MockQuestions
Morningstar Data Analyst Mock Interview
To help you prepare for a Data Analyst interview at Morningstar, here are 40 interview questions and answer examples.
Morningstar was written by William Swansen on June 16th, 2022. Learn more here.
Question 1 of 40
Can you recall a time you were assigned a task that wasn't part of your job description? How did you handle this, and what was the outcome?
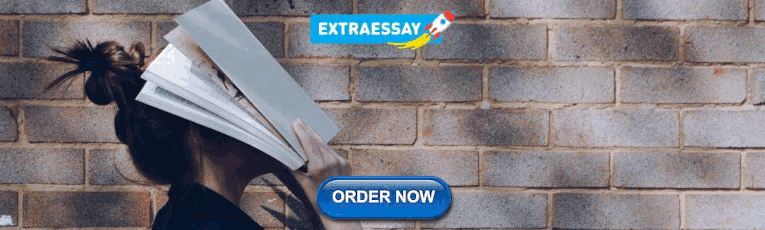
Why the Interviewer Asks This Question
How to answer, answer example.
Some employees are reluctant to do anything that is not part of their job description. However, in today's fast-paced business environment, people are often asked to do extra work that may not have been part of their original assignment when they were hired. Morningstar interviewers seek to understand how you react when asked to do something not part of your normal job and determine how flexible you are.
The best way to answer this is to start by stating that you are always open to pitching in wherever you can to help Morningstar get the job done. Emphasize that you are open to learning new skills that will help you and Morningstar. Then describe an incident to illustrate this.
"I have worked for several small companies where the employees wore many hats. I enjoyed this experience because it allowed me to learn new skills as an information security manager outside of my profession. It helped me grow my portfolio of skills and contributed to each company's success. In one case, I was asked to attend a trade show to research new data analysis tools from a technical perspective. Even though I had no experience in this area, I accepted the assignment with enthusiasm. Attending the show and interfacing with the suppliers taught me a great deal about how products are developed and marketed, which helped me evaluate new product features and make recommendations for adopting them to our management team."
Next Question
Morningstar Data Analyst Interview Questions & Answers
Below is a list of our Morningstar interview questions. Click on any interview question to view our answer advice and answer examples. You may view 5 answer examples before our paywall loads. Afterwards, you'll be asked to upgrade to view the rest of our answers.
1. Can you recall a time you were assigned a task that wasn't part of your job description? How did you handle this, and what was the outcome?
Written by William Swansen on June 16th, 2022
2. Tell me about the audiences to whom you've presented. Were these presentations done in person or remotely?
Hiring managers look for data analysts with strong presentation skills who can present analyses and answer questions clearly and confidently. In many cases, hiring managers look for candidates who can present their findings to people with different backgrounds. Experience communicating with both technical and non-technical audiences is a highly valued skill.
It would be ideal if you had experience presenting to an executive-level audience, but this is not always necessary. When describing your audiences, include approximate size, whether it included executives, and possibly what departments within the company were present. With the rise of remote work, some hiring managers will expect you to discuss presenting analyses via phone or video conference calls since these types of presentations can present unique challenges.
"As a data analyst, I have presented to a wide variety of audiences made up of people with differing backgrounds. The groups ranged in size from one person to 25 people, with the larger ones composed of co-workers from different departments in the company. Most of these presentations were in person, but I have presented a few analyses remotely via video conferencing to smaller audiences. In addition, about one-third of my presentations have had audiences made up of senior managers."
Written by Helen Lee on June 16th, 2022
3. Of the industries you have worked in, from a data perspective, which industry is most similar to the one in which Morningstar competes?
Interviewers at Morningstar will only pose this question if you do not have industry-specific experience related to their company. Without this experience, they want to know how you can apply what you learned from previous positions to the one you are interviewing for. You can be ready to answer this question by conducting research ahead of time.
Morningstar hires candidates based on their skills and abilities to do the job. They also look for a good organizational fit. Specific industry experience is the third most important criterion when evaluating job seekers. If you do not have direct experience within Morningstar's industry, research it before the interview. Come to the interview understanding the unique challenges this industry presents, solutions to overcome these, and practices commonly used by companies within the industry. This will help you answer this question effectively.
"There are a few similarities between the financial services and healthcare industries, specifically related to data and the work of a data analyst. One of the most important is the security of customer or patient data. Both industries work with highly personal and sensitive data that must be kept secure at all times. Because of this, access to data may be more restricted, and analyses may require more time to complete as you navigate the security. To be successful as a data analyst in these industries, you will need to be not only organized but able to present a clear case for the data your analyses require."
4. Describe your experience creating dashboards. If you have experience, what tools have you used to create them?
Dashboards are snapshots of Key Performance Indicators (KPIs) and metrics. Morningstar managers and their teams use them to track a particular business objective or goal. With the input of others, data analysts are often asked to build and update dashboards. Several tools can be used to build dashboards, including Tableau, Power BI, and Excel. In addition to these well-known tools, many free tools are available online.
If you do not have experience building dashboards, discuss how your data visualization skills can help you in this area. Do not describe your experience in too much detail, but include the purpose of the dashboard, the types of data visualizations you used, and a few metrics you included. If the Morningstar interviewer would like more detail, they will ask additional questions.
"I have experience creating dashboards that included Marketing metrics. The metrics included brand awareness, customer satisfaction, and sales by quarter. I used pie charts, bar and line graphs, and tables to present the data in the dashboard. I have created dashboards using both Power BI and Excel."
5. Which, if any, certifications have you earned related to your career as a data analyst?
Hiring managers at Morningstar will be interested in the training you have received for your job as a data analyst. Certifications ensure that you have attained a level of achievement set by industries or specific vendors. It provides a form of measurement for hiring managers to assess skills or knowledge of a particular subject or tool.
When answering this question, briefly explain how your certifications have helped you in your career as a data analyst. If you have yet to earn any certifications, consider mentioning which ones you may be interested in pursuing in the future and why. Interviewers prefer candidates who are willing to learn and interested in constantly improving their skills and competencies.
"Based on my skills, experiences, and education, I have earned the designation of a Certified Analytics Professional. Earning this designation also required me to take a certification exam that measured my knowledge in the field of Data Analytics. I take a recertification exam every three years to ensure that my skills and knowledge are up to date. The requirements of this certification drive me to advance my analytics education and equip me with a stronger toolset to execute my job as a data analyst."
6. Can you explain the difference between data mining and data profiling?
Morningstar interviewers use this question to analyze your competency and qualifications for the data analyst job. Knowing the subtle differences between these two concepts indicates that you've worked in this field and have a good understanding of the practices and procedures data analysts use. You should anticipate many questions like this one during the interview with Morningstar.
This technical question asks you to compare two concepts used by data analysts. Keep your answers brief and to the point when responding to technical questions. The Morningstar interviewer will ask you a follow-up question if they need additional information or want to explore this topic in more detail.
"Data Mining is the process of identifying information in large datasets. It can also be used for sequence recovery and analyzing data clusters. Data profiling helps data analysts at Morningstar identify the dataset's characteristics, including its type, length, frequency, and other identifiers. Both of these techniques help data analysts ensure that the dataset they are working with is appropriate for the task they are trying to complete."
7. And how do you deal with inconsistencies in your data?
The recommendation data analysts provide to decision-makers is only as good as the quality of data they are analyzing. If the data is inconsistent, the conclusions drawn from its analysis could contain errors and be misleading. Morningstar interviewers want to ensure that you are aware of this and have processes to clean and sanitize the datasets you are working with. This ensures that your analysis will produce valid recommendations the Morningstar decision-makers can use confidently.
As an experienced data analyst, you have many different processes and procedures you use in your work. You should review these before the interview at Morningstar to keep them at the top of your mind. During the interview, they'll likely ask many questions about how you do this job. As with any operational question, keep your answer brief and to the point, and always anticipate a follow-up question.
"I use several techniques to identify and correct inconsistencies in my data sets. I prefer the central semantic storage approach to prevent data inconsistencies. That helps create a central area for the data that can be used as a reference when processing the complete data set. If an inconsistency still occurs, I typically use a primary key to link to a table so I can re-enter the correct data. This approach has worked well for me in my previous positions."
8. How would you remove the data in a single cell without affecting the formatting when working with an Excel spreadsheet?
Microsoft Excel spreadsheets are one of the primary tools data analysts use in their profession. Interviewers will likely ask you many questions about how to use Excel spreadsheets to determine your knowledge and experience in this area. By asking the question, they indicate that Morningstar uses Excel and expects its employees to be highly competent with this tool.
One of the mistakes many candidates make during an interview is expounding on a very simple answer and providing too much detail to the interviewer. Interviewers often interpret this as trying to cover up a lack of knowledge or experience with the topic you are discussing. When answering technical questions like this, keep your answer brief and to the point. The Morningstar interviewer will always ask you a follow-up question if they need more information or want to explore the topic in more detail.
"The easiest way to remove data from an Excel spreadsheet cell without impacting the formatting is to select Clear Contents from the Editing menu. That will not affect any other properties of the cell."
9. Can you briefly explain your primary responsibilities as a data analyst?
The interviewer will ask this basic question early in the Morningstar interview. It has several purposes. First, it's an easy way for the interviewer to begin asking you questions related to this job. Second, they can use it to level-set your view of this job compared to Morningstar's. Interviewers want to make sure that you clearly understand the role you are interviewing for and the duties you are expected to perform.
You should review the Morningstar job posting several times when preparing for an interview and highlight the responsibilities and duties. While you may know what a data analyst does, Morningstar may have specific expectations for this role. Your answer to the question should align with the job posting. That will start you on the right track during the interview and convince the interviewer that you understand the role and have the necessary background to perform the job.
"The key responsibilities of a data analyst are to collect, manage, and process data for the organizations they work for. We need to ensure the accuracy of the information and recommendation we proved to help decision-makers make data-driven decisions. I also focus on implementing preventative measures to keep the data secure and clean, using critical thinking to compensate for any abnormalities in the data, and paying strict attention to detail."
10. What are the steps you take when beginning a new data analysis project?
Morningstar prefers to hire experienced data analysts who have developed specific processes and procedures to do their job. Interviewers will ask you about these during the interview to ensure that you have them and to determine if they align with the processes used by their data analytic organization. The more specific and detailed your process, the more comfortable the interviewer will be in recommending you to be hired.
Before attending an interview at Morningstar for a data analyst position, take some time to review the processes, procedures, tools, and concepts used in this job. Having these fresh in your mind will enable you to answer the interviewer's questions about how you perform this job. Keep your answer brief and to the point when responding to a question. The interviewer will always ask you a follow-up question if they want to explore the topic in more detail.
"When starting a new data analysis project, I begin by taking some time to review the project. This ensures that I clearly understand the objectives or problems I have been asked to resolve. Next, I find out how reliable the data is and where it originates. I determine if I need to scrub or sanitize the data before beginning the analysis. I then think about the best methods for modeling it and consider whether the deadline I have been assigned is realistic for the task at hand. After that, I carefully process the data and cross-reference it to a database to ensure accuracy."
11. Describe a project in which quantitative and qualitative data were used to conduct your analysis.
Data analysts should use all the available data to conduct the most impactful analyses. This could include both quantitative and qualitative data. The Morningstar hiring manager wants to know how much experience you have, marrying qualitative to quantitative data. Sometimes it is straightforward, as is the case when working with survey data that has both qualitative and quantitative questions. Other times, it may take creativity to find applicable qualitative data to use in conjunction with your quantitative data.
Morningstar interviewers often ask this question for a data analyst position, so you should anticipate it during the interview. Come to the interview prepared with stories about several projects you've worked on that demonstrate various skills you've used. You can repurpose the stories to address specific questions by including details the Morningstar interviewer asks you about. If you have several projects to choose from, share the project where you used the most creativity in merging the two types of data when answering this question.
"If possible, I always try to incorporate qualitative data to support what the quantitative data tells me. I have been fortunate to have conducted several analyses where qualitative survey data was readily available. However, when working with survey data, I don't think you should limit yourself to the qualitative data from one survey. When appropriate, I have found that there can be valuable qualitative data from other surveys or external sources. For one marketing analysis dealing with a new product evaluation, I reached out to the operations department to utilize qualitative data they had collected from distributors. Using this qualitative data strengthened the validity of my recommendations to the product development group."
12. Tell me about an aspect of your profession that makes you the most satisfied, energized, and productive at work.
The interviewer asks this question to uncover your passion and what you enjoy doing. Morningstar interviewers know that people do their best work when they are passionate about what they do. They will ask questions like this to discover if you are passionate about this job or just doing it for the money.
This is a great question to ask yourself before beginning your job search. It will help you target specific jobs that you enjoy doing and will therefore be good at. That will enable you to answer this question when asked by an interviewer by simply describing a task you enjoyed working on related to the job you are applying for.
"One of my favorite parts about this profession is collaborating with Morningstar employees from other departments. I enjoy working together to determine how to achieve the business's objectives. Participating as a member of a creative team is one of the best aspects of this job."
13. Can you give me an example of when you had to work with someone difficult to get along with? How did you handle the situation?
This is a behavioral question to which the Morningstar interviewer expects a 'STAR' formatted answer: Situation, Task, Action, Result. Interviewers ask behavioral questions to determine how you react to challenging situations in the workplace. Your description of how you handled this in a previous job will indicate what you will do if hired by Morningstar. Behavioral questions typically involve challenges, relationships, conflict, or communication errors.
