- Contact Contact icon Contact
- Training Training icon Training

- Portuguese, Brazil
- Chinese, Simplified
Check the spelling in your query or search for a new term.
Site search accepts advanced operators to help refine your query. Learn more.
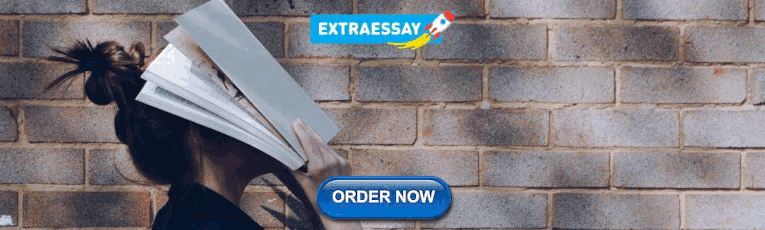
Top 10 areas of green energy research
Magesh Ganesan , Scientist, ACS International India Pvt. Ltd.
February 29, 2024

With so much being published on green energy and sustainability, how can you identify the fastest growing areas of research? Learn how CAS Insights provides a unique view of the green energy research landscape and can help you identify emerging trends sooner. Subscribe to be the first to know when we publish new scientific insights.
Fossil fuels remain our primary energy source, but their limited availability and negative environmental impact have led to events like the 2023 United Nations Climate Change Conference (COP28) to seek green energy alternatives. These are sources that can be replenished in the average human lifespan and have a net zero environmental impact.
Every year, millions of journal articles and patent applications are created, so it can be difficult to identify the signals from the noise. CAS curates, connects, and analyzes the world’s published science inside the CAS Content Collection™ to provide a unique view of the scientific landscape. This enables novel insights that show emerging trends in new research areas. Five broad areas of research were identified that contained the fastest-growing trends: batteries, hydrogen energy, solar cells, new materials, and photothermal energy.
How was the analysis done?
First, CAS identified almost one million indexed documents in the CAS Content Collection™ that were relevant to the green energy space. Then, our researchers analyzed the hidden connections between key concepts using advanced analytics, knowledge graphing, and natural language processing to identify emerging trends in this area. Finally, our expert scientists with dozens of years of experience derived unique insights from the landscape of connections created. Several exciting growth patterns emerged between 2018 and 2022 that are early indicators of opportunities ahead. While many areas of green energy are growing quickly, we identified and prioritized the top ten emerging topics (Figure 1) that will help us reach a more sustainable future.
Batteries, energy storage, and battery recycling
Batteries are the leading method of storing electricity worldwide. Lithium-ion batteries have become commonplace, being used in portable devices and electric vehicles due to their high energy density, while lead-acid batteries are conventionally used for portable and stationary power storage. However, lithium-ion batteries are a fire hazard while lead-acid batteries are notably toxic. This has led to researchers looking for alternate, safer ways to store electricity.
- Aqueous zinc-ion batteries: These batteries are being studied as alternatives to lead-acid batteries because they are naturally occurring, much more environmentally friendly, and notably cheaper and non-toxic.
- Solid-state lithium-ion battery: Standard lithium-ion batteries degrade quickly, are fire hazards, and have high toxicity. However, they are still widely used because of faster charging and easy manufacturing. Solid-state lithium-ion batteries can be charged and discharged many times more than lithium-ion batteries and hold more electricity.
The successful development of these new battery types will make the industry much safer. Not only will solid-state lithium batteries be less of a fire hazard, but the overall pollution will drop, owing to the absence of toxic liquid electrolytes and more sustainable production. Learn more about lithium-ion batteries , the landscape of recyclin g legislation and regulations , and the new breakthroughs that are driving innovation.
Hydrogen energy, green hydrogen economy, and hydrogen storage
Hydrogen has emerged as a promising alternative to fossil fuels, being more environmentally friendly, having higher energy per given weight than gasoline, and more applicable in many energy-related fields.
- Liquid hydrogen storage: Hydrogen has three times the gravimetric density of gasoline but only one-fourth of the volumetric energy density. This means liquid hydrogen is considered the most efficient method of storing hydrogen in its base form, and researchers are seeking new ways to take advantage of it. If properly harnessed, liquid hydrogen storage could enable new fuel cell-driven automobiles and decrease costs in petroleum refining, fertilizer production, and more.
- Water splitting using heterojunction photocatalysts: Photocatalysts have emerged as a sustainable energy source, producing hydrogen using only water and sunlight. The main challenge, however, is identifying or developing them due to low efficiency and unsuitable band positions. Once these hurdles are overcome, the cost of hydrogen is expected to decrease, which could make it the preferred fuel source.
The benefits of these hydrogen production and storage technologies are immense. Urea oxidation will be dual purpose, cleaning water and providing energy simultaneously. More efficient storage methods could facilitate the utilization of hydrogen fuel cells, revolutionizing commercial products like automobiles. These changes would be further bolstered by new water-splitting methods, which would make accessing the necessary hydrogen for these processes much cheaper. Learn more about photocatalysis and new breakthroughs in the landscape of green hydrogen production.
Solar cells
Solar cells have seen an increased interest both academically and commercially. As industries look for more sustainable options, there will be more studies on how to optimize this technology for higher efficiency and lower cost.
- Non-fullerene acceptors for solar cells: The performance of organic solar cells has increased, but development is already underway to replace their most used acceptor, fullerene, with an alternative. These non-fullerene acceptors have more tunable properties, higher thermal and photochemical stability, and can lead to longer device lifetimes. This could lead to more stable, longer-lasting, and cheaper solar cells.
- Stable perovskite solar cells: As researchers look to enhance the efficiency of solar cells, one area of interest is perovskite-based solar cells. These easy-to-fabricate, low-cost cells have reported some of the highest energy efficiencies. However, the current materials used are unstable in certain conditions. The advantages of stable perovskite solar cells remain substantial and could diminish manufacturing costs if this challenge is overcome.
The main hurdle that these two innovations could overcome is cost. By cutting out fullerene or developing cells with perovskite, solar energy could be more affordable to many consumers. Learn more about emerging technologies in materials .
Sustainable chemistry, new materials, and greener alternatives
Noble and toxic metals are frequently used in the energy field. While functional, risks and challenges remain, hindering the industry’s progress. This has led studies to examine more sustainable and efficient alternatives.
- Mxenes: These are two-dimensional materials that incorporate a transition metal and a functional group. Their layered nature makes them strong candidates for energy storage applications like capacitors and batteries while their optical and catalytic properties have potential in photocatalysis and electrocatalysis. Mxenes also contain earth-abundant elements, circumventing the risks associated with noble or toxic metals. Any breakthrough with this material could result in significant cost, environmental, and energy storage benefits.
- Covalent organic frameworks: These are two or three-dimensional structures formed by organic precursor reactions. Forming covalently bonded, porous structures, they are being studied for hydrogen/methane and catalytic/electrocatalytic energy storage applications. Successful covalent organic framework applications could lead to many economic and environmental benefits in energy storage, chemical synthesis, catalysis, and gas separation with special interest in the automobile industry.
These materials have many possible applications. Both being made of earth-abundant materials, they will be more available and sustainable compared to other materials. By replacing current materials with covalent organic frameworks and mxenes, there will also be significant environmental and economic benefits across many industries. Learn more about sustainable catalysts , new biomaterials, and carbon nanotubes that can help build upon new opportunities ahead.
Solar energy, photothermal conversion, and green energy sources
As researchers try to find ways of reducing energy’s environmental impact, special interest is placed on renewable sources. Solar is already gaining commercial and industrial popularity, but further breakthroughs could lead to wider adoption.
- Photothermal energy conversion: The conversion of solar energy to heat, which generates steam, can generate electricity without using other sources. Researchers are studying inorganic and polymeric materials to find a suitable photothermal candidate. Success in this area could lead to a drastic energy cost reduction, owing to the sole reliance on solar energy. It would also have a substantial positive environmental impact by ideally removing the need for fossil fuels.
Unlocking the potential of photothermal energy conversion could lead to immense energy cost savings and cleaner energy sources. Additionally, there has always been a desire to use solar energy to split water atoms for clean hydrogen production. This process of photocatalysis would be critical to using solar energy for clean hydrogen production and could reshape the future for a green hydrogen economy .
Looking ahead
Green energy will remain a large research focus as we seek a net-zero environmental impact thanks to events like COP28. Suitable alternatives are being examined, but there remain challenges and risks that must be overcome before we see real-world applications. As more green energy advances are made, it can be challenging to keep up with the new developments. Subscribe to CAS Insights™ for unique views and the latest updates on green energy alternatives.
Gain new perspectives for faster progress directly to your inbox.
Related Insights
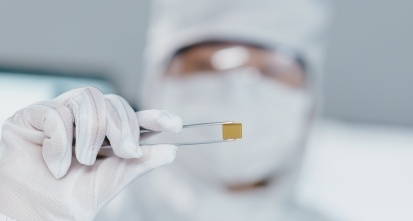
Nuclear power in your pocket? 50-year battery...
March 11, 2024
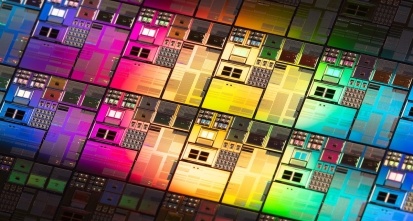
Science Fact Fiction: Can we really recycle...
March 8, 2024
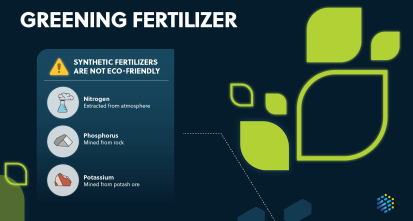
Infographic: Sustainable crops start with green(er)...
Infographic
February 16, 2024
Thank you for visiting nature.com. You are using a browser version with limited support for CSS. To obtain the best experience, we recommend you use a more up to date browser (or turn off compatibility mode in Internet Explorer). In the meantime, to ensure continued support, we are displaying the site without styles and JavaScript.
- View all journals
- Explore content
- About the journal
- Publish with us
- Sign up for alerts
- Perspective
- Published: 18 October 2022
Machine learning for a sustainable energy future
- Zhenpeng Yao ORCID: orcid.org/0000-0001-8286-8257 1 , 2 , 3 , 4 na1 ,
- Yanwei Lum ORCID: orcid.org/0000-0001-7261-2098 5 , 6 na1 ,
- Andrew Johnston 6 na1 ,
- Luis Martin Mejia-Mendoza 2 ,
- Xin Zhou 7 ,
- Yonggang Wen 7 ,
- Alán Aspuru-Guzik ORCID: orcid.org/0000-0002-8277-4434 2 , 8 ,
- Edward H. Sargent ORCID: orcid.org/0000-0003-0396-6495 6 &
- Zhi Wei Seh ORCID: orcid.org/0000-0003-0953-567X 5
Nature Reviews Materials volume 8 , pages 202–215 ( 2023 ) Cite this article
28k Accesses
82 Citations
24 Altmetric
Metrics details
- Computer science
Electrocatalysis
- Energy grids and networks
- Solar cells
Transitioning from fossil fuels to renewable energy sources is a critical global challenge; it demands advances — at the materials, devices and systems levels — for the efficient harvesting, storage, conversion and management of renewable energy. Energy researchers have begun to incorporate machine learning (ML) techniques to accelerate these advances. In this Perspective, we highlight recent advances in ML-driven energy research, outline current and future challenges, and describe what is required to make the best use of ML techniques. We introduce a set of key performance indicators with which to compare the benefits of different ML-accelerated workflows for energy research. We discuss and evaluate the latest advances in applying ML to the development of energy harvesting (photovoltaics), storage (batteries), conversion (electrocatalysis) and management (smart grids). Finally, we offer an overview of potential research areas in the energy field that stand to benefit further from the application of ML.
Similar content being viewed by others
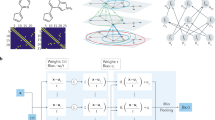
Physics-informed machine learning
George Em Karniadakis, Ioannis G. Kevrekidis, … Liu Yang
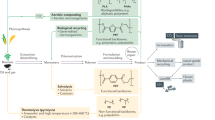
Bioplastics for a circular economy
Jan-Georg Rosenboom, Robert Langer & Giovanni Traverso
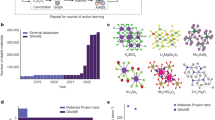
Scaling deep learning for materials discovery
Amil Merchant, Simon Batzner, … Ekin Dogus Cubuk
Introduction
The combustion of fossil fuels, used to fulfill approximately 80% of the world’s energy needs, is the largest single source of rising greenhouse gas emissions and global temperature 1 . The increased use of renewable sources of energy, notably solar and wind power, is an economically viable path towards meeting the climate goals of the Paris Agreement 2 . However, the rate at which renewable energy has grown has been outpaced by ever-growing energy demand, and as a result the fraction of total energy produced by renewable sources has remained constant since 2000 (ref. 3 ). It is thus essential to accelerate the transition towards sustainable sources of energy 4 . Achieving this transition requires energy technologies, infrastructure and policies that enable and promote the harvest, storage, conversion and management of renewable energy.
In sustainable energy research, suitable material candidates (such as photovoltaic materials) must first be chosen from the combinatorial space of possible materials, then synthesized at a high enough yield and quality for use in devices (such as solar panels). The time frame of a representative materials discovery process is 15–20 years 5 , 6 , leaving considerable room for improvement. Furthermore, the devices have to be optimized for robustness and reproducibility to be incorporated into energy systems (such as in solar farms) 7 , where management of energy usage and generation patterns is needed to further guarantee commercial success.
Here we explore the extent to which machine learning (ML) techniques can help to address many of these challenges 8 , 9 , 10 . ML models can be used to predict specific properties of new materials without the need for costly characterization; they can generate new material structures with desired properties; they can understand patterns in renewable energy usage and generation; and they can help to inform energy policy by optimizing energy management at both device and grid levels.
In this Perspective, we introduce Acc(X)eleration Performance Indicators (XPIs), which can be used to measure the effectiveness of platforms developed for accelerated energy materials discovery. Next, we discuss closed-loop ML frameworks and evaluate the latest advances in applying ML to the development of energy harvesting, storage and conversion technologies, as well as the integration of ML into a smart power grid. Finally, we offer an overview of energy research areas that stand to benefit further from ML.
Performance indicators
Because many reports discuss ML-accelerated approaches for materials discovery and energy systems management, we posit that there should be a consistent baseline from which these reports can be compared. For energy systems management, performance indicators at the device, plant and grid levels have been reported 11 , 12 , yet there are no equivalent counterparts for accelerated materials discovery.
The primary goal in materials discovery is to develop efficient materials that are ready for commercialization. The commercialization of a new material requires intensive research efforts that can span up to two decades: the goal of every accelerated approach should be to accomplish commercialization an order-of-magnitude faster. The materials science field can benefit from studying the case of vaccine development. Historically, new vaccines take 10 years from conception to market 13 . However, after the start of the COVID-19 pandemic, several companies were able to develop and begin releasing vaccines in less than a year. This achievement was in part due to an unprecedented global research intensity, but also to a shift in the technology: after a technological breakthrough in 2008, the cost of sequencing DNA began decreasing exponentially 14 , 15 , enabling researchers to screen orders-of-magnitude more vaccines than was previously possible.
ML for energy technologies has much in common with ML for other fields like biomedicine, sharing the same methodology and principles. However, in practice, ML models for different technologies are exposed to additional unique requirements. For example, ML models for medical applications usually have complex structures that take into account regulatory oversight and ensure the safe development, use and monitoring of systems, which usually does not happen in the energy field 16 . Moreover, data availability varies substantially from field to field; biomedical researchers can work with a relatively large amount of data that energy researchers usually lack. This limited data accessibility can constrain the usage of sophisticated ML models (such as deep learning models) in the energy field. However, adaptation has been quick in all energy subfields, with a rapidly increased number of groups recognizing the importance of statistical methods and starting to use them for various problems. We posit that the use of high-throughput experimentation and ML in materials discovery workflows can result in breakthroughs in accelerating development, but the field first needs a set of metrics with which ML models can be evaluated and compared.
Accelerated materials discovery methods should be judged based on the time it takes for a new material to be commercialized. We recognize that this is not a useful metric for new platforms, nor is it one that can be used to decide quickly which platform is best suited for a particular scenario. We therefore propose here XPIs that new materials discovery platforms should report.
Acceleration factor of new materials, XPI-1
This XPI is evaluated by dividing the number of new materials that are synthesized and characterized per unit time with the accelerated platform by the number of materials that are synthesized and characterized with traditional methods. For example, an acceleration factor of ten means that for a given time period, the accelerated platform can evaluate ten times more materials than a traditional platform. For materials with multiple target properties, researchers should report the rate-limiting acceleration factor.
Number of new materials with threshold performance, XPI-2
This XPI tracks the number of new materials discovered with an accelerated platform that have a performance greater than the baseline value. The selection of this baseline value is critical: it should be something that fairly captures the standard to which new materials need to be compared. As an example, an accelerated platform that seeks to discover new perovskite solar cell materials should track the number of devices made with new materials that have a better performance than the best existing solar cell 17 .
Performance of best material over time, XPI-3
This XPI tracks the absolute performance — whether it is Faradaic efficiency, power conversion efficiency or other — of the best material as a function of time. For the accelerated framework, the evolution of the performance should increase faster than the performance obtained by traditional methods 18 .
Repeatability and reproducibility of new materials, XPI-4
This XPI seeks to ensure that the new materials discovered are consistent and repeatable: this is a key consideration to screen out materials that would fail at the commercialization stage. The performance of a new material should not vary by more than x % of its mean value (where x is the standard error): if it does, this material should not be included in either XPI-2 (number of new materials with threshold performance) or XPI-3 (performance of best material over time).
Human cost of the accelerated platform, XPI-5
This XPI reports the total costs of the accelerated platform. This should include the total number of researcher hours needed to design and order the components for the accelerated system, develop the programming and robotic infrastructure, develop and maintain databases used in the system and maintain and run the accelerated platform. This metric would provide researchers with a realistic estimate of the resources required to adapt an accelerated platform for their own research.
Use of the XPIs
Each of these XPIs can be measured for computational, experimental or integrated accelerated systems. Consistently reporting each of these XPIs as new accelerated platforms are developed will allow researchers to evaluate the growth of these platforms and will provide a consistent metric by which different platforms can be compared. As a demonstration, we applied the XPIs to evaluate the acceleration performance of several typical platforms: Edisonian-like trial-test, robotic photocatalysis development 19 and design of a DNA-encoded-library-based kinase inhibitor 20 (Table 1 ). To obtain a comprehensive performance estimate, we define one overall acceleration score S adhering to the following rules. The dependent acceleration factors (XPI-1 and XPI-2), which function in a synergetic way, are added together to reflect their contribution as a whole. The independent acceleration factors (XPI-3, XPI-4 and XPI-5), which may function in a reduplicated way, are multiplied together to value their contributions respectively. As a result, the overall acceleration score can be calculated as S = (XPI-1 + XPI-2) × XPI-3 × XPI-4 ÷ XPI-5. As the reference, the Edisonian-like approach has a calculated overall XPIs score of around 1, whereas the most advanced method, the DNA-encoded-library-based drug design, exhibits an overall XPIs score of 10 7 . For the sustainability field, the robotic photocatalysis platform has an overall XPIs score of 10 5 .
For energy systems, the most frequently reported XPI is the acceleration factor, in part because it is deterministic, but also because it is easy to calculate at the end of the development of a workflow. In most cases, we expect that authors report the acceleration factor only after completing the development of the platform. Reporting the other suggested XPIs will provide researchers with a better sense of both the time and human resources required to develop the platform until it is ready for publication. Moving forward, we hope that other researchers adopt the XPIs — or other similar metrics — to allow for fair and consistent comparison between the different methods and algorithms that are used to accelerate materials discovery.
Closed-loop ML for materials discovery
The traditional approach to materials discovery is often Edisonian-like, relying on trial and error to develop materials with specific properties. First, a target application is identified, and a starting pool of possible candidates is selected (Fig. 1a ). The materials are then synthesized and incorporated into a device or system to measure their properties. These results are then used to establish empirical structure–property relationships, which guide the next round of synthesis and testing. This slow process goes through as many iterations as required and each cycle can take several years to complete.
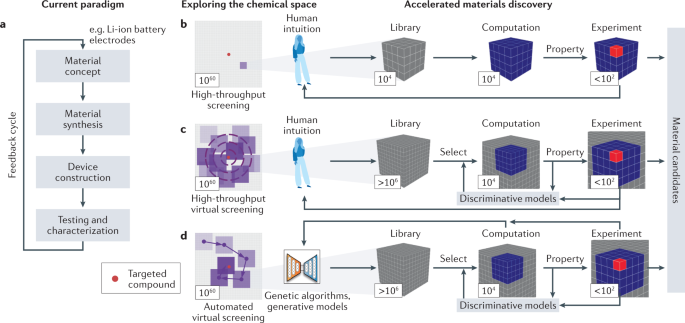
a | Traditional Edisonian-like approach, which involves experimental trial and error. b | High-throughput screening approach involving a combination of theory and experiment. c | Machine learning (ML)-driven approach whereby theoretical and experimental results are used to train a ML model for predicting structure–property relationships. d | ML-driven approach for property-directed and automatic exploration of the chemical space using optimization ML (such as genetic algorithms or generative models) that solve the ‘inverse’ design problem.
A computation-driven, high-throughput screening strategy (Fig. 1b ) offers a faster turnaround. To explore the overall vast chemical space (~10 60 possibilities), human intuition and expertise can be used to create a library with a substantial number of materials of interest (~10 4 ). Theoretical calculations are carried out on these candidates and the top performers (~10 2 candidates) are then experimentally verified. With luck, the material with the desired functionality is ‘discovered’. Otherwise, this process is repeated in another region of the chemical space. This approach can still be very time-consuming and computationally expensive and can only sample a small region of the chemical space.
ML can substantially increase the chemical space sampled, without costing extra time and effort. ML is data-driven, screening datasets to detect patterns, which are the physical laws that govern the system. In this case, these laws correspond to materials structure–property relationships. This workflow involves high-throughput virtual screening (Fig. 1c ) and begins by selecting a larger region (~10 6 ) of the chemical space of possibilities using human intuition and expertise. Theoretical calculations are carried out on a representative subset (~10 4 candidates) and the results are used for training a discriminative ML model. The model can then be used to make predictions on the other candidates in the overall selected chemical space 9 . The top ~10 2 candidates are experimentally verified, and the results are used to improve the predictive capabilities of the model in an iterative loop. If the desired material is not ‘discovered’, the process is repeated on another region of the chemical space.
An improvement on the previous approaches is a framework that requires limited human intuition or expertise to direct the chemical space search: the automated virtual screening approach (Fig. 1d ). To begin with, a region of the chemical space is picked at random to initiate the process. Thereafter, this process is similar to the previous approach, except that the computational and experimental data is also used to train a generative learning model. This generative model solves the ‘inverse’ problem: given a required property, the goal is to predict an ideal structure and composition in the chemical space. This enables a directed, automated search of the chemical space, towards the goal of ‘discovering’ the ideal material 8 .
ML for energy
ML has so far been used to accelerate the development of materials and devices for energy harvesting (photovoltaics), storage (batteries) and conversion (electrocatalysis), as well as to optimize power grids. Besides all the examples discussed here, we summarize the essential concepts in ML (Box 1 ), the grand challenges in sustainable materials research (Box 2 ) and the details of key studies (Table 2 ).
Box 1 Essential concepts in ML
With the availability of large datasets 122 , 125 and increased computing power, various machine learning (ML) algorithms have been developed to solve diverse problems in energy. Below, we provide a brief overview of the types of problem that ML can solve in energy technology, and we then summarize the status of ML-driven energy research. More detailed information about the nuts and bolts of ML techniques can be found in previous reviews 173 , 174 , 175 .
Property prediction
Supervised learning models are predictive (or discriminative) models that are given a datapoint x , and seek to predict a property y (for example, the bandgap 27 ) after being trained on a labelled dataset. The property y can be either continuous or discrete. These models have been used to aid or even replace physical simulations or measurements under certain circumstances 176 , 177 .
Generative materials design
Unsupervised learning models are generative models that can generate or output new examples x ′ (such as new molecules 104 ) after being trained on an unlabelled dataset. This generation of new examples can be further enhanced with additional information (physical properties) to condition or bias the generative process, allowing the models to generate examples with improved properties and leading to the property-to-structure approach called inverse design 52 , 178 .
Self-driving laboratories
Self-driving or autonomous laboratories 19 use ML models to plan and perform experiments, including the automation of retrosynthesis analysis (such as in reinforcement-learning-aided synthesis planning 124 , 179 ), prediction of reaction products (such as in convolutional neural networks (CNNs) for reaction prediction 137 , 138 ) and reaction condition optimization (such as in robotic workflows optimized by active learning 19 , 160 , 180 , 181 , 182 , 183 ). Self-driving laboratories, which use active learning for iterating through rounds of synthesis and measurements, are a key component in the closed-loop inverse design 52 .
Aiding characterization
ML models have been used to aid the quantitative or qualitative analysis of experimental observations and measurements, including assisting in the determination of crystal structure from transmission electron microscopy images 184 , identifying coordination environment 81 and structural transition 83 from X-ray absorption spectroscopy and inferring crystal symmetry from electron diffraction 176 .
Accelerating theoretical computations
ML models can enable otherwise intractable simulations by reducing the computational cost (processor core amount and time) for systems with increased length and timescales 69 , 70 and providing potentials and functionals for complex interactions 68 .
Optimizing system management
ML models can aid the management of energy systems at the device or grid power level by predicting lifetimes (such as battery life 43 , 44 ), adapting to new loads (such as in long short-term memory for building load prediction 95 ) and optimizing performance (such as in reinforcement learning for smart grid control 94 ).
Box 2 Grand challenges in energy materials research
Photovoltaics.
Discover non-toxic (Pd- and Cd-free) materials with good optoelectronic properties
Identify and minimize materials defects in light-absorbing materials
Design effective recombination-layer materials for tandem solar cells
Develop materials design strategies for long-term operational stability 125
Develop (hole/electron) transport materials with high carrier mobility 125
Optimize cell structure for maximum light absorption and minimum use of active materials
Tune materials bandgaps for optimal solar-harvesting performance under complex operation conditions 21 , 22
Develop Earth-abundant cathode materials (Co-free) with high reversibility and charge capacity 4
Design electrolytes with wider electrochemical windows and high conductivity 4
Identify electrolyte systems to boost battery performance and lifetime 4
Discover new molecules for redox flow batteries with suitable voltage 4
Understand correlation between defect growth in battery materials and overall degradation process of battery components
Tune operando (dis)charging protocol for minimized capacity loss, (dis)charging rate and optimal battery life under diversified conditions 7 , 53
Design materials with optimal adsorption energy for maximized catalytic activity 60 , 61
Identify and study active sites on catalytic materials 58
Engineer catalytic materials for extended durability 58 , 60 , 61
Identify a fuller set of materials descriptors that relate to catalytic activity 60 , 61
Engineer multiple catalytic functionalities into the same material 60 , 61
Design multiscale electrode structures for optimized catalytic activity
Correlate atomistic contamination and growth of catalyst particles with electrode degradation process
Tune operando (dis)charging protocol for minimized capacity loss and optimal cell life
ML is accelerating the discovery of new optoelectronic materials and devices for photovoltaics, but major challenges are still associated with each step.
Photovoltaics materials discovery
One materials class for which ML has proved particularly effective is perovskites, because these materials have a vast chemical space from which the constituents may be chosen. Early representations of perovskite materials for ML were atomic-feature representations, in which each structure is encoded as a fixed-length vector comprised of an average of certain atomic properties of the atoms in the crystal structure 21 , 22 . A similar technique was used to predict new lead-free perovskite materials with the proper bandgap for solar cells 23 (Fig. 2a ). These representations allowed for high accuracy but did not account for any spatial relation between atoms 24 , 25 . Materials systems can also be represented as images 26 or as graphs 27 , enabling the treatment of systems with diverse number of atoms. The latter representation is particularly compelling, as perovskites, particularly organic–inorganic perovskites, have crystal structures that incorporate a varying number of atoms, and the organic molecules can vary in size.
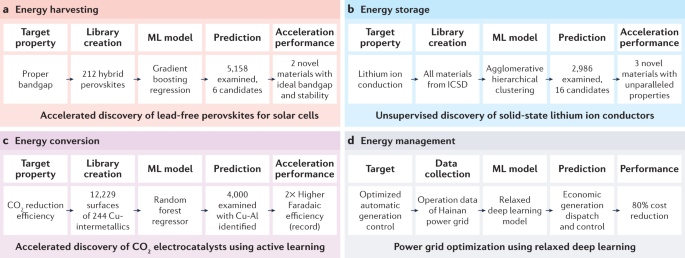
a | Energy harvesting 23 . b | Energy storage 38 . c | Energy conversion 76 . d | Energy management 93 . ICSD, Inorganic Crystal Structure Database; ML, machine learning.
Although bandgap prediction is an important first step, this parameter alone is not sufficient to indicate a useful optoelectronic material; other parameters, including electronic defect density and stability, are equally important. Defect energies are addressable with computational methods, but the calculation of defects in structures is extremely computationally expensive, which inhibits the generation of a dataset of defect energies from which an ML model can be trained. To expedite the high-throughput calculation of defect energies, a Python toolkit has been developed 28 that will be pivotal in building a database of defect energies in semiconductors. Researchers can then use ML to predict both the formation energy of defects and the energy levels of these defects. This knowledge will ensure that the materials selected from high-throughput screening will not only have the correct bandgap but will also either be defect-tolerant or defect-resistant, finding use in commercial optoelectronic devices.
Even without access to a large dataset of experimental results, ML can accelerate the discovery of optoelectronic materials. Using a self-driving laboratory approach, the number of experiments required to optimize an organic solar cell can be reduced from 500 to just 60 (ref. 29 ). This robotic synthesis method accelerates the learning rate of the ML models and drastically reduces the cost of the chemicals needed to run the optimization.
Solar device structure and fabrication
Photovoltaic devices require optimization of layers other than the active layer to maximize performance. One component is the top transparent conductive layer, which needs to have both high optical transparency and high electronic conductivity 30 , 31 . A genetic algorithm that optimized the topology of a light-trapping structure enabled a broadband absorption efficiency of 48.1%, which represents a more than threefold increase over the Yablonovitch limit, the 4 n 2 factor (where n is the refractive index of the material) theoretical limit for light trapping in photovoltaics 32 .
A universal standard irradiance spectrum is usually used by researchers to determine optimal bandgaps for solar cell operation 33 . However, actual solar irradiance fluctuates based on factors such as the position of the Sun, atmospheric phenomena and the season. ML can reduce yearly spectral sets into a few characteristic spectra 33 , allowing for the calculation of optimal bandgaps for real-world conditions.
To optimize device fabrication, a CNN was used to predict the current–voltage characteristics of as-cut Si wafers based on their photoluminescence images 34 . Additionally, an artificial neural network was used to predict the contact resistance of metallic front contacts for Si solar cells, which is critical for the manufacturing process 35 .
Although successful, these studies appear to be limited to optimizing structures and processes that are already well established. We suggest that, in future work, ML could be used to augment simulations, such as the multiphysics models for solar cells. Design of device architecture could begin from such simulation models, coupled with ML in an iterative process to quickly optimize design and reduce computational time and cost. In addition, optimal conditions for the scaling-up of device area and fabrication processes are likely to be very different from those for laboratory-scale demonstrations. However, determining these optimal conditions could be expensive in terms of materials cost and time, owing to the need to construct much larger devices. In this regard, ML, together with the strategic design of experiments, could greatly accelerate the optimization of process conditions (such as the annealing temperatures and solvent choice).
Electrochemical energy storage
Electrochemical energy storage is an essential component in applications such as electric vehicles, consumer electronics and stationary power stations. State-of-the-art electrochemical energy storage solutions have varying efficacy in different applications: for example, lithium-ion batteries exhibit excellent energy density and are widely used in electronics and electric vehicles, whereas redox flow batteries have drawn substantial attention for use in stationary power storage. ML approaches have been widely employed in the field of batteries, including for the discovery of new materials such as solid-state ion conductors 36 , 37 , 38 (Fig. 2b ) and redox active electrolytes for redox flow batteries 39 . ML has also aided battery management, for example, through state-of-charge determination 40 , state-of-health evaluation 41 , 42 and remaining-life prediction 43 , 44 .
Electrode and electrolyte materials design
Layered oxide materials, such as LiCoO 2 or LiNi x Mn y Co 1- x - y O 2 , have been used extensively as cathode materials for alkali metal-ion (Li/Na/K) batteries. However, developing new Li-ion battery materials with higher operating voltages, enhanced energy densities and longer lifetimes is of paramount interest. So far, universal design principles for new battery materials remain undefined, and hence different approaches have been explored. Data from the Materials Project have been used to model the electrode voltage profile diagrams for different materials in alkali metal-ion batteries (Na and K) 45 , leading to the proposition of 5,000 different electrode materials with appropriate moderate voltages. ML was also employed to screen 12,000 candidates for solid Li-ion batteries, resulting in the discovery of ten new Li-ion conducting materials 46 , 47 .
Flow batteries consist of active materials dissolved in electrolytes that flow into a cell with electrodes that facilitate redox reactions. Organic flow batteries are of particular interest. In flow batteries, the solubility of the active material in the electrolyte and the charge/discharge stability dictate performance. ML methods have explored the chemical space to find suitable electrolytes for organic redox flow batteries 48 , 49 . Furthermore, a multi-kernel-ridge regression method accelerated the discovery of active organic molecules using multiple feature training 48 . This method also helped in predicting the solubility dependence of anthraquinone molecules with different numbers and combinations of sulfonic and hydroxyl groups on pH. Future opportunities lie in the exploration of large combinatorial spaces for the inverse design of high-entropy electrodes 50 and high-voltage electrolytes 51 . To this end, deep generative models can assist the discovery of new materials based on the simplified molecular input line entry system (SMILES) representation of molecules 52 .
Battery device and stack management
A combination of mechanistic and semi-empirical models is currently used to estimate capacity and power loss in lithium-ion batteries. However, the models are applicable only to specific failure mechanisms or situations and cannot predict the lifetimes of batteries at the early stages of usage. By contrast, mechanism-agnostic models based on ML can accurately predict battery cycle life, even at an early stage of a battery’s life 43 . A combined early-prediction and Bayesian optimization model has been used to rapidly identify the optimal charging protocol with the longest cycle life 44 . ML can be used to accelerate the optimization of lithium-ion batteries for longer lifetimes 53 , but it remains to be seen whether these models can be generalized to different battery chemistries 54 .
ML methods can also predict important properties of battery storage facilities. A neural network was used to predict the charge/discharge profiles in two types of stationary battery systems, lithium iron phosphate and vanadium redox flow batteries 55 . Battery power management techniques must also consider the uncertainty and variability that arise from both the environment and the application. An iterative Q -learning ( reinforcement learning ) method was also designed for battery management and control in smart residential environments 56 . Given the residential load and the real-time electricity rate, the method is effective at optimizing battery charging/discharging/idle cycles. Discriminative neural network-based models can also optimize battery usage in electric vehicles 57 .
Although ML is able to predict the lifetime of batteries, the underlying degradation mechanisms are difficult to identify and correlate to the state of health and lifetime. To this end, incorporation of domain knowledge into a hybrid physics-based ML model can provide insight and reduce overfitting 53 . However, incorporating the physics of battery degradation processes into a hybrid model remains challenging; representation of electrode materials that encode both compositional and structural information is far from trivial. Validation of these models also requires the development of operando characterization techniques, such as liquid-phase transmission electron microscopy and ambient-pressure X-ray absorption spectroscopy (XAS), that reflect true operating conditions as closely as possible 54 . Ideally, these characterization techniques should be carried out in a high-throughput manner, using automated sample changers, for example, in order to generate large datasets for ML.
Electrocatalysts
Electrocatalysis enables the conversion of simple feedstocks (such as water, carbon dioxide and nitrogen) into valuable chemicals and/or fuels (such as hydrogen, hydrocarbons and ammonia), using renewable energy as an input 58 . The reverse reactions are also possible in a fuel cell, and hydrogen can be consumed to produce electricity 59 . Active and selective electrocatalysts must be developed to improve the efficiency of these reactions 60 , 61 . ML has been used to accelerate electrocatalyst development and device optimization.
Electrocatalyst materials discovery
The most common descriptor of catalytic activity is the adsorption energy of intermediates on a catalyst 61 , 62 . Although these adsorption energies can be calculated using density functional theory (DFT), catalysts possess multiple surface binding sites, each with different adsorption energies 63 . The number of possible sites increases dramatically if alloys are considered, and thus becomes intractable with conventional means 64 .
DFT calculations are critical for the search of electrocatalytic materials 65 and efforts have been made to accelerate the calculations and to reduce their computational cost by using surrogate ML models 66 , 67 , 68 , 69 . Complex reaction mechanisms involving hundreds of possible species and intermediates can also be simplified using ML, with a surrogate model predicting the most important reaction steps and deducing the most likely reaction pathways 70 . ML can also be used to screen for active sites across a random, disordered nanoparticle surface 71 , 72 . DFT calculations are performed on only a few representative sites, which are then used to train a neural network to predict the adsorption energies of all active sites.
Catalyst development can benefit from high-throughput systems for catalyst synthesis and performance evaluation 73 , 74 . An automatic ML-driven framework was developed to screen a large intermetallic chemical space for CO 2 reduction and H 2 evolution 75 . The model predicted the adsorption energy of new intermetallic systems and DFT was automatically performed on the most promising candidates to verify the predictions. This process went on iteratively in a closed feedback loop. 131 intermetallic surfaces across 54 alloys were ultimately identified as promising candidates for CO 2 reduction. Experimental validation 76 with Cu–Al catalysts yielded an unprecedented Faradaic efficiency of 80% towards ethylene at a high current density of 400 mA cm – 2 (Fig. 2c ).
Because of the large number of properties that electrocatalysts may possess (such as shape, size and composition), it is difficult to do data mining on the literature 77 . Electrocatalyst structures are complex and difficult to characterize completely; as a result, many properties may not be fully characterized by research groups in their publications. To avoid situations in which potentially promising compositions perform poorly as a result of non-ideal synthesis or testing conditions, other factors (such as current density, particle size and pH value) that affect the electrocatalyst performance must be kept consistent. New approaches such as carbothermal shock synthesis 78 , 79 may be a promising avenue, owing to its propensity to generate uniformly sized and shaped alloy nanoparticles, regardless of composition.
XAS is a powerful technique, especially for in situ measurements, and has been widely employed to gain crucial insight into the nature of active sites and changes in the electrocatalyst over time 80 . Because the data analysis relies heavily on human experience and expertise, there has been interest in developing ML tools for interpreting XAS data 81 . Improved random forest models can predict the Bader charge (a good approximation of the total electronic charge of an atom) and nearest-neighbour distances, crucial factors that influence the catalytic properties of the material 82 . The extended X-ray absorption fine structure (EXAFS) region of XAS spectra is known to contain information on bonding environments and coordination numbers. Neural networks can be used to automatically interpret EXAFS data 83 , permitting the identification of the structure of bimetallic nanoparticles using experimental XAS data, for example 84 . Raman and infrared spectroscopy are also important tools for the mechanistic understanding of electrocatalysis. Together with explainable artificial intelligence (AI), which can relate the results to underlying physics, these analyses could be used to discover descriptors hidden in spectra that could lead to new breakthroughs in electrocatalyst discovery and optimization.
Fuel cell and electrolyser device management
A fuel cell is an electrochemical device that can be used to convert the chemical energy of a fuel (such as hydrogen) into electrical energy. An electrolyser transforms electrical energy into chemical energy (such as in water splitting to generate hydrogen). ML has been used to optimize and manage their performance, predict degradation and device lifetime as well as detect and diagnose faults. Using a hybrid method consisting of an extreme learning machine, genetic algorithms and wavelet analysis, the degradation in proton-exchange membrane fuel cells has been predicted 85 , 86 . Electrochemical impedance measurements used as input for an artificial neural network have enabled fault detection and isolation in a high-temperature stack of proton-exchange membrane fuel cells 87 , 88 .
ML approaches can also be employed to diagnose faults, such as fuel and air leakage issues, in solid oxide fuel cell stacks. Artificial neural networks can predict the performance of solid oxide fuel cells under different operating conditions 89 . In addition, ML has been applied to optimize the performance of solid oxide electrolysers, for CO 2 /H 2 O reduction 90 , and chloralkali electrolysers 91 .
In the future, the use of ML for fuel cells could be combined with multiscale modelling to improve their design, for example to minimize Ohmic losses and optimize catalyst loading. For practical applications, fuel cells may be subject to fluctuations in energy output requirements (for example, when used in vehicles). ML models could be used to determine the effects of such fluctuations on the long-term durability and performance of fuel cells, similar to what has been done for predicting the state of health and lifetime for batteries. Furthermore, it remains to be seen whether the ML techniques for fuel cells can be easily generalized to electrolysers and vice versa, using transfer learning for example, given that they are essentially reactions in reverse.
Smart power grids
A power grid is responsible for delivering electrical energy from producers (such as power plants and solar farms) to consumers (such as homes and offices). However, energy fluctuations from intermittent renewable energy generators can render the grid vulnerable 92 . ML algorithms can be used to optimize the automatic generation control of power grids, which controls the power output of multiple generators in an energy system. For example, when a relaxed deep learning model was used as a unified timescale controller for the automatic generation control unit, the total operational cost was reduced by up to 80% compared with traditional heuristic control strategies 93 (Fig. 2d ). A smart generation control strategy based on multi-agent reinforcement learning was found to improve the control performance by around 10% compared with other ML algorithms 94 .
Accurate demand and load prediction can support decision-making operations in energy systems for proper load scheduling and power allocation. Multiple ML methods have been proposed to precisely predict the demand load: for example, long short-term memory was used to successfully and accurately predict hourly building load 95 . Short-term load forecasting of diverse customers (such as retail businesses) using a deep neural network and cross-building energy demand forecasting using a deep belief network have also been demonstrated effectively 96 , 97 .
Demand-side management consists of a set of mechanisms that shape consumer electricity consumption by dynamically adjusting the price of electricity. These include reducing (peak shaving), increasing (load growth) and rescheduling (load shifting) the energy demand, which allows for flexible balancing of renewable electricity generation and load 98 . A reinforcement-learning-based algorithm resulted in substantial cost reduction for both the service provider and customer 99 . A decentralized learning-based residential demand scheduling technique successfully shifted up to 35% of the energy demand to periods of high wind availability, substantially saving power costs compared with the unscheduled energy demand scenario 100 . Load forecasting using a multi-agent approach integrates load prediction with reinforcement learning algorithms to shift energy usage (for example, to different electrical devices in a household) for its optimization 101 . This approach reduced peak usage by more than 30% and increased off-peak usage by 50%, reducing the cost and energy losses associated with energy storage.
Opportunities for ML in renewable energy
ML provides the opportunity to enable substantial further advances in different areas of the energy materials field, which share similar materials-related challenges (Fig. 3 ). There are also grand challenges for ML application in smart grid and policy optimization.
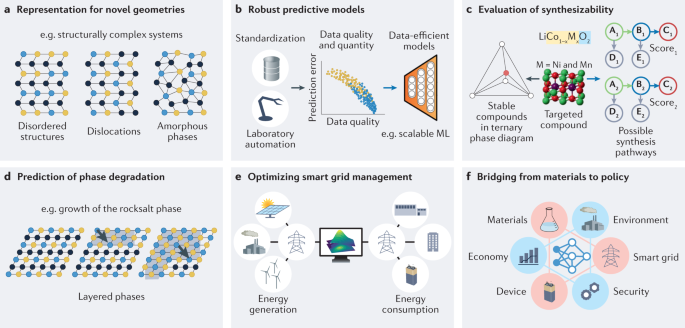
a | Energy materials present additional modelling challenges. Machine learning (ML) could help in the representation of structurally complex structures, which can include disordering, dislocations and amorphous phases. b | Flexible models that scale efficiently with varied dataset sizes are in demand, and ML could help to develop robust predictive models. The yellow dots stand for the addition of unreliable datasets that could harm the prediction accuracy of the ML model. c | Synthesis route prediction remains to be solved for the design of a novel material. In the ternary phase diagram, the dots stand for the stable compounds in that corresponding phase space and the red dot for the targeted compound. Two possible synthesis pathways are compared for a single compound. The score obtained would reflect the complexity, cost and so on of one synthesis pathway. d | ML-aided phase degradation prediction could boost the development of materials with enhanced cyclability. The shaded region represents the rocksalt phase, which grows inside the layered phase. The arrow marks the growth direction. e | The use of ML models could help in optimizing energy generation and energy consumption. Automating the decision-making processes associated with dynamic power supplies using ML will make the power distribution more efficient. f | Energy policy is the manner in which an entity (for example, a government) addresses its energy issues, including conversion, distribution and utilization, where ML could be used to optimize the corresponding economy.
Materials with novel geometries
A ML representation is effective when it captures the inherent properties of the system (such as its physical symmetries) and can be utilized in downstream ancillary tasks, such as transfer learning to new predictive tasks, building new knowledge using visualization or attribution and generating similar data distributions with generative models 102 .
For materials, the inputs are molecules or crystal structures whose physical properties are modelled by the Schrödinger equation. Designing a general representation of materials that reflects these properties is an ongoing research problem. For molecular systems, several representations have been used successfully, including fingerprints 103 , SMILES 104 , self-referencing embedded strings (SELFIES) 105 and graphs 106 , 107 , 108 . Representing crystalline materials has the added complexity of needing to incorporate periodicity in the representation. Methods like the smooth overlap of atomic positions 109 , Voronoi tessellation 110 , 111 , diffraction images 112 , multi-perspective fingerprints 113 and graph-based algorithms 27 , 114 have been suggested, but typically lack the capability for structure reconstruction.
Complex structural systems found in energy materials present additional modelling challenges (Fig. 3a ): a large number of atoms (such as in reticular frameworks or polymers), specific symmetries (such as in molecules with a particular space group and for reticular frameworks belonging to a certain topology), atomic disordering, partial occupancy, or amorphous phases (leading to an enormous combinatorial space), defects and dislocations (such as interfaces and grain boundaries) and low-dimensionality materials (as in nanoparticles). Reduction approximations alleviate the first issue (using, for example, RFcode for reticular framework representation) 8 , but the remaining several problems warrant intensive future research efforts.
Self- supervised learning , which seeks to lever large amounts of synthetic labels and tasks to continue learning without experimental labels 115 , multi-task learning 116 , in which multiple material properties can be modelled jointly to exploit correlation structure between properties, and meta-learning 117 , which looks at strategies that allow models to perform better in new datasets or in out-of-distribution data, all offer avenues to build better representations. On the modelling front, new advances in attention mechanisms 118 , 119 , graph neural networks 120 and equivariant neural networks 121 expand our range of tools with which to model interactions and expected symmetries.
Robust predictive models
Predictive models are the first step when building a pipeline that seeks materials with desired properties. A key component for building these models is training data; more data will often translate into better-performing models, which in turn will translate into better accuracy in the prediction of new materials. Deep learning models tend to scale more favourably with dataset size than traditional ML approaches (such as random forests). Dataset quality is also essential. However, experiments are usually conducted under diverse conditions with large variation in untracked variables (Fig. 3b ). Additionally, public datasets are more likely to suffer from publication bias, because negative results are less likely to be published even though they are just as important as positive results when training statistical models 122 .
Addressing these issues require transparency and standardization of the experimental data reported in the literature. Text and natural language processing strategies could then be employed to extract data from the literature 77 . Data should be reported with the belief that it will eventually be consolidated in a database, such as the MatD3 database 123 . Autonomous laboratory techniques will help to address this issue 19 , 124 . Structured property databases such as the Materials Project 122 and the Harvard Clean Energy Project 125 can also provide a large amount of data. Additionally, different energy fields — energy storage, harvesting and conversion — should converge upon a standard and uniform way to report data. This standard should be continuously updated; as researchers continue to learn about the systems they are studying, conditions that were previously thought to be unimportant will become relevant.
New modelling approaches that work in low-data regimes, such as data-efficient models, dataset-building strategies (active sampling) 126 and data-augmentation techniques, are also important 127 . Uncertainty quantification , data efficiency, interpretability and regularization are important considerations that improve the robustness of ML models. These considerations relate to the notion of generalizability: predictions should generalize to a new class of materials that is out of the distribution of the original dataset. Researchers can attempt to model how far away new data points are from the training set 128 or the variability in predicted labels with uncertainty quantification 129 . Neural networks are a flexible model class, and often models can be underspecified 130 . Incorporating regularization, inductive biases or priors can boost the credibility of a model. Another way to create trustable models could be to enhance the interpretability of ML algorithms by deriving feature relevance and scoring their importance 131 . This strategy could help to identify potential chemically meaningful features and form a starting point for understanding latent factors that dominate material properties. These techniques can also identify the presence of model bias and overfitting, as well as improving generalization and performance 132 , 133 , 134 .
Stable and synthesizable new materials
The formation energy of a compound is used to estimate its stability and synthesizability 135 , 136 . Although negative values usually correspond to stable or synthesizable compounds, slightly positive formation energies below a limit lead to metastable phases with unclear synthesizability 137 , 138 . This is more apparent when investigating unexplored chemical spaces with undetermined equilibrium ground states; yet often the metastable phases exhibit superior properties, as seen in photovoltaics 136 , 139 and ion conductors 140 , for example. It is thus of interest to develop a method to evaluate the synthesizability of metastable phases (Fig. 3c ). Instead of estimating the probability that a particular phase can be synthesized, one can instead evaluate its synthetic complexity using ML. In organic chemistry, synthesis complexity is evaluated according to the accessibility of the phases’ synthesis route 141 or precedent reaction knowledge 142 . Similar methodologies can be applied to the inorganic field with the ongoing design of automated synthesis-planning algorithms for inorganic materials 143 , 144 .
Synthesis and evaluation of a new material alone does not ensure that material will make it to market; material stability is a crucial property that takes a long time to evaluate. Degradation is a generally complex process that occurs through the loss of active matter or growth of inactive phases (such as the rocksalt phases formed in layered Li-ion battery electrodes 145 (Fig. 3d ) or the Pt particle agglomeration in fuel cells 146 ) and/or propagation of defects (such as cracks in cycled battery electrode 147 ). Microscopies such as electron microscopy 148 and simulations such as continuum mechanics modelling 149 are often used to investigate growth and propagation dynamics (that is, phase boundary and defect surface movements versus time). However, these techniques are usually expensive and do not allow rapid degradation prediction. Deep learning techniques such as convolutional neural networks and recurrent neural networks may be able to predict the phase boundary and/or defect pattern evolution under certain conditions after proper training 150 . Similar models can then be built to understand multiple degradation phenomena and aid the design of materials with improved cycle life.
Optimized smart power grids
A promising prospect of ML in smart grids is automating the decision-making processes that are associated with dynamic power supplies to distribute power most efficiently (Fig. 3e ). Practical deployment of ML technologies into physical systems remains difficult because of data scarcity and the risk-averse mindset of policymakers. The collection of and access to large amounts of diverse data is challenging owing to high cost, long delays and concerns over compliance and security 151 . For instance, to capture the variation of renewable resources owing to peak or off-peak and seasonal attributes, long-term data collections are implemented for periods of 24 hours to several years 152 . Furthermore, although ML algorithms are ideally supposed to account for all uncertainties and unpredictable situations in energy systems, the risk-adverse mindset in the energy management industry means that implementation still relies on human decision-making 153 .
An ML-based framework that involves a digital twin of the physical system can address these problems 154 , 155 . The digital twin represents the digitalized cyber models of the physical system and can be constructed from physical laws and/or ML models trained using data sampled from the physical system. This approach aims to accurately simulate the dynamics of the physical system, enabling relatively fast generation of large amounts of high-quality synthetic data at low cost. Notably, because ML model training and validation is performed on the digital twin, there is no risk to the actual physical system. Based on the prediction results, suitable actions can be suggested and then implemented in the physical system to ensure stability and/or improve system operation.
Policy optimization
Finally, research is generally focused on one narrow aspect of a larger problem; we argue that energy research needs a more integrated approach 156 (Fig. 3f ). Energy policy is the manner in which an entity, such as the government, addresses its energy issues, including conversion, distribution and utilization. ML has been used in the fields of energy economics finance for performance diagnostics (such as for oil wells), energy generation (such as wind power) and consumption (such as power load) forecasts and system lifespan (such as battery cell life) and failure (such as grid outage) prediction 157 . They have also been used for energy policy analysis and evaluation (for example, for estimating energy savings). A natural extension of ML models is to use them for policy optimization 158 , 159 , a concept that has not yet seen widespread use. We posit that the best energy policies — including the deployment of the newly discovered materials — can be improved and augmented with ML and should be discussed in research reporting accelerated energy technology platforms.
Conclusions
To summarize, ML has the potential to enable breakthroughs in the development and deployment of sustainable energy techniques. There have been remarkable achievements in many areas of energy technology, from materials design and device management to system deployment. ML is particularly well suited to discovering new materials, and researchers in the field are expecting ML to bring up new materials that may revolutionize the energy industry. The field is still nascent, but there is conclusive evidence that ML is at least able to expose the same trends that human researchers have noticed over decades of research. The ML field itself is still seeing rapid development, with new methodologies being reported daily. It will take time to develop and adopt these methodologies to solve specific problems in materials science. We believe that for ML to truly accelerate the deployment of sustainable energy, it should be deployed as a tool, similar to a synthesis procedure, characterization equipment or control apparatus. Researchers using ML to accelerate energy technology discovery should judge the success of the method primarily on the advances it enables. To this end, we have proposed the XPIs and some areas in which we hope to see ML deployed.
Davidson, D. J. Exnovating for a renewable energy transition. Nat. Energy 4 , 254–256 (2019).
Article Google Scholar
Horowitz, C. A. Paris agreement. Int. Leg. Mater. 55 , 740–755 (2016).
International Energy Agency 2018 World Energy Outlook: Executive Summary https://www.iea.org/reports/world-energy-outlook-2018 (OECD/IEA, 2018).
Chu, S., Cui, Y. & Liu, N. The path towards sustainable energy. Nat. Mater. 16 , 16–22 (2017).
Maine, E. & Garnsey, E. Commercializing generic technology: the case of advanced materials ventures. Res. Policy 35 , 375–393 (2006).
De Luna, P., Wei, J., Bengio, Y., Aspuru-Guzik, A. & Sargent, E. Use machine learning to find energy materials. Nature 552 , 23–27 (2017).
Wang, H., Lei, Z., Zhang, X., Zhou, B. & Peng, J. A review of deep learning for renewable energy forecasting. Energy Convers. Manag. 198 , 111799–111814 (2019).
Yao, Z. et al. Inverse design of nanoporous crystalline reticular materials with deep generative models. Nat. Mach. Intell. 3 , 76–86 (2021).
Rosen, A. S. et al. Machine learning the quantum-chemical properties of metal–organic frameworks for accelerated materials discovery. Matter 4 , 1578–1597 (2021).
Article CAS Google Scholar
Jordan, M. I. & Mitchell, T. M. Machine learning: trends, perspectives, and prospects. Science 349 , 255–260 (2015).
Personal, E., Guerrero, J. I., Garcia, A., Peña, M. & Leon, C. Key performance indicators: a useful tool to assess Smart Grid goals. Energy 76 , 976–988 (2014).
Helmus, J. & den Hoed, R. Key performance indicators of charging infrastructure. World Electr. Veh. J. 8 , 733–741 (2016).
Struck, M.-M. Vaccine R&D success rates and development times. Nat. Biotechnol. 14 , 591–593 (1996).
Moore, G. E. Cramming more components onto integrated circuits. Electronics 38 , 114–116 (1965).
Google Scholar
Wetterstrand, K. A. DNA sequencing costs: data. NHGRI Genome Sequencing Program (GSP) www.genome.gov/sequencingcostsdata (2020).
Rajkomar, A., Dean, J. & Kohane, I. Machine learning in medicine. N. Engl. J. Med. 380 , 1347–1358 (2019).
Jeong, J. et al. Pseudo-halide anion engineering for α-FAPbI3 perovskite solar cells. Nature 592 , 381–385 (2021).
NREL. Best research-cell efficiency chart. NREL https://www.nrel.gov/pv/cell-efficiency.html (2021).
Burger, B. et al. A mobile robotic chemist. Nature 583 , 237–241 (2020).
Clark, M. A. et al. Design, synthesis and selection of DNA-encoded small-molecule libraries. Nat. Chem. Biol. 5 , 647–654 (2009).
Pilania, G., Gubernatis, J. E. & Lookman, T. Multi-fidelity machine learning models for accurate bandgap predictions of solids. Comput. Mater. Sci. 129 , 156–163 (2017).
Pilania, G. et al. Machine learning bandgaps of double perovskites. Sci. Rep. 6 , 19375 (2016).
Lu, S. et al. Accelerated discovery of stable lead-free hybrid organic-inorganic perovskites via machine learning. Nat. Commun. 9 , 3405 (2018).
Askerka, M. et al. Learning-in-templates enables accelerated discovery and synthesis of new stable double perovskites. J. Am. Chem. Soc. 141 , 3682–3690 (2019).
Jain, A. & Bligaard, T. Atomic-position independent descriptor for machine learning of material properties. Phys. Rev. B 98 , 214112 (2018).
Choubisa, H. et al. Crystal site feature embedding enables exploration of large chemical spaces. Matter 3 , 433–448 (2020).
Xie, T. & Grossman, J. C. Crystal graph convolutional neural networks for an accurate and interpretable prediction of material properties. Phys. Rev. Lett. 120 , 145301–145306 (2018).
Broberg, D. et al. PyCDT: a Python toolkit for modeling point defects in semiconductors and insulators. Comput. Phys. Commun. 226 , 165–179 (2018).
Roch, L. M. et al. ChemOS: an orchestration software to democratize autonomous discovery. PLoS ONE 15 , 1–18 (2020).
Wei, L., Xu, X., Gurudayal, Bullock, J. & Ager, J. W. Machine learning optimization of p-type transparent conducting films. Chem. Mater. 31 , 7340–7350 (2019).
Schubert, M. F. et al. Design of multilayer antireflection coatings made from co-sputtered and low-refractive-index materials by genetic algorithm. Opt. Express 16 , 5290–5298 (2008).
Wang, C., Yu, S., Chen, W. & Sun, C. Highly efficient light-trapping structure design inspired by natural evolution. Sci. Rep. 3 , 1025 (2013).
Ripalda, J. M., Buencuerpo, J. & García, I. Solar cell designs by maximizing energy production based on machine learning clustering of spectral variations. Nat. Commun. 9 , 5126 (2018).
Demant, M., Virtue, P., Kovvali, A., Yu, S. X. & Rein, S. Learning quality rating of As-Cut mc-Si wafers via convolutional regression networks. IEEE J. Photovolt. 9 , 1064–1072 (2019).
Musztyfaga-Staszuk, M. & Honysz, R. Application of artificial neural networks in modeling of manufactured front metallization contact resistance for silicon solar cells. Arch. Metall. Mater. 60 , 1673–1678 (2015).
Sendek, A. D. et al. Holistic computational structure screening of more than 12000 candidates for solid lithium-ion conductor materials. Energy Environ. Sci. 10 , 306–320 (2017).
Ahmad, Z., Xie, T., Maheshwari, C., Grossman, J. C. & Viswanathan, V. Machine learning enabled computational screening of inorganic solid electrolytes for suppression of dendrite formation in lithium metal anodes. ACS Cent. Sci. 4 , 996–1006 (2018).
Zhang, Y. et al. Unsupervised discovery of solid-state lithium ion conductors. Nat. Commun. 10 , 5260 (2019).
Doan, H. A. et al. Quantum chemistry-informed active learning to accelerate the design and discovery of sustainable energy storage materials. Chem. Mater. 32 , 6338–6346 (2020).
Chemali, E., Kollmeyer, P. J., Preindl, M. & Emadi, A. State-of-charge estimation of Li-ion batteries using deep neural networks: a machine learning approach. J. Power Sources 400 , 242–255 (2018).
Richardson, R. R., Osborne, M. A. & Howey, D. A. Gaussian process regression for forecasting battery state of health. J. Power Sources 357 , 209–219 (2017).
Berecibar, M. et al. Online state of health estimation on NMC cells based on predictive analytics. J. Power Sources 320 , 239–250 (2016).
Severson, K. A. et al. Data-driven prediction of battery cycle life before capacity degradation. Nat. Energy 4 , 383–391 (2019).
Attia, P. M. et al. Closed-loop optimization of fast-charging protocols for batteries with machine learning. Nature 578 , 397–402 (2020).
Joshi, R. P. et al. Machine learning the voltage of electrode materials in metal-ion batteries. ACS Appl. Mater. Interf. 11 , 18494–18503 (2019).
Cubuk, E. D., Sendek, A. D. & Reed, E. J. Screening billions of candidates for solid lithium-ion conductors: a transfer learning approach for small data. J. Chem. Phys. 150 , 214701 (2019).
Sendek, A. D. et al. Machine learning-assisted discovery of solid Li-ion conducting materials. Chem. Mater. 31 , 342–352 (2019).
Kim, S., Jinich, A. & Aspuru-Guzik, A. MultiDK: a multiple descriptor multiple kernel approach for molecular discovery and its application to organic flow battery electrolytes. J. Chem. Inf. Model. 57 , 657–668 (2017).
Jinich, A., Sanchez-Lengeling, B., Ren, H., Harman, R. & Aspuru-Guzik, A. A mixed quantum chemistry/machine learning approach for the fast and accurate prediction of biochemical redox potentials and its large-scale application to 315000 redox reactions. ACS Cent. Sci. 5 , 1199–1210 (2019).
Sarkar, A. et al. High entropy oxides for reversible energy storage. Nat. Commun. 9 , 3400 (2018).
Choudhury, S. et al. Stabilizing polymer electrolytes in high-voltage lithium batteries. Nat. Commun. 10 , 3091 (2019).
Sanchez-Lengeling, B. & Aspuru-Guzik, A. Inverse molecular design using machine learning: generative models for matter engineering. Science 361 , 360–365 (2018).
Ng, M.-F., Zhao, J., Yan, Q., Conduit, G. J. & Seh, Z. W. Predicting the state of charge and health of batteries using data-driven machine learning. Nat. Mach. Intell. 2 , 161–170 (2020).
Steinmann, S. N. & Seh, Z. W. Understanding electrified interfaces. Nat. Rev. Mater. 6 , 289–291 (2021).
Kandasamy, N., Badrinarayanan, R., Kanamarlapudi, V., Tseng, K. & Soong, B.-H. Performance analysis of machine-learning approaches for modeling the charging/discharging profiles of stationary battery systems with non-uniform cell aging. Batteries 3 , 18 (2017).
Wei, Q., Liu, D. & Shi, G. A novel dual iterative Q-learning method for optimal battery management in smart residential environments. IEEE Trans. Ind. Electron. 62 , 2509–2518 (2015).
Murphey, Y. L. et al. Intelligent hybrid vehicle power control — Part II: online intelligent energy management. IEEE Trans. Vehicular Technol. 62 , 69–79 (2013).
Seh, Z. W. et al. Combining theory and experiment in electrocatalysis: insights into materials design. Science 355 , eaad4998 (2017).
Staffell, I. et al. The role of hydrogen and fuel cells in the global energy system. Energy Environ. Sci. 12 , 463–491 (2019).
Montoya, J. H. H. et al. Materials for solar fuels and chemicals. Nat. Mater. 16 , 70–81 (2017).
Pérez-Ramírez, J. & López, N. Strategies to break linear scaling relationships. Nat. Catal. 2 , 971–976 (2019).
Shi, C., Hansen, H. A., Lausche, A. C. & Norskov, J. K. Trends in electrochemical CO 2 reduction activity for open and close-packed metal surfaces. Phys. Chem. Chem. Phys. 16 , 4720–4727 (2014).
Calle-Vallejo, F., Loffreda, D., Koper, M. T. M. & Sautet, P. Introducing structural sensitivity into adsorption-energy scaling relations by means of coordination numbers. Nat. Chem. 7 , 403–410 (2015).
Ulissi, Z. W. et al. Machine-learning methods enable exhaustive searches for active bimetallic facets and reveal active site motifs for CO 2 reduction. ACS Catal. 7 , 6600–6608 (2017).
Nørskov, J. K., Studt, F., Abild-Pedersen, F. & Bligaard, T. Activity and selectivity maps. In Fundamental Concepts in Heterogeneous Catalysis 97–113 (John Wiley, 2014).
Garijo del Río, E., Mortensen, J. J. & Jacobsen, K. W. Local Bayesian optimizer for atomic structures. Phys. Rev. B 100 , 104103 (2019).
Jørgensen, M. S., Larsen, U. F., Jacobsen, K. W. & Hammer, B. Exploration versus exploitation in global atomistic structure optimization. J. Phys. Chem. A 122 , 1504–1509 (2018).
Jacobsen, T. L., Jørgensen, M. S. & Hammer, B. On-the-fly machine learning of atomic potential in density functional theory structure optimization. Phys. Rev. Lett. 120 , 026102 (2018).
Peterson, A. A. Acceleration of saddle-point searches with machine learning. J. Chem. Phys. 145 , 074106 (2016).
Ulissi, Z. W., Medford, A. J., Bligaard, T. & Nørskov, J. K. To address surface reaction network complexity using scaling relations machine learning and DFT calculations. Nat. Commun. 8 , 14621 (2017).
Huang, Y., Chen, Y., Cheng, T., Wang, L.-W. & Goddard, W. A. Identification of the selective sites for electrochemical reduction of CO to C 2+ products on copper nanoparticles by combining reactive force fields, density functional theory, and machine learning. ACS Energy Lett. 3 , 2983–2988 (2018).
Chen, Y., Huang, Y., Cheng, T. & Goddard, W. A. Identifying active sites for CO 2 reduction on dealloyed gold surfaces by combining machine learning with multiscale simulations. J. Am. Chem. Soc. 141 , 11651–11657 (2019).
Lai, Y., Jones, R. J. R., Wang, Y., Zhou, L. & Gregoire, J. M. Scanning electrochemical flow cell with online mass spectroscopy for accelerated screening of carbon dioxide reduction electrocatalysts. ACS Comb. Sci. 21 , 692–704 (2019).
Lai, Y. et al. The sensitivity of Cu for electrochemical carbon dioxide reduction to hydrocarbons as revealed by high throughput experiments. J. Mater. Chem. A 7 , 26785–26790 (2019).
Tran, K. & Ulissi, Z. W. Active learning across intermetallics to guide discovery of electrocatalysts for CO 2 reduction and H 2 evolution. Nat. Catal. 1 , 696–703 (2018).
Zhong, M. et al. Accelerated discovery of CO 2 electrocatalysts using active machine learning. Nature 581 , 178–183 (2020).
Tshitoyan, V. et al. Unsupervised word embeddings capture latent knowledge from materials science literature. Nature 571 , 95–98 (2019).
Yao, Y. et al. Carbothermal shock synthesis of high-entropy-alloy nanoparticles. Science 359 , 1489–1494 (2018).
Yao, Y. et al. High-throughput, combinatorial synthesis of multimetallic nanoclusters. Proc. Natl Acad. Sci. USA 117 , 6316–6322 (2020).
Timoshenko, J. & Roldan Cuenya, B. In situ/operando electrocatalyst characterization by X-ray absorption spectroscopy. Chem. Rev. 121 , 882–961 (2021).
Zheng, C., Chen, C., Chen, Y. & Ong, S. P. Random forest models for accurate identification of coordination environments from X-ray absorption near-edge structure. Patterns 1 , 100013–100023 (2020).
Torrisi, S. B. et al. Random forest machine learning models for interpretable X-ray absorption near-edge structure spectrum-property relationships. npj Comput. Mater. 6 , 109 (2020).
Timoshenko, J. et al. Neural network approach for characterizing structural transformations by X-ray absorption fine structure spectroscopy. Phys. Rev. Lett. 120 , 225502 (2018).
Marcella, N. et al. Neural network assisted analysis of bimetallic nanocatalysts using X-ray absorption near edge structure spectroscopy. Phys. Chem. Chem. Phys. 22 , 18902–18910 (2020).
Chen, K., Laghrouche, S. & Djerdir, A. Degradation model of proton exchange membrane fuel cell based on a novel hybrid method. Appl. Energy 252 , 113439–113447 (2019).
Ma, R. et al. Data-driven proton exchange membrane fuel cell degradation predication through deep learning method. Appl. Energy 231 , 102–115 (2018).
Jeppesen, C. et al. Fault detection and isolation of high temperature proton exchange membrane fuel cell stack under the influence of degradation. J. Power Sources 359 , 37–47 (2017).
Liu, J. et al. Sequence fault diagnosis for PEMFC water management subsystem using deep learning with t-SNE. IEEE Access. 7 , 92009–92019 (2019).
Ansari, M. A., Rizvi, S. M. A. & Khan, S. Optimization of electrochemical performance of a solid oxide fuel cell using artificial neural network. in 2016 International Conference on Electrical, Electronics, and Optimization Techniques (ICEEOT) 4230–4234 (IEEE, 2016).
Zhang, C. et al. Modelling of solid oxide electrolyser cell using extreme learning machine. Electrochim. Acta 251 , 137–144 (2017).
Esche, E., Weigert, J., Budiarto, T., Hoffmann, C. & Repke, J.-U. Optimization under uncertainty based on a data-driven model for a chloralkali electrolyzer cell. Computer-aided Chem. Eng. 46 , 577–582 (2019).
Siddaiah, R. & Saini, R. P. A review on planning, configurations, modeling and optimization techniques of hybrid renewable energy systems for off grid applications. Renew. Sustain. Energy Rev. 58 , 376–396 (2016).
Yin, L., Yu, T., Zhang, X. & Yang, B. Relaxed deep learning for real-time economic generation dispatch and control with unified time scale. Energy 149 , 11–23 (2018).
Yu, T., Wang, H. Z., Zhou, B., Chan, K. W. & Tang, J. Multi-agent correlated equilibrium Q ( λ ) learning for coordinated smart generation control of interconnected power grids. IEEE Trans. Power Syst. 30 , 1669–1679 (2015).
Marino, D. L., Amarasinghe, K. & Manic, M. Building energy load forecasting using deep neural networks. in IECON Proceedings (Industrial Electronics Conference) 7046–7051 (IECON, 2016).
Ryu, S., Noh, J. & Kim, H. Deep neural network based demand side short term load forecasting. in 2016 IEEE International Conference on Smart Grid Communications (SmartGridComm 2016) 308–313 (IEEE, 2016).
Mocanu, E., Nguyen, P. H., Kling, W. L. & Gibescu, M. Unsupervised energy prediction in a Smart Grid context using reinforcement cross-building transfer learning. Energy Build. 116 , 646–655 (2016).
Lund, P. D., Lindgren, J., Mikkola, J. & Salpakari, J. Review of energy system flexibility measures to enable high levels of variable renewable electricity. Renew. Sustain. Energy Rev. 45 , 785–807 (2015).
Kim, B. G., Zhang, Y., Van Der Schaar, M. & Lee, J. W. Dynamic pricing and energy consumption scheduling with reinforcement learning. IEEE Trans. Smart Grid 7 , 2187–2198 (2016).
Dusparic, I., Taylor, A., Marinescu, A., Cahill, V. & Clarke, S. Maximizing renewable energy use with decentralized residential demand response. in 2015 IEEE 1st International Smart Cities Conference (ISC2 2015 ) 1–6 (IEEE, 2015).
Dusparic, I., Harris, C., Marinescu, A., Cahill, V. & Clarke, S. Multi-agent residential demand response based on load forecasting. in 2013 1st IEEE Conference on Technologies for Sustainability (SusTech 2013) 90–96 (IEEE, 2013).
Bengio, Y., Courville, A. & Vincent, P. Representation learning: a review and new perspectives. IEEE Transactions on Pattern Analysis and Machine Intelligence 35 , 1798–1828 (2013).
Duvenaud, D. et al. Convolutional networks on graphs for learning molecular fingerprints. in Advances In Neural Information Processing Systems 2224–2232 (NIPS, 2015).
Gómez-Bombarelli, R. et al. Automatic chemical design using a data-driven continuous representation of molecules. ACS Cent. Sci. 4 , 268–276 (2018).
Krenn, M., Hase, F., Nigam, A., Friederich, P. & Aspuru-Guzik, A. Self-referencing embedded strings (SELFIES): a 100% robust molecular string representation. Mach. Learn. Sci. Technol. 1 , 045024–045031 (2020).
Jin, W., Barzilay, R. & Jaakkola, T. Junction tree variational autoencoder for molecular graph generation. Mach. Learn. 5 , 3632–3648 (2018).
You, J., Liu, B., Ying, R., Pande, V. & Leskovec, J. Graph convolutional policy network for goal-directed molecular graph generation. Adv. Neural Inf. Process. Syst. 31 , 6412–6422 (2018).
Liu, Q., Allamanis, M., Brockschmidt, M. & Gaunt, A. L. Constrained graph variational autoencoders for molecule design. in Proceedings of the 32nd International Conference on Neural Information Processing Systems (NIPS’18) 7806–7815 (Curran Associates Inc., 2018).
Bartók, A. P., Kondor, R. & Csányi, G. On representing chemical environments. Phys. Rev. B 87 , 184115 (2013).
Ward, L. et al. Including crystal structure attributes in machine learning models of formation energies via Voronoi tessellations. Phys. Rev. B 96 , 024104 (2017).
Isayev, O. et al. Universal fragment descriptors for predicting properties of inorganic crystals. Nat. Commun. 8 , 15679 (2017).
Ziletti, A., Kumar, D., Scheffler, M. & Ghiringhelli, L. M. Insightful classification of crystal structures using deep learning. Nat. Commun. 9 , 2775 (2018).
Ryan, K., Lengyel, J. & Shatruk, M. Crystal structure prediction via deep learning. J. Am. Chem. Soc. 140 , 10158–10168 (2018).
Park, C. W. & Wolverton, C. Developing an improved crystal graph convolutional neural network framework for accelerated materials discovery. Phys. Rev. Mater. 4 , 063801 (2020).
Liu, X. et al. Self-Supervised Learning: Generative or Contrastive (IEEE, 2020).
Ruder, S. An overview of multi-task learning in deep neural networks. Preprint at https://doi.org/10.48550/arXiv.1706.05098 (2017).
Hospedales, T., Antoniou, A., Micaelli, P. & Storkey, A. Meta-learning in neural networks: a survey. IEEE Transactions on Pattern Analysis & Machine Intelligence 44 , 5149–5169 (2020).
Vaswani, A. et al. Attention is all you need. in Proceedings of the 31st International Conference on Neural Information Processing Systems (NIPS’17) 6000–6010 (Curran Associates Inc., 2017).
Veličković, P. et al. Graph attention networks. Preprint at https://doi.org/10.48550/arXiv.1710.10903 (2017).
Battaglia, P. W. et al. Relational inductive biases, deep learning, and graph networks. Preprint at https://doi.org/10.48550/arXiv.1806.01261 (2018).
Satorras, V. G., Hoogeboom, E. & Welling, M. E(n) equivariant graph neural networks. Preprint at https://doi.org/10.48550/arXiv.2102.09844 (2021).
Jain, A. et al. Commentary: The Materials Project: a materials genome approach to accelerating materials innovation. Apl. Mater. 1 , 011002–011012 (2013).
Laasner, R. et al. MatD3: a database and online presentation package for research data supporting materials discovery, design, and dissemination. J. Open Source Softw. 5 , 1945–1947 (2020).
Coley, C. W. et al. A robotic platform for flow synthesis of organic compounds informed by AI planning. Science 365 , 557 (2019).
Hachmann, J. et al. The harvard clean energy project: large-scale computational screening and design of organic photovoltaics on the world community grid. J. Phys. Chem. Lett. 2 , 2241–2251 (2011).
Bıyık, E., Wang, K., Anari, N. & Sadigh, D. Batch active learning using determinantal point processes. Preprint at https://doi.org/10.48550/arXiv.1906.07975 (2019).
Hoffmann, J. et al. Machine learning in a data-limited regime: augmenting experiments with synthetic data uncovers order in crumpled sheets. Sci. Adv. 5 , eaau6792 (2019).
Liu, J. Z. et al. Simple and principled uncertainty estimation with deterministic deep learning via distance awareness. Adv. Neural Inf. Process Syst. 33 , 7498–7512 (2020).
Lakshminarayanan, B., Pritzel, A. & Blundell, C. Simple and scalable predictive uncertainty estimation using deep ensembles. In Proceedings of the 31st International Conference on Neural Information Processing Systems (NIPS’17) 6405–6416 (Curran Associates Inc., 2017).
D’Amour, A. et al. Underspecification presents challenges for credibility in modern machine learning. Preprint at https://doi.org/10.48550/arXiv.2011.03395 (2020).
Barredo Arrieta, A. et al. Explainable artificial intelligence (XAI): concepts, taxonomies, opportunities and challenges toward responsible AI. Inf. Fusion. 58 , 82–115 (2020).
Ribeiro, M. T., Singh, S. & Guestrin, C. “Why should I trust you?” Explaining the predictions of any classifier. in Proceedings of the ACM SIGKDD International Conference on Knowledge Discovery and Data Mining 1135–1144 (2016).
Lundberg, S. & Lee, S.-I. An unexpected unity among methods for interpreting model predictions. Preprint at https://arxiv.org/abs/1611.07478 (2016).
Bach, S. et al. On pixel-wise explanations for non-linear classifier decisions by layer-wise relevance propagation. PLoS ONE 10 , e0130140 (2015).
Sun, W. et al. The thermodynamic scale of inorganic crystalline metastability. Sci. Adv. 2 , e1600225 (2016).
Aykol, M., Dwaraknath, S. S., Sun, W. & Persson, K. A. Thermodynamic limit for synthesis of metastable inorganic materials. Sci. Adv. 4 , eaaq0148 (2018).
Wei, J. N., Duvenaud, D. & Aspuru-Guzik, A. Neural networks for the prediction of organic chemistry reactions. ACS Cent. Sci. 2 , 725–732 (2016).
Coley, C. W. et al. A graph-convolutional neural network model for the prediction of chemical reactivity. Chem. Sci. 10 , 370–377 (2019).
Nagabhushana, G. P., Shivaramaiah, R. & Navrotsky, A. Direct calorimetric verification of thermodynamic instability of lead halide hybrid perovskites. Proc. Natl Acad. Sci. USA 113 , 7717–7721 (2016).
Sanna, S. et al. Enhancement of the chemical stability in confined δ-Bi 2 O 3 . Nat. Mater. 14 , 500–504 (2015).
Podolyan, Y., Walters, M. A. & Karypis, G. Assessing synthetic accessibility of chemical compounds using machine learning methods. J. Chem. Inf. Model. 50 , 979–991 (2010).
Coley, C. W., Rogers, L., Green, W. H. & Jensen, K. F. SCScore: synthetic complexity learned from a reaction corpus. J. Chem. Inf. Model. 58 , 252–261 (2018).
Kim, E. et al. Inorganic materials synthesis planning with literature-trained neural networks. J. Chem. Inf. Model. 60 , 1194–1201 (2020).
Huo, H. et al. Semi-supervised machine-learning classification of materials synthesis procedures. npj Comput. Mater. 5 , 62 (2019).
Tian, C., Lin, F. & Doeff, M. M. Electrochemical characteristics of layered transition metal oxide cathode materials for lithium ion batteries: surface, bulk behavior, and thermal properties. Acc. Chem. Res. 51 , 89–96 (2018).
Guilminot, E., Corcella, A., Charlot, F., Maillard, F. & Chatenet, M. Detection of Pt z + ions and Pt nanoparticles inside the membrane of a used PEMFC. J. Electrochem. Soc. 154 , B96 (2007).
Pender, J. P. et al. Electrode degradation in lithium-ion batteries. ACS Nano 14 , 1243–1295 (2020).
Li, Y. et al. Atomic structure of sensitive battery materials and interfaces revealed by cryo-electron microscopy. Science 358 , 506–510 (2017).
Wang, H. Numerical modeling of non-planar hydraulic fracture propagation in brittle and ductile rocks using XFEM with cohesive zone method. J. Pet. Sci. Eng. 135 , 127–140 (2015).
Hsu, Y.-C., Yu, C.-H. & Buehler, M. J. Using deep learning to predict fracture patterns in crystalline solids. Matter 3 , 197–211 (2020).
Wuest, T., Weimer, D., Irgens, C. & Thoben, K. D. Machine learning in manufacturing: advantages, challenges, and applications. Prod. Manuf. Res. 4 , 23–45 (2016).
De Jong, P., Sánchez, A. S., Esquerre, K., Kalid, R. A. & Torres, E. A. Solar and wind energy production in relation to the electricity load curve and hydroelectricity in the northeast region of Brazil. Renew. Sustain. Energy Rev. 23 , 526–535 (2013).
Zolfani, S. H. & Saparauskas, J. New application of SWARA method in prioritizing sustainability assessment indicators of energy system. Eng. Econ. 24 , 408–414 (2013).
Tao, F., Zhang, M., Liu, Y. & Nee, A. Y. C. Digital twin driven prognostics and health management for complex equipment. CIRP Ann. 67 , 169–172 (2018).
Yun, S., Park, J. H. & Kim, W. T. Data-centric middleware based digital twin platform for dependable cyber-physical systems. in International Conference on Ubiquitous and Future Networks (ICUFN) 922–926 (2017).
Boretti, A. Integration of solar thermal and photovoltaic, wind, and battery energy storage through AI in NEOM city. Energy AI 3 , 100038–100045 (2021).
Ghoddusi, H., Creamer, G. G. & Rafizadeh, N. Machine learning in energy economics and finance: a review. Energy Econ. 81 , 709–727 (2019).
Asensio, O. I., Mi, X. & Dharur, S. Using machine learning techniques to aid environmental policy analysis: a teaching case regarding big data and electric vehicle charging infrastructure. Case Stud. Environ. 4 , 961302 (2020).
Zheng, S., Trott, A., Srinivasa, S., Parkes, D. C. & Socher, R. The AI economist: taxation policy design via two-level deep multiagent reinforcement learning. Sci. Adv. 8 , eabk2607 (2022).
Sun, S. et al. A data fusion approach to optimize compositional stability of halide perovskites. Matter 4 , 1305–1322 (2021).
Sun, W. et al. Machine learning — assisted molecular design and efficiency prediction for high-performance organic photovoltaic materials. Sci. Adv. 5 , eaay4275 (2019).
Sun, S. et al. Accelerated development of perovskite-inspired materials via high-throughput synthesis and machine-learning diagnosis. Joule 3 , 1437–1451 (2019).
Kirman, J. et al. Machine-learning-accelerated perovskite crystallization. Matter 2 , 938–947 (2020).
Langner, S. et al. Beyond ternary OPV: high-throughput experimentation and self-driving laboratories optimize multicomponent systems. Adv. Mater. 32 , 1907801 (2020).
Hartono, N. T. P. et al. How machine learning can help select capping layers to suppress perovskite degradation. Nat. Commun. 11 , 4172 (2020).
Odabaşı, Ç. & Yıldırım, R. Performance analysis of perovskite solar cells in 2013–2018 using machine-learning tools. Nano Energy 56 , 770–791 (2019).
Fenning, D. P. et al. Darwin at high temperature: advancing solar cell material design using defect kinetics simulations and evolutionary optimization. Adv. Energy Mater. 4 , 1400459 (2014).
Allam, O., Cho, B. W., Kim, K. C. & Jang, S. S. Application of DFT-based machine learning for developing molecular electrode materials in Li-ion batteries. RSC Adv. 8 , 39414–39420 (2018).
Okamoto, Y. & Kubo, Y. Ab initio calculations of the redox potentials of additives for lithium-ion batteries and their prediction through machine learning. ACS Omega 3 , 7868–7874 (2018).
Takagishi, Y., Yamanaka, T. & Yamaue, T. Machine learning approaches for designing mesoscale structure of Li-ion battery electrodes. Batteries 5 , 54 (2019).
Tan, Y., Liu, W. & Qiu, Q. Adaptive power management using reinforcement learning. in IEEE/ACM International Conference on Computer-Aided Design, Digest of Technical Papers (ICCAD ) 461–467 (IEEE, 2009).
Ermon, S., Xue, Y., Gomes, C. & Selman, B. Learning policies for battery usage optimization in electric vehicles. Mach. Learn. 92 , 177–194 (2013).
Schmidt, J., Marques, M. R. G., Botti, S. & Marques, M. A. L. Recent advances and applications of machine learning in solid-state materials science. npj Comput. Mater. 5 , 83 (2019).
Pilania, G. Machine learning in materials science: from explainable predictions to autonomous design. Comput. Mater. Sci. 193 , 110360 (2021).
Butler, K. T., Davies, D. W., Cartwright, H., Isayev, O. & Walsh, A. Machine learning for molecular and materials science. Nature 559 , 547–555 (2018).
Kaufmann, K. et al. Crystal symmetry determination in electron diffraction using machine learning. Science 367 , 564–568 (2020).
Chen, C., Zuo, Y., Ye, W., Li, X. & Ong, S. P. Learning properties of ordered and disordered materials from multi-fidelity data. Nat. Comput. Sci. 1 , 46–53 (2021).
Liu, M., Yan, K., Oztekin, B. & Ji, S. GraphEBM: molecular graph generation with energy-based models. Preprint at https://doi.org/10.48550/arXiv.2102.00546 (2021).
Segler, M. H. S., Preuss, M. & Waller, M. P. Planning chemical syntheses with deep neural networks and symbolic AI. Nature 555 , 604–610 (2018).
Granda, J. M., Donina, L., Dragone, V., Long, D.-L. & Cronin, L. Controlling an organic synthesis robot with machine learning to search for new reactivity. Nature 559 , 377–381 (2018).
Epps, R. W. et al. Artificial chemist: an autonomous quantum dot synthesis bot. Adv. Mater. 32 , 2001626 (2020).
MacLeod, B. P. et al. Self-driving laboratory for accelerated discovery of thin-film materials. Sci. Adv. 6 , eaaz8867 (2020).
Li, Z. et al. Robot-accelerated perovskite investigation and discovery. Chem. Mater. 32 , 5650–5663 (2020).
Aguiar, J. A., Gong, M. L., Unocic, R. R., Tasdizen, T. & Miller, B. D. Decoding crystallography from high-resolution electron imaging and diffraction datasets with deep learning. Sci. Adv. 5 , eaaw1949 (2019).
Download references
Acknowledgements
Z.Y. and A.A.-G. were supported as part of the Nanoporous Materials Genome Center by the US Department of Energy, Office of Science, Office of Basic Energy Sciences under award number DE-FG02-17ER16362 and the US Department of Energy, Office of Science — Chicago under award number DE-SC0019300. A.J. was financially supported by Huawei Technologies Canada and the Natural Sciences and Engineering Research Council (NSERC). L.M.M.-M. thanks the support of the Defense Advanced Research Projects Agency under the Accelerated Molecular Discovery Program under cooperative agreement number HR00111920027 dated 1 August 2019. Y.W. acknowledges funding support from the Singapore National Research Foundation under its Green Buildings Innovation Cluster (GBIC award number NRF2015ENC-GBICRD001-012) administered by the Building and Construction Authority, its Green Data Centre Research (GDCR award number NRF2015ENC-GDCR01001-003) administered by the Info-communications Media Development Authority, and its Energy Programme (EP award number NRF2017EWT-EP003-023) administered by the Energy Market Authority of Singapore. A.A.-G. is a Canadian Institute for Advanced Research (CIFAR) Lebovic Fellow. E.H.S. acknowledges funding by the Ontario Ministry of Colleges and Universities (grant ORF-RE08-034), the Natural Sciences and Engineering Research Council (NSERC) of Canada (grant RGPIN-2017-06477), the Canadian Institute for Advanced Research (CIFAR) (grant FS20-154 APPT.2378) and the University of Toronto Connaught Fund (grant GC 2012-13). Z.W.S. acknowledges funding by the Singapore National Research Foundation (NRF-NRFF2017-04).
Author information
These authors contributed equally: Zhenpeng Yao, Yanwei Lum, Andrew Johnston.
Authors and Affiliations
Shanghai Key Laboratory of Hydrogen Science & Center of Hydrogen Science, School of Materials Science and Engineering, Shanghai Jiao Tong University, Shanghai, China
Zhenpeng Yao
Chemical Physics Theory Group, Department of Chemistry and Department of Computer Science, University of Toronto, Toronto, Ontario, Canada
Zhenpeng Yao, Luis Martin Mejia-Mendoza & Alán Aspuru-Guzik
Innovation Center for Future Materials, Zhangjiang Institute for Advanced Study, Shanghai Jiao Tong University, Shanghai, China
State Key Laboratory of Metal Matrix Composites, School of Materials Science and Engineering, Shanghai Jiao Tong University, Shanghai, China
Institute of Materials Research and Engineering, Agency for Science, Technology and Research (A*STAR), Innovis, Singapore, Singapore
Yanwei Lum & Zhi Wei Seh
Department of Electrical and Computer Engineering, University of Toronto, Toronto, Ontario, Canada
Yanwei Lum, Andrew Johnston & Edward H. Sargent
School of Computer Science and Engineering, Nanyang Technological University, Singapore, Singapore
Xin Zhou & Yonggang Wen
Vector Institute for Artificial Intelligence, Toronto, Ontario, Canada
- Alán Aspuru-Guzik
You can also search for this author in PubMed Google Scholar
Contributions
Z.Y., Y.L. and A.J. contributed equally to this work. All authors contributed to the writing and editing of the manuscript.
Corresponding authors
Correspondence to Alán Aspuru-Guzik , Edward H. Sargent or Zhi Wei Seh .
Ethics declarations
Competing interests.
The authors declare no competing interests.
Peer review
Peer review information.
Nature Reviews Materials thanks Shijing Sun and the other, anonymous, reviewer(s) for their contribution to the peer review of this work.
Additional information
Publisher’s note.
Springer Nature remains neutral with regard to jurisdictional claims in published maps and institutional affiliations.
Related links
MatD3 database: https://github.com/HybriD3-database/MatD3
Materials Project: https://materialsproject.org/
Machine learning techniques that can query a user interactively to modify its current strategy (that is, label an input).
(AI). Theory and development of computer systems that exhibit intelligence.
A system for adjusting the power output of multiple generators at different power plants, in response to changes in the load.
A technology development pipeline that incorporates automation to go from idea to realization of technology. ‘Closed’ refers to the concept that the system improves with experience and iterations.
Process of increasing the amount of data through adding slightly modified copies or newly created synthetic data from existing data.
A generative graphical model, or alternatively a class of deep neural network, composed of multiple layers of latent variables, with connections between the layers but not between units within each layer.
(DL). Machine learning subfield that is based on neural networks with representation learning.
The ability to adapt to new, unseen data, drawn from the same distribution as the one used to create the model.
Machine learning techniques that learn to model the data distribution of a dataset and sample new data points.
Degree to which a human can understand a model’s decision. Interpretability can be used to build trust and credibility.
A design method where new materials and compounds are ‘reverse-engineered’ simply by inputting a set of desired properties and characteristics and then using an optimization algorithm to generate a predicted solution.
A special kind of recurrent neural networks that are capable of selectively remembering patterns for a long duration of time.
(ML). Field within artificial intelligence that deals with learning algorithms, which improve automatically through experience (data).
A computerized system composed of multiple interacting intelligent agents.
The combination of ridge regression (a method of estimating the coefficients of multiple-regression models in scenarios where the independent variables are highly correlated) with multiple kernel techniques.
Models that involve the analysis of multiple, simultaneous physical phenomena, which can include heat transfer, fluid flow, deformation, electromagnetics, acoustics and mass transport.
The field of solving problems that have important features at multiple scales of time and/or space.
A neural network is composed of parameterized and optimizable transformations.
A class of artificial neural networks where connections between nodes form a directed or undirected graph along a temporal sequence.
Process of incorporating additional information into the model to constrain its solution space.
Machine learning techniques that make a sequence of decisions to maximize a reward.
Features used in a representation learning model, which transforms inputs into new features for a task.
Technique for solving problems in the planning of chemical synthesis.
A robotic equipment automated chemical synthesis plan.
Design process composed of several stages where materials are iteratively filtered and ranked to arrive to a few top candidates.
Machine learning techniques that involve the usage of labelled data.
Machine learning techniques that adapt a learned representation or strategy from one dataset to another.
Process of evaluating the statistical confidence of model.
Machine learning techniques that learn patterns from unlabelled data.
Rights and permissions
Springer Nature or its licensor holds exclusive rights to this article under a publishing agreement with the author(s) or other rightsholder(s); author self-archiving of the accepted manuscript version of this article is solely governed by the terms of such publishing agreement and applicable law.
Reprints and permissions
About this article
Cite this article.
Yao, Z., Lum, Y., Johnston, A. et al. Machine learning for a sustainable energy future. Nat Rev Mater 8 , 202–215 (2023). https://doi.org/10.1038/s41578-022-00490-5
Download citation
Accepted : 14 September 2022
Published : 18 October 2022
Issue Date : March 2023
DOI : https://doi.org/10.1038/s41578-022-00490-5
Share this article
Anyone you share the following link with will be able to read this content:
Sorry, a shareable link is not currently available for this article.
Provided by the Springer Nature SharedIt content-sharing initiative
This article is cited by
Artificial intelligence-based methods for renewable power system operation.
- Yuanzheng Li
- Yizhou Ding
- Zhigang Zeng
Nature Reviews Electrical Engineering (2024)
Nature-inspired interfacial engineering for energy harvesting
- Baoping Zhang
- Wanghuai Xu
- Zuankai Wang
Bringing digital synthesis to Mars
- Mark D. Symes
- Leroy Cronin
Nature Synthesis (2024)
Accelerating discovery in organic redox flow batteries
Nature Computational Science (2024)
Sustainable moisture energy
- Pengfei Wang
- Tingxian Li
Nature Reviews Materials (2024)
Quick links
- Explore articles by subject
- Guide to authors
- Editorial policies
Sign up for the Nature Briefing newsletter — what matters in science, free to your inbox daily.

