
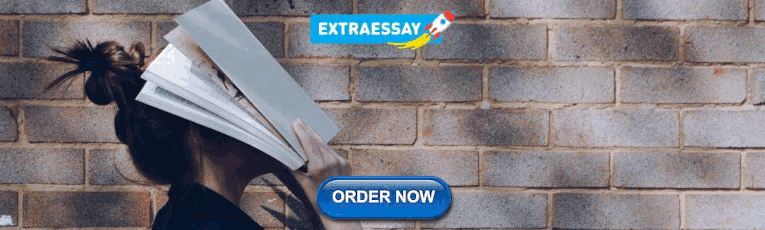
How To Write The Results/Findings Chapter
For qualitative studies (dissertations & theses).
By: Jenna Crossley (PhD Cand). Expert Reviewed By: Dr. Eunice Rautenbach | August 2021
So, you’ve collected and analysed your qualitative data, and it’s time to write up your results chapter – exciting! But where do you start? In this post, we’ll guide you through the qualitative results chapter (also called the findings chapter), step by step.
Overview: Qualitative Results Chapter
- What (exactly) the qualitative results chapter is
- What to include in your results chapter
- How to write up your results chapter
- A few tips and tricks to help you along the way
What exactly is the results chapter?
The results chapter in a dissertation or thesis (or any formal academic research piece) is where you objectively and neutrally present the findings of your qualitative analysis (or analyses if you used multiple qualitative analysis methods ). This chapter can sometimes be combined with the discussion chapter (where you interpret the data and discuss its meaning), depending on your university’s preference. We’ll treat the two chapters as separate, as that’s the most common approach.
In contrast to a quantitative results chapter that presents numbers and statistics, a qualitative results chapter presents data primarily in the form of words . But this doesn’t mean that a qualitative study can’t have quantitative elements – you could, for example, present the number of times a theme or topic pops up in your data, depending on the analysis method(s) you adopt.
Adding a quantitative element to your study can add some rigour, which strengthens your results by providing more evidence for your claims. This is particularly common when using qualitative content analysis. Keep in mind though that qualitative research aims to achieve depth, richness and identify nuances , so don’t get tunnel vision by focusing on the numbers. They’re just cream on top in a qualitative analysis.
So, to recap, the results chapter is where you objectively present the findings of your analysis, without interpreting them (you’ll save that for the discussion chapter). With that out the way, let’s take a look at what you should include in your results chapter.
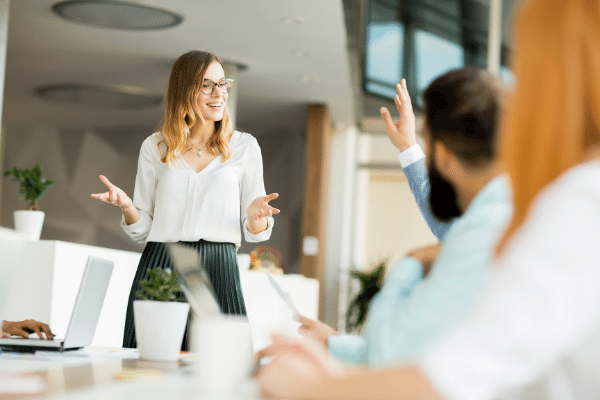
What should you include in the results chapter?
As we’ve mentioned, your qualitative results chapter should purely present and describe your results , not interpret them in relation to the existing literature or your research questions . Any speculations or discussion about the implications of your findings should be reserved for your discussion chapter.
In your results chapter, you’ll want to talk about your analysis findings and whether or not they support your hypotheses (if you have any). Naturally, the exact contents of your results chapter will depend on which qualitative analysis method (or methods) you use. For example, if you were to use thematic analysis, you’d detail the themes identified in your analysis, using extracts from the transcripts or text to support your claims.
While you do need to present your analysis findings in some detail, you should avoid dumping large amounts of raw data in this chapter. Instead, focus on presenting the key findings and using a handful of select quotes or text extracts to support each finding . The reams of data and analysis can be relegated to your appendices.
While it’s tempting to include every last detail you found in your qualitative analysis, it is important to make sure that you report only that which is relevant to your research aims, objectives and research questions . Always keep these three components, as well as your hypotheses (if you have any) front of mind when writing the chapter and use them as a filter to decide what’s relevant and what’s not.
Need a helping hand?
How do I write the results chapter?
Now that we’ve covered the basics, it’s time to look at how to structure your chapter. Broadly speaking, the results chapter needs to contain three core components – the introduction, the body and the concluding summary. Let’s take a look at each of these.
Section 1: Introduction
The first step is to craft a brief introduction to the chapter. This intro is vital as it provides some context for your findings. In your introduction, you should begin by reiterating your problem statement and research questions and highlight the purpose of your research . Make sure that you spell this out for the reader so that the rest of your chapter is well contextualised.
The next step is to briefly outline the structure of your results chapter. In other words, explain what’s included in the chapter and what the reader can expect. In the results chapter, you want to tell a story that is coherent, flows logically, and is easy to follow , so make sure that you plan your structure out well and convey that structure (at a high level), so that your reader is well oriented.
The introduction section shouldn’t be lengthy. Two or three short paragraphs should be more than adequate. It is merely an introduction and overview, not a summary of the chapter.
Pro Tip – To help you structure your chapter, it can be useful to set up an initial draft with (sub)section headings so that you’re able to easily (re)arrange parts of your chapter. This will also help your reader to follow your results and give your chapter some coherence. Be sure to use level-based heading styles (e.g. Heading 1, 2, 3 styles) to help the reader differentiate between levels visually. You can find these options in Word (example below).

Section 2: Body
Before we get started on what to include in the body of your chapter, it’s vital to remember that a results section should be completely objective and descriptive, not interpretive . So, be careful not to use words such as, “suggests” or “implies”, as these usually accompany some form of interpretation – that’s reserved for your discussion chapter.
The structure of your body section is very important , so make sure that you plan it out well. When planning out your qualitative results chapter, create sections and subsections so that you can maintain the flow of the story you’re trying to tell. Be sure to systematically and consistently describe each portion of results. Try to adopt a standardised structure for each portion so that you achieve a high level of consistency throughout the chapter.
For qualitative studies, results chapters tend to be structured according to themes , which makes it easier for readers to follow. However, keep in mind that not all results chapters have to be structured in this manner. For example, if you’re conducting a longitudinal study, you may want to structure your chapter chronologically. Similarly, you might structure this chapter based on your theoretical framework . The exact structure of your chapter will depend on the nature of your study , especially your research questions.
As you work through the body of your chapter, make sure that you use quotes to substantiate every one of your claims . You can present these quotes in italics to differentiate them from your own words. A general rule of thumb is to use at least two pieces of evidence per claim, and these should be linked directly to your data. Also, remember that you need to include all relevant results , not just the ones that support your assumptions or initial leanings.
In addition to including quotes, you can also link your claims to the data by using appendices , which you should reference throughout your text. When you reference, make sure that you include both the name/number of the appendix , as well as the line(s) from which you drew your data.
As referencing styles can vary greatly, be sure to look up the appendix referencing conventions of your university’s prescribed style (e.g. APA , Harvard, etc) and keep this consistent throughout your chapter.
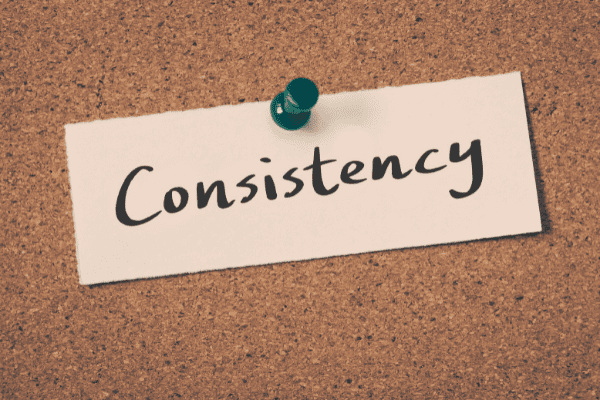
Section 3: Concluding summary
The concluding summary is very important because it summarises your key findings and lays the foundation for the discussion chapter . Keep in mind that some readers may skip directly to this section (from the introduction section), so make sure that it can be read and understood well in isolation.
In this section, you need to remind the reader of the key findings. That is, the results that directly relate to your research questions and that you will build upon in your discussion chapter. Remember, your reader has digested a lot of information in this chapter, so you need to use this section to remind them of the most important takeaways.
Importantly, the concluding summary should not present any new information and should only describe what you’ve already presented in your chapter. Keep it concise – you’re not summarising the whole chapter, just the essentials.
Tips and tricks for an A-grade results chapter
Now that you’ve got a clear picture of what the qualitative results chapter is all about, here are some quick tips and reminders to help you craft a high-quality chapter:
- Your results chapter should be written in the past tense . You’ve done the work already, so you want to tell the reader what you found , not what you are currently finding .
- Make sure that you review your work multiple times and check that every claim is adequately backed up by evidence . Aim for at least two examples per claim, and make use of an appendix to reference these.
- When writing up your results, make sure that you stick to only what is relevant . Don’t waste time on data that are not relevant to your research objectives and research questions.
- Use headings and subheadings to create an intuitive, easy to follow piece of writing. Make use of Microsoft Word’s “heading styles” and be sure to use them consistently.
- When referring to numerical data, tables and figures can provide a useful visual aid. When using these, make sure that they can be read and understood independent of your body text (i.e. that they can stand-alone). To this end, use clear, concise labels for each of your tables or figures and make use of colours to code indicate differences or hierarchy.
- Similarly, when you’re writing up your chapter, it can be useful to highlight topics and themes in different colours . This can help you to differentiate between your data if you get a bit overwhelmed and will also help you to ensure that your results flow logically and coherently.
If you have any questions, leave a comment below and we’ll do our best to help. If you’d like 1-on-1 help with your results chapter (or any chapter of your dissertation or thesis), check out our private dissertation coaching service here or book a free initial consultation to discuss how we can help you.
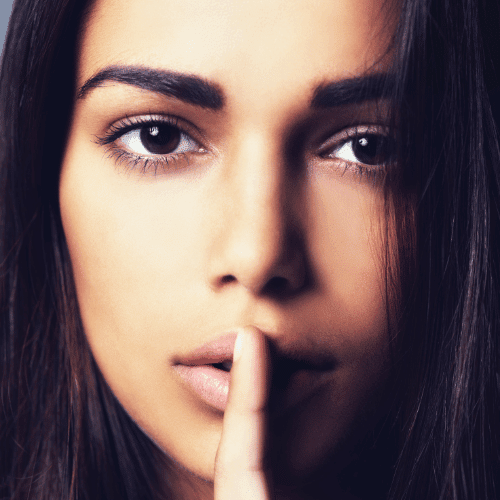
Psst... there’s more!
This post was based on one of our popular Research Bootcamps . If you're working on a research project, you'll definitely want to check this out ...
You Might Also Like:
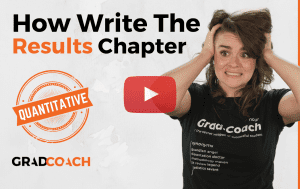
20 Comments
This was extremely helpful. Thanks a lot guys
Hi, thanks for the great research support platform created by the gradcoach team!
I wanted to ask- While “suggests” or “implies” are interpretive terms, what terms could we use for the results chapter? Could you share some examples of descriptive terms?
I think that instead of saying, ‘The data suggested, or The data implied,’ you can say, ‘The Data showed or revealed, or illustrated or outlined’…If interview data, you may say Jane Doe illuminated or elaborated, or Jane Doe described… or Jane Doe expressed or stated.
I found this article very useful. Thank you very much for the outstanding work you are doing.
What if i have 3 different interviewees answering the same interview questions? Should i then present the results in form of the table with the division on the 3 perspectives or rather give a results in form of the text and highlight who said what?
I think this tabular representation of results is a great idea. I am doing it too along with the text. Thanks
That was helpful was struggling to separate the discussion from the findings
this was very useful, Thank you.
Very helpful, I am confident to write my results chapter now.
It is so helpful! It is a good job. Thank you very much!
Very useful, well explained. Many thanks.
Hello, I appreciate the way you provided a supportive comments about qualitative results presenting tips
I loved this! It explains everything needed, and it has helped me better organize my thoughts. What words should I not use while writing my results section, other than subjective ones.
Thanks a lot, it is really helpful
Thank you so much dear, i really appropriate your nice explanations about this.
Thank you so much for this! I was wondering if anyone could help with how to prproperly integrate quotations (Excerpts) from interviews in the finding chapter in a qualitative research. Please GradCoach, address this issue and provide examples.
what if I’m not doing any interviews myself and all the information is coming from case studies that have already done the research.
Very helpful thank you.
This was very helpful as I was wondering how to structure this part of my dissertation, to include the quotes… Thanks for this explanation
This is very helpful, thanks! I am required to write up my results chapters with the discussion in each of them – any tips and tricks for this strategy?
Submit a Comment Cancel reply
Your email address will not be published. Required fields are marked *
Save my name, email, and website in this browser for the next time I comment.
- Print Friendly
How to Analyze Interview Transcripts in Qualitative Research
Rev › Blog › Transcription Blog › How to Analyze Interview Transcripts in Qualitative Research
Studies take time, accuracy, and a drive to provide excellent information, and qualitative research is a critical part of any successful study. You may be wondering how qualitative data adds to a paper or report, given that it’s not the hard “science” we often see highlighted the most often.
How Do You Analyze Qualitative Interviews?
There are two main approaches to qualitative analysis: inductive and deductive . What’s more, there are two types of inductive qualitative analysis to choose from. These are called thematic content analysis and narrative analysis, both of which call for an unstructured approach to research.
Inductive Methods of Analyzing Interview Transcripts
A thematic content analysis begins with weeding out biases and establishing your overarching impressions of the data. Rather than approaching your data with a predetermined framework, identify common themes as you search the materials organically. Your goal is to find common patterns across the data set.
A narrative analysis involves making sense of your interview respondents’ individual stories. Use this type of qualitative data analysis to highlight important aspects of their stories that will best resonate with your readers. And, highlight critical points you have found in other areas of your research.
Deductive Approach to Qualitative Analysis
Deductive analysis , on the other hand, requires a structured or predetermined approach. In this case, the researcher will build categories in advance of their analysis. Then, they’ll map connections in the data to those specific categories.
Each of these qualitative analysis methods lends its benefits to the research effort. Inductive analyses will produce more nuanced findings. Meanwhile, deductive analyses allow the researcher to point to key themes essential to their research.
Successful qualitative research hinges on the accuracy of your data. This can be harder to achieve than with quantitative research. It’s easy to lose important facts and meaning as you transition qualitative data from the source to your published content. This makes transcription a vital tool in maintaining integrity and relaying information in an unbiased way that’s useful for readers and adds appropriate context to the journal or study.
How to Transcribe a Qualitative Interview
Accurate transcription begins early in the interview process, even before you start interviewing. Here are the steps to transcribing a qualitative interview.
1. Collect Feedback for Qualitative Research
There are dozens of ways to gather qualitative data. Recording and accurately transcribing interviews is among the best methods to avoid inaccuracies and data loss, and researchers should consider this approach over simply taking notes firsthand.
Make sure you have a reliable way to record, whether the interview takes place in person, over the phone, or as part of a video call. Depending on the interview method, you may record a video or an audio-only format. Here are some tips depending on where the interview takes place:
- These apps can also be used for over-the-phone interviews.
- For video interviews , we recommend taking advantage of one of our transcription integrations , such as Zoom. Rev also has an API available for those who want to streamline their workflow even further by integrating Rev directly into their processes and platforms.
2. Organize Your Research Recordings
You should ensure that your audio or video files are easy to save, compile, and share. To do this, be sure to adopt easy-to-remember naming conventions as well to ensure they stay organized. An example of a naming convention that is simple to remember and recreate includes “Date.LastNameofSource.Topic”.
3. Transcribe All the Interviews and Focus Group Recordings
The next critical step is transcription. Done manually, this is a long and tedious process that can add hours, days, or even months to your report-writing process. There are dozens of pitfalls when performing transcriptions manually as well, as it can be hard to pick up words spoken in a heavy dialect or quiet tone. You also want to avoid having to transcribe all the “umms” and “ems” that occur when a source is speaking naturally.
Rev provides a variety of transcription services that take the tedium and guesswork out of the research process. You can choose to edit out all of the “umms,” while ensuring that heavy accents or muffled voices are picked up by the recording service.
You can order transcripts from Rev with both audio and video recordings. Once you’ve received your professional transcripts from Rev, you can begin your qualitative analysis.
The 6 Steps of Qualitative Interview Data Analysis
Among qualitative interview data analysis methods, thematic content analysis is perhaps the most common and effective method. It can also be one of the most trustworthy , increasing the traceability and verification of an analysis when done correctly. The following are the six main steps of a successful thematic analysis of your transcripts.
1. Read the Transcripts
By now, you will have accessed your transcript files as digital files in the cloud or have downloaded them to your computer for offline viewing. Start by browsing through your transcripts and making notes of your first impressions. You will be able to identify common themes. This will help you with your final summation of the data.
Next, read through each transcript carefully. Evidence of themes will become stronger, helping you to hone in on important insights.
You must identify bias during this step as well. Biases can appear in the data, among the interviewees, and even within your objectives and methodologies. According to SAGE Publishing , researchers should “acknowledge preconceived notions and actively work to neutralize them” at this early step.
2. Annotate the Transcripts
Annotation is the process of labeling relevant words, phrases, sentences, or sections with codes. These codes help identify important qualitative data types and patterns. Labels can be about actions, activities, concepts, differences, opinions, processes, or whatever you think is relevant. Annotations will help you organize your data for dissemination .
Be generous with your annotations—don’t hold back. You will have an opportunity to eliminate or consolidate them later. It’s best to do more here, so you don’t have to come back to find more opportunities later.
3. Conceptualize the Data
Conceptualizing qualitative data is the process of aligning data with critical themes you will use in your published content. You will have identified many of these themes during your initial review of the transcripts.
To conceptualize, create categories and subcategories by grouping the codes you created during annotation. You may eliminate or combine certain codes rather than using all the codes you created. Keep only the codes you deem relevant to your analysis.
4. Segment the Data
Segmentation is the process of positioning and connecting your categories . This allows you to establish the bulk of your data cohesively. Start by labeling your categories and then describe the connections between them.
You can use these descriptions to improve your final published content.
- Create a spreadsheet to easily compile your data.
- Then, use the columns to structure important variables of your data analysis using codes as tools for reference.
- Create a separate tab for the front of the document that contains a coding table. This glossary contains important codes used in the segmentation process. This will help you and others quickly identify what the codes are referring to.
5. Analyze the Segments
You’re now ready to take a deep dive into your data segments . Start by determining if there is a hierarchy among your categories. Determine if one is more important than the other, or draw a figure to summarize the results. At this stage, you may also want to align qualitative data with any quantitative data you collected.
6. Write the Results
Your analysis of the content is complete—you’re ready to transition your findings into the real body of your content. Use your insights to build and verify theories, answer key questions in your field, and back aims and objectives. Describe your categories and how they are connected using a neutral, objective voice.
Although you will pull heavily from your own research, be sure to publish content in the context of your field. Interpret your results in light of relevant studies, theories, and concepts related to your study.
Why Use Interviews for Qualitative Data
Unlike quantitative data, which is certainly important, a qualitative analysis adds color to academic and business reports. It offers perspective and can make a report more readable, add context, and inspire thoughtful discussion beyond the report.
As we’ve observed, transcribing qualitative interviews is crucial to getting less measurable data from direct sources. They allow researchers to provide relatable stories and perspectives and even quote important contributors directly. Lots of qualitative data from interviews enables authors to avoid embellishment and maintain the integrity of their content as well.
So, how do you conduct interview data analysis on qualitative data to pull key insights and strengthen your reports? Transcribing interviews is one of the most useful tools available for this task.
As a researcher, you need to make the most of recorded interviews . Interview transcripts allow you to use the best qualitative analysis methods. Plus, you can focus only on tasks that add value to your research effort.
Transcription is Essential to Qualitative Research Analysis
Qualitative data is often elusive to researchers. Transcripts allow you to capture original, nuanced responses from your respondents. You get their response naturally using their own words—not a summarized version in your notes.
You can also go back to the original transcript at any time to see what was said as you gain new context. The editable digital transcript files are incredibly easy to work with, saving you time and giving you speaker tags, time marks, and other tools to ensure you can find what you need within a transcript quickly.
When creating a report, accuracy matters, but efficiency matters, as well. Rev offers a seamless way of doing the transcription for you, saving you time and allowing you to focus on high-quality work instead. Consider Rev as your transcription service provider for qualitative research analysis — try Rev’s AI or Human Transcription services today.
Everybody’s Favorite Speech-to-Text Blog
We combine AI and a huge community of freelancers to make speech-to-text greatness every day. Wanna hear more about it?
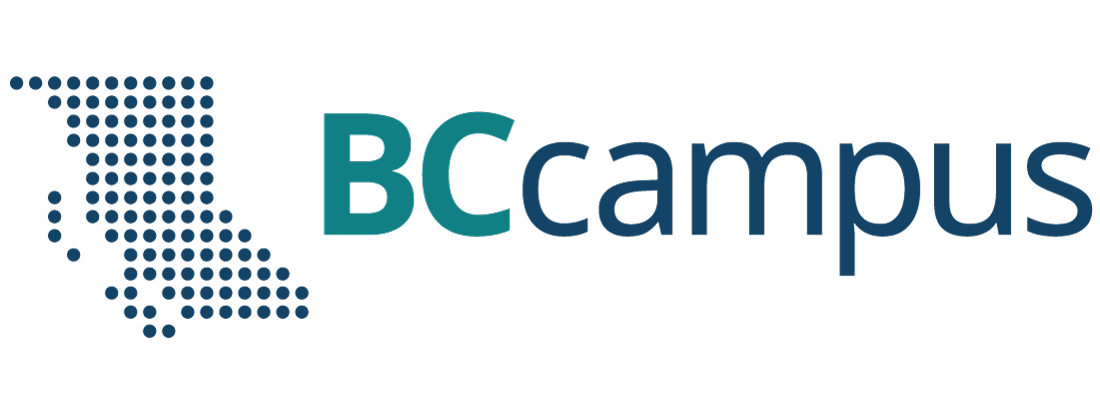
Want to create or adapt books like this? Learn more about how Pressbooks supports open publishing practices.
Chapter 10: Qualitative Data Collection & Analysis Methods
10.5 Analysis of Qualitative Interview Data
Analysis of qualitative interview data typically begins with a set of transcripts of the interviews conducted. Obtaining said transcripts requires either having taken exceptionally good notes during an interview or, preferably, recorded the interview and then transcribed it. To transcribe an interview means to create a complete, written copy of the recorded interview by playing the recording back and typing in each word that is spoken on the recording, noting who spoke which words. In general, it is best to aim for a verbatim transcription, i.e., one that reports word for word exactly what was said in the recorded interview. If possible, it is also best to include nonverbal responses in the written transcription of an interview (if the interview is completed face-to-face, or some other form of visual contact is maintained, such as with Skype). Gestures made by respondents should be noted, as should the tone of voice and notes about when, where, and how spoken words may have been emphasized by respondents.
If you have the time, it is best to transcribe your interviews yourself. If the researcher who conducted the interviews transcribes them herself, that person will also be able to record associated nonverbal behaviors and interactions that may be relevant to analysis but that could not be picked up by audio recording. Interviewees may roll their eyes, wipe tears from their face, and even make obscene gestures that speak volumes about their feelings; however, such non-verbal gestures cannot be recorded, and being able to remember and record in writing these details as it relates to the transcribing of interviews is invaluable.
Overall, the goal of analysis is to reach some inferences, lessons, or conclusions by condensing large amounts of data into relatively smaller, more manageable bits of understandable information. Analysis of qualitative interview data often works inductively (Glaser & Strauss, 1967; Patton, 2001). To move from the specific observations an interviewer collects to identifying patterns across those observations, qualitative interviewers will often begin by reading through transcripts of their interviews and trying to identify codes. A code is a shorthand representation of some more complex set of issues or ideas. The process of identifying codes in one’s qualitative data is often referred to as coding . Coding involves identifying themes across interview data by reading and re-reading (and re-reading again) interview transcripts, until the researcher has a clear idea about what sorts of themes come up across the interviews. Coding helps to achieve the goal of data management and data reduction (Palys & Atchison, 2014, p. 304).
Coding can be inductive or deductive. Deductive coding is the approach used by research analysts who have a well-specified or pre-defined set of interests (Palys & Atchison, 2014, P. 304). The process of deductive coding begins with the analyst utilizing those specific or pre-defined interests to identify “relevant” passages, quotes, images, scenes, etc., to develop a set of preliminary codes (often referred to as descriptive coding ). From there, the analyst elaborates on these preliminary codes, making finer distinctions within each coding category (known as interpretative coding ). Pattern coding is another step an analyst might take as different associations become apparent. For example, if you are studying at-risk behaviours in youth, and you discover that the various behaviours have different characteristics and meanings depending upon the social context (e.g., school, family, work) in which the various behaviours occur, you have identified a pattern (Palys & Atchison, 2014, p. 304).
In contrast, inductive coding begins with the identification of general themes and ideas that emerge as the researcher reads through the data. This process is also referred to as open coding (Palys & Atchison, 2014, p. 305), because it will probably require multiple analyses. As you read through your transcripts, it is likely that you will begin to see some commonalities across the categories or themes that you’ve jotted down (Saylor Academy, 2012). The open coding process can go one of two ways: either the researcher elaborates on a category by making finer, and then even finer distinctions, or the researcher starts with a very specific descriptive category that is subsequently collapsed into another category (Palys & Atchison, 2014, p. 305). In other words, the development and elaboration of codes arise out of the material that is being examined.
The next step for the research analyst is to begin more specific coding, which is known as focused or axial coding . Focused coding involves collapsing or narrowing themes and categories identified in open coding by reading through the notes you made while conducting open coding, identifying themes or categories that seem to be related, and perhaps merging some. Then give each collapsed/merged theme or category a name (or code) and identify passages of data that fit each named category or theme. To identify passages of data that represent your emerging codes, you will need to read through your transcripts several times. You might also write up brief definitions or descriptions of each code. Defining codes is a way of giving meaning to your data, and developing a way to talk about your findings and what your data means (Saylor Academy, 2012).
As tedious and laborious as it might seem to read through hundreds of pages of transcripts multiple times, sometimes getting started with the coding process is actually the hardest part. If you find yourself struggling to identify themes at the open coding stage, ask yourself some questions about your data. The answers should give you a clue about what sorts of themes or categories you are reading (Saylor Academy, 2012). (Lofland and Lofland,1995, p. 2001) identify a set of questions that are useful when coding qualitative data. They suggest asking the following:
- Of what topic, unit, or aspect is this an instance?
- What question about a topic does this item of data suggest?
- What sort of answer to a question about a topic does this item of data suggest (i.e., what proposition is suggested)?
Asking yourself these questions about the passages of data that you are reading can help you begin to identify and name potential themes and categories.
Table 10.3 “ Interview coding” example is drawn from research undertaken by Saylor Academy (Saylor Academy, 2012) where she presents two codes that emerged from her inductive analysis of transcripts from her interviews with child-free adults. Table 10.3 also includes a brief description of each code and a few (of many) interview excerpts from which each code was developed.
Table 10.3 Interview coding
Just as quantitative researchers rely on the assistance of special computer programs designed to help sort through and analyze their data, so, do qualitative researchers. Where quantitative researchers have SPSS and MicroCase (and many others), qualitative researchers have programs such as NVivo ( http://www.qsrinternational.com ) and Atlasti ( http://www.atlasti.com ). These are programs specifically designed to assist qualitative researchers to organize, manage, sort, and analyze large amounts of qualitative data. The programs allow researchers to import interview transcripts contained in an electronic file and then label or code passages, cut and paste passages, search for various words or phrases, and organize complex interrelationships among passages and codes
Research Methods for the Social Sciences: An Introduction Copyright © 2020 by Valerie Sheppard is licensed under a Creative Commons Attribution-NonCommercial-ShareAlike 4.0 International License , except where otherwise noted.
Share This Book
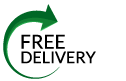
The Plagiarism Checker Online For Your Academic Work
Start Plagiarism Check
Editing & Proofreading for Your Research Paper
Get it proofread now
Online Printing & Binding with Free Express Delivery
Configure binding now
- Academic essay overview
- The writing process
- Structuring academic essays
- Types of academic essays
- Academic writing overview
- Sentence structure
- Academic writing process
- Improving your academic writing
- Titles and headings
- APA style overview
- APA citation & referencing
- APA structure & sections
- Citation & referencing
- Structure and sections
- APA examples overview
- Commonly used citations
- Other examples
- British English vs. American English
- Chicago style overview
- Chicago citation & referencing
- Chicago structure & sections
- Chicago style examples
- Citing sources overview
- Citation format
- Citation examples
- College essay overview
- Application
- How to write a college essay
- Types of college essays
- Commonly confused words
- Definitions
- Dissertation overview
- Dissertation structure & sections
- Dissertation writing process
- Graduate school overview
- Application & admission
- Study abroad
- Master degree
- Harvard referencing overview
- Language rules overview
- Grammatical rules & structures
- Parts of speech
- Punctuation
- Methodology overview
- Analyzing data
- Experiments
- Observations
- Inductive vs. Deductive
- Qualitative vs. Quantitative
- Types of validity
- Types of reliability
- Sampling methods
- Theories & Concepts
- Types of research studies
- Types of variables
- MLA style overview
- MLA examples
- MLA citation & referencing
- MLA structure & sections
- Plagiarism overview
- Plagiarism checker
- Types of plagiarism
- Printing production overview
- Research bias overview
- Types of research bias
- Example sections
- Types of research papers
- Research process overview
- Problem statement
- Research proposal
- Research topic
- Statistics overview
- Levels of measurment
- Frequency distribution
- Measures of central tendency
- Measures of variability
- Hypothesis testing
- Parameters & test statistics
- Types of distributions
- Correlation
- Effect size
- Hypothesis testing assumptions
- Types of ANOVAs
- Types of chi-square
- Statistical data
- Statistical models
- Spelling mistakes
- Tips overview
- Academic writing tips
- Dissertation tips
- Sources tips
- Working with sources overview
- Evaluating sources
- Finding sources
- Including sources
- Types of sources
Your Step to Success
Plagiarism Check within 10min
Printing & Binding with 3D Live Preview
Dissertation Interview – A Short Guide With Helpful Tips
How do you like this article cancel reply.
Save my name, email, and website in this browser for the next time I comment.
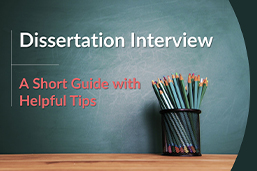
A dissertation interview is a vital tool in academic research, often serving as a primary source in data collection . These structured, semi-structured, or unstructured dialogues provide detailed and firsthand insights into the research topic, supplementing or extending other research methods. The design, execution, and analysis of dissertation interviews require careful planning and a clear understanding of the research question to ensure they contribute effectively to the overarching thesis. This guide aims to guide students with helpful tips for dissertation interviews.
Inhaltsverzeichnis
- 1 Dissertation Interview – In a Nutshell
- 2 Definition: Dissertation interview
- 3 Including a dissertation interview
- 4 Referring to a dissertation interview
- 5 Quoting a dissertation interview
- 6 Using the name of the interviewee from the dissertation interview
Dissertation Interview – In a Nutshell
- Transcribe the interview accurately for inclusion in the dissertation.
- Situations in which you cannot include a dissertation interview transcript in your appendix.
- How to quote a dissertation interview in your dissertation
- What to consider when using the name of the interviewee.
Definition: Dissertation interview
A dissertation interview is a method of primary data collection used in academic research, typically undertaken for a dissertation or thesis. It can be in the form of a structured, semi-structured, or unstructured interview between the researcher and the interviewee(s), with the goal of gaining detailed, firsthand insights into the research topic. The interview questions are formulated based on the research objectives, and the responses are used to support or explore the thesis argument in depth. The information derived from such interviews often complements secondary data or may serve as the primary basis for the research findings.
- Closed questions only allow for a limited number of predetermined answers.
- Open questions encourage individuals to contribute details of their thoughts and feelings.
Including a dissertation interview
You have conducted interviews as part of your descriptive study for your dissertation. How do you incorporate them? There is a high possibility you do not know what is anticipated since no one ever told you.
Transcribing interviews is a condition for using them in dissertations. This may be accomplished with the use of transcribing software. The transcripts of the interviews might be included as an appendix. Due to the length of the appendix, it may be necessary to submit it as a separate document after discussing your dissertation interview with your supervisor. It is essential to have proof that interviews were conducted.

