in Singapore and Malaysia: a multigroup invariance analysis of the technology acceptance model (TAM). Comput. Educ. 53, 1000–1009. doi: 10.1016/j.compedu.2009.05.017
Thibaut, J. W., and Kelley, H. H. (2017). The Social Psychology of Groups . Routledge. doi: 10.4324/9781315135007
Torres, E. N., Milman, A., and Park, S. (2019). Customer delight and outrage in theme parks: a roller coaster of emotions. Int. J. Hospital. Tour. Administr. 16, 1–23. doi: 10.1080/15256480.2019.1641455
Tsiros, M., and Mittal, V. (2000). Regret: a model of its antecedents and consequences in consumer decision making. J. Consum. Res. 26, 401–417. doi: 10.1086/209571
Tzeng, S. Y., Ertz, M., Jo, M. S., and Sarigöll,ü, E. (2021). Factors affecting customer satisfaction on online shopping holiday. Market. Intell. Plann. 8:346. doi: 10.1108/MIP-08-2020-0346
Ul Haq, J., and Bonn, M. A. (2018). Understanding millennial perceptions of human and nonhuman brands. Int. Hospital. Rev . 9:14. doi: 10.1108/IHR-09-2018-0014
Unal, S., and Aydin, H. (2016). Evaluation of consumer regret in terms of perceived risk and repurchase intention. J. Glob. Strateg. Manage. 2, 31–31. doi: 10.20460/JGSM.20161024354
Venkatesh, V., Thong, J. Y., and Xu, X. (2012). Consumer acceptance and use of information technology: extending the unified theory of acceptance and use of technology. MIS Q. 157–178. doi: 10.2307/41410412
Wai, K., Dastane, O., Johari, Z., and Ismail, N. B. (2019). Perceived risk factors affecting consumers' online shopping behaviour. J. Asian Financ. Econ. Bus. 6, 246–260. doi: 10.13106/jafeb.2019.vol6.no4.249
Wang, C. Y., and Mattila, A. S. (2011). A cross-cultural comparison of perceived informational fairness with service failure explanations. J. Serv. Market . 25, 429–439. doi: 10.1108/08876041111161023
Wang, X. (2011). The effect of unrelated supporting service quality on consumer delight, satisfaction, and repurchase intentions. J. Serv. Res. 14, 149–163. doi: 10.1177/1094670511400722
Westbrook, R. A., and Oliver, R. L. (1991). The dimensionality of consumption emotion patterns and consumer satisfaction. J. Consum. Res. 18, 84–91. doi: 10.1086/209243
Whelan, J., and Dawar, N. (2014). Attributions of blame following a product-harm crisis depend on consumers' attachment styles. Mark. Lett. 27, 285–294. doi: 10.1007/s11002-014-9340-z
Woodside, A. G., Frey, L. L., and Daly, R. T. (1989). Linking service quality, customer satisfaction, and behavio. Mark. Health Serv. 9:5. doi: 10.1016/S0022-4359(01)0009-5
Wu, R., and Wang, C. L. (2017). The asymmetric impact of other-blame regret versus self-blame regret on negative word of mouth: empirical evidence from China. Eur. J. Market. doi: 10.1108/EJM-06-2015-0322
Yang, Y., Gong, Y., Land, L. P. W., and Chesney, T. (2020). Understanding the effects of physical experience and information integration on consumer use of online to offline commerce. Int. J. Inf. Manage. 51:102046. doi: 10.1016/j.ijinfomgt.2019.102046
Yap, B. W., and Khong, K. W. (2006). Examining the effects of customer service management (CSM) on perceived business performance via structural equation modelling. Appl. Stochast. Models Bus. Indus. 22, 587–605. doi: 10.1002/asmb.648
Zeelenberg, M., and Pieters, R. (2007). A theory of regret regulation 1.0. J. Consum. Psychol. 17, 3–18. doi: 10.1207/s15327663jcp1701_3
Zeithaml, V. A., Berry, L. L., and Parasuraman, A. (1996). The behavioral consequences of service quality. J. Market. 60, 31–46. doi: 10.1177/002224299606000203
Zhang, H. (2017). Understanding the Consumption Experience of Chinese Tourists: Assessing the Effect of Audience Involvement, Flow and Delight on Electronic Word-of-mouth (eWOM) (Doctoral dissertation).
Zhang, Z., Ye, Q., Song, H., and Liu, T. (2015). The structure of customer satisfaction with cruise-line services: an empirical investigation based on online word of mouth. Curr. Issues Tourism . 18, 450–464.
Keywords: consumer perception, online shopping, actual experiences, customer satisfaction, direct shopping, perceived risk, delight, outrage
Citation: Rao YH, Saleem A, Saeed W and Ul Haq J (2021) Online Consumer Satisfaction During COVID-19: Perspective of a Developing Country. Front. Psychol. 12:751854. doi: 10.3389/fpsyg.2021.751854
Received: 02 August 2021; Accepted: 30 August 2021; Published: 01 October 2021.
Reviewed by:
Copyright © 2021 Rao, Saleem, Saeed and Ul Haq. This is an open-access article distributed under the terms of the Creative Commons Attribution License (CC BY) . The use, distribution or reproduction in other forums is permitted, provided the original author(s) and the copyright owner(s) are credited and that the original publication in this journal is cited, in accordance with accepted academic practice. No use, distribution or reproduction is permitted which does not comply with these terms.
*Correspondence: Junaid Ul Haq, junaid041@yahoo.com
Disclaimer: All claims expressed in this article are solely those of the authors and do not necessarily represent those of their affiliated organizations, or those of the publisher, the editors and the reviewers. Any product that may be evaluated in this article or claim that may be made by its manufacturer is not guaranteed or endorsed by the publisher.

An official website of the United States government
The .gov means it’s official. Federal government websites often end in .gov or .mil. Before sharing sensitive information, make sure you’re on a federal government site.
The site is secure. The https:// ensures that you are connecting to the official website and that any information you provide is encrypted and transmitted securely.
- Publications
- Account settings
Preview improvements coming to the PMC website in October 2024. Learn More or Try it out now .
- Advanced Search
- Journal List
- Elsevier - PMC COVID-19 Collection

The impact of COVID-19 on the evolution of online retail: The pandemic as a window of opportunity
Levente szász.
a Faculty of Economics and Business Administration, Babeș-Bolyai University, 400591, Cluj-Napoca, Teodor Mihali str, 58-60, Romania
Csaba Bálint
b National Bank of Romania, 030031, Bucharest, Lipscani str. 25, sector 3, Romania
Ottó Csíki
Bálint zsolt nagy, béla-gergely rácz, dénes csala.
c Department of Engineering, Lancaster University, Engineering Building, Lancaster University, Lancaster, LA1 4YW, United Kingdom
d Economics Observatory, School of Economics, University of Bristol, Beacon House, Queens Road, Bristol, BS8 1QU, United Kingdom
Lloyd C. Harris
e Alliance Manchester Business School, University of Manchester, Booth St W, Manchester, M15 6PB, United Kingdom
Associated Data
Data will be made available on request.
Pandemic-related shocks have induced an unexpected volatility into the evolution of online sales, making it difficult for retailers to cope with frequently occurring, drastic changes in demand. Relying on a socio-technical approach, the purpose of this paper is to (a) offer a deeper insight into the driving forces of online sales during the pandemic, and (b) investigate whether pandemic-related shocks accelerate the long-term growth of online retail. Novel, high-frequency data on GPS-based population mobility and government stringency is used to demonstrate how time spent in residential areas and governmental restrictions drive the monthly evolution of online sales in 23 countries. We deconstruct these effects into three main phases: lure-in, lock-in, and phase-out. Lastly, using time series analysis, we show that the pandemic has induced a level shift into the long-term growth trend of the online retail sector in the majority of countries investigated.
1. Introduction
The outbreak of the pandemic caused by the spread of a novel type of coronavirus, SARS-CoV-2, has induced an unprecedented shock to the global economy in terms of its speed and encompassing nature, having a significant impact on virtually all countries and economic sectors. During the pandemic, businesses and consumers have been forced continuously to adapt to the immediate and drastic changes brought about by this crisis. Furthermore, there is a general consensus that there will be long lasting global effects and the world economy will return to a “ new normal ” ( Roggeveen and Sethuraman, 2020 ; Sneader and Singhal, 2021 ).
As with similar health-related and economic crises in the past, it is widely accepted that online retail represents a sector that plays a crucial role ( Li et al., 2020 ; Guthrie et al., 2021 ), providing vital access for customers to essential products ( Kirk and Rifkin, 2020 ; Martin-Neuninger and Ruby, 2020 ). Given its significant role, the present paper focuses on the evolution of online retail during the COVID-19 pandemic and analyses the short-term and potential long-lasting effects of this crisis.