Click through the PLOS taxonomy to find articles in your field.
For more information about PLOS Subject Areas, click here .
Loading metrics
Open Access
Peer-reviewed
Research Article
A systematic bibliometric review of clean energy transition: Implications for low-carbon development
Roles Writing – original draft, Writing – review & editing
Affiliation School of Statistics, Shandong University of Finance and Economics, Jinan, China
Roles Data curation, Software
Roles Conceptualization, Writing – review & editing
* E-mail: [email protected]
Affiliation Centre for Corporate Sustainability and Environmental Finance, Department of Applied Finance, Macquarie Business School, Macquarie University, Sydney, Australia

Roles Methodology, Validation
Affiliation School of Humanities and Foreign Languages, Qingdao University of Technology, Qingdao, China
Roles Software, Visualization
- Wei Zhang,
- Binshuai Li,
- Rui Xue,
- Chengcheng Wang,
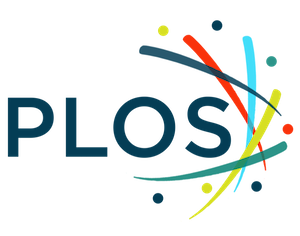
- Published: December 3, 2021
- https://doi.org/10.1371/journal.pone.0261091
- Reader Comments
More voices are calling for a quicker transition towards clean energy. The exploration and exploitation of clean energy such as wind energy and solar energy are effective means to optimise energy structure and improve energy efficiency. To provide in-depth understanding of clean energy transition, this paper utilises a combination of multiple bibliometric mapping techniques, including HistCite, CiteSpace and R Bibliometrix, to conduct a systematic review on 2,191 clean energy related articles obtained from Web of Science (WoS). We identify five current main research streams in the clean energy field, including Energy Transition, Clean Energy and Carbon Emission Policy, Impact of Oil Price on Alternative Energy Stocks, Clean Energy and Economics, and Venture Capital Investments in Clean Energy. Clearly, the effectiveness of policy-driven and market-driven energy transition is an important ongoing debate. Emerging research topics are also discussed and classified into six areas: Clean Energy Conversion Technology and Biomass Energy Utilisation, Optimisation of Energy Generation Technology, Policy-Making in Clean Energy Transition, Impact of Clean Energy Use and Economic Development on Carbon Emissions, Household Use of Clean Energy, and Clean Energy Stock Markets. Accordingly, more and more research attention has been paid to how to improve energy efficiency through advanced clean energy technology, and how to make targeted policies for clean energy transition and energy market development. This article moves beyond the traditional literature review methods and delineates a systematic research agenda for clean energy research, providing research directions for achieving low-carbon development through the clean energy transition.
Citation: Zhang W, Li B, Xue R, Wang C, Cao W (2021) A systematic bibliometric review of clean energy transition: Implications for low-carbon development. PLoS ONE 16(12): e0261091. https://doi.org/10.1371/journal.pone.0261091
Editor: Ghaffar Ali, Shenzhen University, CHINA
Received: July 29, 2021; Accepted: November 23, 2021; Published: December 3, 2021
Copyright: © 2021 Zhang et al. This is an open access article distributed under the terms of the Creative Commons Attribution License , which permits unrestricted use, distribution, and reproduction in any medium, provided the original author and source are credited.
Data Availability: The data underlying the results presented in the study are available from: Zhang, Wei; Li, Binshuai; Xue, Rui; Wang, Chengcheng; Cao, Wei (2021), “Clean Energy Related Literature Data”, Mendeley Data, V1, doi: 10.17632/h9n69648d9.1 .
Funding: This research was supported by the National Social Science Foundation of China (Grant Number: 20BTJ030), the Social Science Planning Foundation of Shandong Province (16CTJJ01) and Youth Innovation Program of Shandong Province (2019REW021). The funders had no role in study design, data collection and analysis, decision to publish, or preparation of the manuscript.
Competing interests: The authors have declared that no competing interests exist.
1 Introduction
Currently, many countries worldwide have proposed and implemented their green recovery plans [ 1 – 3 ]. Public voices for transitioning to clean energy are increasingly high, shifting investors’ attention from traditional energy markets to clean energy markets. Therefore, it is important and urgent to systematically understand how to transition to a “clean” world.
Considering this context, the key research question of this study is to provide a comprehensive understanding of the current progress of the clean energy transition and illustrate a research agenda for emerging areas that await more academic and practical attention. To address the research question, this study provides a systematic literature review of 2,191 articles on clean energy related areas obtained from the Web of Science (WoS) Core Collection database over the period from 1950 to 2020. Using a combination of multiple bibliometric mapping techniques, we identify the main streams of current research and propose important topics for future research, providing comprehensive insights for the developments in clean energy transitions and a theoretical basis for more effective ways to achieve carbon neutrality.
Current main streams of clean energy research identified by bibliometric analysis include Energy Transition, Clean Energy and Carbon Emission Policy, Impact of Oil Price on Alternative Energy Stocks, Clean Energy and Economics, and Venture Capital Investments in Clean Energy.
Specifically, the Energy Transition research stream focuses on the barriers to energy transition at the national and household level [ 4 ]. Given the governments’ dominant role in promoting the clean energy transition [ 5 ], the Clean Energy and Carbon Emission Policy stream concentrates on assessing governments’ related policies and their impacts on carbon emissions. The Impact of Oil Price on Alternative Energy Stocks stream centres around the influencing factors on clean energy stock prices; existing studies show that oil prices, technology stock prices, and interest rates are prominent factors affecting clean energy stock prices [ 6 ]. The Clean Energy and Economics stream tends to apply econometric models to test the causal relationship between clean energy consumption and socio-economic variables such as economic growth [ 7 ] and foreign direct investment (FDI) [ 8 ]. As the soaring demand for clean energy attracts a significant amount of venture capital inflows, especially the private ones [ 9 ], the identification and minimisation of investment risk for investors remains the major topic for current research in Venture Capital Investments in Clean Energy.
We further employ the cluster analysis of articles published in recent five years (2015–2020) to propose the emerging trends and future directions in clean energy research. Clean Energy Conversion Technology and Biomass Energy Utilisation, Optimisation of Energy Generation Technology, Policy-Making in Clean Energy Transition, Impact of Clean Energy Use and Economic Development on Carbon Emissions, Household Use of Clean Energy, and Clean Energy Stock Markets are trending topics in the clean energy transition.
Specifically, a growing trend in Clean Energy Conversion Technology and Biomass Energy Utilisation aims to enhance the efficiency and reliability of the biomass gasification system [ 10 , 11 ]. Research in Optimisation of Energy Generation Technology has been paying more attention to explore ways to effectively integrate new energy resources with traditional ones, construct an efficient hybrid energy system, and resolve the environmental problems incurred from the use of clean energy [ 12 , 13 ]. Because of the significant discrepancies in the influences of local governments’ clean energy policies [ 14 , 15 ], the Policy-Making in Clean Energy Transition research continues to explore how local governments should formulate policies conducive to the development of clean energy. The Impact of Clean Energy Use and Economic Development on Carbon Emissions stream provides policymakers with emission reduction recommendations. It starts to investigate the implications of clean energy use and various economic factors, particularly on carbon productivity and carbon transfer [ 16 ]. The vital issue of Household Use of Clean Energy research is to increase the the heating system’s energy efficiency and to accelerate the energy transfer of clean cooking [ 17 ]. Finally, studies on Clean Energy Stock Markets examine the correlation between clean energy stock prices and the overall stock market, green bond market, electricity market, and coal market [ 18 , 19 ].
Through systematic reviews of current and trending topics in clean energy research, we aim to delineate a critical research agenda for clean energy transition as an effective way to achieve a low-carbon development and carbon neutrality. The article proceeds as follows. Section 2 introduces the literature retrieval process, the bibliometric techniques used and the descriptive information of existing literature on clean energy. Section 3 illustrates the citation map to identify current main streams in clean energy research and provides a critical review of every stream. Section 4 proposes emerging areas and trending topics. Section 5 concludes the article and provides an agenda for future research in the clean energy transition.
2 Research methods
2.1 literature retrieval process.
The method of literature retrieval and bibliometric analysis used in this study is illustrated in Fig 1 . Specifically, we collect basic information and cited references of clean energy articles from Web of Science (WoS) over the period of 1950 to June 2020, with themes limited to “clean energy” and journal sources limited to “SSCI, SCIE, A&HCI.” A total of 2,652 initial articles is retrieved. For validation purposes, we have implemented manual checks to select relevant articles, resulting in 471 irrelevant articles removed. Following Linnenluecke et al. (2017) [ 20 ], we then add another ten most cited clean energy articles into our database. Therefore, we obtain 2,191 articles in our final dataset.
- PPT PowerPoint slide
- PNG larger image
- TIFF original image
https://doi.org/10.1371/journal.pone.0261091.g001
Table 1 shows the basic information of sample articles. The next section will introduce the bibliometric techniques used, i.e., R Bibliometrix, HistCite and CiteSpace, to analyse these clean energy articles.
https://doi.org/10.1371/journal.pone.0261091.t001
2.2 Bibliometric techniques
2.2.1 r bibliometrix..
Bibliometrix is a widely-used R-package developed by Massimo and Corrado (2017) [ 21 ]. It provides access to a wide range of bibliometric functions and excellent visualisation tools. This article uses Bibliometrix to carry out descriptive statistical analysis to illustrate the diagrams for the number of publications over time and the author-keyword-journal connections (Sankey diagram).
2.2.2 HistCite.
HistCite is a citation software developed by Eugene (2004) [ 22 ]. The citation map generated by HistCite is highly useful for mapping out the relationships among highly cited publications [ 23 ]. It is a popular tool for researchers to explore research hotspots and how research themes develop over time. It is an essential tool for bibliometric analysis. This paper utilises HistCite to generate the citation map of 50 highly cited articles as guidance to identify key streams of clean energy research.
2.2.3 CiteSpace.
CiteSpace is a Java visualization application developed by Chen (2017) [ 24 ]. It has powerful bibliometric and visualization functions and is extremely popular in research. It generates a spectrum of colors to depict the literature network’s temporal orders and uses algorithms such as LLR for cluster labeling extraction. This article uses this application to cluster keywords of relevant literature from 2015 to 2020 to identify future research hotspots.
2.3 Descriptive information
2.3.1 publications over time..
Fig 2 illustrates the number of publications from 2000 to 2019. The sample ends at June 2020 and the total number of publications from January 2020 to June 2020 is 274; so to make the diagram more illustrative, we do not include the publication number of 2020. Fig 2 indicates a three-stage development of clean energy research. The first stage (from 2000 to 2010) is the initial stage, with an average of 17.5 articles published per year. The period of 2011–2015 is the developing stage, with an average of 97.4 articles published per year. The publications in the clean energy areas experience a significant increase from 2016, with an average number of 291.5 publications per year (2016–2019). It signals a robust momentum in clean energy research. The clean energy transition is crucially important to mitigate climate change issues and achieve carbon neutrality. Therefore, it is expected to continue to (exponentially) grow in the next few decades.
https://doi.org/10.1371/journal.pone.0261091.g002
2.3.2 Author-Keyword-Journal (AKJ) analysis.
Fig 3 displays the Sankey diagram, i.e., the author-keyword-journal diagram. The three columns in Fig 3 are the top 20 authors, keywords, and source journals in clean energy research, respectively. The Sankey diagram gives a graphical overview of influential clean energy research. The keywords broadly fall into the following categories: Clean Energy Stock Performance, Clean Energy and Economy Growth, Energy Consumption and Carbon Emissions, Clean Energy Power Generation, and Clean Energy Policy. The major publishing journals in the clean energy area include Renewable Energy , Journal of Cleaner Production , Energy Policy , Energy Economics , Applied Energy , etc.
https://doi.org/10.1371/journal.pone.0261091.g003
3 Developments in clean energy transition research
3.1 identification of current research streams.
In this section, we utilise HistCite to generate a citation network map for the top 50 cited articles in clean energy transition research. We then apply the triangulation process [ 23 ] to assign titles for each research stream, laying the foundation for the systematic review of these research themes. Table 2 summarises the citation information of top-cited literature, and Fig 4 illustrates the corresponding citation network map.
https://doi.org/10.1371/journal.pone.0261091.g004
https://doi.org/10.1371/journal.pone.0261091.t002
In Fig 4 , each article is displayed as a node, with a larger-sized node denoting a higher number of citations. The arrows illustrate the citation connections among articles, with arrowheads pointed to the cited ones. Through the triangulation process, we categorise the current clean energy research into the following streams: Energy Transition, Clean Energy and Carbon Emission Policy, Impact of Oil Price on Alternative Energy Stocks, Clean Energy and Economics, and Venture Capital Investments in Clean Energy. In the next section, we provide a comprehensive review of each of these five research streams.
3.2 Review of main research streams
3.2.1 energy transition..
The transition from traditional energy towards clean energy remains the major challenge for the first half of the 21st century [ 4 ]. We discuss the Energy Transition stream from two perspectives: obstacles in clean energy transition and influencing factors on household energy transition.
3 . 2 . 1 . 1 Obstacles in the clean energy transition . Current major challenges to clean energy transition include subsidies to traditional energy, high initial capital cost, high transaction cost, high financing risk, lack of price risk assessment, lack of clean technology, low market acceptance rate, and immature regulatory systems [ 25 – 28 ]. Luthra et al. (2015) [ 29 ] categorised 28 obstacles to the clean energy transition into seven dimensions: economy and finance, market, awareness and information, technology, ecology and geography, culture and behavior, political and government issues. For an in-depth look, the more challenging obstacles are ecological problems, consumers’ lack of awareness of clean technology, inability to obtain solar radiation data, technical complexity, rehabilitation disputes and lack of political commitment.
3 . 2 . 1 . 2 Influencing factors on household energy transition . Household energy use is a substantial part of energy consumption. Investigating the driving factors affecting household energy transition is an effective way to promote clean energy transition. Researchers conduct surveys on households in urban and rural areas in China, India, Brazil, Ethiopia, Guatemala, and other countries. Their results show that 1) household income and fuel prices are the dominant factors affecting household energy transition, 2) household size, household members’ occupations, and education levels are also important factors, and 3) the availability and cost of clean energy alternatives have a significant impact on rural household energy transition [ 30 – 42 ].
3.2.2 Clean energy and carbon emission policy.
The high carbon energy represented by raw coal was still the main factor in promoting the growth of energy-related CO2 emissions [ 43 ]. Appropriate and effective policies are needed to accelerate the clean energy transition. The majority of countries worldwide have set goals to increase the share of clean energy consumption and reduce greenhouse gas (GHG) emissions, resulting in various supportive policies [ 44 ]. Existing policies concentrate around quantity-driven policies. For instance, levying a carbon tax is a typical quantity-driven policy. Guo et al. (2014) [ 5 ] argues that a moderate carbon tax significantly reduces carbon emissions and fossil fuel consumption, with a minimal impact on economic growth. But a more recent study claims that carbon taxes are not always good for the environment [ 45 ]. Another example is feed-in tariffs (FIT), a quantity-driven policy targeted at specific technology [ 46 ]. It is generally regarded as an effective policy for clean energy transition due to its advantages of low costs, low risks, and high innovation incentives [ 47 – 51 ].
3.2.3 Impact of oil price on alternative energy stocks.
The way how oil prices affect stock prices works as follows. On the one hand, rising oil prices increase production and service costs and decrease cash flow turnover, leading to a stock price drop. On the other hand, rising oil prices also indicate the mounting inflation pressure and discount rate, resulting in stock price drop [ 52 ]. As a critical component of the stock market, energy stocks are also highly correlated with oil prices [ 52 – 55 ]. Nevertheless, the negative impact of oil prices may only be a short-term effect for clean energy stocks [ 6 ].
3.2.4 Clean energy and economics.
The clean energy transition is closely related to economic development [ 7 ]. In Fig 4 , the theme of Clean Energy and Economy contains comparatively more nodes (articles), the majority of which use different econometric models to examine the relationship between clean energy consumption and socio-economic variables such as economic growth and FDI. In the short term, there exists a positive correlation and bidirectional causal relationship between clean energy consumption and economic development. In the long run, clean energy consumption will positively affect on economic growth [ 8 , 56 – 60 ]. The empirical results of Paramati et al.(2016) [ 8 ] indicate that there is a unidirectional causality running from FDI to clean energy consumption, with inflows of FDI having a positive impact on the latter. Moreover, the results of Paramati et al.(2016) [ 8 ] also show that the development of the stock market has brought more investment in the clean energy industry and plays a significant role in promoting clean energy transition.
3.2.5 Venture capital investments in clean energy.
Venture capital (VC) is one of the main drivers of technology advancement, especially in new and innovative fields such as clean energy. As the demand for clean energy increases, there has been a surge of venture capital inflows, especially private VCs, into clean energy companies [ 9 , 61 , 62 ]. Currently, clean energy has become the third-largest venture investment field [ 63 ]. In addition, there are also risks embedded in clean energy investments, including market risks, technology risks, human resource risks, and more importantly, regulatory risks [ 64 ]. However, it is feasible to reduce market risks through appropriate business models, reduce technology risks through publicly funded R&D projects, reduce human resource risks through market liberalisation, and reduce regulatory risks through effective government policies [ 64 , 65 ].
4 Emerging research areas
To illustrate the emerging topics in clean energy transition research, we utilise CiteSpace to conduct cluster analysis on sample articles published in recent five years, from 2015 to 2020. The following two sections provide basic information on identified emerging topics and provide a detailed analysis of the relevant literature.
4.1 Identifications of emerging research areas
Fig 5 demonstrates the keyword co-occurrence network map of recent five years’ publications in clean energy transition areas, with a larger circle (keyword) representing more frequent occurrence, and darker colour representing earlier occurrence (publication time). The lines connecting circles (keywords) refer to co-occurrence.
https://doi.org/10.1371/journal.pone.0261091.g005
Using cluster analysis, CiteSpace classifies recent five years’ publications into seven clusters, reflecting seven emerging research topics in clean energy research. The clustered emerging topics include Surface Properties, Fuel Cell, Energy Transition, CO 2 Emission, Household Fuel Use, Oil Price, and Wind Farm. Once again, we apply the triangulation process to define the title of each cluster (area) and provide more details in Table 3 .
https://doi.org/10.1371/journal.pone.0261091.t003
4.2 Analyses of emerging research areas
4.2.1 clean energy conversion technology and biomass energy utilization..
Converting industrial waste and household garbage into clean energy can help deal with the current shortage of clean energy and protect the environment through the recycling process. Studies show that kitchen waste, animal waste, agricultural waste, forestry waste, waste plastics and waste tyres can be converted into clean energy through advanced technologies such as thermochemical conversion or hydrothermal carbonisation [ 10 , 66 – 70 ]. Research on improving these conversion technologies is a trending research hotspot. For example, biomass gasification is a feasible and practical clean energy conversion technology, but it faces crucial challenges to effectively eliminate the tar generated during the gasification process [ 11 , 71 , 72 ]. Another trending research topic in this area is to enhance the efficiency and reliability of biomass gasification. In addition, with the continuous advancement of clean energy conversion technology, how to formulate policies to implement more effective classifications of waste and refuse continues to be an urgent issue to be further explored.
4.2.2 Optimisation of Energy Generation Technology.
Comprehensive utilisation of various energy resources is an ideal approach to alleviate the energy crisis [ 73 ]. Many scholars have investigated how to integrate various new and traditional energy resources, including photovoltaics, batteries, diesel, wind energy, and solar energy, to build a highly effective hybrid energy system [ 12 , 13 , 74 ]. Research on the development of clean energy battery systems, the optimisation of power station scale, and generator systems also receives extensive academic attention [ 75 , 76 ].
Electricity generation from clean energy, such as wind and solar, plays a key role in the clean technology optimisation research [ 77 , 78 ]; however, a series of problems are setting obstacles for it. For instance, wind power generation has a high level of uncertainty, and there are potential exposure risks to the operation of a power grid [ 79 , 80 ]. Therefore, research on wind power generation in recent years tends to focus on wind flow models with the expectation to achieve a more accurate prediction of wind power generation [ 79 , 81 ]. Besides, considering the negative impact of the wind power plant on the environment, researchers have made significant explorations on the environmental effects of wind farms and on the selection of wind farm locations for harnessing wind energy [ 82 – 87 ]. Resolving the problems arising from the use of clean energy is an important topic to be further examined.
4.2.3 Policy-making in clean energy transition.
Regulations and legislations guarantee the secure transition towards clean energy. The government thus plays an essential role in addressing the potential risks incurred by the clean energy transition process. Relevant policies involve electricity price standards, emission trading system, clean energy investment policies, and the use of innovative finance tools in clean energy support [ 14 , 15 , 88 , 89 ]. Tingey and Webb (2020) [ 90 ], Bayulgen (2020) [ 91 ] and Proedrou (2019) [ 92 ] evaluate the practices of local government in the UK, US, and EU in terms of the clean energy transition. Their results indicate that although most local governments have adopted clean energy policies, the effectiveness of these policies varies substantially. To improve the effectiveness of energy policies, the views of different local energy users should be taken into account [ 93 ]. Therefore, what policies local governments should formulate to accelerate clean energy development will continue to be one of the research hotspots in clean energy transition research.
4.2.4 Impact of clean energy use and economic development on carbon emissions.
A large body of literature concentrates on how clean energy, economic growth, land resource use, industrial restructuring, financial market development, the application of new technology and R&D activities affect carbon emissions in recent clean energy areas [ 16 , 94 – 100 ]. And it is likely to be a hot issue worth studying in the future. With the improvement of carbon emission measurement methods, research on the impact of the aforementioned factors on carbon productivity and carbon transfer is attracting increasing scholarly attention [ 101 – 103 ]. Moreover, from a micro point of view, the role of enterprises, as an essential component of the national economy, in environmental governance will become another trending research direction [ 104 ].
4.2.5 Household use of clean energy.
Given that household energy use for heating and cooking is an essential part of energy use, recent studies have made substantial progress on enhancing the heating system’s energy efficiency and advancing the clean energy transition for cooking [ 17 , 105 – 108 ]. Moreover, in terms of the driving factors on the household clean energy transition, more recent literature indicates that household income and energy prices are found to have significant effects on household energy use decisions. Therefore, energy poverty is also an issue worth future research attention [ 109 – 111 ].
4.2.6 Clean energy stock markets.
Without support from the financial markets, the clean energy industry alone cannot secure the desired level of clean energy development. In effect, clean energy stocks have recently become a popular investment asset for investors, especially for those with strong considerations for environmental protection [ 18 , 112 ]. In addition to the follow-up research on the impact of oil price on clean energy stock prices [ 19 , 113 , 114 ], increasingly great attention has been focused on the relationship between clean energy stock investment and its driving factors, including the overall stock market, bond market, electricity market, coal market, gold market, silver market and many more [ 18 , 112 , 115 – 118 ]. Therefore, we reckon that the relationship between clean energy stocks and the financial markets, especially the green bond market [ 119 ] and the carbon market [ 53 ], has great potential to be explored in future clean energy research.
5 Conclusions
Clean energy transition plays a crucial role in post-pandemic green recoveries and carbon neutrality. To advance understanding of clean energy transition, this paper provides a systematic review of existing clean energy literature through a combination of bibliometric analysis techniques. Overall, there has been a surging trend of clean energy research since 2000, especially after 2016, clean energy research has experienced exponential growth.
We collect clean energy literature from the Web of Science (WoS) Core Collection database over the period from 1950 to 2020. Using bibliometric analysis, we identify and provide a comprehensive review of five current main research streams in the clean energy area, including Energy Transition, Clean Energy and Carbon Emission Policy, Impact of Oil Price on Alternative Energy Stocks, Clean Energy and Economics, and Venture Capital Investments in Clean Energy. Main challenges and opportunities facing the current clean energy transition with respect to each research stream are investigated.
To illustrate emerging research topics that attract more recent academic attention, we apply bibliometric cluster analysis to clean energy literature published in recent five years (from 2015 to 2020). Six trending research areas in the clean energy field are proposed and analysed, including Clean Energy Conversion Technology and Biomass Energy Utilisation, Optimisation of Energy Generation Technology, Policy-Making in Clean Energy Transition, Impact of Clean Energy Use and Economic Development on Carbon Emissions, Household Use of Clean Energy, and Clean Energy Stock Markets.
Future research agenda of clean energy awaits theoretical and practical exploration. We propose that the advancement of clean technology is at the heart of clean energy transition and post-pandemic green recovery. Funding for clean energy transition is a critical challenge that needs innovative financial instruments and policy support. Thus green bond markets, carbon taxes and emission trading system (ETS) need in-depth investigation. With more disruptive financing tools available such as crowdfunding, efforts from enterprises and individuals also deserve more attention. In addition, international collaborations on clean energy transition projects are highly recommended. Intensive international collaborations and cooperations are of high importance to achieve the low-carbon development. The completion of the global warming goal needs collective contributions from all countries over the world. A community of common destiny for all of humankind cannot be successfully built with efforts from only a small number of highly engaged countries. The current collaboration in clean energy research lacks worldwide collaborations in climate change actions. Therefore, it is highly recommended that all countries shall shoulder their responsibilities in climate change mitigation and adaptation, with steady growth of environmental investments and frequent collaborations with leading countries in climate change actions.
- View Article
- Google Scholar
- 9. Ghosh S, Nanda R. Venture Capital Investment in the Clean Energy Sector. Harvard Business School Entrepreneurial Management Working Paper. 2010. http://dx.doi.org/10.2139/ssrn.1669445
- PubMed/NCBI
- 63. Wüstenhagen R, Wuebker R, Bürer MJ, Goddard D. Financing fuel cell market development: Exploring the role of expectation dynamics in venture capital investment. In: Pogutz S, Russo A, Migliavacca P, editors. Innovation, markets and sustainable energy. Bocconi University, Italy; 2009. Chapter 8. https://doi.org/10.4337/9781848449329.00019
Articles on Green energy
Displaying 1 - 20 of 54 articles.
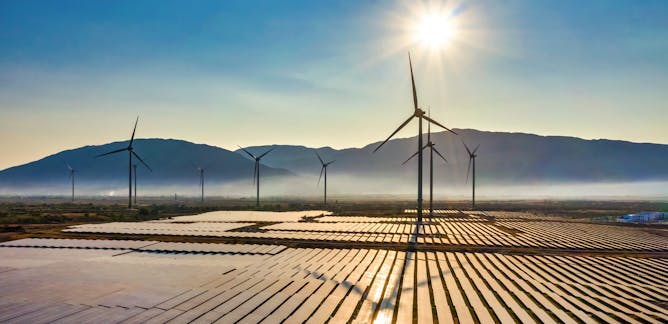
Green economy summit: how can Australia get more from its relationship with Vietnam?
Trang Nguyen , Climateworks Centre and Anna Skarbek , Climateworks Centre
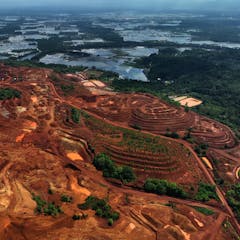
8 ways to ensure Indonesia’s nickel sector is sustainable
Robby Irfany Maqoma , The Conversation and Anggi M. Lubis , The Conversation
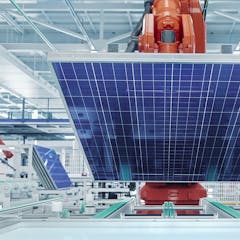
Economists say Australia shouldn’t try to transition to net zero by aping the mammoth US Inflation Reduction Act
Peter Martin , Crawford School of Public Policy, Australian National University
Renewable energy innovation isn’t just good for the climate — it’s also good for the economy
Deborah de Lange , Toronto Metropolitan University
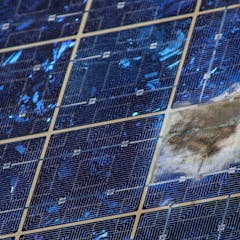
As the world heats up, solar panels will degrade faster – especially in hot, humid areas. What can we do?
Shukla Poddar , UNSW Sydney
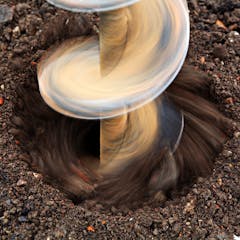
‘Gold’ hydrogen: natural deposits are turning up all over the world – but how useful is it in our move away from fossil fuels?
David Waltham , Royal Holloway University of London
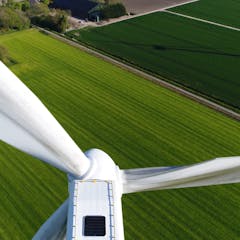
How could Australia actually get to net zero? Here’s how
Anna Skarbek , Climateworks Centre ; Anna Malos , Monash University , and Michael Li , Monash University
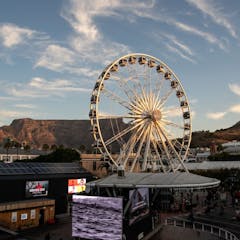
Tourists are returning to South Africa – but the sector will need to go green to deal with the country’s electricity crisis
Gijsbert Hoogendoorn , University of Johannesburg ; Irma Booyens , University of Strathclyde , and Kristy Langerman , University of Johannesburg
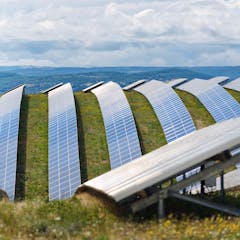
Solar power expected to dominate electricity generation by 2050 – even without more ambitious climate policies
Nadia Ameli , UCL ; Femke Nijsse , University of Exeter , and Jean-Francois Mercure , University of Exeter
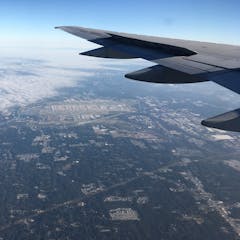
Air travel is in a rut – is there any hope of recapturing the romance of flying?
Christopher Schaberg , Arts & Sciences at Washington University in St. Louis
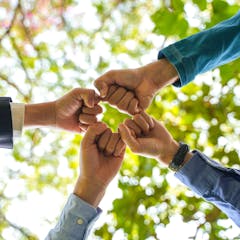
Canada needs to set its businesses up for success in the clean energy transition
Stuart Snaith , University of Victoria ; Alison Jean Parker , University of Victoria , and Douglas A. Stuart , University of Victoria
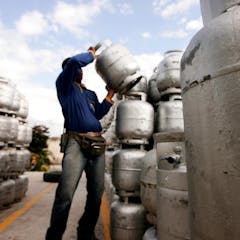
LPG is a fossil fuel. Experts explain why it’s still Africa’s best option for cleaner, greener cooking (for now)
Nigel Bruce , University of Liverpool and Dan Pope , University of Liverpool
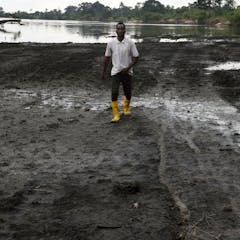
Greenwashing: energy companies make false claims about sustainability – they should be held to account
Ouidad Yousfi , Université de Montpellier and Maha El Kateb , Université de Montpellier
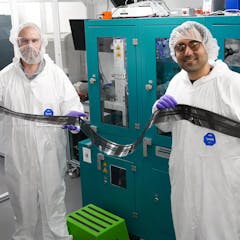
Perovskite: new type of solar technology paves the way for abundant, cheap and printable cells
David Beynon , Swansea University
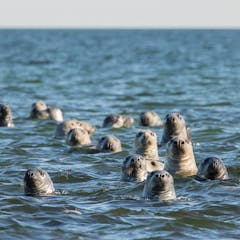
Grey seals are returning to UK waters – but their situation remains precarious
Katrina Davis , University of Oxford and Richard Bevan , Newcastle University
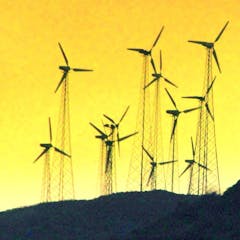
The rise of renewables is not without risk for investors
Frédéric Blanc-Brude , EDHEC Business School ; Laurence Monnier , EDHEC Business School , and Leonard Lum , EDHEC Business School
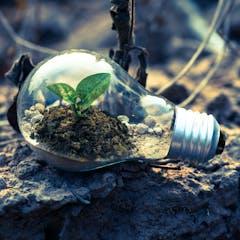
How crowdfunding could raise US$12 billion to boost renewable energy access in Indonesia
Vivi Fitriyanti , The Purnomo Yusgiantoro Center ; Massita Ayu Cindy Putriastuti , The Purnomo Yusgiantoro Center , and Vivid Amalia Khusna , The Purnomo Yusgiantoro Center
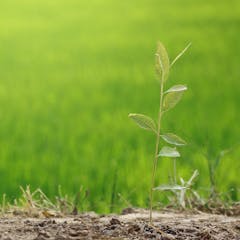
Labor won’t overhaul environment laws until next year. Here are 5 easy wins it could aim for now
James Watson , The University of Queensland
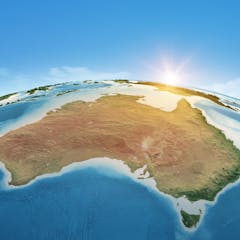
Albanese just laid out a radical new vision for Australia in the region: clean energy exporter and green manufacturer
John Mathews , Macquarie University ; Elizabeth Thurbon , UNSW Sydney ; Hao Tan , University of Newcastle , and Sung-Young Kim , Macquarie University
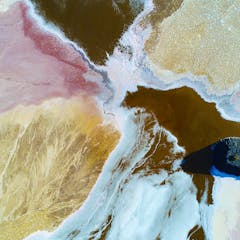
Tapping mineral wealth in mining waste could offset damage from new green economy mines
Anita Parbhakar-Fox , The University of Queensland ; Kamini Bhowany , The University of Queensland ; Kristy Guerin , Geoscience Australia ; Laura Jackson , The University of Queensland , and Partha Narayan Mishra , The University of Queensland
Related Topics
- Clean energy
- Climate change
- Electricity
- Energy transition
- Fossil fuels
- Renewable energy
- Solar power
- Wind energy
Top contributors
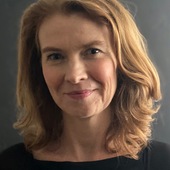
Professor in International Relations / International Political Economy, UNSW Sydney
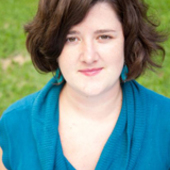
Research Associate, Institute for Sustainable Futures, University of Technology Sydney
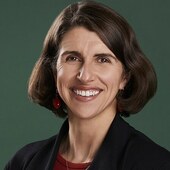
CEO, Climateworks Centre
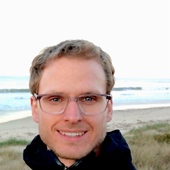
Co-Founder at Relectrify | Graduated PhD in Battery Storage, The University of Melbourne
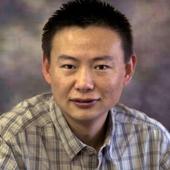
Professor and Associate Dean (Education), University of Nottingham
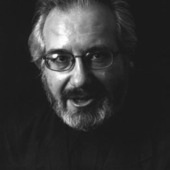
Professor Emeritus, Macquarie Business School, Macquarie University
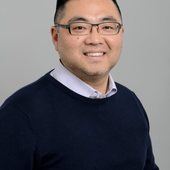
Senior Lecturer in International Relations, Discipline of Politics & International Relations, Macquarie School of Social Sciences, Macquarie University
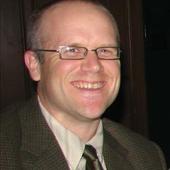
Professor of Forestry Operations, University of the Sunshine Coast
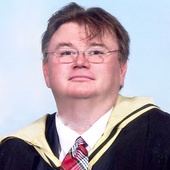
Senior Lecturer in International Economics, University of Dundee
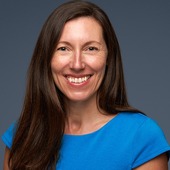
Royal Academy of Engineering Chair in Emerging Technologies - Intelligent & Resilient Ocean Engineering, University of Southampton
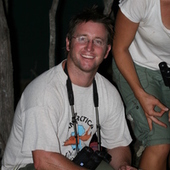
Professor, The University of Queensland
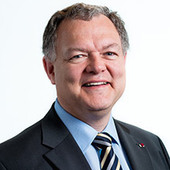
Professor, The University of Melbourne
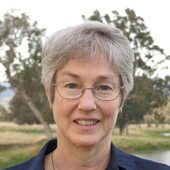
Adjunct Professor, University of New England
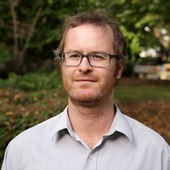
Academic Director, School for International Training, The University of Melbourne
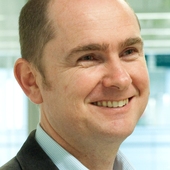
Research Director, Institute for Sustainable Futures, University of Technology Sydney
- X (Twitter)
- Unfollow topic Follow topic
Advertisement
A comprehensive review on green buildings research: bibliometric analysis during 1998–2018
- Environmental Concerns and Pollution control in the Context of Developing Countries
- Published: 16 February 2021
- Volume 28 , pages 46196–46214, ( 2021 )
Cite this article
- Li Ying 1 , 2 ,
- Rong Yanyu ORCID: orcid.org/0000-0003-0722-8510 1 , 3 ,
- Umme Marium Ahmad 1 ,
- Wang Xiaotong 1 , 3 ,
- Zuo Jian 4 &
- Mao Guozhu 1 , 3
17k Accesses
51 Citations
Explore all metrics
Buildings account for nearly 2/5ths of global energy expenditure. Due to this figure, the 90s witnessed the rise of green buildings (GBs) that were designed with the purpose of lowering the demand for energy, water, and materials resources while enhancing environmental protection efforts and human well-being over time. This paper examines recent studies and technologies related to the design, construction, and overall operation of GBs and determines potential future research directions in this area of study. This global review of green building development in the last two decades is conducted through bibliometric analysis on the Web of Science, via the Science Citation Index and Social Sciences Citation Index databases. Publication performance, countries’ characteristics, and identification of key areas of green building development and popular technologies were conducted via social network analysis, big data method, and S-curve predictions. A total of 5246 articles were evaluated on the basis of subject categories, journals’ performance, general publication outputs, and other publication characteristics. Further analysis was made on dominant issues through keyword co-occurrence, green building technologies by patent analysis, and S-curve predictions. The USA, China, and the UK are ranked the top three countries where the majority of publications come from. Australia and China had the closest relationship in the global network cooperation. Global trends of the top 5 countries showed different country characteristics. China had a steady and consistent growth in green building publications each year. The total publications on different cities had a high correlation with cities’ GDP by Baidu Search Index. Also, barriers and contradictions such as cost, occupant comfort, and energy consumption were discussed in developed and developing countries. Green buildings, sustainability, and energy efficiency were the top three hotspots identified through the whole research period by the cluster analysis. Additionally, green building energy technologies, including building structures, materials, and energy systems, were the most prevalent technologies of interest determined by the Derwent Innovations Index prediction analysis. This review reveals hotspots and emerging trends in green building research and development and suggests routes for future research. Bibliometric analysis, combined with other useful tools, can quantitatively measure research activities from the past and present, thus bridging the historical gap and predicting the future of green building development.
Similar content being viewed by others
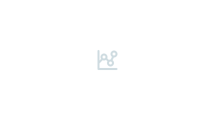
Scientometric of Nearly Zero Energy Building Research: A Systematic Review from the Perspective of Co-Citation Analysis
Mengmeng Wang, Xiaojun Liu, … Baiyu Chen
Determining Technologies Trends and Evolution of Smart Building Technologies by Bibliometric Analysis from 1984 to 2020
Determining Technologies Trends and Evolution of Smart Building Technologies by Bibliometric Analysis from 1984 to 2020
Avoid common mistakes on your manuscript.
Introduction
Rapid urban development has resulted in buildings becoming a massive consumer of energy (Yuan et al. 2013 ), liable for 39% of global energy expenditure and 68% of total electricity consumption in the USA (building). In recent years, green buildings (GBs) have become an alternative solution, rousing widespread attention. Also referred to as sustainable buildings, low energy buildings, and eco-buildings, GBs are designed to reduce the strain on environmental resources as well as curb negative effects on human health by efficiently using natural resources, reducing garbage, and ensuring the residents’ well-being through improved living conditions ( Agency USEP Indoor Air Quality ; Building, n.d ). As a strategy to improve the sustainability of the construction industry, GBs have been widely recognized by governments globally, as a necessary step towards a sustainable construction industry (Shen et al. 2017 ).
Zuo and Zhao ( 2014 ) reviewed the current research status and future development direction of GBs, focusing on connotation and research scope, the benefit-difference between GBs and traditional buildings, and various ways to achieve green building development. Zhao et al. ( 2019 ) presented a bibliometric report of studies on GBs between 2000 and 2016, identifying hot research topics and knowledge gaps. The verification of the true performance of sustainable buildings, the application of ICT, health and safety hazards in the development of green projects, and the corporate social responsibility were detected as future agenda. A scientometrics review of research papers on GB sources from 14 architectural journals between 1992 and 2018 was also presented (Wuni et al. 2019a ). The study reported that 44% of the world participated in research focusing on green building implementation; stakeholder management; attitude assessment; regulations and policies; energy efficiency assessment; sustainability performance assessment; green building certification, etc.
With the transmission of the COVID-19 virus, society is now aware of the importance of healthy buildings. In fact, in the past 20 years, the relationship between the built environment and health has aroused increasing research interest in the field of building science. Public spaces and dispersion of buildings in mixed-use neighborhoods are promoted. Furthermore, telecommuting has become a trend since the COVID-19 pandemic, making indoor air quality even more important in buildings, now (Fezi 2020 ).
The system for evaluating the sustainability of buildings has been established for nearly two decades. But, systems dedicated to identifying whether buildings are healthy have only recently appeared (McArthur and Powell 2020 ). People are paying more and more attention to health factors in the built environment. This is reflected in the substantial increase in related academic papers and the increase in health building certification systems such as WELE and Fitwel (McArthur and Powell 2020 ).
Taking the above into consideration, the aim of this study is to examine the stages of development of GBs worldwide and find the barriers and the hotpots in global trends. This study may be beneficial to foreign governments interested in promoting green building and research in their own nations.
Methodology
Overall description of research design.
Since it is difficult to investigate historical data and predict global trends of GBs, literature research was conducted to analyze their development. The number of published reports on a topic in a particular country may influence the level of industrial development in that certain area (Zhang et al. 2017 ). The bibliometric analysis allows for a quantitative assessment of the development and advancement of research related to GBs and where they are from. Furthermore, it has been shown that useful data has been gathered through bibliometrics and patent analysis (Daim et al. 2006 ).
In this report, the bibliometric method, social network analysis (SNA), CiteSpace, big data method, patent analysis, and S-curve analysis are used to assess data.
Bibliometrics analysis
Bibliometrics, a class of scientometrics, is a tool developed in 1969 for library and information science. It has since been adopted by other fields of study that require a quantitative assessment of academic articles to determine trends and predict future research scenarios by compiling output and type of publication, title, keyword, author, institution, and countries data (Ho 2008 ; Li et al. 2017 ).
Social network analysis
Social network analysis (SNA) is applied to studies by modeling network maps using mathematics and statistics (Mclinden 2013 ; Ye et al. 2013 ). In the SNA, nodes represent social actors, while connections between actors stand for their relationships (Zhang et al. 2017 ). Correlations between two actors are determined by their distance from each other. There is a variety of software for the visualization of SNA such as Gephi, Vosviewer, and Pajek. In this research, “Pajek” was used to model the sequence of and relationships between the objects in the map (Du et al. 2015 ).
CiteSpace is an open-source Java application that maps and analyzes trends in publication statistics gathered from the ISI-Thomson Reuters Scientific database and produces graphic representations of this data (Chen 2006 ; Li et al. 2017 ). Among its many functions, it can determine critical moments in the evolution of research in a particular field, find patterns and hotspots, locate areas of rapid growth, and breakdown the network into categorized clusters (Chen 2006 ).
Big data method
The big data method, with its 3V characters (volume, velocity, and variety), can give useful and accurate information. Enormous amounts of data, which could not be collected or computed manually through conventional methods, can now be collected through public data website. Based on large databases and machine learning, the big data method can be used to design, operate, and evaluate energy efficiency and other index combined with other technologies (Mehmood et al. 2019 ). The primary benefit of big data is that the data is gathered from entire populations as opposed to a small sample of people (Chen et al. 2018 ; Ho 2008 ). It has been widely used in many research areas. In this research, we use the “Baidu Index” to form a general idea of the trends in specific areas based on user interests. The popularity of the keywords could imply the user’s behavior, user’s demand, user’s portrait, etc. Thus, we can analyze the products or events to help with developing strategies. However, it must be noted that although big data can quantitatively represent human behavior, it cannot determine what motivates it. With the convergence of big data and technology, there are unprecedented applications in the field of green building for the improved indoor living environment and controlled energy consumption (Marinakis 2020 ).
- Patent analysis
Bibliometrics, combined with patent analysis, bridges gaps that may exist in historical data when predicting future technologies (Daim et al. 2006 ). It is a trusted form of technical analysis as it is supported by abundant sources and commercial awareness of patents (Guozhu et al. 2018 ; Yoon and Park 2004 ). Therefore, we used patent analysis from the Derwent patent database to conduct an initial analysis and forecast GB technologies.
There are a variety of methods to predict the future development prospects of a technology. Since many technologies are developed in accordance with the S-curve trend, researchers use the S-curve to observe and predict the future trend of technologies (Bengisu and Nekhili 2006 ; Du et al. 2019 ; Liu and Wang 2010 ). The evolution of technical systems generally goes through four stages: emerging, growth, maturity, and decay (saturation) (Ernst 1997 ). We use the logistics model (performed in Loglet Lab 4 software developed by Rockefeller University) to simulate the S-curve of GB-related patents to predict its future development space.
Data collection
The Web of Science (WOS) core collection database is made up of trustworthy and highly ranked journals. It is considered the leading data portal for publications in many fields (Pouris and Pouris 2011 ). Furthermore, the WOS has been cited as the main data source in many recent bibliometric reviews on buildings (Li et al. 2017 ).
Access to all publications used in this paper was attained through the Science Citation Index-Expanded and the Social Sciences Citation Index databases. Because there is no relevant data in WOS before 1998, our examination focuses on 1998 to 2018. With consideration of synonyms, we set a series of green building-related words (see Appendix ) in titles, abstracts, and keywords for bibliometric analysis. For example, sustainable, low energy, zero energy, and low carbon can be substituted for green; housing, construction, and architecture can be a substitute for building (Zuo and Zhao 2014 ).
Analytical procedure
The study was conducted in three stages; data extraction was the first step where all the GB-related words were screened in WOS. Afterwards, some initial analysis was done to get a complete idea of GB research. Then, we made a further analysis on countries’ characteristics, dominant issues, and detected technology hotspots via patent analysis (Fig. 1 ).
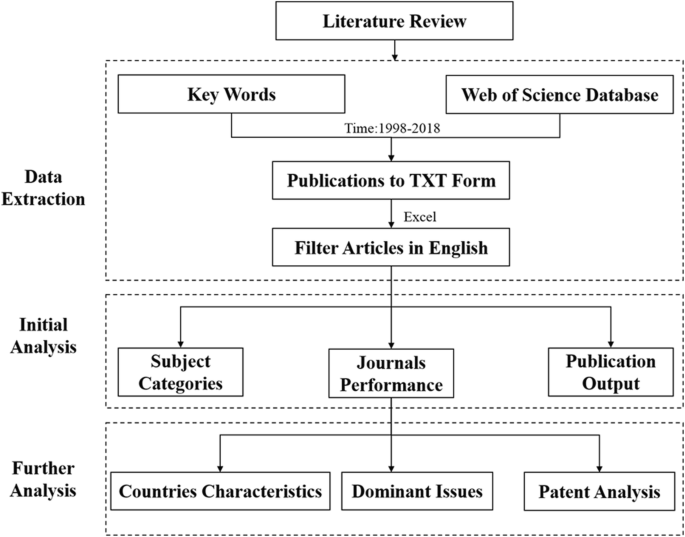
Analytical procedure of the article
Results and analysis
General results.
Of the 6140 publications searched in the database, 88.67% were articles, followed by reviews (6.80%), papers (3.72%), and others (such as editorial materials, news, book reviews). Most articles were written in English (96.78%), followed by German (1.77%), Spanish (0.91%), and other European languages. Therefore, we will only make a further analysis of the types of articles in English publications.
The subject categories and their distribution
The SCI-E and SSCI database determined 155 subjects from the pool of 5246 articles reviewed, such as building technology, energy and fuels, civil engineering, environmental, material science, and thermodynamics, which suggests green building is a cross-disciplinary area of research. The top 3 research areas of green buildings are Construction & Building Technology (36.98%), Energy & Fuels (30.39%), and Engineering Civil (29.49%), which account for over half of the total categories.
The journals’ performance
The top 10 journals contained 38.8% of the 5246 publications, and the distribution of their publications is shown in Fig. 2 . Impact factors qualitatively indicate the standard of journals, the research papers they publish, and researchers associated with those papers (Huibin et al. 2015 ). Below, we used 2017 impact factors in Journal Citation Reports (JCR) to determine the journal standards.
