A Review on Social Network Analysis Methods and Algorithms
Ieee account.
- Change Username/Password
- Update Address
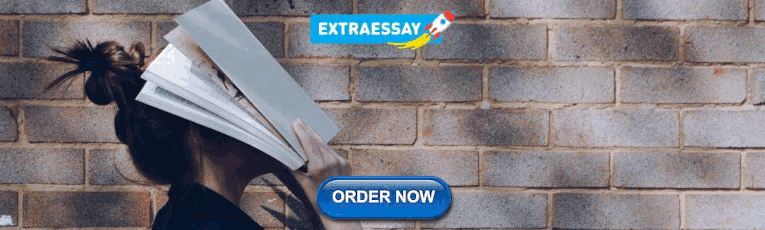
Purchase Details
- Payment Options
- Order History
- View Purchased Documents
Profile Information
- Communications Preferences
- Profession and Education
- Technical Interests
- US & Canada: +1 800 678 4333
- Worldwide: +1 732 981 0060
- Contact & Support
- About IEEE Xplore
- Accessibility
- Terms of Use
- Nondiscrimination Policy
- Privacy & Opting Out of Cookies
A not-for-profit organization, IEEE is the world's largest technical professional organization dedicated to advancing technology for the benefit of humanity. © Copyright 2024 IEEE - All rights reserved. Use of this web site signifies your agreement to the terms and conditions.
Help | Advanced Search
Economics > General Economics
Title: analysis of proximity informed user behavior in a global online social network.
Abstract: Despite the earlier claim of "Death of Distance", recent studies revealed that geographical proximity still greatly influences link formation in online social networks. However, it is unclear how physical distances are intertwined with users' online behaviors in a virtual world. We study the role of spatial dependence on a global online social network with a dyadic Logit model. Results show country-specific patterns for distance effect on probabilities to build connections. Effects are stronger when the possibility for two people to meet in person exists. Relative to weak ties, dependence on proximity is looser for strong social ties.
Submission history
Access paper:.
- HTML (experimental)
- Other Formats
References & Citations
- Google Scholar
- Semantic Scholar
BibTeX formatted citation

Bibliographic and Citation Tools
Code, data and media associated with this article, recommenders and search tools.
- Institution
arXivLabs: experimental projects with community collaborators
arXivLabs is a framework that allows collaborators to develop and share new arXiv features directly on our website.
Both individuals and organizations that work with arXivLabs have embraced and accepted our values of openness, community, excellence, and user data privacy. arXiv is committed to these values and only works with partners that adhere to them.
Have an idea for a project that will add value for arXiv's community? Learn more about arXivLabs .
- Privacy Policy

Home » Social Network Analysis – Types, Tools and Examples
Social Network Analysis – Types, Tools and Examples
Table of Contents

Social Network Analysis
Social Network Analysis (SNA) is an analytical method used to study social structures through the use of networks and graph theory. It identifies the relationships between individuals, organizations, or other entities and examines the patterns and implications of these relationships.
The nodes in the network represent the actors within the networks and the ties or edges represent relationships between the actors. These might be, for example, friendship ties between people, business relationships between companies, or communication patterns between individuals.
By analyzing the network structure and the characteristics of the actors within the network, SNA can reveal properties such as the distribution of resources, the flow of information, or the overall connectivity of the network.
Here are a few key concepts in SNA:
- Centrality : This measures the importance of a node in the network. Various centrality measures exist, each emphasizing a different aspect of a node’s position within the network, such as degree centrality (the number of direct connections a node has), betweenness centrality (the number of times a node acts as a bridge along the shortest path between two other nodes), and eigenvector centrality (the sum of the centrality scores of all nodes that one node is connected to).
- Density : This is a measure of the proportion of possible connections in a network that are actual connections. A high density suggests that the network participants are highly interconnected.
- Clusters or Communities : These are groups of nodes that are more densely connected with each other than with the rest of the network.
- Structural Holes : These are gaps in the network where a node could potentially act as a bridge between two unconnected parts of the network.
Types of Social Network Analysis
Social Network Analysis can be broadly categorized based on the type of networks being analyzed, the level of analysis, and the methodologies employed. Here are a few ways to categorize SNA:
Whole Network Analysis
This type of analysis focuses on the structure and properties of the network as a whole. This might include measures of network cohesion, centralization, and density. It also looks at the overall distribution of relationships and identifies key groups or clusters within the network.
Ego Network Analysis
In this type of analysis, the focus is on a single actor (the ‘ego’) and their immediate network (the ‘alters’). It’s often used when interest is in the personal networks of individuals. Measures can include the size of the network, the composition of the network in terms of the types of ties and nodes, and measures of network density or diversity.
Two-mode (or Bipartite) Network Analysis
This type of SNA is used when there are two different types of nodes, and connections are only possible between nodes of different types (not within types). For example, authors and the books they write, or actors and the movies they appear in. In such a network, you can study the connections between nodes of one type, mediated by nodes of the other type.
Dynamic Network Analysis (DNA)
This is used to study how social networks evolve over time. This could involve studying how ties between actors develop or disappear, or how actors move around within the network. In addition to traditional network measures, DNA also considers measures that are dynamic in nature, such as change in centrality over time.
Semantic Network Analysis
This type of SNA focuses on the relationships between concepts or ideas, rather than individuals or organizations. For instance, semantic network analysis could map out how different scientific concepts are related to each other in the literature.
Social Media Network Analysis
A specialized form of SNA, this deals with the study of social relationships as expressed through social media platforms. It allows for the mapping and measuring of relationships and flows between people, groups, organizations, computers, URLs, and other connected information/knowledge entities.
Social Network Analysis Techniques
Social Network Analysis involves various techniques to understand the structure and patterns of relationships among actors (people, organizations, etc.) in a network. These techniques may be mathematical, visual, or computational, and often involve the use of specialized software. Here are several common SNA techniques:
Network Visualization
One of the most basic SNA techniques involves creating a visual representation of the network. This can help to reveal patterns and structures within the network that may not be immediately obvious from the raw data. There are various ways to create such visualizations, depending on the specifics of the network and the goals of the analysis. Software such as Gephi or Cytoscape can be used for network visualization.
Centrality Measures
These are techniques used to identify the most important nodes within a network. Various measures of centrality exist, each highlighting different aspects of a node’s position in the network. These include degree centrality (the number of connections a node has), betweenness centrality (how often a node appears on the shortest path between other nodes), closeness centrality (how quickly a node can reach all other nodes in the network), and eigenvector centrality (a measure of the influence of a node in a network).
Community Detection
Also known as clustering, this technique aims to identify groups of nodes that are more closely connected with each other than with the rest of the network. This can help to reveal sub-groups or communities within the network.
Structural Equivalence and Blockmodeling
Structural equivalence is a measure of how similarly two nodes are connected to the rest of the network. Nodes that are structurally equivalent often play similar roles in the network. Blockmodeling is a technique used to simplify a network by grouping together structurally equivalent nodes.
Dynamic Network Analysis
This involves studying how a network changes over time. This can help to reveal patterns of network evolution, including how relationships form and dissolve, how centrality measures change over time, and how communities evolve.
Network Correlation and Regression
These are statistical techniques used to identify and test for patterns within the network. For example, one might use these techniques to test whether nodes with certain characteristics are more likely to form connections with each other.
Social Network Analysis Tools
There are several tools available that can be used to conduct Social Network Analysis (SNA). These range from open-source software to commercial offerings, each with their own strengths and weaknesses. Here are a few examples:
- Gephi : Gephi is an open-source, interactive visualization and exploration platform for all kinds of networks and complex systems. It’s user-friendly and allows users to interactively manipulate the network visualization, perform network analysis, and export results in various formats.
- UCINet : UCINet is a comprehensive package for the analysis of social network data as well as other 1-mode and 2-mode data. It’s widely used in social science research.
- NetDraw : Often used in conjunction with UCINet, NetDraw is a free tool for visualizing networks. It supports the visualization of large networks and allows for various customization options.
- Pajek : Pajek is a program for the analysis and visualization of large networks. It’s an extensive tool, offering a range of complex network metrics, and is free for non-commercial use.
- NodeXL : NodeXL is a free, open-source template for Microsoft Excel that allows users to display and analyze network graphs. Its integration with Excel makes it user-friendly, particularly for those already familiar with Excel.
- Cytoscape : Originally designed for biological research, Cytoscape is now a popular open-source software platform for visualizing complex networks and integrating these with any type of attribute data.
- SocioViz : SocioViz is a social media analytics platform for Twitter data, focused on network analysis and visualization. It’s a powerful tool for researchers interested in online social networks.
- NetworkX : NetworkX is a Python package for the creation, manipulation, and study of the structure, dynamics, and functions of complex networks. It integrates well with other scientific Python tools like SciPy and Matplotlib.
- igraph : igraph is a library available in R, Python, and C for creating, manipulating, and analyzing networks. It’s highly efficient and can handle large networks.
- RSiena : RSiena is an R package dedicated to the statistical analysis of network data, with a particular focus on longitudinal social networks.
Social Network Analysis Examples
Social Network Analysis Examples are as follows:
- Public Health – COVID-19 Pandemic : During the COVID-19 pandemic, SNA was used to model the spread of the virus. The interactions between individuals were mapped as a network, helping identify super-spreader events and informing public health interventions.
- Business – Google’s “PageRank” Algorithm : Google’s PageRank algorithm, which determines the order of search engine results, is a type of SNA. It considers web pages as nodes and hyperlinks as connections, determining a page’s importance by looking at the number and quality of links to it.
- Sociology – Stanley Milgram’s “Small World” Experiment : This is one of the most famous social network experiments, where Milgram demonstrated that any two people in the United States are separated on average by only six acquaintances, leading to the phrase “six degrees of separation.”
- Online Social Networks – Facebook’s “People You May Know” Feature : Facebook uses SNA to suggest new friends. The platform analyzes your current network and suggests people you’re likely to know, typically friends of friends or people who share common networks.
- Criminal Network Analysis – Capture of Osama bin Laden : SNA was reportedly used in the operation to capture Osama bin Laden. By mapping the social connections of known associates, intelligence agencies were able to locate the Al-Qaeda leader.
- Academic Research – Collaboration Networks : SNA is used in scientometrics to analyze collaboration networks among researchers . For example, a study on co-authorship networks in scientific articles can reveal patterns of collaboration and the flow of information in different disciplines.
When to use Social Network Analysis
Social Network Analysis is a powerful tool for studying the relationships between entities (like people, organizations, or even concepts) and the overall structure of these relationships. Here are several situations when SNA might be particularly useful:
- Understanding Complex Systems : SNA is well-suited to studying complex, interconnected systems. If you’re interested in not just individual entities but also the relationships between them, SNA can provide valuable insights.