When responding to this question, use the 'STAR' format to frame your answer: Situation, Task, Action, Result. Start by describing the Situation, followed by a brief explanation of the Task you were attempting to complete. Walk the interviewer through the Actions you took, then conclude by discussing the Results you attained and how these benefited the organizations. Describe a situation that you are likely to encounter when hired by Morningstar.
"In one of my recent jobs, I was partnered with another employee who was not open to new ideas and suggestions. Their attitude was 'my way or the highway.' We were tasked to develop a new process for using advanced data analytics techniques more effectively. I asked for their ideas and noted that while they were good, it would be more efficient to modify them slightly. We developed a consensus about the new process by accepting their initial suggestions and only recommending minor modifications. We recommended this to management, and the new process was implemented. This resulted in significant savings for the company and made it easier for my colleague and me to work together on future projects."
14. Please tell me about a time something major didn't go according to plan at work.
Responding to situations that don't go according to plan is a characteristic that Morningstar interviewers look for. They will ask you a question like this to determine how you react to unforeseen circumstances. Your answer provides them with an indication of your flexibility, responsiveness, and creativity.
Since this is a behavioral question, you should utilize the 'STAR' response methodology. Make sure to stay positive, don't blame anyone else for the problem, and don't take full credit for the solution. Demonstrate how you worked with others to resolve the situation. As with any behavioral question, discuss the results you attained and possibly the lessons learned, especially if the outcome wasn't optimum.
"During a recent software update project, the versions of the software we ordered were not correct. We reviewed the purchase order and determined that the software had been ordered incorrectly. Since the software seals were broken, we couldn't return the product, nor was the manufacturer likely to agree to correct this because it was our error. Even though this involved additional expense, my manager and I agreed it would be quicker to order the correct versions of the software so we could proceed with the upgrade. We did this and installed the software without a major delay in the project. This experience taught us to carefully review the software versions we had and needed before ordering new products or performing any upgrades."
15. Describe a situation where you needed to persuade someone about an idea or process.
The Morningstar interviewer will ask you this question because they are interested in learning about your communication and leadership skills and how you apply them to accomplish the tasks required in this role. Persuading other people about your ideas and suggestions is a valuable skill. Interviewers recognize that this will make you more effective in the job and reduce conflict between you and other team members.
You can answer this question using the STAR format, describing how you seek to understand other people's points of view, acknowledging them, then offering them an alternative and the rationale behind it. You can then describe how you addressed any questions and concerns they had and developed a win-win scenario with a positive outcome.
"I take great pride in my ability to convince others of my ideas and suggestions. First, I solicit others' input, carefully listening to their ideas and acknowledging them. If their suggestions are appropriate, we move forward. However, if I believe we can improve on them, I state my ideas and recommendations and explain why I believe in them. I then answer any questions they may have and drive for a consensus in which all the parties are satisfied we are moving in the right direction."
16. Give me an example of a time you led by example. Describe what you did and how your team reacted.
Interviewers ask this question to prompt you to talk about your leadership style, hoping you will describe how you lead from the front by example rather than from the back by exercising your authority. They recognize that teams perform better when they believe in their leadership and are willing to follow their example. By asking this question, the Morningstar interviewer hopes to discern whether you will be an effective leader.
Since this is a behavioral question, format your answer in the STAR framework by first describing a Situation and the Task you were required to complete. Then, tell the interviewer how you Acted by demonstrating the behavior you needed the team to exhibit. Finally, discuss the outcome of the project or task and what your team learned from the example you set. Make sure you communicate how you led by example and how this motivated the team to accomplish the assigned task.
"In my most recent role, my team and I were tasked with implementing a new process. Since none of us had experience in this area, I researched the process and created a training curriculum. I presented this to the team, participating in the exercises which simulated the new process. Together, we debugged the process, created an implementation plan, and launched the process. By fully participating in each aspect of the project, I demonstrated to the team that I was willing to roll up my sleeves and work alongside them to make the project successful. They acknowledged this and expressed their willingness to replicate the process on future projects."
17. Tell me about a difficult decision you had to make in one of your previous roles.
The Morningstar interviewer wants to hear examples of your decision-making process and how you approach challenges or difficult situations. They want to learn more about how decisive you are and whether you are willing to make difficult choices. The interviewer hopes to see that you will put Morningstar's interests ahead of your personal feelings.
When answering this question, choose a situation in which you made a decision involving a personal sacrifice or two equally undesirable outcomes. Explain the choice you made, your rationale, and the outcome. Then briefly discuss what, if anything, you would have done differently based on the outcome.
"During a recent downturn in business, I was required to reduce my staff. Every team member was well qualified and valuable to the organization, so choosing who to let go was difficult. After much consideration, I decided to dismiss one of the more experienced workers, knowing that they could easily find another job within the industry with my recommendation. This allowed me to develop one of the junior staffers, increasing their skills and making them more valuable to the organization. I would make the same decision if presented with this situation again at Morningstar."
18. Can you recall a time your manager was unavailable when a situation arose that demanded an immediate resolution? How did you react?
While this appears to be a question about leadership, it addresses your willingness to take the initiative. The Morningstar interviewer is interested in this because it helps them determine your future growth potential. Morningstar likes to hire individuals who continually improve themselves and develop new skills, allowing them to advance within the company.
When responding to this question, emphasize your willingness to take the initiative rather than complain about the manager's absence. Explain why you felt the need to take action and describe how you did so to resolve the immediate situation and then review the issue and your actions with your manager. Be prepared to answer a follow-up question about what you would do differently next time.
"Recently, my manager was away on vacation and asked me to fill in for them. A conflict arose between our team and another department that demanded an immediate resolution. The manager from the other department insisted we do what they wanted since our manager was not available. Knowing this was not the best solution, I presented an alternative and recommended that we discuss this with the senior leadership team to get their input. The other manager agreed. Working with senior leaders, we developed a compromise that resolved the conflict. When my manager returned from vacation, I briefed them on the incident, and they agreed I had acted appropriately."
19. When was the last occasion you asked for direct feedback from your manager?
The Morningstar interviewer will ask this question to separate you from most applicants because many professionals don't ever seek feedback from their supervisors. Interviewers prefer candidates who constantly seek feedback so they can immediately correct any deficiencies they may have or incorrect actions they are taking. The best professionals know the only way to get better news is to engage their colleagues for feedback and suggestions.
Requesting feedback from the people you work with shows your desire to improve. Most employees avoid receiving feedback, fearing that it will be negative. By actively seeking it, you demonstrate courage, a willingness to be open to criticism, and the initiative to improve. Describe a time you felt you didn't complete a task properly and sought feedback to correct your process so you'd do better next time.
"I have found that one of the best ways to improve myself is to continually seek feedback from the people I work with. My colleagues and management team have perspectives I don't and can spot weaknesses I need to work on to improve. Therefore, I am constantly asking them about my performance. I also ask them for any suggestions they have and resources I can use to improve my skills and expertise. I do this continuously, and it has been very valuable."
20. Why did you choose to interview with Morningstar rather than with others in our industry?
You should anticipate being asked this question in every interview. Employers want to know why you chose to interview with their specific company. They prefer to hire employers who are passionate about their work and the organization. This question is also meant to determine how much research you have done about Morningstar.
If you expect this question during every interview, you can be prepared to answer it based on your research of Morningstar beforehand. Mention the company's recent achievements, business prospects, or work culture. You may also want to refer to Morningstar's challenges and how you can help them address these based on your skills and experience.
"One of the reasons I chose to interview with Morningstar is that my research indicated you are a leader in the financial industry. The products and services you provide have been developed through the innovation and creativity of your staff. As the financial industry pivots towards new technologies, I believe I can help you maintain this leadership position utilizing my information security skills and experience."
21. Working as a data analyst gives you a unique perspective on the data and the tools used while working with that data. Have you ever recommended a change to any of the data processes or tools used? Did anything result from your recommendation?
Hiring managers would like to see that you are confident in your knowledge and experiences as a data analyst and would take the initiative to recommend a change that would benefit Morningstar. Organizations hire candidates who can not only do the job but also bring new processes and procedures into the organization to help them save money, make money, or save time. Demonstrating your ability to do this during the interview will increase your chances of being offered the job at Morningstar.
When sharing your recommendation, include as many details as possible, particularly why you made it. Do not hesitate to share a recommended change that was not implemented. It shows your ability to take the initiative and continually consider process improvement. You can use the STAR format to frame your answer since this is a behavioral question.
"I believe data analysts in non-technical departments are usually the most familiar with the company's data. However, I have worked in companies where data was accessible to several people who were not in an analyst role. This caused confusion over the interpretation of the data. While data dictionaries can be helpful in these situations, I believe it provides a limited explanation. I recommended that those in non-analytical jobs rely on data analysts for data access. This ensured that data was not misinterpreted, which could negatively affect the strategies being created. I built a case by identifying examples of when data was misinterpreted. The company implemented my recommendation and was even willing to hire more data analysts to ensure that there were enough resources to execute my plan."
22. Can you describe the largest data set you had to work with from a past project? How many rows/entries and columns/variables did the data set include, and what kind of data was included—financial, marketing, operational, etc.?
Many hiring managers look for data analysts who can deal with massive data sets with a large number of variables and rows. This requires skills such as organization and the ability to see both the big picture and the details of a project. Morningstar hiring managers will look for these skills in data analysts.
This question is relatively straightforward, and you should not feel compelled to review details about the background of the project and any processes you might have gone through. The Morningstar interviewer is interested in the size and type of data when asking questions like this. Your answer gives them an idea of the scope of projects you have experience with and are comfortable working on.
"The largest data set I worked with was built for a corporate strategy project that required the combined efforts of various departments. This data set had over a million records and 500-600 variables. Included in this data set were marketing and operational data that was eventually loaded into an analytical tool for exploratory analysis. Is this similar to the data sets I will be working with here at Morningstar?"
23. What tools/software do you have experience using in each phase of a data analysis project—from cleaning/preparing data to data exploration to the final presentation?
Data analysts should have experience with various tools as they work with the data and build their analyses. The Morningstar hiring managers will understand that an analyst may use one tool for multiple phases. They also want to learn if your tools are the same ones their data analytics team uses. This indicates that you will come up to speed quickly and begin contributing to the organization's objectives.
If you have experience with the popular tools used in analyzing data, do not hesitate to share it. Highlight your expertise with a particular tool that Morningstar's data team uses to demonstrate your fit with the organization. You can find this out during your pre-interview research and by speaking with current and former Morningstar data analytics team members. If you have used multiple tools for a particular phase throughout your career, communicate that because it showcases your breadth of experiences.
"Throughout my career as a data analyst, I have been fortunate to have opportunities to strengthen my skills using many tools. In the data cleaning or preparation phase, in most cases, I have used Microsoft Excel, and depending on the complexities of the datasets, Microsoft Access if the need arises. I have also used these tools for the data exploration phase. In this phase, I have used several other tools to extract learnings from the data, including statistical programs such as SAS and SPSS and analytical tools such as Tableau and Cognos Analytics. I have also used Tableau and Power BI to present my findings through data visualizations in a dashboard format. In addition, Excel and PowerPoint are some of the more basic tools I will use to build presentations for Morningstar internal clients."
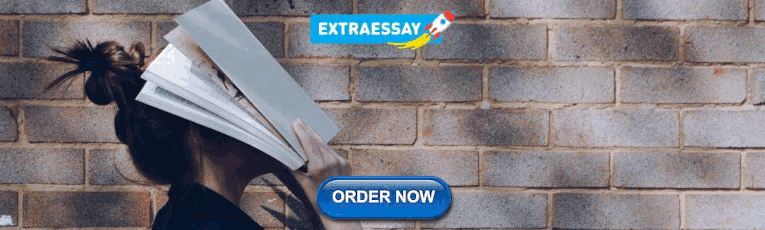
24. Considering all the phases of data analysis, what tools/software work the best for you or do you feel the most comfortable using, and why?
Over a data analyst's career, they have likely had exposure, training, and experiences using different tools, and over time, they begin establishing preferences for specific ones. However, employees' options for data analysis tools are limited to what Morningstar has already chosen. If you have experience working at different companies, you are more likely to have exposure to a variety of analytical tools. This question aims to understand which tools you are comfortable with and not necessarily the number of different tools you have used.
This question may seem similar to one you were asked earlier in the interview. Morningstar interviewers will ask several questions about the same topic during an interview to ensure that your answers are consistent. They also use this process to explore a topic from different perspectives. If you recognize this during the Morningstar interview, try to answer similar questions similarly, providing additional details in the subsequent answers.
"As a data analyst, I find basic tools such as Microsoft Excel and Microsoft Access work the best for me. I feel the most comfortable using these tools because they are the ones I have the most experience using since most--if not all companies--have them readily available. Although most consider these tools basic, I believe with the right training and knowledge, you can accomplish many things using them."
25. It is likely you will work with Morningstar stakeholders who may have little knowledge of data and databases. Describe a time you found yourself working in a situation such as this—what specific challenges did you face, and how did you deal with them?
As with any job, communication is a crucial skill for data analysts. However, communicating with co-workers from multiple departments across Morningstar takes different skills than communicating with co-workers within your department. It may require less technical terms and more time listening to their questions and concerns, which sometimes requires patience.