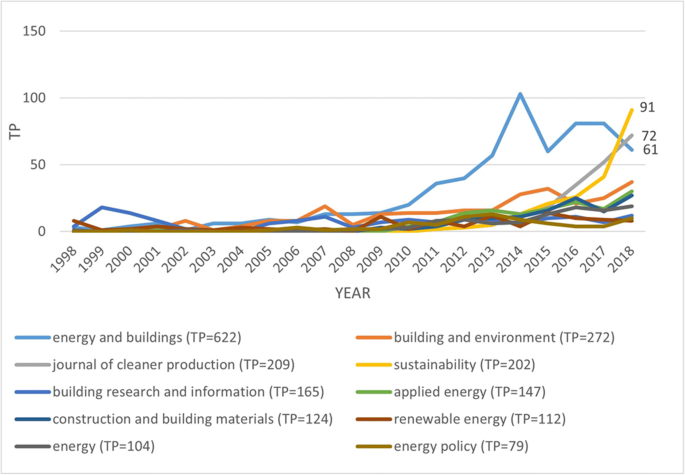
The performance of top10 most productive journals
Publications on green building have appeared in a variety of titles, including energy, building, environment, materials, sustainability, indoor built environment, and thermal engineering. Energy and Buildings, with its impact factor 4.457, was the most productive journal apparently from 2009 to 2017. Sustainability (IF = 2.075) and Journal of Cleaner Production (IF = 5.651) rose to significance rapidly since 2015 and ranked top two journals in 2018.
Publication output
The total publication trends from 1998 to 2018 are shown in Fig. 3 , which shows a staggering increase across the 10 years. Since there was no relevant data before 1998, the starting year is 1998. Before 2004, the number of articles published per year fluctuated. The increasing rate reached 75% and 68% in 2004 and 2007, respectively, which are distinguished in Fig. 3 that leads us to believe that there are internal forces at work, such as appropriate policy creation and enforcement by concerned governments. There was a constant and steady growth in publications after 2007 in the worldwide view.
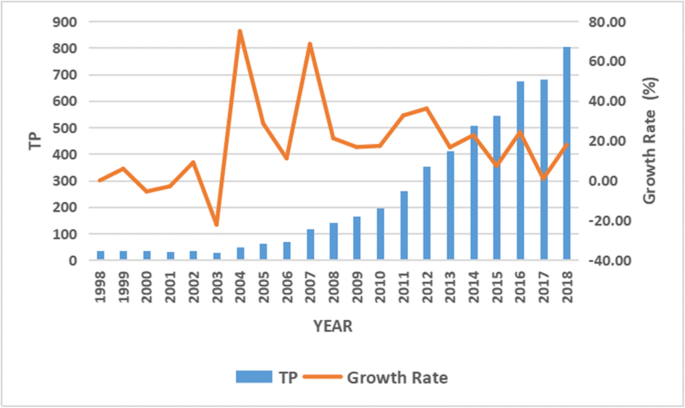
The number of articles published yearly, between 1998 and 2018
The characteristics of the countries
Global distribution and global network were analyzed to illustrate countries’ characteristics. Many tools such as ArcGIS, Bibexcel, Pajek, and Baidu index were used in this part (Fig. 4 ).
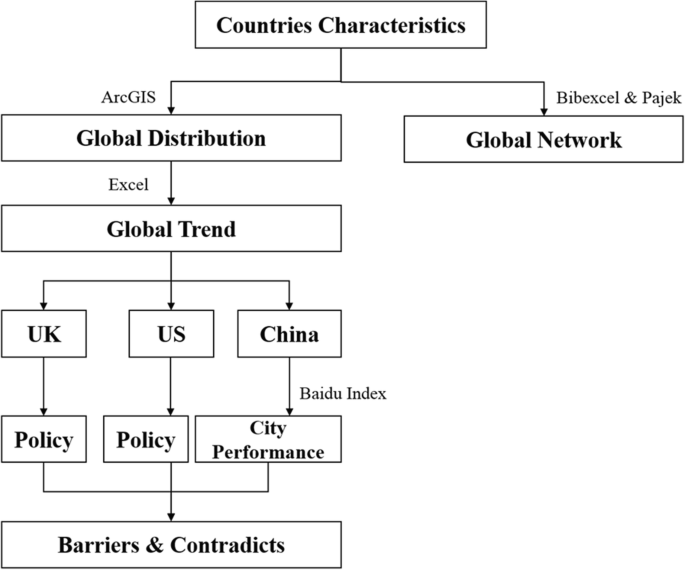
Analysis procedure of countries’ characteristics
Global distribution of publications
By extracting the authors’ addresses (Mao et al. 2015 ), the number of publications from each place was shown in Fig. 5 and Table 1 . Apparently, the USA was the most productive country accounting for 14.98% of all the publications. China (including Hong Kong and Taiwan) and the UK followed next by 13.29% and 8.27% separately. European countries such as Italy, Spain, and Germany also did a lot of work on green building development.
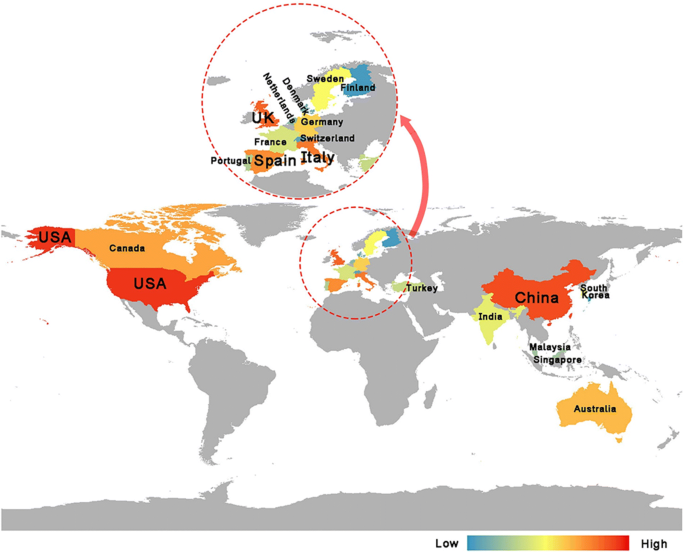
Global geographical distribution of the top 20 publications based on authors’ locations
Global research network
Global networks illustrate cooperation between countries through the analysis of social networks. Academic partnerships among the 10 most productive countries are shown in Fig. 6 . Collaboration is determined by the affiliation of the co-authors, and if a publication is a collaborative research, all countries or institutions will benefit from it (Bozeman et al. 2013 ). Every node denotes a country and their size indicates the amount of publications from that country. The lines linking the nodes denote relationships between countries and their thickness indicates the level of collaboration (Mao et al. 2015 ).
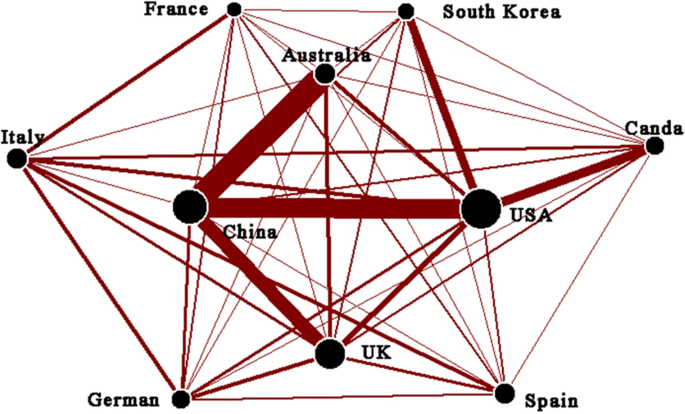
The top 10 most productive countries had close academic collaborative relationships
It was obvious that China and Australia had the strongest linking strength. Secondly, China and the USA, China, and the UK also had close cooperation with each other. Then, the USA with Canada and South Korea followed. The results indicated that cooperation in green building research was worldwide. At the same time, such partnerships could help countries increase individual productivity.
Global trend of publications
The time-trend analysis of academic inputs to green building from the most active countries is shown in Fig. 7 .
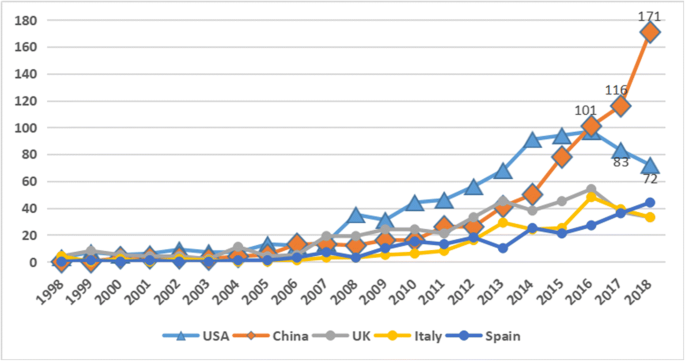
The publication trends of the top five countriesbetween 1998 and 2018 countries areshown in Fig 7 .
Before 2007, these countries showed little growth per year. However, they have had a different, growing trend since 2007. The USA had the greatest proportion of publications from 2007, which rose obviously each year, reaching its peak in 2016 then declined. The number of articles from China was at 13 in 2007, close to the USA. Afterwards, there was a steady growth in China. Not until 2013 did China have a quick rise from 41 publications to 171 in 2018. The UK and Italy had a similar growth trend before 2016 but declined in the last 2 years.
Further analysis on China, the USA, and the UK
Green building development in china, policy implementation in china.
Green building design started in China with the primary goal of energy conservation. In September 2004, the award of “national green building innovation” of the Ministry of Construction was launched, which kicked off the substantive development of GB in China. As we can see from Fig. 7 , there were few publications before 2004 in China. In 2004, there were only 4 publications on GB.
The Ministry of Construction, along with the Ministry of Science and Technology, in 2005, published “The Technical Guidelines for Green Buildings,” proposing the development of GBs (Zhang et al. 2018 ). In June 2006, China had implemented the first “Evaluation Standard for Green Building” (GB/T 50378-2006), which promoted the study of the green building field. In 2007, the demonstration of “100 projects of green building and 100 projects of low-energy building” was launched. In August 2007, the Ministry of Construction issued the “Green Building Assessment Technical Regulations (try out)” and the “Green Building Evaluation Management,” following Beijing, Tianjin, Chongqing, and Shanghai, more than 20 provinces and cities issued the local green building standards, which promoted GBs in large areas in China.
At the beginning of 2013, the State Council issued the “Green Building Action Plan,” so the governments at all levels continuously issued incentive policies for the development of green buildings (Ye et al. 2015 ). The number of certified green buildings has shown a blowout growth trend throughout the country, which implied that China had arrived at a new chapter of development.
In August 2016, the Evaluation Standard for Green Renovation of Existing Buildings was released, encouraging the rise of residential GB research. Retrofitting an existing building is often more cost-effective than building a new facility. Designing significant renovations and alterations to existing buildings, including sustainability measures, will reduce operating costs and environmental impacts and improve the building’s adaptability, durability, and resilience.
At the same time, a number of green ecological urban areas have emerged (Zhang et al. 2018 ). For instance, the Sino-Singapore Tianjin eco-city is a major collaborative project between the two governments. Located in the north of Tianjin Binhai New Area, the eco-city is characterized by salinization of land, lack of freshwater, and serious pollution, which can highlight the importance of eco-city construction. The construction of eco-cities has changed the way cities develop and has provided a demonstration of similar areas.
China has many emerging areas and old centers, so erecting new, energy efficiency buildings and refurbishing existing buildings are the best steps towards saving energy.
Baidu Search Index of “green building”
In order to know the difference in performance among cities in China, this study employs the big data method “Baidu Index” for a smart diagnosis and assessment on green building at finer levels. “Baidu Index” is not equal to the number of searches but is positively related to the number of searches, which is calculated by the statistical model. Based on the keyword search of “green building” in the Baidu Index from 2013 to 2018, the top 10 provinces or cities were identified (Fig. 8 ).
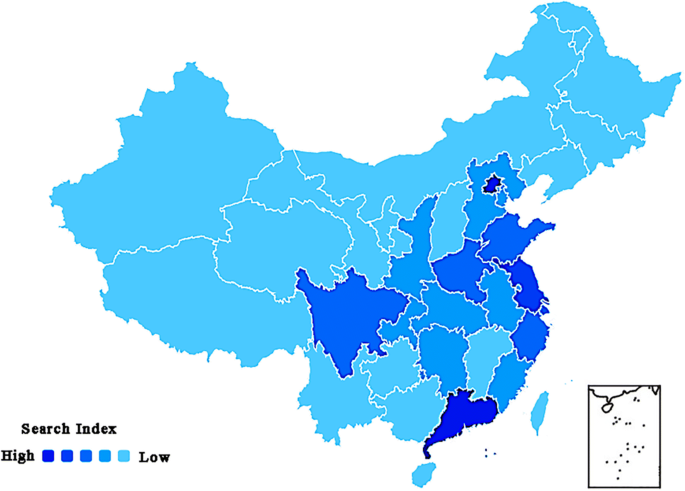
Baidu Search Index of green building in China 2013–2018 from high to low
The top 10 search index distributes the east part and middle part of China, most of which are the high GDP provinces (Fig. 9 ). Economically developed cities in China already have a relatively mature green building market. Many green building projects with local characteristics have been established (Zhang et al. 2018 ).
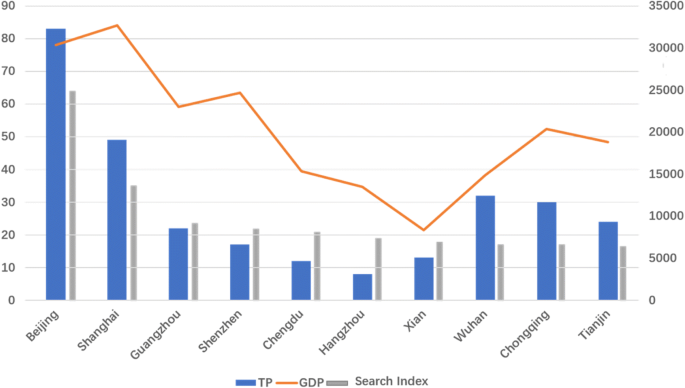
TP GDP & Search Index were highly related
We compared the city search index (2013–2018) with the total publications of different cities by the authors’ address and the GDP in 2018. The correlation coefficient between the TP and the search index was 0.9, which means the two variables are highly related. The correlation coefficient between the TP and GDP was 0.73, which also represented a strong relationship. We inferred that cities with higher GDP had more intention of implementation on green buildings. The stronger the local GDP, the more relevant the economic policies that can be implemented to stimulate the development of green buildings (Hong et al. 2017 ). Local economic status (Yang et al. 2018 ), property developer’s ability, and effective government financial incentives are the three most critical factors for green building implementation (Huang et al. 2018 ). However, Wang et al. ( 2017 ) compared the existing green building design standards and found that they rarely consider the regional economy. Aiming at cities at different economic development phases, the green building design standards for sustainable construction can effectively promote the implementation of green buildings. Liu et al. ( 2020 ) mainly discussed the impact of sustainable construction on GDP. According to the data, there is a strong correlation between the percentage of GDP increments in China and the amount of sustainable infrastructure (Liu et al. 2020 ). The construction of infrastructure can create jobs and improve people’s living standards, increasing GDP as a result (Liu et al. 2020 ).
Green building development in the USA and the UK
The sign that GBs were about to take-off occurred in 1993—the formation of the United States Green Building Council (USGBC), an independent agency. The promulgation of the Energy Policy Act 2005 in the USA was the key point in the development of GBs. The Energy Policy Act 2005 paid great attention to green building energy saving, which also inspired publications on GBs.
Leadership in Energy and Environmental Design (LEED), a popular metric for sustainable buildings and homes (Jalaei and Jrade 2015 ), has become a thriving business model for green building development. It is a widely used measure of how buildings affect the environment.
Another phenomenon worth discussion, combined with Fig. 7 , the increasing rate peaked at 75% in 2004 and 68% in 2007 while the publications of the UK reached the peak in 2004 and 2007. The UK Green Building Council (UKGBC), a United Kingdom membership organization, created in 2007 with regard to the 2004 Sustainable Building Task Group Report: Better Buildings - Better Lives, intends to “radically transform,” all facets of current and future built environment in the UK. It is predicted that the establishment of the UKGBC promoted research on green buildings.
From the China, the USA, and the UK experience, it is predicted that the foundation of a GB council or the particular projects from the government will promote research in this area.
Barriers and contradicts of green building implement
On the other hand, it is obvious that the USA, the UK, and Italian publications have been declining since 2016. There might be some barriers and contradicts on the adoption of green buildings for developed countries. Some articles studied the different barriers to green building in developed and developing countries (Chan et al. 2018 ) (Table 2 ). Because the fraction of energy end-uses is different, the concerns for GBs in the USA, China, and the European Union are also different (Cao et al. 2016 ).
It is regarded that higher cost is the most deterring barrier to GB development across the globe (Nguyen et al. 2017 ). Other aspects such as lack of market demand and knowledge were also main considerations of green building implementation.
As for market demand, occupant satisfaction is an important factor. Numerous GB post-occupancy investigations on occupant satisfaction in various communities have been conducted.
Paul and Taylor ( 2008 ) surveyed personnel ratings of their work environment with regard to ambience, tranquility, lighting, sound, ventilation, heat, humidity, and overall satisfaction. Personnel working in GBs and traditional buildings did not differ in these assessments. Khoshbakht et al. ( 2018 ) identified two global contexts in spite of the inconclusiveness: in the west (mainly the USA and Britain), users experienced no significant differences in satisfaction between green and traditional buildings, whereas, in the east (mainly China and South Korea), GB user satisfaction is significantly higher than traditional building users.
Dominant issues
The dominant issues on different stages.
Bibliometric data was imported to CiteSpace where a three-stage analysis was conducted based on development trends: 1998–2007 initial development; 2008–2015 quick development; 2016–2018 differentiation phase (Fig. 10 ).
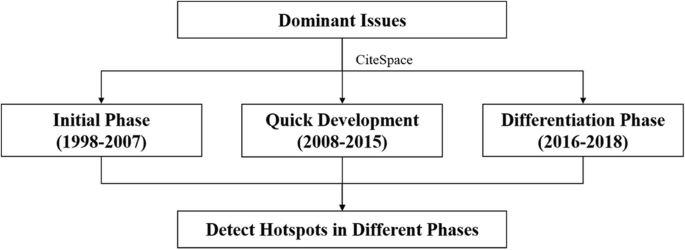
Analysis procedure of dominant issues
CiteSpace was used for word frequency and co-word analysis. The basic principle of co-word analysis is to count a group of words appearing at the same time in a document and measure the close relationship between them by the number of co-occurrences. The top 50 levels of most cited or occurred items from each slice (1998 to 2007; 2008 to 2015; 2016 to 2018) per year were selected. After merging the similar words (singular or plural form), the final keyword knowledge maps were generated as follows.
Initial phase (1998–2007)
In the early stage (Fig. 11 ), “green building” and “sustainability” were the main two clusters. Economics and “environmental assessment method” both had high betweenness centrality of 0.34 which were identified as pivotal points. Purple rings denote pivotal points in the network. The relationships in GB were simple at the initial stage of development.
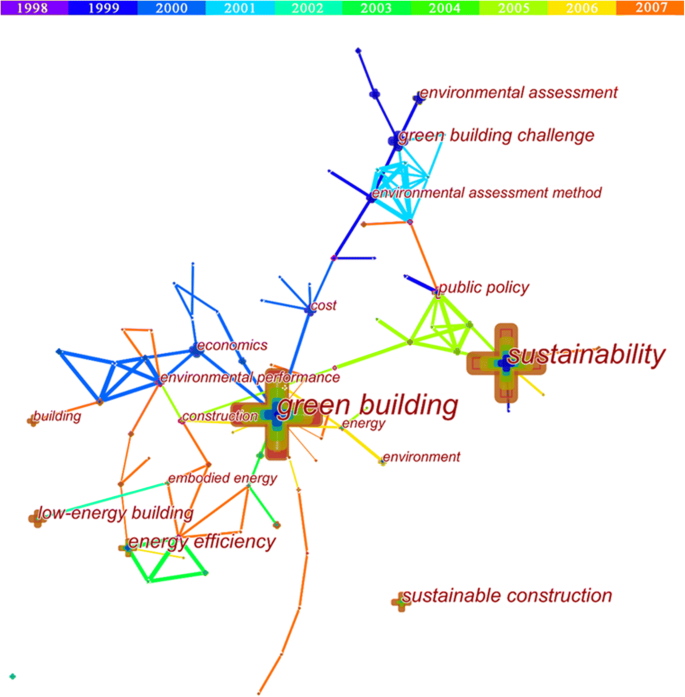
Co-word analysis from 1998–2007
Sustainable construction is further enabled with tools that can evaluate the entire life cycle, site preparation and management, materials and their reusability, and the reduction of resource and energy consumption. Environmental building assessment methods were incorporated to achieve sustainable development, especially at the initial project appraisal stage (Ding 2008 ). Green Building Challenge (GBC) is an exceptional international research, development, and dissemination effort for developing building environmental performance assessments, primarily to help researchers and practitioners in dealing with difficult obstacles in assessing performance (Todd et al. 2001 ).
Quick development (2008–2015)
In the rapid growing stage (Fig. 12 ), pivot nodes and cluster centers were more complicated. Besides “green building” and “sustainability,” “energy efficiency” was the third hotspot word. The emergence of new vocabulary in the keyword network indicated that the research had made progress during 2008 – 2015. Energy performance, energy consumption, natural ventilation, thermal comfort, renewable energy, and embodied energy were all energy related. Energy becomes the most attractive field in achieving sustainability and green building. Other aspects such as “life cycle assessment,” “LEED,” and “thermal comfort” became attractive to researchers.
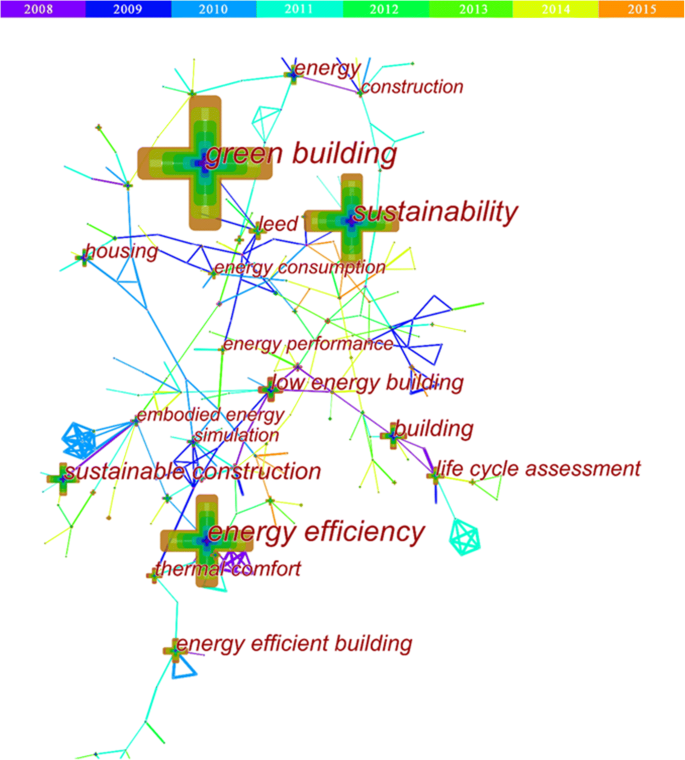
Co-word analysis from 2008–2015
The life cycle assessment (LCA) is a popular technique for the analysis of the technical side of GBs. LCA was developed from environmental assessment and economic analysis which could be a useful method to evaluate building energy efficiency from production and use to end-use (Chwieduk 2003 ). Much attention has been paid to LCA because people began to focus more on the actual performance of the GBs. Essentially, LCA simplifies buildings into systems, monitoring, and calculating mass flow and energy consumption over different stages in their life cycle.
Leadership in Energy and Environmental Design (LEED) was founded by the USGBC and began in the early twenty-first century (Doan et al. 2017 ). LEED is a not-for-profit project based on consumer demand and consensus that offers an impartial GB certification. LEED is the preferred building rating tool globally, with its shares growing rapidly. Meanwhile, UK’s Building Research Establishment Assessment Method (BREEAM) and Japan’s Comprehensive Assessment System for Building Environmental Efficiency (CASBEE) have been in use since the beginning of the twenty-first century, while New Zealand’s Green Star is still in its earlier stages. GBs around the world are made to suit regional climate concerns and need.
In practice, not all certified green buildings are necessarily performing well. Newsham et al. ( 2009 ) gathered energy-use information from 100 LEED-certified non-residential buildings. Results indicated that 28–35% of LEED structures actually consumed higher amounts of energy than the non-LEED structures. There was little connection in its actual energy consumption to its certification grade, meaning that further improvements are required for establishing a comprehensive GB rating metric to ensure consistent performance standards.
Thermal comfort was related to many aspects, such as materials, design scheme, monitoring system, and human behaviors. Materials have been a focus area for improving thermal comfort and reducing energy consumption. Wall (Schossig et al. 2005 ), floor (Ansuini et al. 2011 ), ceiling (Hu et al. 2018 ), window, and shading structures (Shen and Li 2016 ) were building envelopes which had been paid attention to over the years. Windows were important envelopes to improve thermal comfort. For existing and new buildings, rational use of windows and shading structures can enhance the ambient conditions of buildings (Mcleod et al. 2013 ). It was found that redesigning windows could reduce the air temperature by 2.5% (Elshafei et al. 2017 ), thus improving thermal comfort through passive features and reducing the use of active air conditioners (Perez-Fargallo et al. 2018 ). The monitoring of air conditioners’ performance could also prevent overheating of buildings (Ruellan and Park 2016 ).
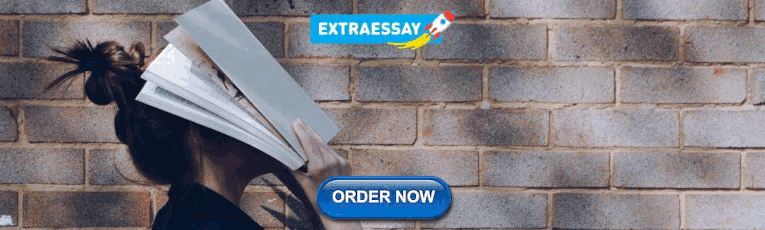
Differentiation phase (2016–2018)
In the years from 2016 to 2018 (Fig. 13 ), “green building,” ”sustainability,” and “energy efficiency” were still the top three hotspots in GB research.
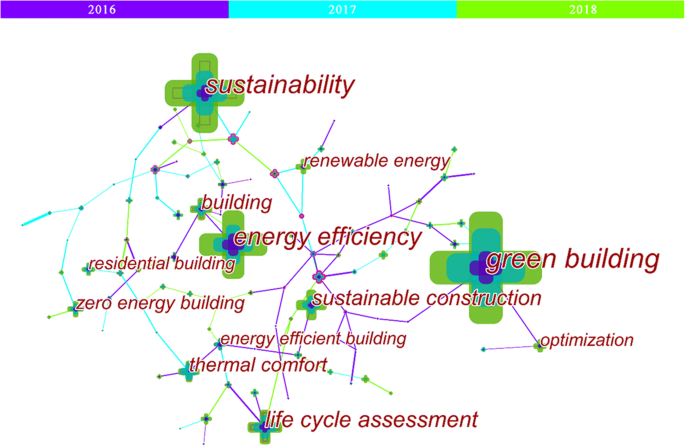
Co-word analysis from 2016–2018
Zero-energy building (ZEB) became a substitute for low energy building in this stage. ZEB was first introduced in 2000 (Cao et al. 2016 ) and was believed to be the solution to the potential ramifications of future energy consumption by buildings (Liu et al. 2019 ). The EU has been using ZEB standards in all of its new building development projects to date (Communuties 2002 ). The USA passed the Energy Independence and Security Act of 2007, aiming for zero net energy consumption of 1 out of every 2 commercial buildings that are yet to be built by 2040 and for all by 2050 (Sartori et al. 2012 ). Energy consumption became the most important factor in new building construction.
Renewable energy was a key element of sustainable development for mankind and nature (Zhang et al. 2013 ). Using renewable energy was an important feature of ZEBs (Cao et al. 2016 ; Pulselli et al. 2007 ). Renewable energy, in the form of solar, wind, geothermal, clean bioenergy, and marine can be used in GBs. Solar energy has been widely used in recent years while wind energy is used locally because of its randomness and unpredictable features. Geothermal energy is mainly utilized by ground source heat pump (GSHP), which has been lauded as a powerful energy system for buildings (Cao et al. 2016 ). Bioenergy has gained much popularity as an alternative source of energy around the globe because it is more stable and accessible than other forms of energy (Zhang et al. 2015 ). There is relatively little use of marine energy, yet this may potentially change depending on future technological developments (Ellabban et al. 2014 ).
Residential buildings receive more attention because people spend 90% of their time inside. Contrary to popular belief, the concentration of contaminants found indoors is more than the concentration outside, sometimes up to 10 times or even 100 times more (agency). The renovation of existing buildings can save energy, upgrade thermal comfort, and improve people’s living conditions.
Energy is a substantial and widely recognized cost of building operations that can be reduced through energy-saving and green building design. Nevertheless, a consensus has been reached by academics and those in building-related fields that GBs are significantly more energy efficient than traditional buildings if designed, constructed, and operated with meticulousness (Wuni et al. 2019b ). The drive to reduce energy consumption from buildings has acted as a catalyst in developing new technologies.
Compared with the article analysis, patents can better reflect the practical technological application to a certain extent. We extracted the information of green building energy-related patent records between 1998 and 2018 from the Derwent Innovations Index database. The development of a technique follows a path: precursor–invention–development–maturity. This is commonly known as an S-type growth (Mao et al. 2018 ). Two thousand six hundred thirty-eight patents were found which were classified into “Derwent Manual Code,” which is the most distinct feature just like “keywords” in the Derwent Innovations Index. Manual codes refer to specific inventions, technological innovations, and unique codes for their applications. According to the top 20 Derwent Manual Code which accounted for more than 80% of the total patents, we classified the hotspots patents into three fields for further S-curve analysis, which are “structure,” “material,” and “energy systems” (Table 3 ).
Sustainable structural design (SSD) has gained a lot of research attention from 2006 to 2016 (Pongiglione and Calderini 2016 ). The S-curve of structure* (Fig. 14 ) has just entered the later period of the growth stage, accounting for 50% of the total saturation in 2018. Due to its effectiveness and impact, SSD has overtime gained recognition and is now considered by experts to be a prominent tool in attaining sustainability goals (Pongiglione and Calderini 2016 ).
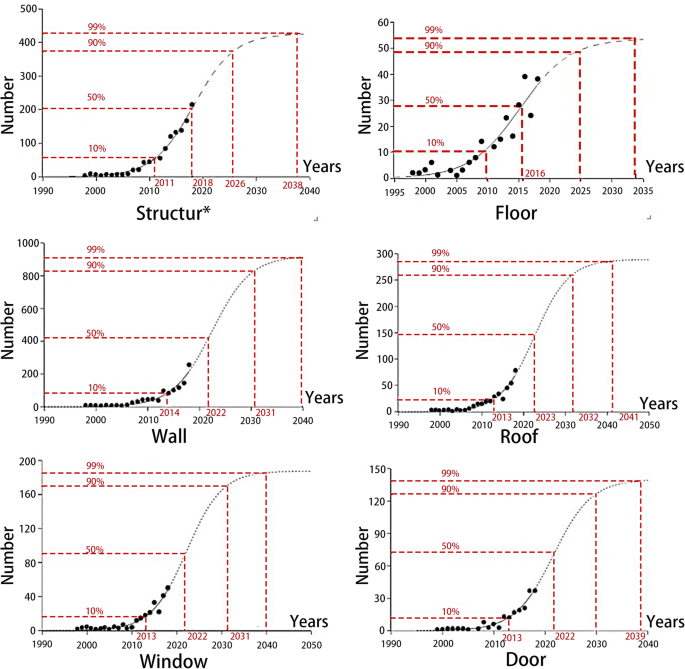
The S-curves of different Structure types from patents
Passive design is important in energy saving which is achieved by appropriately orientating buildings and carefully designing the building envelope. Building envelopes, which are key parts of the energy exchange between the building and the external environment, include walls, roofs, windows, and floors. The EU increased the efficiency of its heat-regulating systems by revamping building envelopes as a primary energy-saving task during 2006 to 2016 (Cao et al. 2016 ).
We analyzed the building envelope separately. According to the S-curve (Fig. 14 ), the number of patents related to GB envelops are in the growth stage. At present, building envelops such as walls, roofs, windows, and even doors have not reached 50% of the saturated quantity. Walls and roofs are two of the most important building envelops. The patent contents of walls mainly include wall materials and manufacturing methods, modular wall components, and wall coatings while technologies about roofs mainly focus on roof materials, the combination of roof and solar energy, and roof structures. Green roofs are relatively new sustainable construction systems because of its esthetic and environmental benefits (Wei et al. 2015 ).
The material resources used in the building industry consume massive quantities of natural and energy resources consumptions (Wang et al. 2018 ). The energy-saving building material is economical and environmentally friendly, has low coefficient heat conductivity, fast curing speed, high production efficacy, wide raw material source and flame, and wear resistance properties (Zhang et al. 2014 ). Honeycomb structures were used for insulating sustainable buildings. They are lightweight and conserve energy making them eco-friendly and ideal for construction (Miao et al. 2011 ).
According to the S-curve (Fig. 15 ), it can be seen that the number of patents on the GB “material” is in the growth stage. It is expected that the number of patents will reach 50% of the total saturation in 2022.
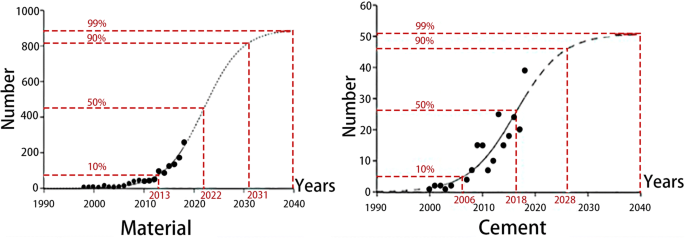
The S-curves of a different material from patents
Building material popularly used comprised of cement, concrete, gypsum, mortar compositions, and boards. Cement is widely used in building material because of its easy availability, strong hardness, excellent waterproof and fireproof performance, and low cost. The S-curve of cement is in the later period of the growth stage, which will reach 90% of the total saturation in 2028. Composite materials like Bamcrete (bamboo-concrete composite) and natural local materials like Rammed Earth had better thermal performance compared with energy-intensive materials like bricks and cement (Kandya and Mohan 2018 ). Novel bricks synthesized from fly ash and coal gangue have better advantages of energy saving in brick production phases compared with that of conventional types of bricks (Zhang et al. 2014 ). For other materials like gypsum or mortar, the numbers of patents are not enough for S-curve analysis. New-type green building materials offer an alternative way to realize energy-saving for sustainable constructions.
Energy system
The energy system mainly included a heating system and ventilation system according to the patent analysis. So, we analyzed solar power systems and air conditioning systems separately. Heat* included heat collecting panels and a fluid heating system.
The results indicated that heat*-, solar-, and ventilation-related technologies were in the growth stage which would reach 50% of the total saturation in 2022 (Fig. 16 ). Photovoltaic technology is of great importance in solar energy application (Khan and Arsalan 2016 ).
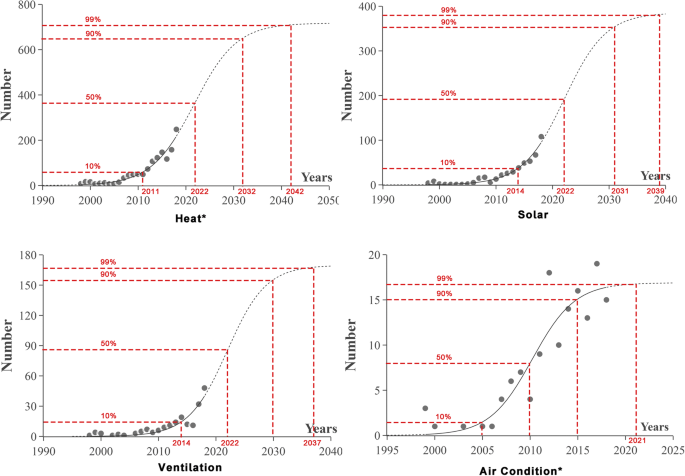
The S-curves of energy systems from patents
On the contrary, air conditioning technologies had entered into the mature stage after a decade of development. It is worth mentioning that the design of the fresh air system of buildings after the COVID-19 outbreak is much more important. With people spending the majority of their time inside (Liu et al. 2019 ), volatile organic compounds, formaldehyde, and carbon dioxide received the most attention worldwide (Wei et al. 2015 ). Due to health problems like sick building syndrome, and more recently since the COVID-19 outbreak, the supply of fresh air can drastically ameliorate indoor air quality (IAQ) (Liu et al. 2019 ). Regulating emissions from materials, enhanced ventilation, and monitoring air indoors are the main methods used in GBs for maintaining IAQ (Wei et al. 2015 ). Air circulation frequency and improved air filtration can reduce the risk of spreading certain diseases, while controlling the airflow between rooms can also prevent cross-infections. Poor indoor air quality and ventilation provide ideal conditions for the breeding and spreading of viruses by air (Chen et al. 2019 ). A diverse range of air filters coupled with a fresh air supply system should be studied. A crucial step forward is to create a cost-effective, energy-efficient, intelligent fresh air supply system (Liu et al. 2017 ) to monitor, filter outdoor PM2.5 (Chen et al. 2017 ), and saving building energy (Liu and Liu 2005 ). Earth-air heat exchanger system (EAHE) is a novel technology that supplies fresh air using underground soil heat (Chen et al. 2019 ).
A total of 5246 journal articles in English from the SCI and SSCI databases published in 1998–2018 were reviewed and analyzed. The study revealed that the literature on green buildings has grown rapidly over the past 20 years. The findings and results are summarized:
Data analysis revealed that GB research is distributed across various subject categories. Energy and Buildings, Building and Environment, Journal of Cleaner Production, and Sustainability were the top journals to publish papers on green buildings.
Global distribution was done to see the green building study worldwide, showing that the USA, China, and the UK ranked the top three countries, accounting for 14.98%, 13.29%, and 8.27% of all the publications respectively. Australia and China had the closest relationship on green building research cooperation worldwide.
Further analysis was made on countries’ characteristics, dominant issues through keyword co-occurrence, green building technology by patent analysis, and S-curve prediction. Global trends of the top 5 countries showed different characteristics. China had a steady and consistent growth in publications each year while the USA, the UK, and Italy were on a decline from 2016. The big data method was used to see the city performance in China, finding that the total publications had a high correlation with the city’s GDP and Baidu Search Index. Policies were regarded as the stimulation for green building development, either in China or the UK. Also, barriers and contradictions such as cost, occupants’ comfort, and energy consumption were discussed about the developed and developing countries.
Cluster and content analysis via CiteSpace identified popular and trending research topics at different stages of development; the top three hotspots were green buildings, sustainability, and energy efficiency throughout the whole research period. Energy efficiency has shifted from low to zero energy buildings or even beyond it in recent years. Energy efficiency was the most important drive to achieve green buildings while LCA and LEED were the two potential ways to evaluate building performance. Thermal comfort and natural ventilation of residential buildings became a topic of interest to the public.
Then, we combined the keywords with “energy” to make further patent analysis in Derwent Innovations Index. “Structure,” “material,” and “energy systems” were three of the most important types of green building technologies. According to S-curve analysis, most of the technologies of energy-saving buildings were on the fast-growing trend, and even though there were conflicts and doubts in different countries on GB adoption, it is still a promising field.
Future directions
An establishment of professional institutes or a series of policies and regulations on green building promulgated by government departments will promote research development (as described in the “Further Analysis on China, the USA, and the UK” section). Thus, a policy enacted by a formal department is of great importance in this particular field.
Passive design is important in energy saving which is ensured by strategically positioning buildings and precisely engineering the building envelope, i.e., roof, walls, windows, and floors. A quality, the passive-design house is crucial to achieving sustained thermal comfort, low-carbon footprint, and a reduced gas bill. The new insulation material is a promising field for reducing building heat loss and energy consumed. Healthy residential buildings have become a focus of future development due to people’s pursuit of a healthy life. A fresh air supply system is important for better indoor air quality and reduces the risk of transmission of several diseases. A 2020 study showed the COVID-19 virus remains viable for only 4 hours on copper compared to 24 h on cardboard. So, antiviral materials will be further studied for healthy buildings (Fezi 2020 ).
With the quick development of big data method and intelligent algorithms, artificial intelligence (AI) green buildings will be a trend. The core purpose of AI buildings is to achieve optimal operating conditions through the accurate analysis of data, collected by sensors built into green buildings. “Smart buildings” and “Connected Buildings” of the future, fitted with meters and sensors, can collect and share massive amounts of information regarding energy use, water use, indoor air quality, etc. Analyzing this data can determine relationships and patterns, and optimize the operation of buildings to save energy without compromising the quality of the indoor environment (Lazarova-Molnar and Mohamed 2019 ).
The major components of green buildings, such as building envelope, windows, and skylines, should be adjustable and versatile in order to get full use of AI. A digital control system can give self-awareness to buildings, adjusting room temperature, indoor air quality, and air cooling/heating conditions to control power consumption, and make it sustainable (Mehmood et al. 2019 ).
Concerns do exist, for example, occupant privacy, data security, robustness of design, and modeling of the AI building (Maasoumy and Sangiovanni-Vincentelli 2016 ). However, with increased data sources and highly adaptable infrastructure, AI green buildings are the future.
This examination of research conducted on green buildings between the years 1998 and 2018, through bibliometric analysis combined with other useful tools, offers a quantitative representation of studies and data conducted in the past and present, bridging historical gaps and forecasting the future of green buildings—providing valuable insight for academicians, researchers, and policy-makers alike.
Agency USEP Indoor Air Quality https://www.epa.gov/indoor-air-quality-iaq.
Ansuini R, Larghetti R, Giretti A, Lemma M (2011) Radiant floors integrated with PCM for indoor temperature control. Energy Build 43:3019–3026. https://doi.org/10.1016/j.enbuild.2011.07.018
Article Google Scholar
Bengisu M, Nekhili R (2006) Forecasting emerging technologies with the aid of science and technology databases. Technol Forecast Soc Chang 73:835–844. https://doi.org/10.1016/j.techfore.2005.09.001
Bozeman B, Fay D, Slade CP (2013) Research collaboration in universities and academic entrepreneurship: the-state-of-the-art. J Technol Transf 38:1–67. https://doi.org/10.1007/s10961-012-9281-8
Building AG Importance of Green Building. https://www.greenbuilt.org/about/importance-of-green-building
Cao XD, Dai XL, Liu JJ (2016) Building energy-consumption status worldwide and the state-of-the-art technologies for zero-energy buildings during the past decade. Energy Build 128:198–213. https://doi.org/10.1016/j.enbuild.2016.06.089
Chan APC, Darko A, Olanipekun AO, Ameyaw EE (2018) Critical barriers to green building technologies adoption in developing countries: the case of Ghana. J Clean Prod 172:1067–1079. https://doi.org/10.1016/j.jclepro.2017.10.235
Chen CC, Lo TH, Tsay YS, Lee CY, Liu KS (2017) Application of a novel formaldehyde sensor with MEMS (micro electro mechanical systems) in indoor air quality test and improvement in medical spaces. Appl Ecol Environ Res 15:81–89. https://doi.org/10.15666/aeer/1502_081089
Chen CM (2006) CiteSpace II: detecting and visualizing emerging trends and transient patterns in scientific literature. J Am Soc Inf Sci Technol 57:359–377. https://doi.org/10.1002/asi.20317
Chen X, Lu WS, Xue F, Xu JY (2018) A cost-benefit analysis of green buildings with respect to construction waste minimization using big data in Hong Kong. J Green Build 13:61–76. https://doi.org/10.3992/1943-4618.13.4.61
Chen XY, Niu RP, Lv LN, Kuang DQ, LOP (2019) Discussion on existing problems of fresh air system. In: 4th International Conference on Advances in Energy Resources and Environment Engineering, vol 237. IOP Conference Series-Earth and Environmental Science. Iop Publishing Ltd, Bristol. doi: https://doi.org/10.1088/1755-1315/237/4/042030
Chwieduk D (2003) Towards sustainable-energy buildings. Appl Energy 76:211–217. https://doi.org/10.1016/s0306-2619(03)00059-x
Communuties CotE (2002) Directive of the European parliament and the council.
Daim TU, Rueda G, Martin H, Gerdsri P (2006) Forecasting emerging technologies: use of bibliometrics and patent analysis. Technol Forecast Soc Chang 73:981–1012. https://doi.org/10.1016/j.techfore.2006.04.004
Ding GKC (2008) Sustainable construction - the role of environmental assessment tools. J Environ Manag 86:451–464. https://doi.org/10.1016/j.jenvman.2006.12.025
Doan DT, Ghaffarianhoseini A, Naismith N, Zhang TR, Ghaffarianhoseini A, Tookey J (2017) A critical comparison of green building rating systems. Build Environ 123:243–260. https://doi.org/10.1016/j.buildenv.2017.07.007
Du HB, Li BL, Brown MA, Mao GZ, Rameezdeen R, Chen H (2015) Expanding and shifting trends in carbon market research: a quantitative bibliometric study. J Clean Prod 103:104–111. https://doi.org/10.1016/j.jclepro.2014.05.094
Du HB, Liu DY, Lu ZM, Crittenden J, Mao GZ, Wang S, Zou HY (2019) Research development on sustainable urban infrastructure from 1991 to 2017: a bibliometric analysis to inform future innovations. Earth Future 7:718–733. https://doi.org/10.1029/2018ef001117
Ellabban O, Abu-Rub H, Blaabjerg F (2014) Renewable energy resources: current status, future prospects and their enabling technology. Renew Sust Energ Rev 39:748–764. https://doi.org/10.1016/j.rser.2014.07.113
Elshafei G, Negm A, Bady M, Suzuki M, Ibrahim MG (2017) Numerical and experimental investigations of the impacts of window parameters on indoor natural ventilation in a residential building. Energy Build 141:321–332. https://doi.org/10.1016/j.enbuild.2017.02.055
Ernst H (1997) The use of patent data for technological forecasting: the diffusion of CNC-technology in the machine tool industry. Small Bus Econ 9:361–381. https://doi.org/10.1023/A:1007921808138
Fezi BA (2020) Health engaged architecture in the context of COVID-19. J Green Build 15:185–212
Guozhu et al (2018) Bibliometric analysis of insights into soil remediation. J Soils Sediments 18:2520–2534
Ho S-Y (2008) Bibliometric analysis of biosorption technology in water treatment research from 1991 to 2004. Int J Environ Pollut 34:1–13. https://doi.org/10.1504/ijep.2008.020778
Article CAS Google Scholar
Hong WX, Jiang ZY, Yang Z, (2017) Iop (2017) Analysis on the restriction factors of the green building scale promotion based on DEMATEL. In: 2nd International Conference on Advances in Energy Resources and Environment Engineering, vol 59. IOP Conference Series-Earth and Environmental Science. Iop Publishing Ltd, Bristol. doi: https://doi.org/10.1088/1755-1315/59/1/012064
Hu J, Kawaguchi KI, Ma JJB (2018) Retractable membrane ceiling on indoor thermal environment of residential buildings. Build Environ 146:289–298. https://doi.org/10.1016/j.buildenv.2018.09.035
Huang N, Bai LB, Wang HL, Du Q, Shao L, Li JT (2018) Social network analysis of factors influencing green building development in China. Int J Environ Res Public Health 15:16. https://doi.org/10.3390/ijerph15122684
Huibin D, Guozhu M, Xi L, Jian Z, Linyuan W (2015) Way forward for alternative energy research: a bibliometric analysis during 1994-2013. Sustain Energy Rev 48:276–286
Jalaei F, Jrade A (2015) Integrating building information modeling (BIM) and LEED system at the conceptual design stage of sustainable buildings. Sustain Cities Soc 18(95-10718):95–107. https://doi.org/10.1016/j.scs.2015.06.007
Kandya A, Mohan M (2018) Mitigating the urban heat island effect through building envelope modifications. Energy Build 164:266–277. https://doi.org/10.1016/j.enbuild.2018.01.014
Khan J, Arsalan MH (2016) Solar power technologies for sustainable electricity generation - a review. Renew Sust Energ Rev 55:414–425. https://doi.org/10.1016/j.rser.2015.10.135
Khoshbakht et al (2018) Are green buildings more satisfactory? A review of global evidence. Habitat Int 74:57–65
Lazarova-Molnar S, Mohamed N (2019) Collaborative data analytics for smart buildings: opportunities and models. Clust Comput 22:1065–1077. https://doi.org/10.1007/s10586-017-1362-x
Li X, Wu P, Shen GQ, Wang X, Teng Y (2017) Mapping the knowledge domains of building information modeling (BIM): a bibliometric approach. Autom Constr 84:195–206. https://doi.org/10.1016/j.autcon.2017.09.011
Liu CY, Wang JC (2010) Forecasting the development of the biped robot walking technique in Japan through S-curve model analysis. Scientometrics 82:21–36
Liu GL et al (2017) A review of air filtration technologies for sustainable and healthy building ventilation. Sustain Cities Soc 32:375–396. https://doi.org/10.1016/j.scs.2017.04.011
Liu J, Liu GQ (2005) Some indoor air quality problems and measures to control them in China Indoor. Built Environ 14:75–81. https://doi.org/10.1177/1420326x05050362
Liu ZB, Li WJ, Chen YZ, Luo YQ, Zhang L (2019) Review of energy conservation technologies for fresh air supply in zero energy buildings. Appl Therm Eng 148:544–556. https://doi.org/10.1016/j.applthermaleng.2018.11.085
Liu ZJ, Pyplacz P, Ermakova M, Konev P (2020) Sustainable construction as a competitive advantage. Sustainability 12:13. https://doi.org/10.3390/su12155946
Maasoumy M, Sangiovanni-Vincentelli A (2016) Smart connected buildings design automation: foundations and trends found trends. Electron Des Autom 10:1–3. https://doi.org/10.1561/1000000043
Mao G, Zou H, Chen G, Du H, Zuo J (2015) Past, current and future of biomass energy research: a bibliometric analysis. Sustain Energy Rev 52:1823–1833. https://doi.org/10.1016/j.rser.2015.07.141
Mao GZ, Shi TT, Zhang S, Crittenden J, Guo SY, Du HB (2018) Bibliometric analysis of insights into soil remediation. J Soils Sediments 18:2520–2534. https://doi.org/10.1007/s11368-018-1932-4
Marinakis V (2020) Big data for energy management and energy-efficient buildings. Energies 13:18. https://doi.org/10.3390/en13071555
McArthur JJ, Powell C (2020) Health and wellness in commercial buildings: systematic review of sustainable building rating systems and alignment with contemporary research. Build Environ 171:18. https://doi.org/10.1016/j.buildenv.2019.106635
Mcleod RS, Hopfe CJ, Kwan A (2013) An investigation into future performance and overheating risks in Passivhaus dwellings. Build Environ 70:189–209
Mclinden D (2013) Concept maps as network data: analysis of a concept map using the methods of social network analysis. Eval Program Plann 36:40–48
Mehmood MU, Chun D, Zeeshan, Han H, Jeon G, Chen K (2019) A review of the applications of artificial intelligence and big data to buildings for energy-efficiency and a comfortable indoor living environment. Energy Build 202:13. https://doi.org/10.1016/j.enbuild.2019.109383
Miao XL, Yao Y, Wang Y, Chu YP (2011) Experimental research on the sound insulation property of lightweight composite paper honeycomb core wallboard. In: Jiang ZY, Li SQ, Zeng JM, Liao XP, Yang DG (eds) Manufacturing Process Technology, Pts 1-5, vol 189-193. Advanced Materials Research. Trans Tech Publications Ltd, Stafa-Zurich, pp 1334–1339. https://doi.org/10.4028/www.scientific.net/AMR.189-193.1334
Chapter Google Scholar
Newsham GR, Mancini S, Energy BJ (2009) Do LEED-certified buildings save energy? Yes, but…. Energy Build 41:897–905
Nguyen HT, Skitmore M, Gray M, Zhang X, Olanipekun AO (2017) Will green building development take off? An exploratory study of barriers to green building in Vietnam. Resour Conserv Recycl 127:8–20
Paul WL, Taylor PA (2008) A comparison of occupant comfort and satisfaction between a green building and a conventional building. Build Environ 43:1858–1870
Perez-Fargallo A, Rubio-Bellido C, Pulido-Arcas JA, Gallego-Maya I, Guevara-Garcia FJ (2018) Influence of adaptive comfort models on energy improvement for housing in cold areas. Sustainability 10:15. https://doi.org/10.3390/su10030859
Pongiglione M, Calderini C (2016) Sustainable structural design: comprehensive literature review. J Struct Eng 142:15. https://doi.org/10.1061/(asce)st.1943-541x.0001621
Pouris A, Pouris A (2011) Scientometrics of a pandemic: HIV/AIDS research in South Africa and the World. Scientometrics 86:541–552
Pulselli RM, Simoncini E, Pulselli FM, Bastianoni S (2007) Emergy analysis of building manufacturing, maintenance and use: Em-building indices to evaluate housing sustainability. Energy Build 39:620–628
Ruellan M, Park H, Bennacer R (2016) Residential building energy demand and thermal comfort: thermal dynamics of electrical appliances and their impact. Energy Build 130:46–54. https://doi.org/10.1016/j.enbuild.2016.07.029
Sartori I, Napolitano A, Voss K (2012) Net zero energy buildings: a consistent definition framework. Energy Build 48:220–232. https://doi.org/10.1016/j.enbuild.2012.01.032
Schossig P, Henning HM, Gschwander S, Haussmann T (2005) Micro-encapsulated phase-change materials integrated into construction materials. Solar Energy Mater Solar Cells 89:297-306
Shen C, Li XT (2016) Solar heat gain reduction of double glazing window with cooling pipes embedded in venetian blinds by utilizing natural cooling. Energy Build 112:173–183. https://doi.org/10.1016/j.enbuild.2015.11.073
Shen L, Yan H, Fan H, Wu Y, Zhang Y (2017) An integrated system of text mining technique and case-based reasoning (TM-CBR) for supporting green building design. S0360132317303797. Build Environ 124:388–401
Todd JA, Crawley D, Geissler S, Lindsey G (2001) Comparative assessment of environmental performance tools and the role of the Green Building Challenge. Build Res Inf 29:324–335
Wang H, Chiang PC, Cai Y, Li C, Wang X, Chen TL, Wei S, Huang Q (2018) Application of wall and insulation materials on green building: a review. Sustainability 10:21. https://doi.org/10.3390/su10093331
Wang J, Liu Y, Ren J, Cho S, (2017) Iop (2017) A brief comparison of existing regional green building design standards in China. In: 2nd International Conference on Advances in Energy Resources and Environment Engineering, vol 59. IOP Conference Series-Earth and Environmental Science. Iop Publishing Ltd, Bristol. doi: https://doi.org/10.1088/1755-1315/59/1/012013
Wei WJ, Ramalho O, Mandin C (2015) Indoor air quality requirements in green building certifications. Build Environ 92:10–19. https://doi.org/10.1016/j.buildenv.2015.03.035
Wuni IY, Shen GQP, Osei-Kyei R (2019a) Scientometric review of global research trends on green buildings in construction journals from 1992 to 2018. Energy Build 190:69–85
Wuni IY, Shen GQP, Osei RO (2019b) Scientometric review of global research trends on green buildings in construction journals from 1992 to 2018 Energy Build 190:69-85 doi: https://doi.org/10.1016/j.enbuild.2019.02.010
Yang XD, Zhang JY, Zhao XB (2018) Factors affecting green residential building development: social network analysis. Sustainability 10:21. https://doi.org/10.3390/su10051389
Ye L, Cheng Z, Wang Q, Lin H, Lin C, Liu B (2015) Developments of Green Building Standards in China. Renew Energy 73:115–122
Ye Q, Li T, Law R (2013) A coauthorship network analysis of tourism and hospitality research collaboration. J Hosp Tour Res 37:51–76
Yoon B, Park Y (2004) A text-mining-based patent network: analytical tool for high-technology trend. J High Technol Manag Res 15:37–50
Yuan XL, Wang XJ, Zuo J (2013) Renewable energy in buildings in China-a review. Renew Sust Energ Rev 24:1–8. https://doi.org/10.1016/j.rser.2013.03.022
Zhang HT, Hu D, Wang RS, Zhang Y (2014) Urban energy saving and carbon reduction potential of new-types of building materials by recycling coal mining wastes. Environ Eng Manag J 13:135–144
Zhang HZ, Li H, Huang BR, Destech Publicat I (2015) Development of biogas industry in Beijing. 2015 4th International Conference on Energy and Environmental Protection. Destech Publications, Inc, Lancaster
Zhang SF, Andrews-Speed P, Zhao XL, He YX (2013) Interactions between renewable energy policy and renewable energy industrial policy: a critical analysis of China’s policy approach to renewable energies. Energy Policy 62:342–353. https://doi.org/10.1016/j.enpol.2013.07.063
Zhang Y, Huang K, Yu YJ, Yang BB (2017) Mapping of water footprint research: a bibliometric analysis during 2006-2015. J Clean Prod 149:70–79. https://doi.org/10.1016/j.jclepro.2017.02.067
Zhang Y, Kang J, Jin H (2018) A review of Green Building Development in China from the perspective of energy saving. Energies 11:18. https://doi.org/10.3390/en11020334
Zhao XB, Zuo J, Wu GD, Huang C (2019) A bibliometric review of green building research 2000-2016. Archit Sci Rev 62:74–88. https://doi.org/10.1080/00038628.2018.1485548
Zuo J, Zhao ZY (2014) Green building research-current status and future agenda: a review. Renew Sust Energ Rev 30:271–281. https://doi.org/10.1016/j.rser.2013.10.021
Download references
Availability of data and materials
The datasets generated and analyzed throughout the current study are available in the Web of Science Core Collection.
This study was supported by The National Natural Science Foundation of China (No.51808385).
Author information
Authors and affiliations.
School of Environmental Science and Engineering, Tianjin University, No. 135 Yaguan Road, Tianjin, 300350, China
Li Ying, Rong Yanyu, Umme Marium Ahmad, Wang Xiaotong & Mao Guozhu
Tianjin University Research Institute of Architectural Design and Urban Planning Co., Ltd, Tianjin, 300072, China
Center for Green Buildings and Sponge Cities, Georgia Tech Tianjin University Shenzhen Institute, Shenzhen, 518071, Guangdong, China
Rong Yanyu, Wang Xiaotong & Mao Guozhu
School of Architecture & Built Environment, The University of Adelaide, Adelaide, Australia
You can also search for this author in PubMed Google Scholar
Contributions
Ying Li conceived the frame of the paper and wrote the manuscript. Yanyu Rong made the data figures and participated in writing the manuscript. Umme Marium Ahmad helped with revising the language. Xiaotong Wang consulted related literature for the manuscript. Jian Zuo contributed significantly to provide the keywords list. Guozhu Mao helped with constructive suggestions.
Corresponding author
Correspondence to Rong Yanyu .
Ethics declarations
Ethics approval and consent to participate.
This manuscript is ethical.
Consent for publication
Not applicable.
Competing interest
The authors declare no competing interest.
Additional information
Responsible Editor: Philippe Garrigues
Publisher’s Note
Springer Nature remains neutral with regard to jurisdictional claims in published maps and institutional affiliations.
Supplementary Information
(DOCX 176 kb)
(From Web of Science Core Collection)
Topic: (“bioclimatic architect*” or “bioclimatic build*” or “bioclimatic construct*” or “bioclimatic hous*” or “eco-architect*” or “eco-build*” or “eco-home*” or “eco-hous*” or “eco-friendly build*” or “ecological architect*” or “ecological build*” or “ecological hous*” or “energy efficient architect*” or “energy efficient build*” or “energy efficient construct*” or “energy efficient home*” or “energy efficient hous*” or “energy efficient struct*” or “energy saving architect*” or “energy saving build*” or “energy saving construct*” or “energy saving home*” or “energy saving hous*” or “energy saving struct*” or “green architect*” or “green build*” or “green construct*” or “green home*” or “low carbon architect*” or “low carbon build*” or “low carbon construct*” or “low carbon home*” or “low carbon hous*” or “low energy architect*” or “low energy build*” or “low energy construct*” or “low energy home*” or “low energy hous*” or “sustainable architect*” or “sustainable build*” or “sustainable construct*” or “sustainable home*” or “sustainable hous*” or “zero energy build*” or “zero energy home*” or “zero energy hous*” or “net zero energy build*” or “net zero energy home*” or “net zero energy hous*” or “zero-carbon build*” or “zero-carbon home*” or “zero-carbon hous*” or “carbon neutral build*” or “carbon neutral construct*” or “carbon neutral hous*” or “high performance architect*” or “high performance build*” or “high performance construct*” or “high performance home*” or “high performance hous*”)
Time span: 1998-2018。 Index: SCI-EXPANDED, SSCI。
Rights and permissions
Reprints and permissions
About this article
Li, Y., Rong, Y., Ahmad, U.M. et al. A comprehensive review on green buildings research: bibliometric analysis during 1998–2018. Environ Sci Pollut Res 28 , 46196–46214 (2021). https://doi.org/10.1007/s11356-021-12739-7
Download citation
Received : 18 October 2020
Accepted : 26 January 2021
Published : 16 February 2021
Issue Date : September 2021
DOI : https://doi.org/10.1007/s11356-021-12739-7
Share this article
Anyone you share the following link with will be able to read this content:
Sorry, a shareable link is not currently available for this article.
Provided by the Springer Nature SharedIt content-sharing initiative
- Green buildings
- Bibliometric analysis
- Energy efficiency
- Find a journal
- Publish with us
- Track your research
The Precourt Institute for Energy is now part of the Stanford Doerr School of Sustainability .