Most of the existing papers studying the interaction between the early-stage of the pandemic and the online retail sector report that in several countries the outbreak of COVID-19 led to an unprecedented surge in online retail demand (e.g., Gao et al., 2020 ; Hobbs, 2020 ; Hwang et al., 2020 ). These observations are supported by commentators suggesting that in 2020 the “share of e-commerce in retail sales grew at two to five times the rate before COVID-19” ( Lund et al., 2021 ). However, only a few studies acknowledge that, beyond the general upswing, the pandemic has increased the volatility of online sales evolution. Furthermore, literature offers little guidance on which factors can explain these changes in online sales during a crisis when traditional market mechanisms do not function as usual. In response, therefore, this paper aims to use large-scale, longitudinal data covering 23 different countries and multiple waves of the pandemic to investigate the drivers of short-term online retail evolution during COVID-19 .
While some researchers have tentatively begun to explore these short-term effects (e.g., Chang and Meyerhoefer, 2021 ; Eger et al., 2021 ), the longer-lasting implications of the pandemic on the online retail sector have yet to be studied empirically. Most scholars emphasize the need to investigate whether the pandemic has truly altered the evolution trajectory of online retail or if the current crisis is merely a single shock after which the sector will return to its traditional evolutionary path as consumers and retail businesses return to their “old habits” in the post-pandemic period ( Sheth, 2020 ; Eger et al., 2021 ; Reardon et al., 2021 ; Schleper et al., 2021 ). Consequently, given the uncertainty of what the “ new normal ” might bring for the online retail market, this paper also intends to use the most recent time series to investigate whether the pandemic has altered the long-term evolution of the sector .
In pursuing the two objectives (investigation of short-term drivers and long-term trend implications), this paper adopts Geels’ (2002) multi-level perspective (MLP) as a theoretical lens to investigate technological transitions in a complex socio-technical context. We interpret the pandemic as a force capable of opening a “window of opportunity” ( Dannenberg et al., 2020 ). Such windows constitute powerful tensions created at the level of the socio-technical landscape that bring a unique possibility for a technological novelty to break through and become more dominant in mass markets ( Geels, 2004 ). Hence, we explore the interplay between the window of opportunity opened by COVID-19 and the growth of the online retail sector. More specifically, we aim to investigate (a) the short-term driving forces behind the exponential evolution of the online retail sector during the pandemic, and (b) whether the pandemic has truly created a window of opportunity for a positive shift in the long-term evolution of online retail. Along with pursuing these objectives we also aim to provide a theoretical contribution to the literature on windows of opportunity, a central concept that has received only limited attention in previous MLP studies ( Geels, 2011 ; Dannenberg et al., 2020 ). In this regard, our paper aims to offer a more detailed insight into how a technological transition path might behave during such a period and to provide a means to evaluate the potential long-term effect of windows of opportunity.
2. Literature review
2.1. the impact of covid-19 on online retail.
Given the crucial role of online retail channels during a pandemic, researchers have examined a variety of ways in which COVID-19 has influenced online shopping. As COVID-19 was first identified in China, initial studies investigated how the outbreak of the crisis has reshaped the retail landscape in China with emphasis on the increasing importance of online channels ( Gao et al., 2020 ; Guo et al., 2020 ; Hao et al., 2020 ; Li et al., 2020 ; Jiang and Stylos, 2021 ). These studies focused on how the outbreak of the pandemic influenced online shopping ( Gao et al., 2020 ; Guo et al., 2020 ), and how online channels helped the population to cope with the emerging health-crisis ( Li et al., 2020 ; Hao et al., 2020 ).
Given the narrow focus of initial studies, authors called for further research in other countries better to understand the global impact of the pandemic on online retail ( Gao et al., 2020 ; Li et al., 2020 ; Jiang and Stylos, 2021 ). Subsequent studies taking this research avenue offered a good cross-section globally by covering multiple different countries but investigated almost exclusively the short-term impacts of COVID-19 on online retail, using data from the first wave of the pandemic ( Table 1 ). Moreover, observers typically argued that the major driving forces behind the exponential proliferation of online channel use in the context of COVID-19, can be grouped in two distinct, but intertwined categories: (a) governmental regulations and restrictions, and (b) pandemic-induced changes in customer behavior. In line with this observation, Shankar et al. (2021) also contend that “many shoppers move a large portion of their business online during the COVID-19 outbreak either by choice or due to regulation …” . Therefore, the next two subsections review the studies that attribute the changes in online sales to one of these two factors.
Summary of the literature on the impact of COVID-19 on the evolution of online retail.
2.1.1. Studies highlighting the impact of changing customer behavior
Adopting a behavioral perspective, Chang and Meyerhoefer (2021) illustrated how the first wave in Taiwan (where no strict stay-at-home orders or business closures were imposed) has shifted consumers’ attention towards online channels. In the early weeks of the pandemic the surge in the number of confirmed cases increased both sales and the number of customers of online food commerce. The change in customer behavior was also induced by the media, as COVID-19 related press articles and Google searches also positively correlated with online food sales.
In a similar manner, Sheth (2020) argued that the pandemic had several powerful and immediate effects on consumer behavior: while facing constraints, consumers improvised and replaced old habits with new ones, such as switching to online retail channels, enabling thereby the “store to come home”. In line with this, Jiang and Stylos (2021) proposed that individual pressures during lockdowns force consumers to create a “new retail purchasing normality” involving higher digital engagement and increased online purchases. Consultancy papers also supported this view. A multi-country survey conducted by McKinsey & Company demonstrated that the pandemic induced a major shift in consumer behavior, at least two thirds of customers having tried new, mostly online forms of shopping ( Sneader and Singhal, 2021 ).
In terms of shifting consumer behavior, Tran (2021) proposed that fear of the pandemic can also drive online purchasing intentions aiming to improve the health safety of the consumer and the surrounding community. Researchers focusing on the second wave of the pandemic ( Chopdar et al., 2022 ; Eger et al., 2021 ) also connected the fear of the virus to increased online shopping. One exception is identified by Mehrolia et al. (2021) , concluding that a considerable majority of Indian customers decided not to order food through online channels during the first wave of the pandemic due to the fear connected to food delivery.
Hao et al. (2020) focused on a different aspect of customer behavior. Their study points out that panic buying (i.e., ordering more than the short-term necessity of the household due to fear), which is a common consumer response during disasters, is more associated with online food retail channels than with traditional channels. Following this idea, Guthrie et al. (2021) use the react-cope-adapt model ( Kirk and Rifkin, 2020 ) to illustrate that during the first month of the pandemic in France consumers reacted by panic buying, dramatically increasing the online purchasing of essential products. This period was followed by coping with the crisis which led to an increase of online orders related to non-essential products. The adapt phase was supposed to show a sustained modification of online purchasing behavior. However, due to limited data available, the authors concluded that long-term behavior changes require further investigation.
2.1.2. Studies highlighting the impact of government regulations
During the pandemic, several governmental restrictions had an immediate impact on online retail. For example, Martin-Neuninger and Ruby (2020) and Hall et al. (2021) identify government-related factors, namely the lockdown period and travel restrictions, as primary reasons behind the surge in online shopping in New Zealand. Hobbs (2020) also argued that initial stay-at-home and distancing orders issued in Canada led to an uptake of the online food retail: while online grocery deliveries were already used by early adopters in the pre-pandemic era, during the outbreak many late-adopter customers tried this channel for the first time. Jílková and Králová (2021) reported similar phenomena in the Czech Republic for all generational cohorts. In summary, unexpected regulations imposed by governments determined an immediate increase in demand for online shopping: existing customers started to use online channels more frequently, while new customers, including older and less tech-savvy generations, turned to online channels for the first time ( Hwang et al., 2020 ; Pantano et al., 2020 ).
From the retailer’s perspective, Reardon et al. (2021) provided several case examples of Asian and Latin American food industry firms strengthening their e-commerce business models or reconfiguring their entire food supply chains as a response to early-stage lockdown policies. Based on a survey among small Belgian retailers, Beckers et al. (2021) found that restrictions have doubled online orders during the first wave of the pandemic. To match the increase in demand, half of the retailers not using online channels before the pandemic opened one during the first months of COVID-19. Based on a literature review, Kirk and Rifkin (2020) also predicted that in order to conform to social distancing regulations, online retail coupled with contactless distribution methods would substantially gain ground during the pandemic. However, results related to the long-lasting effects of the pandemic on online retail are still “speculative in nature” ( Hobbs, 2020 ). Many of the customers who made the shift due to the restrictions might continue to utilize online channels in the long run. Other customers might return to traditional channels as soon as possible ( Beckers et al., 2021 ; Mehrolia et al., 2021 ). Thus, whether online retail can capitalize on the pandemic in the long run is still a subject of debate.
2.1.3. Summary and research questions
A summary of the key studies is provided in Table 1 in chronological order, highlighting the short-term drivers (i.e., government regulations and/or customer behavior, beside the papers narrowly focusing on the effect of the pandemic itself) and potential long-term implications related to the growth of the online retail sector.