Referring to a dissertation interview
Include the transcripts of the interviews in an appendix, and then refer to them throughout your dissertation via paraphrasing. This is how paraphrasing works:
- Interviewee A claims that (Appendix 1).
- Through conversation with B, it became apparent that (Appendix 1)
There may be cases when you cannot include a dissertation interview transcript in your appendix. If we cannot make any references to the interview, it may be cited in the following way if you are using the APA format .
- Person A claims that (Individual conversation, December 24th, 2012).
Quoting a dissertation interview
You must use quotation marks if you take someone else’s statements in a dissertation interview. Finding fascinating quotations will be much simpler if you understand how to pull useful data out of the individual during the dissertation interview. It’s important to maintain professionalism throughout the dissertation interview.
Using the name of the interviewee from the dissertation interview
Do not copy down the interviewee’s name without considering these two things:
If the identity is important to the study and you have obtained the interviewee’s consent to use it, then you can go ahead. You may use a description instead if you are not authorized to use the name.
How is an interview included in a dissertation?
Use a transitional phrase like “according to” or another reference when introducing your interview in the piece. Likewise, tailor your responses to the particular dissertation interview format you are using. Doing so will give your paper a more credible and convincing character.
Is there a certain number of questions posed in a dissertation interview?
Use two or three queries to get started. Research may become overwhelming in scope if excessive questions are asked. For this reason, you should begin with no more than two or three research topics, but some studies may have more.
When writing a dissertation, how long should an interview last?
They normally take 30 minutes to a few hours to complete and are only done once. It’s common practice in many fields to conduct interviews to gather information.
How likely is it that a dissertation interview will be turned down?
The truth is that defending a dissertation is tough and that some students have theirs turned down. All the academics showing you the ropes on how to write a dissertation that will get you accepted have been rejected at some time in their careers.
We use cookies on our website. Some of them are essential, while others help us to improve this website and your experience.
- External Media
Individual Privacy Preferences
Cookie Details Privacy Policy Imprint
Here you will find an overview of all cookies used. You can give your consent to whole categories or display further information and select certain cookies.
Accept all Save
Essential cookies enable basic functions and are necessary for the proper function of the website.
Show Cookie Information Hide Cookie Information
Statistics cookies collect information anonymously. This information helps us to understand how our visitors use our website.
Content from video platforms and social media platforms is blocked by default. If External Media cookies are accepted, access to those contents no longer requires manual consent.
Privacy Policy Imprint
We Trust in Human Precision
20,000+ Professional Language Experts Ready to Help. Expertise in a variety of Niches.
- API Pricing
- Cost estimate
- Customer loyalty program
- Educational Discount
- Non-Profit Discount
- Green Initiative Discount1
Value-Driven Pricing
Unmatched expertise at affordable rates tailored for your needs. Our services empower you to boost your productivity.

- Special Discounts
- Enterprise transcription solutions
- Enterprise translation solutions
- Transcription/Caption API
- AI Transcription Proofreading API
Trusted by Global Leaders
GoTranscript is the chosen service for top media organizations, universities, and Fortune 50 companies.
- API solutions
GoTranscript
One of the Largest Online Transcription and Translation Agencies in the World. Founded in 2005.

6 Easy Steps in Learning How to Analyze Interview Data in Qualitative Studies
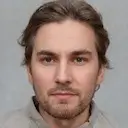
It’s understandable if you find qualitative data analysis quite challenging. Unlike quantitative methodologies, qualitative data analysis doesn’t follow a systematic, rigid, and formulaic process. Once you decide to analyze interview transcripts in qualitative research, the work is slightly more intuitive than tangible.
We won’t sugarcoat things and say that qualitative analysis is an easy task. However, there are practical steps you can take to avoid turning the entire process into something chaotic. In most cases, you’ll start by having accurate interview transcripts that you need to read over and over again.
This article will guide teach you how to transcribe and analyze interview data for qualitative research.
Why It’s Important to Analyze Interview Data in Qualitative Research
Quantitative data may offer rigor to your research. However, it is qualitative analysis that will add color to your business or academic report. By taking this approach, you can add context to your data and make it more readable. It will inspire a more meaningful discussion that goes beyond the hard numbers in your report.
Most of the time, interviews allow you to acquire subjective data from direct sources. As a researcher, you’ll get relatable perspectives and stories. You can also get important quotes from subject matter experts. Interviews provide qualitative data that strengthens the integrity and eliminates embellishment in their research.
Now, how do you acquire the information you need for qualitative data? Well, transcribing interviews provides you with the best resources for qualitative analysis. You can add life to your research by interviewing the following sources:
- Business leaders
- Scientists and investors
- Trial or study participants
- Journal and research authors
You can include sources that may contribute to the quality of the research. Now, whenever you conduct an interview, you should use the right methods to ensure you’ll get the information you need. You must maximize your recorded interviews by transcribing them accurately.
How to Get an Accurate Qualitative Interview Transcript
It’s more challenging to get the information you need in a qualitative interview. However, by taking a systematic approach, it will be easier for you to gather the data you need. Here are some steps to follow:
1. Gather Qualitative Data
Qualitative data can be collected through various means. For one, you can record the interview and take advantage of legal-grade transcription services. Taking this approach will help you avoid data loss and inaccuracies. This method is ideal for focus group discussions and customer interviews. With this process, respondents can be more open in answering the questions.
You may have above-average note-taking skills, but that’s usually unreliable in qualitative research. Whether you’re conducting the interview over the phone, on a video conference call, or in person, you can collect accurate data by recording the conversation. You can either record the interview in an audio-only or video format.
2. Compile Your Interview Recordings Neatly
Your interview video or audio files should be easy to find, identify, and share. Use file-naming conventions that are easy to recall and organize. Ideally, you must include the date of the interview, the source’s last name, and the discussion topic.
No matter what file naming convention you choose, you need to be consistent in the format. This way, it’s easier for you to find the necessary files later when you analyze interview data.
3. Transcribe the Focus Group and Interview Recordings
The next step is to transcribe the recordings. Now, there are plenty of tools online that can automate the process. However, remember that you are working on qualitative research, and you need an accurate transcription.
So, your best bet here is to use 100% human-generated transcription services. GoTranscript offers verbatim results, allowing you to get legal-level accuracy for your qualitative interview recordings. If you’re following tight deadlines, you can opt for a rush order. This way, you’ll get your files within your requested turnaround time and begin your qualitative analysis.
What Are the Methods Used to Analyze Interview Data in Qualitative Research?
When it comes to qualitative data analysis, you can choose from two primary approaches – inductive and deductive. If you opt for the former, you can take an unstructured approach to your research by conducting narrative or thematic content analysis.
Inductive Method for Analyzing Qualitative Interview Transcripts
- Thematic content analysis – This method starts by identifying your overarching impressions of the information and weeding out biases. Instead of establishing a framework for approaching the data, you search the materials organically and look for common themes. You aim to discover the recurring patterns across the data set.
- Narrative content analysis – This method requires you to make sense of the individual stories of your interview respondents. It is the ideal approach for identifying important aspects of the data that resonate well with your readers.
Deductive Method for Analyzing Qualitative Interview Transcripts
Deductive data analysis is a more systematic or structured approach. Before you begin to analyze interview data, you need to develop categories. During the process, you’ll connect information from the data to those pre-determined categories.
Both approaches to data analysis bring benefits to your research. If you want more nuanced findings, the inductive method is the ideal option. Now, if you want to point to key themes that are crucial to your research, opt for deductive analysis.
6 Practical Steps in Performing Qualitative Analysis of Interview Transcripts
Most of the time, researchers opt for thematic content analysis. Many find it to be the most effective and trustworthy method. The approach makes it easier for anyone to verify and trace the findings of the analysis. Here are six practical steps for conducting a thematic analysis of your interview transcripts:
Step 1: Go Through the Transcripts Carefully
At this point, you likely have a digital copy of your transcript files. You may have them stored locally on your computer or in the cloud. Begin by skimming through the transcripts, and along the process, write down your first impressions. This makes to easier to analyze interview data.
Taking this approach will help you identify common patterns in the data. Your quick notes will also be instrumental in helping you come up with a final summation of the data.
Now, you need to read every transcript carefully. After multiple run-throughs, the common themes will become more visible. As a result, you will be able to come up with crucial and significant insights.
During this process, you should also identify biases. Even within your methodologies and objectives, biases will surface in the data and among the interviewees. Sage Publishing recommends that as early as this step, you should actively neutralize the preconceived notions you identify. This way, you can take an objective approach when you begin to analyze interview data.
Step 2: Begin Annotating the Transcripts
Once you get to this step, you need to label the key phrases, terms, sentences, or section codes on the transcript. Annotating your transcripts allows you to identify important qualitative data patterns and types.
The labels can highlight the varying interviewee opinions, concepts, and data differences. This process makes it easier for you to organize your data set for dissemination. As a result, you’re cutting down the time it takes to analyze interview data.
Step 3: Align the Data with Important Themes
This step requires you to conceptualize the qualitative data by aligning it with important themes for your final content. At this phase, you will have identified the common patterns from the initial transcript reviews.
When conceptualizing the data, you need to group the codes from the annotation to create categories and subcategories. You can combine or eliminate certain codes instead of using all of them. You should identify and retain the codes that are relevant to your analysis.
Step 4: Position and Connect Your Categories
Now, you need to segment the data by positioning and connecting your categories. During this step, you will be able to cohesively establish most of your data. You can begin by labeling the categories, and describing how they connect to each other. The descriptions will be instrumental in optimizing your final output. Here are the steps you can take:
- Compile your data conveniently by using a spreadsheet.
- Structure the data’s critical variables by labeling the columns with proper reference codes.
- The document containing the coding table should be on a separate tab. This tab functions as a glossary containing critical codes for the segmentation process. As a result, you can quickly identify what the codes are referring to.
Most researchers prefer doing all these steps electronically for convenience. However, some people prefer having a printed copy so they can highlight segments using pens. In any case, you should save a copy of the transcript files. You can print them out when you need to.
Step 5: Perform a Deep Analysis of the Data Segments
Once you’ve segmented the data, it’s time to perform a deep analysis. You can begin by identifying the categories’ hierarchy. You need to decide if one category is more important than the other. You can also summarize the results by drawing a figure. This stage should also let you align the qualitative data with your quantitative data.
Step 6: Write Your Findings
Once you’ve performed a deep analysis of your data, you’re ready to write the results into a body of content. Establish and verify theories by using your data insights. You can also answer key questions that your field may ask, and don’t forget to adequately support your research goals. Use an objective, neutral voice in describing how your categories connect to each other.
Naturally, you will use your own research and pull insights heavily from it. However, make sure to consider the context of your field when writing your results. Interpret your findings alongside relevant theories, studies, and concepts when you analyze interview data.
Accurate Transcription Is Necessary in Qualitative Research Analysis
Researchers often find qualitative data analysis challenging. As such, it is important to have transcripts that capture the nuanced, unadulterated responses from interviewees. You can get their organic responses because they are using their own words and not the shortened version from your notes.
What’s great about having a transcript is that you can go back to it whenever you gain find new context. However, to ensure a reasonable level of objectivity, you must ensure that your transcript is accurate.
With GoTranscript, you can have a convenient yet efficient way of transcribing your qualitative interviews. You can save time and focus on more important aspects of your research.

- PRO Courses Guides New Tech Help Pro Expert Videos About wikiHow Pro Upgrade Sign In
- EDIT Edit this Article
- EXPLORE Tech Help Pro About Us Random Article Quizzes Request a New Article Community Dashboard This Or That Game Popular Categories Arts and Entertainment Artwork Books Movies Computers and Electronics Computers Phone Skills Technology Hacks Health Men's Health Mental Health Women's Health Relationships Dating Love Relationship Issues Hobbies and Crafts Crafts Drawing Games Education & Communication Communication Skills Personal Development Studying Personal Care and Style Fashion Hair Care Personal Hygiene Youth Personal Care School Stuff Dating All Categories Arts and Entertainment Finance and Business Home and Garden Relationship Quizzes Cars & Other Vehicles Food and Entertaining Personal Care and Style Sports and Fitness Computers and Electronics Health Pets and Animals Travel Education & Communication Hobbies and Crafts Philosophy and Religion Work World Family Life Holidays and Traditions Relationships Youth
- Browse Articles
- Learn Something New
- Quizzes Hot
- This Or That Game New
- Train Your Brain
- Explore More
- Support wikiHow
- About wikiHow
- Log in / Sign up
- Education and Communications
Analyzing Qualitative Interview Data: Step-by-Step Guide
Last Updated: April 13, 2024
This article was co-authored by wikiHow Staff . Our trained team of editors and researchers validate articles for accuracy and comprehensiveness. wikiHow's Content Management Team carefully monitors the work from our editorial staff to ensure that each article is backed by trusted research and meets our high quality standards. This article has been viewed 34,765 times. Learn more...
Interviews are a great source for research projects because they add personal insights and help readers connect to the material. Our guide will show you how to transcribe, categorize, and analyze these interviews to get the most out of them.
Organizing Your Data

- You'll read through the interviews multiple times. Each time, note any new thoughts you have or questions that you need to answer.

- If you recorded the interviews, you'll need to transcribe the recordings, too.
- Transcription might seem tedious, but it's a great way to further immerse yourself in the data.
- You can also choose to use transcription software instead. You can use software to use fonts as indicators and also include features such as time stamps.

- Your social situation
- Your economic situation
- Your personal characteristics

- For example, you might want to use the interviews with teachers as your main focus. Alternatively, you might highlight the voices of parents.

- Some pros of software include finding different methods of categorization, the ability to share your data with other researchers, and it also allows you to better manage large data sets.
- Before purchasing software, read online reviews of different products. Make sure that it will do what you need it to do. For instance, if you want it to transcribe your data set, make sure that it has a transcription function.
- You can also talk to co-workers, professors, or other researchers about which software they like to use.
- One drawback to using software is that you might not familiarize yourself with the data as much if you rely on software. It's really a personal choice.

- For example, you might note that red font indicates interviews with teachers.

- For example, in your interviews about the public school system, you could create a category of data and give it the code “classroom experience”.
- You could also give codes such as “elementary school” and “high school”. Your codes can be really simple! The idea is for them to be very user-friendly to you.
Using Thematic Analysis of the Interviews

- You might notice that parents of high school students are very focused on graduation rates. That might be another theme that you highlight.

- Keep arranging your data until you have a set of themes that support your argument. You want the interviews to satisfactorily answer your research question.

- For example, instead of simply “assessment”, you might describe that theme as “innovations in measuring student success”.

- If you are giving an oral presentation, make visual aids such as a PowerPoint presentation. This will help your audience engage with the material.
- It can be really useful to use video clips of the interviews. Just make sure you have secured the participant's permission!
Choosing Narrative Analysis

- For example, you might go through the minutes of recent school board meetings. You could also look at photos of various classrooms around the city.

- Also make note if you think any of the sources aren't credible or if you think you need to verify the information elsewhere.
- Begin grouping related data to form stories. For example, group all of the material related to school counselors in one group. Ask yourself what type of story the data tells.

- Do any necessary additional resource. For example, you might want to conduct some follow-up interviews.

- Typically, instead of including a lot of hard data, you'll focus on the more personal aspects of the interview. For example, your story might center on students who struggled to make the transition to high school and what sort of challenges they faced.
Expert Q&A
- Don't be afraid to switch approaches. You might start out with a thematic approach and decide that a narrative approach would better suit your themes. Thanks Helpful 0 Not Helpful 0
- Spend a lot of time working with your data. The more familiar you are with your interviews, the more clearly you'll see themes. Thanks Helpful 0 Not Helpful 0

You Might Also Like

- ↑ https://cirt.gcu.edu/research/develop/research_ready/qualitative/7
- ↑ Jeremiah Kaplan. Research & Training Specialist. Expert Interview. 2 September 2021.
- ↑ https://www.bumc.bu.edu/crro/files/2010/07/Hesse-Bieber-4-10.pdf
- ↑ https://www.interaction-design.org/literature/article/how-to-do-a-thematic-analysis-of-user-interviews
About This Article

- Send fan mail to authors
Did this article help you?

Featured Articles

Trending Articles

Watch Articles

- Terms of Use
- Privacy Policy
- Do Not Sell or Share My Info
- Not Selling Info
Get all the best how-tos!
Sign up for wikiHow's weekly email newsletter