Research Areas
Main navigation, renewable energy.

- Fusion Energy
- Renewable Fuels
Energy Conversion & Storage

- Large-Scale Storage
Global Carbon Management

- CO2 Capture & Sequestration
- CO2 Conversion & Use
- Direct Air Capture
- Methane Leakage
- Natural Climate Solutions
Electric Grid Modernization

- Digitization, Control, & Optimization
- Grid Reliability
- Power Electronics
- Superconductors
Policy, Economics & Society

- Energy Equity & Just Transitions
- Finance, Markets, & Business Models
- Legislation, Regulation, & Taxation
- National Security
- Public Opinion
Environmental Impacts

- Air Quality
- Human Health
Energy Efficiency

- Energy-Efficient Computing & Electronics
- Human Behavior & Energy Use
- Water Systems
Industrial Decarbonization

- Additive/3D Printing
- Circular Economy
Sustainable Transportation

- Electric Vehicles
- Heavy-duty transport
Conventional Energy

- Natural Gas
Energy Systems

- Energy Systems Analysis
- Energy Transition Pathways
- Zero-Emission Energy Solutions
Read our research on: Gun Policy | International Conflict | Election 2024
Regions & Countries
How republicans view climate change and energy issues.
Just 12% of Republicans and Republican leaners say dealing with climate change should be a top priority for the president and Congress.
Growing share of Americans favor more nuclear power
A majority of Americans (57%) say they favor more nuclear power plants to generate electricity in the country, up from 43% who said this in 2020.
Why Some Americans Do Not See Urgency on Climate Change
As the Earth’s temperature continues to rise, climate change remains a lower priority for some Americans, and a subset of the public rejects that it’s happening at all. To better understand the perspectives of those who see less urgency to address climate change, the Center conducted a series of in-depth interviews designed to provide deeper insight into the motivations and views of those most skeptical about climate change.
What the data says about Americans’ views of climate change
Two-thirds of Americans say the United States should prioritize developing renewable energy sources over expanding the production of fossil fuels.
How Americans view electric vehicles
About four-in-ten Americans (38%) say they’re very or somewhat likely to seriously consider an electric vehicle (EV) for their next vehicle purchase.
Majorities of Americans Prioritize Renewable Energy, Back Steps to Address Climate Change
Large shares of Americans support the U.S. taking steps to address global climate change and prioritize renewable energy development in the country. Still, fewer than half are ready to phase out fossil fuels completely and 59% oppose ending the production of gas-powered cars.
Home solar panel adoption continues to rise in the U.S.
While residential solar power generates just a fraction of the country’s overall electricity, it has continued to grow rapidly.
Americans support incentives for electric vehicles but are divided over buying one themselves
Overall, two-thirds of Americans support providing incentives to increase the use of electric and hybrid vehicles.
A Majority of Americans Favor Expanding Natural Gas Production To Export to Europe
Yet renewable sources, like wind and solar, remain Americans’ overall priority for domestic production.
Americans Largely Favor U.S. Taking Steps To Become Carbon Neutral by 2050
Majorities of Americans say the United States should prioritize the development of renewable energy sources and take steps toward the country becoming carbon neutral by the year 2050. But just 31% want to phase out fossil fuels completely, and many foresee unexpected problems in a major transition to renewable energy.
Refine Your Results
About Pew Research Center Pew Research Center is a nonpartisan fact tank that informs the public about the issues, attitudes and trends shaping the world. It conducts public opinion polling, demographic research, media content analysis and other empirical social science research. Pew Research Center does not take policy positions. It is a subsidiary of The Pew Charitable Trusts .
- How it works
Useful Links
How much will your dissertation cost?
Have an expert academic write your dissertation paper!
Dissertation Services
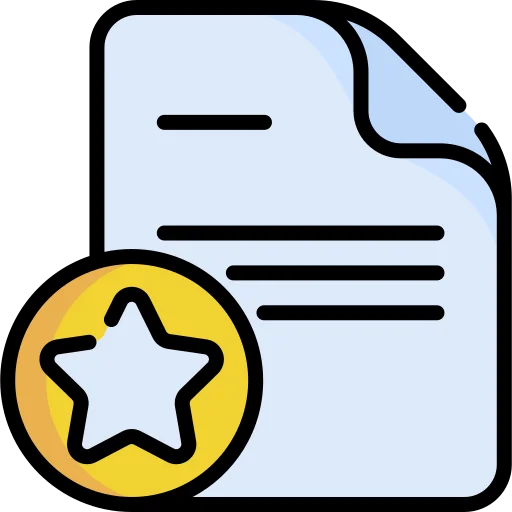
Get unlimited topic ideas and a dissertation plan for just £45.00
Order topics and plan
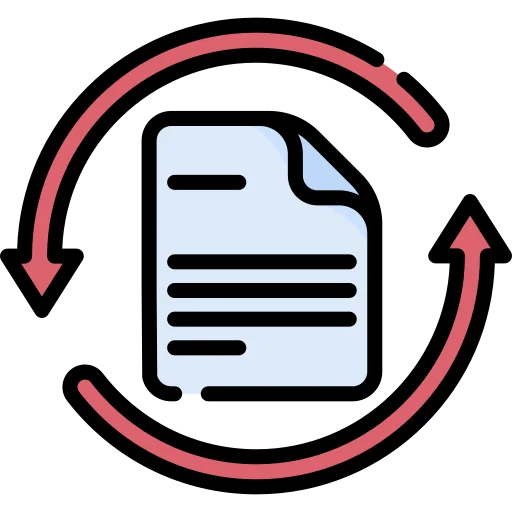
Get 1 free topic in your area of study with aim and justification
Yes I want the free topic
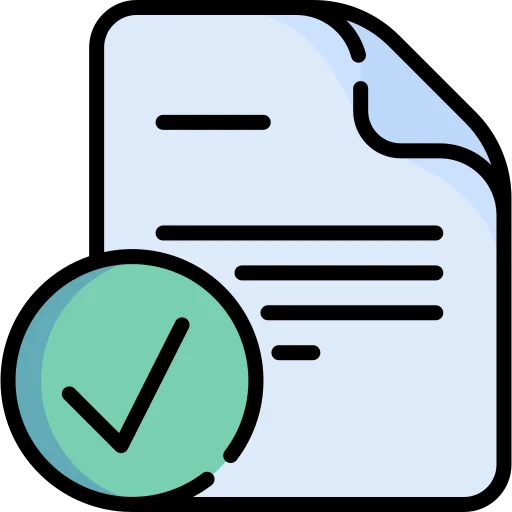
Renewable Energy Dissertation Topics
Published by Carmen Troy at January 5th, 2023 , Revised On August 11, 2023
Renewable energy refers to sustainable energy that can be constantly replenished. These energy sources include solar energy, wind energy, and thermal energy, which are naturally replenishing. In simple words, renewable energy is the energy extracted from natural sources.
Renewable energy has become the need of the hour with potential repercussions on climate. While many used to claim in past that the emergency of climate change is false, the obvious changes today evidently ratify its importance. If not for climate change, renewable energy is essential for increasing the longevity of the earth and thus the species living on it. Therefore, it is a matter of high significance to make some painstaking efforts and ensure the availability of renewable energy resources among all.
Suppose you are aiming to centralise your dissertation on a renewable energy-related theme. In that case, you can look at some of the current, striking, and potential topics suggested by our PHD scholars at ResearchProspect.
You may also want to start your dissertation by requesting a brief research proposal from our writers on any of these topics, which includes an introduction to the problem, research question , aim and objectives, literature review , along with the proposed methodology of research to be conducted. Let us know if you need any help in getting started.
Check our example dissertation to get an idea of how to structure your dissertation .
You can review step by step guide on how to write your dissertation here .
2022 Renewable Energy Dissertation Topics
Topic 1: exploring the economic benefits of increasing biomass conversion – a case study of the uk renewable energy industry..
Research Aim: The present study aims to explore the economic benefits of increasing biomass conversion referring to the case study of the UK renewable energy industry.
Objectives:
- To share a preliminary concept of biomass conversion and its benefits.
- To describe the economic benefits of increasing biomass conversion based on the context of the UK renewable energy industry
- To identify challenges in biomass conversion along with figuring out strategies to eradicate these challenges.
Topic 2: Inspecting the advantages of using solar energy and its role as a solution to the global threat i.e. Climate change.
Research Aim: The present study aims to investigate the benefits of using solar energy and the way it is resolving the problem of climate change.
- To elucidate the benefits of using solar energy and its growing use in different sectors.
- To explain how solar energy can be a solution for a global threat like climate change.
- To provide a stringent set of recommendations for the best possible use of solar energy to eradicate the problem of climate change.
Topic 3: Examining the strategy of embracing renewable energy by the UK retail organisations to fulfil the environmental sustainability goals.
Research Aim: The present study aims to evaluate the strategy of using renewable energy in the UK retail sector to fulfil environmental sustainability goals.
- To express the way renewable energy sources are being relevant in the UK retail industry.
- To analyse how the retail orgnisations in the UK are using renewable energy to fulfil their environmental sustainability goals.
- To share effective ideas about how renewable energy sources can be used properly by the UK retail organisations to fulfil environmental sustainability goals.
Topic 4: Critical assessment of growing concern for sustainability in UK construction industry which is driving renewable energy consumption.
Research Aim: The present study aims to assess the growing concern for sustainability in the UK construction industry that drives overall renewable energy consumption.
- To explain the increasing concern for sustainability in the UK construction industry.
- To examine how renewable energy consumption is increasing in the UK construction industry along with the growing concern for sustainability.
- To recommend the organisations in the UK construction industry to improve the use of renewable energy sources aiming to achieve sustainability goals.
Topic 5: Evaluating the impact of solar energy in sustainability practices in the UK agriculture industry.
Research Aim: The present study aims to evaluate the impacts of using solar energy in sustainability practices in the UK agriculture industry.
- To demonstrate the concept of solar energy consumption and its impacts on sustainability practices.
- To contextualise the use of solar energy in the UK agriculture industry as a part of sustainability practices.
- To provide recommendations for improving the use of solar energy thereby gaining its advantageous effects in the UK agriculture industry.
Renewable Energy Research Topics
Topic. 1: renewable energy: prospects and problems today.
Research Aim: The main aim of the research will be to identify the significance of deploying renewable energy to the masses and its implications in the long run. The research will also discuss whether or not the world is facing challenges in ensuring the availability of renewable energy; if yes, what would be the solutions or alternatives.
Topic. 2: Renewable energy for sustainable development in Africa
Research Aim: Africa leads ahead of all other regions of the world regarding the least access to renewable energy. According to one report, around 600 million people do not have access to electricity in Africa, while 900 million lack access to clean water. This research will study and evaluate how providing renewable energy can foster sustainable development in the region by advancing economic development, improving access to energy, and mitigating climate change.
Topic. 3: Implications of COVID-19 on the biofuel market
Research Aim: Covid-19 posed precarious implications for the global markets as it dismantled the buying capacity of people. It was noted that during the pandemic, the prices of biofuel plummeted dramatically as the consumer need was minimal. Keeping that in mind, you can base your research on what shifts are expected to occur in the bio-fuel market when the pandemic ends.
The prime aim of the research will include studying the impact of COVID-9 on the biofuel market and understanding its influences on biofuel policy support by policymakers.
Topic. 4: Geothermal energy; an untapped abundant energy resource
Research Aim: Geothermal energy is usually viewed as a recent and form of alternative energy. It is cheaper than other green energy sources and is clean and sustainable. It is derived from the earth core and is more eco-friendly than the other fossil fuel sources. In this research, you can explain geothermal energy, its abundance, and how it can be leveraged and supplied to the masses to help escape the energy crisis.
Topic. 5: The Future of Wind Energy
Research Aim: The main aim of the research will be to identify the prospects of wind energy by evaluating the current and prospected policies regarding its utilisation worldwide. The research can also base on modern and future technologies to expand the utilisation and outreach of wind energy.
Topic. 6: Home wind energy: How valuable it is?
Research Aim: Recently more and more people are finding it an excellent idea to install our very own wind turbines and produce clean energy to power homes. But doing that does not come without challenges. The research can discuss the significance of wind energy, check for its practicability, and evaluate its benefits and downsides.
Topic. 7: Economic and environmental benefits of Renewable Energy
Research Aim: All of us are aware that renewable energy has vast benefits, ranging from economic to environmental benefits. The main aim of the research will be to thoroughly discuss the economic and environmental aspects, which are facilitated the most. You can study how countries are thriving economically and structuring workable policies to mitigate climate change and present a model to follow.
How Can ResearchProspect Help?
ResearchProspect writers can send several custom topic ideas to your email address. Once you have chosen a topic that suits your needs and interests, you can order for our dissertation outline service which will include a brief introduction to the topic, research questions , literature review , methodology , expected results and conclusion . The dissertation outline will enable you to review the quality of our work before placing the order for our full dissertation writing service !
Topic. 8: Why it has become more important than ever to focus on renewable energy
Research Aim: The aim of the research will be to identify the key reasons behind the much-needed attention that must be given to renewable energy. It is prime time to focus on renewable energy to ensure sustainable development and handle climate change quickly.
Today, as the world is swiftly transitioning into a technologically driven lifestyle, there are still a lot of people with no access to drinking water and electricity. Moreover, the consumption of artificial resources is responsible for curtailing the longevity of the earth and thus the species living on it. It is essential to take significant steps to help the earth and the people living on it.
Also Read: Environmental Engineering Dissertation Topics
Topic. 9: Is financing Renewable energy costly?
Research Aim: The pivotal aim of the research will be to examine the costs that it would take to finance renewable energy for the masses. Many countries around the world still have no access to clean drinking water, electricity, and therefore technology. These are the main reasons why the countries are underdeveloped, and their inhabitants are below the poverty line.
Topic. 10: Mitigating climate change; can renewable energy help?
Research Aim: The research will evaluate the impact of renewable energy in helping mitigate climate change. It will analyse all key factors that can impeccably play a role in controlling the biggest problem posed to humans.
As the years pass by, the population of humans is also growing. More people means more land acquisition, more pollution, and more requirement for resources. In such a scenario, what is suffering the most is the climate. If it is not addressed today, it will become such a big problem that it will be impossible to handle it easily.
Topic. 11: Living Green: How many have access to Renewable energy
Research Aim: With time, the energy costs are increasing, so are the effects of global warming. It has become more important than ever to ensure living green: Using renewable energy. The main aim of the research would be to do a quantitative analysis of how many people have access to renewable energy.
Topic. 12: Understanding differences between renewable and alternative energy technology
Research Aim: Many people confuse renewable and alternative energy technology and therefore question if there is such thing as renewable energy technology. The research can explain and evaluate the differences between renewable and alternative technology so that people can use them without any doubt in their minds. Renewable energy can be constantly replenished, while alternative energy is an alternative energy source used instead of fossil fuel.
Also Read: Technology Dissertation Topics
Topic. 13: Is solar energy the way forward
Research Aim: There is a persistent controversy on the advantages and disadvantages of solar energy. While some believe that it is of great benefit, it is the other way around for others.
The aim of the research will be to examine solar energy and weigh its pros and cons, and evaluate if it is going to predominate in the future. A qualitative analysis that includes surveying people’s opinions on social energy help clear this ambiguity.
Topic. 14: Approach towards renewable energy in 2030
Research Aim: The research will study the current national and international policies on renewable energy to sketch a draft on the approach towards renewable energy in 2030. Qualitative discourse analysis can help figure out the key indicators that will prompt or prohibit a change in the upcoming years.
Topic. 15: Cost of solar energy in comparison to other renewable energy
Research Aim: The research will conduct a financial analysis on solar energy and draw a comparison against other renewable energy, i.e. hydro, biomass, tidal, and wind energy. It will evaluate the costs against different parameters and on different levels of technology.
Hire an Expert Writer
Orders completed by our expert writers are
- Formally drafted in an academic style
- Free Amendments and 100% Plagiarism Free – or your money back!
- 100% Confidential and Timely Delivery!
- Free anti-plagiarism report
- Appreciated by thousands of clients. Check client reviews
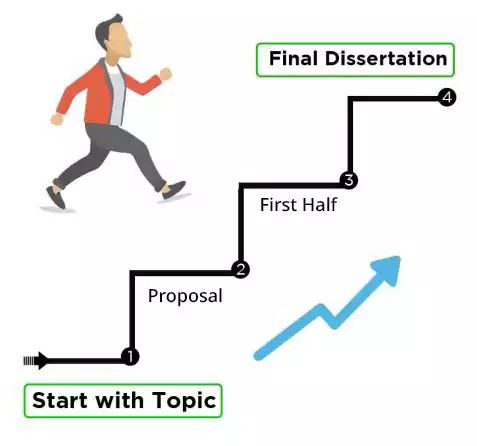
Topic. 16: Trends in Renewable energy
Research Aim: It is necessary to keep an eye on the current trends to make speculations about the future. The researcher can study the trends in renewable energy in 202o or 2021. The research can also draw a comparison in the renewable trends in 2020 and 2021.
Topic. 17: Renewable energy and COVID-19
Research Aim: The research will study and explore the impacts of COVID-19 on renewable energy. It will also explain if the pandemic posed any systematic changes to trends and prospects of renewable energy.
Topic. 18: How does Geothermal energy work?
Research Aim: The research will explain a thorough explanation of how geothermal energy works and why it is more eco-friendly, economical, and valuable than fossil fuel. The researcher can describe describe the steps from scratch until it is utilised as alternative energy.
Topic. 19: Effects of renewable vs non-renewable energy
Research Aim: The researcher will empirically study the small and broad long-run effects of using renewable and non-renewable energy to create a comparison between them.
Topic. 20: A review of tidal energy technologies
Research Aim: Tidal energy is among the most efficient energies; however, it is less common as it is harnessed from tides. The aim of the research will be to study the technological advancement and development regarding the usage as an alternative for energy. The research can list different methods, devices, and technologies that are used to harness tidal energy, and which of them can be the most viable to meet our annual needs.
Free Dissertation Topic
Phone Number
Academic Level Select Academic Level Undergraduate Graduate PHD
Academic Subject
Area of Research
Frequently Asked Questions
How to find renewable energy dissertation topics.
To find renewable energy dissertation topics:
- Research recent advancements.
- Explore environmental concerns.
- Investigate technology potentials.
- Analyze policy and economic aspects.
- Consider global energy needs.
- Select a specific area of interest for your dissertation.
You May Also Like
Most students find it difficult to select the perfect International Business Dissertation Topic for their degree dissertations. In fact, most students choose narrow topics for their international business research papers as a result of the depth and breadth of the field.
Need interesting and manageable Education dissertation topics or thesis? Here are the trending Education dissertation titles so you can choose the most suitable one.
USEFUL LINKS
LEARNING RESOURCES
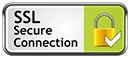
COMPANY DETAILS
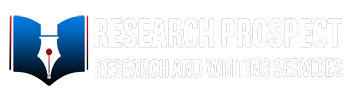
- How It Works