- Identifying Key Actors : SNA can help identify the most important entities in a network based on their position and connections. These might be influential people within a social network, critical servers in a computer network, or key scholars in a field of study.
- Studying Diffusion Processes : If you’re interested in how something (like information, behaviors, diseases) spreads through a network, SNA can be a valuable tool. It allows for the examination of diffusion pathways and identification of nodes that speed up or hinder diffusion.
- Detecting Communities : SNA can be used to identify clusters or communities within a network. These might be groups of friends within a social network, clusters of companies in a business network, or research clusters in scientific collaboration networks.
- Mapping Out Large Systems : In cases where you have a large system of many interconnected entities, SNA can provide a visual representation of the system, making it easier to understand and analyze.
- Investigating Structural Roles : If you’re interested in the roles individuals or entities play within their network, SNA offers methods to classify these roles based on the pattern of their relationships.
Purpose of Social Network Analysis
Social Network Analysis serves a wide range of purposes across different fields, given its versatile nature. Here are several key purposes:
- Understanding Network Structure : One of the key purposes of SNA is to understand the structure of relationships between actors within a network. This includes understanding how the network is organized, the distribution of connections, and the patterns of interaction.
- Identifying Key Actors or Nodes : SNA can identify crucial nodes within a network. These could be individuals with many connections, or nodes that serve as critical links between different parts of the network. In a business, for instance, such nodes might be key influencers or innovators.
- Detecting Subgroups or Communities : SNA can identify clusters or communities within a network, i.e., groups of nodes that are more connected to each other than to the rest of the network. This can be valuable in numerous contexts, from identifying communities in social media networks to detecting collaboration clusters in scientific networks.
- Analyzing Information or Disease Spread : In public health and communication studies, SNA is used to study the patterns and pathways of information or disease spread. Understanding these patterns can be critical for designing effective interventions or campaigns.
- Analyzing Social Capital : SNA can help understand an individual or group’s social capital – the resources they can access through their network relationships. This analysis can offer insights into power dynamics, access to resources, and inequality within a network.
- Studying Network Dynamics : SNA can examine how networks change over time. This could involve studying how relationships form or dissolve, how centrality measures change over time, or how communities evolve.
- Predicting Future Interactions : SNA can be used to predict future interactions or relationships within a network, which can be useful in a variety of settings such as recommender systems, predicting disease spread, or forecasting emerging trends in social media.
Applications of Social Network Analysis
Social Network Analysis has a wide range of applications across different disciplines due to its capacity to analyze relationships and interactions. Here are some common areas where it is applied:
- Public Health : SNA can be used to understand the spread of infectious diseases within a community or globally. It helps identify “super spreaders” and optimizes strategies for vaccination or containment.
- Business and Organizations : Companies use SNA to analyze communication and workflow patterns, enhance collaboration, boost efficiency, and detect key influencers within their organization. It can also be applied in understanding and leveraging informal networks within a business.
- Social Media Analysis : On platforms like Facebook, Twitter, or Instagram, SNA helps analyze user behavior, track information dissemination, identify influencers, detect communities, and develop recommendation systems.
- Criminal Justice : Law enforcement and intelligence agencies use SNA to understand the structure of criminal or terrorist networks, identify key figures, and predict future activities.
- Internet Infrastructure : SNA helps in mapping the internet, identifying critical nodes, and developing strategies for robustness against cyberattacks or outages.
- Marketing : In marketing, SNA can track the diffusion of advertising messages, identify influential consumers for targeted marketing, and understand consumer behavior and brand communities.
- Scientometrics : SNA is used in academic research to map co-authorship networks or citation networks. It can uncover patterns of collaboration and the flow of knowledge in scientific fields.
- Politics and Policy Making : SNA can help understand political alliances, lobby networks, or policy networks, which can be critical for strategic decision-making in politics.
- Ecology : In ecological studies, SNA can help understand the relationships between different species in an ecosystem, providing valuable insights into ecological dynamics.
Advantages of Social Network Analysis
Social Network Analysis offers several advantages when studying complex systems and relationships. Here are a few key advantages:
- Reveals Complex Relationships : SNA allows for the study of relationships between entities (be they people, organizations, computers, etc.) in a way that many other methodologies do not. It emphasizes the importance of these relationships and helps reveal complex interaction patterns.
- Identifies Key Players : SNA can identify the most influential or important nodes in a network, whether they are individuals within a social network, key servers in an internet network, or central scholars in an academic field.
- Unveils Network Structure and Communities : SNA can help visualize the overall structure of a network and can reveal communities or clusters of nodes within a network. This can provide valuable insights into the organization and division of a network.
- Tracks Changes Over Time : Dynamic SNA allows the study of networks over time. This can help to track changes in the network structure, the role of specific nodes, or the flow of information or resources through the network.
- Helps Predict Future Interactions : Based on the analysis of current and past relationships, SNA can be used to predict future interactions, which can be useful in many fields including public health, marketing, and national security.
- Aids in Designing Effective Strategies : The insights gained from SNA can be used to design targeted strategies, whether that’s intervening in the spread of misinformation online, designing a targeted marketing campaign, disrupting a criminal network, or managing collaboration in an organization.
- Versatility : SNA can be applied to a vast array of fields, from sociology to computer science, biology to business, making it a versatile tool.
Disadvantages of Social Network Analysis
While Social Network Analysis is a powerful tool with wide-ranging applications, it also has certain limitations and disadvantages that are important to consider:
- Data Collection Challenges : Collecting complete and accurate network data can be a major challenge. For larger networks, it may be nearly impossible to collect data on all relevant relationships. There’s also a risk of response bias, as people may forget, overlook, or misinterpret their relationships when providing data.
- Time and Resource Intensive : Collecting network data, especially from primary sources, can be extremely time-consuming and expensive. Additionally, analyzing network data can also require significant computational resources for larger networks.
- Complexity : SNA involves complex concepts and measures, which can be difficult to understand without specialized knowledge. This complexity can make it difficult to communicate findings to a non-technical audience.
- Privacy and Ethical Concerns : SNA often involves sensitive data about individuals’ relationships and interactions, raising important privacy and ethical concerns. It’s important to handle this data carefully to respect individuals’ privacy.
- Static Snapshots : Traditional SNA often provides a static snapshot of a network at a particular point in time, which may not capture the dynamic nature of social relationships. While dynamic SNA does exist, it adds additional complexity and data demands.
- Dependence on Quality of Data : The insights and conclusions drawn from SNA are only as good as the data used. Incomplete, inaccurate, or biased data can lead to misleading results.
- Difficulties in Establishing Causality : While SNA can reveal patterns and associations in network data, it can be difficult to establish causal relationships. For instance, do strong connections between two individuals lead to similar behavior, or does similar behavior lead to strong connections?
- Assumptions about Relationships : SNA often assumes that relationships are equally important, which might not always be the case. Different relationships might have different strengths or meanings, which can be challenging to represent in a network.
About the author
Muhammad Hassan
Researcher, Academic Writer, Web developer
You may also like

Digital Ethnography – Types, Methods and Examples

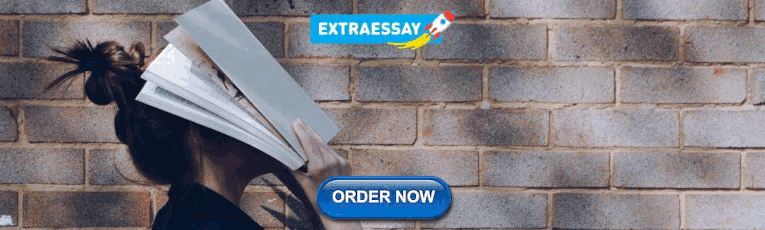
Predictive Analytics – Techniques, Tools and...

Big Data Analytics -Types, Tools and Methods

Diagnostic Analytics – Methods, Tools and...

Blockchain Research – Methods, Types and Examples

What is Data Science? Components, Process and Tools
ORIGINAL RESEARCH article
Associations between social network addiction, anxiety symptoms, and risk of metabolic syndrome in peruvian adolescents—a cross-sectional study.
- 1 Escuela de Medicina Humana, Universidad Señor de Sipán, Chiclayo, Peru
- 2 Escuela de Enfermería, Universidad Señor de Sipán, Chiclayo, Peru
- 3 Research Group for Nutrition and Lifestyle, Universidad Peruana Unión, Lima, Peru
- 4 Departamento de Psicología, Universidad Nacional Mayor de San Marcos, Lima, Peru
- 5 Area de Investigación, Universidad Cesar Vallejo (UCV), Lima, Peru
Background: The link between physical and mental health and screen time in adolescents has been the subject of scientific scrutiny in recent years. However, there are few studies that have evaluated the association between social network addiction (SNA) and metabolic risk in this population.
Objective: This study determined the association between SNA and anxiety symptoms with the risk of metabolic syndrome (MetS) in adolescents.
Methods: A cross-sectional study was conducted in Peruvian adolescents aged 12 to 18 years, who completed a Social Network Addiction Questionnaire and the Generalized Anxiety Disorder 2-item scale (GAD-2), between September and November 2022. A total of 903 participants were included in the study using a non-probability convenience sample. Sociodemographic and anthropometric data were also collected. Binary logistic regression was used to explore the association between SNA and anxiety symptoms with MetS in a cross-sectional analysis.
Results: Males were more likely to have MetS than females (OR = 1.133, p = 0.028). Participants who were 16 years of age or older and those with excess body weight were 2.166, p = 0.013 and 19.414, p < 0.001 times more likely to have MetS, respectively. Additionally, SNA (OR = 1.517, p = 0.016) and the presence of anxiety symptoms (OR = 2.596, p < 0.001) were associated with MetS.
Conclusion: Our findings suggest associations between SNA, anxiety symptoms, and MetS among youth. However, more studies are needed to better understand this association and to deepen the possible clinical and public health implications.
Introduction
Metabolic syndrome (MetS) also known as “insulin resistance syndrome” or “syndrome X” refers to the set of conditions that increase the likelihood of developing cardiovascular and metabolic diseases, including type 2 diabetes mellitus, hypertension, and cardiovascular disease ( 1 ). Central obesity, which can be measured through anthropometric parameters, such as waist and hip circumferences and waist-hip ratio (WHR), constitutes one of the main components of the MetS ( 2 ). In recent decades, the prevalence of obesity in adolescents has increased significantly worldwide ( 3 ), which has been associated with an increased risk of developing cardiometabolic diseases at an early age ( 4 ). In fact, since 1975, obesity rates have increased considerably and have almost tripled in the general population, and in the particular case of children and adolescents, this increase is even greater, reaching almost a five-fold increase ( 3 ). In the national context, in recent years, there has been a steady increase in the number of Peruvian adolescents at high and very high risk of cardiometabolic diseases, as measured by the WHtR ( 5 ), which, in turn, increases mortality risk. In Peru, there is a double burden of morbidity due to, on the one hand, problems associated with infectious diseases and malnutrition by deficit and/or excess, and on the other hand, a progressive increase in non-communicable diseases ( 6 ).