An official website of the United States government
The .gov means it’s official. Federal government websites often end in .gov or .mil. Before sharing sensitive information, make sure you’re on a federal government site.
The site is secure. The https:// ensures that you are connecting to the official website and that any information you provide is encrypted and transmitted securely.
- Publications
- Account settings
Preview improvements coming to the PMC website in October 2024. Learn More or Try it out now .
- Advanced Search
- Journal List
- Am J Pharm Educ
- v.74(8); 2010 Oct 11
Presenting and Evaluating Qualitative Research
The purpose of this paper is to help authors to think about ways to present qualitative research papers in the American Journal of Pharmaceutical Education . It also discusses methods for reviewers to assess the rigour, quality, and usefulness of qualitative research. Examples of different ways to present data from interviews, observations, and focus groups are included. The paper concludes with guidance for publishing qualitative research and a checklist for authors and reviewers.
INTRODUCTION
Policy and practice decisions, including those in education, increasingly are informed by findings from qualitative as well as quantitative research. Qualitative research is useful to policymakers because it often describes the settings in which policies will be implemented. Qualitative research is also useful to both pharmacy practitioners and pharmacy academics who are involved in researching educational issues in both universities and practice and in developing teaching and learning.
Qualitative research involves the collection, analysis, and interpretation of data that are not easily reduced to numbers. These data relate to the social world and the concepts and behaviors of people within it. Qualitative research can be found in all social sciences and in the applied fields that derive from them, for example, research in health services, nursing, and pharmacy. 1 It looks at X in terms of how X varies in different circumstances rather than how big is X or how many Xs are there? 2 Textbooks often subdivide research into qualitative and quantitative approaches, furthering the common assumption that there are fundamental differences between the 2 approaches. With pharmacy educators who have been trained in the natural and clinical sciences, there is often a tendency to embrace quantitative research, perhaps due to familiarity. A growing consensus is emerging that sees both qualitative and quantitative approaches as useful to answering research questions and understanding the world. Increasingly mixed methods research is being carried out where the researcher explicitly combines the quantitative and qualitative aspects of the study. 3 , 4
Like healthcare, education involves complex human interactions that can rarely be studied or explained in simple terms. Complex educational situations demand complex understanding; thus, the scope of educational research can be extended by the use of qualitative methods. Qualitative research can sometimes provide a better understanding of the nature of educational problems and thus add to insights into teaching and learning in a number of contexts. For example, at the University of Nottingham, we conducted in-depth interviews with pharmacists to determine their perceptions of continuing professional development and who had influenced their learning. We also have used a case study approach using observation of practice and in-depth interviews to explore physiotherapists' views of influences on their leaning in practice. We have conducted in-depth interviews with a variety of stakeholders in Malawi, Africa, to explore the issues surrounding pharmacy academic capacity building. A colleague has interviewed and conducted focus groups with students to explore cultural issues as part of a joint Nottingham-Malaysia pharmacy degree program. Another colleague has interviewed pharmacists and patients regarding their expectations before and after clinic appointments and then observed pharmacist-patient communication in clinics and assessed it using the Calgary Cambridge model in order to develop recommendations for communication skills training. 5 We have also performed documentary analysis on curriculum data to compare pharmacist and nurse supplementary prescribing courses in the United Kingdom.
It is important to choose the most appropriate methods for what is being investigated. Qualitative research is not appropriate to answer every research question and researchers need to think carefully about their objectives. Do they wish to study a particular phenomenon in depth (eg, students' perceptions of studying in a different culture)? Or are they more interested in making standardized comparisons and accounting for variance (eg, examining differences in examination grades after changing the way the content of a module is taught). Clearly a quantitative approach would be more appropriate in the last example. As with any research project, a clear research objective has to be identified to know which methods should be applied.
Types of qualitative data include:
- Audio recordings and transcripts from in-depth or semi-structured interviews
- Structured interview questionnaires containing substantial open comments including a substantial number of responses to open comment items.
- Audio recordings and transcripts from focus group sessions.
- Field notes (notes taken by the researcher while in the field [setting] being studied)
- Video recordings (eg, lecture delivery, class assignments, laboratory performance)
- Case study notes
- Documents (reports, meeting minutes, e-mails)
- Diaries, video diaries
- Observation notes
- Press clippings
- Photographs
RIGOUR IN QUALITATIVE RESEARCH
Qualitative research is often criticized as biased, small scale, anecdotal, and/or lacking rigor; however, when it is carried out properly it is unbiased, in depth, valid, reliable, credible and rigorous. In qualitative research, there needs to be a way of assessing the “extent to which claims are supported by convincing evidence.” 1 Although the terms reliability and validity traditionally have been associated with quantitative research, increasingly they are being seen as important concepts in qualitative research as well. Examining the data for reliability and validity assesses both the objectivity and credibility of the research. Validity relates to the honesty and genuineness of the research data, while reliability relates to the reproducibility and stability of the data.
The validity of research findings refers to the extent to which the findings are an accurate representation of the phenomena they are intended to represent. The reliability of a study refers to the reproducibility of the findings. Validity can be substantiated by a number of techniques including triangulation use of contradictory evidence, respondent validation, and constant comparison. Triangulation is using 2 or more methods to study the same phenomenon. Contradictory evidence, often known as deviant cases, must be sought out, examined, and accounted for in the analysis to ensure that researcher bias does not interfere with or alter their perception of the data and any insights offered. Respondent validation, which is allowing participants to read through the data and analyses and provide feedback on the researchers' interpretations of their responses, provides researchers with a method of checking for inconsistencies, challenges the researchers' assumptions, and provides them with an opportunity to re-analyze their data. The use of constant comparison means that one piece of data (for example, an interview) is compared with previous data and not considered on its own, enabling researchers to treat the data as a whole rather than fragmenting it. Constant comparison also enables the researcher to identify emerging/unanticipated themes within the research project.
STRENGTHS AND LIMITATIONS OF QUALITATIVE RESEARCH
Qualitative researchers have been criticized for overusing interviews and focus groups at the expense of other methods such as ethnography, observation, documentary analysis, case studies, and conversational analysis. Qualitative research has numerous strengths when properly conducted.
Strengths of Qualitative Research
- Issues can be examined in detail and in depth.
- Interviews are not restricted to specific questions and can be guided/redirected by the researcher in real time.
- The research framework and direction can be quickly revised as new information emerges.
- The data based on human experience that is obtained is powerful and sometimes more compelling than quantitative data.
- Subtleties and complexities about the research subjects and/or topic are discovered that are often missed by more positivistic enquiries.
- Data usually are collected from a few cases or individuals so findings cannot be generalized to a larger population. Findings can however be transferable to another setting.
Limitations of Qualitative Research
- Research quality is heavily dependent on the individual skills of the researcher and more easily influenced by the researcher's personal biases and idiosyncrasies.
- Rigor is more difficult to maintain, assess, and demonstrate.
- The volume of data makes analysis and interpretation time consuming.
- It is sometimes not as well understood and accepted as quantitative research within the scientific community
- The researcher's presence during data gathering, which is often unavoidable in qualitative research, can affect the subjects' responses.
- Issues of anonymity and confidentiality can present problems when presenting findings
- Findings can be more difficult and time consuming to characterize in a visual way.
PRESENTATION OF QUALITATIVE RESEARCH FINDINGS
The following extracts are examples of how qualitative data might be presented:
Data From an Interview.
The following is an example of how to present and discuss a quote from an interview.
The researcher should select quotes that are poignant and/or most representative of the research findings. Including large portions of an interview in a research paper is not necessary and often tedious for the reader. The setting and speakers should be established in the text at the end of the quote.
The student describes how he had used deep learning in a dispensing module. He was able to draw on learning from a previous module, “I found that while using the e learning programme I was able to apply the knowledge and skills that I had gained in last year's diseases and goals of treatment module.” (interviewee 22, male)
This is an excerpt from an article on curriculum reform that used interviews 5 :
The first question was, “Without the accreditation mandate, how much of this curriculum reform would have been attempted?” According to respondents, accreditation played a significant role in prompting the broad-based curricular change, and their comments revealed a nuanced view. Most indicated that the change would likely have occurred even without the mandate from the accreditation process: “It reflects where the profession wants to be … training a professional who wants to take on more responsibility.” However, they also commented that “if it were not mandated, it could have been a very difficult road.” Or it “would have happened, but much later.” The change would more likely have been incremental, “evolutionary,” or far more limited in its scope. “Accreditation tipped the balance” was the way one person phrased it. “Nobody got serious until the accrediting body said it would no longer accredit programs that did not change.”
Data From Observations
The following example is some data taken from observation of pharmacist patient consultations using the Calgary Cambridge guide. 6 , 7 The data are first presented and a discussion follows:
Pharmacist: We will soon be starting a stop smoking clinic. Patient: Is the interview over now? Pharmacist: No this is part of it. (Laughs) You can't tell me to bog off (sic) yet. (pause) We will be starting a stop smoking service here, Patient: Yes. Pharmacist: with one-to-one and we will be able to help you or try to help you. If you want it. In this example, the pharmacist has picked up from the patient's reaction to the stop smoking clinic that she is not receptive to advice about giving up smoking at this time; in fact she would rather end the consultation. The pharmacist draws on his prior relationship with the patient and makes use of a joke to lighten the tone. He feels his message is important enough to persevere but he presents the information in a succinct and non-pressurised way. His final comment of “If you want it” is important as this makes it clear that he is not putting any pressure on the patient to take up this offer. This extract shows that some patient cues were picked up, and appropriately dealt with, but this was not the case in all examples.
Data From Focus Groups
This excerpt from a study involving 11 focus groups illustrates how findings are presented using representative quotes from focus group participants. 8
Those pharmacists who were initially familiar with CPD endorsed the model for their peers, and suggested it had made a meaningful difference in the way they viewed their own practice. In virtually all focus groups sessions, pharmacists familiar with and supportive of the CPD paradigm had worked in collaborative practice environments such as hospital pharmacy practice. For these pharmacists, the major advantage of CPD was the linking of workplace learning with continuous education. One pharmacist stated, “It's amazing how much I have to learn every day, when I work as a pharmacist. With [the learning portfolio] it helps to show how much learning we all do, every day. It's kind of satisfying to look it over and see how much you accomplish.” Within many of the learning portfolio-sharing sessions, debates emerged regarding the true value of traditional continuing education and its outcome in changing an individual's practice. While participants appreciated the opportunity for social and professional networking inherent in some forms of traditional CE, most eventually conceded that the academic value of most CE programming was limited by the lack of a systematic process for following-up and implementing new learning in the workplace. “Well it's nice to go to these [continuing education] events, but really, I don't know how useful they are. You go, you sit, you listen, but then, well I at least forget.”
The following is an extract from a focus group (conducted by the author) with first-year pharmacy students about community placements. It illustrates how focus groups provide a chance for participants to discuss issues on which they might disagree.
Interviewer: So you are saying that you would prefer health related placements? Student 1: Not exactly so long as I could be developing my communication skill. Student 2: Yes but I still think the more health related the placement is the more I'll gain from it. Student 3: I disagree because other people related skills are useful and you may learn those from taking part in a community project like building a garden. Interviewer: So would you prefer a mixture of health and non health related community placements?
GUIDANCE FOR PUBLISHING QUALITATIVE RESEARCH
Qualitative research is becoming increasingly accepted and published in pharmacy and medical journals. Some journals and publishers have guidelines for presenting qualitative research, for example, the British Medical Journal 9 and Biomedcentral . 10 Medical Education published a useful series of articles on qualitative research. 11 Some of the important issues that should be considered by authors, reviewers and editors when publishing qualitative research are discussed below.
Introduction.
A good introduction provides a brief overview of the manuscript, including the research question and a statement justifying the research question and the reasons for using qualitative research methods. This section also should provide background information, including relevant literature from pharmacy, medicine, and other health professions, as well as literature from the field of education that addresses similar issues. Any specific educational or research terminology used in the manuscript should be defined in the introduction.
The methods section should clearly state and justify why the particular method, for example, face to face semistructured interviews, was chosen. The method should be outlined and illustrated with examples such as the interview questions, focusing exercises, observation criteria, etc. The criteria for selecting the study participants should then be explained and justified. The way in which the participants were recruited and by whom also must be stated. A brief explanation/description should be included of those who were invited to participate but chose not to. It is important to consider “fair dealing,” ie, whether the research design explicitly incorporates a wide range of different perspectives so that the viewpoint of 1 group is never presented as if it represents the sole truth about any situation. The process by which ethical and or research/institutional governance approval was obtained should be described and cited.
The study sample and the research setting should be described. Sampling differs between qualitative and quantitative studies. In quantitative survey studies, it is important to select probability samples so that statistics can be used to provide generalizations to the population from which the sample was drawn. Qualitative research necessitates having a small sample because of the detailed and intensive work required for the study. So sample sizes are not calculated using mathematical rules and probability statistics are not applied. Instead qualitative researchers should describe their sample in terms of characteristics and relevance to the wider population. Purposive sampling is common in qualitative research. Particular individuals are chosen with characteristics relevant to the study who are thought will be most informative. Purposive sampling also may be used to produce maximum variation within a sample. Participants being chosen based for example, on year of study, gender, place of work, etc. Representative samples also may be used, for example, 20 students from each of 6 schools of pharmacy. Convenience samples involve the researcher choosing those who are either most accessible or most willing to take part. This may be fine for exploratory studies; however, this form of sampling may be biased and unrepresentative of the population in question. Theoretical sampling uses insights gained from previous research to inform sample selection for a new study. The method for gaining informed consent from the participants should be described, as well as how anonymity and confidentiality of subjects were guaranteed. The method of recording, eg, audio or video recording, should be noted, along with procedures used for transcribing the data.
Data Analysis.
A description of how the data were analyzed also should be included. Was computer-aided qualitative data analysis software such as NVivo (QSR International, Cambridge, MA) used? Arrival at “data saturation” or the end of data collection should then be described and justified. A good rule when considering how much information to include is that readers should have been given enough information to be able to carry out similar research themselves.
One of the strengths of qualitative research is the recognition that data must always be understood in relation to the context of their production. 1 The analytical approach taken should be described in detail and theoretically justified in light of the research question. If the analysis was repeated by more than 1 researcher to ensure reliability or trustworthiness, this should be stated and methods of resolving any disagreements clearly described. Some researchers ask participants to check the data. If this was done, it should be fully discussed in the paper.
An adequate account of how the findings were produced should be included A description of how the themes and concepts were derived from the data also should be included. Was an inductive or deductive process used? The analysis should not be limited to just those issues that the researcher thinks are important, anticipated themes, but also consider issues that participants raised, ie, emergent themes. Qualitative researchers must be open regarding the data analysis and provide evidence of their thinking, for example, were alternative explanations for the data considered and dismissed, and if so, why were they dismissed? It also is important to present outlying or negative/deviant cases that did not fit with the central interpretation.
The interpretation should usually be grounded in interviewees or respondents' contributions and may be semi-quantified, if this is possible or appropriate, for example, “Half of the respondents said …” “The majority said …” “Three said…” Readers should be presented with data that enable them to “see what the researcher is talking about.” 1 Sufficient data should be presented to allow the reader to clearly see the relationship between the data and the interpretation of the data. Qualitative data conventionally are presented by using illustrative quotes. Quotes are “raw data” and should be compiled and analyzed, not just listed. There should be an explanation of how the quotes were chosen and how they are labeled. For example, have pseudonyms been given to each respondent or are the respondents identified using codes, and if so, how? It is important for the reader to be able to see that a range of participants have contributed to the data and that not all the quotes are drawn from 1 or 2 individuals. There is a tendency for authors to overuse quotes and for papers to be dominated by a series of long quotes with little analysis or discussion. This should be avoided.
Participants do not always state the truth and may say what they think the interviewer wishes to hear. A good qualitative researcher should not only examine what people say but also consider how they structured their responses and how they talked about the subject being discussed, for example, the person's emotions, tone, nonverbal communication, etc. If the research was triangulated with other qualitative or quantitative data, this should be discussed.
Discussion.
The findings should be presented in the context of any similar previous research and or theories. A discussion of the existing literature and how this present research contributes to the area should be included. A consideration must also be made about how transferrable the research would be to other settings. Any particular strengths and limitations of the research also should be discussed. It is common practice to include some discussion within the results section of qualitative research and follow with a concluding discussion.
The author also should reflect on their own influence on the data, including a consideration of how the researcher(s) may have introduced bias to the results. The researcher should critically examine their own influence on the design and development of the research, as well as on data collection and interpretation of the data, eg, were they an experienced teacher who researched teaching methods? If so, they should discuss how this might have influenced their interpretation of the results.
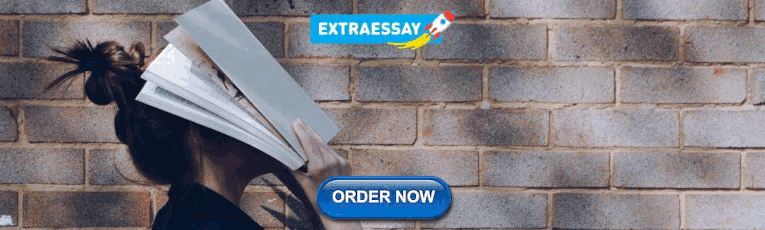
Conclusion.
The conclusion should summarize the main findings from the study and emphasize what the study adds to knowledge in the area being studied. Mays and Pope suggest the researcher ask the following 3 questions to determine whether the conclusions of a qualitative study are valid 12 : How well does this analysis explain why people behave in the way they do? How comprehensible would this explanation be to a thoughtful participant in the setting? How well does the explanation cohere with what we already know?
CHECKLIST FOR QUALITATIVE PAPERS
This paper establishes criteria for judging the quality of qualitative research. It provides guidance for authors and reviewers to prepare and review qualitative research papers for the American Journal of Pharmaceutical Education . A checklist is provided in Appendix 1 to assist both authors and reviewers of qualitative data.
ACKNOWLEDGEMENTS
Thank you to the 3 reviewers whose ideas helped me to shape this paper.
Appendix 1. Checklist for authors and reviewers of qualitative research.
Introduction
- □ Research question is clearly stated.
- □ Research question is justified and related to the existing knowledge base (empirical research, theory, policy).
- □ Any specific research or educational terminology used later in manuscript is defined.
- □ The process by which ethical and or research/institutional governance approval was obtained is described and cited.
- □ Reason for choosing particular research method is stated.
- □ Criteria for selecting study participants are explained and justified.
- □ Recruitment methods are explicitly stated.
- □ Details of who chose not to participate and why are given.
- □ Study sample and research setting used are described.
- □ Method for gaining informed consent from the participants is described.
- □ Maintenance/Preservation of subject anonymity and confidentiality is described.
- □ Method of recording data (eg, audio or video recording) and procedures for transcribing data are described.
- □ Methods are outlined and examples given (eg, interview guide).
- □ Decision to stop data collection is described and justified.
- □ Data analysis and verification are described, including by whom they were performed.
- □ Methods for identifying/extrapolating themes and concepts from the data are discussed.
- □ Sufficient data are presented to allow a reader to assess whether or not the interpretation is supported by the data.
- □ Outlying or negative/deviant cases that do not fit with the central interpretation are presented.
- □ Transferability of research findings to other settings is discussed.
- □ Findings are presented in the context of any similar previous research and social theories.
- □ Discussion often is incorporated into the results in qualitative papers.
- □ A discussion of the existing literature and how this present research contributes to the area is included.
- □ Any particular strengths and limitations of the research are discussed.
- □ Reflection of the influence of the researcher(s) on the data, including a consideration of how the researcher(s) may have introduced bias to the results is included.
Conclusions
- □ The conclusion states the main finings of the study and emphasizes what the study adds to knowledge in the subject area.