- Green Office Model online course
- Case studies
- Membership Programme for Green Offices
- Ambassador Track
- Green Office Alumni Network
- GOM Sustainability Network
- Vision of the Movement
80 sustainability research topics for students to explore green campus issues
You’re planning your thesis, paper or capstone? You want to do a student research project with impact. We have outlined a range of sustainability research topics for you. The list specifically focuses on how to green your campus . Take action to make your university more sustainable!
Our list of sustainability research topics helps students investigate green campus issues.
Sustainability research topics: Education
Some sustainability research topics on education for sustainable development :
- What are the strengths and weaknesses of different definitions of sustainability education? Which definition could your university adopt?
- To what extent is sustainability education already implemented in the curriculum of your university?
- What are the strengths and limitations of advancing sustainability education within your curriculum?
- Where does your university stand with regards to sustainability education compared to other institutions of higher education?
- What is the demand among students for more, different or better sustainability education?
- How can existing sustainability projects on campus be used for educational purposes, e.g. visit solar cells on rooftops as part of engineering classes?
Sign-up to the FREE online course to establish your Green Office
5 weeks. One email per week. Unsubscribe at any time. By signing up, you agree to our data policy .
- What definition of sustainability research should your university embrace?
- To what extent is sustainability research already practised at your university?
- What are the strengths and weaknesses of the institution’s sustainability research portfolio compared to other institutions of higher education?
- What are the drivers of and barriers to sustainability research at your university?
- How could sustainability research help students to study sustainability issues on campus and inform practical change projects?
- What are the opportunities and costs associated with promoting sustainability research? What could a plan of action look like to strategically advance it?
Some sustainability research topics on community engagement and awareness:
- What are the perceptions of and attitudes towards sustainability by students and staff?
- What are ways to promote sustainable lifestyles among students?
- To what extent are students and staff aware of the UN Sustainable Development Goals (SDGs) ?
- How aware are students and staff about the institution’s sustainability ambitions?
- What are the benefits and disadvantages of approaches to communicate the university’s sustainability efforts better?
- What are the challenges to involve students and staff in the university’s sustainability efforts?
- Which ways to increase the engagement of the campus community exist, for example by organising sustainability events ?
For inspiration, read our post on 10 projects to engage students on the SDGs .
Explore sustainability topics for research papers on different issues related to greening campus operations:
- What are the opportunities and costs of improving the building insulations to save energy?
- What lighting systems exist on the market that are more energy efficient?
- What would a business case look like to install a new lighting system?
- Where are the main consumers of energy on campus?
- What innovative energy technologies are developed at the institution itself? To what extent could those be directly installed and tested in buildings?
- What lux values are sufficient for work and study places so that places are appropriately lit without wasting too much electricity?
- What are the strengths and weaknesses of different sustainable building standards?
- Which building standards would be most appropriate to inform the institution’s sustainable building policy?
- What are the costs and benefits associated with different types of green roofs?
- On which buildings could green roofs be installed?
- To what extent are catering and food products certified as organic or fair trade food?
- How much and why do students attach importance to organic and fair trade products sold in the cafeteria?
- How can students and employees be made more aware of the multiple benefits – e.g. health, environment, economics – of sustainable (organic, fair trade, local) food ?
- How much are students willing to pay for more organic or fair trade products?
- What types and amounts of waste are produced by whom and where at the institution?
- How did waste streams develop over the last years?
- What are innovative practices in reducing waste going to landfill or incineration? How could those be applied?
- What are the costs and benefits associated with waste recycling ?
- What options exist to switch from paper-based to more digital forms of working and studying to reduce paper consumption?
- What are the environmental, economic, and social benefits and disadvantages of different options to advance more digital working and studying?
More sustainability research topics on campus operations:
Biodiversity
- What species live at different campus locations?
- To what extent do students, faculty and staff value this biodiversity?
- What are ways to enhance biodiversity on campus?
Greenhouse-gase (GHG)
- What are the pros and cons of different GHG accounting standards?
- Which standard should the institution use to develop a GHG emissions inventory ?
- Where are GHG emissions released at the institution?
- How big is the institution’s GHG footprint?
Procurement
- What does sustainable procurement mean in the context of a university?
- How is procurement currently organised? To what extent are sustainability criteria already applied in tenders?
- To what extent could the university implement sustainability criteria that go beyond the legal minimum to advance the environmental, economic and social benefits of tenders?
- What are the largest consumers of water?
- What is the direct and indirect water-footprint of the institution?
- What are opportunities and costs to reduce water usage?
Transportation and mobility
- How do students and staff currently travel to the university and as part of their study or work?
- What is the environmental impact of these travel behaviours? How could the impact be reduced?
- What best practices exist among companies and other institutions of higher education to reduce staff travel or incentivize different travel behaviours?
Behaviour change
- What is the potential to reduce resource consumption through behaviour change?
- What are the best practices of behaviour change interventions at institutions of higher education?
- To what extent could these projects be also applied at your university?
Sustainability research topics on governance, strategy and reporting
Sustainability research topics on governance issues:
- What does sustainability mean for institutions of higher education?
- How does a comprehensive concept of a sustainable institution of higher education look like?
- How could the university’s long-term sustainability vision look like? How could this vision be realized through a roadmap?
- What are innovative ways to develop sustainability strategies for a university through a bottom-up approach?
- What ethical imperatives would demand that institutions of higher education care for their impact on the planet, people and profit?
- What are the responsibilities of institutions of higher education to contribute to global challenges, such as poverty, gender inequality, and climate change?
Monitoring and reporting
- What data is important to monitor the institution’s environmental impact? How can this data be collected and analysed?
- What are the advantages and disadvantages of different sustainability reporting standards?
- Which sustainability reporting standards should the university adhere to?
- What are efficient ways to organize sustainability reporting within the organization?
- What is the best way to communicate results among students, staff and outside actors?
- What are the strengths and weaknesses of different methodologies (e.g. payback or Net Present Value) to calculate the financial costs and benefits of sustainability investments?
- Which methodology should the institution apply?
- To what extent could sustainability projects be financed through a revolving loan fund?
- What are the possibilities to involve outside organizations through energy contracting?
- What subsidies are available at the European, national and city level to develop a green campus?
- How could the university use these financing options to advance its energy transition?
- What are approaches to integrate negative externalities into the accounting schemes of the university?
- What would be the opportunities, benefits and risks associated with establishing an energy company that’s owned by the university?
- What are the best practices to finance energy efficiency and renewable energy projects at public institutions around the world?
- How can incentive schemes be changed so that energy end-users directly benefit from reductions in energy usage?
We hope this list inspired you to find a sustainability topic for research papers.
- 12 sustainability documentaries to educate yourself and get inspired
- Green guide: 15 tips for a sustainable student lifestyle
- 12 sustainability teaching resources about green campuses
- Open access
- Published: 20 December 2023
Emerging new themes in green finance: a systematic literature review
- H. M. N. K. Mudalige ORCID: orcid.org/0000-0002-4497-4750 1
Future Business Journal volume 9 , Article number: 108 ( 2023 ) Cite this article
2745 Accesses
Metrics details
There is a need for an extensive understanding of the emerging themes and trends within the domain of green finance, which is still evolving. By conducting a systematic literature review on green finance, the purpose of this study is to identify the emerging themes that have garnered significant attention over the past 12 years. In order to identify the emerging themes in green finance, bibliometric analysis was performed on 978 publications that were published between 2011 and 2023 and were taken from the databases of Scopus and Web of Science. The author examined annual scientific production, journal distribution, countries scientific production, most relevant authors, most frequent words, areas where empirical research is lacking, words' frequency over time, trend topics, and themes of green finance. The outcome of the review identified the following seven themes: (i) green finance and environmental sustainability; (ii) green finance and investments; (iii) green finance and innovation; (iv) green finance policy/green credit guidelines; (v) green finance and economy; (vi) green finance and corporate social responsibility; (vii)trends/challenges/barriers/awareness of green finance. The analysis of these emerging themes will contribute to the existing corpus of knowledge and provide valuable insights into the landscape of green finance as it evolves.
Introduction
Cities will face their greatest challenges ever during the next 30 years, and three-quarters of the world's population will reside in urban areas by 2050 due to the unparalleled rate of urbanization as a result of population growth, resource scarcity, such as peak oil, water shortages, and food security [ 100 ].
One of the main challenges in building and maintaining sustainable cities is discovering the sources required to fund vital infrastructure, development, and maintenance activities that have a sustainable future. To achieve the creation of sustainable cities, there is a need for green projects via green financial bonds, green banks, carbon market tools, other new financial instruments, new policies, fiscal policy, a green central bank, fintech, community-based green funds, and expanding the financing of investments that provide environmental benefits [ 26 , 78 ].
It is evident that green financing plays a crucial role in promoting sustainable initiatives. Thus, a transition from a rising economy to a green economy necessitates that a country's leadership offers green financing [ 112 ]. To assure green economic growth, nations around the world have invested in green projects to promote, invent, and employ environmentally friendly technologies to safeguard the environment and maximize environmental performance [ 55 ]. Because of new stakeholders' and institutions' understanding of environmental issues, regulatory authorities are likely to seek out extra ecologically acceptable financial resources. In an effort to establish environmental legitimacy, this type of environmental proactivity will be required when new methods of providing financial resources and green financing arise.
In numerous ways, the impact of adopting green financing is proven. First, green finance provides financial support for firms engaged in green innovation, including the purchase of green equipment, the introduction of new environmentally efficient technologies, and the training of their personnel. Second, green funding from various projects can assist stakeholders (organizations, governments, and regulators) in spending R&D funds on environmental challenges and minimize the associated risk with green legislation. Lastly, green policies have higher costs than conventional practices, and green finance can assist an organization in covering these expenses without encountering significant financial obstacles. As a result, green finance-driven economic growth can significantly support green policies, lessen environmental pollution, and build sustainable cities [ 128 ].
There have previously been systematic literature reviews conducted in the green finance area. However, a study's reliance on one database can exclude some recent developments in green finance from its analysis [ 93 ]. Findings from several databases could be compared and contrasted to create a more all-encompassing view of the area. Therefore, this study focuses on using Scopus and WoS databases.
Though additional methods, such as systematic literature reviews (SLR) and more complex network analyses such as co-occurrence of index terms, citations, co-citations, and bibliometric coupling, are available, previously conducted studies used a fundamental bibliometric technique [ 23 ]. A more detailed picture of the green finance study setting may emerge from an examination of the identification of various themes.
As part of a systematic review of the literature concerning emerging trends in green finance, it is critical to ascertain the dominant themes that are present in the field. By adopting this methodology, an intentional emphasis is placed on maintaining the review's relevance and excluding any studies that are obsolete. In addition, by identifying and classifying these themes, one can gain significant knowledge regarding the ever-changing characteristics of green finance, thereby illuminating the latest advancements and patterns. A study conducted by Pasupuleti and Ayyagari [ 99 ] identified different themes in green finance, but the researchers were only focused on polluting companies. By amalgamating insights from the literature review, one can attain a holistic comprehension of the current state of research in the field of green finance. Additionally, this process identifies areas where additional inquiry is necessary. Engaging in such an undertaking provides advantages not only to the scholarly community but also carries practical implications for policymakers, practitioners, and investors, assisting them in formulating effective policies and investment strategies and making well-informed decisions.
Green finance research is growing rapidly. However, the rising themes and trends in green finance literature must be comprehended. A comprehensive literature review can summarize current knowledge, identify research gaps, and identify the field's most relevant topics. This study seeks to uncover green finance's emerging themes through a rigorous literature review. This research aims to advance green finance knowledge by synthesizing and analyzing a wide range of scholarly articles.
Methods and methodology
Study selection process and methods.
In this study, a systematic literature review (SLR) was applied. It used inclusion criteria, analysis techniques, and a more objective method of article selection. As recommended for SLRs [ 65 ] with regard to the article selection process, the PRISMA article selection steps were adhered to. The steps are "identification," "screening," and "included". The steps that were taken in this study are shown in Fig. 1 .
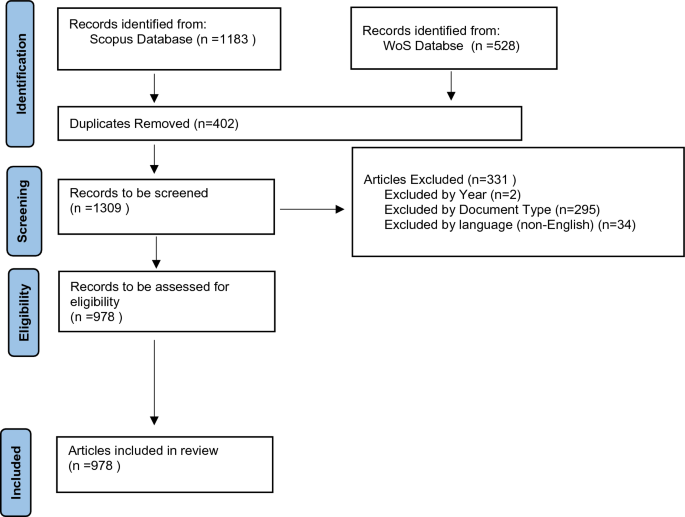
PRISMA article selection flow diagram. Note : Search algorithm; “green finance” . Sources (s) Authors Construct, 2023
In the identification phase, the search terms, search criteria, databases, and data extraction technique are chosen. The keyword to use in the search was "green finance" as the study is aimed at identifying emerging themes in green finance.
The identified articles need to be screened in accordance with the PRISMA guidelines. The tasks carried out at the screening were the screening, retrieval, and evaluation of each article's eligibility. According to Priyashantha et al. in [ 103 ], articles in each task that did not meet the inclusion criteria were removed. The "empirical studies" published in "Journals" from "2011–2023" in "English" were the inclusion criteria for screening the articles. In 2023, up to May, the journal articles were chosen.
This screening was carried out both manually and automatically. Utilizing Scopus' and Web of Science's (WoS) automatic article screening features by study type, language, report type, and publication date, articles achieving the inclusion criteria "empirical studies" published in "English" "journals" from "2011–2023″ were included. The other publication types such as conference papers, book chapters, reviews, research notes, editor's comments, short surveys, and unpublished data, as well as non-English articles and articles published within the considered year range, were excluded. The full versions of the screened articles were then retrieved for the eligibility assessment, the next stage of screening. The author manually evaluated each article's eligibility.
Study risk of bias assessment
Researcher bias in article selection and analysis lowers the quality of reviews [ 8 , 102 ]. Avoiding bias in article selection and analysis requires using a review protocol, adhering to a systematic, objective article selection procedure, using objective analysis methods [ 8 , 102 ], and performing a parallel independent quality assessment of articles by two or more researchers [ 8 ]. By adhering to all of these requirements, the risk of bias in the articles was removed.
Methods of analysis
Biblioshiny and VOSviewer were used for bibliometric analysis. Green finance literature was captured by Scopus and WoS. These databases were used exclusively to get a representative sample of journal articles to study green finance articles. The data were collected and analyzed using Biblioshiny. Select databases can be systematically extracted and analyzed with the software. It collects year-by-year article distribution, journal distribution, country-specific scientific production, most relevant authors, most frequent words, word frequency over time, trend topics, density visualization, etc.
Trends and patterns were found by analyzing green finance paper distribution by year. This analysis shows green finance research's growth. By analyzing article distribution by year, we may also establish green financing and rising theme trends. To identify green finance research publications, article distribution was studied. Academic journal distribution can indicate green finance's prominence in various academic journals. Analyzing scientific production by region reveals regional green finance research tendencies. Scientific production across nations identifies knowledge-producing regions.
Analyzing influential green finance authors helps identify their contributions. This strategy acknowledges influential scholars. The research's most frequently used words reveal the fundamental questions and ideas of environmentally responsible economics. This analysis reveals the discipline's primary topics and studies. By counting words, it may focus on green finance's most important and widely used components. Word frequency can show how green finance's focus has shifted. By tracking word usage, it can identify trending topics. This analysis reveals changing green finance research priorities. Biblioshiny explores green financial trends. This study reveals new topics, research gaps, and subject interests. The trend themes allow us to evaluate green finance studies.
Results and findings
Study selection.
The PRISMA flow diagram illustrates that during the identification step, 528 articles from the WoS database and 1183 articles from the Scopus database that include the term "green finance" were identified. There were 402 duplicates, which were removed. The overall number of articles remained at 1302 at that point. Further attempts were made to include papers on empirical investigations in the final versions that were published in English. 34 non-English articles were thus disregarded. In addition, 295 papers from conferences, book chapters, reviews, news articles, notes, letters, abstracts, and brief surveys were not included. Two articles were disqualified because they were published before 2011. The next step was to retrieve the remaining 978 articles and transfer their pertinent data to an MS Excel file, including the article's title, abstract, keywords, authors' names and affiliations, journal name, citation counts, and year of publication. After that, each article was examined by a third party to determine whether it met the requirements for its eligibility.
Study characteristics
Main information.
This study examined 978 studies by 1830 authors from 59 countries. They've been published in 281 publications. The average number of citations each article received was 12.37. There were a total of 2206 keywords and 44,712 references. This information is detailed in Table 1 .
Annual scientific production
The fluctuations in green financing for scientific production are depicted in Fig. 2 . In 2011, two articles were published that demonstrated interest in this research. No publications were released in 2012, indicating a paucity of research or interest. The trend persisted in 2013 with two articles. One publication appeared in 2014, indicating a halt in research. Since 2015, scientific output has gradually increased. In 2015, three articles contributed to the development of green finance research. Two articles survived in 2016. With eleven articles published in 2017, green finance has become a significant area of study. In 2018, 23 articles were published; in 2019, there will be 42. With 45 publications in 2020, green finance research remains robust. Green finance research increased to 132 publications in 2021. This significant increase in articles on the subject indicates a growing interest in the matter. The publication of 403 research articles in 2022 represents a notable increase. This increase reflects the expanding literature on green finance and its academic significance.
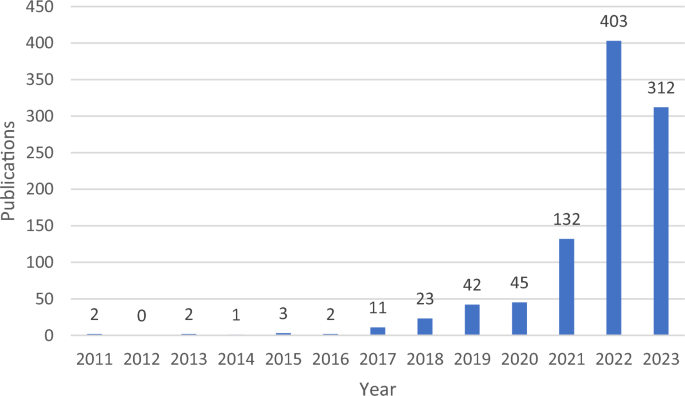
Year-wise research article distribution. Source (s): Author created, 2023
Journal distribution
Table 2 consists of a list of journals that were included in the sample and had more than six relevant papers published inside the journals. The majority of the journals that publish articles relating to green finance are, unsurprisingly, those that focus on environmental science, renewable energy, and sustainability. This is despite the fact that finance is considered an essential component of green financing. Not a single journal in the field of finance was able to attract more than 10 papers.
Based on the number of papers, Environmental Science and Pollution Research emerges as the top journal, demonstrating a strong focus on comprehending the intersection between environmental science, pollution, and financial aspects. The prevalence of journals focused on renewable energy and sustainability, each of which publishes 50 papers, demonstrates the growing interest in examining the financial aspects of sustainable development and renewable energy sources. The fact that Resources Policy was included in the list of 49 papers indicates that a significant emphasis was placed on understanding the financial implications of resource management and extraction.
Green finance is interdisciplinary in nature, exploring the connections between finance and various environmental issues, as evidenced by the existence of interdisciplinary journals like Frontiers in Environmental Science. The existence of journals like Finance Research Letters and Economic Research-Ekonomska Istrazivanja highlights the importance of economic and financial analysis in the context of green finance.
Countries scientific production
The analysis of region frequencies in the provided data in Fig. 3 reveals intriguing patterns and highlights the varying levels of research focus in various countries. The analysis is focused on the top ten countries for scientific production on green finance.
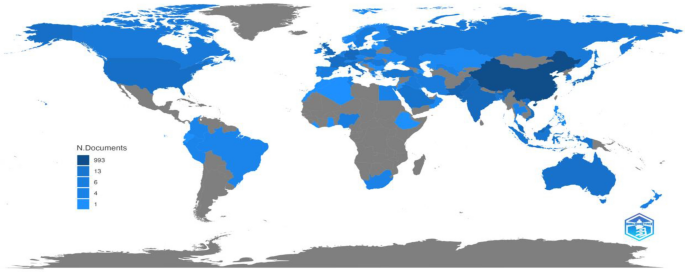
China is the part of the world most frequently mentioned, with a striking frequency of 993. This suggests a significant research interest in comprehending and analyzing diverse aspects of China's economy, policies, and development. Given China's status as the world's most populous nation and its growing global influence, it is unsurprising that researchers have devoted considerable effort to examining China's position in various fields, including finance, sustainability, and innovation.
Pakistan follows with a frequency of 79, indicating a notable but relatively lower research emphasis. Researchers may have investigated particular Pakistan-related topics, such as its economy, governance, or social issues. Pakistan may be of particular interest to a subset of researchers, or there may be a paucity of relevant literature in the analyzed dataset.
With a frequency of 60, the UK is the third-most-mentioned region. This demonstrates a sustained interest in researching various aspects of the UK, such as its economy, financial sector, and policies. It is possible that the historical significance of the UK, particularly in terms of finance and international relations, contributed to its prominence in literature.
Most relevant authors
The prominent and active contributors to the discipline are shown in Fig. 4 . Wang Y has significantly added to the body of literature. The top authors have a constant record of publishing, which shows a dedication to knowledge advancement and suggests a high level of expertise in their field of study.
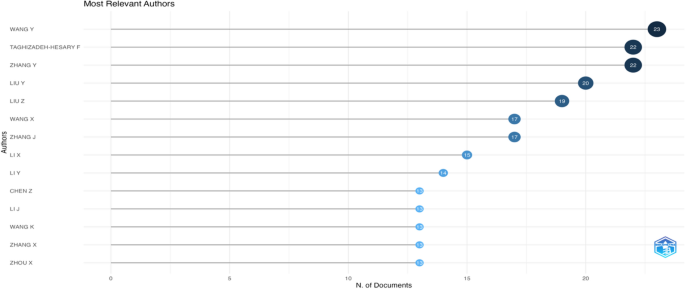
In this section, the findings that conform to the aims of the research are reported. The conclusions were generated through the use of trend themes, keyword co-occurrence analysis, "most frequent words," and "word frequency over time." During the course of the investigation, both the "keyword co-occurrence; network visualization" and the "density visualization" methods were applied.
Most frequent words
The analysis of the most frequent words sheds light on the emerging themes in the field of green finance, as illustrated in Table 3 and Fig. 5 . A significant emphasis on China, which appears 253 times in the literature, is one of the important observations. This indicates that China's initiatives and role in the context of sustainable finance and green investment are gaining increasing recognition. China's approach to green finance and its potential implications for global sustainability initiatives are likely the primary focus of researchers and policymakers.
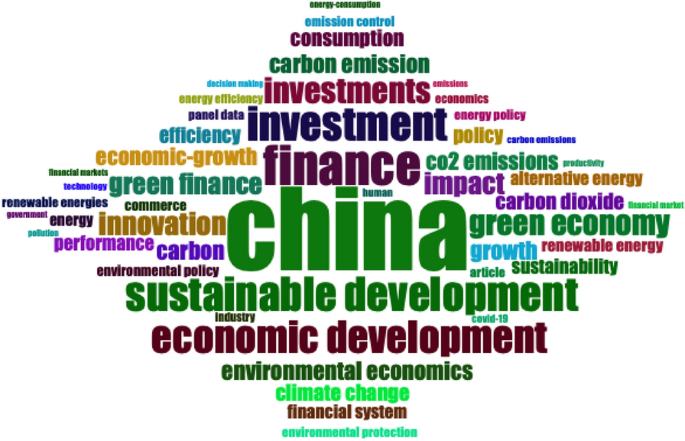
The term "finance" appears 122 times, emphasizing the importance of financial mechanisms and instruments to the advancement of green initiatives. This emphasizes the significance of financial institutions, policies, and frameworks that support environmental protection and sustainable development. The frequency of the term "investment" (103) emphasizes the significance of allocating financial resources to environmentally friendly businesses and initiatives.
The 105 occurrences of "sustainable development" indicate the close relationship between green finance and broader sustainability goals. This indicates that researchers and practitioners recognize the need to align financial decisions with environmental, social, and governance (ESG) factors in order to achieve long-term sustainable development objectives.
The terms "green economy" (75) and "environmental economics" (57) refer to the integration of environmental considerations into economic systems and decision-making procedures. This emphasizes the importance of transitioning to environmentally sustainable economic models and policies.
The frequency of terms such as "carbon," "carbon emissions," and "carbon dioxide" (55, 55, and 51 times, respectively) indicates a focus on mitigating greenhouse gas emissions and addressing climate change via financial mechanisms. This is consistent with the worldwide drive for decarbonization and the transition to low-carbon economies.
In addition, the terms "innovation" (71), "impact" (67), and "efficiency" (49) emphasize the significance of technological advancements, measurable outcomes, and resource optimization in green finance. These ideas illustrate the ongoing pursuit of innovative strategies and solutions to promote positive environmental impact while maximizing resource utilization.
The terms "sustainability" (44), "policy" (49), and "financial system" (41) highlight the need for policy frameworks and a robust financial system to facilitate the incorporation of sustainability considerations into mainstream finance. These themes emphasize the critical role that regulations, incentives, and institutional arrangements play in promoting green finance practices and nurturing a sustainable economy.
In addition, the terms "climate change" (50) and "alternative energy" (42) suggest an emphasis on addressing climate-related issues and investigating renewable and sustainable energy sources. This demonstrates an acknowledgment of the role of green finance in the transition to a low-carbon, resilient future.
The relationships between the keywords depicted as nodes are displayed in Fig. 6 's keyword co-occurrence network visualization. The link shows how each keyword relates to the others. In particular, the thickness of the line indicates how strong the relationship is. As a result, Fig. 8 illustrates how China and green finance are connected by a thicker line, showing that the majority of green finance research is carried out in China. Additionally, the connection between finance, sustainable development, and investments in green finance shows their connection to green finance. In Fig. 6 , the nodes are grouped into the red, green, and blue clusters. These clusters contain the keywords listed in Table 3 for each one. The various clusters in Fig. 6 demonstrate how different areas of research had distinct effects on green financing. When keywords are grouped together, it indicates that the topics they refer to are quite likely to be the same. As a result, the red, green, and blue clusters in Fig. 6 highlight common themes, while Table 4 provides explanations for the clusters.
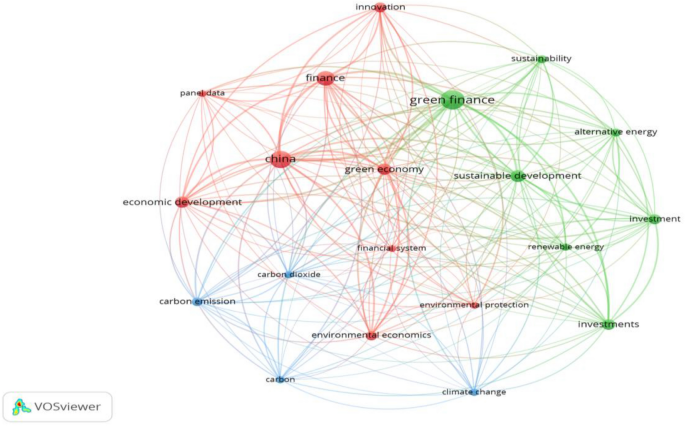
The keyword co-occurrence network visualization
Areas where empirical research is lacking
Figure 7 displays the density visualization map that the VOSviewer generated. The VoSviewer manual states that a node with a red background denotes sufficient research for established knowledge and that it is evident that more study on green finance is still needed. On the other hand, keyword nodes with a green background show that there hasn't been much research on those particular keywords. Other than finance and China, the other keywords in the figure are therefore in the green background, which denotes insufficient research.
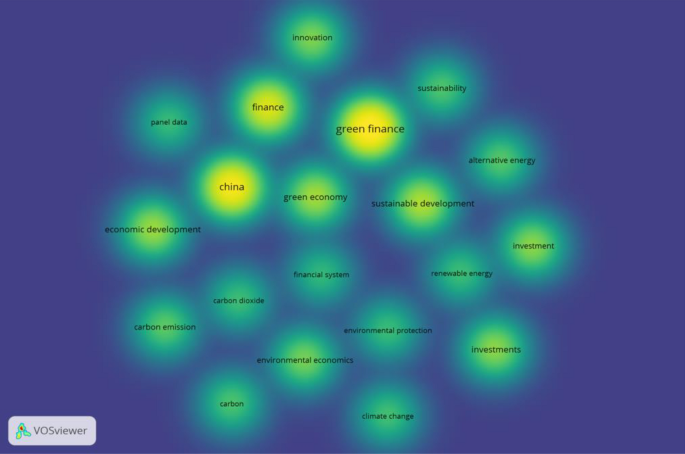
The keyword co-occurrence density visualization
Word’s frequency over time
The analysis of words' frequency over time in Fig. 8 reveals a number of significant trends. Beginning in 2018, the frequency of the term "China" increases considerably, with a significant rise in 2022 and a peak of 253 occurrences in 2023. This indicates a growing emphasis on China's role in green finance and its expanding prominence in the academic literature.
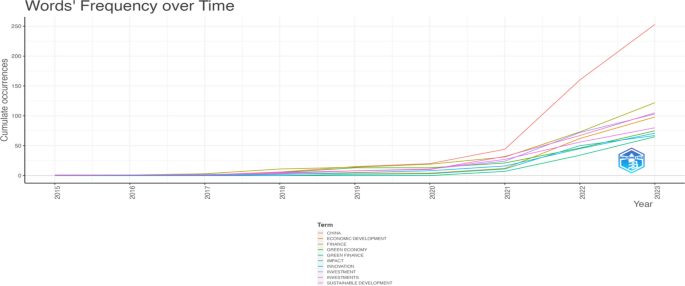
The persistent occurrence of the term "finance" over the years indicates the sustained significance of financial mechanisms and instruments in the context of green finance research. Its increasing frequency over time demonstrates the continued emphasis placed on financial aspects of the field.
The consistent growth of the term "sustainable development" from 2016 to 2019 indicates a growing recognition of the connection between green finance and broader sustainability objectives. However, after 2019, its occurrence remains comparatively stable, indicating that sustainable development has become a well-established and consistent theme in the literature.
Similarly, the term "investment" has maintained a consistent presence throughout the years, indicating a continued emphasis on allocating financial resources to green and sustainable initiatives. Its frequency fluctuates but remains relatively high throughout the period under consideration.
The frequency of the term "economic development” increased gradually until 2021, after which it remained relatively stable. This indicates that researchers have acknowledged the need to incorporate economic development and sustainable practices, resulting in a continued emphasis on this topic.
Similar to the term "investments," it has maintained a consistent presence throughout the years. This demonstrates a persistent desire to investigate investment opportunities and strategies within the context of green finance.
The frequency of the term "green economy” increased until 2020, after which it stabilized. This demonstrates an ongoing commitment to transitioning to a greener and more sustainable economy.
The terms "innovation" and "impact" have exhibited a general upward trend over the years. This suggests that innovative approaches to measuring the impact of green finance initiatives and projects are gaining importance.
The term "green finance" has been used significantly more frequently, particularly after 2021. This demonstrates the increasing interest and focus on the specific discipline of green finance, reflecting its emergence as a distinct research area within the context of sustainable finance as a whole.
Trend topics
Insights into novel areas and their developments over time can be gained from an analysis of trend themes using author keywords in the bibliometric data, as shown in Fig. 9 .
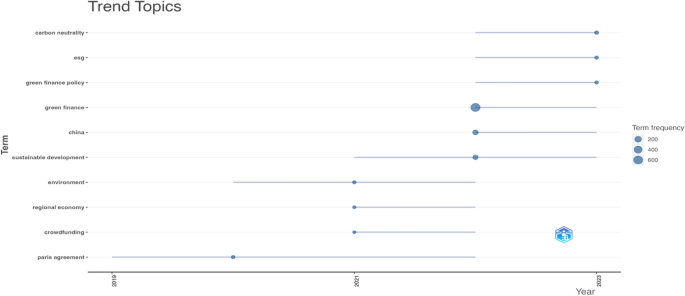
Trend Topics
There are nine times where the "Paris Agreement" is mentioned as a subject. It was consistently present from 2019 to 2022, demonstrating a strong interest in comprehending the ramifications and execution of this global climate agreement. The Paris Agreement's effects on environmental regulations and attempts to slow down climate change were probably among the topics on which researchers concentrated.
Seven uses of the word "environment" show that it is a recurring subject. This implies maintaining a focus on environmental concerns and the interactions between human actions and the environment as a whole. It's likely that academics and researchers have examined numerous environmental concerns and their effects on various industries and regulations.
Six occurrences of "regional economy" are found in the literature. This shows a rise in interest in learning about the dynamics and growth of regional economies and how they relate to sustainable practices. The emphasis on regional economies indicates that scholars are looking at the regional and context-specific elements affecting sustainable development and economic progress.
Another subject with five mentions per topic is "crowdfunding". This shows that crowdsourcing is becoming more and more popular as a method of finance, especially for sustainable projects. Crowdfunding's ability to assist green projects, as well as the opportunities and challenges that come with it, has probably been studied by researchers.
With 631 occurrences, the topic "green finance" stands out due to its very high frequency and demonstrates its rising importance in the literature. This demonstrates a rise in interest in the nexus between finance and environmental sustainability. The methods, laws, and procedures that encourage financial investments in green projects and companies have probably been studied by academics and policymakers.
With 92 mentions, "China" stands out as being quite popular. In the context of green finance and sustainable development, this suggests a strong focus on China's participation. Researchers are probably looking at China's policies and initiatives and how they may affect international sustainability efforts.
The phrase "sustainable development" also comes up 70 times, demonstrating a steadfast interest in learning and implementing sustainable practices in a variety of fields. There is a good chance that academics have looked into the frameworks, policies, and tactics that help achieve long-term sustainable development goals.
Seventeen times are mentioned when the term "carbon neutrality" is brought up, which shows that efforts to achieve it are becoming more and more of a priority. To minimize greenhouse gas emissions and combat climate change, researchers have probably looked into a variety of strategies and regulations.
ESG (environmental, social, and governance) is a term with a frequency of ten references, which reflects the growing understanding of the significance of ESG aspects in investment choices and company practices. The incorporation of ESG factors into financial analysis and decision-making processes has probably been researched by researchers and practitioners.
Last but not least, the phrase "green finance policy" is used nine times, showing that policies that support and oversee green finance efforts are a particular emphasis of the study. It's likely that academics and policymakers have looked at how well these policies work and how they affect the growth of sustainable practices and investments.
In conclusion, study subjects that have attracted interest over time are shown by an analysis of trend topics in the bibliometric data. These themes show the continued attempts to understand and manage environmental concerns through research, policy, and finance, from global agreements like the Paris Agreement to specific topics like green finance and sustainable development.
Themes of green finance
This study uncovered a variety of topics relating to green finance as well as potential areas for further research. The descriptions of the themes are presented in Fig. 10 . Different themes related to green finance, along with significant studies that contributed significantly, are discussed below.
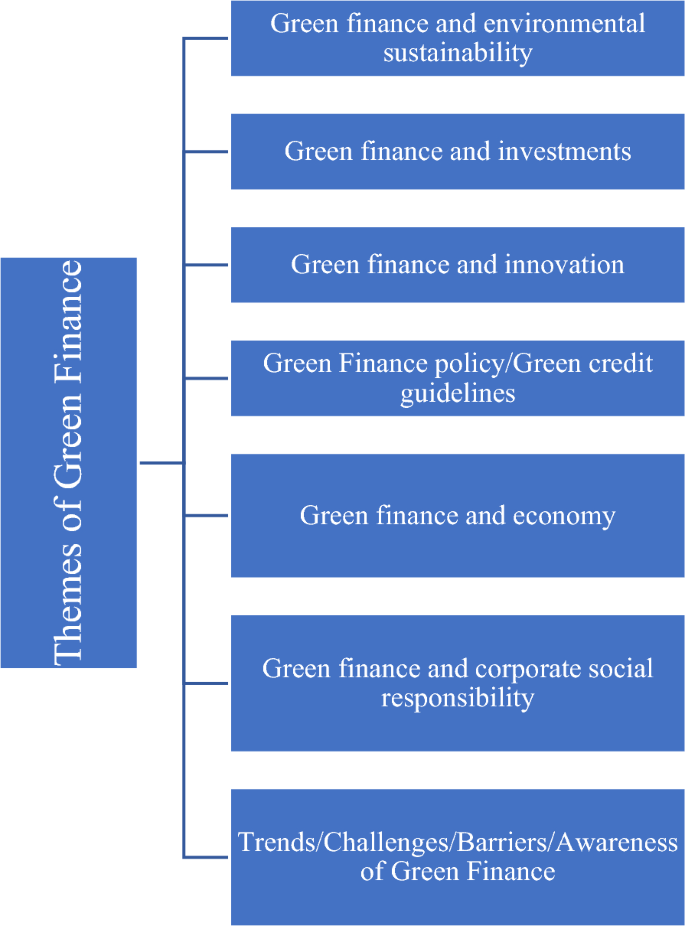
Green finance and environmental sustainability
In recent years, there has been a growing emphasis on the significance of green finance and environmental sustainability, leading to increased attention and focus in both academic research and practical applications. The world is currently experiencing an unparalleled environmental crisis, with issues like resource depletion, biodiversity loss, and climate change becoming more pressing. Green finance, which falls under the umbrella of sustainable finance, centers its attention on investments and financial methods that not only yield economic profits but also contribute to favorable environmental consequences.
Existing research mostly focuses on green finance and environmental sustainability in Asian countries, with specific focus on China. Green finance's function in low-carbon development has been thoroughly studied in relation to carbon emissions [ 13 , 147 ]. Green financing and renewable energy growth have also received attention, aiding China's clean energy revolution [ 4 , 12 , 20 , 21 , 40 , 49 , 51 , 56 , 61 , 67 , 72 , 75 , 76 , 80 , 85 , 89 , 97 , 104 , 105 , 107 , 109 , 110 , 119 , 121 , 129 , 135 , 144 , 145 , 149 , 169 , 172 ]. Environmental rules and green finance have also been studied to determine how well they promote sustainable financing [ 19 , 22 , 62 , 114 , 123 , 145 , 159 ].
When it comes to the study of regions outside of Asia, such as Africa, South America, and parts of Europe, there is a significant knowledge gap. It may be helpful to gain useful insights into regional variances and strategies if one is able to comprehend the various ways in which these various regions approach green financing and environmental sustainability initiatives.
Green finance and investments
Following a global shift toward sustainable and ecologically responsible economic practices, green finance and investments have developed dramatically.
Green bond quality and effectiveness, notably in China, is a major study topic. Green bonds finance ecologically friendly projects, therefore verifying their quality is crucial to green financial markets. To help green bonds meet sustainability goals, researchers have studied their quality procedures and standards [ 3 , 6 , 9 , 10 , 33 , 34 , 35 , 38 , 79 , 92 , 95 , 108 , 115 , 164 ]. The relationship between green and non-green investments is another frequent research topic. Researchers have studied the hedging or diversification impacts of these two assets. This study examines how green and non-green investments affect portfolio strategies, risk management, and the financial environment [ 1 , 116 ]. Another interesting relationship is natural resource richness, FDI, and regional eco-efficiency. Given global agreements like COP26, scholars are studying how natural resources and FDI effect regional ecological efficiency as states attempt to combine economic growth with environmental sustainability [ 15 , 36 , 42 , 143 , 157 ].
A key feature of green finance study is how financial institutions, integrate green investment and financing teams. The green finance agenda requires understanding how bank’s structure and behave to encourage sustainable investment. Green financial instrument creation and effect are another study topic. Researchers have examined green finance products including green bonds and minibonds to determine their performance and impact on environmental and sustainability goals. This field helps design policies and strategies to optimize industrial structures and promote sustainable development.
Green finance research examines how it affects industrial structures. Studies have examined how green finance initiatives including loans and investments optimize and shift industrial sectors toward sustainability. These findings are crucial for governments and business stakeholders seeking financial incentives for eco-friendly operations [ 12 , 31 , 46 , 57 , 85 , 96 , 124 , 130 , 139 ].
Green finance market interactions with financial variables must also be assessed for sustainable financial development. Researchers examine the relationship between green financial indices and other financial indicators to better understand how green finance affects the financial landscape [ 27 , 32 , 48 , 68 , 137 ].
Green finance and investments have many unexplored areas, presenting research opportunities. The behavioral dimensions of green investment focus on the psychological drivers and biases that influence investment choices; subnational and local initiatives, which are frequently ignored despite their crucial role in ecological action; cross-country comparisons to provide a more holistic view of effective green finance practices; the role and impact of green finance in emerging economies; and innovative green financial instruments like blockchain. Examining these lesser-known aspects could improve our understanding of sustainability in the financial sector and offer insightful information to investors, financial institutions, and legislators that want to make a positive impact on a more sustainable and environmentally friendly future.
Green finance and innovation
The convergence of green finance and innovation is a crucial topic that addresses the pressing global concerns of environmental sustainability and financial stability. Much study has been done on green finance and innovation, yet various themes and gaps emerge, demonstrating its complexity.
Green financing policies and instruments promote innovation, especially in environmental technologies and renewable energy. Many studies have studied how green funding affects green innovation and if it promotes sustainable technology. They've studied green bonds, green banking, and green finance reform laws, offering empirical evidence that financial incentives combined with green practices can stimulate environmental innovation [ 16 , 41 , 44 , 47 , 52 , 64 , 70 , 81 , 87 , 107 , 133 , 152 , 162 ].
The role of environmental legislation in green financing and innovation is another common theme. Researchers have studied how these restrictions affect green finance's impact on technology. Studying how financial policies and regulatory frameworks interact has helped explain the complex dynamics affecting innovation in environmentally sensitive industries [ 11 , 29 , 54 , 84 , 120 , 126 , 132 , 151 , 152 , 174 ].
Nevertheless, there are obvious gaps in the existing knowledge within the field. The effects of green finance on innovation have been extensively studied, but a better knowledge of the factors driving innovation in other areas is needed. Further study may reveal how green funding might boost innovation in non-environmental industries. How can financial mechanisms support sustainable transportation, agricultural, and urban planning innovation.
Further research is needed on education and the human element in green innovation. How green finance, educational investments, and innovation interact can help individuals, businesses, and societies develop a sustainable future. Green finance and innovation's impact on environmental adaptation and resilience also understudied. More research is needed to determine how financial mechanisms and new solutions may help communities and organizations adapt to climate change.
Green finance policy/green credit guidelines
Climate change and environmental degradation are major worldwide issues. Green finance, which promotes environmentally and socially responsible investments, is a key instrument in this battle. Research and discussion have focused on how green finance policies affect the economy and environment.
The switch to renewable energy is crucial to fighting climate change globally. This transition relies on green financing initiatives. Researchers are investigating how well such regulations promote renewable energy. They examined how green finance regulations affect renewable energy output, investment, and job development in this growing sector. Understanding these implications helps improve green finance initiatives for sustainability [ 18 , 98 , 118 ].
China and other nations have implemented green finance pilot programs to test the waters and stimulate innovation. This research evaluates pilot policy implementation and impacts. Scholars use synthetic control and other tools to study how these initiatives affect green innovation. The results help determine the real-world implications of such experiments and their potential for wider use [ 48 , 113 , 121 , 131 , 146 , 162 ].
Green financing policies vary worldwide. Comparative research of green financing rules can highlight policy differences among jurisdictions. Researchers compared the EU and Russia's green financing laws. These studies emphasize differences, similarities, and the potential influence of these policies on green finance development, promoting cross-border cooperation and knowledge exchange [ 60 , 125 ].
Monitoring and measuring green finance progress is essential for future development. Researchers are developing green finance indices to assess green finance in a country or region. These indices help policymakers, investors, and the public understand green finance's growth and potential [ 141 ].
Despite significant and informative research on green finance policies and their effects on the economy and environment, several research gaps and opportunities for additional investigation remain. First, a thorough evaluation of the durability and long-term sustainability of green finance policies is lacking in the literature. Many studies focus on short-term outcomes, but long-term planning and implementation need understanding these policies' long-term implications. Second, green finance policies' cross-border effects need greater study. As the global economy grows more interconnected, it's important to understand how regional policies affect others and the possibility for international collaboration. Green finance and social effects as creating employment and community development are understudied. Such studies could illuminate these policies' overall impact. Finally, additional multidisciplinary research combining economics, environmental science, and social science are needed to comprehend green finance policies' complex implications. Scholars can fill these gaps to improve our understanding of this crucial topic and inform sustainable policymaking.
Green finance and economy
The relationship between carbon intensity and economic development is a growing topic in green finance research. How nations may shift to low-carbon economies while maintaining economic growth has been studied. Several studies have quantified how green finance policies reduce carbon emissions and boost economic growth [ 63 , 71 , 122 , 155 , 175 ].
The study of the impact of green financing on agriculture, particularly in China, is gaining attention. Green financing impacts agricultural trade, sustainability, and food security, according to researchers [ 37 , 140 ]. Given its connection with economics, food production, and sustainability, this type of researches is crucial.
Efficient utilization of natural resources in Asian countries has gained attention for promoting green economic growth. Researchers have studied how nations might maximize economic gains from natural resources while reducing environmental harm. Addressing sustainable economic development concerns requires this area [ 86 , 101 , 146 , 166 ].
The significance of judicial quality in reducing emissions without hindering economic growth is a common issue in green finance research. Researchers examine how strong legal systems can enforce environmental laws and promote green practices while boosting the economy [ 154 ].
Even while the previously stated research topics have unquestionably enhanced our understanding of the intricacies of green finance, there are still a number of uncharted territories and research gaps that need to be investigated further. Currently, research on green finance mostly focuses on economic and environmental concerns. Integrated research combining economic, environmental, and social science is needed. It can provide a holistic view of green finance policy' many implications. The globalization of green finance policy has significant implications and cross-border effects. These policies' worldwide spillover effects and country collaboration are rarely studied. Research is lacking on how regional policies affect others and international cooperation.