Based on the literature, we derive two main conclusions that serve as basis for our research questions. First, as demonstrated in Table 1 , there is a plethora of mostly anecdotal, non-empirically-based evidence that during the pandemic (and beside the pandemic itself) two major factors, i.e., government restrictions and consumer behavior changes, drove a significant initial surge in online shopping. Second, extant studies failed to offer insights into how these factors drive online sales during the entire period of the current pandemic ( Schleper et al., 2021 ). Therefore, we cover the full period of COVID-19 to date and provide more conclusive empirical evidence on how these two factors influence the evolution of online retail.
RQ1. How do changes in customer behavior and government regulations drive the evolution of online retail during the pandemic?
Moreover, the long-term implications of this change in online retail use have remained, so far, a subject of anecdotal speculation ( Table 1 ). However, changes to the retail sector might become a constant in the “ new normal ”, and further research is needed “to understand the short-term and long-term impact of the pandemic on consumer behavior and provide guidance on how retailers should cope with those changes” ( Roggeveen and Sethuraman, 2020 ). Hobbs (2020) suggested that COVID-19 prompted sceptics and late-adopters to use online retail channels, and these new customers are likely to continue to shop online even after the pandemic. More cautious voices, however, asked the question whether the pandemic has “swung the pendulum too far and too fast towards online shopping” ( Gauri et al., 2021 ), which may potentially result in an unsustainable boost to online retail. Thus, the extent to which this shift will lead to a fundamental leap in the long-term role of online retailing is unknown.
RQ2. What trend-shifting impact does the pandemic have on the long-term evolution of online retail?
In answering RQ1 and RQ2 we also aim to extend the scope of existing research ( Table 1 ) in four different aspects. Given that COVID-19 is a global phenomenon, we aim to cover a larger geographical region compared to the majority of previous studies focusing on a single country. Second, in contrast with existing research mostly investigating a single branch of the online retail sector, we propose to analyze the online retail sector as a whole, covering the sales of all types of products. Third, we integrate novel measures into the analysis that have emerged during this pandemic (mobility indicators, government stringency index) to be able better to explain the evolution of the online retail sector during this crisis. Fourth, we investigate a longer period before and during the pandemic than previous studies to infer long-term implications.
2.2. A socio-technical approach to study the evolution of online retail during COVID-19
The multi-level perspective (MLP) has been established as insightful in studying COVID-19 related developments in the online retail sector ( Dannenberg et al., 2020 ). Consequently, we use the MLP as a theoretical lens to study the short and long-term evolution of online retail. Geels (2002) argues that the central tenet of MLP is that technological transitions are not only dependent on the development of the technology itself, but also pivot on the broader socio-technical context. In line with this view, technological transition represents a change from one socio-technical configuration (regime) to other: beyond the substitution of an older technology with a newer one, such transitions include changes in other socio-technical dimensions such as infrastructures, policies, user practices, and markets ( Geels, 2002 , 2004 ).
According to the MLP, technological transitions are shaped by the interaction between developments unfolding on three analytical levels ( Geels, 2002 , 2004 , 2011 ):
- • Technological niches represent the micro-level of the MLP. Niches are quasi-protected spaces where radical innovations are developed (e.g., R&D laboratories, subsidized development projects, or specific user categories supporting emerging innovations). They are unstable socio-technical configurations where innovations are carried out by a limited number of actors. Processes in the niche are gradually linked together and stabilize in time into a dominant design that allows for the radical innovation to break through to the next level.
- • Socio-technical regimes represent the meso-level of the MLP. Regimes refer to “the semi-coherent set of rules that orient and coordinate the activities of social groups” ( Geels, 2011 ) creating thereby a “deep structure” that ensures the stability of the current socio-technical system. Nevertheless, the semi-coherence of these rules allows for a dynamic stability which enables further incremental innovation, with small adjustments accumulating into stable technological transition paths. A socio-technical regime is formed by the co-evolution of different sub-regimes, each with its own set of rules and dynamics: user and market, technological, science, policy, and socio-cultural sub-regimes. According to Geels (2004) , the socio-technical regime can be understood as the meta-coordination of the different sub-regimes that determines technology adoption and use.
- • The socio-technical landscape represents the macro-level of the MLP. The landscape provides a wider, technology-external context for the interactions of actors within the niche and the socio-technical regime. Actors cannot influence elements of the landscape on the short-run, and changes at the landscape level take place usually slowly, representing longer-term, deep structural tendencies (e.g., macroeconomic processes, cultural patterns, political trends).
An important implication of the MLP is that the future evolution of a (new) technology does not only depend on the processes within the niche, but also on the interactions between different levels; including the regime and landscape levels. Geels and Schot (2007) contend that the general pattern of technology transition involves all three levels: (1) niche innovations align and gain internal momentum, (2) landscape developments put pressure on existing regimes, and (3) regimes destabilize creating an opportunity for niche innovations to break through to mass markets.
In terms of the interplay between COVID-19 and online retailing, another important concept linked to the MLP is the “window of opportunity”. Geels (2002) argues that windows of opportunity are created when tensions appear in the current socio-technical regime or when landscape developments put a pressure on the current regime for internal restructuring. These tensions loosen the rules of the socio-technical regime and create opportunities for technologies to escape the niche-level and become more deeply embedded in the regime. Competition with the existing technology becomes more intensive, triggering wider changes in the regime, where the new technology may replace the old one in the long run ( Geels, 2004 ).
Dannenberg et al. (2020) conclude that COVID-19 represents a critical landscape development that puts pressure on the socio-technical configuration of the retail sector. In line with our literature review, they suggest that two sub-regimes were particularly affected: policy regime (government regulations) and, user and market regime (sudden change in customer behavior). The authors further argue that these two major changes have opened a window of opportunity for online grocery retail to gain substantial market share. In this regard, RQ1 aims to investigate how the developments within these two dimensions influence the evolution of the online retail sector during the opening up of a window of opportunity ( Fig. 1 ). Given that, to date, the MLP offers little insight into the evolution of a technology during a window of opportunity ( Dannenberg et al., 2020 ), answering RQ1 should enrich this theoretical framework by explicating the forces that drive technology transitions during tensions in the landscape and the socio-technical regime (i.e., during a window of opportunity).

COVID-19 and the trajectory of online retail evolution (adapted from: Geels, 2002 ; Dannenberg et al., 2020 ).
Concerning the long-term impact of this window of opportunity, we investigate whether it enables the online retail sector to gain a significantly higher share of the whole retail sector on the long run (technology trajectory in Fig. 1 ) to the detriment of offline channels ( Helm et al., 2020 ). However, in the long run, MLP is not necessarily about mapping “winning” technologies that entirely replace/reconfigure existing regimes: it is just as possible that the breakthrough of a new technology will lead to a symbiosis with incumbent socio-technical regimes ( Geels, 2002 ; Genus and Coles, 2008 ). Thus, in our case, the question is more about the relative share of online retail and physical retail within the retail sector (cf. omnichannel retailing, Gauri et al., 2021 ). Beside speculation, current literature offers little guidance in this regard. Dannenberg et al. (2020) suggest that even if the pandemic has led to an upswing of online shopping, there is no indication for a fundamental long-term shift from physical to online retail. The authors, however, base their assumptions on a limited set of data, both from a temporal (March–May 2020) and from a geographical/sectoral perspective (German grocery retail). On the other hand, many other authors advocate a breakthrough of online retail as a result of taking advantage of the window of opportunity created by the pandemic (e.g., Chang and Meyerhoefer, 2021 ; Hobbs, 2020 ; Tran, 2021 ). Answering RQ2 is designed to explicate and illuminate further this debate.
3. Data and variables
3.1. data used in short-term analysis (rq1).
To investigate RQ1, we use as dependent variable the monthly evolution of online retail sales during the pandemic (Feb 2020–Jan 2022) in European countries. We rely on Beckers et al. (2021) who define online retail channel use as the selling of goods via mail, phone, website, or social media. Therefore, we adopt NACE-level retail trade data published by Eurostat using the index of deflated turnover (i.e., turnover in real terms, 2015 = 100) for the “Retail sale via mail order houses or via Internet” sector. Seasonally and calendar adjusted time series data is used to assess the monthly changes during the pandemic in this sector, shortly denoted from now on “online retail” ( ΔOnline_retail ). In terms of countries, the Eurostat database was deemed the most suitable to study our research questions as it provides online retail data for 23 European countries (20 countries of the European Union, plus Norway, UK, and Turkey, covering thereby all major economies from Europe). This sample offers a rich variety of pandemic-related contexts: each of these countries was hit by the pandemic to a different extent and the reaction of authorities was also fairly diverse ( Hale et al., 2021 ). Fig. 2 illustrates the evolution of the ΔOnline_retail variable in these countries.

Monthly changes in online retail turnover during the pandemic in the countries investigated.
To investigate this volatile evolution, two novel measures are used as explanatory variables that have been introduced recently as a response to the need to track social phenomena more frequently and more precisely during the pandemic.