Social networks are online platforms that allow users to create personal profiles and establish connections with other users in their network ( 7 ). In recent years, the use of social networks has become an increasingly frequent and popular activity among adolescents ( 8 ). Social network platforms such as TikTok, Facebook, Twitter, among others, can represent a valuable opportunity to connect and engage adolescents with messages related to the adoption of healthy lifestyles, such as proper diet and physical activity, which can be a preventive element against MetS ( 9 ). However, excessive exposure to these digital platforms could have negative consequences on the physical and mental health of this population group ( 8 ). There are few studies that examine the relationship between SNA and MetS. However, we found a recent study that evaluated the link between adolescent social networks and health in adulthood, suggesting that adolescents’ social network position has lasting consequences for MetS in adulthood ( 10 ). However, most studies have focused on body mass index (BMI), pointing to a possible relationship between social media addiction, excessive use of media, and an increased risk of developing obesity in adolescents ( 11 – 13 ), which is related to cardiometabolic problems ( 1 , 4 ).
Anxiety symptoms are one of the most prevalent psychiatric conditions in adolescence, affecting approximately 1 in 4 adolescents ( 14 ). These symptoms may represent risk factors for anxiety disorders encompassing various conditions such as agoraphobia, panic disorder, specific phobias, separation anxiety disorder, social anxiety disorder, and generalized anxiety disorder ( 15 ). Studies have shown that anxiety symptoms are associated with an increased risk of cardiovascular disease and other metabolic problems in young adults ( 16 , 17 ). Although there is less research examining these connections in adolescents, a study recently found a statistically significant relationship between anxiety and some metabolic risk factors in this population ( 18 ). Another study conducted in adolescents reported a relationship between anxiety symptoms and insulin resistance ( 19 ), which may lead to worsening metabolic outcomes in at-risk youth.
Therefore, considering the above, it is important to deepen our understanding of this relationship and explore the possible clinical and public health implications. In this context, the present study aims to examine the association between SNA, anxiety symptoms, and MetS in adolescents, with the intention of contributing to a better understanding of these phenomena and to the implementation of effective preventive and therapeutic interventions.
Materials and methods
Design and study participants.
A descriptive cross-sectional study was conducted during September and November 2022. The sample was selected using non-probabilistic sampling ( 20 , 21 ). The researchers chose to use non-probabilistic purpose sampling because it is relevant to obtain data from respondents for this study ( 21 – 23 ). Data were collected using a survey consisting of the following: (a) sociodemographic data (e.g., age, sex, origin, place of residence, among others); (b) a validated questionnaire and scale to assess symptoms of SNA and anxiety symptoms, respectively; (c) in addition, information was collected on body weight status, height, and waist circumference, to subsequently estimate BMI, height-for-age (H/A), and waist-to-height ratio (WHtR).
The survey was distributed to participants enrolled in two public schools in the districts of Reque and Morrope, located in the city of Chiclayo, Peru. Data collection was possible due to the support of the directors of both schools and the teachers of each of the classrooms we selected. The sample size was calculated using Free Statistic Calculators version 4.0 ( 24 ). For the multiple regression analysis, an effect size of 0.10, a statistical power of 0.80, 5 explanatory variables and a probability level of 0.05 were considered. According to this calculation, a minimum sample size of 134 participants was required. However, in this study, a total of 903 students participated voluntarily, which far exceeds the calculated sample size. Participants of both sexes, those without any pathology, and those within the selected age range (12–18 years) were included. However, adolescents whose parents did not give their written informed consent were excluded from the study. Furthermore, 23 records were excluded due to missing data. The final sample was 903 participants.
Ethical aspects
The study was carried out after receiving the approval of the Research Ethics Committee of the Universidad Señor de Sipán (Registration and reference number: 0085-17052022-CIEI). Subsequently, the directors of both schools were contacted to request and obtain permission to meet with the parents of potential participants. This meeting was to explain to all parents the purpose of the study. Furthermore, considering that the participants were minors, a procedure was implemented to guarantee participation with the consent of the parents or legal guardians. After providing initial explanations about the purpose of the study, an informed consent question was included to be answered by parents or legal guardians as a prerequisite for adolescents to participate in the survey. Therefore, informed consent was obtained from all subjects prior to their participation in the study.
Measurement instruments
Social network addiction questionnaire.
This instrument was originally developed by Escurra and Salas in 2014 and was constructed using a sample of 380 participants (36.3% men and 63.7% women) in Lima, Peru ( 25 ). This questionnaire is made up of 24 items on a 5-point Likert scale ranging from never to always (assigned scores from 0 to 4). In addition, it has 23 direct items and 1 inverse item. A higher score indicates a higher level of SNA. The validity and reliability of the instrument was analyzed, showing a Cronbach’s Alpha coefficient (α) = 0.95, therefore, it has adequate internal consistency. Additionally, the instrument evaluation was carried out on a sample of 744 adolescents aged 17 to 19 years, reporting a reliability of α = 0.86 ( 26 ). In the current study, the internal consistency of the instrument was also tested, evidencing α = 0.89.
Generalized anxiety disorder (GAD-2)
The Generalized Anxiety Disorder 2-item Scale was used to measure emotional state. This instrument is composed of item 1 of the GAD-7 “Feeling of nervousness, anxiety, or being on edge” and by item 2 “Not being able to stop worrying or controlling worries” and is assessed through the question: “Indicate how often you have experienced the following problems in the last 15 days” ( 27 , 28 ). These items have 4 response options where never = 0, less than half of the days = 1, more than half of the days = 2, and almost every day = 3. A cutoff score greater than or equal to 3 on GAD-2 is an indicator of a probable clinically relevant anxiety disorder, while a score less than 3 indicates the absence of anxiety symptoms ( 29 ). Total scores range from 0 to 6 ( 28 , 30 , 31 ). In this study, the version adapted and validated for the Peruvian population was used and presented an adequate Cronbach’s α coefficient (α = 0.81) ( 32 ).
Sociodemographic data
Sociodemographic and economic data were collected through a registration form, which is composed of sociodemographic factors such as age in years (11–12 and 16–18), sex (male and female), residence (urban and rural), level of education of parents (elementary, technical, and university), marital status of parents (married, cohabiting, single, divorced, and widowed), family income in “soles (PEN)” (<2,149.00 PEN, 2,149.00 PEN–10,746.00 PEN, and > 10,746.00 PEN), among others.
Anthropometric data
Weight and height were measured using a calibrated SECA 700 mechanical column scale with a capacity of 220 kg and a measuring range of 60 to 200 cm (SECA®, Hamburg, Germany). Anthropometric evaluation was performed by a professional nutritionist in the early hours of the day for one week. Furthermore, the measurements were performed with the participants walking barefoot and wearing the minimum amount of clothing. The BMI was calculated, and the classification was made according to the parameters established by the World Health Organization. A BMI z score was determined and classified as follows: “underweight,” BMI z-score < −1; “normal,” BMI z score −1 to 1; and “overweight” (z > 1) ( 33 ).
Height/age (H/A)
Furthermore, H / A was calculated and classified based on the reference data corresponding to the Peruvian standards in the public health system: >2 standard deviation (SD), “normal or adequate” (H/A ≥ −2 to ≤2 SD), “low” (H/A < −2 to −3 SD), and “severe low” (H/A < −3). For the purposes of the current study, it is necessary to specify that short and very severe height was recategorized as short height ( 34 , 35 ).
Waist-to-height ratio (WHtR)
Waist circumference (WC) was measured in triplicate using a Cescorf (Cescorf Equipamentos Para Esporte Ltda—Epp, Brazil) self-retractable metallic steel tape measure. Measurement of the WC was made considering the midpoint of the axillary line, in the distance that goes from the ridge of the last rib to the iliac spine ( 36 ).
After obtaining WC measurements, WHtR was determined by dividing the waist circumference of each participant by their respective height. This anthropometric parameter is valued for its ability to provide an accurate indication of the distribution of adipose tissue in the body ( 33 , 37 ). It is a simple index that offers immediate identification and interpretation, being particularly useful in the early identification of abdominal obesity in children ( 38 ). As a result, it makes it possible to anticipate potential risks related to cardiometabolic disorders ( 2 , 37 , 39 ). In the evaluation process, a cutoff point of 0.5 was identified. That is, participants who had a WHtR index greater than 0.5 were classified as adolescents at risk of MetS ( 2 , 33 , 37 , 39 , 40 ).
Statistical analysis
A Microsoft Excel spreadsheet was used for data collection and coding. Then, for data processing and analysis, the IBM SPSS statistical software package, version 26 (SPSS Inc., Chicago, IL, United States) was used. Descriptive analysis of the variables was performed using tables of absolute frequencies and percentages. To explore whether sociodemographic data, anthropometric data, SNA, and anxiety symptoms were different according to sex and MetS, the chi-square statistical test was used. Finally, an exploration of the association of the factors influencing MetS (dependent variable) was carried out using a binary logistic regression model. We considered sex, age, overweight, SNA, and anxiety symptoms as independent variables. These variables had a probability value ( p -value) of less than 0.05 in a preliminary bivariate analysis, and therefore were incorporated in the bivariate logistic regression analysis.
A total of 903 schoolchildren voluntarily decided to participate in the study; of these, 56% were female. The highest proportion (68.8%) were between 12 and 15 years of age. Regarding the level of education of parents, 76.6% of mothers and 69.2% of fathers reported basic education. Most parents were married (46.4%), had a monthly income <2,149.00 (74.6%), and reported having more than two sons (57.9%). More than half of the respondents reported that they live with their parents (55.3%). The sociodemographic characteristics of the participants are shown in Table 1 .