The experience you share should reflect how you adapted to working with people who may not have spoken your "language." Many times, this requires you to have the ability to look at a situation from different perspectives and beyond just your own. You can demonstrate this skill by answering the Morningstar interview questions using simple, easy-to-understand language and avoiding acronyms, jargon, and technical language. The exception is if the Morningstar interviewer is your hiring manager or a prospective colleague.
"I have run into this situation frequently as a data analyst. In most cases, stakeholders want answers to questions that are not available because of the limitations of the data that is collected or of the database structure. In these situations, I worked with the stakeholder to develop an analysis to answer related questions that may give them an answer as close to what they are looking for as possible. In the process, I tried to offer them a basic understanding of the data available and the database structures--nothing too detailed as I thought this might confuse them. In the long term, we developed a project to investigate whether we can collect the unavailable data. This ensured them that I understood their needs and was willing to work hard at trying to get them what they needed."
26. Describe an analysis project you worked on where the results were surprising to you and others involved in the project.
When launching an analysis, most analysts have a prediction of the outcome based on information from past projects. However, there will likely be times when the results are unexpected. The Morningstar interviewer seeks to understand how you react to unexpected results. They hope to learn that you keep an open mind and use this type of situation as a learning experience or an opportunity to explore a new track for your analysis.
Your answer to this question will give the Morningstar interviewer a glimpse of the type of analytical projects you have worked on and your enthusiasm for them. When describing your project, show some passion for the lessons you drew from it. Also, consider including what action you and the other stakeholders took due to the unexpected results.
"In my experience working with customer profiling projects, analyses usually do not show surprising results, particularly for established brands. However, while conducting one routine analysis, I was able to identify a customer subsegment that had the potential to provide additional value to the company if it was offered the right product and services with a relevant message. It felt as if I struck gold--the opportunity to add value to a subset of an existing customer base through new products and services was invaluable. It was surprising to everyone involved that we could identify a subsegment from this customer base. From there, we began strategizing with product development and brand managers to develop a plan for this new subsegment."
27. Do you have any experience working with statistical models? If so, please describe in as much detail as possible the statistical model you worked on and your role in creating it or using it to answer a business question.
Not all data analysts will have experience working with statistical models. Interviewers, in most cases, will only ask this question if the job description mentioned statistical modeling. Having read your resume, they may be aware of your experience or lack of experience with statistical models and are asking the question to either confirm this or have you describe what you know about this topic and how you will be able to learn to use it when hired by Morningstar.
If you are surprised by this question, be upfront about your experience. If you have not had any direct involvement with statistical modeling work, highlight what you know about it and any training or exposure you have had to it. The Morningstar interviewer will appreciate your honesty and willingness to learn a new skill. Remember, statistical modeling work can include building, using, or maintaining it.
"As a data analyst, I have had experience working with statisticians to help them build their models. Although I do not have direct experience building the model, I have aided them by analyzing data and ensuring they have access to the appropriate data. The model was built to help the sales team identify customers most likely to purchase additional products and services and when they would be most apt to make that decision. This model increased the sales team's efficiency so we didn't waste time with customers who were unlikely to purchase again in the near future. I aided in identifying the appropriate variables used in the model and evaluating the efficacy of the model upon completion."
28. Morningstar, like most large companies, houses its data in multiple data warehouses. In one of your more complex analytical projects, how many data warehouses did you have to query to gather all the required data?
The technical complexity of your work as a data analyst may vary depending on the size of the companies you have previously worked for. Strong technical skills are an important attribute of a data analyst's background. Having experience retrieving data from multiple data warehouses demonstrates your understanding of databases, data structures, and programming languages.
The Morningstar interviewer uses this question to determine how you perform the duties required by this job. This specific question gives them an idea of the scope of work you've done in your previous roles. Keep your answer brief and to the point when responding to operational questions. The interviewer will ask you a follow-up question if they need additional information.
"In the larger companies I have worked at as a data analyst, I have had to work with multiple data warehouses to retrieve the appropriate data. For a particular corporate-wide initiative, I queried against four different data warehouses. Once I retrieved the records and variables I needed, I built one large dataset I worked off of to complete my analysis. Does this sound similar to how Morningstar manages its data?"
29. Querying, cleaning/preparing, analyzing, presenting, and communicating findings are some of the main steps in a data analysis project. Of those, which ONE step do you enjoy most?
Interviewers at Morningstar will ask this question for several reasons. The first is to determine if you are familiar with each step in the data analysis process. The second is to determine which of these you favor. This question may indicate that the data analytics team delegates specific processes to individual members of the team based on their aptitude, skills, and preferences.
It is acceptable to prefer completing one task over another. However, you would be expected to have experience performing all these tasks. Avoid showing aversion to any of the steps in the process when indicating which you enjoy performing most, including explaining why it's your favorite. This will illustrate your strengths to the interviewer.
"If I had to select one step as a favorite, it would be analyzing the data. I enjoy developing a variety of hypotheses and searching for evidence to support or refute them. While following my analytical plan, I have stumbled upon interesting and unexpected information from the data. I believe there is always something to be learned from the data, whether big or small, that will help me in future analytical projects here at Morningstar."
30. Describe a project where the stakeholders' questions couldn't be answered due to data limitations. What advice, if any, did you give the stakeholders?
At some point, data analysts will encounter data obstacles when conducting analytical projects. Hiring managers at Morningstar want to know how you would deal with these situations, particularly when working directly with stakeholders who may not have a strong understanding of the data. Your answer to this question will also reflect your ability to problem-solve.
Since this behavioral question asks you to describe a situation you encountered in a previous job, you can structure your answer using the STAR format. Describe the situation, talk about the task you were asked to complete, provide an overview of your actions, and then talk about the results you attained. Your answer should demonstrate your tact and diplomacy when communicating with other project stakeholders. This is important since the project you worked on did not provide the information they were looking for.
"Years ago, I worked at a company where the executives wanted to initiate a customer segmentation project. However, the data collected in the customer data warehouse was not robust enough to create a meaningful customer segmentation plan. Understanding the importance of this project to the stakeholders, I was able to work with the data warehouse team to outline a handful of data initiatives that would move us closer to a customer segmentation plan. By the time I moved on from this company, the initiatives were progressing well towards its ultimate goal."
31. Think of a project where you worked with a relatively large data set. Describe the process you took to gather and prepare the data for analysis.
Working with large data sets can present challenges, so the Morningstar hiring managers want to know that you have the experience to handle them if they arise. They want to ensure you have encountered challenges in your previous work and learn about the steps you took to overcome them. They are also interested in whether the project you worked on and the impediments you encountered are similar to the ones the Morningstar data analyst team handles.
Walk the interviewer through the project you plan to present step-by-step. Share any challenges you might have faced and how you successfully overcame them. If you have been fortunate enough not to face any challenges, stick to the details of your project and the steps you took while working with the data.
"I have had experience working with large data sets delivered to us from outside vendors. These data sets were often survey responses for marketing research projects with large sample sizes. Upon receiving the data set, I checked the validity of the data by running predetermined frequencies and queries. Doing so would often reveal issues, such as missing data, data type issues, and errors in skip patterns within the survey. I would work with the vendor to correct these issues before beginning further analyses of the data. Once we resolved the data issues, I would load the data into a data analysis tool to begin my analysis. Sometimes I would work with a data engineer to load it into an appropriate tool that could handle the size of the data set."
32. Talk about your knowledge of statistics and how you used this knowledge in your analytical projects.
At the minimum, data analysts should have knowledge and experience using basic statistics, including mean, median, and mode, and be able to conduct significance testing. A more advanced level of statistics may be required, but this would be specified in the job description. Data analysts should not only know how to calculate these basic statistics but should also be able to interpret them about the business. The Morningstar interviewer will ask your question to confirm all of this and determine your level of expertise.
You may be tempted to provide the Morningstar interviewer with a great deal of information about your ability to use statistics. This may result in a long, drawn-out answer. Try to avoid this. When you are asked about your capabilities, keep your answers brief and to the point. The interviewer will use follow-up questions to explore this topic in more detail if they want to.
"I use statistics regularly as a data analyst. For the majority of my work, I calculate basic statistics such as the mean and standard variances. I also conduct significance testing frequently to determine if measurement differences between two populations are statistically significant and worth highlighting for further investigation. In addition, for a few projects, I have worked with correlation coefficients to determine the relationship between two variables in a data set."
33. What scripting language have you used in your past projects as a data analyst? Which are you most confident using?
The interviewer will ask this question to determine if the scripting languages you use are similar to those used by Morningstar's data analytics organization. This will indicate that you can be onboarded quickly and come up to speed with their processes and procedures rapidly. The sooner you contribute to Morningstar's objectives, the more valuable you are as an employee. This will set you apart from other candidates.
Companies like Morningstar work with multiple scripting languages, so knowing more than one is an asset. If you do not have extensive experience programming with the main language used by the company, highlight your eagerness to learn or strengthen your skills with new scripting languages. Many find that, at a general level, their experience with one scripting language helps them learn new ones.
"I have primarily used SQL in my projects as a data analyst and am the most comfortable with it. I have had experience executing some basic Python commands and have made it my goal to receive additional training for it. I have found that my extensive SQL experience has helped me learn Python more easily."
34. How many years of SQL programming do you have? In your most recent position as a data analyst, for what percentage of your analytical projects did you utilize your SQL skills?
SQL is the most well-known scripting language, and many believe it is the easiest to learn. To be marketable, data analysts should learn SQL and gain experience using it. This question is straightforward--the Morningstar interviewer wants to gauge the strength of your SQL programming skills.
Even though this information is probably available in your resume, the Morningstar interviewer asks the question to confirm what they've already read. If you only have a few years of experience, emphasize how your skills have grown with each subsequent project. You can provide examples of projects you worked on using your SQL programming skills to illustrate your answer.
"I have seven years of experience programming with SQL. Over this period, I have used SQL for over 90% of my projects. At times, I have used multiple languages for different phases of projects, but find SQL to be the language I turn to the most."
35. There are many analytical tools data analysts can include in their toolbox. Excel continues to be a common tool for many. Which Excel functions do you have experience using? Describe in detail how you used Excel for one of your analytical projects.
Excel is by far the most utilized tool in the field of data analytics. Interviewers will ask you several questions about this topic to determine your expertise using this tool. They are interested in your expertise in both the basic functionality of Excel and your ability to use more advanced techniques within the tool. They expect you to provide examples for both of these.
If you are an Excel expert, it would be challenging to list all the functions you have experience using. Instead, concentrate on highlighting the more difficult ones, particularly statistical functions. If you have experience utilizing the more challenging functions, Morningstar hiring managers will presume you have experience using the more basic ones. Be sure to highlight your pivot table skills and ability to create graphs in Excel. If you have not attained these skills yet, investing in training to learn them is worthwhile.
"As a data analyst, I have used Excel almost daily. It has become an essential tool in all phases of my analytical projects. I have used Pivot tables to check and clean data sets and analyze them. I have also used statistical functions to calculate standard deviations, correlation coefficients, percentiles, and quartiles in the analysis phase. In addition, I have used the graphing function in Excel to develop visual summaries of the data. For example, I regularly worked on customer satisfaction surveys and received raw data from external vendors. I would take this data, bring it into Excel, and use sort functions and pivot tables to verify the data was clean and loaded correctly. As part of the analysis phase, I always worked with pivot tables to segment the data. In addition, if the analysis called for it, I used the statistical functions I mentioned earlier. Building tables and graphs in Excel allowed me to tie my analyses together visually. I could often complete the tasks in one file, making everything I worked on easily accessible."
36. Do you think creativity is a good skill for a Morningstar data analyst, and if so, how have you used it in your career?
When considering a data analyst's skills, creativity is not top of mind for many. Instead, plenty of people would consider technical and math or statistical skills to be at the top of the list. However, data analysts use their creativity in various ways, including developing analytical plans, finding solutions to data issues, and presenting data visually. Creativity is about 'thinking outside the box.' Be prepared to share in more detail how you used your creativity for a specific project.
You'll note that this is a two-part question. One of the most important skills you can use during an interview is active listening. Listen carefully to the interviewer's question and allow them to finish before you begin to formulate your answer. Interviewers are not only interested in your answers to the questions, but they want to make sure that you answer each part of the question. Doing so demonstrates your attention to detail and ability to communicate effectively.
"As a Morningstar data analyst, there is no question that creativity is an important skill to have. Creativity has gotten me past the data roadblocks in past projects. It has also helped me find new and interesting ways to present analytical results to clients. More specifically, creativity is important when validating data before analyzing it. There have been a few times when I began analyzing data only to find there were some 'abnormal' results. I stepped back and created new and 'non-routine' data checks to identify issues causing the atypical results."
37. What experience, if any, do you have with web analytics? If you have experience, what tools have you used?
An increasing number of data analyst job postings include web analytics experience as a preferred or required skill. At times, companies like Morningstar may choose to separate the roles. Still, in many cases, they prefer data analysts to have a holistic view and, therefore, choose to integrate web analytics into their job description.
When sharing your web analytics experience, give some detail on the measurements you were tracking and the general scope of the project. If you don't have a specific experience with web analytics, convey this to the Morningstar interviewer and then discuss how you would go about learning about this practice. Interviewers can immediately recognize if you're trying to bluff your way through a question and prefer you to answer honestly.