Dissertation Interviews
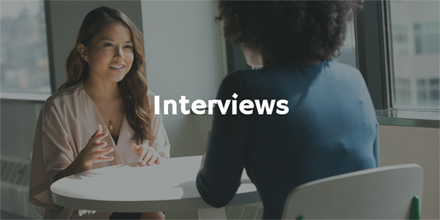
This blog will look into holding interviews as a dissertation research method. Many students choose to hold interviews to collect primary data for their research. This blog should help students collect good data. A Dissertation Interview is one type of research method . Dissertation Interviews can be combined with case studies , and/or questionnaires in your research. Holding dissertation interviews has important ethical considerations .
Who to Interview
“How many interviews are needed?” is a frequent question from students. I use this example:
If my research was to establish the strategy for a particular company I might need to interview:
- 50 shop-floor employees – and I probably wouldn’t get a good answer!
- 10 managers – who between them might come up with nearly the exact wording. (I may have to interpret the result)
- 3 senior mangers – who may say exactly the same and correct thing
- 1 CEO – who wrote the strategy!
In short – you want to interview experts. The literature review shows that you are a master of knowledge in your topic area. Collecting data is showing that you have seen an application of this knowledge by practitioners. It is best to collect data from experts in the field – those with experience at applying the knowledge.
One suggestion is to find a topic expert from a professional body to interview. You could use LinkedIn to try and identify such an expert. This type of interview can compliment interviews within a case study company.
Types of Interview
The research methodology chapter should mention the type of interview to be use.
- Structured. You will ask a set of questions carefully thought out beforehand. If a question cannot be answered, the respondent will move onto the next question without discussion.
- Semi Structured. You will ask a set of questions prepared beforehand. If a question cannot be answered, you can explain your reasons for asking the question or clarify the question in order to assist with gaining a comment.
- Unstructured. There will be no prepared questions. After introductions you could simply say “Tell me about….”.You could also show some sort of model or concept for the interviewee to comment on.
Structured interviews can be quick to gather information. They also ask all people the same set of questions. This will assist repeatability. Unstructured interviews can gain a deeper insight into the thoughts of the interviewee.
You need to explain how and why the particular interviewees were chosen.
Preparing to Interview
The questions should arise from the Literature Review . In the case of the structured interview the exact reasons for asking each question needs to be established. It is a good idea to test the interview questions for clarity on a fellow student:
- Are the questions clear?
- Are they interpreted correctly?
- Can the answers be analysed?
Spending time getting the questions correct will lead to better research.
Holding the Interview
For face-to-face or virtual interviews:
- Be prompt – but be patient and be prepared to wait for the interviewee
- Politeness costs nothing. The interviewee does not have to give up their time
- Be prepared with copies of the questions, paper for taking notes, or recording systems
- Have a copy of the participant information leaflet and consent forms ready for signatures
- Respect the interviewees time
- Don’t just ask your research questions, set a context for the interviewee with some background questions.
Being prepared helps you to appear professional and organised. The interviewee is more likely to spend time with you.
Interview Writing Up
Once the interview data is collected, the results need to be written up. I am a firm believer of every chapter in the dissertation having a single purpose. Therefore I suggest:
- Full interview transcripts can be placed in the appendix if desired
- Results Chapter: State the number of interviews held, dates held, and positions of the interviewees. This is where you may want to establish the ‘expertise’ of the interviewees. Results from each question could be presented in a tabular format.
- Analysis Chapter : Interview questions can be analysed using content analysis. It may be sufficient to compare answers for the same questions, and to cross reference the academic theory.
- Save any discussion for the discussion chapter.
Imperfections in the interviews need to be reported. These can actually help the discussion chapter, and provide information for the recommendations and further work sections.
Collecting primary data via interviews can be supplemented with using a case study.
Interviews take time to prepare and write up. Showing a comprehensive understandings of the interview process will help gain a better dissertation mark!
AbleSim Project Management Simulations YouTube Channel
AbleSim have a YouTube channel dedicated to work with Project Management Simulations, Masters Dissertation Support and MS Project.
Subscribe to this channel here!
Project Management Training YouTube Channel
Andrew Bell has a YouTube channel dedicated to Project Management Training with over 10 hours of video arranged by 97 videos in 20 playlists.
Follow 🔔 AbleSim 🔔 on twitter @AbleSim
Join the conversation #pmsim
- Tweets could not be loaded at this time.
Like us on Facebook
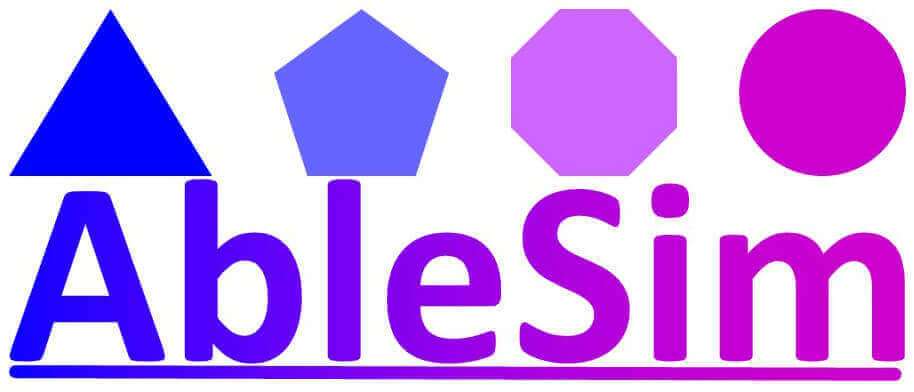
- Privacy Overview
- Strictly Necessary Cookies
- 3rd Party Cookies
This website uses cookies so that we can provide you with the best user experience possible.
Cookie information is stored in your browser and performs functions such as recognising you when you return to our website and helping our team to understand which sections of the website you find most interesting and useful.
Strictly necessary cookies should be enabled at all times so that we can save your preferences for cookie settings.
If you disable this cookie, we will not be able to save your preferences. This means that every time you visit this website you will need to enable or disable cookies again.
This website uses Google Analytics to collect anonymous information such as the number of visitors to the site, and the most popular pages.
Keeping this cookie enabled helps us to improve our website.
Please enable Strictly Necessary Cookies first so that we can save your preferences!
- Online Degree Explore Bachelor’s & Master’s degrees
- MasterTrack™ Earn credit towards a Master’s degree
- University Certificates Advance your career with graduate-level learning
- Top Courses
- Join for Free
Top Data Scientist Interview Questions and Tips
Explore this guide discussing what you can expect during a data science interview and example data science interview questions. You'll also learn how best to prepare for a data science interview, including tips on practice and job research.
![dissertation interview data [Featured image] Woman in an interview](https://d3njjcbhbojbot.cloudfront.net/api/utilities/v1/imageproxy/https://images.ctfassets.net/wp1lcwdav1p1/3NXFLoWKJD1qNCxQUaOT2h/92d7250204d31ea51648b5b49735399f/iStock-1168255943.jpg?w=1500&h=680&q=60&fit=fill&f=faces&fm=jpg&fl=progressive&auto=format%2Ccompress&dpr=1&w=1000)
You've landed an interview for your dream job as a data scientist and are ready to show off your knowledge and expertise to the hiring manager. But, as a data-oriented professional, you know that the best way to improve your chances of success is by preparing in advance with practice questions and answers.
This article will explore some of the most common questions posed to data scientists in job interviews and provide tips for answering them. Finally, it will also introduce you to cost-effective online courses that can help you master your next interview.
Top data science interview questions
Preparation is key to ensuring you enter your next data science interview confidently. Below, you'll find a list of the most common types of data scientist interview questions, covering everything from coding and data modelling to algorithms and statistics.
Coding and programming questions
Coding is an essential skill for data science roles, regardless of the company in which you're working. As a result, interviewers will likely ask you about your prior experience with common programming languages like Python, R, and SQL. Typically, these questions involve data manipulation using code devised to test your programming, problem-solving, and innovation skills. During the interview, you'll likely be required to use a computer or whiteboard to complete the questions, or you may be asked to talk through the problem verbally and explain your thought process. Here are some potential coding and programming questions you could be asked:
"What would you do if a categorisation, an aggregation, and a ratio came up in the same query?"
"Calculate the Jaccard similarity between two sets: the size of the intersection divided by the size of the union."
"Write a program that prints numbers from one through to 50 in a language of your choice."
"List all orders, including customer information, using a basic SQL query."
Data modelling techniques questions
After coding, questions on data modelling techniques will likely be asked during your job interview. In particular, interviewers will likely want to know if you're familiar with different data models and their uses. Interviewers ask questions of this type to test your knowledge of building statistical models and implementing machine learning models, such as linear regression models, logistic regression models, and decision tree models. During your interview, here are some questions that you might encounter:
"How should you maintain a deployed model?"
"Can you name a disadvantage of using the linear model?"
"What is regularisation in regression?"
"What is a confusion matrix?"
Questions on algorithms
Algorithms undergird much of the work that you'll be doing as a data scientist. Questions on algorithms are primarily designed to test how you think about a problem and demonstrate your knowledge. During your interview, you'll likely be asked to explain the purposes of different algorithms, how they might help solve other problems, and demonstrate your knowledge of various machine learning algorithms. As a result, you should brush up on your knowledge of common algorithms such as linear regression and logistic regression. While the exact questions you'll be asked will vary from one interview to another, here are some of the most common forms they may take:
"How would you reverse a linked list?"
"The recommendations, 'People who bought this also bought…' seen on many e-commerce sites, resulting from which algorithm?"
"If we are looking to predict the probability of death from heart disease based on three risk factors: age, gender, and high cholesterol levels, what is the most appropriate algorithm to use?"
"How often should an algorithm be updated?"
Statistics and probability questions
Statistics are a cornerstone concept in data science. Unsurprisingly, interviewers ask questions about statistics in a data science interview to test your knowledge of statistical theory and associated principles. This is your chance to showcase your knowledge of common statistical analysis methods and concepts to refresh your knowledge before the big day. Some common topics to review include random sampling, systematic sampling, and probability distribution. During your interview, questions of this type may take the following forms:
"What is the law of large numbers?"
"What is selection bias?"
"What is the process of working towards a random forest?"
"What is an example of a data type with a non-Gaussian distribution?"
Questions on product sense and business applications
Many employers are more interested in effective data scientists' impact on their bottom line than in exploring the field academically. In effect, you should be asked how your work contributes to the growth of the business and the development of the goods or services it sells. These questions are specific to the industry and how you use data science. Answering them effectively can show that you can apply your data science knowledge to a business capacity rather than just understanding theory. Questions will likely be particular to the role, but use the following as a guide:
"We are looking to improve a new feature for our product. What metrics would you track to ensure it’s a good idea?"
"If we were looking to grow X metric on X feature, how might we achieve that?"
"Tell me about a time you set about aligning data projects with company goals."
"When measuring the impact of a search toolbar change, which metric would you use?"
Tips for preparing for your data science interview
Thoroughly practising for your interview is the best way to ensure its success. To help you prepare for the big day, here are some ways to ensure you are ready for whatever comes up.
1. Research the position and the company
If you want to know what may be asked in your data science interview, the best place to start is by researching the role you are applying for and the company itself.
Check out company websites, social media pages, reviews, and even speak to people working there. The more you can learn about the work culture, the company’s values, and the methods and systems they use, the better you can tailor your answers and demonstrate that you are fully aligned with their goals.
By researching your role, you can also better predict some of the questions you may encounter. Go through the job description and see what is expected, as this will likely be what you are evaluated on. Ensure you have an example prepared for each point and a good bank of potential answers to any question.
2. Understand the job description roles, and responsibilities
As you review the job description and responsibilities, try to get a clear sense of what will be expected of you. If there is anything in the job description that you don’t understand, search the internet, look up the terms, or call the company and ask for clarification.
If you fully understand expectations, tailoring your answers and giving highly relevant examples will be easier. Demonstrating the value you will add to the business with clear responses and concrete examples will highlight your qualifications for the position and the real-world effects of your work.
3. Practice answering commonly asked questions
After finishing the research, and with some help from the questions in this article, you should know what to expect in the interview. Write down these questions and practice your answers. It might feel strange, but the best way to do this is to speak out loud as if you are talking to the interviewer. Doing it aloud means you can hear how your answers will sound and help you practice your volume, speed, and body language. The more you practice, the easier the answers will come to you and the more prepared you will be to recall the information during the interview.
Have your questions ready
While it’s important to think about the questions you’ll have to answer, it’s also essential to have some questions ready that you will ask at the end of the interview.
Many overlook this, but it is an excellent way to learn more about the role, decide whether it is for you, and show interest in the position and company. Some examples of questions include:
• What is the metric on which my performance will be evaluated?
• How will the projects I work on align with key business goals?
• What are the top three reasons you like working here?
• What are the most immediate projects that need to be addressed?
Further support
Regardless of your experience level, interviews can be nerve-wracking undertakings that have the potential to shake your self-confidence. Through preparation, you can enter your following interview with your head held high.
As you're looking for your next data science job, consider taking a cost-effective online course through Coursera to prepare for your next interview. For example, Big Interview’s The Art of the Job Interview will teach proven techniques in five beginner-friendly classes to help you turn your job interviews into job offers.
Keep reading
Coursera staff.
Editorial Team
Coursera’s editorial team is comprised of highly experienced professional editors, writers, and fact...
This content has been made available for informational purposes only. Learners are advised to conduct additional research to ensure that courses and other credentials pursued meet their personal, professional, and financial goals.
Download Interview guide PDF
- Data Structure Interview Questions
Download PDF
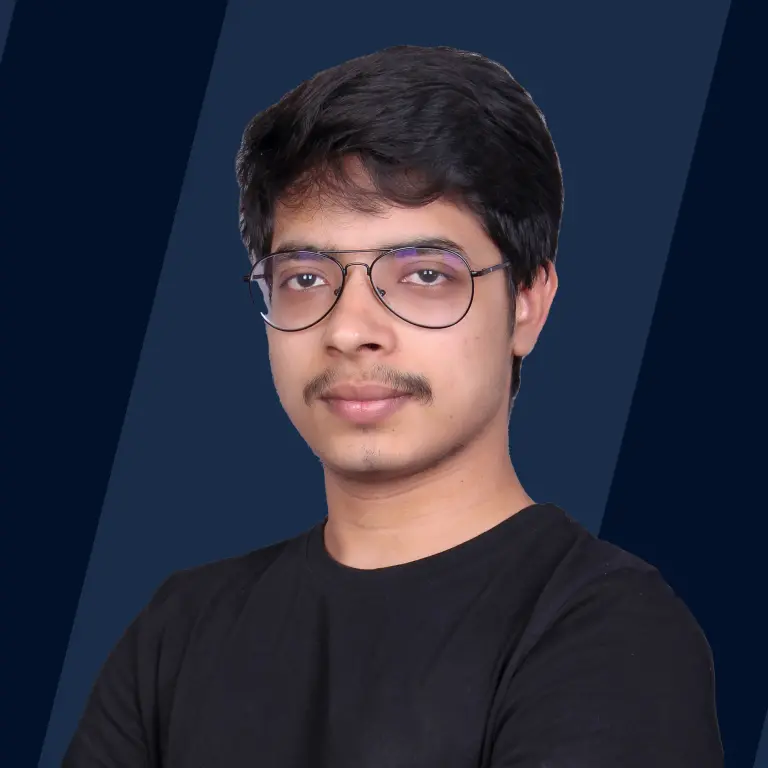
Data structures are the building blocks of any computer program as they help in organizing and manipulating data in an efficient manner. Without data structures, the computer would be unable to understand how to follow a program's instructions properly. It also defines their relationship with one another.
Arrays , Linked Lists , Stacks, Queues, and others are examples of Data Structure. Data structures also provide clarity, organization and structure to the program's code while also helping the programmer ensure that each line of code performs its function correctly.
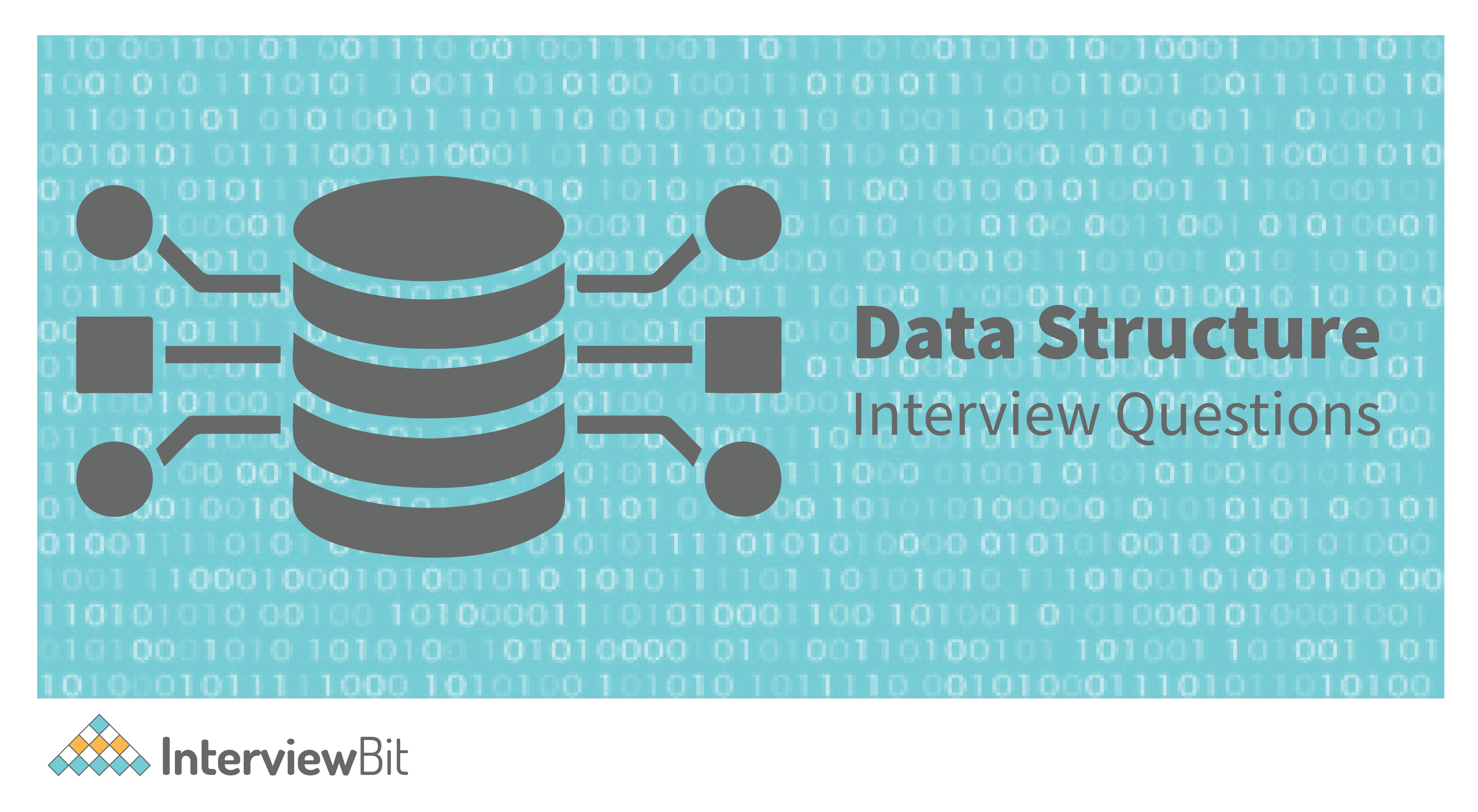
In this article, we've compiled the answers to the most frequently asked Data Structure Interview Questions so that you may better prepare for your job interview.
Basic Data Structure Interview Questions for Freshers
Data structure interview questions for experienced, data structure mcq, 1. what are data structures.
A data structure is a mechanical or logical way that data is organized within a program. The organization of data is what determines how a program performs. There are many types of data structures, each with its own uses. When designing code, we need to pay particular attention to the way data is structured. If data isn't stored efficiently or correctly structured, then the overall performance of the code will be reduced.
2. Why Create Data Structures?
Data structures serve a number of important functions in a program. They ensure that each line of code performs its function correctly and efficiently, they help the programmer identify and fix problems with his/her code, and they help to create a clear and organized code base.
3. What are some applications of Data structures?
Following are some real-time applications of data structures:
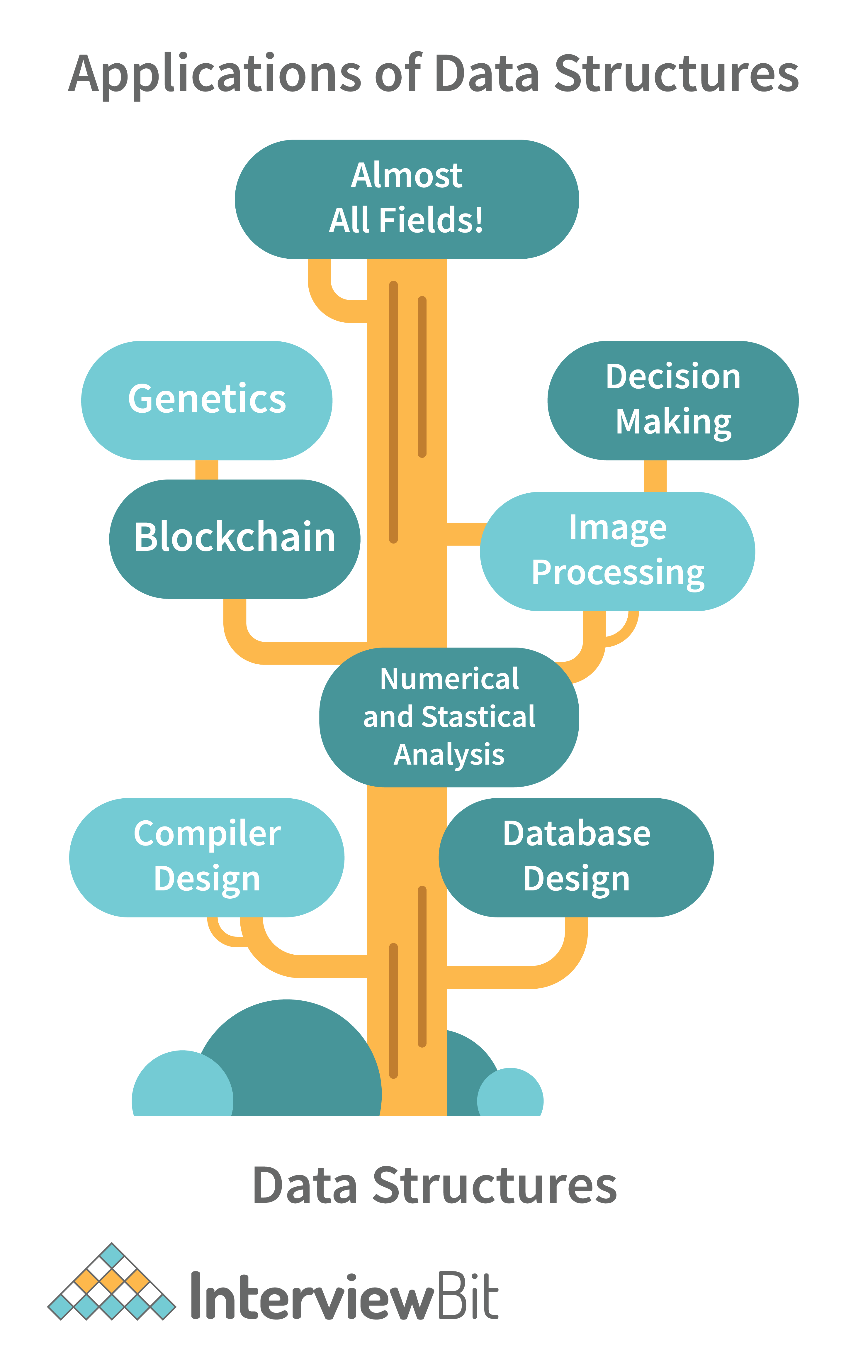
- Decision Making
- Image Processing
- Numerical and Statistical Analysis
- Compiler Design
- Database Design and many more
4. Explain the process behind storing a variable in memory.
- The required amount of memory is assigned first.
- Then, it is stored based on the data structure being used.
- Using concepts like dynamic allocation ensures high efficiency and that the storage units can be accessed based on requirements in real-time.
5. Can you explain the difference between file structure and storage structure?
- File Structure: Representation of data into secondary or auxiliary memory say any device such as a hard disk or pen drives that stores data which remains intact until manually deleted is known as a file structure representation.
- Storage Structure : In this type, data is stored in the main memory i.e RAM, and is deleted once the function that uses this data gets completely executed.
The difference is that the storage structure has data stored in the memory of the computer system, whereas the file structure has the data stored in the auxiliary memory.
Learn via our Video Courses
6. describe the types of data structures.
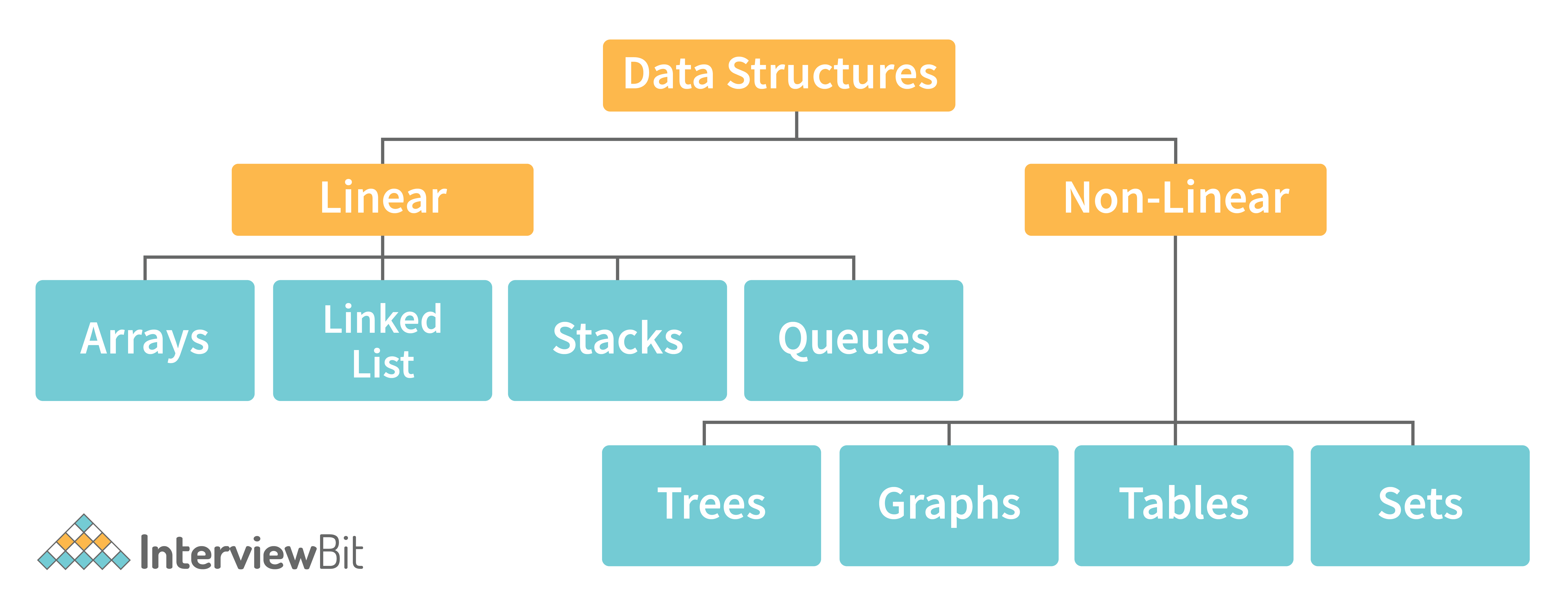
- Linear Data Structure: A data structure that includes data elements arranged sequentially or linearly, where each element is connected to its previous and next nearest elements, is referred to as a linear data structure. Arrays and linked lists are two examples of linear data structures.
- Non-Linear Data Structure: Non-linear data structures are data structures in which data elements are not arranged linearly or sequentially. We cannot walk through all elements in one pass in a non-linear data structure, as in a linear data structure. Trees and graphs are two examples of non-linear data structures.
7. What is a stack data structure? What are the applications of stack?
A stack is a data structure that is used to represent the state of an application at a particular point in time. The stack consists of a series of items that are added to the top of the stack and then removed from the top. It is a linear data structure that follows a particular order in which operations are performed. LIFO (Last In First Out) or FILO (First In Last Out) are two possible orders. A stack consists of a sequence of items. The element that's added last will come out first, a real-life example might be a stack of clothes on top of each other. When we remove the cloth that was previously on top, we can say that the cloth that was added last comes out first.
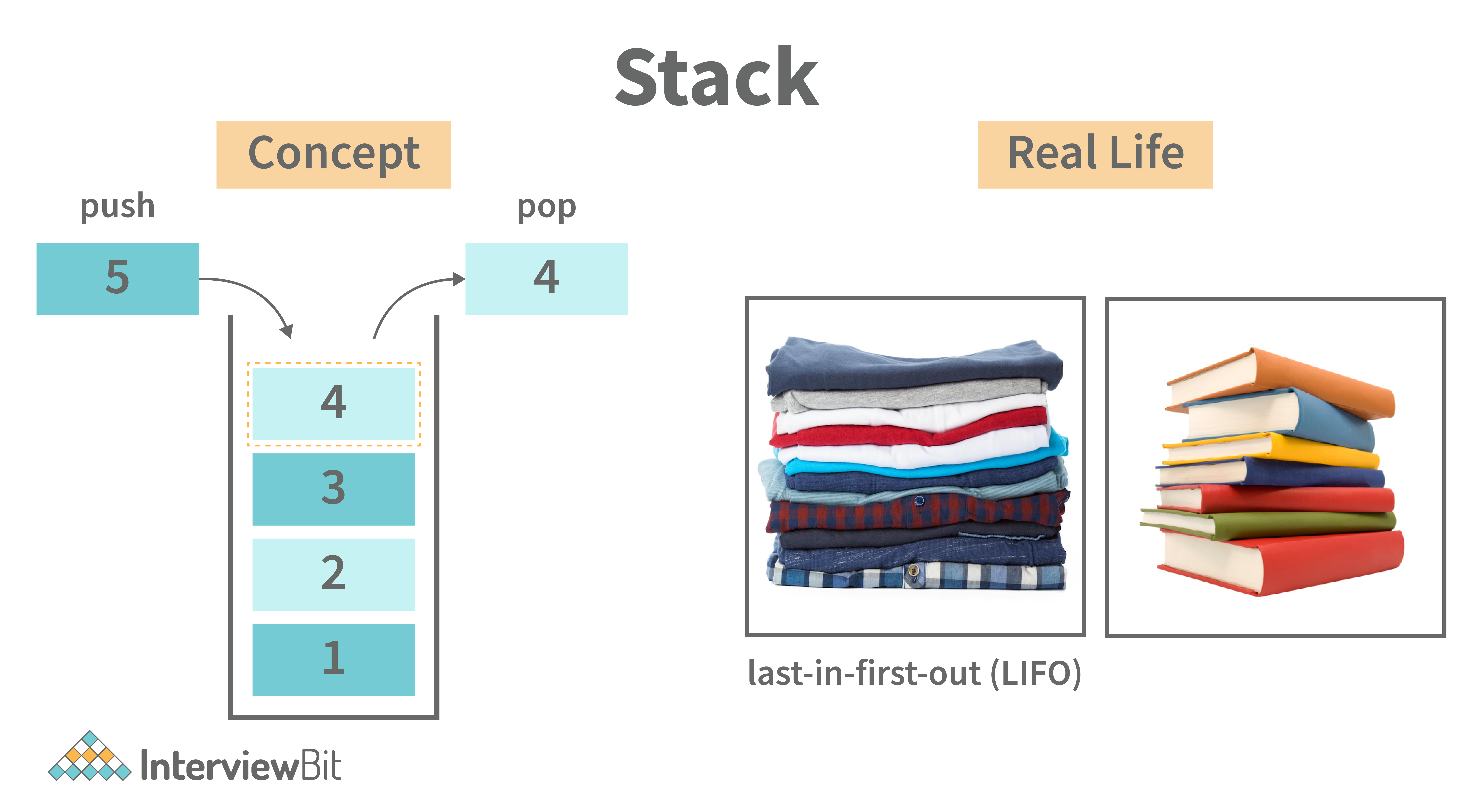
Following are some applications for stack data structure:
- It acts as temporary storage during recursive operations
- Redo and Undo operations in doc editors
- Reversing a string
- Parenthesis matching
- Postfix to Infix Expressions
- Function calls order
8. What are different operations available in stack data structure?
Some of the main operations provided in the stack data structure are:
- push: This adds an item to the top of the stack. The overflow condition occurs if the stack is full.
- pop: This removes the top item of the stack. Underflow condition occurs if the stack is empty.
- top: This returns the top item from the stack.
- isEmpty: This returns true if the stack is empty else false.
- size: This returns the size of the stack.
9. What is a queue data structure? What are the applications of queue?
A queue is a linear data structure that allows users to store items in a list in a systematic manner. The items are added to the queue at the rear end until they are full, at which point they are removed from the queue from the front. Queues are commonly used in situations where the users want to hold items for a long period of time, such as during a checkout process. A good example of a queue is any queue of customers for a resource where the first consumer is served first.
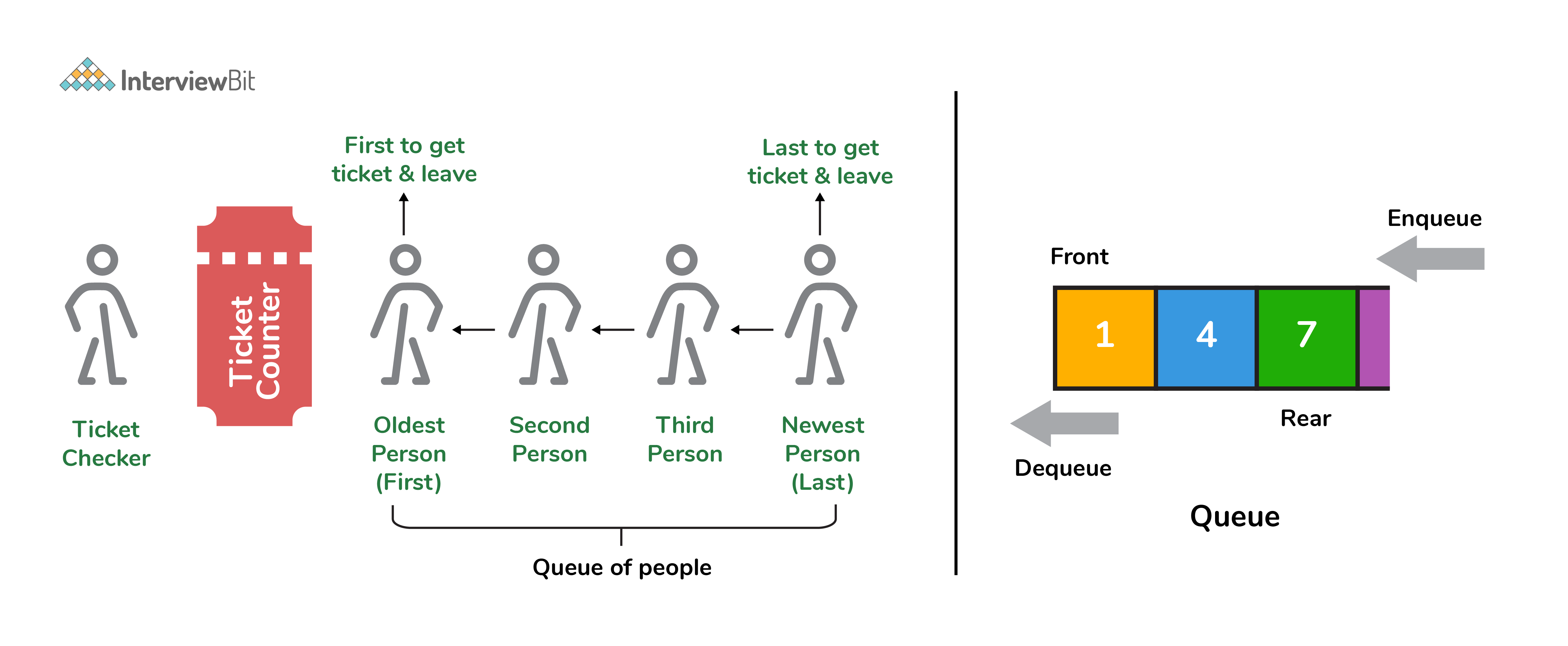
Following are some applications of queue data structure:
- Breadth-first search algorithm in graphs
- Operating system: job scheduling operations, Disk scheduling, CPU scheduling etc.
- Call management in call centres
10. What are different operations available in queue data structure?
- enqueue: This adds an element to the rear end of the queue. Overflow conditions occur if the queue is full.
- dequeue: This removes an element from the front end of the queue. Underflow conditions occur if the queue is empty.
- isEmpty: This returns true if the queue is empty or else false.
- rear: This returns the rear end element without removing it.
- front: This returns the front-end element without removing it.
- size: This returns the size of the queue.
11. Differentiate between stack and queue data structure.
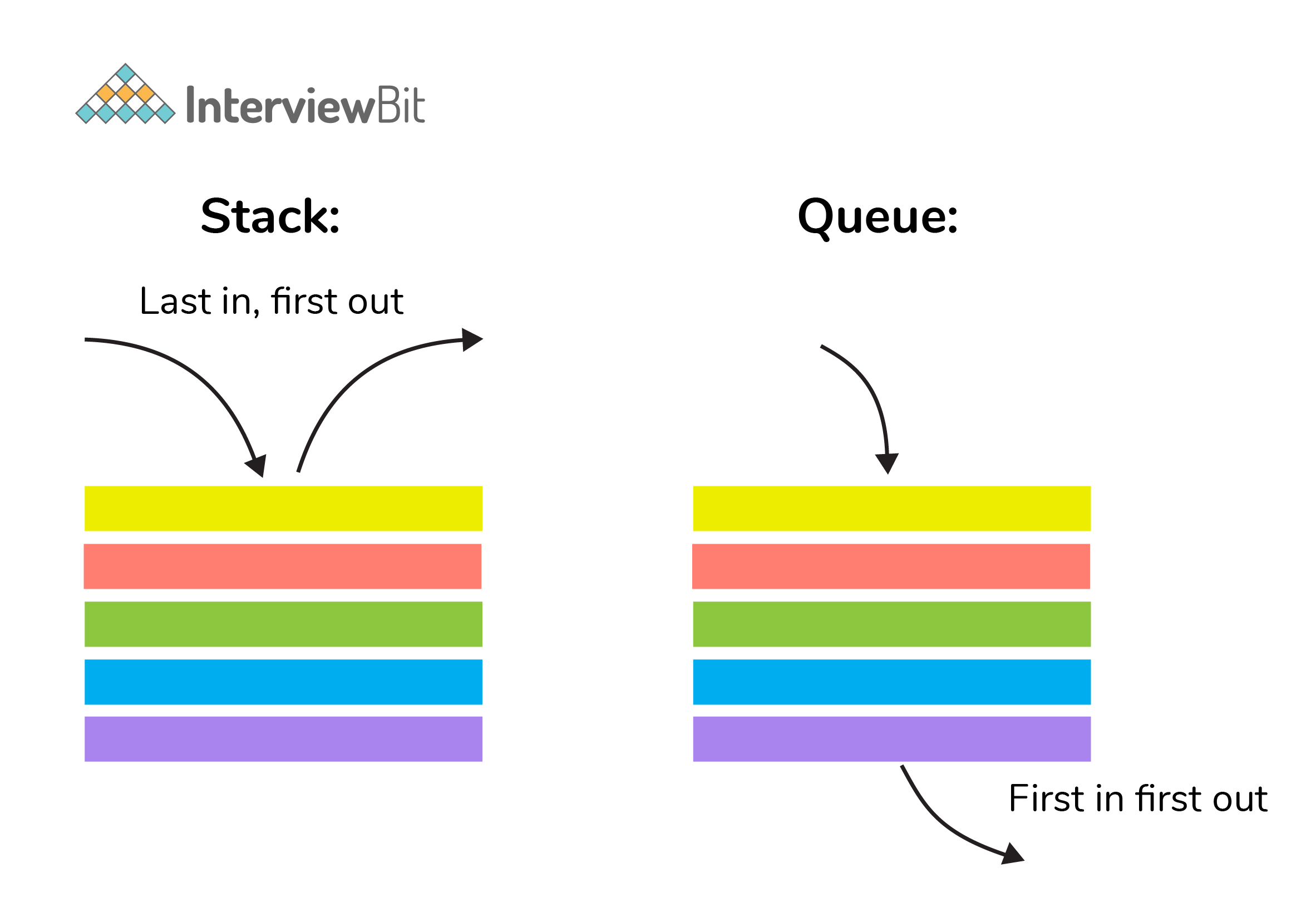
12. How to implement a queue using stack?
A queue can be implemented using two stacks . Let q be the queue and stack1 and stack2 be the 2 stacks for implementing q . We know that stack supports push, pop, and peek operations and using these operations, we need to emulate the operations of the queue - enqueue and dequeue. Hence, queue q can be implemented in two methods (Both the methods use auxillary space complexity of O(n)):
1. By making enqueue operation costly:
- Here, the oldest element is always at the top of stack1 which ensures dequeue operation occurs in O(1) time complexity.
- To place the element at top of stack1, stack2 is used.
- Enqueue: Here time complexity will be O(n)
- Dequeue: Here time complexity will be O(1)
2. By making the dequeue operation costly:
- Here, for enqueue operation, the new element is pushed at the top of stack1 . Here, the enqueue operation time complexity is O(1).
- In dequeue, if stack2 is empty, all elements from stack1 are moved to stack2 and top of stack2 is the result. Basically, reversing the list by pushing to a stack and returning the first enqueued element. This operation of pushing all elements to a new stack takes O(n) complexity.
- Enqueue: Time complexity: O(1)
- Dequeue: Time complexity: O(n)
13. How do you implement stack using queues?
- A stack can be implemented using two queues. We know that a queue supports enqueue and dequeue operations. Using these operations, we need to develop push, pop operations.
- Let stack be ‘s’ and queues used to implement be ‘q1’ and ‘q2’. Then, stack ‘s’ can be implemented in two ways:
1. By making push operation costly:
- This method ensures that the newly entered element is always at the front of ‘q1’ so that pop operation just dequeues from ‘q1’.
- ‘q2’ is used as auxillary queue to put every new element in front of ‘q1’ while ensuring pop happens in O(1) complexity.
- Push element to stack s: Here push takes O(n) time complexity.
- Pop element from stack s: Takes O(1) time complexity.
2. By making pop operation costly:
- In push operation, the element is enqueued to q1.
- In pop operation, all the elements from q1 except the last remaining element, are pushed to q2 if it is empty. That last element remaining of q1 is dequeued and returned.
- Push element to stack s: Here push takes O(1) time complexity.
- Pop element from stack s: Takes O(n) time complexity.
14. What is array data structure? What are the applications of arrays?
An array data structure is a data structure that is used to store data in a way that is efficient and easy to access. It is similar to a list in that it stores data in a sequence. However, an array data structure differs from a list in that it can hold much more data than a list can. An array data structure is created by combining several arrays together. Each array is then given a unique identifier, and each array’s data is stored in the order in which they are created.
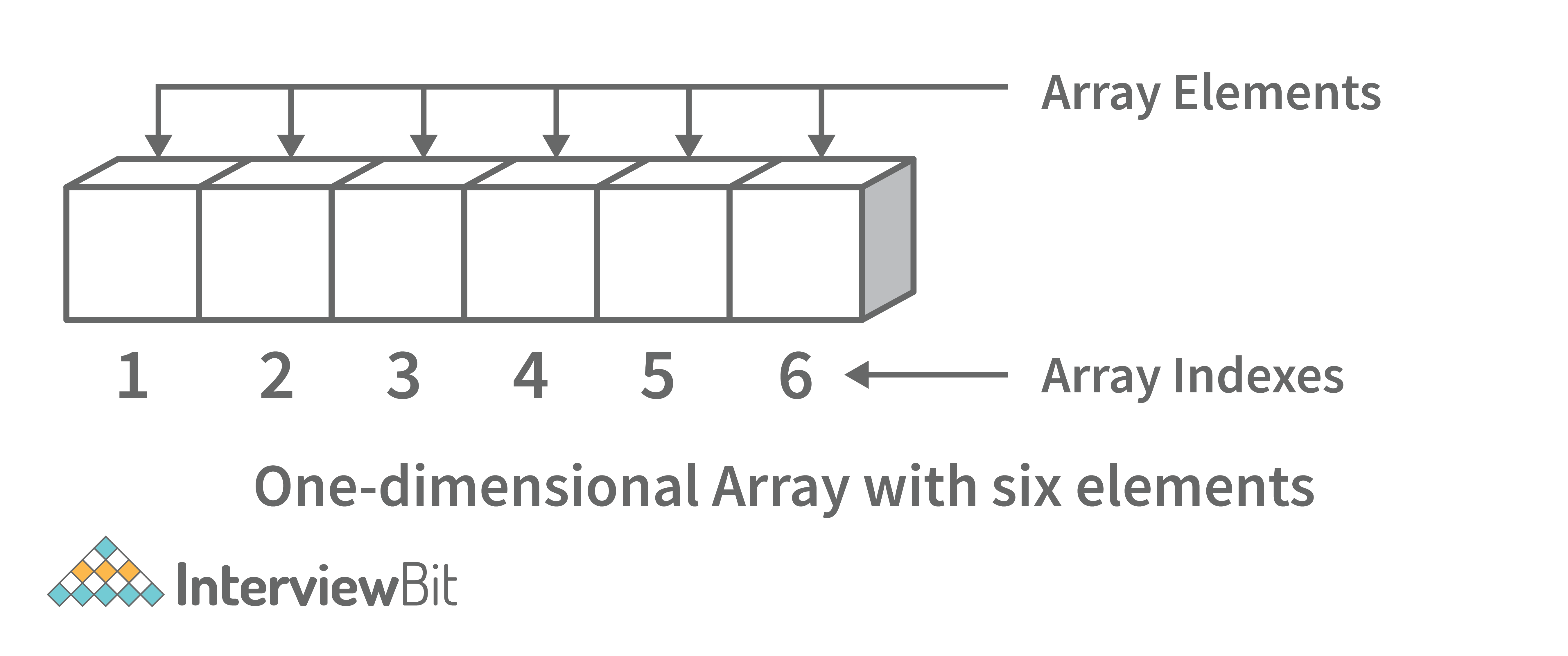
Array data structures are commonly used in databases and other computer systems to store large amounts of data efficiently. They are also useful for storing information that is frequently accessed, such as large amounts of text or images.
15. Elaborate on different types of array data structure
There are several different types of arrays:
- One-dimensional array: A one-dimensional array stores its elements in contiguous memory locations, accessing them using a single index value. It is a linear data structure holding all the elements in a sequence.