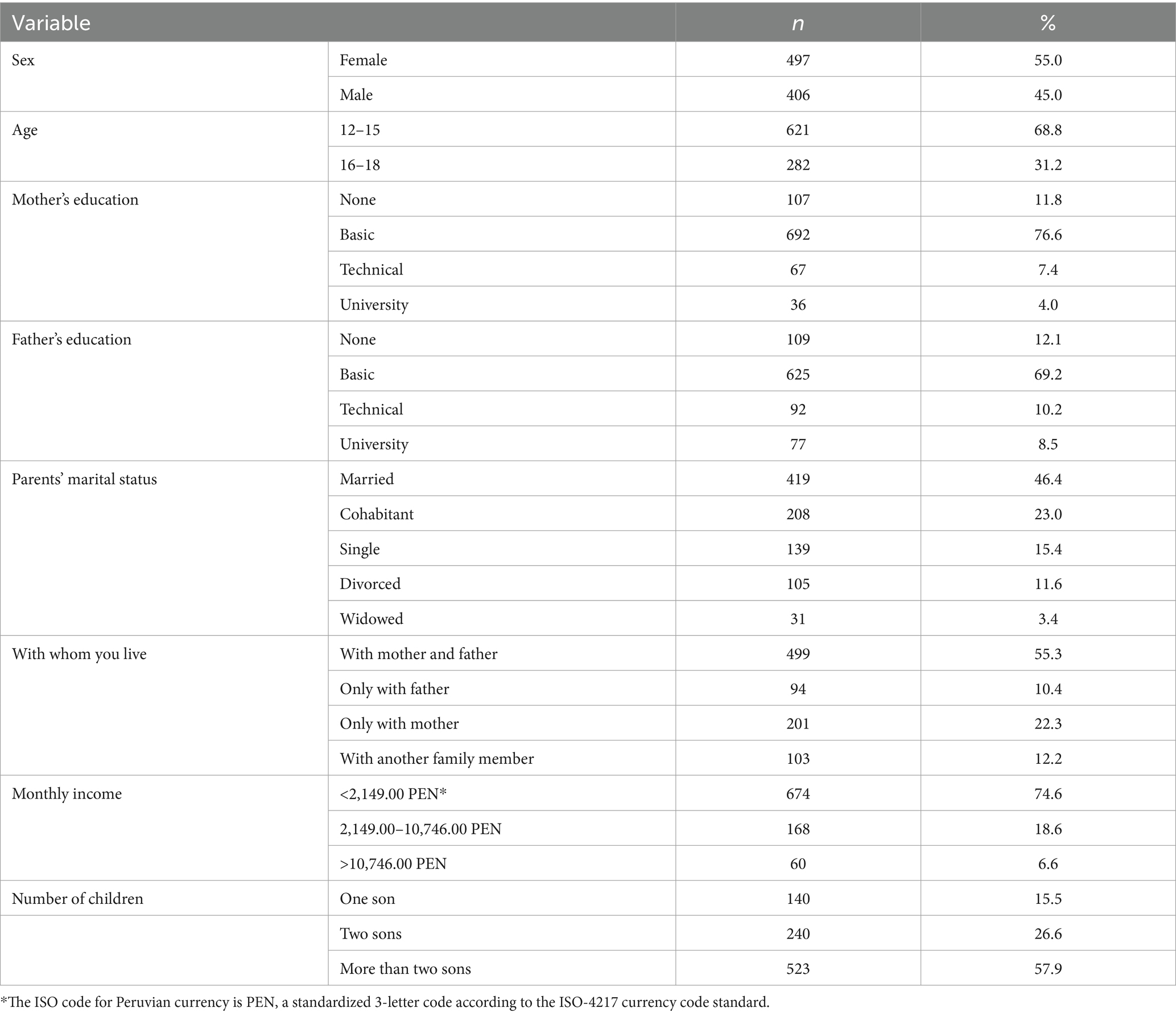
Table 1 . Sociodemographic characteristics of the participants ( N = 903).
The results of the association between H/A, BMI, SNA, and anxiety symptoms are shown in Table 2 . MetS was generally observed among men (67.1%, p = 0.046), in the age range of 16–18 (78.3%, p < 0.001), in those with excess body weight (90.1%, p < 0.001), SNA (85.5%, p = 0.039) and anxiety symptoms (63.2%, p = 0.007).
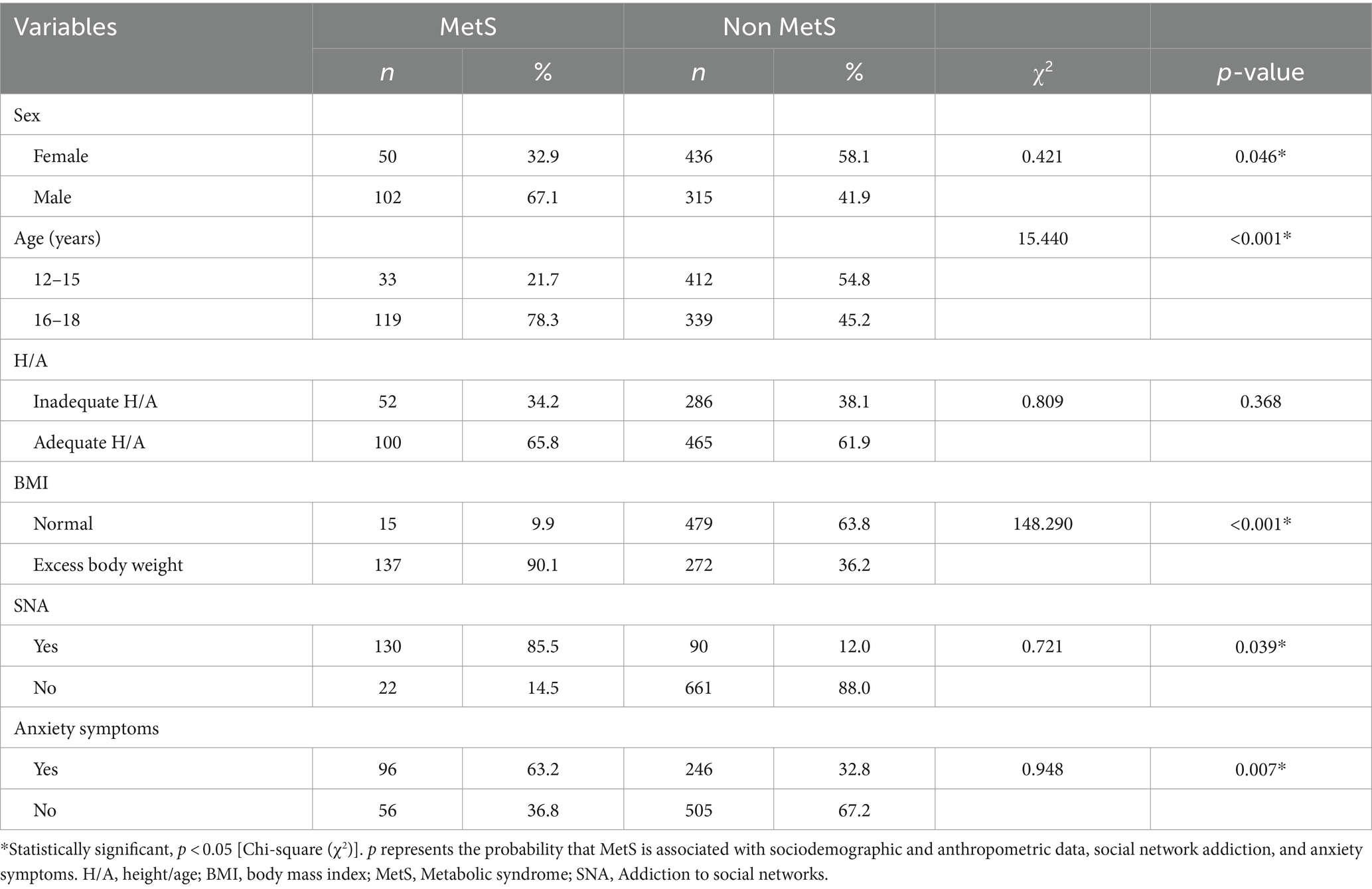
Table 2 . Chi-square analysis of MetS.
A binary logistic regression model was used to explore the variables that predict the probability that adolescents will present MetS and the results are shown in Table 3 . In this analysis, males were more likely to present MetS than females (OR = 1.133, p = 0.028). Participants who were 16 years of age or older and those with excess body weight were 2.166, p = 0.013 and 19.414, p < 0.001 times more likely to have MetS, respectively. Furthermore, SNA (OR = 1.517, p = 0.016) and the presence of anxiety symptoms (OR = 2.596, p < 0.001) were associated with the risk of MetS.
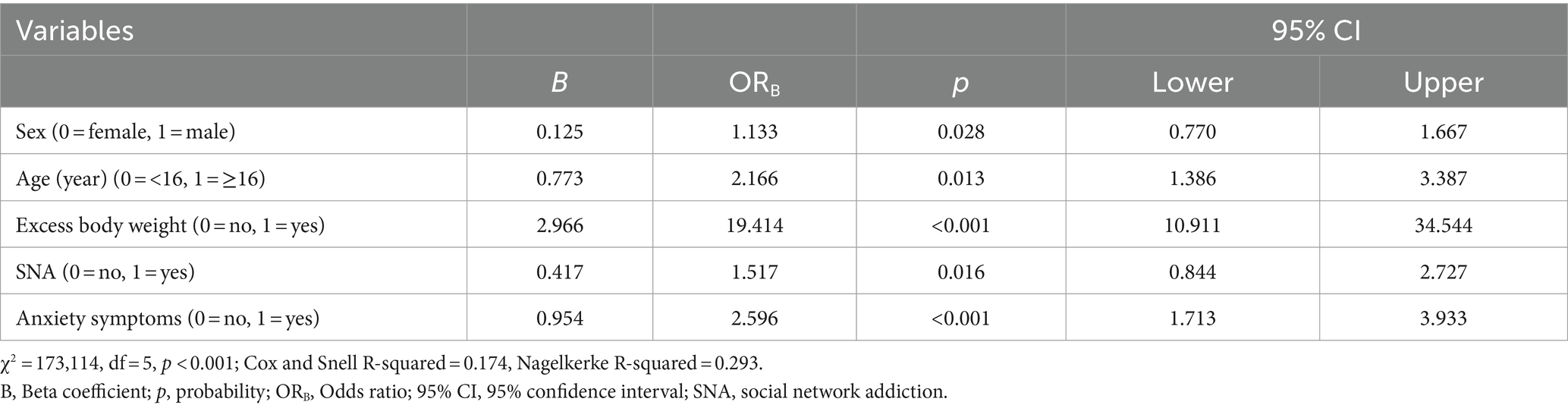
Table 3 . Binary logistic regression analysis of factors associated with MetS.
In this cross-sectional study, we determined the association between SNA and anxiety symptoms with the risk of MetS in Peruvian adolescents aged 12 to 18 years. The main findings were as follows: Male sex, participants who were 16 years of age or older and those who had excess body weight were more likely to present MetS. Furthermore, it is highlighted that SNA and anxiety symptoms were associated with MetS.