"Using Google Analytics, I have used web analytics as part of a larger marketing campaign evaluation project. The web metrics I tracked included open rate, click-through rate, average time on page, and conversion rate. In addition, I built funnels within Google Analytics to measure where visitors were dropping off before converting. By tracking these web metrics in conjunction with non-web marketing efforts, I was able to recommend the best marketing channels to use to target specific segments."
38. As a data analyst, what skills do you believe are important for working on a Morningstar team with people with different backgrounds and varying roles and responsibilities?
As a data analyst, you will likely have opportunities to work on cross-functional teams. Members of these teams have various backgrounds, differing priorities, and varied skill sets. Of course, there are general skills that would be helpful in any team environment. However, Morningstar hiring managers would be interested in skills that may be somewhat unique to a data analyst facing a team environment. If possible, think of skills that go beyond basic ones like communication.
If the Morningstar interviewer is your hiring manager, they already know about the technical skills needed for this role. Therefore, your answer should address soft skills such as communication, creativity, adaptability, and decision-making. Your answer should demonstrate to them that you understand that the job of a data analyst is more complex than just crunching numbers.
"I believe data analysts have the unique challenge of communicating technical and statistical concepts that many others on the Morningstar team may not understand. Data analysts must be able to explain these concepts in a way that is easily understood by everyone. To successfully do so, data analysts need to have the ability to apply these technical and statistical concepts to the business--specifically to those parts of the business represented on your team. This is possible when data analysts have a more holistic view of the company."
39. How would you rate your writing skills? How do you use this form of communication as a data analyst?
Although data analysts spend much of their time working with numbers, strong writing skills are equally important. They need to interpret the results of their analyses into words to present to stakeholders. Data analysts should be able to tell the 'story' with words and numbers. The Morningstar interviewer will ask this question to understand your communication skills. Your answer will provide them with an example of how you communicate.
If you have not had many opportunities to use or strengthen your writing skills, state the extra measures you are willing to take to change that, whether through training or by proactively seeking opportunities. If you already have strong writing skills, offer to provide the Morningstar interviewer with an example of this using a document you created in one of your previous roles. Make sure to purge any proprietary information before sending this to the interviewer.
"I have a high level of confidence in my writing skills. As a data analyst, I have had many opportunities to strengthen these skills. Whether through email communications with team members or more formal analytical summaries, I find I can get my point across clearly and concisely. I continuously look for ways to exercise and strengthen my writing skills."
40. In your opinion, what soft skills do you believe will be most important in your role as a Morningstar data analyst, and why?
Soft skills are personal attributes that help people work well with others and perform their jobs at a high level. Many refer to these skills as 'non-tangible' and 'non-technical.' As with most jobs, data analysts must have strong soft skills because they do not work in isolation. Therefore their work habits and performance affect others on their team. Morningstar interviewers want to know that you understand the importance of these types of skills.
Knowing the soft skills related to a data analyst job is critical to performing well during the interview at Morningstar. You can find this information by searching for it on the internet. Some common soft skills employers prefer are interpersonal communication, leadership, creativeness, innovation, adaptability, flexibility, and the willingness to learn. Use your past work experiences to support why you find a particular soft skill important, and try to include how you have developed it over time.
"Personally, as a data analyst, I have found leadership skills to be one of the most important soft skills. In my experience, exercising leadership skills does not require a person to be in a managerial role. In a team environment, leadership skills are displayed when you take the initiative to guide and help others. In many cases, data analysts are in a position where they need to educate others on the data and how to interpret it. I have found it crucial to speak up and become an expert in interpreting the company data. Being able to take the initiative has become easier for me over time. I have strengthened this skill by educating myself and finding more opportunities to share my learnings with my team. As a result, I became more confident and built myself up as a leader in this area amongst my team members."
- +91-8208413258
- [email protected]
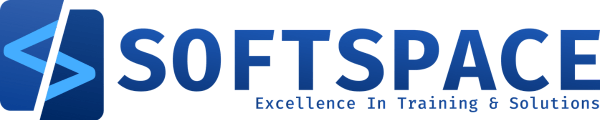
39 Data Analyst Interview Questions & Answers | 2024 Updated
Are you gearing up for a data analyst interview? Whether you’re a seasoned professional or just starting your career in data analytics, being well-prepared is key to success. In today’s data-driven world, companies are constantly on the lookout for skilled analysts who can turn raw data into actionable insights. To help you ace your next interview, we’ve compiled a comprehensive list of essential data analyst interview questions, complete with in-depth answers and expert tips.
From fundamental concepts like statistical analysis and data visualization to more advanced topics such as machine learning and big data technologies, this guide covers the breadth of knowledge expected in a data analyst role. We’ll explore questions that test your technical skills, problem-solving abilities, and even your communication prowess – all crucial aspects of a successful data analyst’s toolkit.
Whether you’re facing a technical screening, a behavioural interview, or a case study challenge, this blog post will equip you with the knowledge and confidence to showcase your skills effectively. Let’s dive into the world of data analyst interviews and set you on the path to landing your dream data analyst job !
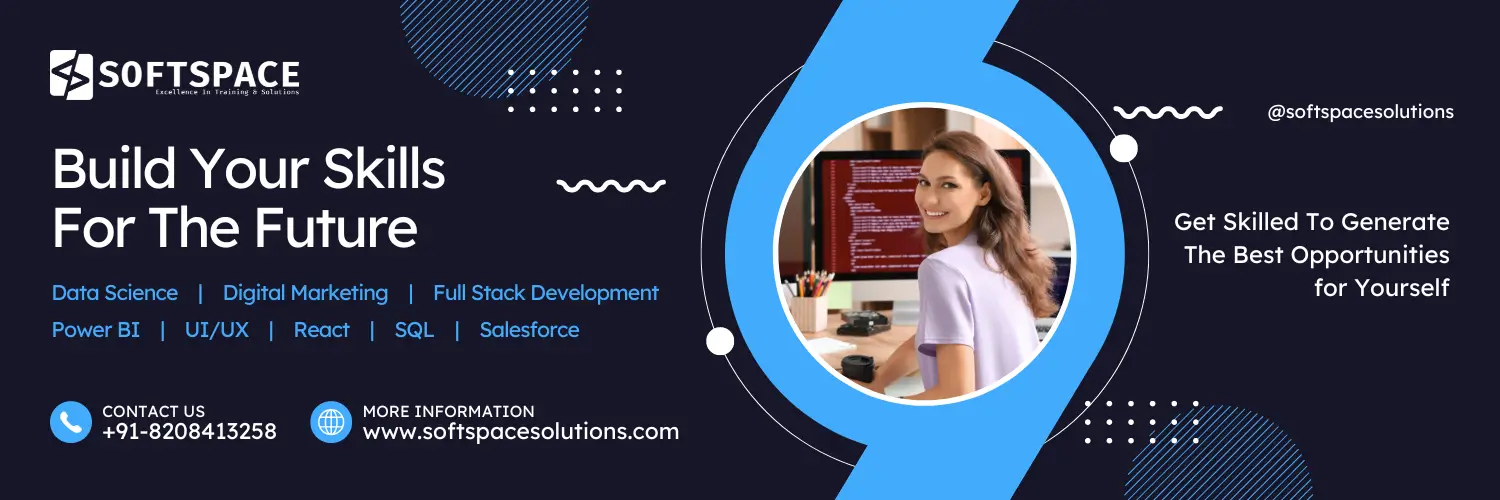
Data Analyst Interview Questions And Answers
Q1: What is the difference between supervised and unsupervised learning?
A: Supervised learning and unsupervised learning are two fundamental categories in machine learning:
Supervised learning uses labelled data to train models. In this approach, the algorithm learns from a dataset where the correct outcomes are already known. The goal is to learn a function that maps input variables to output variables. Examples include regression (predicting continuous values) and classification (predicting categories). Common algorithms are linear regression, logistic regression, decision trees, and support vector machines.
Unsupervised learning, on the other hand, works with unlabeled data. The algorithm tries to find patterns or structures in the data without predefined outcomes. It’s often used for exploratory data analysis, feature learning, and discovering hidden patterns. Common techniques include clustering (like K-means), dimensionality reduction (such as Principal Component Analysis), and association rule learning.
Q2: Explain the concept of data normalization.
A: Data normalization is a preprocessing technique used to standardize the range of independent variables or features of data. The goal is to change the values of numeric columns in the dataset to use a common scale, without distorting differences in the ranges of values or losing information.
Normalization is important because features with large values can disproportionately influence many machine learning algorithms, even if they’re not more important than features with smaller values. There are several methods of normalization:
- Min-Max Scaling: Scales values to a fixed range, usually 0 to 1.
- Z-score Normalization: Scales data to have a mean of 0 and a standard deviation of 1.
- Decimal Scaling: Moves the decimal point of values.
Normalization can significantly improve the performance and training stability of many machine learning algorithms, especially those that use gradient descent optimization.
Q3: What is the purpose of exploratory data analysis (EDA)?
A: Exploratory Data Analysis (EDA) is an approach to analyzing datasets to summarize their main characteristics, often with visual methods. The primary purpose of EDA is to:
- Understand the data: Get a sense of what the data looks like, its structure, and its properties.
- Detect patterns and anomalies: Identify trends, relationships, and outliers in the data.
- Test hypotheses: Generate hypotheses about the underlying structure of the data.
- Check assumptions: Assess assumptions made about the data for further statistical analysis.
- Support selection of appropriate statistical tools and techniques.
EDA typically involves:
- Calculating summary statistics (mean, median, mode, standard deviation)
- Creating visualizations (histograms, box plots, scatter plots)
- Examining distributions of variables
- Identifying correlations between variables
EDA is crucial because it guides the data scientist’s choice of further techniques, helps in feature selection, and provides insights that can be valuable for stakeholders.
Q4: How do you handle missing data in a dataset?
A: Handling missing data is a critical step in data preprocessing. The approach depends on the nature of the data and the reason for missingness. Common strategies include:
- Listwise deletion: Remove entire rows with any missing values.
- Pairwise deletion: Use all available data in each analysis.
- Mean/Median/Mode imputation: Replace missing values with the mean, median, or mode of the column.
- Regression imputation: Predict missing values based on other variables.
- Multiple imputation: Create multiple complete datasets, analyze each, and combine results.
- Some algorithms like Random Forests can work with missing data.
- K-Nearest Neighbors (KNN) imputation
- Expectation-Maximization algorithm
The choice depends on factors like the amount of missing data, the mechanism of missingness (MCAR, MAR, or MNAR), and the specific requirements of the analysis or model.
Q5: What is the difference between correlation and causation?
A: Correlation and causation are often confused, but they represent different types of relationships between variables:
Correlation is a statistical measure that describes the size and direction of a relationship between two or more variables. A correlation indicates that:
- As one variable changes, the other tends to change in a specific way.
- The relationship can be positive (both increase together) or negative (one increases as the other decreases).
- Correlation does not imply that changes in one variable cause changes in the other.
Causation, on the other hand, implies that changes in one variable directly cause changes in another. To establish causation:
- There must be a logical sequence of events (temporal precedence).
- The relationship should persist when controlling for other variables.
- Alternative explanations should be ruled out.
The phrase “correlation does not imply causation” is a reminder that finding a correlation between variables does not necessarily mean that one causes the other. Establishing causation typically requires controlled experiments or more advanced statistical techniques like causal inference methods.
Q6: Explain the concept of overfitting in machine learning.
A: Overfitting is a common problem in machine learning where a model learns the training data too well, including its noise and fluctuations, rather than learning the underlying pattern. This results in a model that performs excellently on the training data but poorly on new, unseen data.
Key aspects of overfitting include:
- High complexity: Overfitted models are often unnecessarily complex, with too many parameters relative to the amount of training data.
- Poor generalization: The model fails to generalize well to new data, showing a significant drop in performance on the test set compared to the training set.
- Noise sensitivity: The model captures random fluctuations in the training data as if they were meaningful patterns.
To prevent overfitting, techniques such as:
- Cross-validation
- Regularization (L1, L2)
- Early stopping
- Ensemble methods
- Increasing training data
- Feature selection
are commonly used. The goal is to find the right balance between model complexity and generalization ability.
Q7: What is the purpose of cross-validation in model evaluation?
A: Cross-validation is a resampling procedure used to evaluate machine learning models on a limited data sample. Its main purposes are:
- Model performance estimation: It provides a more accurate measure of model performance, especially when data is limited.
- Detecting overfitting: By testing the model on multiple subsets of data, it helps identify if the model is overfitting to the training data.
- Model selection: It aids in choosing between different models or hyperparameters by comparing their cross-validated performance.
- Bias-variance tradeoff assessment: It helps in understanding if the model has high bias (underfitting) or high variance (overfitting).
The most common type is k-fold cross-validation:
- The dataset is divided into k subsets or “folds”.
- The model is trained on k-1 folds and tested on the remaining folds.
- This process is repeated k times, with each fold serving as the test set once.
- The results are averaged to give an overall performance estimate.
Cross-validation provides a more robust evaluation of model performance than a single train-test split, especially for smaller datasets.
Q8: Describe the steps in a typical data analysis project.
A: A typical data analysis project usually follows these steps:
- Clearly define the question or problem to be solved.
- Identify key stakeholders and their requirements.
- Gather relevant data from various sources.
- Ensure data quality and relevance.
- Handle missing values, outliers, and inconsistencies.
- Format data appropriately for analysis.