Green finance and corporate social responsibility
Fostering CSR requires understanding how environmental regulations affect companies' sustainable strategies. Researchers should examine how CSR goals can be better aligned with regulations to improve environmental and social outcomes. Researchers have studied green finance-CSR approaches to promote sustainability. This research seeks to understand how green finance initiatives like green bonds and sustainable investment practices affect CSR performance [ 173 ]. Businesses and investors looking to maximize their environmental and social impact must understand these mechanisms.
One intriguing research topic is empirical evidence from heavily polluting enterprises, especially in China. This study shows how green finance can reduce environmental harm and promote CSR in industries with a high environmental impact [ 45 , 66 ]. Researchers can find ways to help heavily polluting companies become more sustainable by studying their experiences.
Bangladesh banks' CSR and green finance practices have also been studied [ 168 ]. This study studies how green financing affects financial institution CSR and environmental performance. Financial organizations can use these results to incorporate environmental responsibility while being profitable. Another relevant research topic is post-pandemic CSR practices as a business strategy to combat volatility and drive energy and environmental transition [ 53 ]. Understanding how CSR and green finance can help companies whether economic downturns and pandemics are crucial. This research can help businesses adapt to changing business conditions.
Further studies can explore socially responsible mutual funds and low-carbon economies. The impact of the investment industry on sustainability and environmental responsibility can be better understood by scholars by examining how these funds affect company behavior and investment decisions. Investors and businesses pursuing sustainable development may find these insights to be beneficial.
Green bond issuance is growing, thus study on its effects on company performance and CSR is needed. Investors seeking to support environmentally responsible businesses and companies contemplating green finance must have a comprehensive understanding of the repercussions on associated with green financing.
Trends/challenges/barriers/awareness of green finance
Regional patterns in China's green finance trends are well-studied, but little is known about applying these findings elsewhere, especially in countries with similar environmental issues [ 24 , 30 , 83 , 88 ]. Analysis of green finance growth by sector is common; however, there may be a knowledge vacuum about how sectors might learn from each other to create more successful sectoral plans [ 28 , 50 , 142 ].
Analyzing the structural barriers to green financing is vital, but also understanding how consumers, financial institutions, and governments can work together to close this gap is crucial. Political and institutional restrictions in green financing have been extensively examined, but cross-national comparisons might reveal similar concerns and inventive solutions. Cultural variety is crucial in ethical and green finance, but the challenges of adapting cultural methods to different places may not be adequately examined [ 7 ].
There were 213 papers pertaining to green finance research that were published between the years 2011 and 2021. However, between 2022 and May 2023, there was an enormous increase in the number of publications, which was 715. These publications can be found in Scopus and WoS. This spike can be associated with a number of causes that have encouraged both academia and industry to focus on sustainable and environmentally friendly practices. These drivers can be found in both the public and private sectors.
To begin, there has been a growing awareness of the urgent need to address climate change and its adverse impacts on the world. An increasing number of demands for action have accompanied this recognition. Green finance provides a means by which funds can be directed toward projects and investments that promote environmental sustainability, such as the development of sustainable infrastructure, clean technologies, and renewable sources of energy. In addition, global initiatives such as the Paris Agreement have put pressure on governments and financial institutions to align their strategies with climate goals, which has led to an increased demand for research on green finance practices and regulations [ 58 ]. Additionally, investors and consumers are becoming more aware of the environmental impact of their financial actions, which is contributing to an increase in demand for environmentally responsible investing products and services [ 39 ]. As a direct consequence of these developing tendencies, researchers and academics have developed responses to them, adding to the expanding body of literature on green finance.
993, more than any other nation, are references to China. This shows a keen interest in learning about China's economy, politics, and development. Researchers have concentrated on China's position in finance, sustainability, and innovation given its status as the world's largest population country and its growing global relevance due to its critical role in fostering sustainable and low-carbon development. Reduced energy use and waste are the goals of energy efficiency measures, which also have a positive effect on the environment by reducing greenhouse gas emissions. Researchers want to comprehend the procedures, regulations, and financial tools that can successfully encourage and support energy efficiency projects, which will ultimately contribute to a greener and more sustainable future. This is why they are focused on energy efficiency within the context of green finance [ 2 , 14 , 60 , 67 , 69 , 74 , 106 , 117 , 134 , 136 , 156 , 160 , 170 ].
The construction of pilot zones for green finance reform and innovations (GFRI) is a significant step the Chinese government has taken to build a green economy. Many authors have conducted surveys on China's GFRI policy and its impact on innovations. The GFRI policy program supports green innovation in large, polluting companies and urban green development by enhancing total factor productivity in pilot cities, emphasizing the importance of debt finance in corporate green innovation [ 40 , 82 , 148 , 150 , 153 , 158 ]. A different study by Wang et al. in 2022 [ 127 ] discovered that while the GFRP generally plays a positive role in fostering green technology innovation capabilities, the extent to which it has an impact varies depending on the region's resources, environment, and level of economic development, with middle- and high-income areas seeing a more noticeable impact. Wang et al. in 2022 [ 127 ] propose a green finance index, employing statistical indicators from 2011 to 2019, to analyze China's green finance development and predict its growth from 2020 to 2024. New energy, green mobility, and new energy vehicles have boosted China's green finance index during the previous nine years, according to research.
The Green Financial Reform and Innovation Pilot Zones (GFPZ) policy's effect on the ESG ratings of Chinese A-share listed firms between 2014 and 2020 is examined in another study. The findings showed that the GFPZ policy raises ESG scores, which are mainly based on social responsibility, and helps businesses in the pilot zones do better financially and environmentally [ 17 ]. In 2023, Shao and Huang [ 111 ] reviewed China's green finance policy mix, showing a shift toward market-based approaches and greater private sector engagement, influenced by dynamic vertical interactions between different levels of government.
Chen et al. [ 14 ] examined the response of China's equity funds to institutional pressure on green finance in 2021. The results showed that funds with negative screening strategies, which exclude environmentally harmful investments, have higher green investment levels and higher financial returns, while funds with positive screening strategies face negative investor reactions despite their green investments.
A study done by Lv et al. [ 88 ] found that while green finance development in China is improving, regional disparities and a polarization trend exist, requiring measures to narrow the gap and promote coordinated development across economic regions. Because it is crucial for striking a balance between economic development, environmental conservation, and social well-being, researchers in green finance concentrate on sustainability. The authors focused on studies on sustainable investment options, analyzed how environmental, social, and governance aspects are incorporated into financial decision-making, and evaluated how sustainability affects financial performance. Researchers are expected to advance ethical and sustainable financial practices and help the world accomplish its sustainability goals by studying sustainability within the context of green finance [ 5 , 25 , 43 , 46 , 59 , 73 , 77 , 90 , 91 , 94 , 104 , 109 , 138 , 161 , 163 , 165 , 167 , 171 ].
In conclusion, research on green finance has primarily focused on Asian countries, particularly China, where it plays a crucial role in low-carbon development and renewable energy growth. However, there is a significant knowledge gap in regions outside Asia, such as Africa, South America, and parts of Europe. Further research is needed to understand regional variances and strategies in these areas.
Studies have examined various aspects of green finance, including green bond quality, the relationship between green and non-green investments, and the impact of green finance on environmental and sustainability goals. Behavioral dimensions of green investment, subnational and local initiatives, cross-country comparisons, and the role of green finance in emerging economies have also been explored. Additionally, the role of green finance in stimulating innovation in environmental technologies and renewable energy has been studied, but there are gaps in understanding its impact on non-environmental industries and the human element in green innovation.
Further research is needed to understand the role of environmental legislation in green finance, its impact on technology, and its cross-border effects. The durability and long-term sustainability of green finance policies should also be examined, along with their social effects such as employment creation and community development. The relationship between carbon intensity and economic development, as well as the alignment of corporate social responsibility goals with environmental regulations, are important areas for investigation.
There is a need for more research on applying the findings from China's green finance trends to other countries facing similar environmental issues. Structural barriers to green financing should be analyzed, and the collaboration between consumers, financial institutions, and governments in closing this gap should be explored. Cultural diversity in ethical and green finance should also be considered, along with the challenges of adapting cultural methods to different places. Overall, further research in these areas can contribute to a more sustainable and environmentally friendly future.
When compared to other fields of study, it is clear that research on green finance has not been investigated to the same extent. In contrast to the less-researched areas of carbon, carbon emissions, climate change, financial systems, policymaking, agriculture, CSR, supply chain, risk management, corporate strategy, regional planning, and governance, green financing has been well-liked with investments, sustainable developments, green innovations, and green economies. On the other hand, taking into account the growing attention paid to sustainability on a worldwide scale and the pressing need to find solutions to the problems posed by the environment, it is quite likely that research into green finance will become more important in the years to come.
The increasing significance of sustainable development and the change to an economy with lower carbon emissions will require the development of innovative financial solutions to support green initiatives and assist the shift toward a financial system that is more friendly to the environment and more sustainable. It is anticipated that researchers will devote a greater amount of attention to green finance as the level of awareness regarding the environmental and social impacts of financial activities continues to rise. These researchers will investigate topics such as sustainable investment strategies, green bond markets, sustainable banking practices, and the incorporation of environmental considerations into financial decision-making. In addition to this, the incorporation of environmentally friendly financial practices into policy frameworks and regulatory measures further emphasizes the requirement for research in this particular area. In general, it is projected that research on green finance will pick up steam in the years to come because it plays such an important role in the process of sculpting a financially sustainable and resilient.
Availability of data and materials
SCOPUS and WoS databases.
Abbreviations
Corporate social responsibility
Financial Technology
Green finance reform and innovations
Green Financial Reform and Innovation Pilot Zones
- Systematic literature review
Akhtaruzzaman M, Banerjee AK, Ghardallou W, Umar Z (2022) Is greenness an optimal hedge for sectoral stock indices? Econ Model. https://doi.org/10.1016/j.econmod.2022.106030
Article Google Scholar
An Q, Lin C, Li Q, Zheng L (2023) Research on the impact of green finance development on energy intensity in China. Front Earth Sci 11:1118939. https://doi.org/10.3389/feart.2023.1118939
Azhgaliyeva D, Kapsalyamova Z, Mishra R (2022) Oil price shocks and green bonds: An empirical evidence. Energy Econ. https://doi.org/10.1016/j.eneco.2022.106108
Bai J, Chen Z, Yan X, Zhang Y (2022) Research on the impact of green finance on carbon emissions: evidence from china. Econ Res-Ekon Istraz 35(1):6965–84. https://doi.org/10.1080/1331677X.2022.2054455
Bai X, Wang K-T, Tran TK, Sadiq M, Trung LM, Khudoykulov K (2022) Measuring china’s green economic recovery and energy environment sustainability: econometric analysis of sustainable development goals. Econ Anal Policy. https://doi.org/10.1016/j.eap.2022.07.005
Barua S, Chiesa M (2019) ‘Sustainable financing practices through green bonds: What affects the funding size? Bus Strategy Environ. https://doi.org/10.1002/bse.2307
Borbely, Emese. ‘The Relevance of Cultural Diversity in Ethical and Green Finance’. In Sustainable Economic Development: Green Economy and Green Growth , edited by W. L. Filho, D. M. Pociovalisteanu, and A. Q. AlAmin, 67–82. Cham: Springer International Publishing Ag, 2017. https://doi.org/10.1007/978-3-319-45081-0_4 .
Brereton P, Kitchenham B, Budgen D, Turner M, Khalil M (2007) Lessons from applying the systematic literature review process within the software engineering domain. J Syst Softw 80(4):571–583. https://doi.org/10.1016/J.JSS.2006.07.009
Cerqueti R, Deffains-Crapsky C, Storani S (2023) Green Finance Instruments: Exploring Minibonds Issuance in Italy. Corpor Soc Responsib Environ Manag. https://doi.org/10.1002/csr.2467
Chang K, Liu L, Luo D, Xing K (2023) The impact of green technology innovation on carbon dioxide emissions: the role of local environmental regulations. J Environ Manag. https://doi.org/10.1016/j.jenvman.2023.117990
Chang L, Moldir M, Zhang Y, Nazar R (2023) Asymmetric impact of green bonds on energy efficiency: fresh evidence from quantile estimation. Util Policy. https://doi.org/10.1016/j.jup.2022.101474
Chen D, Haiqing H, Chang C-P (2023) Green finance, environment regulation, and industrial green transformation for corporate social responsibility. Corpor Soc Responsib Environ Manag. https://doi.org/10.1002/csr.2476
Chen J, Li L, Yang D, Wang Z (2023) The dynamic impact of green finance and renewable energy on sustainable development in China. Front Environ Sci 10:1097181. https://doi.org/10.3389/fenvs.2022.1097181
Chen J, Siddik AB, Zheng G-W, Masukujjaman M, Bekhzod S (2022) The effect of green banking practices on banks environmental performance and green financing: an empirical study. Energies. https://doi.org/10.3390/en15041292
Chen Q, Ning Bo, Pan Y, Xiao J (2022) Green finance and outward foreign direct investment: evidence from a Quasi-natural experiment of green insurance in China. Asia Pacific J Manag 39(3):899–924. https://doi.org/10.1007/s10490-020-09750-w
Chen S, Xie G (2023) Assessing the linkage among green finance, technology, and education expenditure: evidence from G7 economies. Environ Sci Pollut Res. https://doi.org/10.1007/s11356-023-25625-1
Chen Z, Ling H, He X, Liu Z, Chen D, Wang W (2022) Green financial reform and corporate ESG performance in China: empirical evidence from the green financial reform and innovation pilot zone. Int J Environ Res Public Health. https://doi.org/10.3390/ijerph192214981
Cheng Z, Kai Z, Zhu S (2023) Does green finance regulation improve renewable energy utilization? Evidence from energy consumption efficiency. Renew Energy. https://doi.org/10.1016/j.renene.2023.03.083
Cho H, Lehner OM, Nilavongse R (2020) Combining financial and ecological sustainability in bank capital regulations. J Appl Account Res. https://doi.org/10.1108/JAAR-10-2020-0221
Chu H, Hongjuan Y, Chong Y, Li L (2023) Does the development of digital finance curb carbon emissions? Evidence from county data in China. Environ Sci Pollut Res. https://doi.org/10.1007/s11356-023-25659-5
Cui Y, Wang G, Irfan M, Desheng W, Cao J (2022) The effect of green finance and unemployment rate on carbon emissions in China. Front Environ Sci 10:887341. https://doi.org/10.3389/fenvs.2022.887341
Deng W, Zhang Z (2023) Environmental regulation intensity, green finance, and environmental sustainability: empirical evidence from China based on spatial metrology. Environ Sci Pollut Res. https://doi.org/10.1007/s11356-023-26946-x
Desalegn G, Fekete-Farkas M, Tangl A (2022) The effect of monetary policy and private investment on green finance: evidence from Hungary. J Risk Financ Manag 15(3):117. https://doi.org/10.3390/jrfm15030117
Ding R, Du Y, Du L, Fu J, Chen S, Wang K, Xiao W, Peng L, Liang J (2023) Green finance network evolution and prediction: fresh evidence from China. Environ Sci Pollut Res. https://doi.org/10.1007/s11356-023-27183-y
Dong C, Hao Wu, Zhou J, Lin H, Chang L (2023) Role of renewable energy investment and geopolitical risk in green finance development: empirical evidence from BRICS countries. Renew Energy. https://doi.org/10.1016/j.renene.2023.02.115
Duchêne S (2020) Review of Handbook of Green Finance. Available at from: https://www.sciencedirect.com/science/article/abs/pii/S0921800920311873 .
Fabozzi FJ, Tunaru DE, Tunaru RS (2022) The interconnectedness between green finance indexes and other important financial variables. J Portfolio Manag. https://doi.org/10.3905/jpm.2022.1.392
Falcone PM, Sica E (2019) Assessing the opportunities and challenges of green finance in Italy: an analysis of the biomass production sector. Sustainability 11(2):517. https://doi.org/10.3390/su11020517
Fang Y, Shao Z (2023) How does green finance affect cleaner industrial production and end-of-pipe treatment performance? Evidence from China. Environ Sci Pollut Res 30(12):33485–33503. https://doi.org/10.1007/s11356-022-24513-4
Feng W, Bilivogui P, Wu J, Mu X (2023) Green finance: current status, development, and future course of actions in China. Environ Res Commun 5(3):035005. https://doi.org/10.1088/2515-7620/acc1c7
Gao J, Wu D, Xiao Q, Randhawa A, Liu Q, Zhang T (2023) green finance, environmental pollution and high-quality economic development—a study based on China’s provincial panel Data. Environ Sci Pollut Res. https://doi.org/10.1007/s11356-022-24428-0
Gao L, Tian Q, Meng F (2023) The impact of green finance on industrial reasonability in China: empirical research based on the spatial panel durbin model. Environ Sci Pollut Res. https://doi.org/10.1007/s11356-022-18732-y
García-Lamarca M, Ullström S (2022) Everyone wants this market to grow: the affective post-politics of municipal green bonds. Environ Plann E: Nat Space. https://doi.org/10.1177/2514848620973708
Gilchrist D, Yu J, Zhong R (2021) The limits of green finance: a survey of literature in the context of green bonds and green loans. Sustainability. https://doi.org/10.3390/su13020478
Glomsrød S, Wei T (2018) Business as unusual: the implications of fossil divestment and green bonds for financial flows, economic growth and energy market. Energy Sustain Dev. https://doi.org/10.1016/j.esd.2018.02.005
Gong W (2023) A study on the effects of natural resource abundance and foreign direct investment on regional eco-efficiency in China under the target of COP26. Resour Policy. https://doi.org/10.1016/j.resourpol.2023.103529
Guo J, Zhang K, Liu K (2022) Exploring the mechanism of the impact of green finance and digital economy on China’s green total factor productivity. Int J Environ Res Public Health 19(23):16303. https://doi.org/10.3390/ijerph192316303
Hadaś-Dyduch M, Puszer B, Czech M, Cichy J (2022) Green bonds as an instrument for financing ecological investments in the V4 countries. Sustainability. https://doi.org/10.3390/su141912188
Hales R, Dai J, Fu T (2023) Green finance: opportunities and challenges for the financial sector. J Financ Serv Res 1–23
Han S, Zhang Z, Yang S (2022) Green finance and corporate green innovation: based on china’s green finance reform and innovation pilot policy. J Environ Public Health 2022:1833377. https://doi.org/10.1155/2022/1833377
Han Y, Tan S, Zhu C, Liu Y (2023) Research on the emission reduction effects of carbon trading mechanism on power industry: plant-level evidence from China. Int J Climate Change Strateg Manag. https://doi.org/10.1108/IJCCSM-06-2022-0074
Hawash MK, Taha MA, Hasan AA, Braiber HT, Mahdi RAA, Thajil KM, Albakr AMA (2022) Financial inclusion, foreign direct investment, green finance and green credit effect on iraq manufacturing companies sustainable economic development: a case on static panel data. CUAD Econ 45(128):53–60. https://doi.org/10.32826/cude.v1i128.706
He C, Yan G (2020) Path selections for sustainable development of green finance in developed coastal areas of China. J Coast Res. https://doi.org/10.2112/JCR-SI104-014.1
He J, Iqbal W, Fangli Su (2023) Nexus between renewable energy investment, green finance, and sustainable development: role of industrial structure and technical innovations. Renew Energy. https://doi.org/10.1016/j.renene.2023.04.010
He L, Zhong T, Gan S (2022) Green finance and corporate environmental responsibility: evidence from heavily polluting listed enterprises in China. Environ Sci Pollut Res 29(49):74081–74096. https://doi.org/10.1007/s11356-022-21065-5
Hu J, Zhang H (2023) Has green finance optimized the industrial structure in China? Environ Sci Pollut Res 30(12):32926–32941. https://doi.org/10.1007/s11356-022-24514-3
Huang Di (2022) Green finance, environmental regulation, and regional economic growth: from the perspective of low-carbon technological progress. Environ Sci Pollut Res 29(22):33698–33712. https://doi.org/10.1007/s11356-022-18582-8
Huang H, Zhang J (2021) Research on the environmental effect of green finance policy based on the analysis of pilot zones for green finance reform and innovations. Sustainability 13(7):3754. https://doi.org/10.3390/su13073754
Huang J, An L, Peng W, Guo L (2023) Identifying the role of green financial development played in carbon intensity: evidence from China. J Clean Prod. https://doi.org/10.1016/j.jclepro.2023.136943
Jain K, Gangopadhyay M, Mukhopadhyay K (2022) Prospects and challenges of green bonds in renewable energy sector: case of selected Asian economies. J Sustain Finance Invest. https://doi.org/10.1080/20430795.2022.2034596
Jia Q (2023) The impact of green finance on the level of decarbonization of the economies: an analysis of the United States’, China’s, and Russia’s current agenda. Bus Strateg Environ 32(1):110–119. https://doi.org/10.1002/bse.3120
Jiang K, Chen Z, Chen F (2022) Green creates value: evidence from China. J Asian Econ. https://doi.org/10.1016/j.asieco.2021.101425
Karagiannopoulou S, Sariannidis N, Ragazou K, Passas I, Garefalakis A (2023) Corporate social responsibility: a business strategy that promotes energy environmental transition and combats volatility in the post-pandemic world. Energies. https://doi.org/10.3390/en16031102
Khalatur S, Dubovych O (2022) Financial engineering of green finance as an element of environmental innovation management. Market Manag Innov 1:232–46. https://doi.org/10.21272/mmi.2022.1-17
Khan KI, Nasir A, Rashid T (2022) Green practices: a solution for environmental deregulation and the future of energy efficiency in the post-COVID-19 era. Front Energy Res. https://doi.org/10.3389/fenrg.2022.878670
Kong F (2022) A better understanding of the role of new energy and green finance to help achieve carbon neutrality goals, with special reference to China. Sci Prog 105(1):00368504221086361. https://doi.org/10.1177/00368504221086361
Kong H, Xu Y, Zhang R, Tang D, Boamah V, Wu G, Zhou B (2023) Research on the upgrading of China’s regional industrial structure based on the perspective of green finance. Front Environ Sci 11:972559. https://doi.org/10.3389/fenvs.2023.972559
Kroeze C, Ntziachristos L, Blok K, Hausler R (2022) Energy and climate governance for sustainable development. Annu Rev Environ Resour 47:1–27
Google Scholar
Lan J, Wei Y, Guo J, Li Q, Liu Z (2023) The effect of green finance on industrial pollution emissions: evidence from China. Resour Policy 80:103156. https://doi.org/10.1016/j.resourpol.2022.103156
Larsen M (2023) Adding “origination” to diffusion theory: contrasting the roles of china and the EU in green finance. Rev Int Polit Econ. https://doi.org/10.1080/09692290.2023.2204532
Lee C-C, Zhang J, Hou S (2023) The impact of regional renewable energy development on environmental sustainability in China. Resour Policy. https://doi.org/10.1016/j.resourpol.2022.103245
Lee C-C, Ho S-J (2022) Impacts of export diversification on energy intensity, renewable energy, and waste energy in 121 countries: Do environmental regulations matter? Renew Energy. https://doi.org/10.1016/j.renene.2022.09.079
Lee C-C, Wang F, Lou R, Wang K (2023) How does green finance drive the decarbonization of the economy? Empirical evidence from China. Renew Energy 204:671–684. https://doi.org/10.1016/j.renene.2023.01.058
Lee JW (2020) Green finance and sustainable development goals: the case of China. J Asian Finance Econ Bus 7(7):577–86. https://doi.org/10.13106/jafeb.2020.vol7.no7.577
Liberati A, Altman DG, Tetzlaff J, Mulrow C, Gøtzsche PC, Ioannidis JPA, Clarke M, Devereaux PJ, Kleijnen J, Moher D (2009) The PRISMA statement for reporting systematic reviews and meta-analyses of studies that evaluate health care interventions: explanation and elaboration. PLoS Med 6(7):e1000100. https://doi.org/10.1371/journal.pmed.1000100
Li C, Sampene AK, Agyeman FO, Brenya R, Wiredu J (2022) The role of green finance and energy innovation in neutralizing environmental pollution: empirical evidence from the MINT economies. J Environ Manag 317:115500. https://doi.org/10.1016/j.jenvman.2022.115500
Li C, Umair M (2023) Does green finance development goals affects renewable energy in China. Renew Energy. https://doi.org/10.1016/j.renene.2022.12.066
Li C, Gan Y (2021) The spatial spillover effects of green finance on ecological environment-empirical research based on spatial econometric model. Environ Sci Pollut Res 28(5):5651–5665. https://doi.org/10.1007/s11356-020-10961-3
Li C, Feng X, Li X, Zhou Y (2023) Effect of green credit policy on energy firms’ growth: evidence from China. Econ Res-Ekon Istraz. https://doi.org/10.1080/1331677X.2023.2177701
Li G, Jia X, Khan AA, Khan SU, Ali MAS, Luo J (2023) Does green finance promote agricultural green total factor productivity? Considering green credit, green investment, green securities, and carbon Finance in China. Environ Sci Pollut Res. https://doi.org/10.1007/s11356-022-24857-x
Li J, Dong K, Taghizadeh-Hesary F, Wang K (2022) 3G in China: How green economic growth and green finance promote green energy? Renew Energy 200:1327–1337. https://doi.org/10.1016/j.renene.2022.10.052
Li W, Fan Y (2023) Influence of green finance on carbon emission intensity: empirical evidence from China based on spatial metrology. Environ Sci Pollut Res. https://doi.org/10.1007/s11356-022-23523-6
Li X, Wang Z, Yu Y, Chen Y (2023) Does green finance promote the social responsibility fulfilment of highly polluting enterprises?–Empirical evidence from China. Econ Res-Ekon Istraz. https://doi.org/10.1080/1331677X.2022.2153719
Li Z, Kuo T-H, Siao-Yun W, The Vinh L (2022) Role of green finance, volatility and risk in promoting the investments in renewable energy resources in the post-Covid-19. Resour Policy 76:102563. https://doi.org/10.1016/j.resourpol.2022.102563
Li Z, Lu X, Wang S, Li X, Li H (2023) The threshold effect of environmental regulation in the nexus between green finance and total factor carbon productivity: evidence from a dynamic panel threshold model. Environ Sci Pollut Res. https://doi.org/10.1007/s11356-023-25214-2
Liang J, Song X (2022) Can green finance improve carbon emission efficiency? Evidence from China. Fron Environ Sci 10:955403. https://doi.org/10.3389/fenvs.2022.955403
Lin C, Zhang X, Gao Z, Sun Y (2023) The development of green finance and the rising status of China’s manufacturing value chain. Sustainability 15(8):6395. https://doi.org/10.3390/su15086395
Lin J-D (2023) Explaining the quality of green bonds in China. J Clean Product. https://doi.org/10.1016/j.jclepro.2023.136893
Lin L, Hong Y (2022) Developing a green bonds market: lessons from China. Eur Bus Organ Law Rev. https://doi.org/10.1007/s40804-021-00231-1
Lin R, Wang Z, Gao C (2023) Re-examining resources taxes and sustainable financial expansion: an empirical evidence of novel panel methods for China’s provincial data. Resour Policy. https://doi.org/10.1016/j.resourpol.2022.103284
Liu C, Xiong M (2022) green finance reform and corporate innovation: evidence from China. Financ Res Lett 48:102993. https://doi.org/10.1016/j.frl.2022.102993
Liu H, Yao P, Latif S, Aslam S, Iqbal N (2022) Impact of green financing, FinTech, and financial inclusion on energy efficiency. Environ Sci Pollut Res. https://doi.org/10.1007/s11356-021-16949-x
Liu H, Zhu Q, Khoso WM, Khoso AK (2023) Spatial pattern and the development of green finance trends in China. Renewable Energy. https://doi.org/10.1016/j.renene.2023.05.014
Liu X, Nie W (2022) Study on the coupling coordination mechanism of green technology innovation, environmental regulation, and green finance. Environ Sci Pollut Res 29(47):71796–71809. https://doi.org/10.1007/s11356-022-20905-8
Liu X, Zhang Y (2023) Green finance, environmental technology progress bias and cleaner industrial structure. Environ Dev Sustain. https://doi.org/10.1007/s10668-023-03062-x
Liu Y, Xia L (2023) Evaluating low-carbon economic peer effects of green finance and ICT for sustainable development: a chinese perspective. Environ Sci Pollut Res. https://doi.org/10.1007/s11356-022-24234-8
Liu Z, Zheng S, Zhang X, Mo L (2023) The impact of green finance on export technology complexity: evidence from China. Sustainability 15(3):2625. https://doi.org/10.3390/su15032625
Lv C, Bian B, Lee C-C, He Z (2021) Regional gap and the trend of green finance development in China. Energy Econ 102:105476. https://doi.org/10.1016/j.eneco.2021.105476
Lyu Y, You Wu, Zhang J (2023) How industrial structure distortion affects energy poverty? Evidence from China. Energy. https://doi.org/10.1016/j.energy.2023.127754
Ma H, Miao X, Wang Z, Wang X (2023) How does green finance affect the sustainable development of the regional economy? Evidence from China. Sustainability 15(4):3776. https://doi.org/10.3390/su15043776
Mao Q, Ma X, Shi L, Jing Xu (2021) Effect of green finance on regional economic development: evidence from China. Transform Bus Econ 20(3C):505–525
Mejía-Escobar JC, González-Ruiz JD, Franco-Sepúlveda G (2021) Current state and development of green bonds market in the Latin America and the Caribbean. Sustainability. https://doi.org/10.3390/su131910872
Mohanty S, Nanda SS, Soubhari T, Vishnu NS, Biswal S, Patnaik S (2023) Emerging research trends in green finance: a bibliometric overview. J Risk Financ Manag. https://doi.org/10.3390/jrfm16020108
Muganyi T, Yan L, Sun H-P (2021) Green finance, fintech and environmental protection: evidence from China. Environ Sci Ecotechnol 7:100107. https://doi.org/10.1016/j.ese.2021.100107
Naeem MA, Conlon T, Cotter J (2022) Green Bonds and other assets: evidence from extreme risk transmission. J Environ Manag. https://doi.org/10.1016/j.jenvman.2021.114358
Nie L, Chen P, Liu X, Shi Q, Zhang J (2022) Coupling and coordinative development of green finance and industrial-structure optimization in china: spatial-temporal difference and driving factors. Int J Environ Res Public Health 19(17):10984. https://doi.org/10.3390/ijerph191710984
Pan J, Chen X, Luo X, Zeng X, Liu Z, Lai W, Yue Xu, Chuangxin Lu (2022) Analysis of the impact of China’s energy industry on social development from the perspective of low-carbon policy. Energy Reports Elsevier Ltd. https://doi.org/10.1016/j.egyr.2022.05.052
Pan Y, Dong F (2023) Green Finance policy coupling effect of fossil energy use rights trading and renewable energy certificates trading on low carbon economy: taking China as an example. Econ Anal Policy 77:658–679. https://doi.org/10.1016/j.eap.2022.12.014
Pasupuleti A, Ayyagari LR (2023) A thematic study of green finance with special reference to polluting companies: a review and future direction. Environ Process 10:24. https://doi.org/10.1007/s40710-023-00642-x
Pearson L, Newton P, Roberts P (2014) Resilient sustainable cities: a future, vol 10. Routledge, Abingdon, p 9780203593066
Phung Thanh Q (2022) Economic effects of green bond market development in Asian economies. J Risk Finance. https://doi.org/10.1108/JRF-08-2022-0216
Priyashantha KG, De Alwis AC, Welmilla I (2022) Disruptive human resource management technologies: a systematic literature review. Eur J Manag Bus Econ
Priyashantha KG, De Alwis AC, Welmilla I (2021) Gender stereotypes change outcomes: a systematic literature review. J Human Appl Soc Sci. https://doi.org/10.1108/JHASS-07-2021-0131
Qin, Jiahong, and Jianhua Cao. ‘Carbon Emission Reduction Effects of Green Credit Policies: Empirical Evidence From China’. Frontiers in Environmental Science . Frontiers Media S.A., 2022. https://doi.org/10.3389/fenvs.2022.798072 .
Qin M, Zhang X, Li Y, Badarcea RM (2023) Blockchain market and green finance: the enablers of carbon neutrality in China. Energy Econ 118:106501. https://doi.org/10.1016/j.eneco.2022.106501
Ran C, Zhang Y (2023) Does green finance stimulate green innovation of heavy-polluting enterprises? Evidence from green finance pilot zones in China. Environ Sci Pollut Res. https://doi.org/10.1007/s11356-023-26758-z
Ran Q, Liu Lu, Razzaq A, Meng Y, Yang X (2023) Does green finance improve carbon emission efficiency? Experimental Evidence from China. Environ Sci Pollut Res 30(16):48288–48299. https://doi.org/10.1007/s11356-023-25571-y
Rao H, Chen D, Shen F, Shen Y (2022) ‘Can green bonds stimulate green innovation in enterprises? Evidence from China. Sustainability. https://doi.org/10.3390/su142315631
Ren X, Shao Q, Zhong R (2020) Nexus between green finance, non-fossil energy use, and carbon intensity: empirical evidence from China based on a vector error correction model. J Clean Prod 277:122844. https://doi.org/10.1016/j.jclepro.2020.122844
Ren Y, Jian Yu, Shuhua Xu, Tang J, Zhang C (2023) Green finance and industrial low-carbon transition: evidence from a quasi-natural experiment in China. Sustainability 15(6):4827. https://doi.org/10.3390/su15064827
Shao J, Huang P (2023) The policy mix of green finance in China: an evolutionary and multilevel perspective. Climate Policy. https://doi.org/10.1080/14693062.2023.2202181
Shen Y, Su Z-W, Malik MY, Umar M, Khan Z, Khan M (2020) Does green investment, financial development and natural resources rent limit carbon emissions? A provincial panel analysis of China. Sci. Total Environ. 755(2020):142538
Shi F, Ding R, Li H, Hao S (2022) Environmental regulation, digital financial inclusion, and environmental pollution: an empirical study based on the spatial spillover effect and panel threshold effect. Sustainability. https://doi.org/10.3390/su14116869
Shi M, Jia Z, Mehmood U (2023) Exploring the roles of green finance and environmental regulations on CO2es: defining the roles of social and economic globalization in the next eleven nations’. Environ Sci Pollut Res. https://doi.org/10.1007/s11356-023-26327-4
Sisodia G, Joseph A, Dominic J (2022) Whether corporate green bonds act as armour during crises? evidence from a natural experiment. Int J Manag Finance. https://doi.org/10.1108/IJMF-10-2021-0501
Song M, Zheng H, Shen Z, Chen B (2023) How financial technology affects energy transformation in China. Technol Forecast Soc Change. https://doi.org/10.1016/j.techfore.2022.122259
Sun C (2023) How are green finance, carbon emissions, and energy resources related in Asian sub-regions? Resour Policy. https://doi.org/10.1016/j.resourpol.2023.103648
Sun H, Chen F (2022) The impact of green finance on China’s regional energy consumption structure based on system GMM. Resour Policy 76:102588. https://doi.org/10.1016/j.resourpol.2022.102588
Sun J, Zhai N, Miao J, Sun H (2022) Can green finance effectively promote the carbon emission reduction in “local-neighborhood” areas?—Empirical evidence from China. Agriculture 12(10):1550. https://doi.org/10.3390/agriculture12101550
Sun Y, Sun Y, Li X (2021) Constructing a green financial innovation system with the PPP environmental protection industry fund. Int J Technol Manag. https://doi.org/10.1504/IJTM.2021.115272
Tang D, Fu B, Boamah V (2023) Implementation effect of China’s green finance pilot policy based on synthetic control method: a green innovation perspective. Environ Sci Pollut Res. https://doi.org/10.1007/s11356-023-25977-8
Tang W-Q, Meng B, Li-Bo W (2020) The impact of regulatory and financial discrimination on China’s low-carbon development: considering firm heterogeneity. Adv Climate Change Res. https://doi.org/10.1016/j.accre.2020.06.002
Tariq A, Hassan A (2023) Role of green finance, environmental regulations, and economic development in the transition towards a sustainable environment. J Clean Prod. https://doi.org/10.1016/j.jclepro.2023.137425
Tian Fa, Hou S (2022) The impact of green finance on industrial land use efficiency: evidence from 279 cities in China. Sustainability 14(10):6184. https://doi.org/10.3390/su14106184
Tsindeliani I, Proshunin M, Sadovskaya T, Tropskaya S, Davydova M, Popkova Z (2023) Comparative legal aspects of the EU and Russia policy in the field of green financing. International Journal of Sustainable Development and Planning. 1:1
Umar M, Safi A (2023) Do green finance and innovation matter for environmental protection? A case of OECD economies. Energy Econ 119:106560. https://doi.org/10.1016/j.eneco.2023.106560
Wang B, Zhao W (2022) Interplay of renewable energy investment efficiency, shareholder control and green financial development in China. Renew Energy. https://doi.org/10.1016/j.renene.2022.08.122
Wang B, Wang Y, Cheng X, Wang J (2023) Green finance, energy structure, and environmental pollution: evidence from a spatial econometric approach. Environ Sci Pollut Res. https://doi.org/10.1007/s11356-023-27427-x
Wang B, Wang C (2023) Green finance and technological innovation in heavily polluting enterprises: evidence from China. Int J Environ Res Public Health. https://doi.org/10.3390/ijerph20043333
Wang C, Li X-w, Wen H-X, Nie P-Y (2021) Order financing for promoting green transition. J Clean Prod. https://doi.org/10.1016/j.jclepro.2020.125415
Wang C, Gong W, You W, Liu Y (2023) the development level of green finance in chengdu-chongqing twin-city economic circle of china based on grey correlation model. Polish J Environ Stud. https://doi.org/10.15244/pjoes/157380
Wang E, Nie J, Zhan H (2022) The impact of carbon emissions trading on the profitability and debt burden of listed companies. Sustainability. https://doi.org/10.3390/su142013429
Wang F, Wang R, He Z (2021) The impact of environmental pollution and green finance on the high-quality development of energy based on spatial dubin model. Resour Policy 74:102451. https://doi.org/10.1016/j.resourpol.2021.102451
Wang F, Sun Z, Feng H (2022) Can media attention promote green innovation of chinese enterprises? Regulatory effect of environmental regulation and green finance. Sustainability 14(17):11091. https://doi.org/10.3390/su141711091
Wang G, Li S, Yang L (2022) Research on the pathway of green financial system to implement the realization of china’s carbon neutrality target. Int J Environ Res Public Health. https://doi.org/10.3390/ijerph19042451
Wang J, Tang J, Guo K (2022) Green bond index prediction based on CEEMDAN-LSTM. Front Energy Res. https://doi.org/10.3389/fenrg.2021.793413
Wang K-H, Zhao Y-X, Jiang C-F, Li Z-Z (2022) Does green finance inspire sustainable development? Evidence from a global perspective. Econ Anal Policy 75:412–426. https://doi.org/10.1016/j.eap.2022.06.002
Wang L, Dilanchiev A, Haseeb M (2022) The environmental regulation and policy assessment effect on the road to green recovery transformation. Econ Anal Policy. https://doi.org/10.1016/j.eap.2022.10.006
Wang M, Li X, Wang S (2021) Discovering research trends and opportunities of green finance and energy policy: a data-driven scientometric analysis. Energy Policy 154:112295. https://doi.org/10.1016/j.enpol.2021.112295
Wang W, Li Y (2022) Can green finance promote the optimization and upgrading of industrial structures?—Based on the intermediary perspective of technological progress. Front Environ Sci 10:919950. https://doi.org/10.3389/fenvs.2022.919950
Wang Y, Zhao Na, Lei X, Long R (2021) Green finance innovation and regional green development. Sustainability 13(15):8230. https://doi.org/10.3390/su13158230
Weber, Olaf, and Amr ElAlfy. The Development of Green Finance by Sector . Edited by M. Migliorelli and P. Dessertine. Rise of Green Finance in Europe: Opportunities and Challenges for Issuers, Investors and Marketplaces . Basingstoke: Palgrave, 2019. https://doi.org/10.1007/978-3-030-22510-0_3 .
Wei Z, Huang L (2022) Invading the dynamics of economic growth and CO 2 emission: panel data error correction model (ECM) approach. Environ Sci Pollut Res. https://doi.org/10.1007/s11356-022-20189-y
Xia L, Liu Y, Yang X (2023) The response of green finance toward the sustainable environment: the role of renewable energy development and institutional quality. Environ Sci Pollut Res. https://doi.org/10.1007/s11356-023-26430-6
Xu A, Zhu Y, Wang W (2023) Micro green technology innovation effects of green finance pilot policy-from the perspectives of action points and green value. J Bus Res 159:113724. https://doi.org/10.1016/j.jbusres.2023.113724
Xu C, Li X (2023) The efficiency of natural resource consumption and government administration concerning green economic growth in Asian countries. Resour Policy. https://doi.org/10.1016/j.resourpol.2023.103569
Xu J, Moslehpour M, Tran TK, Dinh KC, Ngo TQ, Huy PQ (2023) The role of institutional quality, renewable energy development and trade openness in green finance: empirical evidence from South Asian countries. Renewable Energy. https://doi.org/10.1016/j.renene.2023.03.015
Xu Li, Xu C (2022) Does green finance and energy policy paradox demonstrate green economic recovery: role of social capital and public health. Front Public Health 10:951527. https://doi.org/10.3389/fpubh.2022.951527
Yan Chunxiao, Tan Qi (2023) Has the level of green finance development improved carbon emission performance?—Empirical evidence from China. Manager Decis Econ. https://doi.org/10.1002/mde.3891
Yan Z, Cui C, Liao C (2021) The impact of green finance on clean power generation: evidence based on China. Strateg Plann Energy Environ. https://doi.org/10.13052/spee1048-5236.4046
Yang S, Zhang H, Zhang Q, Liu T (2022) Peer effects of enterprise green financing behavior: evidence from China. Front Environ Sci. https://doi.org/10.3389/fenvs.2022.1033868
Yin X, Chen D, Ji J (2023) How does environmental regulation influence green technological innovation? Moderating effect of green finance. J Environ Manag. https://doi.org/10.1016/j.jenvman.2023.118112
Yu H, Zhao Y, Qiao G, Ahmad M (2023) ‘Can green financial reform policies promote enterprise development? Empirical evidence from China. Sustainability. https://doi.org/10.3390/su15032692
Yuan H, Zou L, Feng Y (2023) How to achieve emission reduction without hindering economic growth? The role of judicial quality. Ecol Econ. https://doi.org/10.1016/j.ecolecon.2023.107839
Yun Na (2023) Nexus among carbon intensity and natural resources utilization on economic development: econometric analysis from China. Resour Policy. https://doi.org/10.1016/j.resourpol.2023.103600
Zeng Y, Wang F, Jun Wu (2022) The impact of green finance on urban haze pollution in China: a technological innovation perspective. Energies 15(3):801. https://doi.org/10.3390/en15030801
Zhai W (2023) Risk assessment of China’s foreign direct investment in “one belt, one road”: taking the green finance as a research perspective. Socio-Econ Plann Sci. https://doi.org/10.1016/j.seps.2023.101558
Zhan Y, Wang Y, Zhong Y (2022) Effects of Green Finance and financial innovation on environmental quality: new empirical evidence from China. Econ Res-Ekon Istraz. https://doi.org/10.1080/1331677X.2022.2164034
Zhang C, Cheng X, Ma Y (2022) Research on the impact of green finance policy on regional green innovation-based on evidence from the pilot zones for green finance reform and innovation’. Front Environ Sci 10:896661. https://doi.org/10.3389/fenvs.2022.896661
Zhang D, Chen XH, Lau CKM, Cai Y (2023) The causal relationship between green finance and geopolitical risk: implications for environmental management. J Environ Manag 327:116949. https://doi.org/10.1016/j.jenvman.2022.116949
Zhang D (2023) ‘Does green finance really inhibit extreme hypocritical ESG risk? A greenwashing perspective exploration. Energy Econ. https://doi.org/10.1016/j.eneco.2023.106688
Zhang J, Li F, Ding X (2022) Will green finance promote green development: based on the threshold effect of R&D investment. Environ Sci Pollut Res 29(40):60232–60243. https://doi.org/10.1007/s11356-022-20161-w
Zhang Jun (2023) Assessing the effect of the improvement of environmental damage compensation legal system and green finance project on the re-establishment of the ecological environment. Environ Sci Pollut Res. https://doi.org/10.1007/s11356-023-26877-7
Zhang S, Yang Z, Wang S (2020) Design of green bonds by double-barrier options’. Discr Contin Dyn Syst Series S. https://doi.org/10.3934/dcdss.2020110
Zhao J, Taghizadeh-Hesary F, Dong K, Dong X (2023) How green growth affects carbon emissions in China: the role of green finance. Econ Res-Ekon Istraz. https://doi.org/10.1080/1331677X.2022.2095522
Zhao M, Duan X (2023) Assessing financial performance through green bond markets and energy reliance in asian economies. Environ Sci Pollut Res. https://doi.org/10.1007/s11356-023-27173-0
Zhao X, Guo Y, Feng T (2023) Towards green recovery: natural resources utilization efficiency under the impact of environmental information disclosure. Resour Policy. https://doi.org/10.1016/j.resourpol.2023.103657
Zheng G-W, Siddik AB, Masukujjaman M, Fatema N (2021) Factors affecting the sustainability performance of financial institutions in Bangladesh: the role of green finance. Sustainability 13(18):10165. https://doi.org/10.3390/su131810165
Zheng M, Du Q, Wang Q-J (2023) Nexus between green finance and renewable energy development in China. Emerg Markets Finance Trade 59(4):1205–18. https://doi.org/10.1080/1540496X.2022.2119811
Zhou C, Qi S (2023) Does green finance restrain corporate financialization? Environ Sci Pollut Res. https://doi.org/10.1007/s11356-023-27476-2
Zhou C, Qi S, Li Y (2022) China’s green finance and total factor energy efficiency. Front Energy Res 10:1076050. https://doi.org/10.3389/fenrg.2022.1076050
Zhou K, Tao Y, Wang S, Luo H (2023) Does green finance drive environmental innovation in china? Emerg Markets Finance Trade. https://doi.org/10.1080/1540496X.2023.2190847
Zhou X, Cui Y (2019) Green bonds, corporate performance, and corporate social responsibility. Sustainability. https://doi.org/10.3390/su11236881
Zhou X, Juntao Du (2021) Does environmental regulation induce improved financial development for green technological innovation in China? J Environ Manag. https://doi.org/10.1016/j.jenvman.2021.113685
Zhu Y, Zhang J, Duan C (2023) How does green finance affect the low-carbon economy? Capital allocation, green technology innovation and industry structure perspectives. Econ Res-Ekon Istraz. https://doi.org/10.1080/1331677X.2022.2110138
Download references
Acknowledgements
Not applicable.
Not relevant.
Author information
Authors and affiliations.
Department of Business Finance, Faculty of Management, University of Peradeniya, Peradeniya, Sri Lanka
H. M. N. K. Mudalige
You can also search for this author in PubMed Google Scholar
Contributions
Single author.
Corresponding author
Correspondence to H. M. N. K. Mudalige .
Ethics declarations
Ethics approval and consent to participate, consent for publication.
Consent is given to the publisher
Competing interests
Additional information, publisher's note.
Springer Nature remains neutral with regard to jurisdictional claims in published maps and institutional affiliations.
Rights and permissions
Open Access This article is licensed under a Creative Commons Attribution 4.0 International License, which permits use, sharing, adaptation, distribution and reproduction in any medium or format, as long as you give appropriate credit to the original author(s) and the source, provide a link to the Creative Commons licence, and indicate if changes were made. The images or other third party material in this article are included in the article's Creative Commons licence, unless indicated otherwise in a credit line to the material. If material is not included in the article's Creative Commons licence and your intended use is not permitted by statutory regulation or exceeds the permitted use, you will need to obtain permission directly from the copyright holder. To view a copy of this licence, visit http://creativecommons.org/licenses/by/4.0/ .
Reprints and permissions
About this article
Cite this article.
Mudalige, H.M.N.K. Emerging new themes in green finance: a systematic literature review. Futur Bus J 9 , 108 (2023). https://doi.org/10.1186/s43093-023-00287-0
Download citation
Received : 31 August 2023
Accepted : 30 November 2023
Published : 20 December 2023
DOI : https://doi.org/10.1186/s43093-023-00287-0
Share this article
Anyone you share the following link with will be able to read this content:
Sorry, a shareable link is not currently available for this article.
Provided by the Springer Nature SharedIt content-sharing initiative
- Bibliometric analysis
- Green finance