Previous studies have documented the influence of sex and age on the anthropometric profile, more precisely MetS, measured through the WHtR ( 4 , 41 – 43 ). The results of the logistic regression analysis of the current study revealed that men were more likely to have MetS compared to females; furthermore, we found that a higher proportion of men had MetS. It should be noted that the current study used WHtR to determine MetS. Similarly, the findings of the research conducted in Brazilian adolescents showed that boys had a higher mean WHtR and a higher WHtR at the 95 th percentile ( 41 ). Similarly, other studies in adolescents reported that boys were more likely to report MetS factors, such as high blood pressure, elevated cholesterol, glucose, and triglyceride levels ( 42 , 43 ). Although in some studies MetS patterns do not differ in both sexes ( 44 ), however, there are mechanisms that support evidence of sex differences ( 42 , 43 , 45 , 46 ). In general, it has been found that men have higher visceral adipose tissue, intramyocellular and intrahepatic lipids than women, which could partly explain why they have a higher MetS ( 46 ). On the other hand, males tend to have higher blood pressure and cholesterol levels than women from puberty onward, which can also contribute to a higher cardiovascular risk ( 42 , 43 ). In hormonal terms, it has been found that testosterone levels in men can negatively affect glucose metabolism and increase the risk of insulin resistance and type 2 diabetes, which are considered cardiometabolic complications ( 45 ).
Chronological age remains one of the strongest predictors of cardiometabolic events ( 47 ). In the present study, we found that participants who were 16 or older tended to report MetS; furthermore, an age ≥ 16 years was significantly associated with MetS. These findings are similar to the results reported in a recent study conducted in Peruvian adolescents where the highest proportion of those with MetS, measured by WHtR, were aged 15 to 17 years vs. 12 to 14 years ( 4 ). In addition, these results confirm the findings of research that measured waist circumference and WHtR in US children and adolescents ( 48 ). This study showed that the relative changes in WHtR increased with increasing age, and that the greatest relative change was observed in men and women between 18 and 19 years of age ( 48 ). Chronological age is an important determinant of health, since it coincides with the critical moments of increasing body fat and, therefore, of the development of diseases ( 49 ). In fact, as age increases, the risk of developing various chronic cardiometabolic diseases and conditions increases ( 48 , 50 ). In addition, the physical and mental changes that occur with age can affect the quality of life and a person’s ability to perform daily activities ( 51 ). However, it is important to note that the premature onset of age-related diseases in younger people suggests a discrepancy between chronological and biological age, pointing out that chronological age is not always representative of true biological age, because several disease/morbidity factors may be related to biological age ( 50 ). Beyond this discrepancy, it is important to consider age as an important factor in assessing adolescent health and in planning long-term disease prevention and treatment strategies.
Global obesity and MetS, measured using WHtR, are two anthropometric factors that are associated with the onset and development of noncommunicable diseases ( 1 ). The measurement of both factors is particularly important in adolescents, since adolescence is a high-risk stage and one of the most critical periods of life, due to constant changes in lifestyle ( 52 ). Evidence for the association between global obesity and MetS has been demonstrated in both adolescents and the general population ( 4 , 49 , 53 , 54 ). In our study, we found that those who had excess body weight were more likely to present MetS. These results are consistent with the findings of a study conducted in Spanish schoolchildren aged 6 to 15 years, showing a relationship between excess body weight and abdominal obesity, a metabolic risk factor ( 49 ). Similarly, the results of a recent study conducted in 506 adolescents aged 10 to 19 years of age from different schools in Brazil reported that normal weight obesity, which is defined as excess body fat in normal weight individuals, is associated with MetS, assessed through waist circumference ( 53 ). Some possible justification why obesity is related to increased waist circumference in adolescents is the fact that excess body fat accumulates predominantly in the abdominal region ( 1 ). It is worth mentioning that abdominal fat, also known as visceral fat, is metabolically active and can release pro-inflammatory fatty acids and adipokines, which contribute to the development of insulin resistance, dyslipidemia and other MetS factors ( 53 , 54 ). Therefore, assessment of MetS measured through WHtR in obese and normal weight adolescents can be useful to identify those with a higher risk of developing cardiovascular and metabolic diseases.
Another relevant finding of this study is the fact that SNA is associated with MetS. This connection is especially relevant in the current times since the use of social networks has become a widespread and popular activity among adolescents ( 8 ). Although there is a paucity of research analyzing the relationship between SNA and MetS, our findings are in line with a recent study that evaluated the relationship between adolescents’ social networks and their health in adulthood suggesting that their position in their social network during adolescence has lasting implications for MetS in adulthood ( 10 ). It should be noted that most studies have focused on BMI, pointing to a possible relationship between SNA and excessive media use with an increased risk of developing obesity in children and adolescents ( 11 – 13 , 55 ), which is related to cardiometabolic problems ( 1 , 4 ). Therefore, it is important to highlight that excessive exposure to digital platforms could have negative effects on the cardiovascular health of this population group through the onset of obesity ( 56 ). This suggests that the effect of SNA on MetS could be mediated by obesity. Although it is important to note that structural equation modeling was not used in this study to explore these relationships in depth, our findings provide a clear picture of how cardiometabolic disease is influenced by SNA and obesity. This sets the groundwork for future research using mediation models, which will help to better understand these relationships. The mechanism for this association may be that excessive and sedentary use of social networks reduces the time that would be devoted to physical activities ( 57 ). Studies that have provided evidence to support this theory show that when the amount of time adolescents spend in front of screens is reduced, their level of physical activity increases ( 12 ). Another reason for this relationship is the fact that it has been suggested that the consumption of hypercaloric foods can increase in parallel with the time spent on the media and social networks ( 58 , 59 ). This hypothesis is supported by research showing that high use of social networks among adolescents is associated with unhealthy dietary behaviors ( 60 ), and that energy intake in adolescents decreases when sedentary behaviors are reduced ( 59 ).
Finally, in this study, we found that anxiety symptoms are associated with MetS. Although little research has been done on the relationship between anxiety and metabolic risk factors in adolescents, however, a recent study has found a significant association between anxiety and some metabolic risk factors in this population group ( 18 ). Similarly, another study conducted in adolescents reported a relationship between anxiety symptoms and insulin resistance ( 19 ), which may lead to worsening metabolic outcomes in at-risk youth. Furthermore, studies have shown that anxiety symptoms are associated with an increased risk of cardiovascular disease and other metabolic problems in young adults ( 16 , 17 ). Anxiety is a common affective disorder in children and adolescents, affecting approximately 1 in 12 children and 1 in 4 adolescents, and is one of the most common mental health problems in these populations ( 14 ). One of the possible reasons why anxiety symptoms are associated with MetS is that this disorder can contribute to increased intake of unhealthy foods and decreased physical activity ( 61 ). In particular, it is possible that people with anxiety are more likely to have a sedentary lifestyle, increasing the risk of obesity and cardiovascular disease ( 61 ). Furthermore, anxiety symptoms can increase the release of stress hormones, such as cortisol, which can promote abdominal fat gain and insulin resistance ( 19 , 62 ).
Limitations and future research
When interpreting the results of this study, certain limitations should be taken into account, which will benefit future lines of research. First, the study was a cross-sectional design; therefore, it does not allow establishing the possibility of causality, that is, it cannot be considered that having SNA, anxiety symptoms, or excess body weight can lead to an increase in WHtR; therefore, longitudinal studies that follow participants over time are needed to determine whether the initial presence of SNA, anxiety symptoms, or overweight predicts future increases in WHtR. Second, in relation to the anthropometric data, this is cross-sectional information, where a single measurement was taken for each student and there is no follow-up data to evaluate the evolution of weight and height over time. Therefore, the results presented are based on single measurements taken in different age groups. Considering this, we cannot evaluate how these anthropometric parameters evolve with age. However, in the specific case of WHtR, an advantage of using this index is that it does not appear to be age dependent at certain levels and therefore it may be possible to use a single cut-off value for all children ( 63 ). Nonetheless, it is important to point out that there is no consensus on a single WHtR cut-off point to predict the risk of MetS risk in adolescents ( 64 ). Given the lack of consensus on a single WHtR cut-off point for predicting the risk of MetS in adolescents, further studies are needed to explore and validate uniform criteria. This could include comparative analyses of different cut-off values in different adolescent populations to identify those that are most predictive of MetS risk. Third, data on SNA and anxiety symptoms were self-reported, which may lead to measurement errors. However, both instruments were validated in the Peruvian population. Therefore, future research on anxiety symptoms could be based on medical diagnoses rather than self-report alone. Medical diagnoses provide a more objective and detailed assessment of anxiety status by combining clinical observations, medical records, and, in some cases, psychometric tests administered by professionals ( 65 ). Finally, it is important to note that the inability to generalize the findings to a larger population, due to the type of sampling used and the number of participants involved, is an obvious limitation in the current study. Therefore, it is important to interpret the results with caution and within the specific context of the selected sample group. In addition, studies using probability sampling methods are suggested to verify and expand on the findings of the current study.
Public health implications
Despite these limitations, we believe that the current study is of public health relevance due to its potential impact on the long-term health of adolescents. Adolescents are a vulnerable and growing population; therefore, habits and behaviors acquired at this stage can influence their health in adulthood. In addition, SNA, anxiety symptoms, and obesity are mental and physical health problems that have been increasing in the adolescent population in recent years. If these problems are related to an increased risk of MetS, they need to be addressed early and effectively. Therefore, it is important to conduct further research to confirm the relationship between these factors and to develop and implement preventive and treatment interventions targeting this vulnerable population. Furthermore, it is essential that health professionals, educators, and public health authorities inform and educate both adolescents and their parents about the importance of a healthy and balanced life, including responsible use of social networks, management of stress and anxiety, and maintaining a healthy weight.
The findings of this cross-sectional study suggest that men were more likely to have MetS compared to females; furthermore, we found that a higher proportion of men had higher MetS. Furthermore, it is notable that participants 16 years or older tended to report a higher level of MetS; furthermore, an age ≥ 16 years was significantly associated with the risk of MetS. Similarly, excess body weight, SNA, and anxiety symptoms were associated with the risk of MetS. Given the impact of MetS on health, more efforts are needed to better understand the associated factors for the implementation of effective preventive and therapeutic interventions.
Data availability statement
The raw data supporting the conclusions of this article will be made available by the authors, without undue reservation.
Ethics statement
The studies involving humans were approved by Research Ethics Committee of the Universidad Señor de Sipán (Registration and reference number: 0085-17052022-CIEI). The studies were conducted in accordance with the local legislation and institutional requirements. Written informed consent for participation in this study was provided by the participants’ legal guardians/next of kin.