- Perform initial investigations on data.
- Use statistical and visualization techniques to understand data characteristics.
- Create new features or transform existing ones to improve model performance.
- Select appropriate analytical or machine learning techniques.
- Train and validate models.
- Assess model performance using relevant metrics.
- Perform cross-validation and test on holdout data.
- Derive insights from the model outputs.
- Relate findings back to the original problem.
- Create clear, informative visualizations of results.
- Prepare reports or presentations for stakeholders.
- Implement the model in a production environment.
- Set up systems to monitor model performance over time.
This process is often iterative, with feedback loops between steps as new insights emerge or requirements change.
Q9: What is the difference between a bar chart and a histogram?
A: While bar charts and histograms may look similar, they serve different purposes and are used for different types of data:
- Used for categorical data or discrete numeric data.
- Each bar represents a distinct category.
- Bars are usually separated by spaces.
- The height of each bar represents the frequency or value for that category.
- Can be vertical or horizontal.
- Often used to compare different groups or categories.
- Used for continuous numerical data.
- Represents the distribution of a continuous variable.
- Bars are usually adjacent to each other without spaces.
- The area of each bar represents the frequency or probability of data falling within that interval.
- The x-axis represents intervals of the continuous variable.
- Used to show the shape of a data distribution (e.g., normal, skewed, bimodal).
Key differences:
- Data type: Bar charts for categorical, histograms for continuous.
- Purpose: Bar charts compare categories, and histograms show distributions.
- Interpretation: In bar charts, height is key; in histograms, area is important.
Understanding this difference is crucial for choosing the appropriate visualization for your data and interpreting it correctly.
Q10: How do you determine the appropriate sample size for a study?
A: Determining the appropriate sample size is crucial for ensuring the validity and reliability of a study. The process involves several considerations:
- Confidence Level: Typically set at 95% or 99%, it represents how confident you want to be in your results.
- Margin of Error: The amount of error you’re willing to tolerate, often expressed as a percentage.
- Population Variability: An estimate of how much variance exists in the population. If unknown, 50% is often used as it provides the most conservative estimate.
- Population Size: For very large populations, this becomes less important.
- Effect Size: In comparative studies, how large a difference do you expect or want to detect?
- Statistical Power: The probability of detecting an effect if it exists, typically set at 80% or higher.
Calculation methods:
- For simple random sampling, formulas exist that incorporate these factors.
- For more complex designs, power analysis software can be used.
- In qualitative research, concepts like data saturation are often used instead.
Practical considerations:
- Budget and resources available for the study.
- Time constraints.
- Ethical considerations in certain fields (e.g., medical trials).
It’s important to note that larger sample sizes generally lead to more precise estimates and greater statistical power, but there’s often a point of diminishing returns where increasing sample size provides minimal additional benefit.
Q11: What is the difference between variance and standard deviation?
A: Variance and standard deviation are both measures of variability in a dataset, but they differ in interpretation and units:
- Measures the average squared deviation from the mean.
- Calculated by summing the squared differences from the mean and dividing by n-1 (for sample variance) or n (for population variance).
- Expressed in squared units of the original data.
- Formula: σ² = Σ(x – μ)² / n (for population)
Standard Deviation:
- The square root of the variance.
- Measures the average distance between each data point and the mean.
- Expressed in the same units as the original data.
- Formula: σ = √(Σ(x – μ)² / n) (for population)
- Units: Variance is in squared units, and standard deviation is in the original units.
- Interpretation: Standard deviation is often easier to interpret due to being in original units.
- Use cases: Variance is often used in statistical calculations, while standard deviation is commonly used for reporting and interpretation.
Both measures are important in statistics and data analysis for understanding the spread of data and are used in various statistical tests and machine learning algorithms.
Q12: Explain the concept of p-value in hypothesis testing.
A: The p-value is a fundamental concept in statistical hypothesis testing. It represents the probability of obtaining test results at least as extreme as the observed results, assuming that the null hypothesis is true.
Key points about p-values:
- Definition: The p-value is the probability of observing a test statistic as extreme as the one calculated, given that the null hypothesis is true.
- Interpretation: A small p-value (typically ≤ 0.05) suggests strong evidence against the null hypothesis, favouring the alternative hypothesis.
- Null Hypothesis: The assumption of no effect or no difference, which the researcher tries to reject.
- Significance Level (α): The threshold below which the p-value is considered statistically significant, commonly set at 0.05 or 0.01.
- Decision Making: If p ≤ α, reject the null hypothesis; if p > α, fail to reject the null hypothesis.
- P-value does not measure the probability that the hypothesis is true.
- It doesn’t indicate the size or importance of an observed effect.
- Limitations: P-values can be affected by sample size and don’t provide information about effect size or practical significance.
Understanding p-values is crucial for interpreting statistical analyses, but they should be used in conjunction with other statistical measures and practical considerations.
Q13: What is the purpose of A/B testing in data analysis?
A: A/B testing, also known as split testing, is a method used to compare two versions of a variable (web page, app feature, marketing email, etc.) to determine which one performs better. Its purposes include:
- Decision Making: Provides data-driven evidence to support business decisions.
- Performance Optimization: Helps improve user experience, conversion rates, or other key metrics.
- Risk Mitigation: Allows testing of changes on a small scale before full implementation.
- User Behavior Understanding: Offers insights into how users interact with different versions.
- Continuous Improvement: Facilitates ongoing refinement of products or strategies.
- Formulate a hypothesis about a change.
- Create two versions: A (control) and B (variation).
- Randomly divide the audience between versions.
- Collect and analyze data on key performance metrics.
- Determine the statistical significance of results.
- Implement the winning version or iterate further.
Considerations:
- Sample Size: Ensure sufficient participants for statistical validity.
- Duration: Run long enough to account for variations (e.g., day-of-week effects).
- Significance Testing: Use appropriate statistical tests to validate results.
- Segmentation: Consider how different user segments respond.
A/B testing is widely used in digital marketing, product development, and user experience design to make data-informed decisions.
Q14: How do you handle outliers in a dataset?
A: Handling outliers is an important step in data preprocessing. The approach depends on the nature of the outliers and the specific analysis requirements. Here are several strategies:
- Statistical: Z-score, Interquartile Range (IQR)
- Visualization: Box plots, scatter plots
- Machine Learning: Isolation Forest, Local Outlier Factor
- Delete outliers if they’re due to errors or irrelevant to the analysis.
- Caution: Ensure removal doesn’t introduce bias or lose important information.
- Log transformation or other mathematical functions to reduce the impact of extreme values.
- Winsorization: Cap extreme values at a specified percentile (e.g., 5th and 95th).
- Analyze outliers separately to understand their nature and potential insights.
- Use techniques less sensitive to outliers (e.g., median instead of mean, robust regression).
- Replace outliers with more typical values (e.g., mean, median) if appropriate.
- Generate binary features indicating the presence of outliers.
- Understanding the domain and context is crucial.
- The choice of method should depend on the reason for the outliers and the goals of the analysis.
- Document and justify the approach taken for transparency.
Proper handling of outliers can significantly improve model performance and the reliability of statistical analyses.
Q15: What is the difference between parametric and non-parametric statistical tests?
A: Parametric and non-parametric tests are two broad categories of statistical tests, each with distinct characteristics and assumptions:
Parametric Tests:
- Data follows a known probability distribution (often normal distribution).
- Parameters of the distribution are known or can be estimated.
- Examples: t-test, ANOVA, Pearson correlation
- More powerful when assumptions are met.
- Provide more information about the data.
- Less robust when assumptions are violated.
- May not be suitable for small sample sizes.
Non-Parametric Tests:
- Do not assume a specific distribution of the data.
- Often based on ranks or orders of data rather than actual values.
- Examples: Mann-Whitney U test, Kruskal-Wallis test, Spearman correlation
- More robust against outliers and extreme values.
- Suitable for ordinal data and small sample sizes.
- Applicable when parametric assumptions are not met.
- Generally less powerful than parametric tests when parametric assumptions are met.
- May not provide as much information about the data.
Key Differences:
- Distribution Assumptions: Parametric tests assume a specific distribution; non-parametric tests do not.
- Data Type: Parametric tests typically require interval or ratio data; non-parametric tests can be used with ordinal data.
- Central Tendency: Parametric tests use means; non-parametric tests often use medians.
- Power: Parametric tests are generally more powerful when their assumptions are met.
Choosing between parametric and non-parametric tests depends on the data characteristics, sample size, and research questions. It’s important to check the assumptions and choose the most appropriate test for the given situation.
Q16: What is the purpose of dimensionality reduction in data analysis?
A: Dimensionality reduction is a technique used to reduce the number of features in a dataset while retaining as much important information as possible. Its purposes include:
- Improved Model Performance: Reducing irrelevant or redundant features can improve model accuracy and reduce overfitting.
- Computational Efficiency: Fewer dimensions mean faster training times and less computational resources required.
- Visualization: Reducing data to 2 or 3 dimensions allows for easier visualization and interpretation.
- Noise Reduction: Eliminating less important features can help reduce noise in the data.
- Addressing the Curse of Dimensionality: As dimensions increase, the amount of data needed to generalize accurately grows exponentially.
- Feature Extraction: Creating new, more informative features from combinations of original features.
Common techniques include:
- Principal Component Analysis (PCA)
- t-SNE (t-Distributed Stochastic Neighbor Embedding)
- Autoencoders
- Linear Discriminant Analysis (LDA)
- Factor Analysis
When applying dimensionality reduction, it’s important to balance information retention with dimension reduction and to validate that the reduced dataset still captures the essential patterns in the data.
Q17: Explain the concept of multicollinearity in regression analysis.
A: Multicollinearity occurs in regression analysis when two or more independent variables are highly correlated with each other. This situation can lead to several problems:
- Unstable Coefficients: Small changes in the model or data can lead to large changes in the coefficients of the correlated variables.
- Difficult Interpretation: It becomes challenging to determine the individual effect of each variable on the dependent variable.
- Increased Standard Errors: The standard errors of the coefficients increase, potentially making some variables appear statistically insignificant when they should be significant.
- Reduced Model Reliability: The overall model may still have a good fit, but individual predictors may not be reliable.
Detection methods:
- Correlation Matrix: Look for high correlations between independent variables.
- Variance Inflation Factor (VIF): VIF > 5-10 typically indicates problematic multicollinearity.
- Condition Number: A large condition number of the correlation matrix indicates multicollinearity.
Addressing multicollinearity:
- Remove one of the correlated variables.
- Combine correlated variables into a single feature.
- Use regularization techniques like Ridge or Lasso regression.
- Collect more data if possible.
- Use dimensionality reduction techniques.
Understanding and addressing multicollinearity is crucial for building reliable and interpretable regression models.
Q18: What is the difference between classification and regression in machine learning?
A: Classification and regression are two fundamental types of supervised learning tasks in machine learning:
Classification:
- Purpose: Predicts a discrete class label or category.
- Output: Categorical variables (e.g., yes/no, red/blue/green).
- Examples: Spam detection, image recognition, medical diagnosis.
- Algorithms: Logistic Regression, Decision Trees, Random Forests, Support Vector Machines, Neural Networks.
- Evaluation Metrics: Accuracy, Precision, Recall, F1-score, ROC-AUC.
Regression:
- Purpose: Predicts a continuous numerical value.
- Output: Continuous variables (e.g., price, temperature, age).
- Examples: House price prediction, sales forecasting, temperature estimation.
- Algorithms: Linear Regression, Polynomial Regression, Decision Trees, Random Forests, Neural Networks.
- Evaluation Metrics: Mean Squared Error (MSE), Root Mean Squared Error (RMSE), Mean Absolute Error (MAE), R-squared.
- Nature of Output: Discrete categories vs. continuous values.
- Problem Type: Grouping vs. estimating.
- Evaluation Methods: Classification metrics vs. regression metrics.
- Decision Boundaries: Classification often involves finding decision boundaries between classes, while regression fits a continuous function.
Some algorithms, like Decision Trees and Neural Networks, can be used for both classification and regression tasks with appropriate modifications.
Q19: How do you handle imbalanced datasets in machine learning?
A: Imbalanced datasets, where one class significantly outnumbers the other(s), can lead to biased models. Here are strategies to handle them:
- Oversampling: Increase instances of the minority class (e.g., SMOTE – Synthetic Minority Over-sampling Technique).
- Undersampling: Reduce instances of the majority class (e.g., Random Undersampling).
- Combination: Use both over- and under-sampling (e.g., SMOTETomek).
- Class Weighting: Assign higher weights to the minority class in the loss function.
- Bagging-based: Random Forests with balanced bootstrap.
- Boosting-based: AdaBoost, Gradient Boosting with class weighting.
- Anomaly Detection: Treat the minority class as anomalies, especially useful for extreme imbalances.
- Data Augmentation: Generate synthetic examples of the minority class.
- Use algorithms less sensitive to imbalance (e.g., decision trees).
- Adjust the decision threshold in probabilistic classifiers.
- Collect More Data: If possible, gather more samples of the minority class.
- Change the Performance Metric: Use metrics like F1-score, ROC-AUC, or Cohen’s Kappa instead of accuracy.
- Cost-Sensitive Learning: Adjust the algorithm to account for the costs of misclassification.
The choice of method depends on the specific problem, dataset characteristics, and the goals of the analysis. It’s often beneficial to try multiple approaches and compare their performance.