- Two-dimensional array: A two-dimensional array is a tabular array that includes rows and columns and stores data. An M × N two-dimensional array is created by grouping M rows and N columns into N columns and rows.
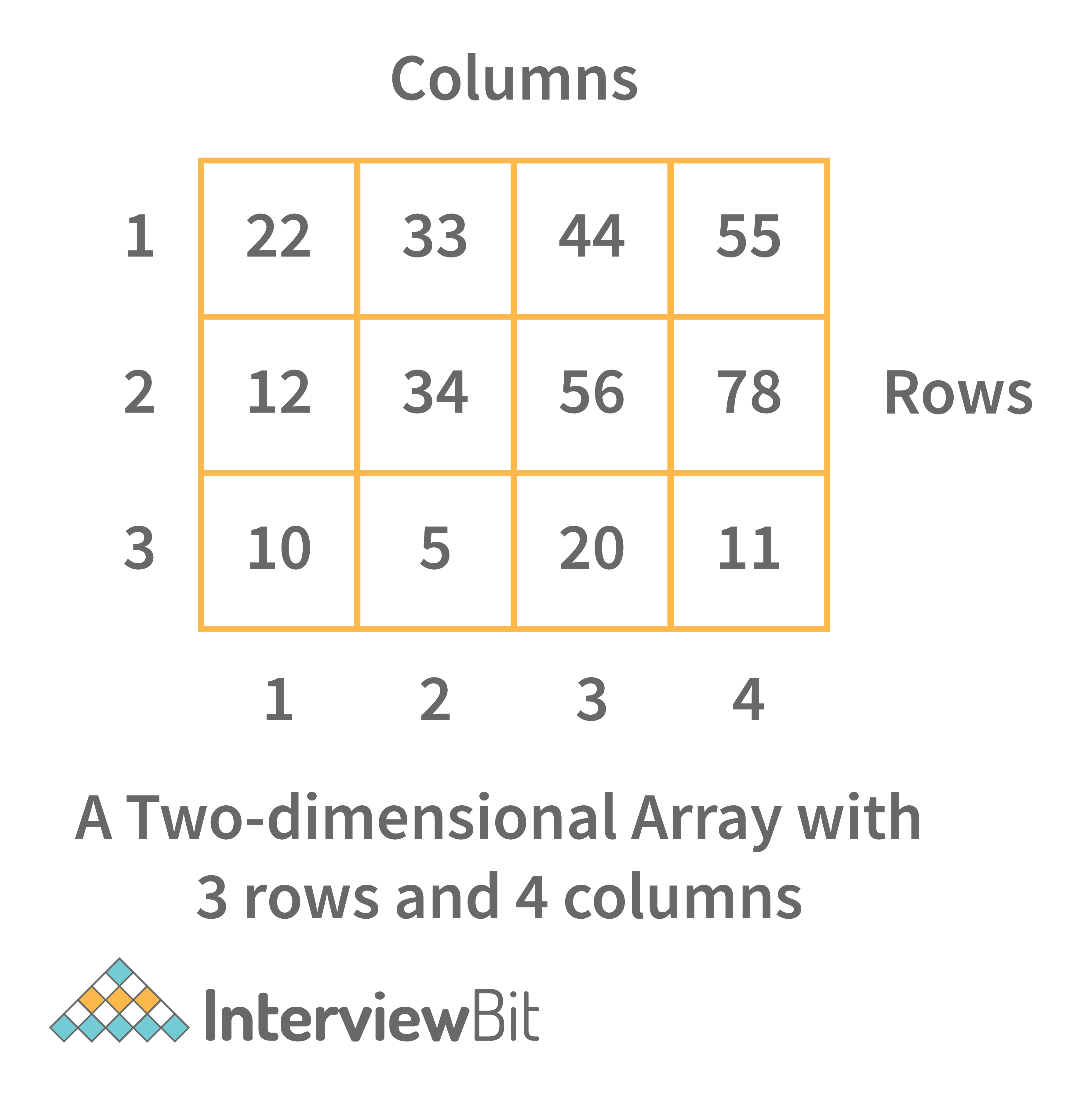
- Three-dimensional array: A three-dimensional array is a grid that has rows, columns, and depth as a third dimension. It comprises a cube with rows, columns, and depth as a third dimension. The three-dimensional array has three subscripts for a position in a particular row, column, and depth. Depth (dimension or layer) is the first index, row index is the second index, and column index is the third index.
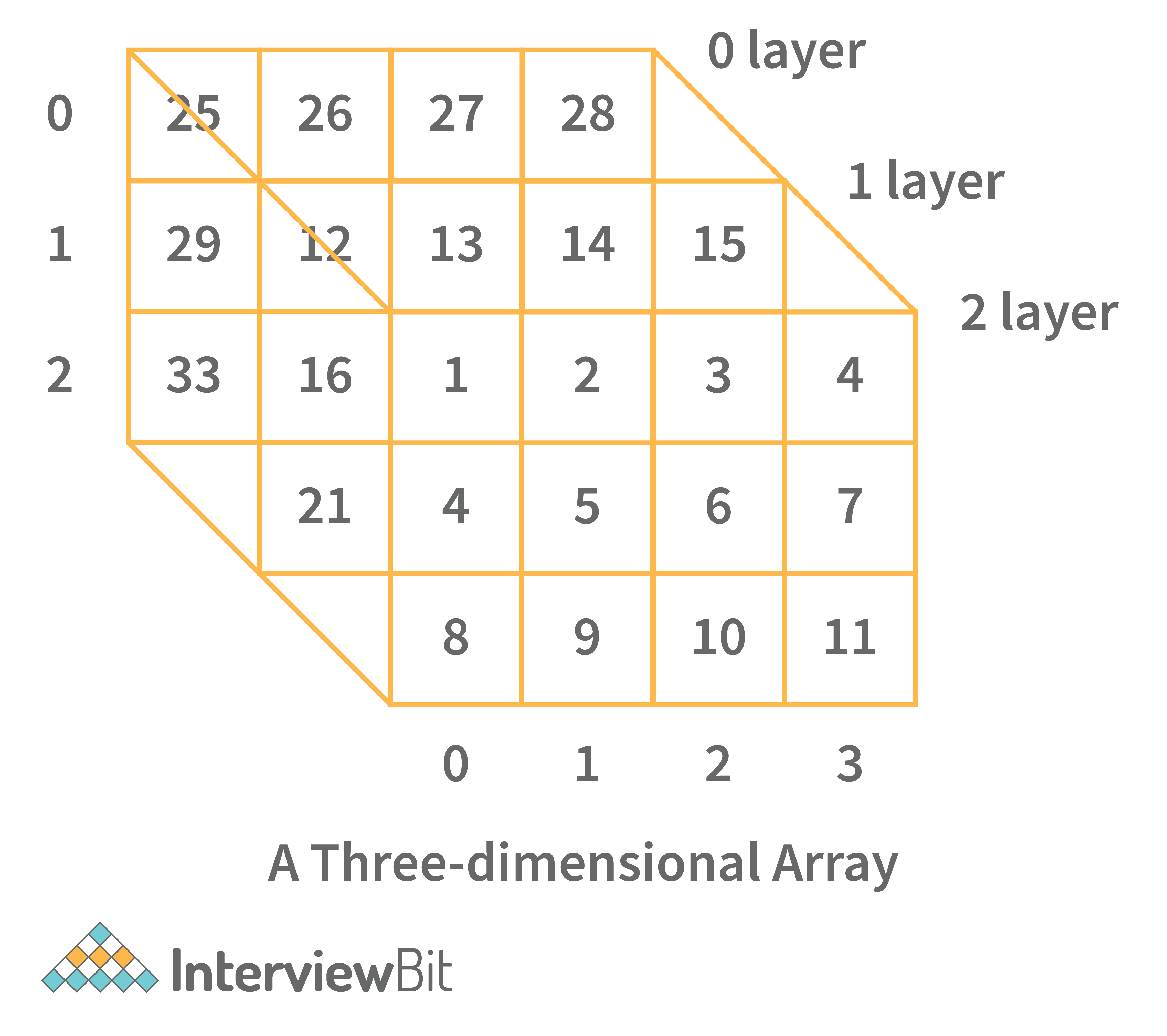
16. What is a linked list data structure? What are the applications for the Linked list?
A linked list can be thought of as a series of linked nodes (or items) that are connected by links (or paths). Each link represents an entry into the linked list, and each entry points to the next node in the sequence. The order in which nodes are added to the list is determined by the order in which they are created.
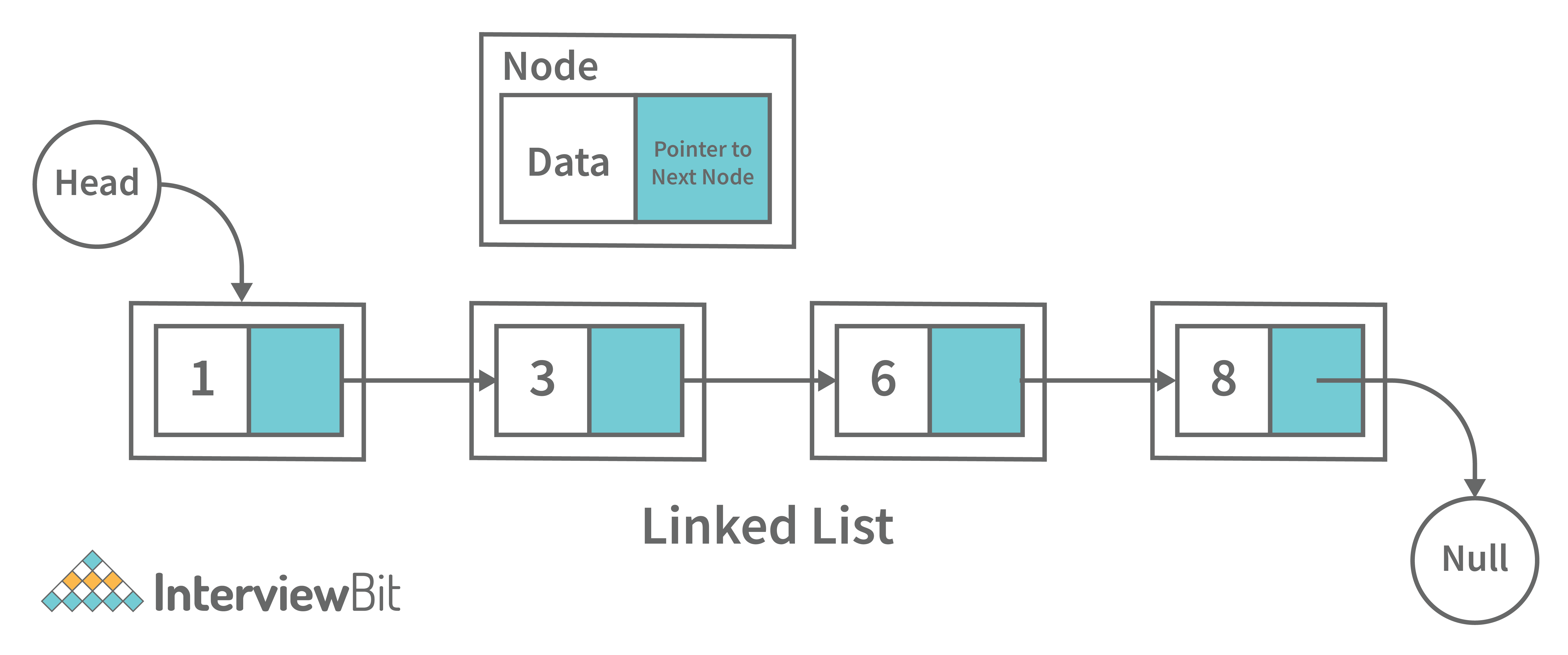
Following are some applications of linked list data structure:
- Stack, Queue, binary trees, and graphs are implemented using linked lists.
- Dynamic management for Operating System memory.
- Round robin scheduling for operating system tasks.
- Forward and backward operation in the browser.
17. Elaborate on different types of Linked List data structures?
Following are different types of linked lists:
1. Singly Linked List: A singly linked list is a data structure that is used to store multiple items. The items are linked together using the key. The key is used to identify the item and is usually a unique identifier. In a singly linked list, each item is stored in a separate node. The node can be a single object or it can be a collection of objects. When an item is added to the list, the node is updated and the new item is added to the end of the list. When an item is removed from the list, the node that contains the removed item is deleted and its place is taken by another node. The key of a singly linked list can be any type of data structure that can be used to identify an object. For example, it could be an integer, a string, or even another singly linked list. Singly-linked lists are useful for storing many different types of data. For example, they are commonly used to store lists of items such as grocery lists or patient records. They are also useful for storing data that is time sensitive such as stock market prices or flight schedules.
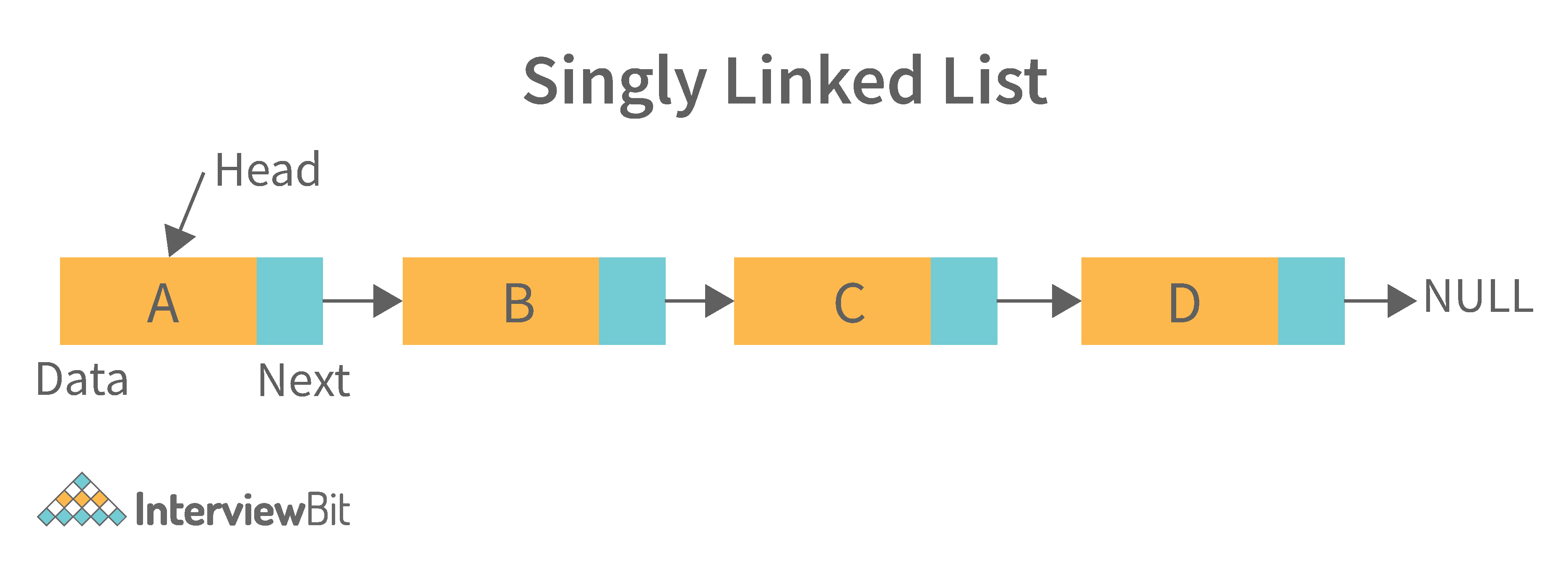
2. Doubly Linked List: A doubly linked list is a data structure that allows for two-way data access such that each node in the list points to the next node in the list and also points back to its previous node. In a doubly linked list, each node can be accessed by its address, and the contents of the node can be accessed by its index. It's ideal for applications that need to access large amounts of data in a fast manner. A disadvantage of a doubly linked list is that it is more difficult to maintain than a single-linked list. In addition, it is more difficult to add and remove nodes than in a single-linked list.