Author contributions
JS: Conceptualization, Funding acquisition, Investigation, Methodology, Project administration, Supervision, Visualization, Writing – original draft, Writing – review & editing. SO-G: Conceptualization, Data curation, Funding acquisition, Investigation, Project administration, Resources, Writing – original draft. GL-T: Conceptualization, Methodology, Project administration, Resources, Visualization, Writing – original draft. IL-D-M: Conceptualization, Data curation, Funding acquisition, Writing – original draft. FB-C: Funding acquisition, Investigation, Methodology, Writing – original draft. EL-L: Conceptualization, Funding acquisition, Methodology, Project administration, Writing – review & editing. YC-M: Conceptualization, Data curation, Investigation, Project administration, Visualization, Writing – original draft, Writing – review & editing. AS-B: Investigation, Methodology, Validation, Visualization, Writing – review & editing. CR-V: Formal Analysis, Investigation, Methodology, Supervision, Visualization, Writing – review & editing.
The author(s) declare financial support was received for the research, authorship, and/or publication of this article. The study was financed by the Universidad Señor de Sipán through the Vice Rectorate for Research, Grant: 082-2022-PD-USS and approved by RESOLUCIÓN DE DIRECTORIO N° 079-2022-PD-USS.
Acknowledgments
The authors are grateful for the support provided by the Vice Rectorate for Research and the Research Department of the School of Sciences of Sipan University. We also thank Dr. Millones-Gómez Pablo A. and Dr. Pérez-Delgado Orlando for their administrative support in the execution of the study. Finally, we thank all parents and students who decided to participate in this study.
Conflict of interest
The authors declare that the research was conducted in the absence of any commercial or financial relationships that could be construed as a potential conflict of interest.
Publisher’s note
All claims expressed in this article are solely those of the authors and do not necessarily represent those of their affiliated organizations, or those of the publisher, the editors and the reviewers. Any product that may be evaluated in this article, or claim that may be made by its manufacturer, is not guaranteed or endorsed by the publisher.
1. Chung, ST, Onuzuruike, AU, and Magge, SN. Cardiometabolic risk in obese children. Ann N Y Acad Sci . (2018) 1411:166:183. doi: 10.1111/nyas.13602
Crossref Full Text | Google Scholar
2. Widjaja, NA, Arifani, R, and Irawan, R. Value of waist-to-hip ratio as a predictor of metabolic syndrome in adolescents with obesity. Acta Biomed . (2023) 94:e2023076. doi: 10.23750/abm.v94i3.13755
PubMed Abstract | Crossref Full Text | Google Scholar
3. PAHO/WHO. Obesity Prevention – PAHO/WHO | Pan American Health Organization [Internet]; (2021). Available at: https://www.paho.org/en/topics/obesity-prevention (Accessed May 10, 2023)
Google Scholar
4. López Malque, JJ, Chanducas Lozano, B, Calizaya-Milla, YE, Calizaya-Milla, SE, Morales-García, WC, and Saintila, J. Relationship between sleep quality, eating habits, and anthropometric profile in adolescents: a cross-sectional survey. Retos . (2023) 48:341–8. doi: 10.47197/retos.v48.96283
5. Tarqui-Mamani, C, Alvarez-Dongo, D, and Espinoza-Oriundo, P. Riesgo cardiovascular según circunferencia abdominal en peruanos. An Fac Med . (2017) 78:287–91. doi: 10.15381/anales.v78i3.13760
6. Curi-Quinto, K, Ortiz-Panozo, E, and López de Romaña, D. Malnutrition in all its forms and socio-economic disparities in children under 5 years of age and women of reproductive age in Peru. Public Health Nutr . (2020) 23:s89–s100. doi: 10.1017/S136898001900315X
7. Mader, L, Müller, KW, Wölfling, K, Beutel, ME, and Scherer, L. Is (disordered) social networking sites usage a risk factor for dysfunctional eating and exercise behavior? Int J Environ Res Public Health . (2023) 20:3484. doi: 10.3390/ijerph20043484
8. Bozzola, E, Spina, G, Agostiniani, R, Barni, S, Russo, R, Scarpato, E, et al. The use of social media in children and adolescents: scoping review on the potential risks. Int J Environ Res Public Health . (2022) 19:9960. doi: 10.3390/ijerph19169960
9. Pilař, L, Stanislavská, LK, Kvasnička, R, Hartman, R, and Tichá, I. Healthy food on Instagram social network: vegan, homemade and clean eating. Nutrients . (2021) 13:1991. doi: 10.3390/nu13061991
10. Kim, J, and Park, K. Longitudinal evidence on adolescent social network position and cardiometabolic risk in adulthood. Soc Sci Med . (2022) 301:114909. doi: 10.1016/j.socscimed.2022.114909
11. Khajeheian, D, Colabi, AM, Shah, NBAK, Radzi, CWJBWM, and Jenatabadi, HS. Effect of social media on child obesity: application of structural equation modeling with the Taguchi method. Int J Environ Res Public Health . (2018) 15:1–22. doi: 10.3390/ijerph15071343
12. Durmus, G, Ortabag, T, and Ozdemir, S. Determining the relationship between obesity and problematic internet use among adolescents. Iran J Public Health . (2021) 50:1796–804. doi: 10.18502/ijph.v50i9.7052
13. Koca, SB, Paketci, A, and Buyukyilmaz, G. The relationship between internet usage style and internet addiction and food addiction in obese children compared to healthy children. Turk Arch Pediatr . (2023) 58:205–11. doi: 10.5152/TurkArchPediatr.2023.22183
14. Dobnik, RB . Anksiozne motnje pri otrocih in mladostnikih. Psihološka obzorja . (2020) 29:1–8. doi: 10.20419/2020.29.505
15. Steinsbekk, S, Ranum, B, and Wichstrøm, L. Prevalence and course of anxiety disorders and symptoms from preschool to adolescence: a 6-wave community study. J Child Psychol Psychiatry . (2022) 63:527–34. doi: 10.1111/jcpp.13487
16. Skogberg, N, Castaneda, AE, Agyemang, C, Koponen, P, Lilja, E, and Laatikainen, T. The association of depressive and anxiety symptoms with the metabolic syndrome and its components among Russian, Somali, and Kurdish origin adults in Finland: a population-based study. J Psychosom Res . (2022) 159:110944. doi: 10.1016/j.jpsychores.2022.110944
17. Patterson, SL, Marcus, M, Goetz, M, Vaccarino, V, and Gooding, HC. Depression and anxiety are associated with cardiovascular health in young adults. J Am Heart Assoc . (2022) 11:e027610. doi: 10.1161/JAHA.122.027610
18. Ahmadi, N, Farahzadi, MH, Mohammadi, MR, Mostafavi, SA, Moeeini, SS, Shahvazian, N, et al. Relationship between anxiety disorders and anthropometric indices, risk factors, and symptoms of cardiovascular disorder in children and adolescents. Iran J Psychiatry . (2021) 16:409–17. doi: 10.18502/ijps.v16i4.7228
19. Bruggink, SM, Shomaker, LB, Kelly, NR, Drinkard, BE, Chen, KY, Brychta, RJ, et al. Insulin sensitivity, depression/anxiety, and physical fitness in at-risk adolescents. Sports Med Int Open . (2019) 3:E40–7. doi: 10.1055/a-0889-8653
20. Ato, M, López, JJ, and Benavente, A. A classification system for research designs in psychology. An Psicol . (2013) 29:1038–59.
21. Jager, J, Putnick, DL, and Bornstein, MH. More than just convenient: the scientific merits of homogeneous convenience samples. Monogr Soc Res Child Dev . (2017) 82:13–30. doi: 10.1111/mono.12296
22. Jiatong, W, Wang, Z, Alam, M, Murad, M, Gul, F, and Gill, SA. The impact of transformational leadership on affective organizational commitment and job performance: the mediating role of employee engagement. Front Psychol . (2022) 13:13. doi: 10.3389/fpsyg.2022.831060
23. Althubaiti, A . Sample size determination: a practical guide for health researchers. J Gen Fam Med . (2023) 24:72–8. doi: 10.1002/jgf2.600
24. Soper D. Software. A-priori sample size calculator for multiple regression; (2022). Available at: https://www.danielsoper.com/statcalc/ (Accessed January 4, 2022)
25. Escurra, M, and Salas, E. Contruccion y validacion del cuestionario de adiccion a redes sociales. Liberabit . (2014) 20:73–91.
26. González Alcántara, KE . Valoración psicométrica del Cuestionario de Adicción a Redes Sociales (ARS) en adolescentes mexicanos. Rev Psicol Clín Con Niños Adolesc . (2021) 8:26–34. doi: 10.21134/rpcna.2021.08.3.3
27. Staples, LG, Dear, BF, Gandy, M, Fogliati, V, Fogliati, R, Karin, E, et al. Psychometric properties and clinical utility of brief measures of depression, anxiety, and general distress: the PHQ-2, GAD-2, and K-6. Gen Hosp Psychiatry . (2019) 56:13–8. doi: 10.1016/j.genhosppsych.2018.11.003
28. Kroenke, K, Spitzer, RL, Williams, JBW, Monahan, PO, and Löwe, B. Anxiety disorders in primary care: prevalence, impairment, comorbidity, and detection. Ann Intern Med . (2007) 146:317–25. doi: 10.7326/0003-4819-146-5-200703060-00004
29. Huang, Y, Fietze, I, and Penzel, T. Analysis of the correlations between insomnia and mental health during the COVID-19 pandemic in Germany. Somnologie . (2022) 26:89–97. doi: 10.1007/s11818-022-00347-7
30. Plummer, F, Manea, L, Trepel, D, and McMillan, D. Screening for anxiety disorders with the GAD-7 and GAD-2: a systematic review and diagnostic meta analysis. Gen Hosp Psychiatry . (2016) 39:24–31. doi: 10.1016/j.genhosppsych.2015.11.005
31. Christensen, H, Batterham, PJ, Grant, J, Griffiths, KM, and MacKinnon, AJ. A population study comparing screening performance of prototypes for depression and anxiety with standard scales. BMC Med Res Methodol . (2011) 11:1–9. doi: 10.1186/1471-2288-11-154
32. Franco-Jimenez, RA, and Nuñez-Magallanes, A. Propiedades psicométricas del GAD-7, GAD-2 y GAD-Mini en universitarios peruanos. Propós Represent . (2022) 10:2022. doi: 10.20511/pyr2022.v10n1.1437
33. Hirschler, V, Aranda, C, De Luján, M, Maccalini, G, and Jadzinsky, M. Can waist circumference identify children with the metabolic syndrome? Arch Pediatr Adolesc Med . (2005) 159:740–4. doi: 10.1001/archpedi.159.8.740
34. Tarqui-Mamani, CB, Alvarez-Dongo, D, Espinoza-Oriundo, PL, Tarqui-Mamani, CB, Alvarez-Dongo, D, and Espinoza-Oriundo, PL. Analysis of the trend of height in Peruvian children and adolescents; 2007–2013. Rev Esp Nutr Hum Diet . (2018) 22:64–71. doi: 10.14306/452
35. Instituto Nacional de Salud. Informes y publicaciones. Guía técnica para la valoración nutricional antropométrica de la persona adolescente; (2015). Available at: https://www.gob.pe/institucion/minsa/informes-publicaciones/305911-guia-tecnica-para-la-valoracion-nutricional-antropometrica-de-la-persona-adolescente (Accessed May 4, 2023)
36. Wang, J, Thornton, JC, Bari, S, Williamson, B, Gallagher, D, Heymsfield, SB, et al. Comparisons of waist circumferences measured at 4 sites. Am J Clin Nutr . (2003) 77:379–84. doi: 10.1093/ajcn/77.2.379
37. Yoo, EG . Waist-to-height ratio as a screening tool for obesity and cardiometabolic risk. Korean J Pediatr . (2016) 59:425–31. doi: 10.3345/kjp.2016.59.11.425
38. Eslami, M, Pourghazi, F, Khazdouz, M, Tian, J, Pourrostami, K, Esmaeili-Abdar, Z, et al. Optimal cut-off value of waist circumference-to-height ratio to predict central obesity in children and adolescents: a systematic review and meta-analysis of diagnostic studies. Front Nutr . (2023):9. doi: 10.3389/fnut.2022.985319
39. Xiong, F, Garnett, SP, Cowell, CT, Biesheuvel, C, Zeng, Y, Long, CL, et al. Waist circumference and waist-to-height ratio in Han Chinese children living in Chongqing, south-West China. Public Health Nutr . (2011) 14:20–6. doi: 10.1017/S136898001000042X
40. Browning, LM, Hsieh, SD, and Ashwell, M. A systematic review of waist-to-height ratio as a screening tool for the prediction of cardiovascular disease and diabetes: 0·5 could be a suitable global boundary value. Nutr Res Rev . (2010) 23:247–69. doi: 10.1017/S0954422410000144
41. de Pádua Cintra, I, Zanetti Passos, MA, Dos Santos, LC, da Costa Machado, H, and Fisberg, M. Waist-to-height ratio percentiles and cutoffs for obesity: a cross-sectional study in Brazilian adolescents. J Health Popul Nutr . (2014) 32:411–9.