The first variable is a proxy of changes in general customer behavior: population mobility . Shankar et al. (2021) argue that during a period characterized by dramatic and frequent changes in shopping behaviors, high-frequency, mobile GPS data can offer better information for retailers. Therefore, we integrate into our analysis the mobility data provided by Google® through their Community Mobility Reports ( Google, 2021 ), comprising several types of mobilities grouped by the destination/location of the mobility. Based on Beckers et al. (2021) who argue that COVID-19 has temporarily put an end to hypermobility cutting short consumers’ physical range around their homes, we select the residential component ( ΔResidential ) from the different forms of mobility, arguing that the changes in residential mobility (i.e., amount of time spent at home) could be the strongest component to explain changes in online shopping. Given that there might be some time needed for online shopping behavior to adjust to changes in mobility, the one-month lagged version of the variable is also used in our model ( ΔResidential(-1) ).
The second explanatory variable incorporated in our analysis is related to government restrictions . We use data from the Oxford COVID-19 Government Response Tracker, more precisely the values of the COVID-19 Stringency Index which aggregates the stringency of lockdown-type governmental measures, such as school closures, travel restrictions, bans on public gatherings, workplace closures, etc. ( Hale et al., 2021 ). This represents the most suitable proxy to measure the type of regulations connected by previous literature to online channel use during the pandemic ( Table 1 ). The index provides a multi-country panel of daily frequency, measured as a percentage value; 100% representing the highest level of stringency. To match the frequency of the dependent variable, the monthly change of the index is computed as explanatory variable ( ΔGovernment_stringency ). The one-month lagged variant is also introduced in the analysis ( ΔGovernment_stringency(-1) ).
Beside the two novel explanatory variables generated during the pandemic, we integrate several control variables in our analysis. These variables assess the income and purchasing power of the population (GDP/capita and unemployment level in each country), the level of urbanization (density of the population in each country), the level of education (percentage of the population attending tertiary education), the pervasiveness of online channels (Internet penetration), and the actual pervasiveness of online shopping (Online retail share in the retail sector) ( Hortaçsu and Syverson, 2015 ). Data for all countries analyzed are retrieved from the Eurostat database. The unemployment variable has a monthly frequency ( Δ Unemployment ), while the other variables ( GDP/capita, Internet penetration, Tertiary education, Population density, Online retail share ) change on a yearly basis. Descriptive statistics for the monthly variables are provided in Table 2 . The correlation matrix is included in Appendix A.
Descriptive statistics of the main variables included in the short-term analysis.
3.2. Data used in long-term analysis (RQ2)
To evaluate the trend-shifting potential of the pandemic in the online retail sector, the same retail trade data is used as for the short-term analysis, covering however a longer period of time between Jan 2000 and Jan 2022 ( Online_Retail ). To offer an overview of the long-term evolution of our focal variable, we present a boxplot containing data for all countries aggregated to annual averages, normalized on a 0–100 scale ( Fig. 3 , left). Primary visual inspection suggests that two periods can be distinguished in terms of the dynamism of the sector (2000–2010 characterized by slower growth pace versus 2011–2021 showing stronger momentum), while the relatively higher values of the last two boxplots indicate that it is beneficial to investigate whether the pandemic has induced a level shift into the evolution of online retail.

Long-term evolution of online retail turnover (left) and online retail market share (right) in the countries investigated (normalized: min = 0, max = 100).
Furthermore, to assess whether the online retail sector could exploit the window of opportunity opened by the pandemic, we compute another variable as a proxy measuring the share of online retail in total retail sales. For this purpose, we calculate the ratio between the indices of deflated turnover of online retail and the “Retail trade, except of motor vehicles and motorcycles” sector, this latter being a proxy for total retail sales ( Online_Retail_Ratio ≈ Online_Retail/Total_Retail ) ( Fig. 3 , right). The ratio approach is also consistent with theory (symbiotic technologies: Geels, 2002 ) and previous research ( Hortaçsu and Syverson, 2015 ).
4. Analysis and results
4.1. short-term analysis (rq1), 4.1.1. panel regression analysis.
To illuminate the impact of mobility and government restrictions on the monthly evolution of online sales, we have elected to implement a panel regression model. We have performed three random-effects and three cross-section fixed-effects panel regressions. We opted for the panel specification because it enables us to harness the rich structure of our data and to account for the unobserved heterogeneity present in the data. We perform 2 × 3 = 6 regressions because of the different methodology (fixed vs. random effects), and the 3 combinations resulting from including only the government stringency variables, only the residential mobility variables, and both. Five control variables were nearly collinear in the fixed effects case; therefore Table 3 presents only the estimates for these variables in the random effects case. Our main specification is the following:
where C i j t and β ( C ) j are the independent variables and their coefficients, i is the index of countries, t of time, and j of the equation variables.
Regression models.
Notes: t-values in parentheses; *significant at 0.05; **significant at 0.01.
Results of the fixed effects specifications of our panel regression model (equations 1 to 3) indicate that our first variable of interest, residential mobility, and its one-period lag, have a significant impact on the monthly change in online retail sales, both variables having the expected positive sign. The same can be pointed out for the government stringency and lag variables. However, when we include both residential mobility and government stringency, only the first remains significant, due to high collinearity between the two explanatory variables. The results are similar in the random effects case (equations 4 to 6). The goodness-of-fit statistics (adjusted R-squared, F-statistic) are quite high for panel regressions, indicating that the explanatory variables introduced in the panel explain a large proportion of the variation of the monthly change in online retail sales.
Thus, results altogether indicate that both residential mobility and government stringency are significant predictors of online retail channel use: as residential mobility increases (i.e., people spend more time at home) and, alternatively, as government stringency increases (i.e., anti-COVID-19 measures become stricter) the use of online retail channels increases. Furthermore, the impact of all control variables is insignificant, meaning that mobility and government stringency indicators provide a better explanation for the variation of online retail sales during the pandemic than traditional variables that have been used to explain the evolution of the online retail sector in pre-pandemic periods.
4.1.2. Detailed analysis of short-term effects
While panel regression results show that both residential mobility and government stringency are good predictors of the evolution of online sales, relationships between variables are rarely perfectly linear. Therefore, we provide a more detailed analysis on the interplay between these variables. Fig. 4 illustrates the monthly evolution of online sales (vertical axis) together with the monthly percentage change in residential mobility (horizontal axis) for the entire period of the pandemic, each dot representing one country in one month.

Monthly evolution of online sales and residential mobility during the pandemic in the countries investigated.
Beside the general positive relationship between the two variables, the scatter plot also indicates that three different forces can be identified that shape the evolution of online retail sales during the pandemic. First, there are periods in which mobility is restricted more and more to residential areas, and consumers adapt by significantly increasing their monthly spending on online retail channels (as high as +30–50% during the first wave of the pandemic). This process is exactly what was expected during the pandemic: as the mobility range of people is restricted primarily to their homes, they turn to online retail channels more frequently. This process is termed the “lure-in” phase. Typical months during which the lure-in phase was dominant were Mar 2020, Apr 2020, Oct–Nov 2020, Nov 2021, and Jan 2022 ( Fig. 5 ).

Monthly evolution of online sales and residential mobility during different phases of the pandemic in the countries investigated.
However, it is also observable that when consumers are not confined to residential areas and start increasing their mobility outside their homes (i.e., residential mobility decreases), a decrease in online spending does not follow automatically, as people tend to continue to use, or even increase the usage of, online retail channels. Additionally, in many cases a large drop in residential mobility is paired with no significant change in online retail sales. These cases are labelled as the “lock-in” phase, which means that temporarily consumers remain users of online channels even if their mobility would allow them to use offline channels more intensively. Thus, mobility restrictions have an immediate (lure-in), but also a lagged (lock-in) impact on online retail channel use, in line with the significance of lagged variables in our panel regression model ( Table 3 ). The most typical months in which several European countries went through this lock-in phase were May 2020, Jun 2020, Feb 2021, Mar 2021 ( Fig. 5 ). This phase is not as consistent on a monthly basis as the lure-in phase, several countries experiencing a negative change in online channel use, concurrently with the decrease of residential mobility.
Lastly, there is also a “phase-out” period denoting cases where online retail use decreases, while time spent at home generally decreases. During these months a part of the former online shopping volume of customers is most probably replaced by (or allocated back to) offline channels. Furthermore, in some rare instances residential mobility has a slight increase, while consumers still decrease their online spending. Predominantly phase-out months include Jul 2020, May–Jul 2021, Dec 2021 ( Fig. 5 ).
The same three phases can be observed if the residential mobility indicator on the vertical axis is replaced by the government stringency index ( Fig. 6 , Fig. 7 ). In summary, there is a clear lure-in phase which was noticeable especially during the beginning of the first and second wave of the pandemic (Mar–Apr, 2020; Oct–Nov 2020): sudden drops in mobility and severe governmental restrictions clearly prompt customers to shop online. This effect has some “stickiness” (lock-in phase) because as governmental restrictions are eased, certain customers continue to use (or even increase the use of) online retail channels. Nevertheless, after a relatively short period the lock-in effect fades and customers drop their online shopping volume significantly (phase-out), countervailing to some extent the argument of the pandemic-induced upward boost of the online retail sector. Thus, while illuminating in other respects, this analysis, in itself, is unhelpful regarding the longer-term implications of the pandemic for the online retail sector. The next section aims to address this deficiency.