3. Circular Linked List: A circular linked list is a unidirectional linked list where each node points to its next node and the last node points back to the first node, which makes it circular.
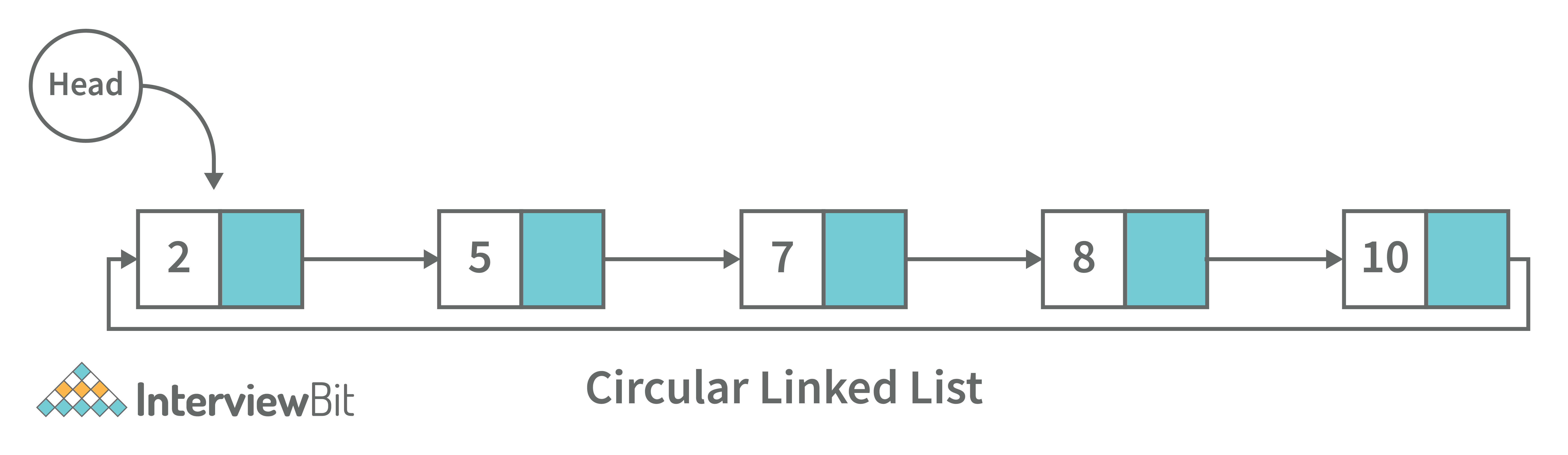
4. Doubly Circular Linked List: A doubly circular linked list is a linked list where each node points to its next node and its previous node and the last node points back to the first node and first node’s previous points to the last node.
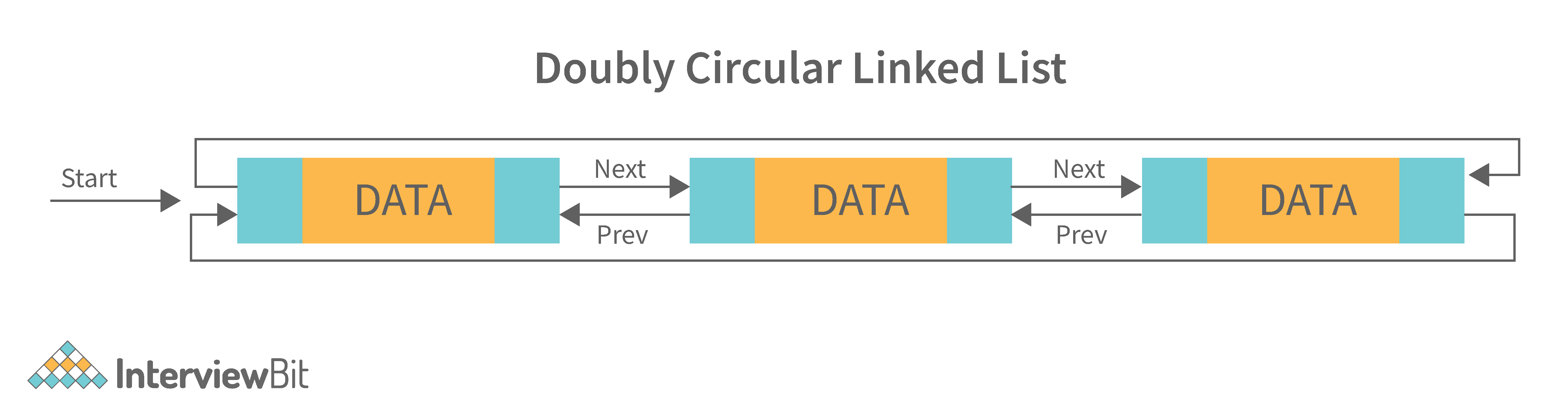
5. Header List: A list that contains the header node at the beginning of the list, is called the header-linked list. This is helpful in calculating some repetitive operations like the number of elements in the list etc.
18. Difference between Array and Linked List.
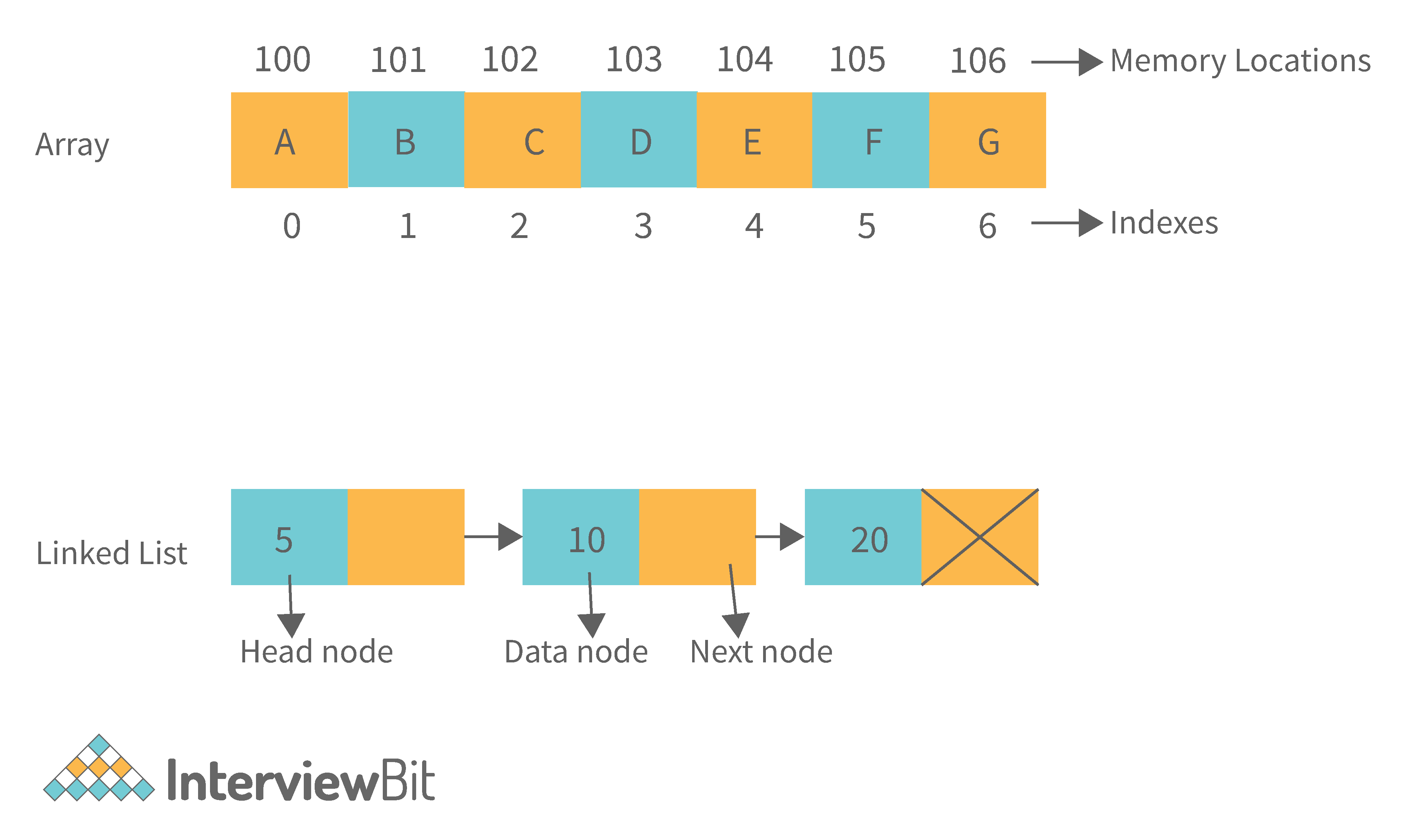
19. What is an asymptotic analysis of an algorithm?
Asymptotic analysis of an algorithm defines the run-time performance as per its mathematical boundations. Asymptotic analysis helps us articulate the best case(Omega Notation, Ω), average case(Theta Notation, θ), and worst case(Big Oh Notation, Ο) performance of an algorithm.
20. What is hashmap in data structure?
Hashmap is a data structure that uses an implementation of a hash table data structure which allows access to data in constant time (O(1)) complexity if you have the key.
21. What is the requirement for an object to be used as key or value in HashMap?
- The key or value object that gets used in the hashmap must implement equals() and hashcode() method.
- The hash code is used when inserting the key object into the map and the equals method is used when trying to retrieve a value from the map.
22. How does HashMap handle collisions in Java?
- The java.util.HashMap class in Java uses the approach of chaining to handle collisions. In chaining, if the new values with the same key are attempted to be pushed, then these values are stored in a linked list stored in a bucket of the key as a chain along with the existing value.
- In the worst-case scenario, it can happen that all keys might have the same hashcode, which will result in the hash table turning into a linked list. In this case, searching a value will take O(n) complexity as opposed to O(1) time due to the nature of the linked list. Hence, care has to be taken while selecting hashing algorithm.
23. What is the time complexity of basic operations get() and put() in HashMap class?
The time complexity is O(1) assuming that the hash function used in the hash map distributes elements uniformly among the buckets.
1. What is binary tree data structure? What are the applications for binary trees?
A binary tree is a data structure that is used to organize data in a way that allows for efficient retrieval and manipulation. It is a data structure that uses two nodes, called leaves and nodes, to represent the data. The leaves represent the data and the nodes represent the relationships between the leaves. Each node has two children, called siblings, and each child has one parent. The parent is the node that is closest to the root of the tree. When a node is deleted from the tree, it is deleted from both its child and its parent.
Following are some applications for binary tree data structure:
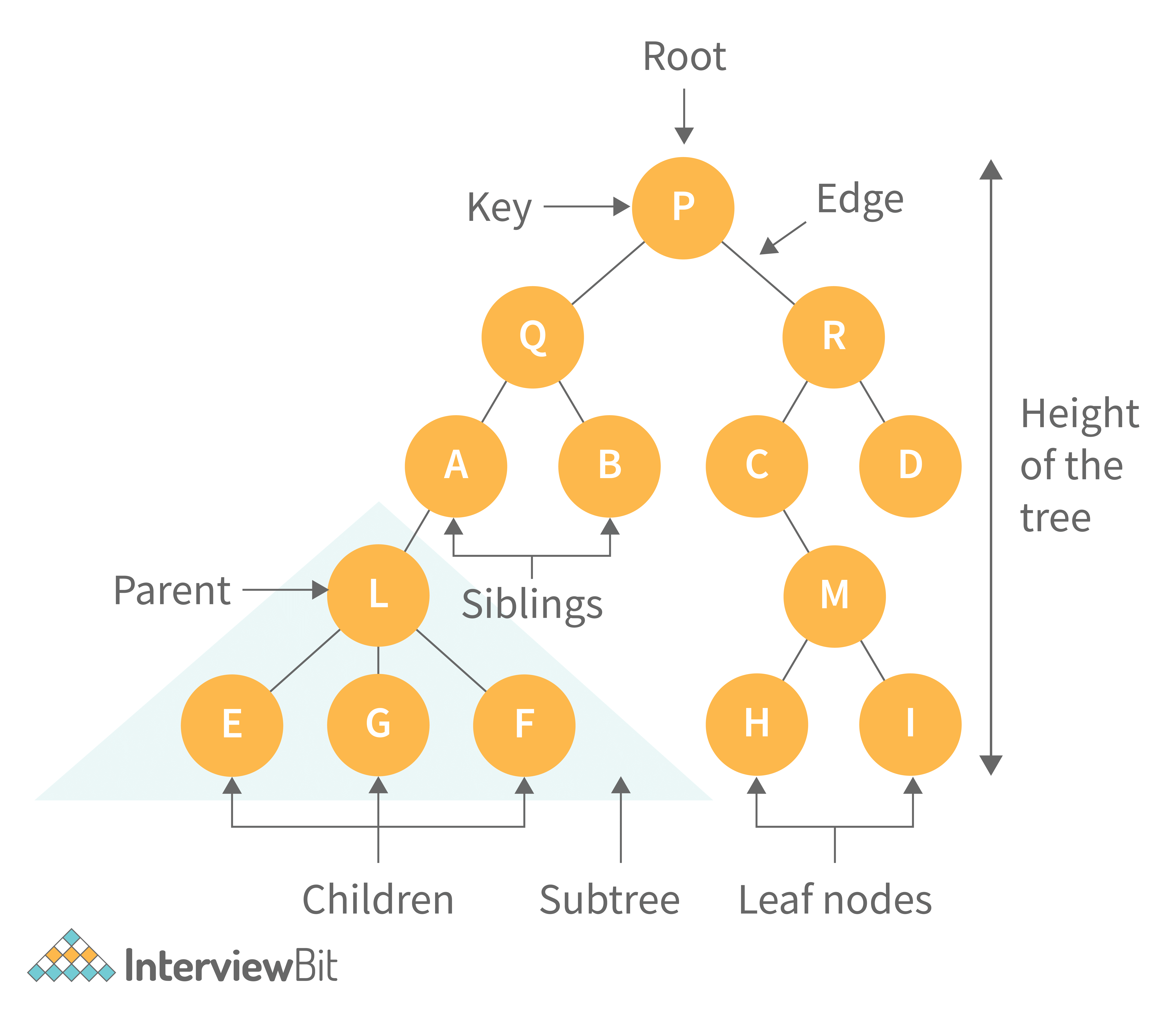
- It's widely used in computer networks for storing routing table information.
- Decision Trees.
- Expression Evaluation.
- Database indices.
2. What is binary search tree data structure? What are the applications for binary search trees?
A binary search tree is a data structure that stores items in sorted order. In a binary search tree, each node stores a key and a value. The key is used to access the item and the value is used to determine whether the item is present or not. The key can be any type of value such as an integer, floating point number, character string, or even a combination of these types. The value can be any type of items such as an integer, floating point number, character string, or even a combination of these types. When a node is added to the tree, its key is used to access the item stored at that node. When a node is removed from the tree, its key is used to access the item stored at that node.
A binary search tree is a special type of binary tree that has a specific order of elements in it. It has three basic qualities:
- All elements in the left subtree of a node should have a value less than or equal to the parent node's value, and
- All elements in the right subtree of a node should have a value greater than or equal to the parent node's value.
- Both the left and right subtrees must be binary search trees too.
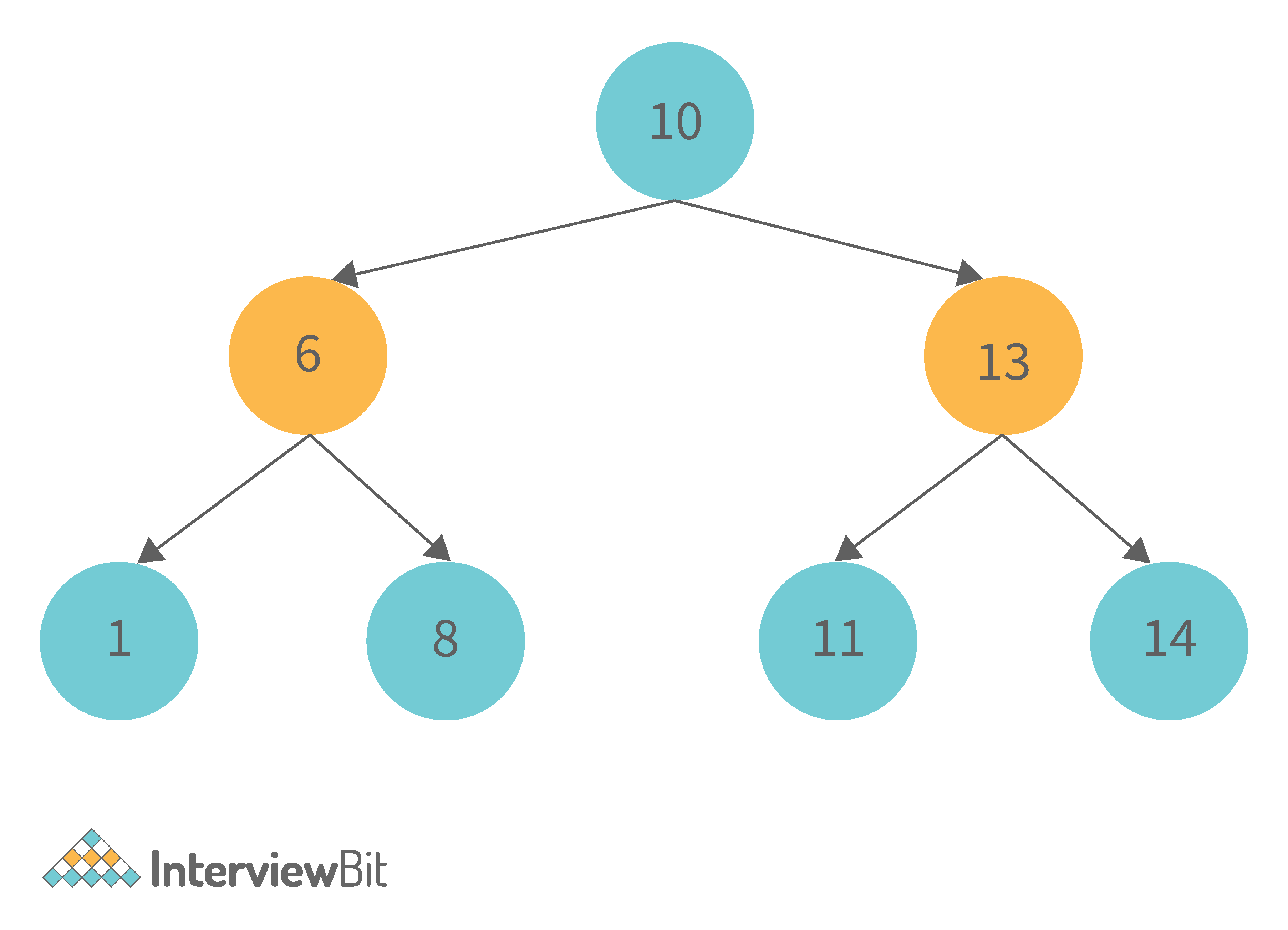
- It is used for indexing and multi-level indexing.
- It is used for implementing various search algorithms.
- It is helpful in organizing a sorted stream of data.
3. What are tree traversals?
Tree traversal is the process of visiting all the nodes of a tree. Since the root (head) is the first node and all nodes are connected via edges (or links) we always start with that node. There are three ways which we use to traverse a tree −
1. Inorder Traversal:
- Step 1. Traverse the left subtree, i.e., call Inorder(root.left)
- Step 2. Visit the root.
- Step 3. Traverse the right subtree, i.e., call Inorder(root.right)
- Inorder traversal in Java:
- Uses: In binary search trees (BST), inorder traversal gives nodes in ascending order.
2. Preorder Traversal:
- Step 1. Visit the root.
- Step 2. Traverse the left subtree, i.e., call Preorder(root.left)
- Step 3. Traverse the right subtree, i.e., call Preorder(root.right)
- Preorder traversal in Java:
- Preorder traversal is commonly used to create a copy of the tree.
- It is also used to get prefix expression of an expression tree.
3. Postorder Traversal:
- Step 1. Traverse the left subtree, i.e., call Postorder(root.left)
- Step 2. Traverse the right subtree, i.e., call Postorder(root.right)
- Step 3. Visit the root.
- Postorder traversal in Java:
- Postorder traversal is commonly used to delete the tree.
- It is also useful to get the postfix expression of an expression tree.
Consider the following tree as an example, then:
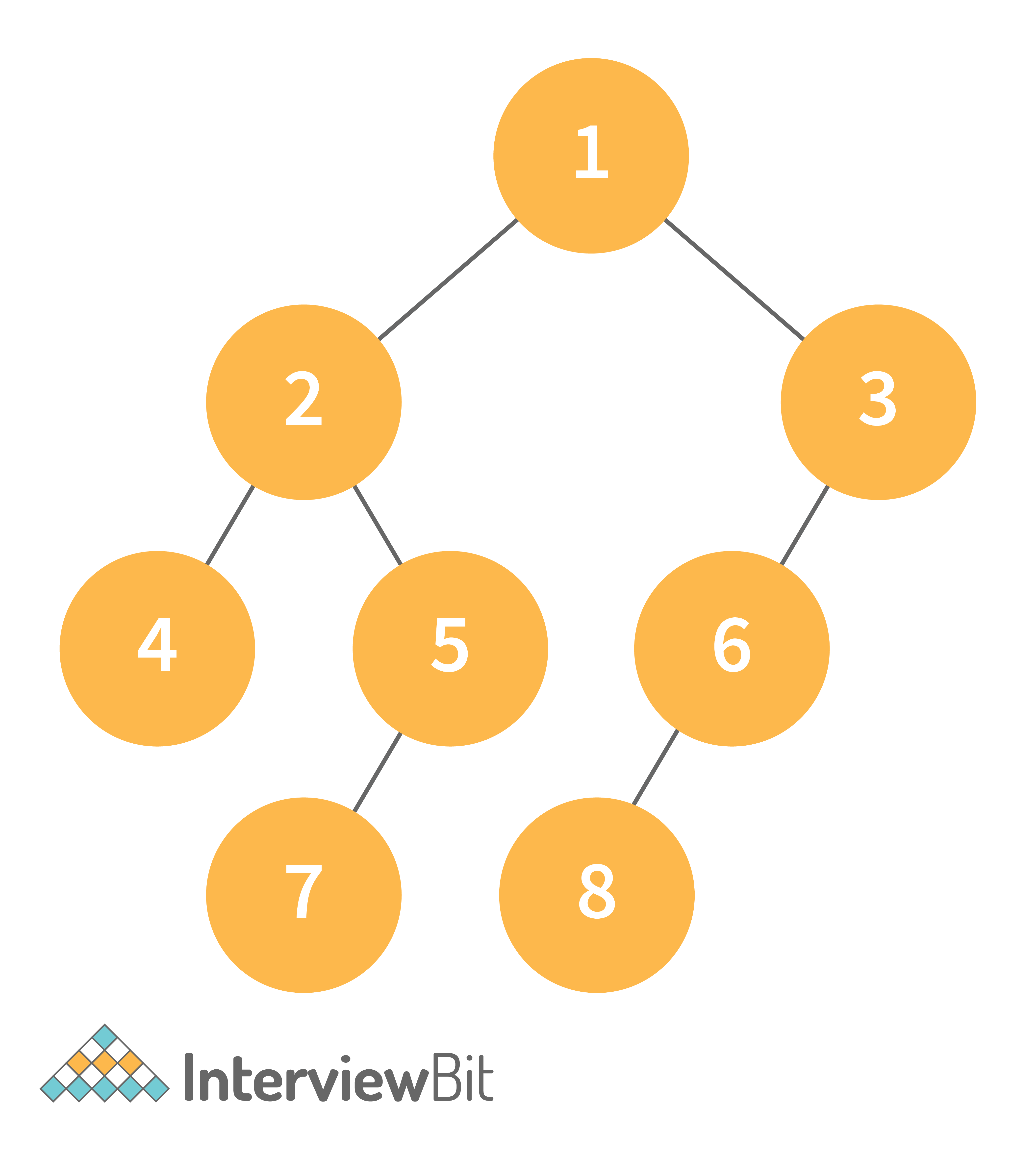
- Inorder Traversal => Left, Root, Right : [4, 2, 5, 1, 3]
- Preorder Traversal => Root, Left, Right : [1, 2, 4, 5, 3]
- Postorder Traversal => Left, Right, Root : [4, 5, 2, 3, 1]
4. What is a deque data structure and its types? What are the applications for deque?
A deque can be thought of as an array of items, but with one important difference: Instead of pushing and popping items off the end to make room, deques are designed to allow items to be inserted at either end. This property makes deques well-suited for performing tasks such as keeping track of inventory, scheduling tasks, or handling large amounts of data.
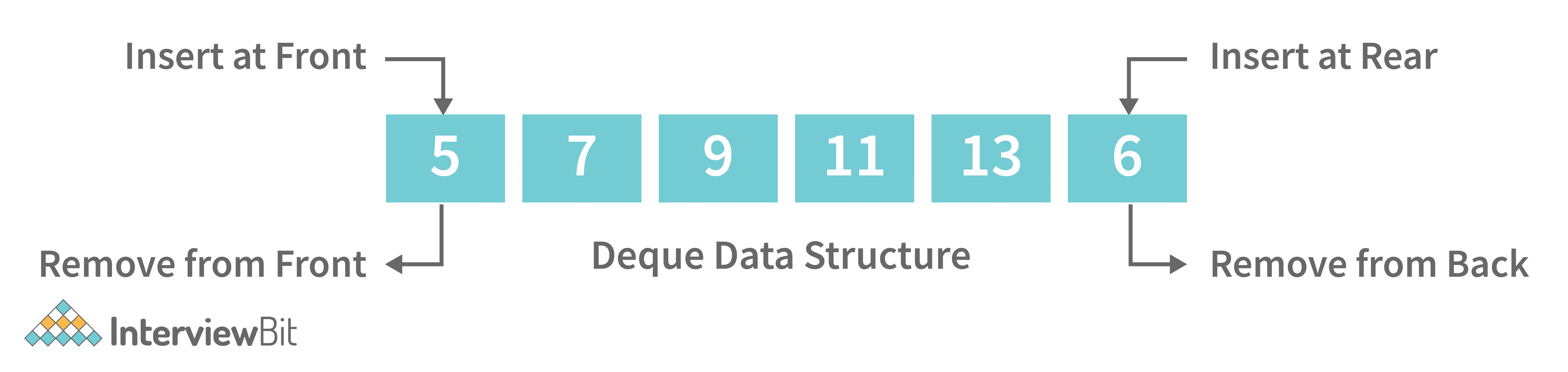
There are two types of deque:
- Input Restricted Deque: Insertion operations are performed at only one end while deletion is performed at both ends in the input restricted queue.
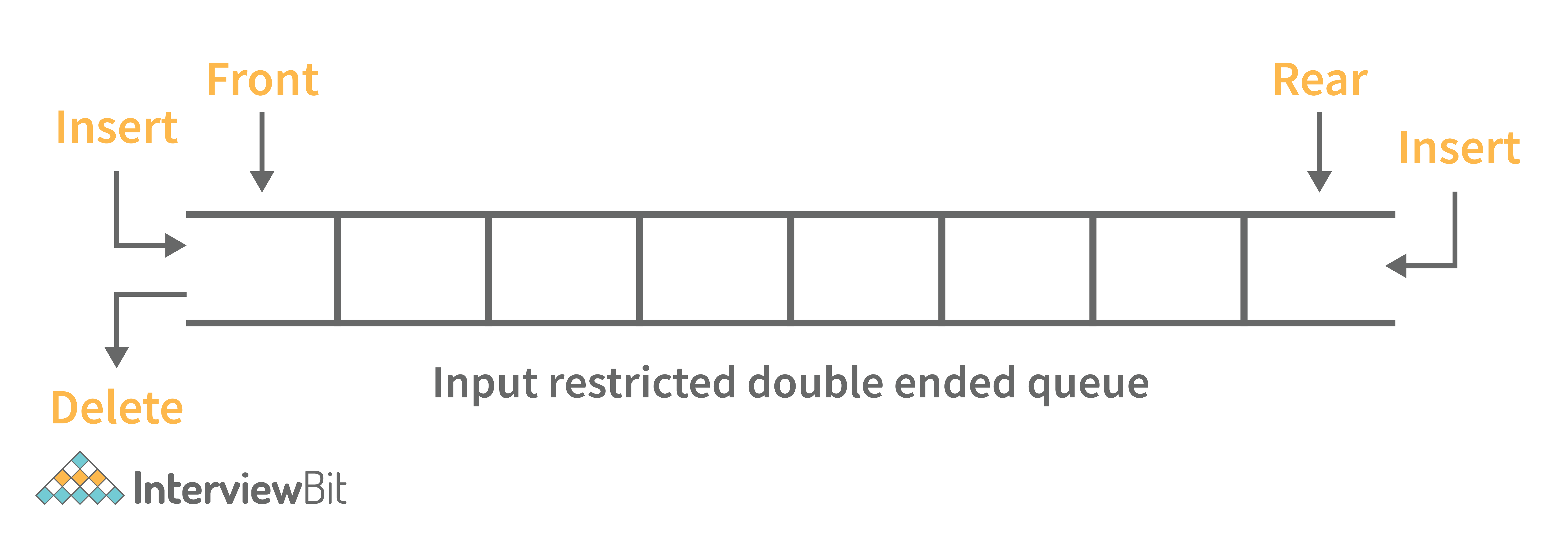
- Output Restricted Deque: Deletion operations are performed at only one end while insertion is performed at both ends in the output restricted queue.
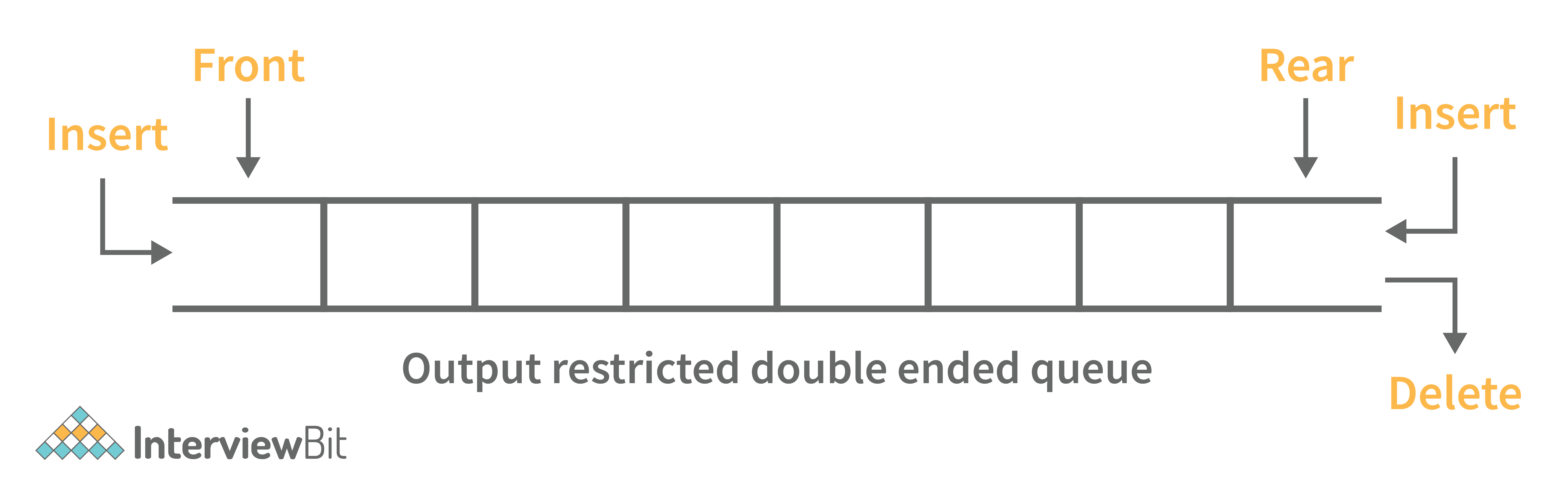
Following are some real-time applications for deque data structure:
- It can be used as both stack and queue, as it supports all the operations for both data structures.
- Web browser’s history can be stored in a deque.
- Operating systems job scheduling algorithm.
5. What are some key operations performed on the Deque data structure?
Following are the key operations available deque:
- insertFront() : This adds an element to the front of the Deque.
- insertLast() : This adds an element to the rear of the Deque.
- deleteFront() : This deletes an element from the front of the Deque.
- deleteLast() :This deletes an element from the front of the Deque.
- getFront() : This gets an element from the front of the Deque.
- getRear() : This gets an element from the rear of the Deque.
- isEmpty() : This checks whether Deque is empty or not.
- isFull() : This checks whether Deque is full or not.
6. What is a priority queue? What are the applications for priority queue?
Priority Queue is an abstract data type that is similar to a queue in that each element is assigned a priority value. The order in which elements in a priority queue are served is determined by their priority (i.e., the order in which they are removed). If the elements have the same priority, they are served in the order they appear in the queue.
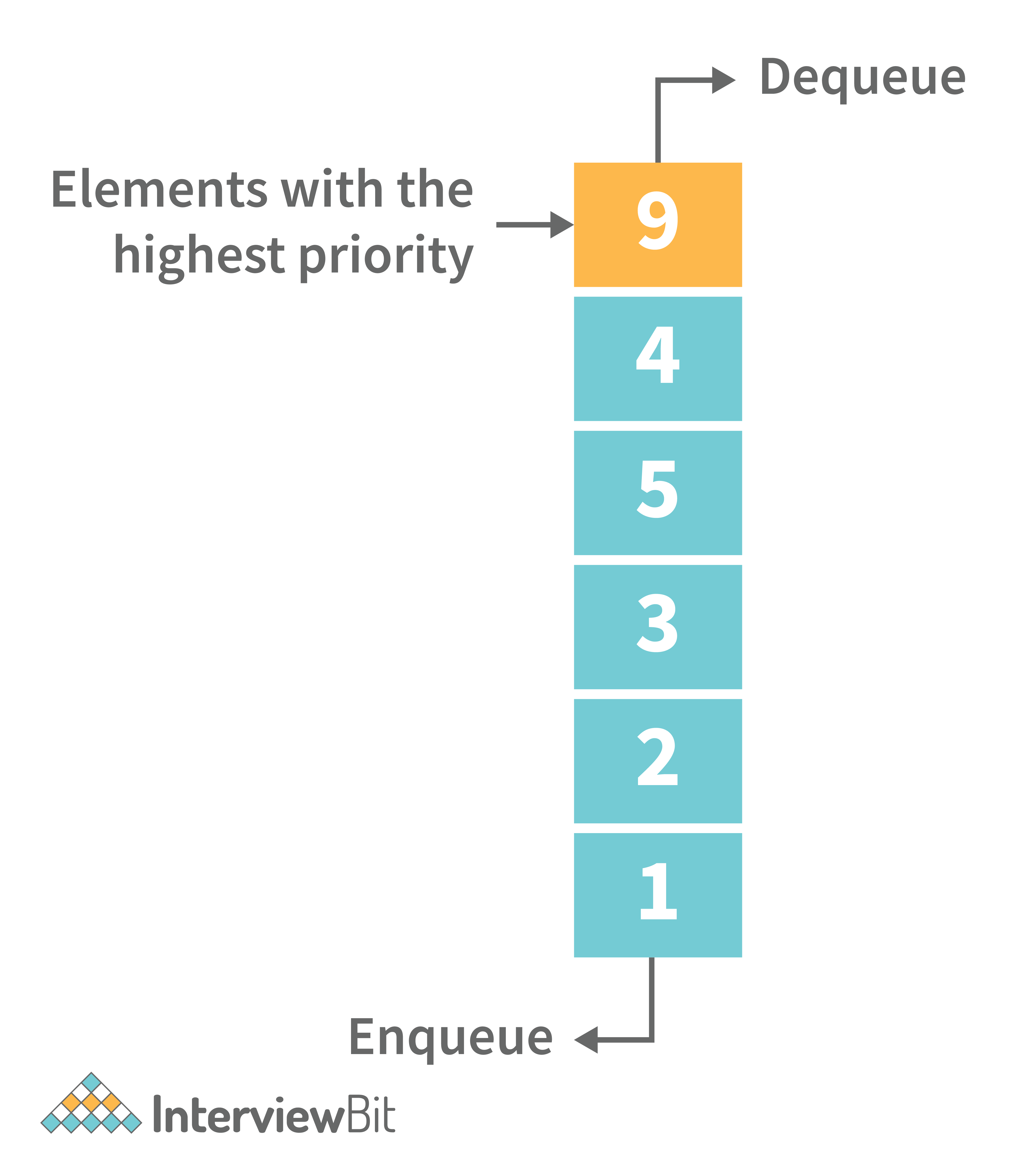
Following are some real-time applications for priority queue:
- Used in graph algorithms like Dijkstra, Prim’s Minimum spanning tree etc.
- Huffman code for data compression
- Finding Kth Largest/Smallest element
7. Compare different implementations of priority queue
The following table contains an asymptotic analysis of different implementations of a priority queue:
8. What is graph data structure and its representations? What are the applications for graphs?
A graph is a type of non-linear data structure made up of nodes and edges. The nodes are also known as vertices, and edges are lines or arcs that connect any two nodes in the graph.
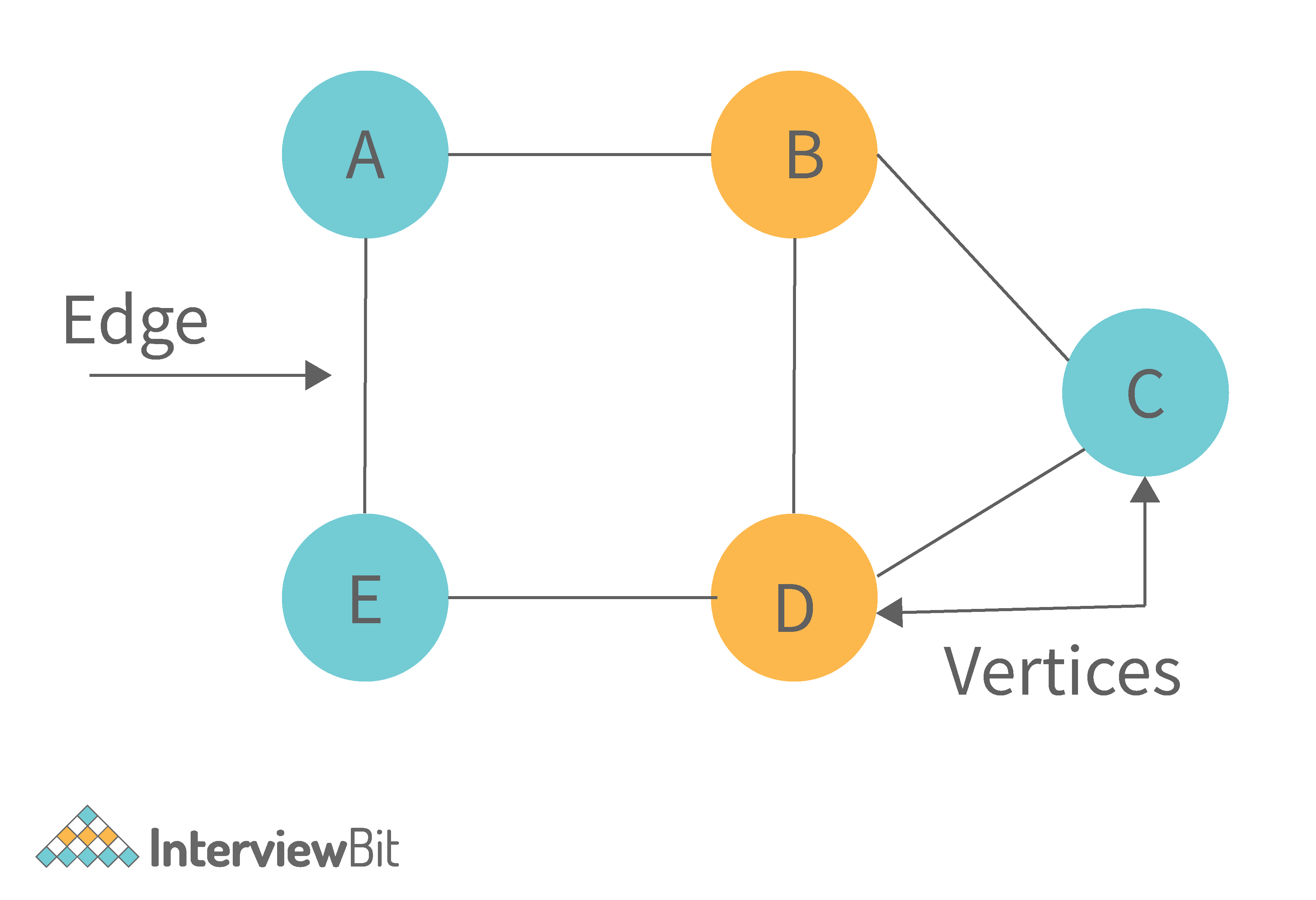
The following are the two most common graph representations:
1. Adjacency Matrix : Adjacency Matrix is a two-dimensional array with the dimensions V x V, where V is the number of vertices in a graph. Representation is simpler to implement and adhere to. It takes O(1) time to remove an edge. Queries such as whether there is an edge from vertex 'u' to vertex 'v' are efficient and can be completed in O(1).
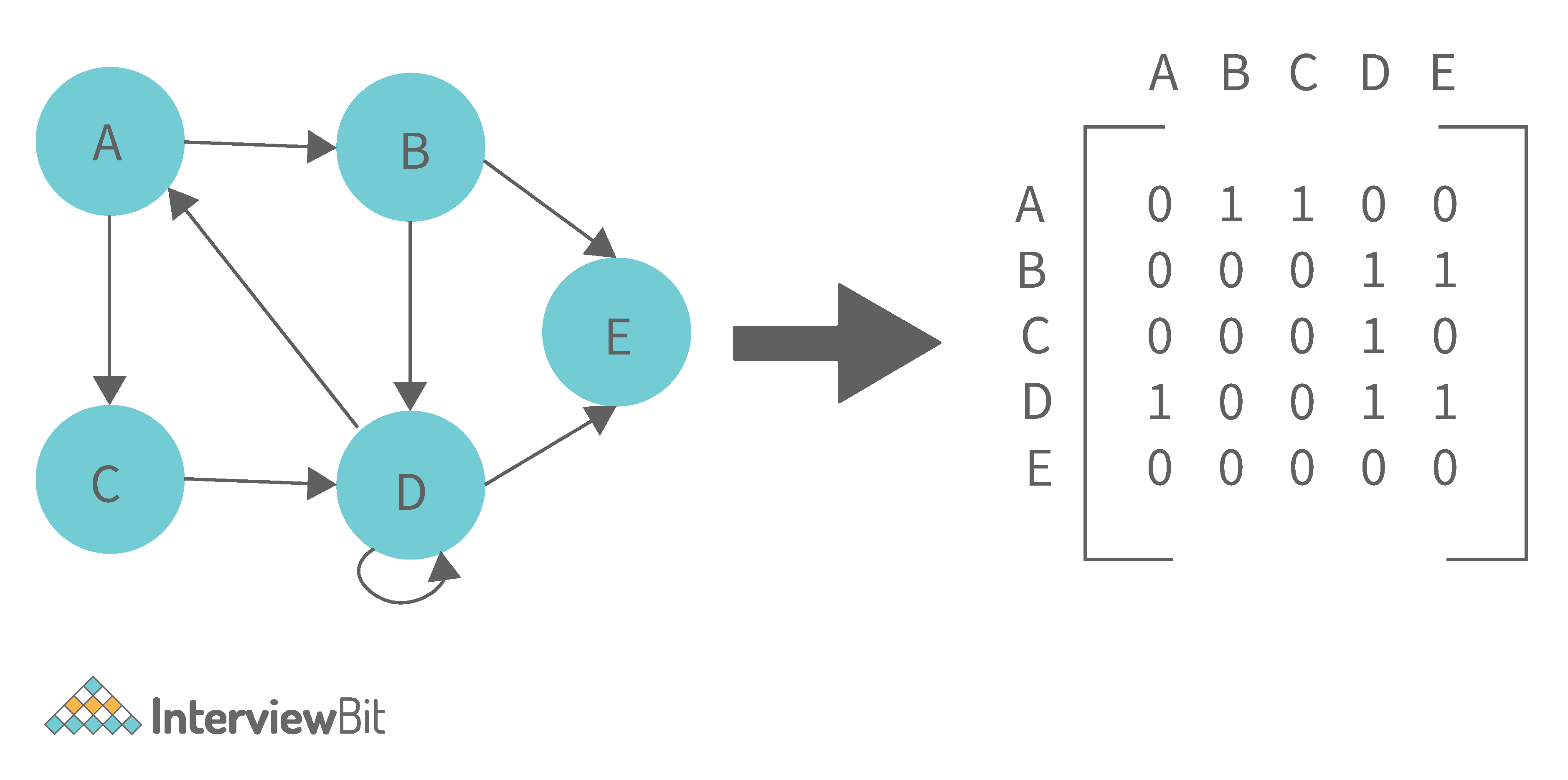
One of the cons of this representation is that even if the graph is sparse (has fewer edges), it takes up the same amount of space. Adding a vertex takes O(V^2). It also takes O(V) time to compute all of a vertex's neighbours, which is not very efficient.
2. Adjacency List: In this method, each Node holds a list of Nodes that are directly connected to that vertex. Each node at the end of the list is connected with null values to indicate that it is the last node in the list. This saves space O(|V|+|E|). In the worst-case scenario, a graph can have C(V, 2) edges, consuming O(V^2) space. It is simpler to add a vertex. It takes the least amount of time to compute all of a vertex's neighbours.
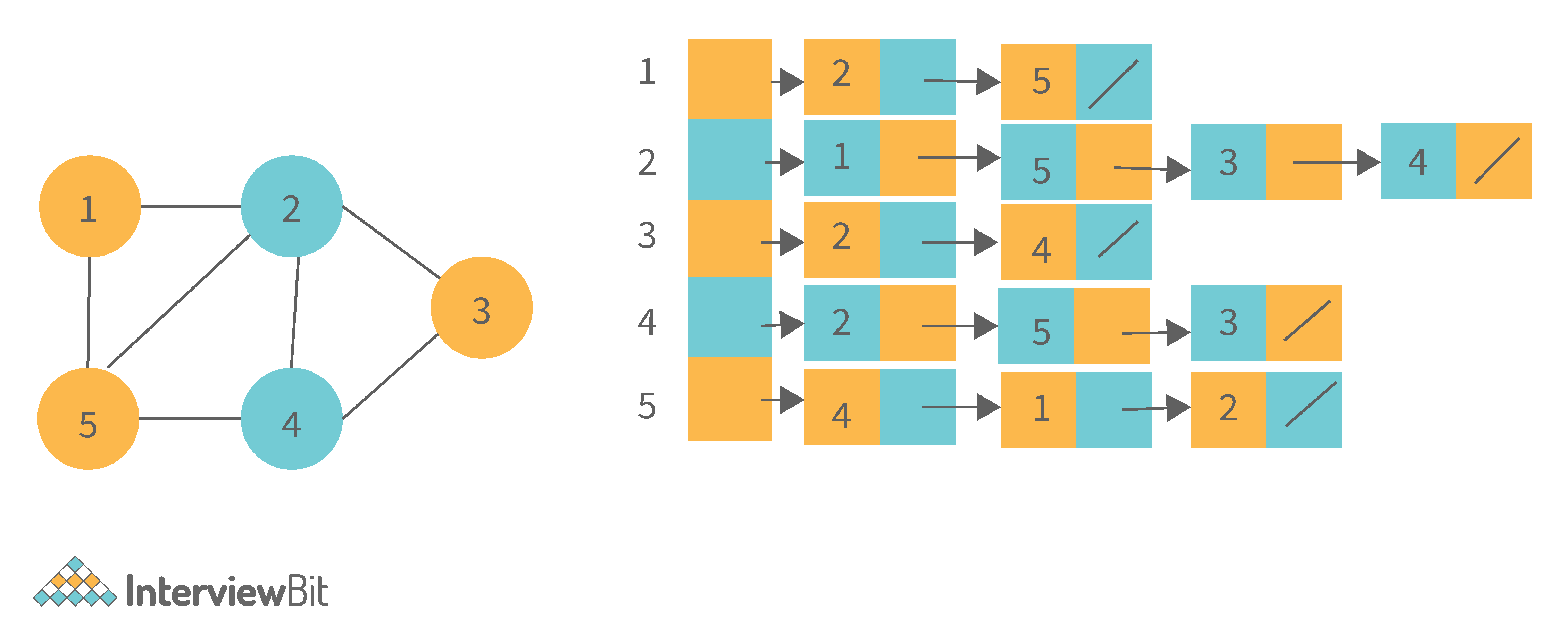
One of the cons of this representation is that queries such as "is there an edge from vertex u to vertex v?" are inefficient and take O (V) in the worst case.
9. What is the difference between the Breadth First Search (BFS) and Depth First Search (DFS)?
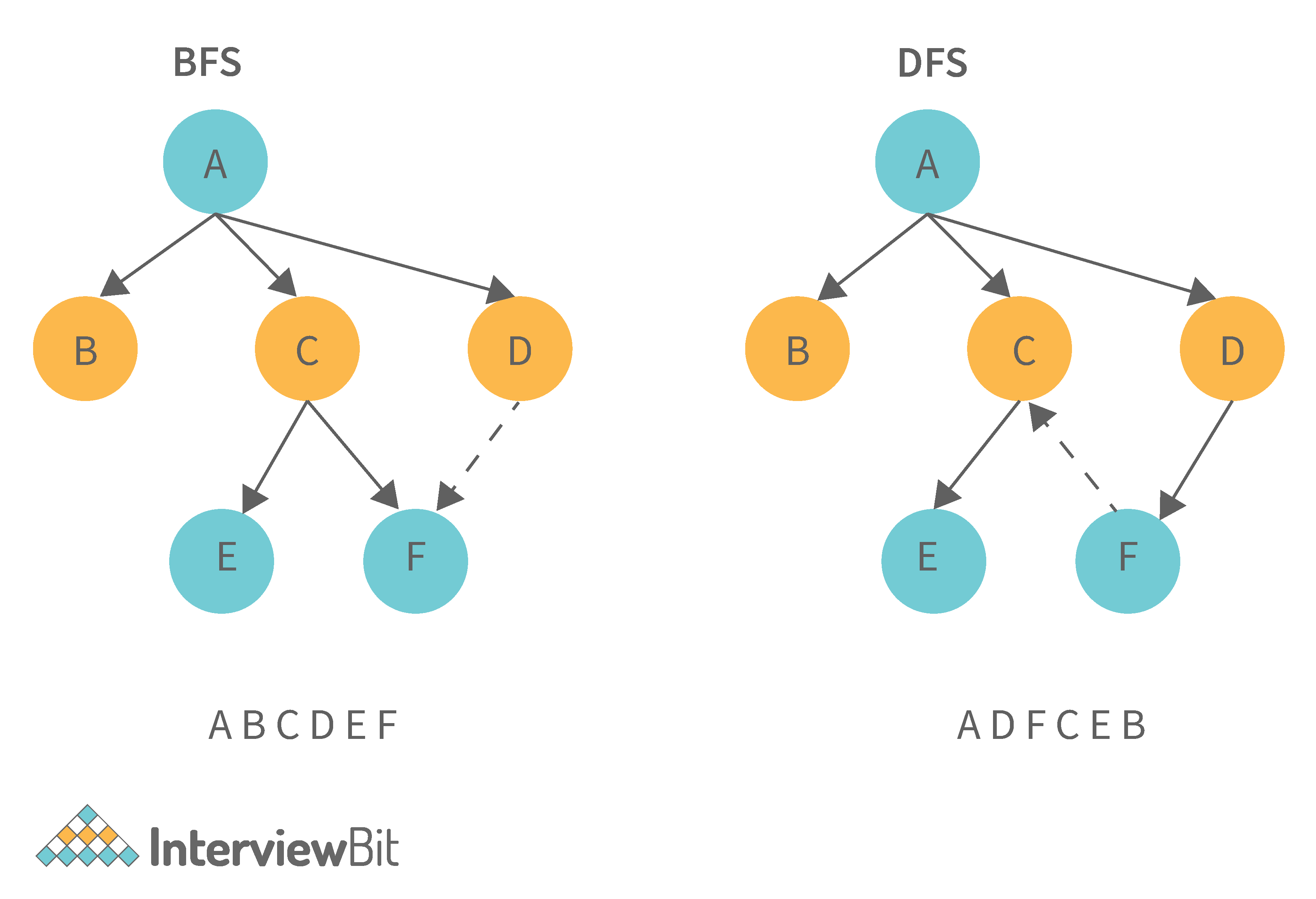
10. What is AVL tree data structure, its operations, and its rotations? What are the applications for AVL trees?
AVL trees are height balancing binary search trees named after their inventors Adelson, Velski, and Landis. The AVL tree compares the heights of the left and right subtrees and ensures that the difference is less than one. This distinction is known as the Balance Factor.
BalanceFactor = height(left-subtree) − height(right-subtree)
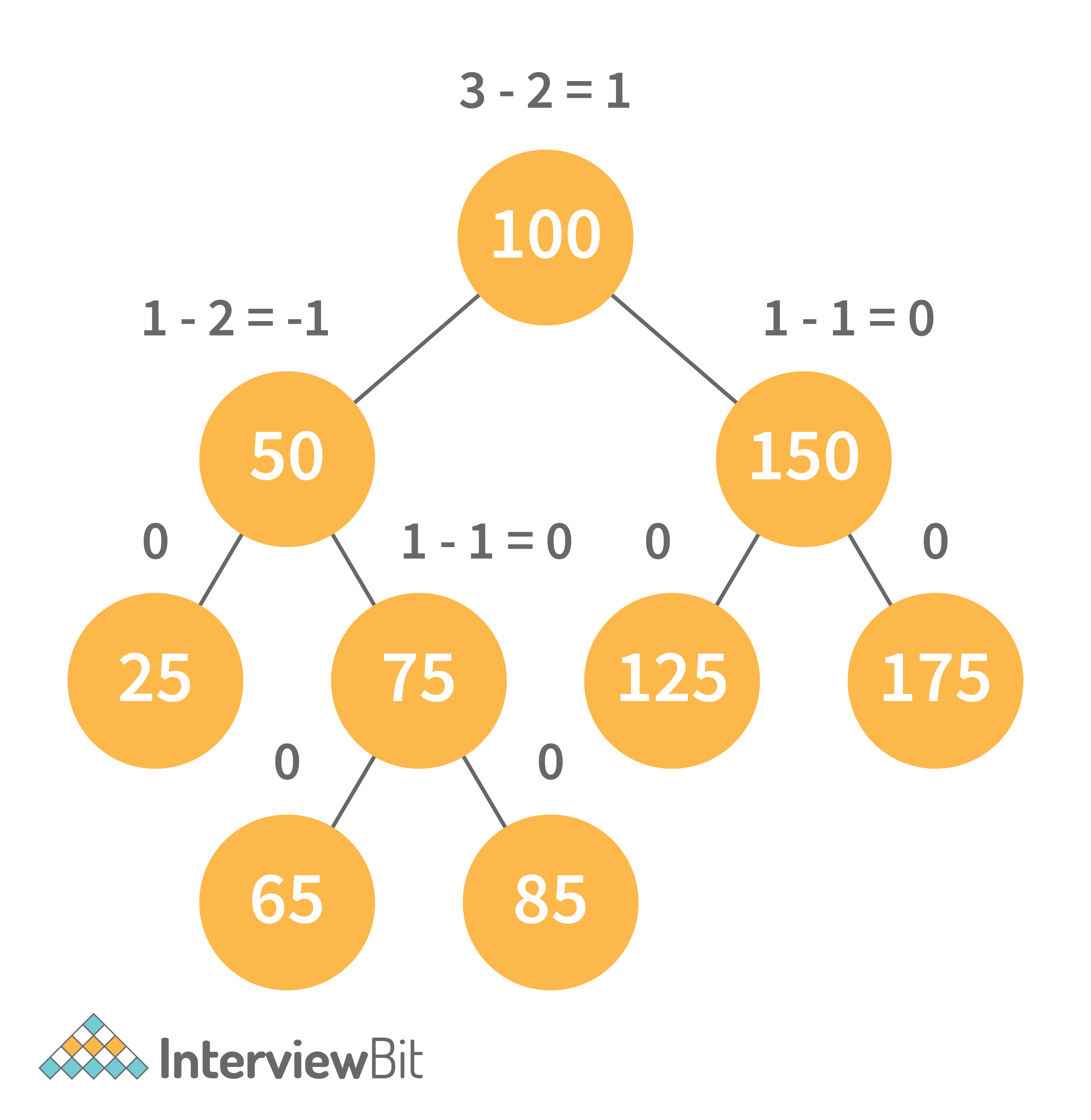
We can perform the following two operations on AVL tree:
- Insertion: Insertion in an AVL tree is done in the same way that it is done in a binary search tree. However, it may cause a violation in the AVL tree property, requiring the tree to be balanced. Rotations can be used to balance the tree.
- Deletion: Deletion can also be performed in the same manner as in a binary search tree. Because deletion can disrupt the tree's balance, various types of rotations are used to rebalance it.
An AVL tree can balance itself by performing the four rotations listed below:
- Left rotation: When a node is inserted into the right subtree of the right subtree and the tree becomes unbalanced, we perform a single left rotation.
- Right rotation: If a node is inserted in the left subtree of the left subtree, the AVL tree may become unbalanced. The tree then requires right rotation.
- Left-Right rotation: The RR rotation is performed first on the subtree, followed by the LL rotation on the entire tree.
- Right-Left rotation: The LL rotation is performed first on the subtree, followed by the RR rotation on the entire tree.
Following are some real-time applications for AVL tree data structure:
- AVL trees are typically used for in-memory sets and dictionaries.
- AVL trees are also widely used in database applications where there are fewer insertions and deletions but frequent data lookups are required.
- Apart from database applications, it is used in applications that require improved searching.
11. What is a B-tree data structure? What are the applications for B-trees?
The B Tree is a type of m-way tree that is commonly used for disc access. A B-Tree with order m can only have m-1 keys and m children. One of the primary reasons for using a B tree is its ability to store a large number of keys in a single node as well as large key values while keeping the tree's height relatively small.
A B-tree of order 4 is shown below in the image:
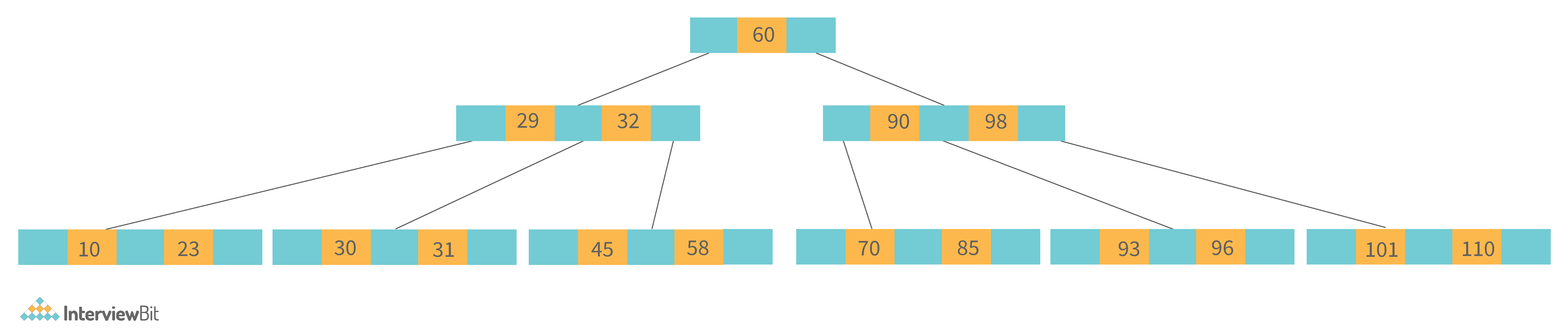
Following are the key properties of a B-tree data structure:
- All of the leaves are at the same height.
- The term minimum degree 't' describes a B-Tree. The value of t is determined by the size of the disc block.
- Except for root, every node must have at least t-1 keys. The root must contain at least one key.
- All nodes (including root) can have no more than 2*t - 1 keys.
- The number of children of a node is equal to its key count plus one.
- A node's keys are sorted in ascending order. The child of two keys k1 and k2 contains all keys between k1 and k2.
- In contrast to Binary Search Tree, B-Tree grows and shrinks from the root.
Following are real-time applications of a B-Tree data structure:
- It is used to access data stored on discs in large databases.
- Using a B tree, you can search for data in a data set in significantly less time.
- The indexing feature allows for multilevel indexing.
- The B-tree approach is also used by the majority of servers.
12. Define Segment Tree data structure and its applications.
A segment Tree is a binary tree that is used to store intervals or segments. The Segment Tree is made up of nodes that represent intervals. Segment Tree is used when there are multiple range queries on an array and changes to array elements.
The segment tree of array A[7] will look like this:
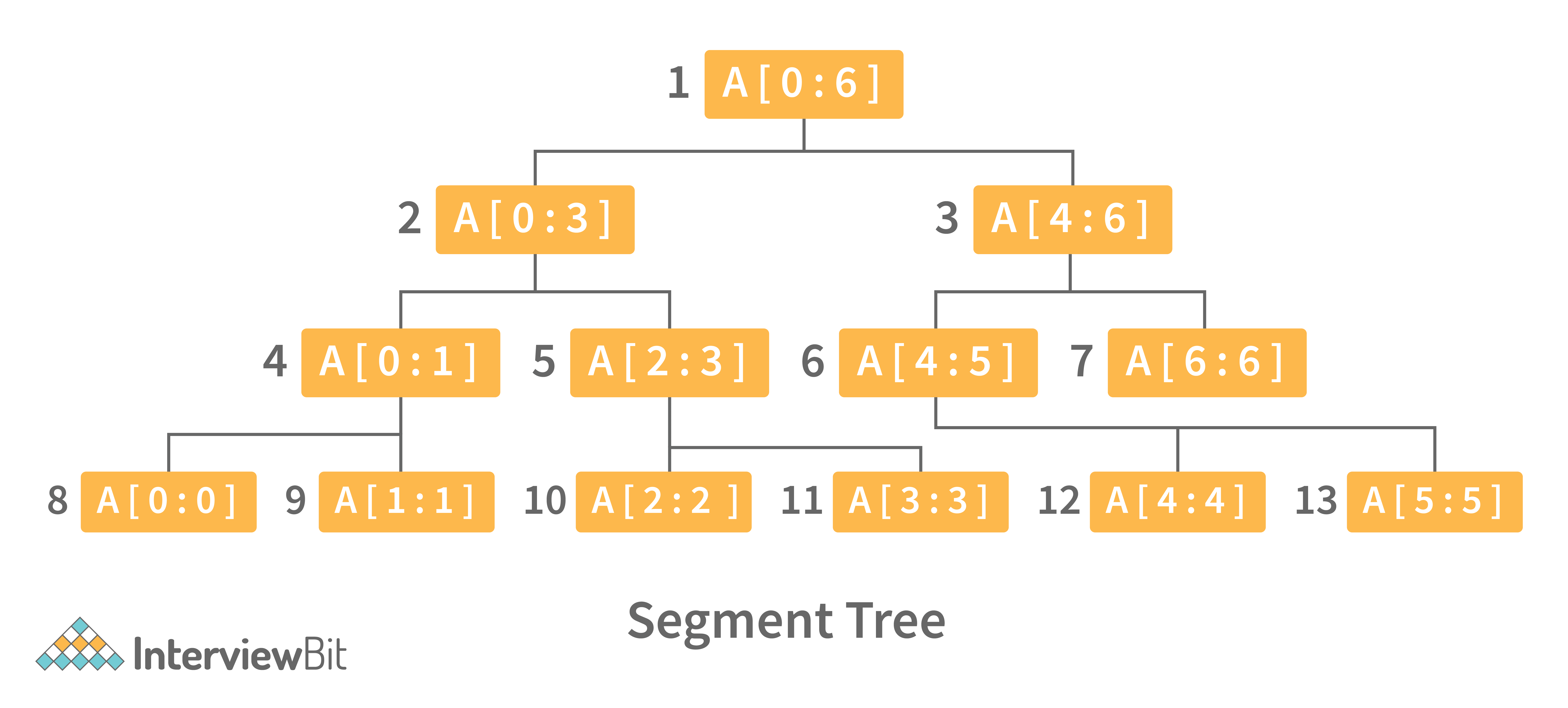
Following are key operations performed on the Segment tree data structure:
- Building Tree: In this step, we create the structure and initialize the segment tree variable.
- Updating the Tree: In this step, we change the tree by updating the array value at a point or over an interval.
- Querying Tree: This operation can be used to run a range query on the array.
Following are real-time applications for Segment Tree:
- Used to efficiently list all pairs of intersecting rectangles from a list of rectangles in the plane.
- The segment tree has become popular for use in pattern recognition and image processing.
- Finding range sum/product, range max/min, prefix sum/product, etc
- Computational geometry
- Geographic information systems
- Static and Dynamic RMQ (Range Minimum Query)
- Storing segments in an arbitrary manner
13. Define Trie data structure and its applications
The word "Trie" is an abbreviation for "retrieval." Trie is a data structure that stores a set of strings as a sorted tree. Each node has the same number of pointers as the number of alphabet characters. It can look up a word in the dictionary by using its prefix. Assuming that all strings are formed from the letters 'a' to 'z' in the English alphabet, each trie node can have a maximum of 26 points.
Trie is also referred to as the digital tree or the prefix tree. The key to which a node is connected is determined by its position in the Trie. Trie allows us to insert and find strings in O(L) time, where L is the length of a single word. This is clearly faster than BST. Because of how it is implemented, this is also faster than Hashing. There is no need to compute a hash function. There is no need to handle collisions (like we do in open addressing and separate chaining)
Another benefit of Trie is that we can easily print all words in alphabetical order, which is not easy with hashing. Trie can also perform prefix search (or auto-complete) efficiently.