42. Vasquez, F, Correa-Burrows, P, Blanco, E, Gahagan, S, and Burrows, R. A waist-to-height ratio of 0.54 is a good predictor of metabolic syndrome in 16-year-old male and female adolescents. Pediatr Res . (2019) 85:269–74. doi: 10.1038/s41390-018-0257-8
43. Barstad, LH, Júlíusson, PB, Johnson, LK, Hertel, JK, Lekhal, S, and Hjelmesæth, J. Gender-related differences in cardiometabolic risk factors and lifestyle behaviors in treatment-seeking adolescents with severe obesity. BMC Pediatr . (2018) 18:61. doi: 10.1186/s12887-018-1057-3
44. Zhou, P . Gender differences in cardiovascular risks of obese adolescents in the Bronx. J Clin Res Pediatr Endocrinol . (2010) 2:67–71. doi: 10.4274/jcrpe.v2i2.67
45. Grossmann, M . Testosterone and glucose metabolism in men: current concepts and controversies. J Endocrinol . (2014) 220:R37–55. doi: 10.1530/JOE-13-0393
46. Schorr, M, Dichtel, LE, Gerweck, AV, Valera, RD, Torriani, M, Miller, KK, et al. Sex differences in body composition and association with cardiometabolic risk. Biol Sex Differ . (2018) 9:1–10. doi: 10.1186/s13293-018-0189-3
47. Kaptoge, S, Pennells, L, De Bacquer, D, Cooney, MT, Kavousi, M, Stevens, G, et al. World Health Organization cardiovascular disease risk charts: revised models to estimate risk in 21 global regions. Lancet Glob Health . (2019) 7:e1332–45. doi: 10.1016/S2214-109X(19)30318-3
48. Li, C, Ford, ES, Mokdad, AH, and Cook, S. Recent trends in waist circumference and waist-height ratio among US children and adolescents. Pediatrics . (2006) 118:e1390–8. doi: 10.1542/peds.2006-1062
49. Pérez-Ríos, M, Santiago-Pérez, MI, Leis, R, Martínez, A, Malvar, A, Hervada, X, et al. Exceso ponderal y obesidad abdominal en niños y adolescentes gallegos. An Pediatr (Engl Ed) . (2018) 89:302–8. doi: 10.1016/j.anpedi.2017.11.007
50. Emami, M, Agbaedeng, TA, Thomas, G, Middeldorp, ME, Thiyagarajah, A, Wong, CX, et al. Accelerated biological aging secondary to cardiometabolic risk factors is a predictor of cardiovascular mortality: a systematic review and meta-analysis. Can J Cardiol . (2022) 38:365–75. doi: 10.1016/j.cjca.2021.10.012
51. Youssef, A, Keown-Stoneman, C, Maunder, R, Wnuk, S, Wiljer, D, Mylopoulos, M, et al. Differences in physical and mental health-related quality of life outcomes 3 years after bariatric surgery: a group-based trajectory analysis. Surg Obes Relat Dis . (2020) 16:1837–49. doi: 10.1016/j.soard.2020.06.014
52. de Vasconcelos, HCA, Fragoso, LVC, Marinho, NBP, de Araújo, MFM, de Freitas, RWJF, Zanetti, ML, et al. Correlation between anthropometric indicators and sleep quality among Brazilian university students. Rev Esc Enferm . (2013) 47:851–8. doi: 10.1590/S0080-623420130000400012
53. Cota, BC, Priore, SE, Ribeiro, SAV, Juvanhol, LL, de Faria, ER, de Faria, FR, et al. Cardiometabolic risk in adolescents with normal weight obesity. Eur J Clin Nutr . (2022) 76:863–70. doi: 10.1038/s41430-021-01037-7
54. Correa-Rodríguez, M, González-Ruíz, K, Rincón-Pabón, D, Izquierdo, M, García-Hermoso, A, Agostinis-Sobrinho, C, et al. Normal-weight obesity is associated with increased cardiometabolic risk in young adults. Nutrients . (2020) 12:1106. doi: 10.3390/nu12041106
55. Chassiakos, YR, Radesky, J, Christakis, D, Moreno, MA, Cross, C, Hill, D, et al. Children and adolescents and digital media. Pediatrics . (2016) 138: 1–18. doi: 10.1542/peds.2016-2593
56. Burrows, R, Correa-Burrows, P, Rogan, J, Cheng, E, Blanco, E, and Gahagan, S. Long-term vs. recent-onset obesity: their contribution to cardiometabolic risk in adolescence. Pediatr Res . (2019) 86:776–82. doi: 10.1038/s41390-019-0543-0
57. Asiamah, N, Agyemang, SM, Yarfi, C, Jnr, RAM, Muhonja, F, Khan, HTA, et al. Associations of social networks with physical activity enjoyment among older adults: walkability as a modifier through a STROBE-compliant analysis. Int J Environ Res Public Health . (2023) 20:3341. doi: 10.3390/ijerph20043341
58. Sampasa-Kanyinga, H, Chaput, JP, and Hamilton, HA. Associations between the use of social networking sites and unhealthy eating behaviours and excess body weight in adolescents. Br J Nutr . (2015) 114:1941–7. doi: 10.1017/S0007114515003566
59. Jensen, ML, Dillman Carpentier, FR, Corvalán, C, Popkin, BM, Evenson, KR, Adair, L, et al. Television viewing and using screens while eating: associations with dietary intake in children and adolescents. Appetite . (2022) 168:105670. doi: 10.1016/j.appet.2021.105670
60. Kucharczuk, AJ, Oliver, TL, and Dowdell, EB. Social media’s influence on adolescents′ food choices: a mixed studies systematic literature review. Appetite . (2022) 168:105765. doi: 10.1016/j.appet.2021.105765
61. Helgadóttir, B, Forsell, Y, and Ekblom, Ö. Physical activity patterns of people affected by depressive and anxiety disorders as measured by accelerometers: a cross-sectional study. PLoS One . (2015) 10:e0115894. doi: 10.1371/journal.pone.0115894
62. Lenze, EJ, Mantella, RC, Shi, P, Goate, AM, Nowotny, P, Butters, MA, et al. Elevated cortisol in older adults with generalized anxiety disorder is reduced by treatment: a placebo-controlled evaluation of escitalopram. Am J Geriatr Psychiatr . (2011) 19:482–90. doi: 10.1097/JGP.0b013e3181ec806c
63. Aeberli, I, Gut-Knabenhans, M, Kusche-Ammann, R, Molinari, L, and Zimmermann, M. Waist circumference and waist-to-height ratio percentiles in a nationally representative sample of 6-13 year old children in Switzerland. Swiss Med Wkly . (2011) 141:1–16. doi: 10.4414/smw.2011.13227
64. Muñoz-Hernando, J, Luque, V, Ferré, N, Feliu, A, Closa-Monasterolo, R, Gutiérrez-Marin, D, et al. Diagnosis accuracy of waist-to-height ratio to predict cardiometabolic risk in children with obesity. Pediatr Res . (2023) 93:1294–301. doi: 10.1038/s41390-022-02223-4
65. Roseberry, K, Le-Niculescu, H, Levey, DF, Bhagar, R, Soe, K, Rogers, J, et al. Towards precision medicine for anxiety disorders: objective assessment, risk prediction, pharmacogenomics, and repurposed drugs. Mol Psychiatry . (2023) 28:2894–912. doi: 10.1038/s41380-023-01998-0
Keywords: adolescents, anxiety symptoms, cardiometabolic risk, metabolic syndrome, obesity, social network addiction
Citation: Saintila J, Oblitas-Guerrero SM, Larrain-Tavara G, Lizarraga-De-Maguiña IG, Bernal-Corrales FdC, López-López E, Calizaya-Milla YE, Serpa-Barrientos A and Ramos-Vera C (2024) Associations between social network addiction, anxiety symptoms, and risk of metabolic syndrome in Peruvian adolescents—a cross-sectional study. Front. Public Health . 12:1261133. doi: 10.3389/fpubh.2024.1261133
Received: 18 July 2023; Accepted: 16 April 2024; Published: 01 May 2024.