Monthly evolution of online sales and government stringency during the pandemic in the countries investigated.

Monthly evolution of online sales and government stringency during different phases of the pandemic in the countries investigated.
4.2. Long-term analysis (RQ2)
To investigate the potential trend-shifting impact of the pandemic in the online retail sector, a two-step approach is applied. First, to establish a basis for comparison, we analyze the 20-years trend of the sector without considering the specific effect of the pandemic. Second, based on the long-term trend established, we focus on the period of the pandemic, and use outlier detection methods to estimate whether the pandemic has induced a level shift in the long-term trend of the sector.
4.2.1. Long-term trend analysis
Online retail sales and online retail market shares show an increasing tendency during the last 20+ years ( Fig. 3 ). While the retail sector as a whole had a slight increasing tendency during this period, the average annual growth rate of the online retail sector was clearly higher. This difference is most visible during the last ten years when the online retail sector has been constantly on an increasing trajectory, thereby raising its market share within the total retail sector. Thus, the online retail sector has been benefitting from continuous market share gains with a relatively lower growth pace in the early period (2001–2010), and with rapid increases in the last period (2011–2021). These differences are illustrated in Fig. 8 .

Average annual growth rates in the retail sector in European countries (%).
Next, we use unit root tests to statistically demonstrate that there is an underlying long-term growth trend in the data ( Chatfield and Xing, 2019 ), both in terms of monthly online retail turnover ( Online_Retail ) and in terms of online retail market share ( Online_Retail_Ratio ). Applying the most widely used Augmented Dickey-Fuller (ADF) test, we aim to show that there is a systematic, persistent stochastic trend in the time series (i.e., an upward tendency in our case). Unit root test results confirm that in most of the countries investigated the null hypothesis of one unit root cannot be rejected: the p-values are above 0.05 in 23 cases out of 24 in case of the Online_Retail variable and in 21 cases out of 24 for Online_Retail_Ratio . Thus, for the vast majority of countries neither Online_Retail , nor Online_Retail_Ratio is stationary, indicating that there is an (upward) long-term stochastic trend in the time series. Furthermore, unit root test results also imply that any positive or negative shock (such as the pandemic) during the period investigated has a persistent effect on the trend. Nevertheless, further investigation is needed to determine whether this shock applies for the pandemic period as well.
4.2.2. Outlier detection during the pandemic
Outlier detection is used to determine whether the pandemic has caused a level shift in the Online_Retail , and especially in the Online_Retail_Ratio time series. For this purpose, we use ARIMA 1 models with specific dummy regressors on both time series, implemented in JDemetra+ which is a proprietary software developed by the National Bank of Belgium in cooperation with the Deutsche Bundesbank and Eurostat. The software has been officially recommended since 2015 to the members of the European Statistical System and the European System of Central Banks as a tool for seasonal adjustment and other connected time series issues, such as outlier detection. In general, outliers are represented by abrupt changes in a time series caused by unexpected natural or socioeconomic effects, such as the pandemic. Three main types of outliers can be identified ( Fig. 9 ): (a) additive outlier (AO), which changes the time series for one period only, returning to the original trend afterwards, (b) level shift (LS) that causes a permanent (upward or downward) change in the level of a time series, and (c) transitory change (TC) whose effect of changing the time series is faded out over a limited number of periods ( IMF, 2018 ). Here, we specifically look for LS type outliers: a positive LS would suggest that online retail turnover and its market share registered a sudden increase during the pandemic, and that therefore the pandemic has accelerated the underlying growth trend of online retail.

Level shift versus other outlier types (source: IMF, 2018 ).
JDemetra+ uses the traditional TRAMO 2 methodology ( Gómez and Maravall, 1996 ; Findley et al., 2017 ) where TRAMO is designed to perform outlier detection as well. 3 Although this is a widely used framework in economics and connected disciplines, its applications in retailing are quite scarce which offers us the possibility to shed additional light on the effect of the pandemic on the online retail sector. In particular, TRAMO uses regression models with ARIMA errors as follows:
where z t is the original data series, β = ( β 1 , … β n ) is a vector of regression coefficients, y t = ( y 1 t , … y n t ) represents n regression variables (in our case LS, AO and TC outliers), while x t is the disturbance that follows the general ARIMA process.
Using the TRAMO method, we analyze the full Jan 2000–Jan 2022 time period for outliers in each country involved in the analysis, complemented by the aggregated time series on the EU-27 level. Both Online_Retail and Online_Retail_Ratio time series were analyzed for all three types of outliers. However, in light of RQ2, only LS type outliers are listed in Table 4 that were identified during 2020. It should be noted that 2021 LS outliers are not (yet) taken into consideration here because they are situated at the end of our time series data (i.e., further data is needed by TRAMO to determine whether a 2021 LS will remain significant and persist in the long run). In contrast, LS outliers in 2020 have already proven that they induced a persistent upward shock into the long-term trend of the online retail sector. Table 4 lists all significant level shifts (p < .05) detected during 2020. Full results are presented in Appendix B .
Level shift (LS) detection during the pandemic.
The results of LS detection indicate that at the level of the EU-27, as well as in most of the countries investigated there was at least one positive LS in the online retail trend during the first year of the pandemic. This strongly suggests that COVID-19 has induced a boost both to online retail turnover and to its market share, supporting the window of opportunity concept. Out of the 23 countries analyzed, only 9 where had no significant LS. However, these cases represent smaller European countries, the largest ones (Germany, France, Italy, Spain, UK) all experiencing positive significant LSs. Furthermore, some of the countries (Italy, Lithuania, Norway) experienced multiple significant LSs during 2020 which further strengthens our conclusion related to the long-term implications of the pandemic. While there are two anomalous negative LSs in the Online_Retail_Ratio as well ( Table 4 ), we suggest that these do not contradict our results, as these are all overcompensated by multiple positive LSs in the same countries (Italy and Norway), the magnitude of which is significantly higher than that of the negative LSs. Nevertheless, these negative LSs could be a sign of a significant “phase-out” effect, as discussed in the short-term analysis.
5. Summary and discussion
Two important gaps were addressed in this paper: (RQ1) how can factors related to consumer behavior (mobility) and regulations (government stringency) explain the volatile evolution of online retail sales during the pandemic, and (RQ2) what long-term trend-shifting effects can be identified during the pandemic in the evolution trajectory of online retail.
First, our results confirm that the two indicators proposed to estimate changes in consumer behavior ( Residential mobility ) and in government regulations ( Government stringency ) can significantly explain the hectic short-term evolution of the online retail sector during the pandemic. Released for the first time during the pandemic, these two indicators are significantly above and beyond the explanatory power of traditional variables used to predict online channel use in pre-pandemic periods. The more people are confined to residential areas, and the stricter government restrictions are, the more customers turn to online channels. These results offer empirical support to previous studies that proposed that changes in mobility ( Shankar et al., 2021 ) and pandemic-related government regulations ( Hwang et al., 2020 ) could provide a better measure to estimate changes in online sales.
Second, using these newly introduced variables, our study goes beyond demonstrating the simple linear relationship between these variables and online retail turnover, to describe in more detail how online shopping habits change during the pandemic. This is a novel approach compared to existing studies that simply argue that the pandemic is linked to the increased use of online channels (e.g., Chang and Meyerhoefer, 2021 ; Hwang et al., 2020 ; Eger et al., 2021 ). Using government stringency and mobility data, we offer a more nuanced understanding of how online shopping behavior evolves during the different stages of the pandemic, an issue currently hotly debated in the literature ( Kirk and Rifkin, 2020 ; Guthrie et al., 2021 ; Schleper et al., 2021 ). Three different phases are distinguished in this paper: (1) a lure-in phase; (2) a temporary lock-in phase; and (3) a phase-out period. Furthermore, the same phases seem to repeat during different waves of the pandemic, starting with a strong lure-in phase, followed by a mix of lock-in and phase-out periods.
Third, using advanced outlier detection methods, we show that the faster growth trend that characterized online retail in the past decade has experienced a new positive level shift during 2020 in most of the countries investigated. In only a couple of months during the pandemic, online retail has gained extra market share against offline retail that in normal circumstances would have probably taken several years. Thus, our empirical findings confirm the predictions of some researchers (e.g., Chang and Meyerhoefer, 2021 ; Tran, 2021 ), and actively address the questions posed by other researchers (e.g., Sheth, 2020 ; Guthrie et al., 2021 ), by establishing that the pandemic has indeed induced a persistent upward shift into the growth trajectory of online retail. These level shifts were especially visible in the larger economies of Europe. Thus, our results are concordant with several other studies that suggest that many firms managed to quickly overcome infrastructural challenges and build up the necessary online capacities ( Guo et al., 2020 ; Beckers et al., 2021 ; Reardon et al., 2021 ), while customers will continue to use online retail channels more intensively in post-lockdown periods as well ( Hobbs, 2020 ; Eger et al., 2021 ; Hall et al., 2021 ). Even if some customers return to traditional shopping channels ( Hobbs, 2020 ; Sheth, 2020 ), our results indicate that for a large segment of customers the pandemic-induced shock outweighs the potential phase-out effect, shifting their long-term orientation towards online channels.