- Bioenergy Technologies Office
- Accomplishments
- National Biotechnology & Biomanufacturing Initiative
- Bold Goals for U.S. Biotechnology & Biomanufacturing
- 2023 Multi-Year Program Plan
- Bioproduct Production
- CO2 Utilization
- Deconstruction & Fractionation
- Synthesis & Upgrading
- Conversion Technologies Related Links
- Sustainability
- Data, Modeling, & Analysis Related Links
- Feedstock-Conversion Interface R&D
- Feedstock Technologies Related Links
- Algal Logistics
- Algal Production
- Algal Related Links
- Development
- Infrastructure
- Systems Development & Integration Related Links
- Sustainable Aviation Fuels
- Sustainable Marine Fuels
- Waste-to-Energy
- CO2 Reduction & Upgrading for e-Fuels Consortium
- Consortium for Computational Physics & Chemistry
- Co-Optimization of Fuels & Engines
- Feedstock-Conversion Interface Consortium
- Bioenergy FAQs
- Biofuel Basics
- Biopower Basics
- Bioproduct Basics
- Biomass Feedstocks
- Career Exploration Wheel
- Career Grid
- Workforce Training Opportunities Map
- Algae Technology Educational Consortium
- Workforce Development Strategy
- Bioenergy Jobs at BETO
- BRIDGES Events
- BRIDGES Webinar Recordings and Presentations
- Internships & Fellowships
- Teacher & Classroom Resources
- BioenergizeME Infographic Challenge
- Wood Heater Design Challenge
- Funding Opportunities
- Analytical Tools
- Bioenergy Publications
- Bioenergy Related Links
- State Biomass Contacts
- BioProse: Bioenergy R&D Blog
- Bioenergy News
- Bioeconomy 2020
- Bioeconomy 2018
- Bioeconomy 2017
- Bioenergy 2016
- Bioenergy 2015
- Biomass 2014
- Biomass 2013
- Biomass 2012
- Biomass 2011
- Inaccessible
- Biomass 2009
- Peer Review 2023
- Peer Review 2021
- Peer Review 2019
- Peer Review 2017
- Peer Review 2015
- Peer Review 2013
- Peer Review 2011
- Past Meetings & Workshops
- Bioenergy Newsletter
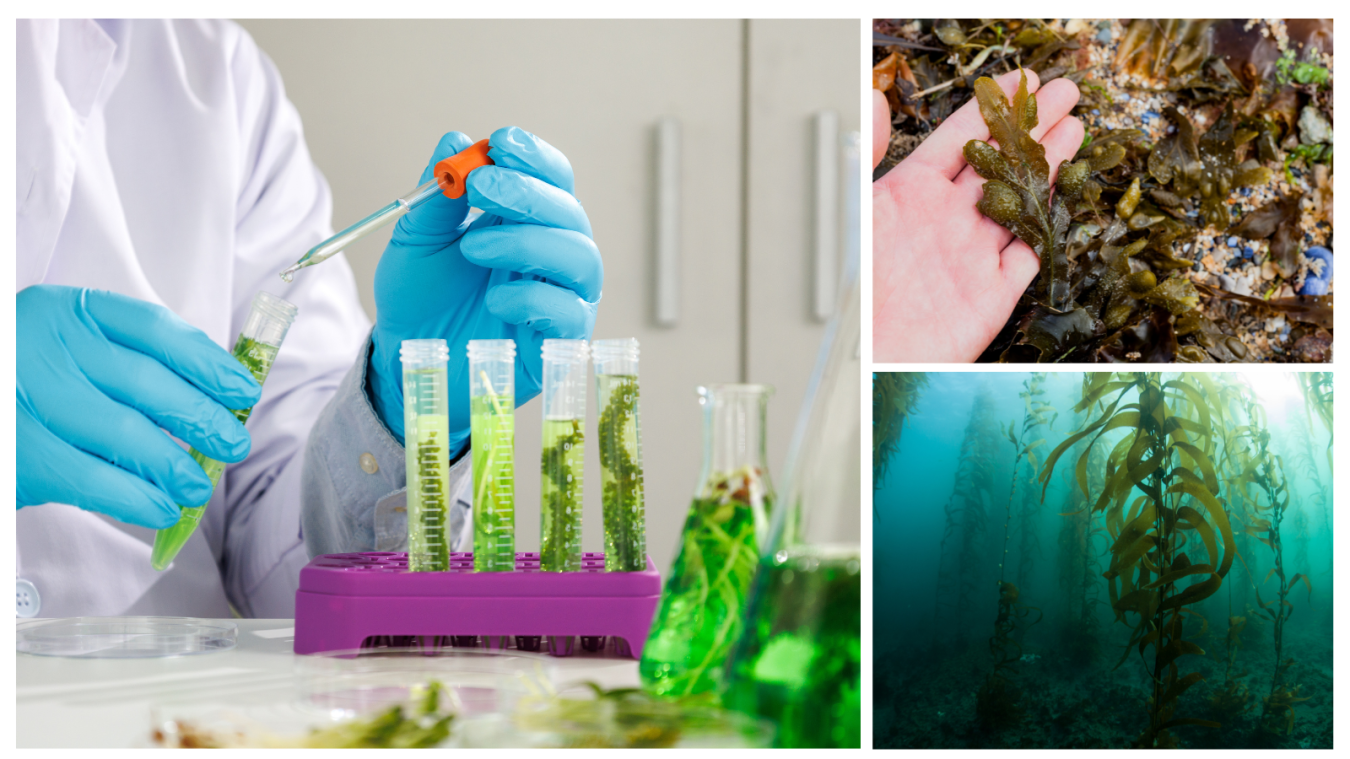
Office: Bioenergy Technologies Office (BETO) & Office of Fossil Energy and Carbon Management (FECM)
FOA number: DE-FOA-0003274
FOA Amount: $18.8 million
Phase 1 Selections to be Announced : ~September 2024
Link to Apply: MACRO: Mixed Algae Conversion Research Opportunity
Today, the U.S. Department of Energy’s Bioenergy Technologies Office (BETO) and Office of Fossil Energy and Carbon Management (FECM) announced the MACRO: Mixed Algae Conversion Research Opportunity funding opportunity announcement (FOA). The FOA will award up to $18.8 million to address research and development (R&D) challenges in converting algae, such as seaweeds and other wet waste feedstocks, to biofuels and bioproducts that can decarbonize domestic transportation, industry, and communities.
Seaweeds, also known as macroalgae, are an emerging biomass resource with unique benefits compared to land-based biomass systems. However, they are underutilized and are difficult to convert due to their variability, unique chemical make-up, and storage instability. Overcoming these conversion challenges will help build algae biomass supply chains, accelerate their demand, and ultimately, drive the U.S. bioeconomy by enabling greater volumes of sustainable aviation fuel (SAF) and carbon dioxide (CO 2 ) conversion to algae.
A wide range of biomass resources, such as readily available seaweeds and wet waste feedstocks, will be critical to reach the Biden-Harris administration’s decarbonization goal of an equitable, clean energy future of net-zero emissions, economy-wide by no later than 2050. This FOA will also support the government-wide targets of the Sustainable Aviation Fuel Grand Challenge , the Executive Order on Advancing Biotechnology and Biomanufacturing Innovation for a Sustainable, Safe, and Secure American Bioeconomy , and the Clean Fuels & Products Shot™ .
Topic Areas
Aligning with BETO and FECM’s strategic program goals, the FOA will fund selected projects in two focus areas:
- Topic Area 1 : Conversion of Seaweeds to Low Carbon Fuels and Bioproducts This Topic Area, funded by BETO, will focus on laboratory scale R&D on conversion of seaweeds and seaweed blends with other wet wastes to renewable fuels and bioproducts to enable these readily available feedstocks to access new markets. It aims to address gaps in storage, mobilization, and conversion of readily available algae, including offshore farmed seaweeds, seaweed wastes, and blends of seaweed with other waste algae or blends of seaweed with other wet wastes to low-carbon fuels and bioproducts to enable these readily available feedstocks to access new markets. If successful, these efforts will enable market expansion, address community waste management challenges, and reduce greenhouse gas reductions.
- Topic Area 2 : Conversion of Algal Biomass for Low Carbon Agricultural Bioproducts This Topic Area, funded by FECM, will support the use of anthropogenic CO 2 streams from industrial sources or utilities to grow micro- and macroalgae for low-carbon bioproducts. It aims to utilize CO 2 emissions streams from utilities or industrial sources to grow algae for source material and create value-added bioproducts (exclusive of fuels). Applications are sought that utilize anthropogenic (e.g., fossil fuel derived) CO 2 emissions, including concentrated CO 2 supplied from DAC technologies, in the cultivation process and then convert macro- and/or micro-algae into low-carbon agricultural applications or bioproducts such as animal feed. Applications are encouraged to focus on optimization of the technologies and processes for the conversion of cultivated algae biomass to bioproducts and clearly describe the end use bioproducts targeted.
Both topic areas contribute to BETO’s strategic goals for SAFs and other low-carbon bioproducts , as well as FECM’s aims to use CO 2 emissions to grow algae and convert these feedstocks into low-carbon agricultural bioproducts.
BETO anticipates making approximately five to six financial assistance awards lasting from 24 to 36 months under this FOA. FECM intends to award three to four financial assistance awards that will run up to 24 months in length.
The FOA concept paper deadline is 5:00 p.m. ET, on May 10, 2024 , and full applications are due at 5:00 p.m. ET, on June 27, 2024 . More information on the MACRO FOA and how to apply can be found on EERE Exchange . Additional information on the FOA and applicant eligibility is also available on Grants.gov .
An informational webinar for potential applicants will be held on Wednesday, April 17, 2024, at 1:00 p.m. ET .
Additional Information
Visit BETO’s funding announcement page for other upcoming funding opportunities.
Teaming Partner List
BETO has compiled a Teaming Partner List to encourage collaboration and facilitate the formation of project teams. The list allows organizations wishing to apply for funding to explore partnering with other prospective applicants. Partnerships can provide additional expertise and resources to successfully meet FOA goals.
Please include the following information in your partner list submission:
Topic Field - Please enter the Subtopic Area you are interested in. If you would like to be included in multiple Subtopic Areas, please complete separate submissions.
Background, Interests, Capabilities Field - Please include information about your organization type/characteristics in the “Background, Interest, Capabilities” field to help other participants identify your expertise and resources. BETO also seeks applications from diverse teams of minority-serving institutions (MSIs), including Historically Black Colleges and Universities (HBCUs), Minority Business Enterprises, Minority-Owned Businesses, Woman-Owned Businesses, Veteran-Owned Businesses, and tribal entities; workforce education and training providers; and labor organizations.
Any organization that would like to be included on this list should submit the required information via the Teaming Partner List on EERE Exchange.
Disclaimer: By submitting a request to be included on the Teaming Partner List, the requesting organization consents to the publication of the above-referenced information. By enabling and publishing the Teaming Partner List, EERE is not endorsing, sponsoring, or otherwise evaluating the qualifications of the individuals and organizations that are self-identifying themselves for placement on this Teaming Partner List. EERE will not pay for the provision of any information, nor will it compensate any applicants or requesting organizations for the development of such information.
Technology Verification
All applications selected for award negotiations under Topic Area 1 of this FOA are required to participate in a verification process led by DOE’s identified external third-party non-conflicted verification team. This verification process provides technical assistance to both BETO and the project by providing an in-depth analysis of key technical and economic metrics to ensure transparency and increase the likelihood of project success.
FutureVU: Sustainability