Reviewed by:
Copyright © 2024 Saintila, Oblitas-Guerrero, Larrain-Tavara, Lizarraga-De-Maguiña, Bernal-Corrales, López-López, Calizaya-Milla, Serpa-Barrientos and Ramos-Vera. This is an open-access article distributed under the terms of the Creative Commons Attribution License (CC BY) . The use, distribution or reproduction in other forums is permitted, provided the original author(s) and the copyright owner(s) are credited and that the original publication in this journal is cited, in accordance with accepted academic practice. No use, distribution or reproduction is permitted which does not comply with these terms.
*Correspondence: Jacksaint Saintila, [email protected] ; Yaquelin E. Calizaya-Milla, [email protected]
Disclaimer: All claims expressed in this article are solely those of the authors and do not necessarily represent those of their affiliated organizations, or those of the publisher, the editors and the reviewers. Any product that may be evaluated in this article or claim that may be made by its manufacturer is not guaranteed or endorsed by the publisher.
Social Network Analysis and Mining
- Serves a wide range of researchers from computer science, network science, social sciences, mathematical sciences, medical, biological, financial, management, and political sciences.
- 92% of authors who answered a survey reported they would definitely or probably publish in the journal again.
- It covers a broad spectrum of topics from social sciences, mathematics, statistics, physics, network science, and computer science.
- Aims to create a platform that encourages the integration of varied techniques in the analysis and mining of social networks.
This is a transformative journal , you may have access to funding.
- Reda Alhajj
Latest articles
Overview of the twitter conversation around #14f 2021 catalonia regional election: an analysis of echo chambers and presence of social bots.
- Raul Broto Cervera
- Cristina Pérez-Solà
- Albert Batlle
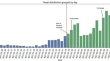
A temporal graph model to study the dynamics of collective behavior and performance in team sports: an application to basketball
- Quentin Bourgeais
- Rodolphe Charrier
- Ludovic Seifert
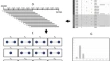
A comprehensive survey on community detection methods and applications in complex information networks
- Abdelhani Diboune
- Hachem Slimani
- Kadda Beghdad Bey
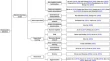
Uncertainty-aware graph neural network for semi-supervised diversified recommendation
- Thomas Tran
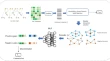
Sentiment analysis of tweets on social security and medicare
- Unmesh Kumar Chakravarty
- Shaikh Arifuzzaman
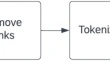
Journal updates
Asonam 2024 journal track.
Submission Deadline: May 31, 2024
CfP: Role of Social Network Analysis in Human Behaviour and Emotion Analysis for Business Intelligence
Submission Deadline: October 10, 2023
Guest Editors: Dr. Jungpil Shin, Dr. Md. Al Mehedi Hasan, Dr. Yong Seok Hwang
Call for Papers: Pervasive Health: Social Media Analytics and Sensing for Healthcare and well-being
Artificial intelligence (AI) is changing the landscape of healthcare and modern personalized precision medicine. With the increasing availability of healthcare data and rapid progress of machine learning algorithms, AI and big data analytics are enabling unprecedented analysis of healthcare. Social media networks, when combined with Big Data applications and health policymaking, enable the development of smart public health applications that will result in high-quality health delivery and reduced costs.
Special Issue on Collective intelligence on Web and Social Media
Deadline for Submission: 28 February, 2023
Journal information
- ACM Digital Library
- EI Compendex
- Emerging Sources Citation Index
- Google Scholar
- Japanese Science and Technology Agency (JST)
- Norwegian Register for Scientific Journals and Series
- OCLC WorldCat Discovery Service
- TD Net Discovery Service
- UGC-CARE List (India)
Rights and permissions
Springer policies
© Springer-Verlag GmbH Austria, part of Springer Nature
- Find a journal
- Publish with us
- Track your research
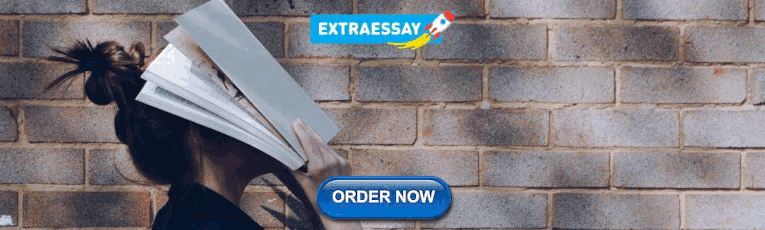
IMAGES
VIDEO
COMMENTS
Social network-based applications like Facebook, Twitter, and Instagram have been used by people of all age groups and backgrounds for the last few years. It is a rich platform for sharing knowledge amongst users online. This information is shared as feelings, opinions, interests, events, or comments in large volumes and varied forms of data. Many multidisciplinary researchers have conducted ...
Social network analysis (SNA) is a core pursuit of analyzing social networks today. In addition to the usual statistical techniques of data analysis, these networks are investigated using SNA ...
Social network analysis is the application of network science on social networks, i.e., social phenomena are represented and studied by data on overlapping dyads as the units of observation (Brandes et al., 2013c ). Consequently, graphs are a straightforward and convenient mathematical representation that will be the basis of this article.
Social network analysis is a large and growing body of research on the measurement and analysis of relational structure. Here, we review the fundamental concepts of network analysis, as well as a range of methods currently used in the field. Issues pertaining to data collection, analysis of single networks, network comparison, and analysis of ...
This paper is structured as follows: Section 2 contains the scientometric analysis performed to highlight the different research areas with high impact in SNA research field. Section 3 provides an analysis of the different concepts and research works that belongs to the SNA research field from the Computer Science point-of-view. The different application domains in SNA, as well as the emerging ...
Social network analysis (SNA) is a core pursuit of analyzing social networks today. In addition to the usual statistical techniques of data analysis, these networks are investigated using SNA measures. It helps in understanding the dependencies between social entities in the data, characterizing their behaviors and their effect on the network ...
Social network analysis is an applicable method for investigating relations and interactions. Social network analysis (SNA) is an approach rooted in anthropology, sociology and social psychology for assessing social structures [3, 16].The social network perspective illustrates social systems as networks of various relationships [2, 12].SNA centers upon the structure and design of relationships ...
This review of social network analysis focuses on identifying recent trends in interpersonal social networks research in organizations, and generating new research directions, with an emphasis on conceptual foundations. It is organized around two broad social network topics: structural holes and brokerage and the nature of ties. New research directions include adding affect, behavior, and ...
Online social networks (OSNs) are part of daily life of human beings. Millions of users are connected through online social networks. Due to very large number of users and huge amount of data, social network analysis is a challenging task. The emergence of deep learning techniques has enabled to carry out a rigorous analysis of OSNs. A lot of research is carried out in the area of social ...
Just like the basic logic of entropy, social network analysis estimates the probabilities of basic units of interactions through exponential random graph models, as Equation (2) presents: (2) In Equation (2), the variable Y encompasses the entire set of potential ties within a fixed social network comprising n actors.
The potential of data mining techniques for the analysis of available data sources is beginning to be recognised across the social sciences (see Savage and Burrows 2007), and the formation of this journal and of Advances in Social Network Analysis and Mining (ASONAM) is a mark of its potential for the field of social network analysis.The new techniques of network analysis are most appropriate ...
The representation and analysis of community network structure remains at the forefront of network research in the social sciences today, with growing interest in unraveling the structure of computer-supported virtual communities that have proliferated in recent years ( 12 ). By the 1960s, the network perspective was thriving in anthropology.
Social network analysis is the process of investigating social structures through the use of networks and graph theory. It combines a variety of techniques for analyzing the structure of social networks as well as theories that aim at explaining the underlying dynamics and patterns observed in these structures. It is an inherently interdisciplinary field which originally emerged from the ...
Of course, it will maximize the power of social network analysis if we directly and explicitly test, in the data analysis stage, the causal linkages (or lack of it, for that matter) among the ...
human societies, social networks have been there forging individual and collective behavior. In the academia, research on social networks can be traced to the rst decades of the twentieth century (Rice, 1927), while probably the most in uential early work on social network analysis was the seminal paper\Contacts and In uence"(de Sola Pool and ...
pairwise ties, a social network should not be equated with social group. There are two concepts of a social group: realist and nominalist. The realist concept is most commonly used in sociological parlance. According to this concept, it is an entity consisting of social actors such as individuals, families, and so on and is set apart from the rest.
1. Introduction. The SNA is a theory investigating the relations and interactions based on anthropology, sociology, and social psychology to assess social structures (Erçetin and Neyişci, 2014).The social structure in a network theory comprises individuals or organisations named nodes linked through one or more types of interdependencies, such as friendship, kinship, financial exchange ...
Abstract. In this article, social network analysis (SNA) is defined and historical development process is explained. A comprehensive literature search has been conducted for this purpose. SAA is a ...
Despite the earlier claim of "Death of Distance", recent studies revealed that geographical proximity still greatly influences link formation in online social networks. However, it is unclear how physical distances are intertwined with users' online behaviors in a virtual world. We study the role of spatial dependence on a global online social network with a dyadic Logit model. Results show ...
Introduction. The close of the fifteenth anniversary of the special issue of the journal Social Networks on 'Ethical dilemmas in social network research' 1 provides the chance for both a critical assessment and a reconceptualization of the matters at stake. If the motivation for that earlier publication was a glaring lack of references and sources for practitioners of social network ...
Pajek: Pajek is a program for the analysis and visualization of large networks. It's an extensive tool, offering a range of complex network metrics, and is free for non-commercial use. NodeXL: NodeXL is a free, open-source template for Microsoft Excel that allows users to display and analyze network graphs.
This paper presents the analysis of journals publishing articles on social network analysis (SNA). The dataset consists of articles from the Web of Science database obtained by searching for "social network*", works intensively cited, written by the most prominent authors, and published in the main SNA journals up to July 2018. There were 8943 journals in 70,792 articles with complete ...
Using network analysis, it quantifies cooperative relations between states on space matters to examine the current state of international cooperation in space and its evolving dynamics. It finds that international cooperation in space has become increasingly defined by the emergence of distinct clusters of states, termed 'space blocs'.
Associations between social network addiction, anxiety symptoms, and risk of metabolic syndrome in Peruvian adolescents—a cross-sectional study ... In this analysis, males were more likely to present MetS than females (OR = 1.133, p = 0.028). Participants who were 16 years of age or older and those with excess body weight were 2.166, ...
Overview. Social Network Analysis and Mining is a multidisciplinary journal focusing on theoretical and experimental work related to social network analysis and mining. Serves a wide range of researchers from computer science, network science, social sciences, mathematical sciences, medical, biological, financial, management, and political ...
Huntington LS, Webster KE, Devitt BM, Scanlon JP, Feller JA. Factors associated with an increased risk of recurrence after a first-time patellar dislocation: a systematic review and meta-analysis. Am J Sports Med. 2020;48(10):2552-2562.