6. Conclusion
This paper analyzed short-term drivers (RQ1) and long-term implications of the pandemic (RQ2) in the online retail sector, relying on the MLP’s socio-technical approach as a theoretical lens. COVID-19 is operationalized within the MLP as an exogeneous landscape event that induced a shock on the regime level. This shock opened a window of opportunity for online retail to exponentially grow and significantly increase its share against traditional retail channels.
6.1. Theoretical implications
Our research shows that during a window of opportunity created by a landscape event, forces within the socio-technical regime that shape the long-term trajectory of a technology change radically. Geels and Schot (2007) argue that strong landscape pressures (such as a pandemic) destabilize actual socio-technical regimes creating tensions that open windows of opportunity for technologies to emerge. Our short-term analysis related to RQ1 offers additional insights into how these regime tensions function. Panel regression results indicate that during unstable periods (when windows of opportunity are created by landscape pressures), certain sub-regimes take over the force that shapes technological transitions, while other sub-regimes become negligible. In our study, the policy regime (strict government restrictions) and the user preferences and market regime (reorientation of shopping behaviors due to reduced mobility) were responsible for creating the tension on the regime-level. Conversely, other sub-regimes on the same level, such as technological regimes (e.g., technical infrastructure used in online retail), science regime (e.g., technical knowledge used to operate online transactions), and socio-cultural regimes (e.g., distrust of certain segments of the population in online retail), had no significant impact on the way online retail was evolving. Thus, we propose that windows of opportunity are created when one or more particular regimes exert pressures that take over the place of other regimes in creating the forces that shape technological transitions. When a window of opportunity is open, these new forces remain dominant and might even alter other regimes.
Second, our long-term analysis suggests that COVID-19 can be regarded as a shock-type landscape development that creates tension in the current socio-technical regime to create a window of opportunity for online retail. Results of our long-term analysis suggest that the quasi-stable socio-technical regime of the last decades enabled a gradual and constant growth of online retail in Europe, attaining continuously increasing market shares throughout the years. However, as the pandemic generated a window of opportunity for this sector, online retail was able to capitalize on this opportunity in most countries, receiving a significant boost to its previous growth tendency.
Third, as a more general research implication for retail, our study demonstrates that high-frequency indicators that emerged during the pandemic, such as data on population mobility and on government stringency can be used to better assess fundamental socio-economic processes during crises. These two types of indicators provide a more complex, real-time assessment of ongoing socio-economic processes, making them more suitable to make predictions or explain phenomena in a volatile context.
6.2. Practical implications
Through demonstrating that mobility and government stringency has a positive impact on the evolution of online sales, we offer an important tool to retail practitioners to monitor and anticipate potential large variations in online demand. While mobile GPS data has already been used to track retail store traffic, our analysis suggests that tracking customer movements outside brick-and-mortar stores can also provide an anchor during volatile times. Such high-frequency, near-real-time data could become the primary input for managers to keep up with sudden pandemic-related developments, and potentially with post-pandemic shopping behavior changes as well.
Online retailers that have already capitalized on this pandemic should also take into consideration that a sudden pandemic-related growth in sales could be followed by a temporary lock-in phase. However, retailers should continue to work on keeping (newly acquired) customers, as a phase-out period might rapidly occur. Conversely, our long-term analysis, suggests that actors in the online retail sector should expect that, on average, the phase-out effect is outweighed by the pandemic-induced boost in online sales, creating much potential on the long-run for online retailers to capture the benefits of the positive level shift in the growth trajectory of the sector.
6.3. Limitations and further research
A first set of limitations is related to the nature of data employed in our study. While Eurostat provides the most reliable macroeconomic data, comparable across countries, on the evolution of the (online) retail sector, aspects of the data were not ideal. Several countries had missing data on the most recent values of the online retail turnover index, and some European countries (e.g., Switzerland) could not be involved in our study at all. While all largest retail markets have been included in our sample, results of the study can nevertheless not be universally generalized beyond the 23 countries involved in the analysis.
In respect of GPS-based mobility and government stringency data, we have shown that these variables are suitable to explain the large variations in online retail sales during the pandemic. However, whether and to what extent these data can be used to keep up with developments in the online retail sector beyond the pandemic remains unknown but represents a promising direction for future research.
Another set of limitations stems from the results described in this paper. While our outlier detection could empirically demonstrate the pandemic-induced level shift in the long-term evolution of the online sector, statistically significant shifts were not observed in all the countries investigated. It remains an important future research avenue to explain why some countries, including the largest European economies, experienced level shifts during the pandemic, while others have not.
Lastly, this paper focused on the evolution of the online retail sector, explaining its volatile evolution during the pandemic and demonstrating how the sector could take advantage of the window of opportunity created by COVID-19. Our results could provide a starting point for investigating other technologies and solutions, such as video conferencing, home delivery or VR-solutions, to evaluate whether and to what extent they have capitalized on pandemic-induced opportunities, thereby shaping how the “ new normal ” might look like in a post-pandemic world.
Acknowledgement
This research was partially supported by the PN-III-P1-1.1-TE-2019-1773 research project for young independent research teams funded by UEFISCDI Romania.
1 Autoregressive Integrated Moving Average.
2 Time series Regression with ARIMA noise, Missing values and Outliers.
3 A comprehensive description of the procedure and its technical implementation in JDemetra+ is provided by Eurostat’s website .
Appendix A. – Correlation matrix
*significant at the p < .001 level.
Appendix B. Complete results of outlier detection
Outlier detection with TRAMO in the Online_Retail time series (Jan 2000–Jan 2022)
Content of cells: (a) type of outlier: LS – level shift, TC – transitory change, AO – Additive outlier; (b) month of occurrence in parentheses; (c) magnitude of outlier [t-value].
Outlier detection with TRAMO in the Online_Retail_Ratio time series (Jan 2000–Jan 2022)
Data availability
- Beckers J., Weekx S., Beutels P., Verhetsel A. COVID-19 and retail: the catalyst for e-commerce in Belgium? J. Retailing Consum. Serv. 2021; 62 [ Google Scholar ]
- Chang H.H., Meyerhoefer C.D. COVID‐19 and the demand for online food shopping services: empirical Evidence from Taiwan. Am. J. Agric. Econ. 2021; 103 (2):448–465. [ Google Scholar ]
- Chatfield C., Xing H. Chapman and hall/CRC; 2019. The Analysis of Time Series: an Introduction with R. [ Google Scholar ]
- Chopdar P.K., Paul J., Prodanova J. Mobile shoppers’ response to Covid-19 phobia, pessimism and smartphone addiction: does social influence matter? Technol. Forecast. Soc. Change. 2022; 174 [ Google Scholar ]
- Dannenberg P., Fuchs M., Riedler T., Wiedemann C. Digital transition by COVID‐19 pandemic? The German food online retail. Tijdschr. Econ. Soc. Geogr. 2020; 111 (3):543–560. [ PMC free article ] [ PubMed ] [ Google Scholar ]
- Eger L., Komárková L., Egerová D., Mičík M. The effect of COVID-19 on consumer shopping behaviour: generational cohort perspective. J. Retailing Consum. Serv. 2021; 61 [ Google Scholar ]