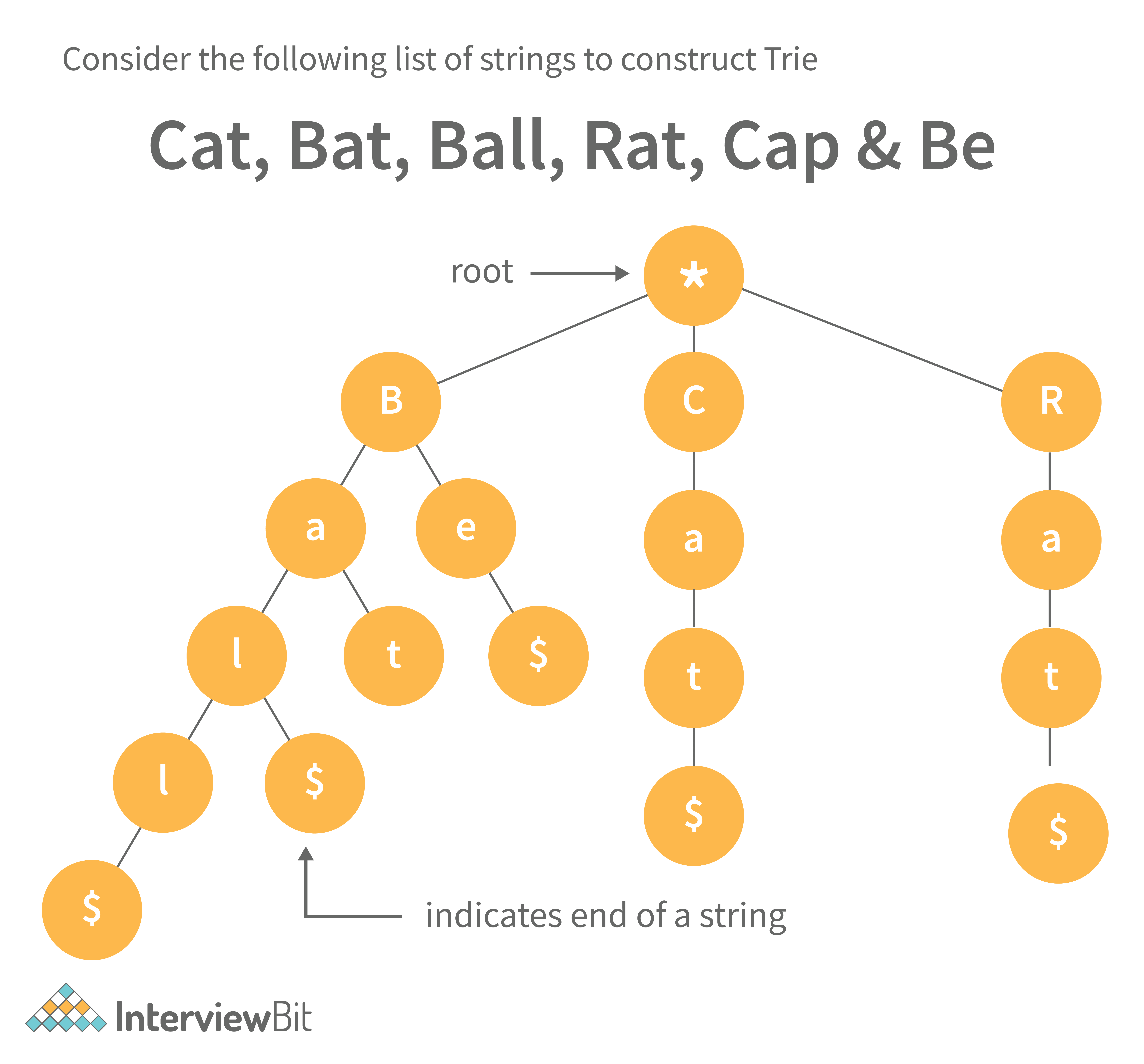
The main disadvantage of tries is that they require a large amount of memory to store the strings. We have an excessive number of node pointers for each node
Following are some real-time applications for Trie data structure:
- Auto-Complete and Search for Search Engines
- Genome Analysis
- Data Analytics
- Browser History
- Spell Checker
14. Define Red-Black Tree and its applications
Red Black Trees are a type of self-balancing binary search tree. Rudolf Bayer invented it in 1972 and dubbed it "symmetric binary B-trees."
A red-black tree is a Binary tree in which each node has a colour attribute, either red or black. By comparing the node colours on any simple path from the root to a leaf, red-black trees ensure that no path is more than twice as long as any other, ensuring that the tree is generally balanced.
Red-black trees are similar to binary trees in that they both store their data in two's complementary binary formats. However, red-black trees have one important advantage over binary trees: they are faster to access. Because red-black trees are so fast to access, they are often used to store large amounts of data.
Red-black trees can be used to store any type of data that can be represented as a set of values.
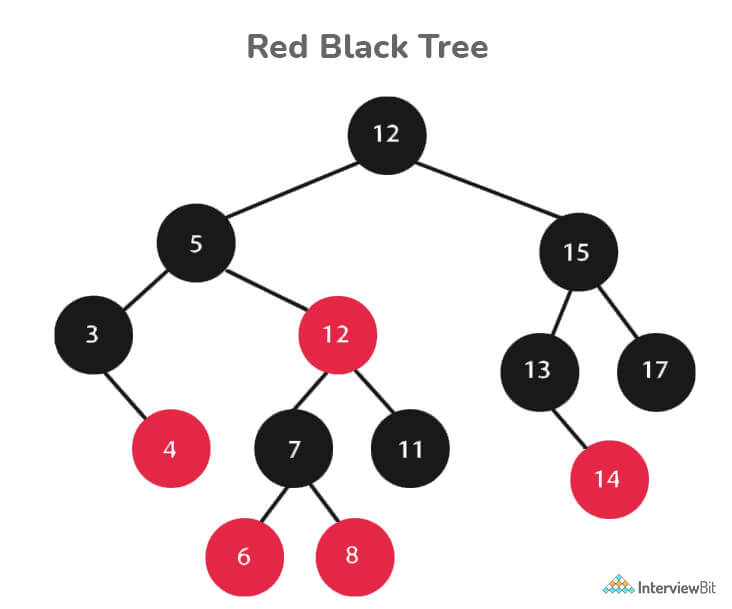
Every Red-Black Tree Obeys the Following Rules:
- Every node is either red or black.
- The tree's root is always black.
- There are no two red nodes that are adjacent.
- There is the same number of black nodes on every path from a node to any of its descendant's NULL nodes.
- All of the leaf nodes are black.
Following are some real-time applications for the Red-Black Tree data structure:
- The majority of self-balancing BST library functions in C++ or Java use Red-Black Trees.
- It is used to implement Linux CPU Scheduling.
- It is also used to reduce time complexity in the K-mean clustering algorithm in machine learning.
- MySQL also employs the Red-Black tree for table indexes in order to reduce searching and insertion time.
15. Which data structures are used for implementing LRU cache?
LRU cache or Least Recently Used cache allows quick identification of an element that hasn’t been put to use for the longest time by organizing items in order of use. In order to achieve this, two data structures are used:
- Queue – This is implemented using a doubly-linked list. The maximum size of the queue is determined by the cache size, i.e by the total number of available frames. The least recently used pages will be near the front end of the queue whereas the most recently used pages will be towards the rear end of the queue.
- Hashmap – Hashmap stores the page number as the key along with the address of the corresponding queue node as the value.
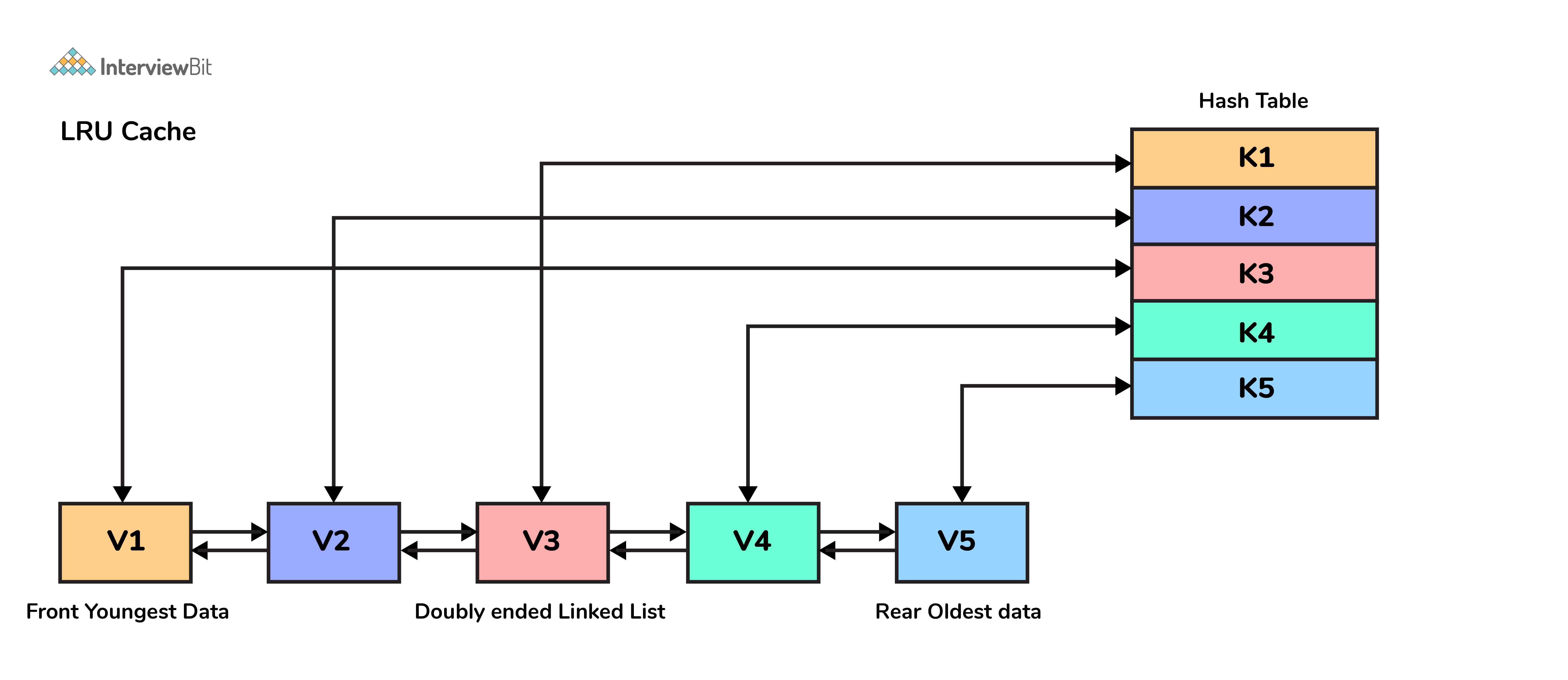
16. What is a heap data structure?
Heap is a special tree-based non-linear data structure in which the tree is a complete binary tree. A binary tree is said to be complete if all levels are completely filled except possibly the last level and the last level has all elements as left as possible. Heaps are of two types:
- In a Max-Heap the data element present at the root node must be the greatest among all the data elements present in the tree.
- This property should be recursively true for all sub-trees of that binary tree.
- In a Min-Heap the data element present at the root node must be the smallest (or minimum) among all the data elements present in the tree.
Data Structure Coding Interview Questions
1. write a program to remove duplicates from a sorted array in place.
- Input : {1, 1, 1, 2, 3, 3, 6, 6, 7}
- Output : {1, 2, 3, 6, 7}
- Explanation : The given input has only 1,2,3,6, and 7 as unique elements, hence the output only lists them out.
- Time Complexity : O(n)
- Space Complexity: O(1)
2. Write a function for zigzag traversal in a binary tree
- Output: [1, 3, 2, 4, 5, 6, 8, 7]
- Explanation: Zigzag Traversal first iterates the given level of the tree from left to right and then the next level as the right to the level.
- Space Complexity: O(n)
3. Write a function to sort a linked list of 0s, 1s and 2s
- Input: 0->1->0->2->1->0->2->1
- Output: 0->0->0->1->1->1->2->2
- Explanation : All 0’s will come first then 1s and then 2s. This can be done in O(n) time by counting the occurrences of all three and rearranging them in the linked list.
4. Write a function to detect cycle in an undirected graph
- Input: n = 4, e = 4 , 0 1, 1 2, 2 3, 3 1
- Output: Yes
- Explanation : The graph is represented as follows in adjacency list representation: 0->1 1->2 2->3 3->1
From the above representation, we can see that there exists a cycle: 1→2→3→1
- Time Complexity: O(V+E)
- Space Complexity: O(V)
5. Write a function to convert an infix expression to postfix expression
- Input: a+b*(c^d)
- Output: abcd^*+
- Time Complexity: O(n)
6. Write a function to find the maximum for each and every contiguous subarray of size k.
- Input: N = 9, K = 3 arr[] = {1, 2, 3, 1, 4, 5, 2, 3, 6}
- Output: {3, 3, 4, 5, 5, 5, 6}
- Explanation : In the first subarray of size 3: {1,2,3}, the value 3 is maximum, similarly for all such subarrays for size 3.
- Space Complexity: O(k)
7. Write a function to merge two sorted binary search tree
/ \
5 9
Output: 3 4 5 6 7 9 12
- Time Complexity: O(m+n)
- Space Complexity: O(height of the first tree + height of the second tree)
8. Write a function to print all unique rows of the given matrix.
{{1, 1, 1, 0, 0},
{0, 1, 0, 0, 1},
{1, 0, 1, 1, 0},
{1, 1, 1, 0, 0}}
{{1, 1, 1, 0, 0},
{0, 1, 0, 0, 1},
{1, 0, 1, 1, 0}}
- Time Complexity: O( ROW x COL )
- Space Complexity: O( ROW )
9. Write a function to find number of subarrays with product less than K
- Input: arr = [1, 6, 2, 3, 2, 1], k = 12
10. Find the subsequence of length 3 with the highest product from a sequence of non-negative integers, with the elements in increasing order.
- Input: n = 8 arr[ ] = {6, 7, 10, 1, 2, 3, 11, 12}
- Output: {10, 11, 12}
The three increasing elements of the given arrays are 10, 11, and 12, which form a three-size subsequence with the highest product.
- Time Complexity: O(nlog(n))
11. Write a function to implement Quicksort on Doubly Linked List
- Input: 8<->10<->1<->7<->6
- Output: 1<->6<->7<->8<->10
- Time Complexity: O(n^2) in the worst case when the list is already sorted. O(nlog(n)) in the best and average case.
12. Write a function to connect nodes at the same level of a binary tree
Input: 100
/ \
13 15
/ \ \
14 1 20
Output: 100-> NULL
/ \
13 -> 15 -> NULL
/ \ \
14 -> 1 -> 20 -> NULL
13. Write a function to find number of structurally unique binary trees are possible
Input: N = 3
Output: 5 for N = 3, there are 5 possible BSTs:
1 3 3 2 1
\ / / / \ \
3 2 1 1 3 2
/ / \ \
2 1 2 3
- Time Complexity: O(n)
14. Implement LRU(Least Recently Used) Cache
- Time Complexity: O(1) to get an element
15. Write a function to determine whether duplicate elements in a given array are within a given distance of each other.
- Input: arr[] = {1, 2, 3, 4, 2, 1, 2} range=3
- Output: True
16. Write a recursive function to calculate the height of a binary tree in Java.
- Consider that every node of a tree represents a class called Node as given below:
- Then the height of the binary tree can be found as follows:
17. Write Java code to count number of nodes in a binary tree
int countNodes (Node root) { int count = 1 ; //Root itself should be counted if (root == null ) return 0 ; else { count += countNodes(root.left); count += countNodes(root.right); return count; } }
18. Print Left view of any binary trees.
- The main idea to solve this problem is to traverse the tree in pre order manner and pass the level information along with it. If the level is visited for the first time, then we store the information of the current node and the current level in the hashmap. Basically, we are getting the left view by noting the first node of every level.
- At the end of traversal, we can get the solution by just traversing the map.
- Consider the following tree as example for finding the left view:
- Left view of a binary tree in Java:
19. Given an m x n 2D grid map of '1’s which represents land and '0’s that represents water return the number of islands (surrounded by water and formed by connecting adjacent lands in 2 directions - vertically or horizontally).
Assume that the boundary cases - which are all four edges of the grid are surrounded by water., constraints are:, m == grid.length n == grid[i].length 1 <= m, n <= 300 grid[i][j] can only be ‘0’ or ‘1’., input: grid = [ [“1” , “1” , “1” , “0” , “0”], [“1” , “1” , “0” , “0” , “0”], [“0” , “0” , “1” , “0” , “1”], [“0” , “0” , “0” , “1” , “1”] ], 20. what is topological sorting in a graph.
- Topological sorting is a linear ordering of vertices such that for every directed edge ij, vertex i comes before j in the ordering.
- Topological sorting is only possible for Directed Acyclic Graph (DAG).
- jobs scheduling from the given dependencies among jobs.
- ordering of formula cell evaluation in spreadsheets
- ordering of compilation tasks to be performed in make files,
- data serialization
- resolving symbol dependencies in linkers.
- Topological Sort Code in Java:
In this post, we covered the most important and frequently asked Data Structures interview questions. We've also included some pointers and tricks to help you prepare for the interview. When preparing for the product-based companies interview, keep in mind that the Data Structures interview is a critical component of the process. It is critical that you are well prepared for the interview because it will determine whether or not you are hired. As a result, it is critical to begin planning as soon as possible.
Additional Interview Resources
- Programming: DSA - https://www.interviewbit.com/courses/programming/
- Data Structure MCQ - https://www.interviewbit.com/data-structure-mcq/
- Best Books for Data Structures and Algorithms - https://www.interviewbit.com/blog/data-structures-and-algorithms-books/
- Best Data Structures and Algorithms Course - https://www.interviewbit.com/blog/best-courses-for-data-structures-and-algorithms/
- Free Java DSA Certification Course - https://www.scaler.com/topics/course/dsa-beginners-java/
- Algorithm Interview Questions - https://www.interviewbit.com/algorithm-interview-questions/
- Master Data Structures and Algorithms With the Scaler Academy Program - https://www.scaler.com/courses/data-structures-and-algorithms/
- Difference Between Array and Linked List - https://www.scaler.com/topics/difference-between-array-and-linked-list/
Coding Problems
Which of the following is a non-linear data structure?
Which of the following data structures is linear?
Which data structure is used for implementing recursion?
Which of the following data structures are indexed structures?
Stack data structure cannot be used for
Which of the following data structures is preferred in the implementation of a database system?
How can memory be saved when storing colour information in a Red-Black tree?
Which of the following data structures is best for searching words in dictionaries?
Which of the following statement is true:
What is the worst-case time complexity of inserting n elements into an empty linked list that must be kept in sorted order?
Which of the following data structure can’t store the non-homogeneous data elements?
A directed graph is _______ if there is a path from each vertex to every other vertex in the graph.
In what traversal we process all of a vertex’s descendants before we move to an adjacent vertex?
In circular queue, the value of REAR would be?
Which of the following statement is true?
- Statement i) Using singly linked lists and circular list, it is not possible to traverse the list backwards.
- Statement ii) To find the predecessor, it is required to traverse the list from the first node in case of singly linked list.
The binary search method needs no more than ________ comparisons.
Which of the following are the properties of a binary tree?
Which of the following scenario is true for the statement - “Arrays are best data structures”?
Which of the following code snippet is used to convert decimal to binary numbers?
What will be the final elements on the stack if the following sequence of operations are executed?
Dijkstra’s Algorithm cannot be applied on which of the following?
- Privacy Policy
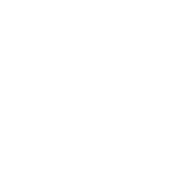
- Practice Questions
- Programming
- System Design
- Fast Track Courses
- Online Interviewbit Compilers
- Online C Compiler
- Online C++ Compiler
- Online Java Compiler
- Online Javascript Compiler
- Online Python Compiler
- Interview Preparation
- Java Interview Questions
- Sql Interview Questions
- Python Interview Questions
- Javascript Interview Questions
- Angular Interview Questions
- Networking Interview Questions
- Selenium Interview Questions
- Data Science Interview Questions
- System Design Interview Questions
- Hr Interview Questions
- Html Interview Questions
- C Interview Questions
- Amazon Interview Questions
- Facebook Interview Questions
- Google Interview Questions
- Tcs Interview Questions
- Accenture Interview Questions
- Infosys Interview Questions
- Capgemini Interview Questions
- Wipro Interview Questions
- Cognizant Interview Questions
- Deloitte Interview Questions
- Zoho Interview Questions
- Hcl Interview Questions
- Highest Paying Jobs In India
- Exciting C Projects Ideas With Source Code
- Top Java 8 Features
- Angular Vs React
- 10 Best Data Structures And Algorithms Books
- Best Full Stack Developer Courses
- Best Data Science Courses
- Python Commands List
- Data Scientist Salary
- Maximum Subarray Sum Kadane’s Algorithm
- Python Cheat Sheet
- C++ Cheat Sheet
- Javascript Cheat Sheet
- Git Cheat Sheet
- Java Cheat Sheet
- Data Structure Mcq
- C Programming Mcq
- Javascript Mcq
1 Million +
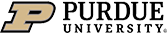
Playing With(out) Golden Hands: The Intersections of Video Game Controllers and Gamer Identity
Since the Electronic Software Association (ESA) began reporting data for the video game industry in 2002, women have represented nearly half of the game playing population. However, despite this stable statistic, the industry’s ideal “Gamer” is consistently depicted as a young, white, cisgender, heterosexual, able-bodied male, and the games industry frequently targets this idealized identity through advertising and game design. This has resulted in a culture that is notably toxic towards women and marginalized players, built on an assumption of meritocracy within games—or the expectation that every player begins each game with the same advantages, disadvantages, and skills as every other player. While the construction of gamer identity has received extensive scholarly attention, gaming peripherals—such as video game controllers—are either minimized or left entirely out of the conversation. This dissertation, informed by feminist methodologies in technical communication and game studies, uses a mixed-methods approach involving archival research, visual analysis, surveys, and interviews to understand the intersections of video game controllers and gamer identity. Using Microsoft’s Xbox as a case study, the findings demonstrate how a dominant narrative has controlled controller design decisions through iterative processes. This has resulted in controllers that are more uncomfortable, more unusable, and more frustrating for and viewed more negatively by women and marginalized players. For each controller iteration, women and marginalized participants rated controllers significantly lower. Though the total improvement score (TIS) from first iteration to current iteration were similar between women and marginalized participants and cismale participants, the lower starting point for women and marginalized participants resulted in a lower ending point. Design decisions across controller iterations privilege cismale experiences, reifying gamer identity through controller design and resulting in not just an ideal gamer identity, but an ideal gamer body.
Degree Type
- Doctor of Philosophy
Campus location
- West Lafayette
Advisor/Supervisor/Committee Chair
Additional committee member 2, additional committee member 3, additional committee member 4, usage metrics.
- Technical writing
- Digital writing
- Media industry studies
- Communication technology and digital media studies
- Media studies
- Computer gaming and animation