Twenty-five and counting: Vanderbilt University continues to expand its green building certifications
Posted by hamiltcl on Friday, April 12, 2024 in FutureVU , Green Building .
THINGS TO KNOW:
- Vanderbilt University now has 25 LEED certified buildings
- Vanderbilt has PETAL and WELL-designed buildings (certifications pending)
- The Commons was the first set of buildings to receive LEED certification on campus
- When certified, Nicholas S. Zeppos Residential College will be Vanderbilt’s first Platinum LEED-certified building
- In 2020, Vanderbilt was honored with the 2020 Leadership Award from the U.S. Green Building Council
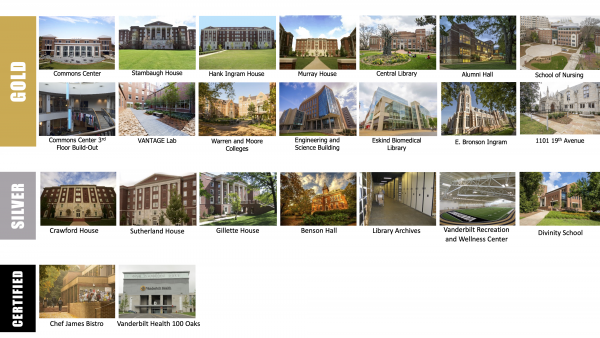
When Vanderbilt University constructed its first building in 1875, it was efficiently designed for multipurpose use—with classrooms, laboratories, library, museum and chapel under one roof. As architect William Crawford Smith designed Kirkland Hall, then known as Main Building, he employed the use of flexible spaces, which is one of the tenets of sustainably designed buildings still in use today. His flexible design and construction stood the test of time as Kirkland Hall morphed its identity over the years.
Today, the university builds on Smith’s legacy by keeping sustainability at the forefront of its design process for new buildings—and when evaluating existing buildings.
LEADERSHIP IN ENERGY AND ENVIRONMENTAL DESIGN (LEED)
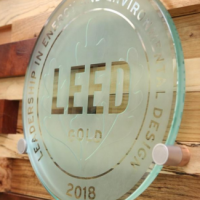
LEED is broken down by a set of goals:
- Reduce contribution to global climate change
- Enhance individual human health
- Protect and restore water resources
- Protect and enhance biodiversity and ecosystem
- Promote sustainable and regenerative materials
- Enhance community quality of life
The Commons
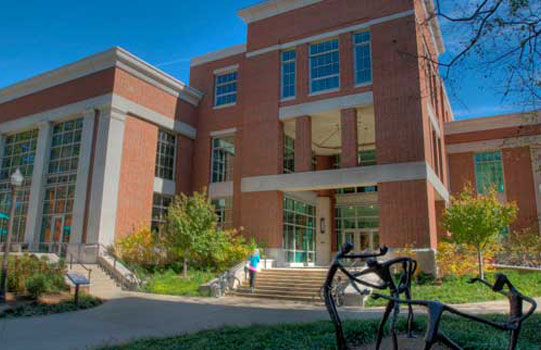
In 2009 Vanderbilt opened the doors to its first set of LEED-certified buildings. The Commons has seven LEED-certified buildings, four Gold and three Silver. When unveiled, this project made history as the largest collection of LEED-certified buildings in Tennessee.
- Nicholas S. Zeppos Residential College
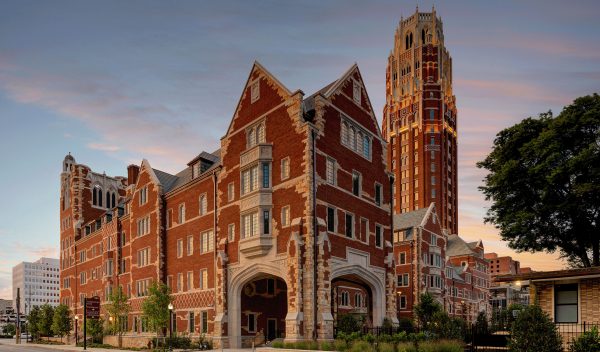
For a building to achieve a LEED Platinum certification, it must earn 80+ points from the USBGC.
Zeppos College is set to achieve this certification through design elements such as:
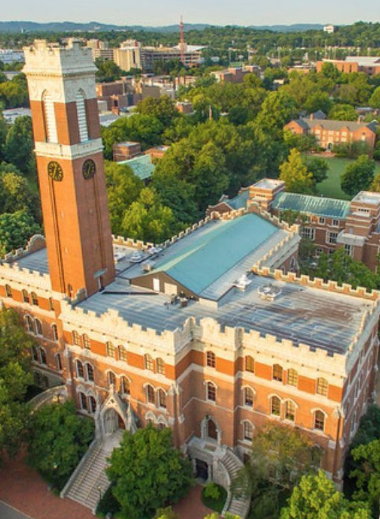
- Increased natural light throughout the building and campus views from regularly occupied areas
- Proximity to modes of transportation such as the nearby bike racks and the WeGo and VandyRide bus stops
- Regionally sourced materials used in the design and construction
- Products developed with recycled content such as the carpeting, floors in the restrooms and metal studs and concrete blocks used in the structure
- High-efficiency flush and low-flow plumbing fixtures to reduce potable water use
- Overall energy performance
Learn more about the design elements it takes to earn a Platinum LEED certification.
PETAL AND WELL BUILDINGS
Vanderbilt has two other types of sustainably designed buildings: WELL and PETAL (certifications pending).
VANDERBILT UNIVERSITY SCHOOL OF NURSING
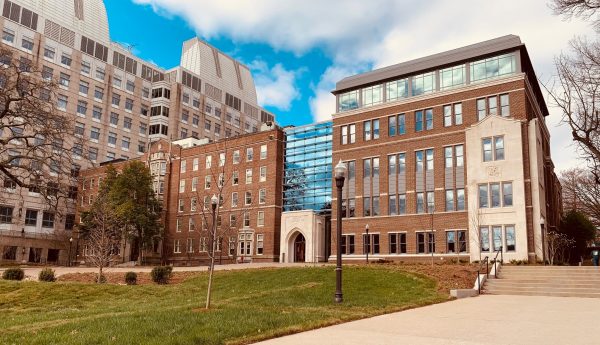
The WELL Building Standard is a performance-based system of measuring, certifying and monitoring features that affect human health and well-being in a built environment, including air quality, water, nourishment, light, fitness, comfort and mind.
Explore this interactive map of the building >>
THE CONNECTOR BUILDING
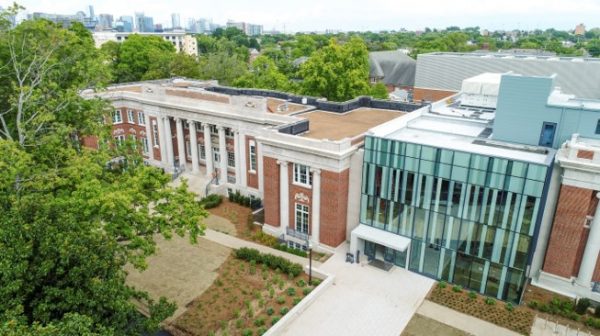
The Connector Building, which is between the newly renovated One Magnolia and Mayborn Buildings on the Peabody campus, was strategically designed to achieve a LEED certification and Vanderbilt’s first Living Building Challenge PETAL certification for materials (certifications pending).
To qualify for a PETAL certification, administered by the International Living Future Institute, a building must be designed to:
- Sourcing materials that are Red List free
- Tracking embodied carbon from construction
- Using salvaged materials and responsibly sourced wood and stone
- Striving to eliminate waste during construction
- Sourcing materials from local industries
In the Connector Building, there are a series of educational signs to highlight the sustainable elements that qualify it as a PETAL-certified building.
THE FUTURE
The university has many buildings with various certifications pending:
- Rothschild Residential College
- Kirkland Hall
- 17th and Horton
- Engineering and Science Building (fifth and sixth floors)
- Owen Graduate School of Management
Additional information will be released as the university earns LEED, WELL and PETAL certifications.
LEARN MORE ABOUT FUTUREVU SUSTAINABILITY
Tags: featured , FutureVU , Green Building
Comments are closed
- Annual Report
- What You Can Do
- Subscribe to Newsletter
Quick Links
- PeopleFinder
- Media Center
- E-Books & White Papers
- Knowledge Center
White Hydrogen Is Ready to Fuel the Multibillion-Dollar Hydrogen Economy
by BIS Research , on April 10, 2024
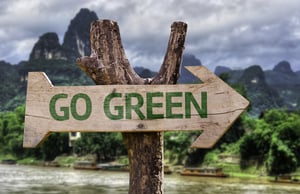
This shift is further fueled by progress and investments in sectors such as the water electrolysis market, hydrogen fuel cell market, green ammonia market, green methanol market, ammonia crackers market, and the hydrogen storage and transportation market. Combined, these innovative technologies can guide us towards a more eco-friendly future.
Technology at the Core of the Hydrogen Economy
At the core of the hydrogen economy’s progress are technologies that streamline the production, storage, and application of hydrogen as a fuel. The water electrolysis market plays a role by enabling the creation of green hydrogen through the process of water splitting, utilizing energy sources. This innovation not only offers a method for producing hydrogen but also drives the growth of the hydrogen fuel cell market by providing a clean alternative to traditional fossil fuels in power generation and transport.
Similarly, advancements in the green ammonia and green methanol markets illustrate the adaptability of hydrogens, in producing chemicals in an environmentally friendly way. Furthermore, these innovations are crucial for the progress of the ammonia crackers market, growing at CAGR of 25.7% , and the hydrogen storage and transportation market, growing at a CAGR of 18.74% , to guarantee the efficient distribution and utilization of hydrogen in various industries.
Hydrogen Economy: Key Drivers and Opportunities
The rise of the hydrogen industry is being driven by the push for decarbonization advancements in technology and substantial financial investments. The focus on white hydrogen, known for its eco-extraction methods, highlights the potential for innovation and environmental responsibility within the sector. The white (natural) hydrogen market, though currently at a very nascent stage, is expected to reach 115.09 million tons in production from 2029-2033 .
Recent investments, like a funding round for a hydrogen company supported by Bill Gates, showcase the market's growth prospects and investor confidence. Additionally, the shift towards achieving net zero emissions has brought attention to the green hydrogen market, emphasizing its role in reducing carbon footprints across sectors. The green hydrogen market is projected to grow at a CAGR of 67.19% between 2023-20233 and reach $141.29 billion by 2033 . This expanding market offers opportunities for green technology development, job creation, and establishing a supply chain for clean energy.
White Hydrogen: A Significant Breakthrough
The emergence and emphasis on white hydrogen represent a significant milestone. Unlike other types of hydrogen, white hydrogen is produced through natural reserves, similar to natural gas but without the negative impact on carbon emissions associated with traditional fossil fuels. This groundbreaking discovery not only broadens the range of hydrogen sources but also underscores the importance of white hydrogens in transitioning towards cleaner energy alternatives. Its ability to be harnessed with minimal environmental impact positions white hydrogen as a key player in the global move towards cleaner energy solutions, offering a promising avenue for sustainable industrial and energy sectors.
Hydrogen Economy: A Way Forward
The hydrogen economy showcases a collective dedication in combating climate change as various stakeholders pave the path toward new solutions. The unique characteristics of white hydrogen and its minimal environmental footprint serve as proof that it could play a role in the energy portfolio. White hydrogen holds promise in contributing to our energy sources due to its qualities and limited environmental impact. The hydrogen economy isn't an alternative; it's a progression towards a cleaner more resilient planet, for future generations.
Understanding the Hydrogen Market Better
In an ever-evolving economy, having market insights is key. By utilizing the critical information available in market research reports, stakeholders can better understand trends, future prospects, and the competitive environment to make well-informed decisions and strategic plans.
Check out recent reports by BIS Research on these topics and other related markets:
- White Hydrogen Market
- Green Hydrogen Market
- PEM Electrolyzer Market
- Power Supply Equipment Market for Water Electrolysis
- Multiple-Element Gas Container Market
- Global Virtual Pipeline Market
- Hydrogen Fuel Cell Market
- Hydrogen Fueling Station Market
- Ammonia Crackers Market
- Low-Carbon Aluminum Market
- Sustainable Steel Market
- Hydrogen Storage and Transportation Market
These market research reports by BIS Research not only shed light on the dynamics of the hydrogen market but also outline potential areas for growth and challenges ahead. The data and expertise helps ensure that stakeholders are well prepared to seize the growing opportunities in the hydrogen economy and gain a foothold at the forefront of the transition toward sustainable energy solutions.
About the Publisher: BIS Research is a global market intelligence, research and advisory company that focuses on emerging technology trends that are likely to disrupt the market. Its team includes industry veterans, experts, and analysts with diverse backgrounds in consulting, investment banking, government, and academia.
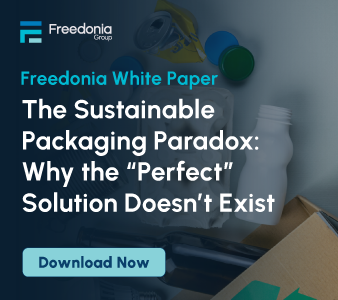
About This Blog
Our goal is to help you better understand your customer, market, and competition in order to help drive your business growth.
Popular Posts
- A CEO’s Perspective on Harnessing AI for Market Research Excellence
- How to Use Market Research for Onboarding and Training Employees
- 10 Global Industries That Will Boom in the Next 5 Years
- Primary Data vs. Secondary Data: Market Research Methods
- 10 Booming Industries in the U.S. to Watch in 202 4 and Beyond
Recent Posts
Posts by topic.
- Industry Insights (818)
- Market Research Strategy (271)
- Food & Beverage (133)
- Healthcare (124)
- The Freedonia Group (120)
- How To's (107)
- Market Research Provider (89)
- Manufacturing & Construction (80)
- Pharmaceuticals (77)
- Packaged Facts (76)
- Telecommunications & Wireless (70)
- Heavy Industry (69)
- Marketing (58)
- Profound (56)
- Retail (56)
- Transportation & Shipping (54)
- Software & Enterprise Computing (53)
- House & Home (50)
- Materials & Chemicals (47)
- Consumer Electronics (45)
- Medical Devices (45)
- Energy & Resources (42)
- Public Sector (40)
- Demographics (37)
- Biotechnology (36)
- Business Services & Administration (36)
- Education (36)
- Custom Market Research (35)
- Diagnostics (34)
- Academic (33)
- E-commerce & IT Outsourcing (32)
- Travel & Leisure (32)
- Financial Services (29)
- Computer Hardware & Networking (26)
- Simba Information (24)
- Kalorama Information (21)
- Knowledge Centers (19)
- Apparel (18)
- Cosmetics & Personal Care (17)
- Social Media (16)
- Advertising (14)
- Big Data (14)
- Market Research Subscription (14)
- Holiday (11)
- Emerging Markets (8)
- Associations (1)
- Religion (1)
MarketResearch.com 6116 Executive Blvd Suite 550 Rockville, MD 20852 800.298.5699 (U.S.) +1.240.747.3093 (International) [email protected]
From Our Blog
Subscribe to blog, connect with us.


A new spin on organic shampoo makes it sudsier, longer lasting
While there's no regulation in the U.S. for what's in organic shampoos, they tend to contain ingredients perceived as safe or environmentally friendly. However, these "clean" shampoos separate and spoil faster than those made with synthetic stabilizers and preservatives. Now, researchers in ACS Sustainable Chemistry & Engineering demonstrate that a simple process -- spinning organic shampoo at high speeds -- improved the final products' shelf lives and ability to clean hair.
Natural emulsifiers, such as xanthan gum and cetyl alcohol, are sometimes used to prevent organic shampoo from separating and enhance its soapy lather. But they don't remain effective for as long as synthetic emulsifiers used in conventional haircare products. So, Youhong Tang, Colin Raston and colleagues looked to a technology invented by Raston -- a vortex fluidic device (VFD). The team wanted to see if the high-speed spinning platform could work as well as a chemical to improve the lather and shelf stability of organic shampoo without adding to the list of ingredients on the back of the bottle.
Previously, the researchers determined that VFD's intense micromixing could produce clean biodiesel from used cooking oil and homogenize raw milk, but they hadn't used the platform to process more goopy liquids, such as shampoos. They guessed that it could also minimize aggregation of oil droplets and glass microspheres, components in some organic shampoo formulations, thereby maximizing the stability of these personal care products. To test the theory, the researchers added fresh store-bought organic shampoo to a glass tube tilted 45 degrees and spun it for 15 minutes at 4,500 revolutions per minute. (Their previous work determined this angle and spinning speed were the optimal conditions for processing.)
After 30 days of storage, an unprocessed organic shampoo sample turned from white to yellow and separated into two layers, whereas the spun shampoo didn't change color and remained homogenized. In tests comparing the products' foaming and cleansing abilities, the researchers found that the spun shampoo's lather lasted longer and cleaned human hair more thoroughly. And on a microscopic level, the spun shampoo contained more evenly dispersed oil droplets and glass microspheres compared to the original product. The team says that result confirmed the hypothesis that VFD processing could be a "green" one-step technique for more stable, emulsified viscous liquids.
Next, they plan to characterize how processing with VFD affects individual components in organic shampoos -- like natural surfactants (soaps) and botanical extracts -- so they can fine-tune the technique for a broader range of organic shampoos, other natural personal care products and cosmetics.
The authors acknowledge the Australian Research Council Industrial Transformation Training Centre for Green Chemistry in Manufacturing for funding, and Plantworx Pty Ltd for funding and materials.
- Organic Chemistry
- Materials Science
- Inorganic Chemistry
- Energy and Resources
- Electronics
- Organic chemistry
- Energy conservation
- Citric acid
- Automobile emissions control
Story Source:
Materials provided by American Chemical Society . Note: Content may be edited for style and length.
Journal Reference :
- Xuejiao Cao, Shan He, Xuan Luo, Jingrong Gao, Youhong Tang, Colin L. Raston. Stability and Cleansing Function Enhancement of Organic Shampoo by a Vortex Fluidic Device . ACS Sustainable Chemistry & Engineering , 2024; 12 (14): 5533 DOI: 10.1021/acssuschemeng.3c08159
Cite This Page :
Explore More
- Ozone Removes Mating Barriers Between Fly ...
- Parkinson's: New Theory On Origins and Spread
- Clash of Stars Solves Stellar Mystery
- Secure Quantum Computing at Home
- Ocean Currents: Collapse of Antarctic Ice ...
- Pacific Cities Much Older Than Previously ...
- The Milky Way in Ancient Egyptian Mythology
- Physical Activity Best in the Evening
- How the Body Switches out of 'Fight' Mode
- New Drug Prevents Flu-Related Lung Damage
Trending Topics
Strange & offbeat.
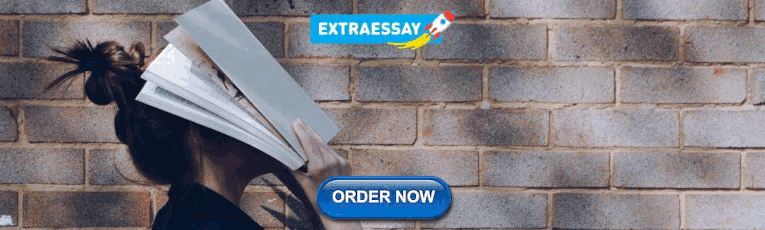
IMAGES
COMMENTS
While many areas of green energy are growing quickly, we identified and prioritized the top ten emerging topics (Figure 1) that will help us reach a more sustainable future. Figure 1: Normalized growth in the number of publications between 2013-2022 for the emerging research topics. Batteries, energy storage, and battery recycling
A view of wind turbines drives down home values — but only briefly. House prices drop by 1% if wind turbines are close and visible, but they rebound quickly. Research Highlights 20 Mar 2024 ...
More energy efficiency means less pollution, and energy efficiency has increased by around 2% annually in the past few years. But meeting the target for 2030 — to double the rate of the 1990 ...
Green-hydrogen is an emerging area of study, with most of the research aligned to Affordable and Clean Energy (SDG 7) and Climate Action (SDG 13). 50% of the publications from 2016-to 2021 are from the Top 10% Journal Percentiles, confirming the high-quality output and the interest of the top journals in the topic of green-hydrogen.
Y.W. acknowledges funding support from the Singapore National Research Foundation under its Green Buildings Innovation Cluster (GBIC award number NRF2015ENC-GBICRD001-012) administered by the ...
This Research Topic aims to fill these literature gaps that are gradually appealing. This Research Topic will provide insights about:(1) Key drivers of the quick deployment of green energy technologies.(2) Specific challenges and barriers, hindering the penetration and acceptance of green energy technologies.(3) Future aspects o...
Through systematic reviews of current and trending topics in clean energy research, we aim to delineate a critical research agenda for clean energy transition as an effective way to achieve a low-carbon development and carbon neutrality. ... Int J Green Energy. 2017; 14(4): 368-378. View Article Google Scholar 113. Dutta A. Oil and energy ...
From Figure 2, it is noted that the energy sector inn form of electricity and heat production is the largest contributor of green house gases with about 34%, industry at 24% followed by agriculture, forestry and other land activities accounting for 21%, transportation with 14%, while buildings contributed about 6% while the building sector is least with 6% in 2018 (Lamb et al., 2021).
Deborah de Lange, Toronto Metropolitan University. Recent research about energy industry restructuring options for a green transition indicates that innovation in renewable energy positively ...
Being the primary engine of global economic activity, energy obtained from non-renewable sources plays a large role in environmental damage. To move toward clean and green energy and achieve net-zero carbon emissions, it is crucial to develop reliable and sustainable alternatives to fossil fuels as well as smart and sustainable energy technology. The seventh Sustainable Development Goal (SDG 7 ...
Explore the latest full-text research PDFs, articles, conference papers, preprints and more on GREEN ENERGY. Find methods information, sources, references or conduct a literature review on GREEN ...
Dissertation Topics Ideas on Sustainability and Green Technology for 2021. Topic 1: Research on sustainable gardens. Topic 2: Sustainable outdoor designs using recycled materials. Topic 3: Pollution-free disposal and recycling of trash. Topic 4: Importance of gardening- awareness and ideas for the city, terrace/roof gardening.
And he's not alone. Teams around the globe are creating a bevy of novel "hydrovoltaic" devices able to convert the energy inherent in evaporation, rainfall, and small water flows into usable energy. "It's a really burgeoning field," Yao says. But so far, these devices have mostly been one-off lab demonstrations.
Cluster and content analysis via CiteSpace identified popular and trending research topics at different stages of development; the top three hotspots were green buildings, sustainability, and energy efficiency throughout the whole research period. Energy efficiency has shifted from low to zero energy buildings or even beyond it in recent years.
2. Climate, energy and environmental policy. By Alec Tyson, Brian Kennedy and Cary Funk. A majority of Americans consider climate change a priority today so that future generations can have a sustainable planet, and this view is held across generations. Looking to the future, the public is closely divided on what it will take to address climate ...
Explore the latest questions and answers in Green Energy, and find Green Energy experts. Questions (107) Publications (10,000) Questions related to Green Energy. 1. 2. Dariusz Prokopowicz. asked a ...
EERE's Solar Energy Technologies Office (SETO) is seeking to support postdoctoral researchers to apply and advance cutting-edge data science to drive toward the national solar cost reduction goals. Areas of interest include: Novel analysis of Green Button (smart meter) and PV performance data with the Durable Module Materials (DuraMAT) Consortium.
The Precourt Institute for Energy is now part of the Stanford Doerr School of Sustainability. Search this site Submit Search. Menu. About. ... Research Funding Opportunities. Precourt Pioneering Projects; Seed Grants; Stanford Energy Research Year in Review; Stanford Energy Postdoctoral Fellowship; Request for Proposals; Education. Students ...
Americans Largely Favor U.S. Taking Steps To Become Carbon Neutral by 2050. Majorities of Americans say the United States should prioritize the development of renewable energy sources and take steps toward the country becoming carbon neutral by the year 2050. But just 31% want to phase out fossil fuels completely, and many foresee unexpected ...
Renewable Energy Research Topics. Topic. 1: Renewable energy: Prospects and Problems Today. Topic. 2: Renewable energy for sustainable development in Africa. Topic. 3: Implications of COVID-19 on the biofuel market. Topic. 4: Geothermal energy; an untapped abundant energy resource. Topic. 5: The Future of Wind Energy.
April 11, 2024. Source: Duke University. Summary: New research is shedding light on one barrier to a clean energy future: corrosion. Using nanoscale imaging techniques, researchers have captured ...
You want to do a student research project with impact. We have outlined a range of sustainability research topics for you. The list specifically focuses on how to green your campus. Take action to make your university more sustainable! Our list of sustainability research topics helps students investigate green campus issues.
Optimizing high-dimensional, expensive, black-box problems within integrated energy system. AMIR SAFARI. Mohsen Assadi. Mohan Kolhe. Amin Hajizadeh. Adel Younis. 145 views.
The relationship between carbon intensity and economic development is a growing topic in green finance research. How nations may shift to low-carbon economies while maintaining economic growth has been studied. ... Kong F (2022) A better understanding of the role of new energy and green finance to help achieve carbon neutrality goals, with ...
Generating geothermal energy requires a permeable subsurface to efficiently release heat when cold fluids are forced into the rock. This research reveals the optimum times for efficient energy ...
The U.S. Department of Energy's Bioenergy Technologies Office (BETO) and Office of Fossil Energy and Carbon Management (FECM) today announced the MACRO: Mixed Algae Conversion Research Opportunity funding opportunity announcement (FOA). The FOA will award up to $18.8 million to address research and development (R&D) challenges in converting algae, such as seaweeds and other wet waste ...
LEADERSHIP IN ENERGY AND ENVIRONMENTAL DESIGN (LEED) LEED, the world's most widely used green building rating system, is managed by the U.S. Green Building Council. LEED is broken down by a set of goals: Reduce contribution to global climate change Enhance individual human health Protect and restore water resources
Biden's Green-Energy Price Shock. The cost of electricity has climbed by 29.4% since January 2021. "When the global market is flooded by artificially cheap Chinese products, the viability of ...
by BIS Research, on April 10, 2024. In the pursuit of sustainability, the hydrogen economy is emerging as a compelling energy solution. White hydrogen and green hydrogen are at the forefront of this shift—reshaping how we produce, store, and consume energy. This shift is further fueled by progress and investments in sectors such as the water ...
Apr. 19, 2023 — Organic solar cells show great promise for clean energy applications. However, photovoltaic modules made from organic semiconductors do not maintain their efficiency for long ...