- Findley D.F., Lytras D.P., McElroy T.S. Detecting seasonality in seasonally adjusted monthly time series. Statistics. 2017; 3 [ Google Scholar ]
- Gao X., Shi X., Guo H., Liu Y. To buy or not buy food online: the impact of the COVID-19 epidemic on the adoption of e-commerce in China. PLoS One. 2020; 15 (8) [ PMC free article ] [ PubMed ] [ Google Scholar ]
- Gauri D.K., Jindal R.P., Ratchford B., Fox E., Bhatnagar A., Pandey A., Navallo J.R., Fogarty J., Carr S., Howerton E. Evolution of retail formats: past, present, and future. J. Retailing. 2021; 97 (1):42–61. [ Google Scholar ]
- Geels F.W. Technological transitions as evolutionary reconfiguration processes: a multi-level perspective and a case-study. Res. Pol. 2002; 31 (8–9):1257–1274. [ Google Scholar ]
- Geels F.W. From sectoral systems of innovation to socio-technical systems: insights about dynamics and change from sociology and institutional theory. Res. Pol. 2004; 33 (6–7):897–920. [ Google Scholar ]
- Geels F.W. The multi-level perspective on sustainability transitions: responses to seven criticisms. Environ. Innov. Soc. Transit. 2011; 1 (1):24–40. [ Google Scholar ]
- Geels F.W., Schot J. Typology of sociotechnical transition pathways. Res. Pol. 2007; 36 (3):399–417. [ Google Scholar ]
- Genus A., Coles A.M. Rethinking the multi-level perspective of technological transitions. Res. Pol. 2008; 37 (9):1436–1445. [ Google Scholar ]
- Gómez V., Maravall A. Banco de España. Servicio de Estudios; 1996. Programs TRAMO and SEATS: Instructions for the User (Beta Version: September 1996) [ Google Scholar ]
- Google L.L.C. 2021. Google COVID-19 Community Mobility Reports. https://www.google.com/covid19/mobility/ [ Google Scholar ]
- Guo H., Liu Y., Shi X., Chen K.Z. The role of e-commerce in the urban food system under COVID-19: lessons from China. China Agric. Econ. Rev. 2020; 13 (2):436–455. [ Google Scholar ]
- Guthrie C., Fosso-Wamba S., Arnaud J.B. Online consumer resilience during a pandemic: an exploratory study of e-commerce behavior before, during and after a COVID-19 lockdown. J. Retailing Consum. Serv. 2021; 61 [ Google Scholar ]
- Hale T., Angrist N., Goldszmidt R., Kira B., Petherick A., Phillips T., et al. A global panel database of pandemic policies (Oxford COVID-19 Government Response Tracker) Nat. Human Behav. 2021; 5 (4):529–538. [ PubMed ] [ Google Scholar ]
- Hall M.C., Prayag G., Fieger P., Dyason D. Beyond panic buying: consumption displacement and COVID-19. J. Serv. Manag. 2021; 32 (1):113–128. [ Google Scholar ]
- Hao N., Wang H., Zhou Q. The impact of online grocery shopping on stockpile behavior in Covid-19. China Agric. Econ. Rev. 2020; 12 (3):459–470. [ Google Scholar ]
- Helm S., Kim S.H., Van Riper S. Navigating the ‘retail apocalypse’: a framework of consumer evaluations of the new retail landscape. J. Retailing Consum. Serv. 2020; 54 [ Google Scholar ]
- Hobbs J.E. Food supply chains during the COVID‐19 pandemic. Can. J. Agric. Econ. 2020; 68 (2):171–176. doi: 10.1111/cjag.12237. [ CrossRef ] [ Google Scholar ]
- Hortaçsu A., Syverson C. The ongoing evolution of US retail: a format tug-of-war. J. Econ. Perspect. 2015; 29 (4):89–112. [ Google Scholar ]
- Hwang E., Nageswaran L., Cho S. 2020. Impact of COVID-19 on Omnichannel Retail: Drivers of Online Sales during Pandemic. SSRN 3657827. [ Google Scholar ]
- IMF . Statistics Department; 2018. Quarterly National Accounts Manual . International Monetary Fund. [ Google Scholar ]
- Jiang Y., Stylos N. Triggers of consumers’ enhanced digital engagement and the role of digital technologies in transforming the retail ecosystem during COVID-19 pandemic. Technol. Forecast. Soc. Change. 2021; 172 [ Google Scholar ]
- Jílková P., Králová P. Digital consumer behaviour and eCommerce trends during the COVID-19 crisis. Int. Adv. Econ. Res. 2021:1–3. [ Google Scholar ]
- Kirk C.P., Rifkin L.S. I'll trade you diamonds for toilet paper: consumer reacting, coping and adapting behaviors in the COVID-19 pandemic. J. Bus. Res. 2020; 117 :124–131. [ PMC free article ] [ PubMed ] [ Google Scholar ]
- Li J., Hallsworth A.G., Coca‐Stefaniak J.A. Changing grocery shopping behaviours among Chinese consumers at the outset of the COVID‐19 outbreak. Tijdschr. Econ. Soc. Geogr. 2020; 111 (3):574–583. [ PMC free article ] [ PubMed ] [ Google Scholar ]
- Lund S., Madgavkar A., Manyika J., Smit S., Ellingrud K., Meaney M., Robinson O. McKinsey Global Institute; 2021. The Future of Work after COVID-19. [ Google Scholar ]
- Martin-Neuninger R., Ruby M.B. What does food retail research tell us about the implications of coronavirus (COVID-19) for grocery purchasing habits? Front. Psychol. 2020; 11 doi: 10.3389/fpsyg.2020.01448. [ PMC free article ] [ PubMed ] [ CrossRef ] [ Google Scholar ]
- Mehrolia S., Alagarsamy S., Solaikutty V.M. Customers response to online food delivery services during COVID‐19 outbreak using binary logistic regression. Int. J. Consum. Stud. 2021; 45 (3):396–408. [ PMC free article ] [ PubMed ] [ Google Scholar ]
- Pantano E., Pizzi G., Scarpi D., Dennis C. Competing during a pandemic? Retailers’ ups and downs during the COVID-19 outbreak. J. Bus. Res. 2020; 116 :209–213. [ PMC free article ] [ PubMed ] [ Google Scholar ]
- Reardon T., Heiman A., Lu L., Nuthalapati C.S., Vos R., Zilberman D. “Pivoting” by food industry firms to cope with COVID‐19 in developing regions: e‐commerce and “copivoting” delivery intermediaries. Agric. Econ. 2021; 52 (3):459–475. doi: 10.1111/agec.12631. [ PMC free article ] [ PubMed ] [ CrossRef ] [ Google Scholar ]
- Roggeveen A.L., Sethuraman R. How the COVID-19 pandemic may change the world of retailing. J. Retailing. 2020; 96 (2):169–171. [ Google Scholar ]
- Schleper M.C., Gold S., Trautrims A., Baldock D. Pandemic-induced knowledge gaps in operations and supply chain management: COVID-19's impacts on retailing. Int. J. Oper. Prod. Manag. 2021; 41 (3):193–205. [ Google Scholar ]
- Shankar V., Kalyanam K., Setia P., Golmohammadi A., Tirunillai S., Douglass T., Hennessey J., Bull J.S., Waddoups R. How technology is changing retail. J. Retailing. 2021; 97 (1):13–27. [ Google Scholar ]
- Sheth J. Impact of Covid-19 on consumer behavior: will the old habits return or die? J. Bus. Res. 2020; 117 :280–283. [ PMC free article ] [ PubMed ] [ Google Scholar ]
- Sneader K., Singhal S. McKinsey and Company; 2021. The Next Normal Arrives: Trends that Will Define 2021- and beyond. [ Google Scholar ]
- Tran L.T.T. Managing the effectiveness of e-commerce platforms in a pandemic. J. Retailing Consum. Serv. 2021; 58 doi: 10.1016/j.jretconser.2020.102287. [ CrossRef ] [ Google Scholar ]
- E-Commerce ›
Digital Shopping Behaviour
Industry-specific and extensively researched technical data (partially from exclusive partnerships). A paid subscription is required for full access.
Perceived impact of AI on online shopping experience in the U.S. 2024
A 2024 survey carried out in the United States showed that only nine percent of shoppers believed artificial intelligence (AI) improved their shopping experience. Accoring to most surveyed consumers, AI impact on the shopping experience depended on how the technology is used.
Perceived impact of AI on online shopping experience in the United States in 2024
- Immediate access to 1m+ statistics
- Incl. source references
- Download as PNG, PDF, XLS, PPT
Additional Information
Show sources information Show publisher information Use Ask Statista Research Service
Bizrate Insights
United States
February 2024
1,458 respondents
Other statistics on the topic Artificial intelligence in U.S. e-commerce
- Funding of chatbot/conversational AI startups worldwide 2023
- Consumers able to identify AI on product recommendations in the U.S. 2023, by age
- Most used shopping technologies in the U.S. 2023-2024
B2C E-Commerce
- Use of AI in B2C e-commerce operations in the United States 2023
To download this statistic in XLS format you need a Statista Account
To download this statistic in PNG format you need a Statista Account
To download this statistic in PDF format you need a Statista Account
To download this statistic in PPT format you need a Statista Account
As a Premium user you get access to the detailed source references and background information about this statistic.
As a Premium user you get access to background information and details about the release of this statistic.
As soon as this statistic is updated, you will immediately be notified via e-mail.
… to incorporate the statistic into your presentation at any time.
You need at least a Starter Account to use this feature.
- Immediate access to statistics, forecasts & reports
- Usage and publication rights
- Download in various formats
You only have access to basic statistics. This statistic is not included in your account.
- Instant access to 1m statistics
- Download in XLS, PDF & PNG format
- Detailed references
Business Solutions including all features.