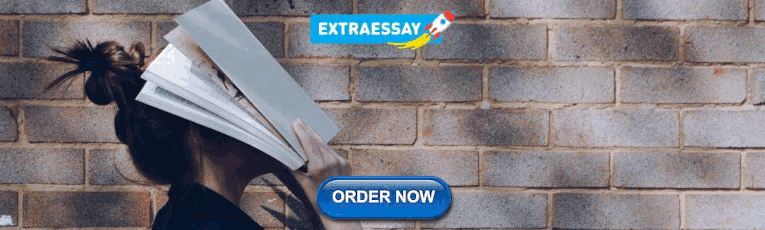
IMAGES
VIDEO
COMMENTS
Including interviews in your dissertation. To present interviews in a dissertation, you first need to transcribe your interviews. You can use transcription software for this. You can then add the written interviews to the appendix. If you have many or long interviews that make the appendix extremely long, the appendix (after consultation with ...
Introduction. When you incorporate original interview data into your writing, you are developing new ideas by using quotations and often sources that no one else has accessed. Drawing from interviews can liven up your writing, ground your big concepts within the specific circumstances of particular individuals, and introduce you to insights you ...
Step 1 - Initial coding. The first step of the coding process is to identify the essence of the text and code it accordingly. While there are various qualitative analysis software packages available, you can just as easily code textual data using Microsoft Word's "comments" feature.
If you need 10 interviews, it is a good idea to plan for 15. Likely, a few will cancel, delay, or not produce useful data. 5. Not keeping your golden thread front of mind. We touched on this a little earlier, but it is a key point that should be central to your entire research process.
The results chapter in a dissertation or thesis (or any formal academic research piece) is where you objectively and neutrally present the findings of your qualitative analysis (or analyses if you used multiple qualitative analysis methods ). This chapter can sometimes be combined with the discussion chapter (where you interpret the data and ...
The 6 Steps of Qualitative Interview Data Analysis. Among qualitative interview data analysis methods, thematic content analysis is perhaps the most common and effective method. It can also be one of the most trustworthy, increasing the traceability and verification of an analysis when done correctly. The following are the six main steps of a ...
Introduction. In medical education research, the qualitative research interview is a viable and highly utilized data-collection tool (DiCicco-Bloom and Crabtree Citation 2006; Jamshed Citation 2014).There are a range of interview formats, conducted with both individuals and groups, where semi-structured interviews are becoming increasingly prevalent in medical education research.
Qualitative fieldwork often generates a considerable data corpus. Hours of interviews or focus groups, pages of observational notes, and countless documents can be retrieved during the data collection. The amount of data collected is often conditioned on the length of the fieldwork phase. Thus, one of the first steps in qualitative analysis is ...
Additionally, interviews can be tailored specifically to the knowledge and experience of the interviewee. Designing and structuring the interview Qualitative interviews can range from highly exploratory to addressing specific hypotheses. As a result, the structure of interviews can range from loose conversations to structured exchanges in
A great way of getting the data you need for your dissertation research question is by interviewing people. You can approach interviewing in a number of ways; the methods you choose depend on what you're trying to find out. The kinds of methods you can choose include: Life history. Paired.
Coding involves identifying themes across interview data by reading and re-reading (and re-reading again) interview transcripts, until the researcher has a clear idea about what sorts of themes come up across the interviews. Coding helps to achieve the goal of data management and data reduction (Palys & Atchison, 2014, p. 304).
A dissertation interview is a method of primary data collection used in academic research, typically undertaken for a dissertation or thesis. It can be in the form of a structured, semi-structured, or unstructured interview between the researcher and the interviewee(s), with the goal of gaining detailed, firsthand insights into the research topic.
Here are some steps to follow: 1. Gather Qualitative Data. Qualitative data can be collected through various means. For one, you can record the interview and take advantage of legal-grade transcription services. Taking this approach will help you avoid data loss and inaccuracies.
Interviewing. This is the most common format of data collection in qualitative research. According to Oakley, qualitative interview is a type of framework in which the practices and standards be not only recorded, but also achieved, challenged and as well as reinforced.[] As no research interview lacks structure[] most of the qualitative research interviews are either semi-structured, lightly ...
There is much literature using primary interview data, but often, the primary data represent either a small sample size or a limited regional pool. Additionally, there often is a lack of continuity or connection between the different primary research in literature, which makes them difficult to combine. ... (Unpublished doctoral dissertation ...
This article addresses the practical and ethical challenges faced when anonymising qualitative interview data. We draw on examples from our interview study with more than 50 individuals who have a relative with a chronic disorder of consciousness, i.e. who are in a vegetative or minimally conscious state with little or no awareness of themselves or their environment.
1. Familiarize yourself with your data to become more informed. Read the interviews to start getting to know your sources. Then read the data again, this time making notes of your impressions. Go through the data set several times, and determine which interviews are useful and which you might set aside. [1]
The purpose of this paper is to help authors to think about ways to present qualitative research papers in the American Journal of Pharmaceutical Education. It also discusses methods for reviewers to assess the rigour, quality, and usefulness of qualitative research. Examples of different ways to present data from interviews, observations, and ...
1 Answer to this question. Answer: Analyzing and presenting qualitative data in a research paper can be difficult. The Methods section is where one needs to justify and present the research design. As you have rightly said, there are stipulations on the word count for a manuscript. To present the interview data, you can consider using a table.
The analysis of the collected interview data hopes to answer the question, 'How do women view, use, and incorporate the website Pinterest into their daily lives?' To assist in this analysis, a conceptual and theoretical framework that draws from ... of interest for this dissertation, the use of interviews to explore the variety of opinions
Summary. Collecting primary data via interviews can be supplemented with using a case study. Interviews take time to prepare and write up. Showing a comprehensive understandings of the interview process will help gain a better dissertation mark! AbleSim Project Management Simulations YouTube Channel.
Traditionally, focus group research is "a way of collecting qualitative data, which—essentially—involves engaging a small number of people in an informal group discussion (or discussions), 'focused' around a particular topic or set of issues" (Wilkinson, 2004, p. 177).Social science researchers in general and qualitative researchers in particular often rely on focus groups to ...
1. Research the position and the company. If you want to know what may be asked in your data science interview, the best place to start is by researching the role you are applying for and the company itself. Check out company websites, social media pages, reviews, and even speak to people working there.
15. Elaborate on different types of array data structure. There are several different types of arrays: One-dimensional array: A one-dimensional array stores its elements in contiguous memory locations, accessing them using a single index value. It is a linear data structure holding all the elements in a sequence.
This dissertation, informed by feminist methodologies in technical communication and game studies, uses a mixed-methods approach involving archival research, visual analysis, surveys, and interviews to understand the intersections of video game controllers and gamer identity. Using Microsoft's Xbox as a case study, the findings demonstrate ...