Q20: What is the purpose of feature scaling in machine learning?
A: Feature scaling is a preprocessing technique used to standardize the range of independent variables or features of data. Its purposes include:
- Improved Algorithm Performance: Many algorithms perform better when features are on a similar scale.
- Faster Convergence: Gradient descent converges faster for scaled features.
- Preventing Dominance: Ensures that larger-scale features don’t dominate smaller-scale features.
- Improved Interpretability: Makes coefficients in linear models more comparable.
- Necessary for Certain Algorithms: Some algorithms (e.g., Neural Networks, K-Nearest Neighbors) require scaled features to work properly.
Common scaling methods:
- Scales features to have mean=0 and variance=1.
- Formula: z = (x – μ) / σ
- Scales features to a fixed range, usually [0, 1].
- Formula: x_scaled = (x – min(x)) / (max(x) – min(x))
- Uses statistics that are robust to outliers.
- Often based on median and interquartile range.
- Useful for highly skewed features.
- Apply scaling after splitting data into train and test sets to prevent data leakage.
- Be cautious with tree-based models, which are generally invariant to monotonic transformations of features.
- Remember to apply the same scaling to new data during prediction.
Feature scaling is a crucial step in data preprocessing that can significantly impact the performance and interpretability of many machine learning models.
Q21: What is the difference between a data warehouse and a data lake?
A: Data warehouses and data lakes are both storage repositories for big data, but they differ in several key aspects:
Data Warehouse:
- Structure: Highly structured, schema-on-write approach.
- Data Type: Processed data, typically from transactional systems.
- Purpose: Designed for business intelligence, reporting, and structured queries.
- Users: Business analysts, data analysts.
- Data Quality: High, as data is cleaned and transformed before loading.
- Speed: Fast query performance due to optimized structure.
- Cost: Generally more expensive due to data preparation and storage requirements.
- Structure: Raw or minimally processed data, schema-on-read approach.
- Data Type: Can store structured, semi-structured, and unstructured data.
- Purpose: Flexible storage for various types of analytics, including machine learning and data discovery.
- Users: Data scientists, data engineers, and analysts with advanced skills.
- Data Quality: Varies, as it contains raw data.
- Speed: Can be slower for queries due to lack of optimization.
- Cost: Generally less expensive for storage, but may require more processing time.
- Data warehouses are optimized for fast queries on structured data, while data lakes provide flexibility for storing and analyzing diverse data types.
- Data warehouses require significant upfront design, while data lakes allow for more agile data storage and analysis.
The choice between them depends on the organization’s needs, data types, and analytical requirements.
Q22: Explain the concept of confidence intervals in statistics.
A: A confidence interval is a range of values that is likely to contain the true population parameter with a certain level of confidence. Key points include:
- Definition: An estimated range of values that is likely to include an unknown population parameter.
- Point estimate: The single value estimate of the parameter.
- Margin of error: The range around the point estimate.
- Confidence level: Usually 95% or 99%, indicating the probability that the interval contains the true parameter.
- Interpretation: If we were to repeat the sampling process many times, about 95% (for a 95% confidence interval) of the intervals would contain the true population parameter.
- Sample size: Larger samples lead to narrower intervals.
- Variability in the data: More variability leads to wider intervals.
- Confidence level: Higher confidence levels result in wider intervals.
- Calculation: Typically involves the point estimate, standard error, and a critical value from a t-distribution or normal distribution.
- Estimating population parameters.
- Assessing the precision of estimates.
- Hypothesis testing (by checking if a hypothesized value falls within the interval).
Understanding confidence intervals is crucial for interpreting statistical results and making inferences about populations based on sample data.
Q23: What is the purpose of regularization in machine learning models?
A: Regularization is a technique used to prevent overfitting in machine learning models. Its purposes include:
- Prevent Overfitting: Discourages learning a more complex or flexible model, to reduce the risk of fitting noise in the training data.
- Improve Generalization: Helps the model perform well on unseen data, not just the training set.
- Feature Selection: Some regularization techniques can lead to sparse models, effectively performing feature selection.
- Stability: Makes the model more stable by reducing its sensitivity to individual data points.
Common regularization techniques:
- Adds the absolute value of coefficients to the loss function.
- Can lead to sparse models by driving some coefficients to exactly zero.
- Adds the squared magnitude of coefficients to the loss function.
- Shrinks all coefficients but doesn’t typically make them exactly zero.
- Combines L1 and L2 regularization.
- Randomly “drops out” a proportion of neurons during training.
- Stops training when performance on a validation set starts to degrade.
- Artificially increases the training set size, which can have a regularizing effect.
The choice of regularization technique depends on the specific problem, model type, and desired outcomes. Proper use of regularization can significantly improve model performance and reliability.
Q24: How do you evaluate the performance of a clustering algorithm?
A: Evaluating clustering algorithms can be challenging since they are unsupervised learning methods. However, several approaches can be used:
- Silhouette Score: Measures how similar an object is to its own cluster compared to other clusters.
- Calinski-Harabasz Index: Ratio of between-cluster dispersion to within-cluster dispersion.
- Davies-Bouldin Index: Ratio of within-cluster distances to between-cluster distances.
- Adjusted Rand Index: Measures the similarity between two clusterings, adjusted for chance.
- Normalized Mutual Information: Measures the mutual dependence between the clustering and the true labels.
- Purity: Proportion of the total number of objects that were correctly clustered.
- Plot the explained variation as a function of the number of clusters and look for an elbow point.
- Plot silhouette scores for different numbers of clusters to find the optimal number.
- Use dimensionality reduction techniques (e.g., PCA, t-SNE) to visualize clusters in 2D or 3D.
- Assess if the clusters make sense in the context of the problem domain.
- Evaluate how consistent the clustering results are across different subsamples of the data.
- Assess if the clustering results provide actionable insights or improve business outcomes.
It’s often beneficial to use a combination of these methods to get a comprehensive evaluation of clustering performance. The choice of evaluation method should align with the goals of the clustering task and the nature of the data.
Q25: What is the difference between bagging and boosting in ensemble learning?
A: Bagging (Bootstrap Aggregating) and Boosting are two popular ensemble learning techniques, but they differ in their approach:
- Approach: Creates multiple subsets of the original dataset with replacement, trains a model on each subset, and combines predictions through voting or averaging.
- Goal: Reduce variance and avoid overfitting.
- Training: Models are trained independently and in parallel.
- Weighting: Each model typically has equal weight in the final prediction.
- Example Algorithm: Random Forests
- Approach: Trains models sequentially, with each new model focusing on the errors of the previous models.
- Goal: Reduce bias and increase predictive power.
- Training: Models are trained sequentially, with each model learning from the mistakes of the previous ones.
- Weighting: Models are weighted based on their performance.
- Example Algorithms: AdaBoost, Gradient Boosting Machines (e.g., XGBoost)
- Error Handling: Bagging focuses on reducing variance while boosting aims to reduce bias.
- Model Independence: In bagging, models are independent; in boosting, they are dependent on previous models.
- Overfitting: Bagging is less prone to overfitting compared to boosting.
- Computational Efficiency: Bagging can be parallelized, while boosting is inherently sequential.
Both techniques have their strengths and are used in different scenarios depending on the nature of the problem and the characteristics of the data.
Q26: What is the purpose of cross-entropy loss in machine learning?
A: Cross-entropy loss, also known as log loss, is a loss function commonly used in classification problems, especially in neural networks. Its purposes include:
- Measure Model Performance: It quantifies the difference between predicted probability distributions and actual distributions.
- Optimize Classification Models: It provides a clear optimization objective for training classifiers.
- Handle Probabilistic Outputs: It’s particularly useful for models that output probabilities (e.g., softmax in neural networks).
- Penalize Confident Mistakes: It heavily penalizes predictions that are both wrong and confident.
- Multi-class Classification: It naturally extends to multi-class problems.
Key points:
- For binary classification, it’s calculated as: -[y log(p) + (1-y) log(1-p)], where y is the true label and p is the predicted probability.
- For multi-class problems, it uses the categorical cross-entropy formula.
- It’s often used with logistic regression and neural networks.
- Minimizing cross-entropy is equivalent to maximizing the likelihood of the observed data under the model.
Understanding cross-entropy loss is crucial for effectively training and evaluating many types of classification models.
Q27: Explain the concept of time series decomposition.
A: Time series decomposition is a technique used to break down a time series into its constituent components. The main components are:
- Trend: The long-term progression of the series (increasing, decreasing, or stable).
- Seasonality: Regular patterns that repeat at fixed intervals (e.g., daily, weekly, monthly).
- Cyclical: Fluctuations that don’t have a fixed frequency.
- Residual (or Irregular): The random variation left after other components are accounted for.
Common decomposition models:
- Additive: Original = Trend + Seasonality + Residual
- Multiplicative: Original = Trend * Seasonality * Residual
Methods for decomposition:
- Classical Decomposition: Uses moving averages to estimate trend and seasonality.
- X-11 Method: More sophisticated approach used by statistical agencies.
- STL (Seasonal and Trend decomposition using Loess): Versatile method that can handle any type of seasonality.
Purposes of decomposition:
- Understanding underlying patterns in the data.
- Forecasting future values by projecting components separately.
- Removing seasonality for clearer trend analysis.
- Anomaly detection by examining residuals.
Time series decomposition is a fundamental technique in time series analysis, providing insights into the underlying structure of temporal data.
Q28: What is the difference between correlation and covariance?
A: Correlation and covariance are both measures of the relationship between two variables, but they differ in several important ways:
Covariance:
- Measures the direction of the linear relationship between two variables.
- Not standardized, so its value depends on the scale of the variables.
- Formula: Cov(X,Y) = E[(X – μX)(Y – μY)]
- Range: Can be any real number.
Correlation:
- Measures both the strength and direction of the linear relationship.
- Standardized measure, always between -1 and 1.
- Formula: Corr(X,Y) = Cov(X,Y) / (σX * σY)
- Range: Always between -1 (perfect negative correlation) and 1 (perfect positive correlation).
- Scale: Covariance is affected by the scale of variables; correlation is scale-invariant.
- Interpretation: Correlation is easier to interpret due to its standardized range.
- Units: Covariance is in units of X times units of Y; correlation is unitless.
- Use cases: Correlation is more commonly used for general relationship analysis; covariance is often used in more technical applications like portfolio theory.
Understanding both measures is important for data analysis, as they provide complementary information about relationships between variables.
Q29: How do you handle missing time series data?
A: Handling missing time series data is crucial for accurate analysis and forecasting. Here are several approaches:
- Listwise deletion: Remove entire time periods with missing data.
- Pairwise deletion: Use available data for each calculation.
- Mean/Median Imputation: Replace missing values with the mean or median of the series.
- Last Observation Carried Forward (LOCF): Use the last known value to fill subsequent missing values.
- Next Observation Carried Backward (NOCB): Use the next known value to fill preceding missing values.
- Linear Interpolation: Estimate missing values using a straight line between known points.
- Spline Interpolation: Use a curved line for smoother estimates.
- Seasonal Adjustment: Use seasonal patterns to estimate missing values.
- Moving Average: Use a window of surrounding values to estimate the missing point.
- ARIMA Models: Use autoregressive integrated moving average models to forecast missing values.
- Kalman Filtering: Estimate missing values based on past and future observations.
- Multiple Imputation: Create multiple plausible imputed datasets and combine results.
- K-Nearest Neighbors: Estimate based on similar time periods.
- Random Forests: Can handle missing values internally.
- Use domain knowledge to inform appropriate imputation strategies.
- The choice of method depends on the pattern of missingness, the nature of the time series, and the analysis goals.
- It’s important to assess the impact of the chosen method on subsequent analyses.
- For critical applications, it’s often beneficial to compare results using different imputation methods.
Q30: What is the purpose of feature importance in machine learning models?
A: Feature importance is a technique used to assign scores to input features based on how useful they are at predicting a target variable. Its purposes include:
- Model Interpretation: This helps understand which features are driving the predictions, making the model more interpretable.
- Feature Selection: Identifies the most relevant features, allowing for dimensionality reduction by removing less important ones.
- Model Improvement: Guides feature engineering efforts by highlighting areas where new or transformed features might be beneficial.
- Domain Insights: Provides valuable information about the underlying processes generating the data.
- Debugging: Helps identify potential issues in the model or data by revealing unexpected importance patterns.
- Reducing Overfitting: Focusing on the most important features, can lead to simpler, more generalizable models.
- Efficient Resource Allocation: In domains where gathering data is expensive, it helps focus efforts on collecting the most impactful features.
Methods for calculating feature importance:
- Tree-based methods: Feature importance in Random Forests or Gradient Boosting Machines.
- Permutation Importance: Measures the decrease in model performance when a feature is randomly shuffled.
- Coefficient Magnitude: In linear models, the absolute value of coefficients can indicate importance.
- SHAP (SHapley Additive exPlanations) Values: Game theoretic approach to feature importance.
- Feature importance can be affected by multicollinearity among features.
- Different methods may produce different rankings, so it’s often useful to compare multiple approaches.
- Importance doesn’t imply causality; it only indicates predictive power in the context of the model.
Understanding and utilizing feature importance is crucial for developing effective and interpretable machine learning models.
Q31: What is the difference between precision and recall in classification metrics?
A: Precision and recall are two important metrics used to evaluate classification models, especially for imbalanced datasets:
- Definition: The proportion of true positive predictions among all positive predictions.