Statistics on " Artificial intelligence (AI) in U.S. e-commerce "
- Global spending on AI 2023, by industry
- Distribution of overall AI companies worldwide 2023, by country
- Distribution of global spending on AI 2023, by industry
- U.S. investments in generative AI 2021-2023, by category
- Most used AI technologies among retail businesses in the United States 2024
- Main applications of gen AI in the U.S. grocery retail 2023
- Use of AI in B2B e-commerce operations in the United States 2023
- Main tasks Amazon sellers and brands use AI for 2024
- Main challenges in implementing AI-based retail solutions in the U.S. 2023
- Outlook for Artificial Intelligence use in the retail industry in the U.S. 2021-2024
- Perceived impact of AI technologies on retail in the U.S. 2024
- Shoppers interested in generative AI uses in the U.S. 2023
- Positive aspects of using gen AI for holiday shopping in the U.S. 2023
- Consumers able to identify AI on product recommendations in the U.S. 2023, by gender
- Consumers able to identify AI on product recommendations in the U.S. 2023, by income
- Use of generative AI capabilities during holiday shopping in the U.S. 2023
- Ways AI has been improving CX U.S. 2023
- Shopping experiences with e-commerce chatbots in the U.S. 2023
- Main advantages of using e-commerce chatbots in the U.S. 2023
- U.S. shoppers using chatbots and ChatGPT 2023
Other statistics that may interest you Artificial intelligence (AI) in U.S. e-commerce
Market overview
- Premium Statistic Global spending on AI 2023, by industry
- Premium Statistic Distribution of overall AI companies worldwide 2023, by country
- Premium Statistic Distribution of global spending on AI 2023, by industry
- Premium Statistic U.S. investments in generative AI 2021-2023, by category
Business adoption
- Premium Statistic Most used AI technologies among retail businesses in the United States 2024
- Premium Statistic Main applications of gen AI in the U.S. grocery retail 2023
- Premium Statistic Use of AI in B2C e-commerce operations in the United States 2023
- Premium Statistic Use of AI in B2B e-commerce operations in the United States 2023
- Basic Statistic Main tasks Amazon sellers and brands use AI for 2024
- Premium Statistic Main challenges in implementing AI-based retail solutions in the U.S. 2023
Shopping experience
- Premium Statistic Outlook for Artificial Intelligence use in the retail industry in the U.S. 2021-2024
- Premium Statistic Perceived impact of AI technologies on retail in the U.S. 2024
- Premium Statistic Shoppers interested in generative AI uses in the U.S. 2023
- Premium Statistic Positive aspects of using gen AI for holiday shopping in the U.S. 2023
- Premium Statistic Consumers able to identify AI on product recommendations in the U.S. 2023, by age
- Premium Statistic Consumers able to identify AI on product recommendations in the U.S. 2023, by gender
- Premium Statistic Consumers able to identify AI on product recommendations in the U.S. 2023, by income
- Premium Statistic Use of generative AI capabilities during holiday shopping in the U.S. 2023
Spotlight: chatbots
- Basic Statistic Funding of chatbot/conversational AI startups worldwide 2023
- Premium Statistic Ways AI has been improving CX U.S. 2023
- Premium Statistic Most used shopping technologies in the U.S. 2023-2024
- Premium Statistic Shopping experiences with e-commerce chatbots in the U.S. 2023
- Premium Statistic Main advantages of using e-commerce chatbots in the U.S. 2023
- Basic Statistic U.S. shoppers using chatbots and ChatGPT 2023
Further Content: You might find this interesting as well
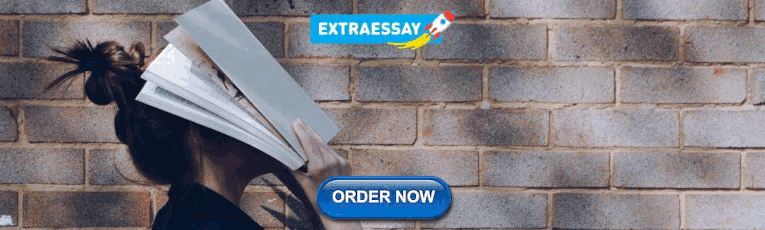
COMMENTS
This paper extends the existing knowledge of the online shopping behaviour of consumers in the e-commerce context, thus revealing and sharing insights that are relevant to individuals and businesses with a common interest in the theoretical implications, including the managerial strategies that would be suitable for growing B2C e-commerce in ...
1. Introduction. Online shopping is a common, globally found activity (Erjavec and Manfreda, 2021; Shao et al., 2022).In 2020, retail e-commerce sales worldwide amounted to 4.28 trillion United States (U.S.) dollars and this is projected to grow to 5.4 trillion U.S. dollars in 2022 (Coppola, 2021).Within this vast market, customers will often make spontaneous, unplanned, unreflective and ...
first stream of research focuses on consumers online shopping behavior at specific online shops. For example, an early study in this domain was Gefen et al. (2003) who explain ed why
Online shopping provides flexibility in the place and time of shopping activities. The current study applies the concepts and guidelines of the systematic review and meta-analysis to the most recent evidence on the intensity of online shopping, intending to resolve the controversies arising from past research in this area.
This article attempts to take stock of this environment to critically assess the research gaps in the domain and provide future research directions. Applying a well-grounded systematic methodology following the TCCM (theory, context, characteristics and methodology) framework, 197 online consumer shopping behaviour articles were reviewed.
These studies examined the online behavior of consumers in numerous dimensions and postulated perceptions behind online shopping behavior and attributes (Jarvenpaa and Todd, 1996; Chang and Kannan, 2006), consumer information process styles, online store layouts, (Park and Kim, 2003), behavioral and normative beliefs about technology adoption ...
Abstract. This chapter provides an overview of recent research related to online shopping and the conceptual frameworks that have guided that research. Specifically, the chapter addresses research related to who shops online and who does not, what attracts consumers to shop online, how and what consumers do when shopping online, and factors ...
2.1 Online Shopping During COVID-19. World Health Organization (WHO) in early 2020 announced Covid19 as a pandemic. Due to the restrictions imposed by governments across the world, 52% consumers adhered to the social distancing norms by wearing masks to prevent any infection spread by the virus in the communities (Andrienko 2020).Many consumers shifted to e-commerce platforms for purchases ...
The measures for attitude toward online shopping were adapted from Jarvenpaa et al. (1999). The study of Wu and Chen ... Siqueira-Junior: Analyzed and interpreted the data; Contributed reagents, materials, analysis tools or data; Wrote the paper. Funding statement. This research has received support from the University of Valle ...
The purpose of the research was to find out the problems that consumers face during their shopping through online stores.,A quantitative research method was adopted for this research in which a survey was conducted among the users of online shopping sites.,As per the results total six factors came out from the study that restrains consumers to ...
Finding also revealed that purchase intention significant positively influence online shopping behavior. For future research, sample from working adults and other variables that related to online shopping were to be included to minimise sampling bias. ... Working Papers on Information Systems, 9-37. Dabrowska, A., 2011. Consumer Behaviour in ...
The author found that the main factors that affect online shopping are convenience and attractive pricing/discount. Advertising and recommendations were among the least effective. In the study by Lian and Yen (2014), authors tested the two dimensions (drivers and barriers) that might affect intention to purchase online.
Recent years have seen an increase in the number of research papers related to e-tailing [1, 2]. ... Bryła, P.: Organic food online shopping in Poland. Br. Food J. 120(5), 1015-1027 (2018) Article Google Scholar Osman, R., Hwang, F.: A method to study how older adults navigate in an online grocery shopping site.
Online shopping is a process whereby consumers directly buy goods, services etc. from a. seller without an intermediary service over the Internet. Shoppers can visit web stores. from the comfort ...
This paper intends to examine online shopping. experiences from three aspects: the physical, ideological and pragmatic dimensions. As an exploratory research study, a qualitative research method ...
Introduction. Online shopping is the act of buying a product or service through any e-stores with the help of any website or app. Tarhini et al. (2021) stated that shopping through online channels is actively progressing due to the opportunity to save time and effort. Furthermore, online shopping varies from direct e-store and indirect e-store about their perception against the actual experience.
The research paper provides practical guidelines for online-based business organizations on how to effectively use social media platforms for business target advertising and promotional activities. ... this study used an online purposive sampling technique to investigate the impact of social media on consumers' online shopping behavior. The ...
Online shopping is a form of electronic commerce which allows consumers to directly buy goods. or services from a sell er over the Internet using a web browser. The online stores are using ...
In summary, unexpected regulations imposed by governments determined an immediate increase in demand for online shopping: existing customers started to use online channels more frequently, while new customers, including older and less tech-savvy generations, turned to online channels for the first time (Hwang et al., 2020; Pantano et al., 2020).
The paper also suggests some recommendations regarding online shopping in Kosovo ... M. N. and Ashouri, M. I., 2015. Consumer attitudes towards online shopping. Internet Research. 25(5), pp:707 ...
The fast-paced growth of e-commerce is rapidly changing consumers' shopping habits and shaping the future of the retail industry. While online retailing has allowed companies to overcome geographic barriers to selling and helped them achieve operational efficiencies, offline retailers have struggled to compete with online retailers, and many retailers have chosen to operate both online and ...
Perceived impact of AI on online shopping experience in the U.S. 2024. A 2024 survey carried out in the United States showed that only nine percent of shoppers believed artificial intelligence (AI ...
N. Jamila Dani (2017) "A Study on Consumers Attitude Towards Online Shopping" International Journal of Research in Management and Business Studies, Vol,4. Issue. (SPL 2) PP:42-46.