- Formula: TP / (TP + FP)
- Focuses on: Minimizing false positives.
- Use case: When the cost of false positives is high (e.g., spam detection).
Recall (also known as Sensitivity):
- Definition: The proportion of true positive predictions among all actual positive instances.
- Formula: TP / (TP + FN)
- Focuses on: Minimizing false negatives.
- Use case: When the cost of false negatives is high (e.g., disease diagnosis).
- Focus: Precision focuses on the accuracy of positive predictions, while recall focuses on finding all positive instances.
- Trade-off: Often, improving one metric leads to a decrease in the other.
- Use cases: The importance of each metric depends on the specific problem and the costs associated with different types of errors.
Understanding both metrics is crucial for a comprehensive evaluation of classification models, especially in scenarios with class imbalance.
Q32: Explain the concept of dimensionality curse in machine learning.
A: The curse of dimensionality refers to various phenomena that arise when analyzing data in high-dimensional spaces that do not occur in low-dimensional settings. Key aspects include:
- Data Sparsity: As dimensions increase, the amount of data needed to generalize accurately grows exponentially.
- Distance Concentration: In high dimensions, the distance between any two points becomes almost constant, making nearest neighbor-based methods less effective.
- Model Complexity: More dimensions often require more complex models, increasing the risk of overfitting.
- Computational Cost: Many algorithms scale poorly with increasing dimensions.
- Visualization Difficulty: It becomes challenging to visualize and understand data in high dimensions.
- Feature Interaction: The number of potential feature interactions grows exponentially with dimensions.
Implications:
- Increased risk of overfitting
- Reduced effectiveness of distance-based methods
- Need for more training data
- Importance of feature selection and dimensionality reduction techniques
Mitigating strategies:
- Dimensionality reduction (e.g., PCA, t-SNE)
- Regularization techniques
- Using models that handle high-dimensional data well (e.g., decision trees, random forests)
Understanding the curse of dimensionality is crucial for effectively handling high-dimensional datasets and choosing appropriate modelling strategies.
Q33: What is the purpose of the confusion matrix in classification problems?
A: A confusion matrix is a table used to describe the performance of a classification model. Its purposes include:
- Comprehensive Performance Evaluation: It provides a detailed breakdown of correct and incorrect classifications for each class.
- Visualization of Model Performance: It offers a clear, tabular view of model predictions versus actual values.
- Calculation of Various Metrics: It serves as the basis for computing important evaluation metrics like accuracy, precision, recall, and F1-score.
- Identification of Error Types: It distinguishes between different types of errors (false positives and false negatives).
- Class-Specific Performance: It allows assessment of how well the model performs for each individual class.
- Imbalanced Dataset Handling: It’s particularly useful for evaluating performance on imbalanced datasets where accuracy alone can be misleading.
Predicted Pos Neg Actual Pos TP FN Neg FP TN
where: TP = True Positives TN = True Negatives FP = False Positives FN = False Negatives
From this, various metrics can be derived:
- Accuracy = (TP + TN) / (TP + TN + FP + FN)
- Precision = TP / (TP + FP)
- Recall = TP / (TP + FN)
- F1-Score = 2 * (Precision * Recall) / (Precision + Recall)
Q34: How does the random forest algorithm work?
A: Random Forest is an ensemble learning method that operates by constructing multiple decision trees and merging their predictions. Key aspects of its functioning include:
- Creates multiple subsets of the original dataset through random sampling with replacement.
- Each subset is used to train a different decision tree.
- At each split in the tree, only a random subset of features is considered.
- This introduces diversity among the trees.
- Each tree is grown to its maximum depth without pruning.
- Trees are typically created using algorithms like CART (Classification and Regression Trees).
- For classification, the final prediction is the mode of the predictions from individual trees.
- For regression, it’s the average of individual tree predictions.
- Uses the samples not included in each bootstrap sample to estimate the model’s performance.
Advantages:
- Reduces overfitting compared to individual decision trees.
- Handles high-dimensional data well.
- Can capture complex interactions between features.
- Provides feature importance measures.
- Less interpretable than single decision trees.
- Can be computationally intensive for large datasets.
- Requires tuning of hyperparameters like the number of trees and features considered at each split.
Random Forest is widely used due to its versatility, good performance, and ability to handle various types of data without extensive preprocessing.
Q35: What is the purpose of regularization in neural networks?
A: Regularization in neural networks serves several important purposes:
- Prevent Overfitting: It helps the model generalize better to unseen data by preventing it from fitting the training data too closely.
- Reduce Model Complexity: Encourages simpler models that are less likely to overfit.
- Improve Generalization: Helps the model perform well on new, unseen data.
- Feature Selection: Some regularization techniques can effectively perform feature selection by reducing the impact of less important features.
- Stability: Makes the model more robust to small changes in the input data.
Common regularization techniques in neural networks include:
- L1 and L2 Regularization: Add penalties to the loss function based on the magnitude of weights.
- Dropout: Randomly “drops out” a proportion of neurons during training, forcing the network to learn redundant representations.
- Early Stopping: Stops training when performance on a validation set starts to degrade.
- Data Augmentation: Artificially increases the training set size by applying transformations to existing data.
- Batch Normalization: Normalizes the inputs of each layer, which can have a regularizing effect.
- Weight Decay: Gradually reduces the weight values over time during training.
The choice of regularization technique depends on the specific problem, network architecture, and available data. Often, a combination of techniques is used for optimal results.
Q36: Explain the concept of gradient descent in machine learning.
A: Gradient descent is an optimization algorithm used to minimize a function by iteratively moving in the direction of steepest descent as defined by the negative of the gradient. In machine learning, it’s commonly used to minimize the loss function. Key aspects include:
- Objective: Find the minimum of a function (typically the loss function in ML).
- Start with initial parameter values.
- Calculate the gradient (direction of steepest increase) of the loss function.
- Update parameters in the opposite direction of the gradient.
- Repeat until convergence or a specified number of iterations.
- Learning Rate: Determines the size of steps taken in each iteration. Crucial for convergence and optimization speed.
- Batch Gradient Descent: Uses entire dataset for each update.
- Stochastic Gradient Descent (SGD): Uses a single random sample for each update.
- Mini-batch Gradient Descent: Uses a small random subset of data for each update.
- Momentum: Adds a fraction of the previous update to the current one.
- AdaGrad: Adapts learning rates for each parameter.
- RMSprop: Adapts learning rates using a moving average of squared gradients.
- Adam: Combines ideas from RMSprop and momentum.
- Local minima: Can get stuck in suboptimal solutions.
- Saddle points: Areas where the gradient is zero but not a minimum.
- Choosing appropriate learning rates.
Understanding gradient descent is crucial for training many machine learning models, especially neural networks.
Q37: What is the difference between parametric and non-parametric models?
A: Parametric and non-parametric models differ in their assumptions about the underlying data distribution:
Parametric Models:
- Assumption: Assume a fixed functional form for the relationship between inputs and outputs.
- Parameters: Have a fixed number of parameters, regardless of the amount of training data.
- Examples: Linear Regression, Logistic Regression, Neural Networks.
- Simple and easy to interpret.
- Require less data to train.
- Faster to train and make predictions.
- May not capture complex relationships if the assumed form is incorrect.
- Can underfit if the model is too simple for the data.
Non-Parametric Models:
- Assumption: Do not assume a specific functional form for the relationship.
- Parameters: Number of parameters grows with the amount of training data.
- Examples: K-Nearest Neighbors, Decision Trees, Support Vector Machines with non-linear kernels.
- Flexible, can capture complex relationships in data.
- Make fewer assumptions about the underlying data distribution.
- Can perform well with high-dimensional data.
- Require more data to train effectively.
- Can be computationally intensive.
- Risk of overfitting if not properly regularized.
- Flexibility: Non-parametric models are generally more flexible but require more data.
- Interpretability: Parametric models are often easier to interpret.
- Scalability: Parametric models typically scale better to large datasets.
- Assumptions: Parametric models make stronger assumptions about the data distribution.
The choice between parametric and non-parametric models depends on the amount of available data, the complexity of the underlying relationship, and the specific requirements of the problem at hand.
Q38: How do you handle multicollinearity in regression analysis?
A: Multicollinearity occurs when independent variables in a regression model are highly correlated with each other. Here are methods to handle it:
- Identify highly correlated variables using correlation matrices.
- Consider removing one of the correlated variables.
- Calculate VIF for each predictor.
- Remove variables with high VIF (typically > 5 or 10).
- Use techniques like Lasso or Ridge regression that can automatically select or de-emphasize redundant features.
- Transform correlated variables into a set of uncorrelated principal components.
- Use these components as predictors instead of original variables.
- Create a new variable that captures the information from correlated predictors (e.g., an average or sum).
- Use L1 (Lasso) or L2 (Ridge) regularization to reduce the impact of correlated variables.
- A technique that finds a linear regression model by projecting predicted variables and observable variables into a new space.
- Use expert knowledge to select the most relevant variables among correlated ones.
- Subtracting the mean from predictor variables can sometimes help reduce multicollinearity.
- Sometimes, multicollinearity is a result of a small sample size.
- The choice of method depends on the severity of multicollinearity and the specific requirements of the analysis.
- It’s important to balance addressing multicollinearity with maintaining the interpretability and predictive power of the model.
- Some level of multicollinearity is often present and may not always be problematic if it’s not severe.
Properly handling multicollinearity is crucial for building reliable and interpretable regression models.
Q39: What is the purpose of cross-validation in model evaluation?
A: Cross-validation is a resampling procedure used to evaluate machine learning models on a limited data sample. Its purposes include:
- Provides a more robust estimate of model performance than a single train-test split.
- Helps understand how well the model might perform on unseen data.
- By testing on multiple subsets of data, it helps identify if the model is overfitting to the training data.
- Allows comparison of different models or hyperparameters to choose the best performing one.
- Helps in finding the optimal hyperparameters for a given model.
- Provides insight into how well the model generalizes to independent datasets.
- Makes efficient use of limited data by using all observations for both training and validation.
- Helps in understanding if the model has high bias (underfitting) or high variance (overfitting).
Common Cross-Validation Techniques:
- K-Fold Cross-Validation: Data is divided into k subsets, with each subset serving as the test set once.
- Leave-One-Out Cross-Validation: Special case of k-fold where k equals the number of observations.
- Stratified K-Fold: Ensures that the proportion of samples for each class is roughly the same in each fold.
- Time Series Cross-Validation: Adapts cross-validation for time-dependent data.
- The choice of cross-validation method depends on the size of the dataset, the problem type, and computational resources.
- It’s important to ensure that the cross-validation procedure mimics the real-world application of the model.
- Cross-validation should be used in conjunction with a final holdout test set for unbiased evaluation.
Cross-validation is a fundamental technique in machine learning for robust model evaluation and selection, helping to build more reliable and generalizable models.
As we conclude our exploration of data analyst interview questions, it’s clear that the field of data analytics is both challenging and rewarding. The questions we’ve covered span a wide range of topics, reflecting the diverse skill set required in this dynamic profession. From statistical concepts and programming skills to business acumen and communication abilities, successful data analysts must be well-rounded professionals.
Remember, while technical knowledge is crucial, employers also value candidates who can think critically, solve complex problems, and effectively communicate their findings. As you prepare for your interviews, focus not just on memorizing answers, but on understanding the underlying concepts and their real-world applications.
Keep in mind that the field of data analytics is constantly evolving. Stay current with the latest trends, tools, and techniques in the industry. Continuous learning and adaptability are key traits that will set you apart in your career.
Lastly, approach your interviews with confidence. Your preparation and passion for data will shine through as you engage with interviewers. Each interview is not just an evaluation but an opportunity to showcase your unique perspective and value as a data analyst.
We hope this guide serves as a valuable resource in your interview preparation. Good luck in your job search, and may your future be filled with exciting data-driven discoveries and impactful insights!
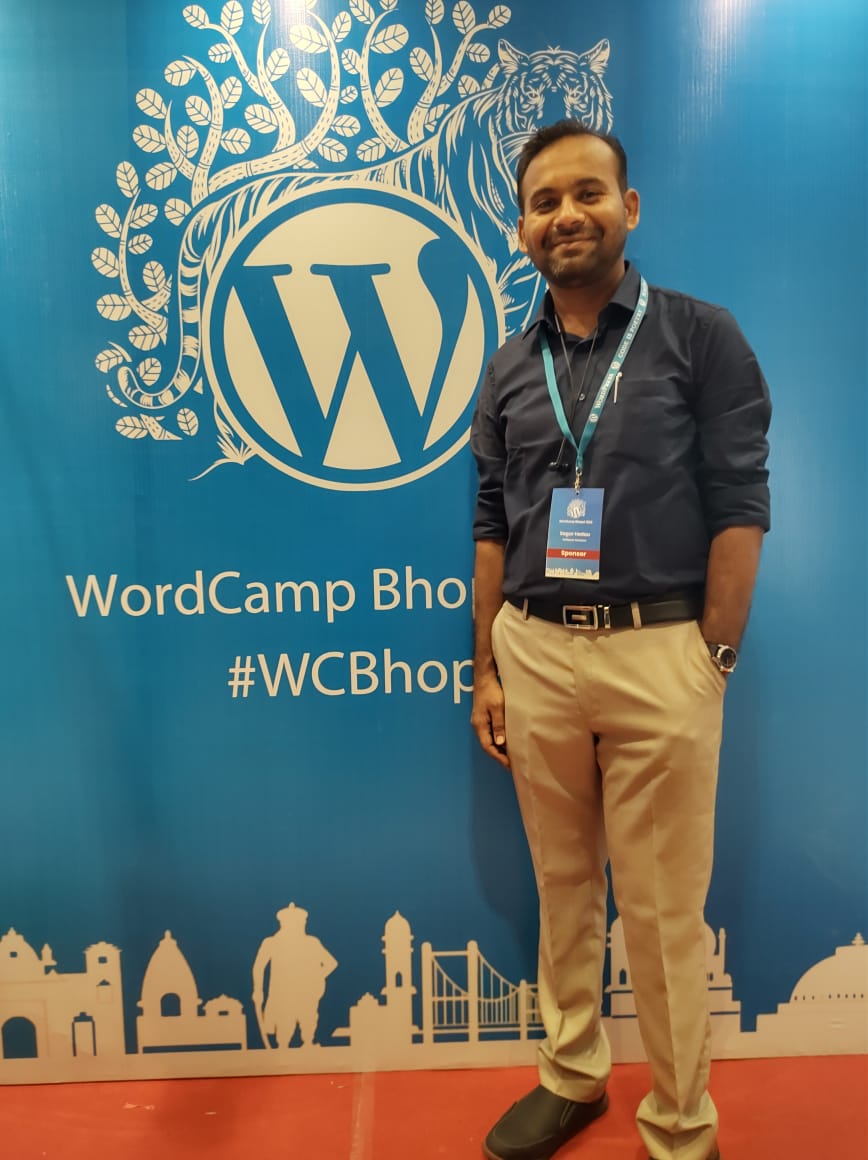
13+ Yrs Experienced Career Counsellor & Skill Development Trainer | Educator | Digital & Content Strategist. Helping freshers and graduates make sound career choices through practical consultation. Guest faculty and Digital Marketing trainer working on building a skill development brand in Softspace Solutions. A passionate writer in core technical topics related to career growth.
Related Posts
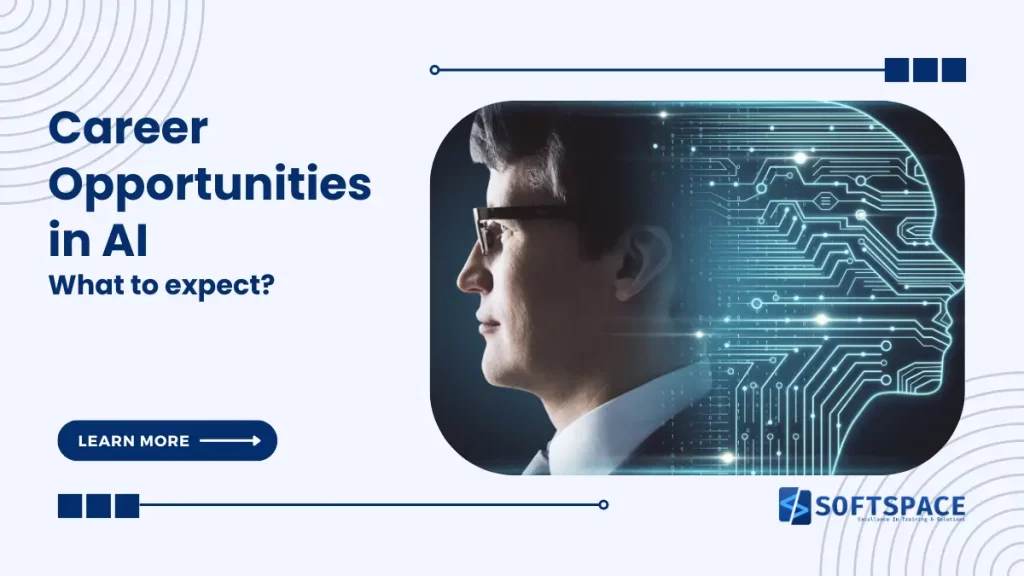
10 Super Career Opportunities in AI | What are you picking?
Explore the top 10 super career opportunities in AI! Discover which cutting-edge roles you can pursue in the fast-growing field of artificial intelligence.
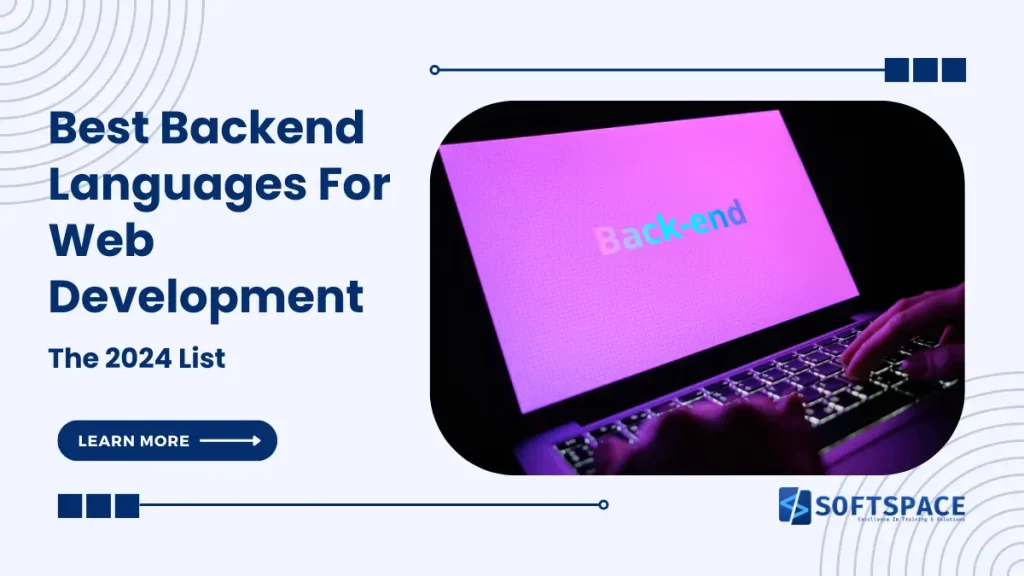
Best Backend Languages For Web Development | The 2024 List
Discover the best backend languages for web development like JavaScript (Node.js), Python, Java, and more. Which is the best and recommended today ion 2024?
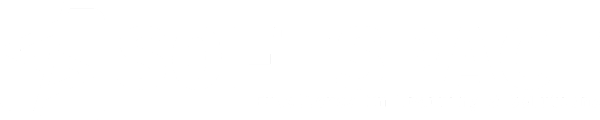
Build skills & your online presence with us. We offer coherent solutions in skill development and building your brand presence online.
Online Courses
Ui/ux courses.
- UI/UX Course
- Web Designing
Analytics Courses
- Data Science
Marketing & CRM
- Digital Marketing
- Website Development
- Career Counselling
© All rights reserved
Copyright @ 2024 | softspace solutions.
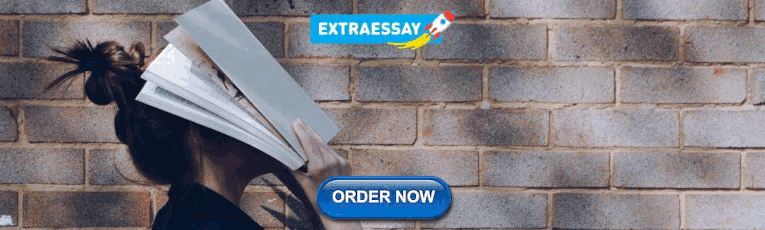
COMMENTS
102 Morningstar Data Research Analyst interview questions and 101 interview reviews. ... In technical and managerial round , questions asked are dependent upon the role which you are applying. Interview questions [1] Question 1. Difference between parent and subsidiary companies ... You have to give aptitude test first which is based on basic ...
"Here at Morningstar, your financial advisors rely on the most accurate and up-to-date data to present to clients. I would take great pride in my job as a data analyst to provide the best data possible. In fact, the pride I take in interpreting complicated data initially attracted me to Morningstar as a company.
Practice 40 Morningstar Data Analyst interview questions. Written by professional interviewers with 40 answer examples. ... I was asked to attend a trade show to research new data analysis tools from a technical perspective. ... may indicate that the data analytics team delegates specific processes to individual members of the team based on ...
The process continued with two rounds of interviews, but unfortunately, I was rejected after the first round. During the interview, I was asked to introduce myself and answer some behavioral questions. read more. Interview questions [1] Question 1. 1. Pros and cons of joining morningstar 2. Tell me about yourself 3.
97 Morningstar Data Research Analyst interview questions and 96 interview reviews. Free interview details posted anonymously by Morningstar interview candidates. ... Morningstar Data Research Analyst Interview Questions. Updated 12 Mar 2024. Search job titles. Find Interviews. ... You have to give aptitude test first which is based on basic ...
89 Morningstar Data Research Analyst interview questions and 88 interview reviews. Free interview details posted anonymously by Morningstar interview candidates.
The interview was only 2 days , i interviewed in July & they responded in October with the joining date & CTC. read more. Interview questions [1] Question 1. 1st and 2nd rounds are mostly Finance based on stocks, IPOs, capital markets, IFRS, GAAP, accounting standards etc, basically almost everything Finance.
Morningstar interview details: 6 interview questions and 6 interview reviews posted anonymously by Morningstar interview candidates. ... (Earlier Known As Data Research Analyst Interview Questions. Updated Oct 11, 2022. Search job titles. Find Interviews. ... Once your resume has been shortlisted, there are three rounds. First is an aptitude ...
I interviewed at Morningstar (Mumbai) in 1/9/2017. Interview. 3 rounds of interview , aptitude, technical questions like merger and acquisition, amortization ,basics of fundamental, ev first round is of aptitude test second is technical round ,it was fast process completed in one day. Interview questions [1]
It was online consist of 3 rounds starting with aptitude followed by 2 virtual rounds of interview. First round was taken by team leader and the last round was conducted by the manager overall good experience. Interview questions [1] Question 1. Strengths and weakness and situational based questions.
Data Research Analyst Interview. I interviewed at Morningstar (Vāshi) You have to give aptitude test first which is based on basic finance questions such as ratio analysis , balance sheet and income statements etc. Then you have to give interview HR and technical interview.
Morningstar Data Research Analyst 1 interview questions and answers interview rounds and process 2024 GD topics test pattern shared by 6 candidates interviewed with Morningstar. ... Question related to aptitude to test analytical skills and English question to know the grammatical ability to speak in English. 3
Morningstar Data Research Analyst Interview Questions for Experienced shared by 12 candidates 2024 recruitment process
Morningstar interview questions and answers interview rounds and process 2024 GD topics test pattern shared by 214 candidates interviewed with Morningstar ... Top skills recommended for Morningstar Data Research Analyst interview Insights by AmbitionBox Data Collection ... The most common rounds in the Morningstar interview process are Aptitude ...
Aptitude Test Round Data Research Analyst - Equity Operations Expected questions in Aptitude test - 30 basic qts about Capital markets, Corporate Finanace and Accounts related qts and 30 qts related QA, DI, VA, LR, VR, etc. ( ps. if you have given any of the MBA entrance tests then this would be real easy for you. ) 60 qts in hour
The process took 1 day. I interviewed at Morningstar (Mumbai) in 1 May 2017. Interview. 1. Aptitude test (Finance related). Writing test and solving problems includes finance and accounts) 2. Technical round (Require knowledge related to finance and share market) Diluted share and Anti-diluted shares 3.
How To Crack Morningstar Research Associate Interview ? Morningstar Aptitude Test & Interview Qus_____My Job Updates ...
Morningstar Data Research Analyst interview questions and answers interview rounds and process 2024 GD topics test pattern shared by 45 candidates interviewed with Morningstar. ... It was basic Aptitude Test with Equity Based Questions. 3 Assignment Round .
Aptitude test, operationa interview and HR interview , then they will give u offer letter regarding joining, work in shifts uk us , morning, afternoon, night. Interview process was quite smooth and nice company to work with. Interview questions [1] Question 1. What is FS, Minority interest, EPS.
From fundamental concepts like statistical analysis and data visualization to more advanced topics such as machine learning and big data technologies, this guide covers the breadth of knowledge expected in a data analyst role. We'll explore questions that test your technical skills, problem-solving abilities, and even your communication ...
A Research Analyst Aptitude Test is a pre-employment evaluation to determine a candidate's research analysis skills and abilities. The test assesses proficiency in numerical ability, data analysis, abstract reasoning, critical thinking, reasoning ability, attention to detail, and verbal ability. The test can be used by recruiters and hiring ...
The most common topics and skills that interviewers at Morningstar expect are Research Analysis, Data Collection, Data Research, Equity and Finance. What are the top questions asked in Morningstar Data Research Analyst interview for freshers?
24 Morningstar MDP Associate interview questions and 26 interview reviews. Free interview details posted anonymously by Morningstar interview candidates. ... Data research analyst (102) Research associate (63) Mdp program (39) Intern (32) Software engineer (29) Mdp associate (25) Analyst (23) Equity research analyst (19) ... aptitude test with ...
Morningstar Senior Data Research Analyst interview questions and answers interview rounds and process 2024 GD topics test pattern shared by 3 candidates interviewed with Morningstar. ... Question related to aptitude to test analytical skills and English question to know the grammatical ability to speak in English. 3